- 1MARE - Marine and Environmental Sciences Centre, Agência Regional para o Desenvolvimento da Investigação Tecnologia e Inovação (ARDITI), Funchal, Portugal
- 2cE3c/Azorean Biodiversity Group, Departamento de Biologia, Faculdade de Ciências e Tecnologia, Universidade dos Açores, Ponta Delgada, Portugal
- 3Oceanic Observatory of Madeira (OOM), Funchal, Portugal
- 4School of Earth Sciences, University of Bristol, Bristol, United Kingdom
- 5Lobosonda - Madeira Whale Watching, Estreito da Calheta, Portugal
- 6ITI/LARSyS, Técnico Lisboa, Universidade de Lisboa, Lisbon, Portugal
Species distributional estimates are an essential tool to improve and implement effective conservation and management measures. Nevertheless, obtaining accurate distributional estimates remains a challenge in many cases, especially when looking at the marine environment, mainly due to the species mobility and habitat dynamism. Ecosystems surrounding oceanic islands are highly dynamic and constitute a key actor on pelagic habitats, congregating biodiversity in their vicinity. The main objective of this study was to obtain accurate fine-scale spatio-temporal distributional estimates of cetaceans in oceanic islands, such as the Madeira archipelago, using a long-term opportunistically collected dataset. Ecological Niche Models (ENM) were built using cetacean occurrence data collected on-board commercial whale watching activities and environmental data from 2003 to 2018 for 10 species with a diverse range of habitat associations. Models were built using two different datasets of environmental variables with different temporal and spatial resolutions for comparison purposes. State-of-the-art techniques were used to iterate, build and evaluate the MAXENT models constructed. Models built using the long-term opportunistic dataset successfully described distribution patterns throughout the study area for the species considered. Final models were used to produce spatial grids of species average and standard deviation suitability monthly estimates. Results provide the first fine-scale (both in the temporal and spatial dimension) cetacean distributional estimates for the Madeira archipelago and reveal seasonal/annual distributional patterns, thus providing novel insights on species ecology and quantitative data to implement better dynamic management actions.
Introduction
While the construction of species distributional estimates is a crucial topic to conserve, protect, manage and monitor biodiversity (Rodríguez et al., 2007), it stills remains a challenge to obtain accurate and reliable products to ensure practical management actions (Araújo et al., 2019). One of the main tools used for these purposes are ecological miche models (ENMs). A class of methods that use occurrence data together with environmental data to make a correlative model of the environmental conditions that meet a species’ ecological requirements and predict the relative suitability of habitat (Warren and Seifert, 2011). Challenges are even more significant when estimating species distributions in the marine environment, where there are many factors to take into account, such as the species mobility and habitat dynamism (Redfern et al., 2006; Fernandez et al., 2017), or the difficulties of sampling at oceanic environments, primarily when referring to cetacean populations (Tyne et al., 2016).
Lately, several studies found that non-traditional data sources (such as opportunistic or citizen science data; e.g., Catlin-Groves, 2012; Embling et al., 2015) can be a cost-effective solution to overcome some of the challenges mentioned, allowing to produce relatively accurate cetacean abundance and distributional estimates (e.g., Fernandez et al., 2018; Robbins et al., 2019). When studying species in dynamic habitats, opportunistic surveys (and citizen science) can have many advantages over traditional methods. Formal and dedicated surveys provide poor coverage in time because of financial and logistic constraints, meaning that they cannot capture long-term variation in species distributions and occurrence.
Oceanic islands are a key actor on pelagic habitats, congregating biodiversity in their vicinity, primarily due to the “island-mass effect” (Doty and Oguri, 1956) or “island stirring” (Mann and Lazier, 1991), which is the topographic disturbance of oceanic flow by an island and its effects on the marine ecosystem. Several oceanographic features, such as wakes and eddies or vortices (e.g., Aristegui et al., 1994; Caldeira et al., 2002), are originated due to the presence of islands and have a direct effect on the local and regional productivity (Barton et al., 2000). Due to their dynamic nature, their effects on marine species are not well understood.
Cetaceans are marine mammals in the order Cetacea, which includes whales, dolphins, and porpoises. They have a strong influence on the marine ecosystems: as consumers of fish and invertebrates, as prey to other predators, as reservoirs of carbon, as vertical and horizontal vectors for nutrients and as detrital sources of energy and habitat in the deep-sea (Roman et al., 2014). Whales and dolphins are essential to ensure the correct functioning of the marine ecosystems worldwide, with a vital role in the biogeochemical cycles at biome and Earth system scale (Albouy et al., 2020; Norris et al., 2020). A high diversity of cetaceans (∼30 species) has been recorded in the Madeira Archipelago (NE Atlantic), including species featured in the Red List of the International Union for Conservation of Nature as Endangered, Vulnerable, and Data Deficient (Freitas et al., 2012; Alves et al., 2018). These waters host populations with some degree of residency, such as the short-finned pilot whale (Globicephala macrorhynchus) or the bottlenose dolphin (Tursiops truncatus) (Alves et al., 2013b; Dinis et al., 2016a). Other deep-diving cetacean species, such as the sperm whale (Physeter macrocephalus) and Blainville’s beaked whale (Mesoplodon densirostris), are among the most sighted species by commercial whale watching companies with some periodicity.
Moreover, baleen whales occur frequently in the archipelago, especially the Bryde’s whale (Balaenoptera edeni), with a relatively high occurrence rate but with a very high interannual variation (Alves et al., 2018). Recent studies revealed cetacean interconnectivity among neighboring archipelagos, such as the Canaries and the Azores, with re-sightings of individuals from several species among the three archipelagos (e.g., Alves et al., 2019; Dinis et al., 2021). Additionally, Madeira constitutes an area of interest for several (at least ten) cetacean species due to being used for traveling, feeding, resting, socializing and calving (Alves et al., 2018). However, despite its ecological importance, up to date, there are no reliable spatio-temporal distributional estimates for any of the cetacean species present in the area.
In this study, we provide, for the first time, a fine-scale spatio-temporal approach to obtain distributional estimates of cetaceans around the Madeira archipelago. Having a better knowledge of the species suitability throughout the year is critical for their conservation through maritime spatial planning and management of human activities. We built niche models for the ten most sighted cetacean species in Madeira, using two different datasets of environmental variables with different temporal and spatial resolutions. Models were iterated and selected using several state-of-the-art evaluation techniques.
Materials and Methods
Study Area and Data Collection
The Madeira archipelago is in the NE Atlantic (33°N, 17°W) and is mainly influenced by a branch of the Gulf Stream, the Azores Current system. Caldeira et al. (2002) suggested that the archipelago’s latitude might be the subtropical front, where cold-temperate waters from the north meet warm tropical waters from the south. The island mass effect of the archipelago is easily noticeable from satellite imagery, with wakes being formed on leeward areas and lee eddies spinning of both flanks of Madeira Island. Moreover, upwelling was detected near the island’s coasts, with the region between Madeira and Desertas Islands being particularly dynamic (Caldeira et al., 2002).
Cetacean occurrences were collected in an opportunistically way on-board commercial whale-watching vessels departing from two harbors (Calheta and Funchal) separated over 30 km on the South Coast of Madeira Island (Figure 1). The occurrences were collected by three operators (see section “Acknowledgments”) during their regular touristic trips from January 2003 until December 2018, with a total of 3,138 days sampled. Experienced observers from the companies collected location and species identification of each encounter. We applied a database filtering and cleaning to remove duplicate observations and incorrect GPS points clearly outside of the study area (or on land). A total of 8,607 sightings from 23 different species were selected during this period, from which the ten most sighted species were used in the present study (Table 1). Detailed methodological procedures on the data collection on-board commercial whale-watching vessels are given in Alves et al. (2018).
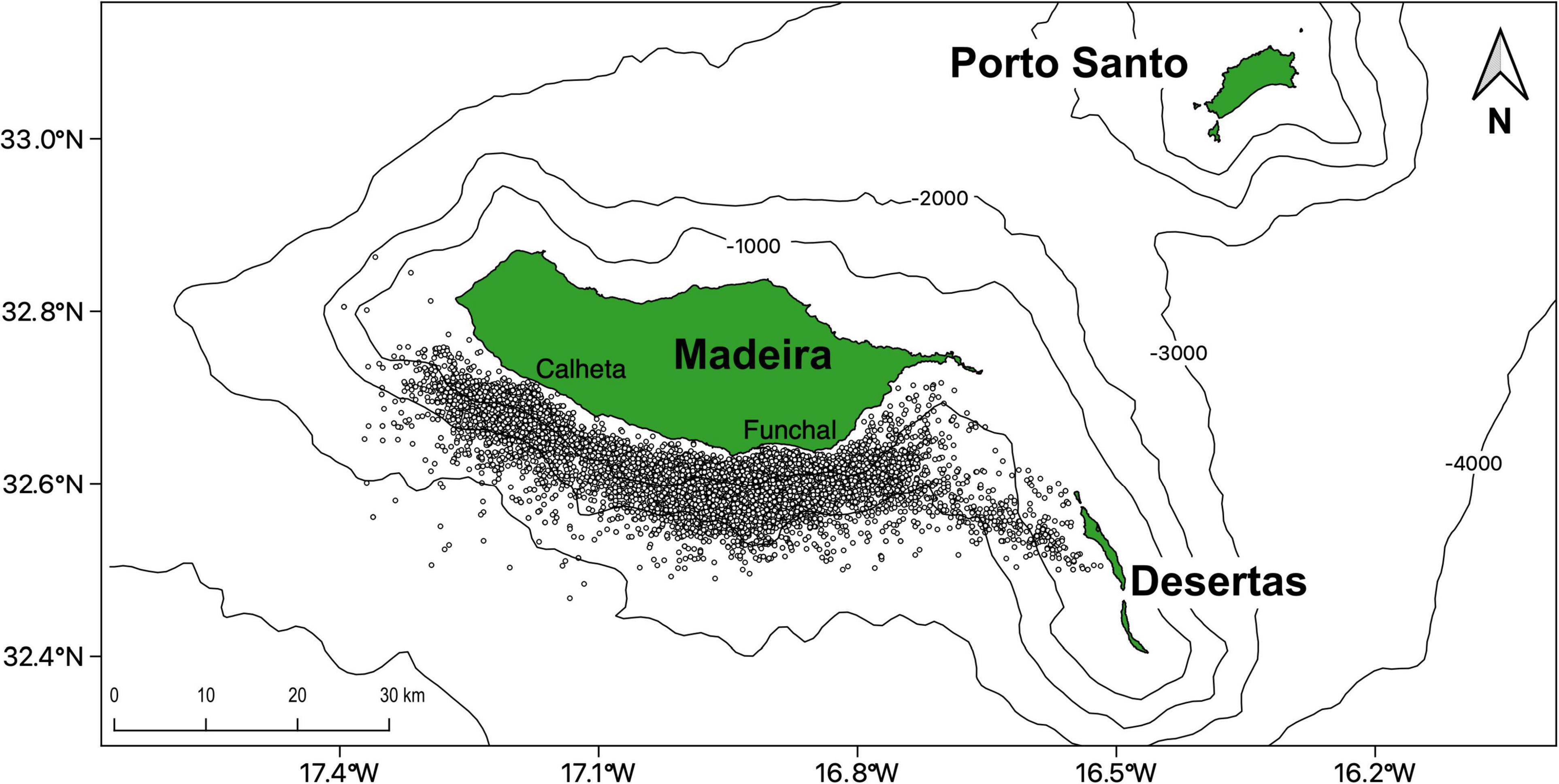
Figure 1. Cetacean sightings for 23 species (n = 8,607) pooled together, collected by the commercial whale watching companies departing from Funchal and Calheta in Madeira Island (Portugal) from 2003 until 2018.
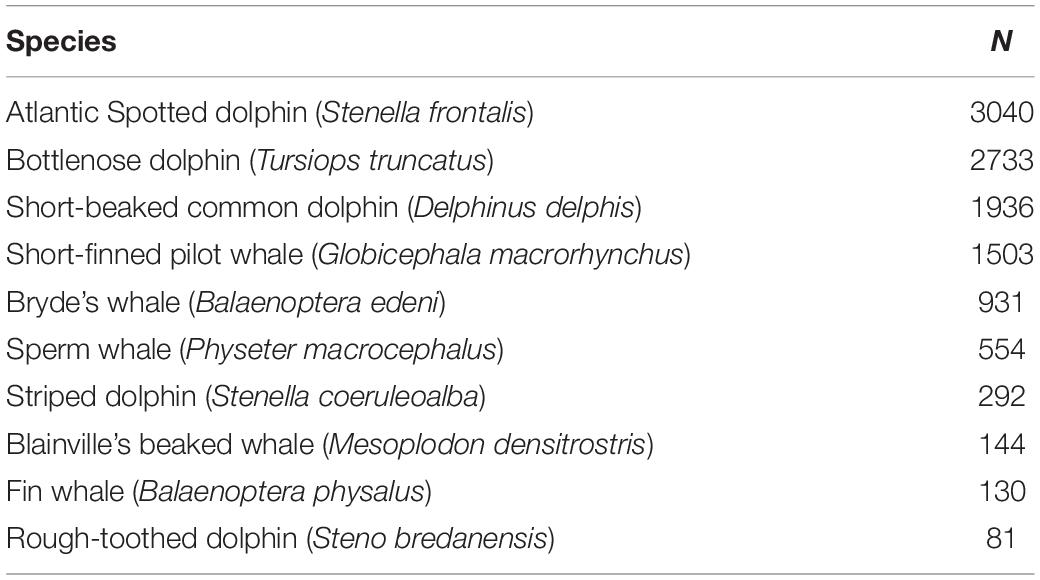
Table 1. Number of sightings for the 10 most sighted species (out of 23) by commercial whale watching companies from 2003 until 2018 used in the present study (n = 8,607).
Occurrences and Background Data
All occurrences records were projected onto 2 and 8 km grids to match the resolution of the two environmental datasets. Observations data collected on whale-watching operations might have a different source of biases due to the nature of the touristic activity. For example, it is not unusual that the same group of animals is visited more than once in a very similar location, both in space and time, which creates autocorrelation problems in contiguous grids. Therefore, a filtering approach was applied to remove potentially related sightings. A spatial thinning procedure was applied to all the sightings for both temporal groupings (1- and 8-days), using the spThin R package (Aiello-Lammens et al., 2015). Different sizes of the exclusion radius were tested (2, 4, and 6 km), selecting at the end a value of 2 km, which was the best compromise to reduce related sightings and still keep a good amount of observations. This agrees with the relatively small size of the sampled area (around 2,100 km2) by the whale-watching boats. Furthermore, during the modeling analysis, occurrences were also resampled to one occurrence per pixel for each temporal grouping.
Due to the sampling effort’s opportunistic nature, we applied a Minimum Sampled Area (MSA) approach, as Fernandez et al. (2017) used. All the sightings for each specific temporal scale were pooled together using a Minimum Convex Polygon, adding a 2 km buffer. Grids intersecting the polygon were taken as potentially sampled areas, therefore classified as background. The amount of effort per temporal unit (day or 8-days) was considered using the number of sea trips performed on a specific period. For each analysis, random background datasets (n = 10,000) were created, using the effort as a weighting factor.
Environmental Variables
A set of 19 environmental variables were used to calibrate the models (Table 2). Six terrain variables (depth, slope, and distance to the 1,000 m bathymetric lines, valley depth, distance to canyon-like features, and distance to major canyons) were derived from a digital elevation model (DEM) using the bathymetric dataset from the Instituto Hidrográfico of Portugal and interpolated using QGIS 3.1 at a resolution of 1 km. Physical features, such as the depth and the slope, can directly influence the distribution of cetaceans (e.g., Moore et al., 2000; Azzellino et al., 2008). Depth was directly read from the DEM; slope and distances to the 1,000 m bathymetric lines were calculated using QGIS 3.1. Moreover, other morphological features, such as canyons, can play an essential role in cetacean distributions. Canyons and other similar features can affect cetacean abundance patterns due to a series of physical features that enhance primary productivity and convert it to potential prey biomass (Moors-Murphy, 2014). These effects are even more noticeable when dealing with deep-diving cetacean species, which might directly rely on these areas for feeding purposes (Breen et al., 2020).
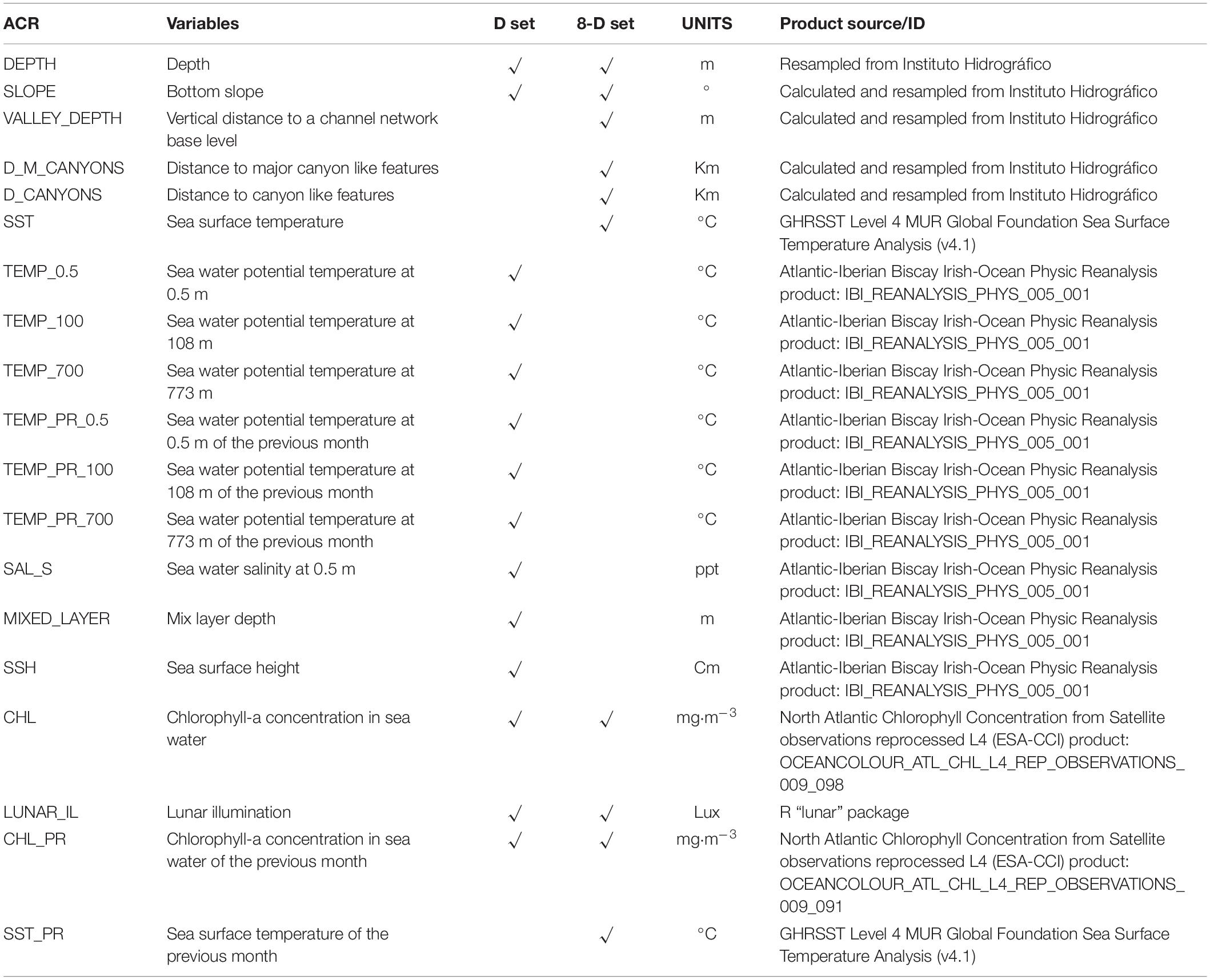
Table 2. Variables used to construct the two different assemblages of layers for the present analysis: the (1) “D” set with a spatial resolution of 7.8 km and a temporal resolution of 1 day, and the (2) “8-D” set, with a spatial resolution of 2 km and a temporal resolution of 8-days and 1 month.
We calculated a series of morphological variables to include the effects of morphological features as prey aggregation areas into the models. The valley depth refers to the vertical distance to a channel network base level; it was calculated using the QGIS module “Relative Heights and Slope Positions” based on Boehner and Selige (2006). The canyon-like features were calculated using the topographic position index (TPI), which measures where a point is in the overall landscape/seascape to identify features such as ridges, canyons, or midslopes (Wright and Heyman, 2008). We computed the TPI with the SAGA GIS1 implementation (based on Guisan et al., 1999; Weiss, 2001), using a radius of 3,000 m. We selected features corresponding to V-shape river valleys and deep narrow canyons (Weiss, 2001). We applied a spatial filter (<3km) to eliminate artifacts and minor features. Another layer focusing only on the major canyon-like features was identified, selecting features with an extension larger than 25 squared km. Distance to the edge of these structures was calculated.
Nine of the surface and deep waters oceanographic variables used for model building were obtained through the Copernicus Marine system, with a daily, 8-day, and monthly temporal resolution; through the IBI (Iberian Biscay Irish) Ocean Reanalysis system (see Table 2). The IBI model numerical core is based on the NEMO v3.6 ocean general circulation model run at 1/12° horizontal resolution, assimilating altimeter data, in situ temperature, vertical salinity profiles, and satellite sea surface temperature. The surface chlorophyll (Chl-a) data was obtained from the ESA Ocean Color CCI Remote Sensing Reflectance data (by merging layers from SeaWiFS, MODIS-Aqua, MERIS, and VIIRS sensors and realigning the spectra to that of the SeaWiFS sensor) using the regional OC5CCI chlorophyll algorithm. Moreover, the Sea Surface Temperature (SST) was obtained from the Group for High-Resolution Sea Surface Temperature (GHRSST), a global, gap-free, gridded, daily 1 km dataset created by merging multiple Level-2 satellite SST datasets. Depending on the analysis performed, layers were scaled to the desired temporal and spatial resolution.
We applied a variance inflation factor (VIF) approach as implemented in the R package usdm (Naimi, 2015) to test for collinearity. Two of the environmental layers had collinearity issues, the distance to 1,000 m bathymetric line and the distance to major canyons. Due to the potential ecological importance of canyons, we excluded the distance to 1,000 m bathymetric isoline, keeping all the canyon-related variables (see the final variables selected for the analysis in Table 2).
Two different sets of environmental layers were constructed. The first assemblage (the daily set) aimed to detect the effect of dynamic variables at a coarse spatial resolution (7.8 km) and included a set of nine oceanographic variables at different depths with a daily resolution. The cumulative effect of variables (temperature and Chl-a) was measured as the mean values for the 30 days previous to the sightings. The second group of layers (the 8-day set) aimed to detect the influence of topographic features on a fine-scale resolution (2 km), including a set of 4 oceanographic variables with an 8-day (Table 2). The 30-day mean values of temperature and Chl-a were included to test for those variables’ cumulative effects.
Modeling Building and Evaluation
Due to the opportunistic nature of the data used in this study, without real absences, we used a presence-background algorithm MAXENT (Phillips et al., 2006) to infer the ecological niche model of the selected species.
The kuenm package (Cobos et al., 2019a) in R was used to select the most important variables, build and evaluate the MAXENT models. Data was introduced using the sightings with data (SWD) formatting, adding the temporality factor. Models were built with 75% occurrences for training purposes. For each species or family, we created thousands of candidate models (more than 10,000 for each group) by combining all the potential different sets of environmental predictors, three values of regularization multiplier (1, 1.5, 2), and five possible combinations (“l,” “lq,” “lqp,” “lqpt,” and “lqpth”) of the feature classes (linear = l, quadratic = q, product = p, threshold = t, and hinge = h). The regularization parameter allows MAXENT to limit the model complexity (protecting it against overfitting), adding a penalty for each term included in the model and higher weights given to a term (Phillips et al., 2006). Overfitted models excel in predicting non-independent evaluation data, leading to an inappropriate automatic selection of low regularization values; better model performance is generally achieved at slightly (or substantially) higher regularization parameters (Radosavljevic and Anderson, 2014). Feature classes are used in MAXENT to build the responses curves. Depending on the feature classes selected, different response curves can be obtained, from very simple to highly complex non-linear curves (Merow et al., 2013).
Model evaluation was carried out following the implementation of kuenm based on statistical significance, through partial ROC (Receiver Operator Curve), with 500 iterations and 50% of data for bootstrapping (Peterson et al., 2008), omission rates with a threshold of E = 5% (Anderson et al., 2003) and model complexity based on the findings of Warren and Seifert (2011) on the AICc index (indicates how well models fit the data while penalizing complexity to favor simple models). Best models were selected according to (1) significant models with (2) omission rates ≤5%. Then, from among this model set, models with delta AICc values of ≤2 were chosen as final models. The exhaustive method implemented in kuenm ensures better performance of the models selected and reduces both overfitting and underfitting when comparing it to a classical heuristic method (Cobos et al., 2019b).
The best models were selected by the kuenm process, only in one case (fin whales on the daily scenario); we did not select the best option given by kuenm, but one of the other potential candidates selected during the process. In this case, the best model was slightly overfitted to coastal areas due to the relatively low number of observations for the species and the bias associated with the whale-wacthing activity. Therefore, we selected a model with no restrictions on the Depth variable, as we know that fin whales are also frequently sighted offshore.
To select between the two different environmental datasets tested (with different temporal and spatial resolution), we used an expert-based omission criterion (areas/time being classified as unsuitable when they are not). Experts evaluation and knowledge were based on empirical observations of the target species around the archipelago in different periods outside the study area. However, as these datasets were not available at the moment of the evaluation, we used the expert-based omission criterion as a complementary validation method.
Projections of the models were made for each combination of year/month from 2003 to 2018 using a clamping approach to avoid extrapolations to areas outside of the range of the training conditions (Merow et al., 2013). Finally, the mean suitability values and standard deviation for all months through all years were calculated.
Results
Model Performance
A total of 87,300 model solutions were generated for the 8-day dataset, and 300,300 were generated for the daily data. The best results were selected according to their relative predictive and explanatory capabilities. According to expert knowledge criteria, models built using the 8-day dataset, with a higher spatial resolution and more topographic-based variables, had a better predictive performance. For 8 of the 10 species considered in the present study (G. macrorhyncus, P. macrocephalus, M. densirostris, S. frontalis, T. truncatus, D. delphis, S. coeruleoalba, and S. bredanensis), the 8-day scenario produced better results (Table 3). Only two species (B. edeni and B. physalus) produced better estimates when using the daily dataset. The results are presented per functional ecological groups: (i) deep-divers (short-finned pilot whales, sperm whales and Blainville’s beaked whale), (ii) delphinids (bottlenose dolphins, rough-toothed dolphins, Atlantic spotted dolphins, short-beaked common dolphins and striped dolphins), and (iii) balaenopterids (fin whales and Bryde’s whales).
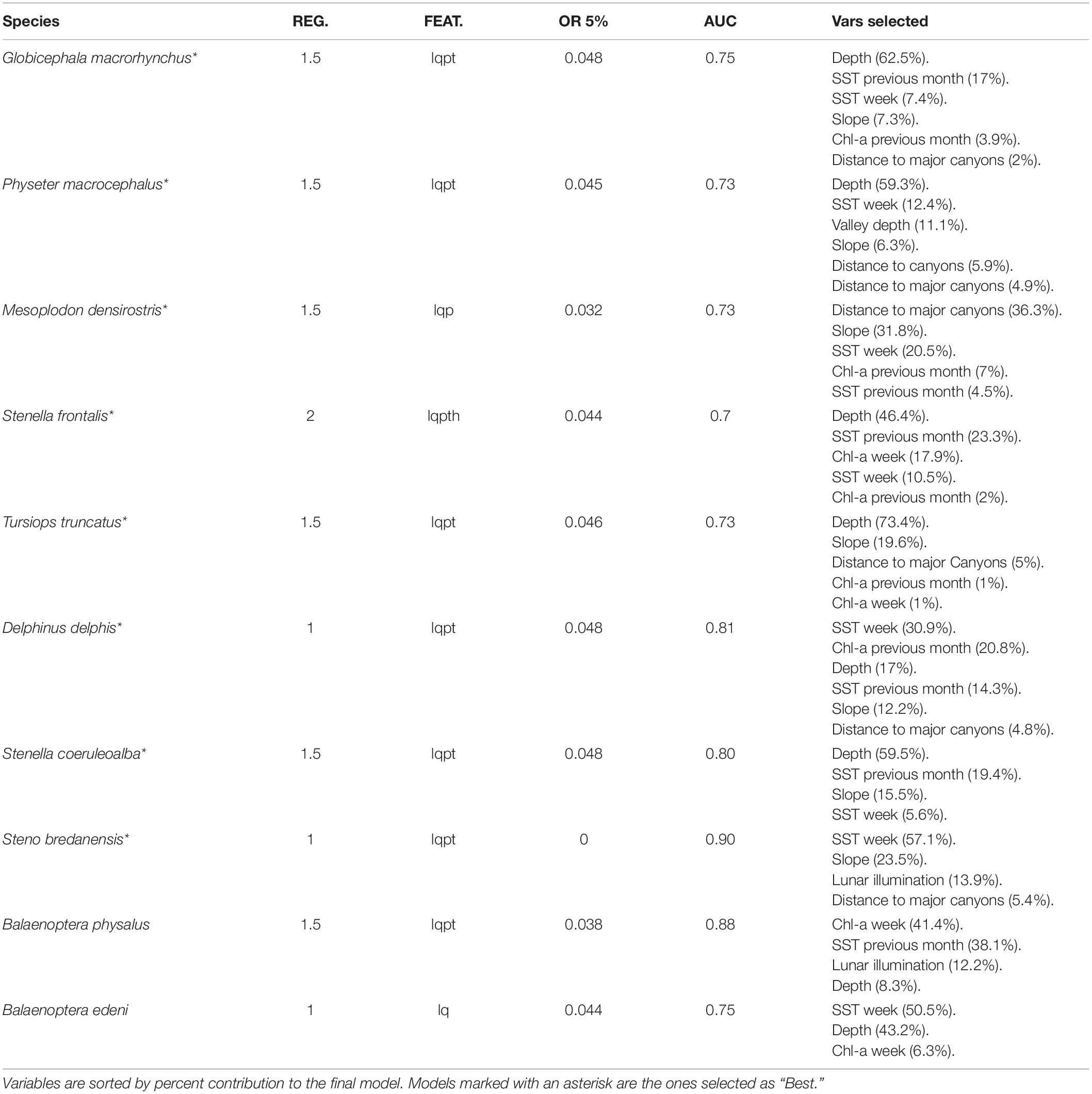
Table 3. Eight-days models regularization multiplier (REG.), feature classes (linear = l, quadratic = q, product = p, threshold = t, and hinge = h), omission rate at 5% (OR 5%), area under the curve (AUC), and variables selected (with its contribution) for each species.
Deep-Divers
All the deep-diving species higher suitability values were related to the bathymetry or other topographic variables. The short-finned pilot whales (G. macrorhynchus) suitable areas were related with depths between 1,000 and 2,000 m with intermediate slope values and a slight preference for regions closer to major canyons (Figure 2). The SST played a relevant role in the species niche, with a preference for warm waters (18–24°C) and low Chl-a values. The daily analysis results showed an influence of the shallow mixed layer in the suitability. Models projections suggest a patched temporal and spatial distribution of the species, with highly suitable areas found at the South-east and North of Madeira. Seasonal variability was found, with lower suitability values from March to July. However, high standard deviation values were found in March and April (Supplementary Figure 1). Lastly, the daily models showed a subtle influence of the lunar illumination on the species, with higher suitability indexes with lower values.
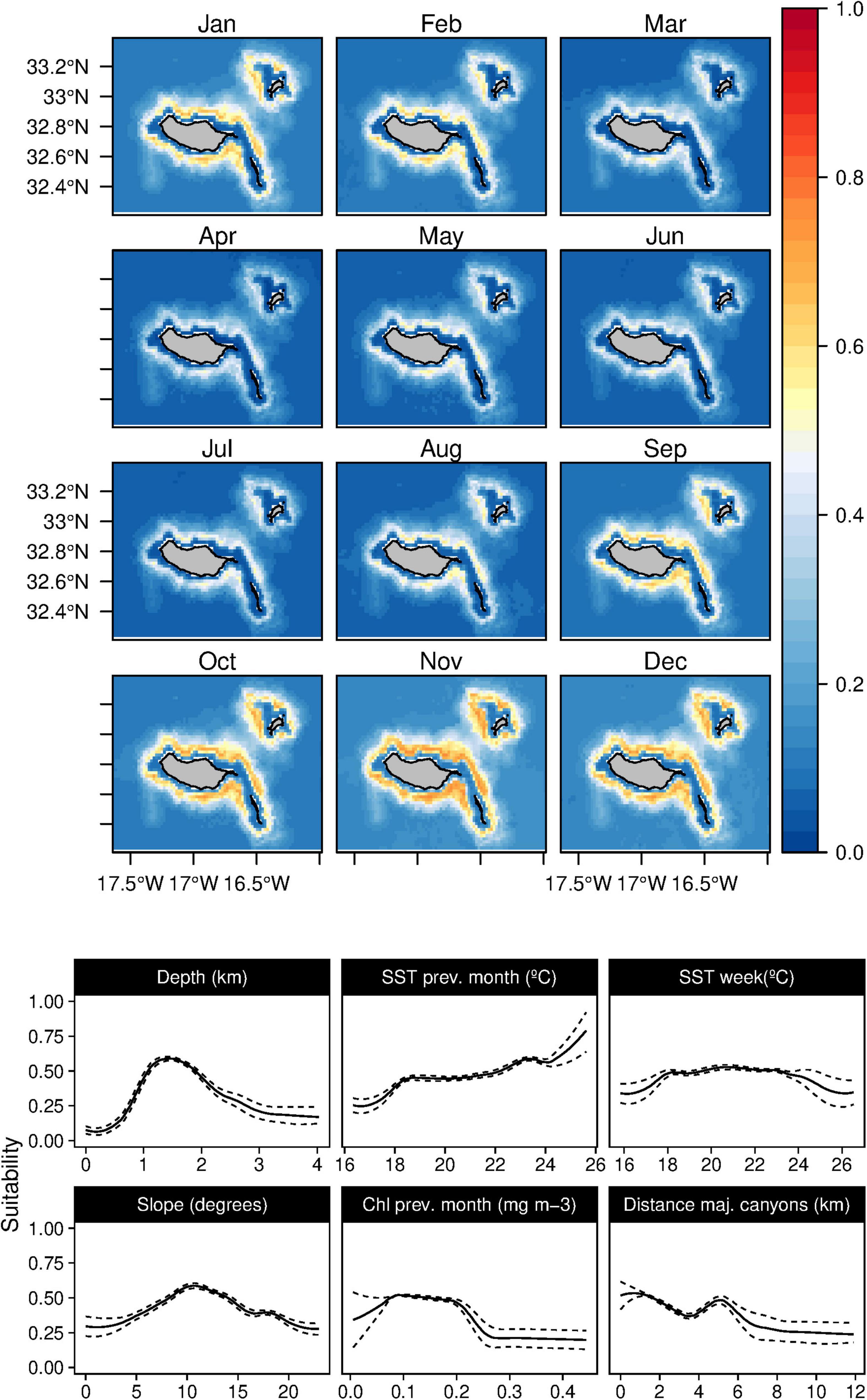
Figure 2. Mean monthly suitability maps (above) and smoothed response curves with standard deviation (below) in the Madeira archipelago for Globicephala macrorhynchus for the “8-days” models. In the maps, red represents more suitable and blue less suitable. Responses curves are ordered by percent contribution of the environmental variables.
Sperm whales (P. macrocephalus) habitat was related with waters deeper than 1 km, with an intermediate slope and closer to any type of canyons (Figure 3). The analysis suggested a relation with areas with low valley depths and close to canyons on mid-slope areas. Only one oceanographic variable was found relevant for the model selected (the SST), denoting a slight preference of the species for warmer waters. Low standard deviation values of suitability were found (Supplementary Figure 2) for all the months.
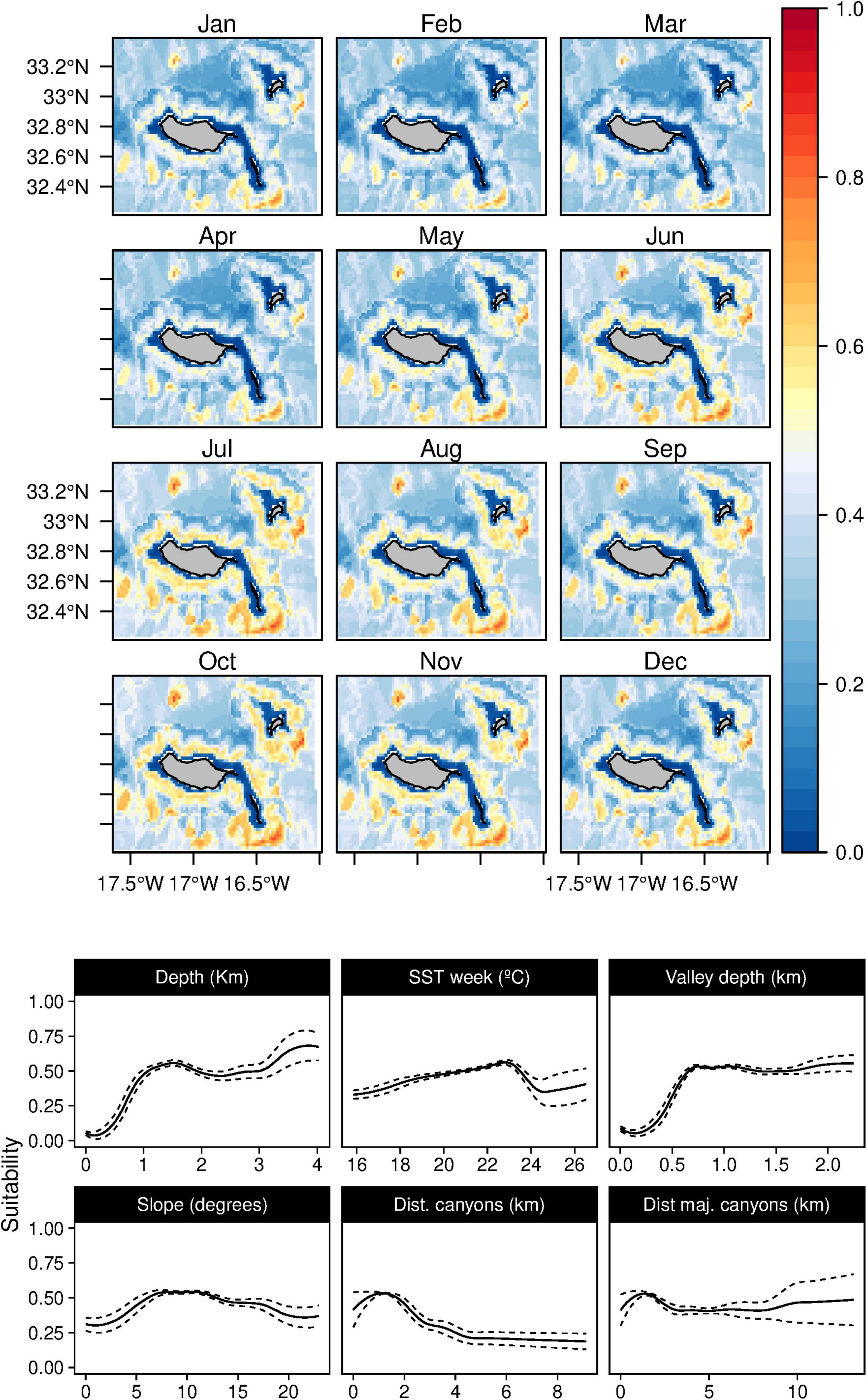
Figure 3. Mean monthly suitability maps (above) and smoothed response curves with standard deviation (below) in the Madeira archipelago for Physeter macrocephalus for the “8-days” models. In the maps, red represents more suitable and blue less suitable. Responses curves are ordered by percent contribution of the environmental variables.
Blainville’s beaked whales (M. densirostris) models showed a significant contribution of the major canyons, slope, and SST, with a total contribution of 88.6% on the model construction (Table 3). The species was related to areas with major canyons, with high slope values and a positive correlation with warm waters (Figure 4). Moreover, a marginal effect of the Chl-a and SST on the previous month was also observed. This species showed a patchy distribution primarily in the vicinity of canyons and a temporal preference for summer and early autumn. Nonetheless, the standard deviation maps (Supplementary Figure 3) show relatively high values from January to April.
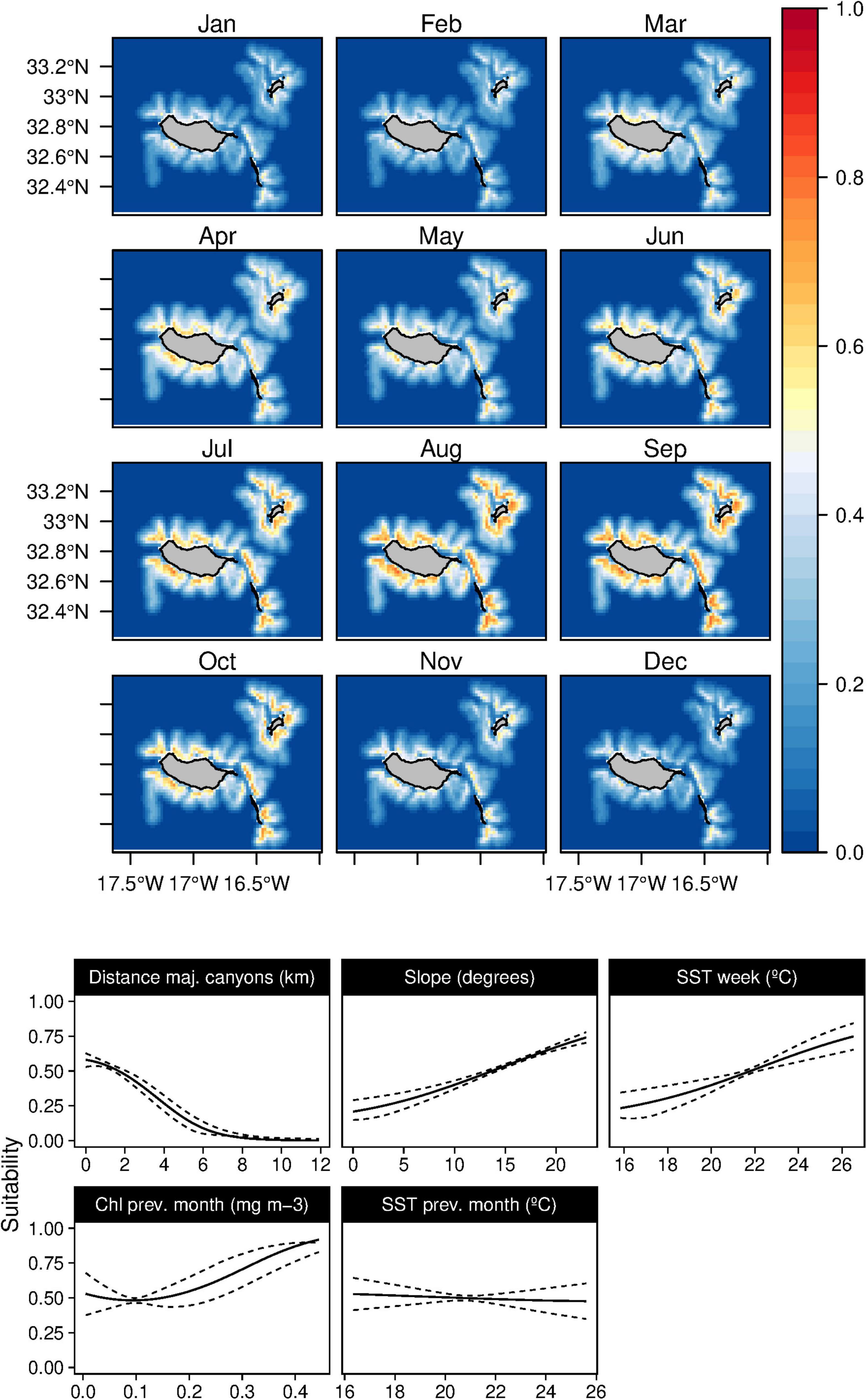
Figure 4. Mean monthly suitability maps (above) and smoothed response curves with standard deviation (below) in the Madeira archipelago for Mesoplodon densirostris for the “8-days” models. In the maps, red represents more suitable and blue less suitable. Responses curves are ordered by percent contribution of the environmental variables.
Delphinids
The suitability for bottlenose dolphins (T. truncatus) was found to be primarily related to the bathymetry, slope, and distance to the major canyons, contributing up to 98% to the selected model (Table 3). The species suitability was positively linked to relatively shallow waters with high slope values and somehow close to major canyons. Even if marginal, there is also a preference for waters with high Chl-a values (Figure 5). These outcomes produced a generalist distribution of the species on Madeira’s coastal areas with almost no temporal variability, also reflected on the low values of standard deviation maps (Supplementary Figure 4).
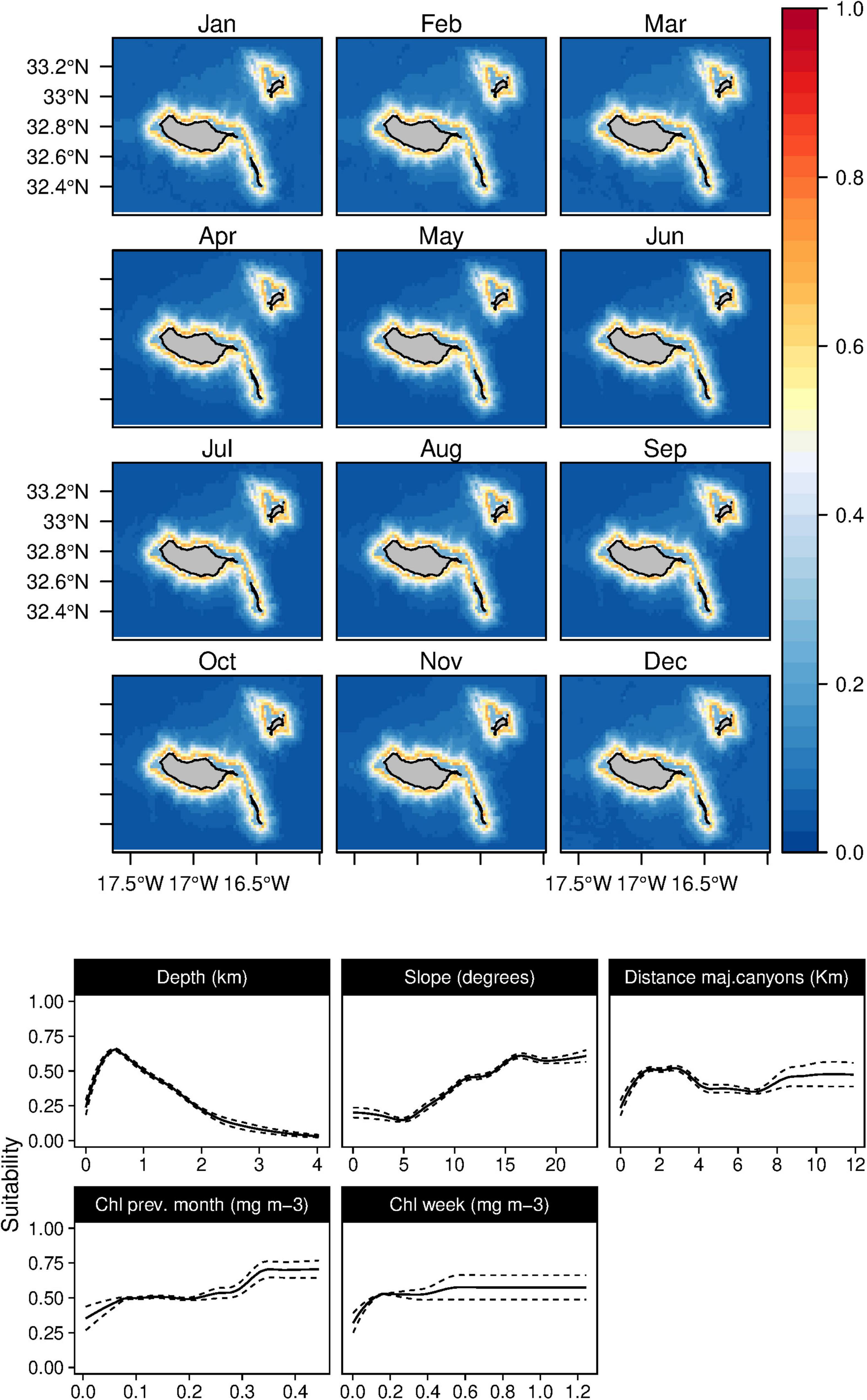
Figure 5. Mean monthly suitability maps (above) and smoothed response curves with standard deviation (below) in the Madeira archipelago for Tursiops truncatus for the “8-days” models. In the maps, red represents more suitable and blue less suitable. Responses curves are ordered by percent contribution of the environmental variables.
The weekly SST was found to be the most influential factor (Figure 6) determining the rough-toothed dolphins (S. bredanensis) niche, being linked to warmer waters (above 20°C). The daily scenario models also showed a relation between warm waters and shallow mixed layer depth. Topographically, the niche was linked with mid and high slope values in the proximity of major canyons. The results revealed a relation between rough-toothed dolphin niche and low lunar illumination values. Relatively high suitability values for the species were found from July to October, with high standard deviation values in June and November (Supplementary Figure 5).
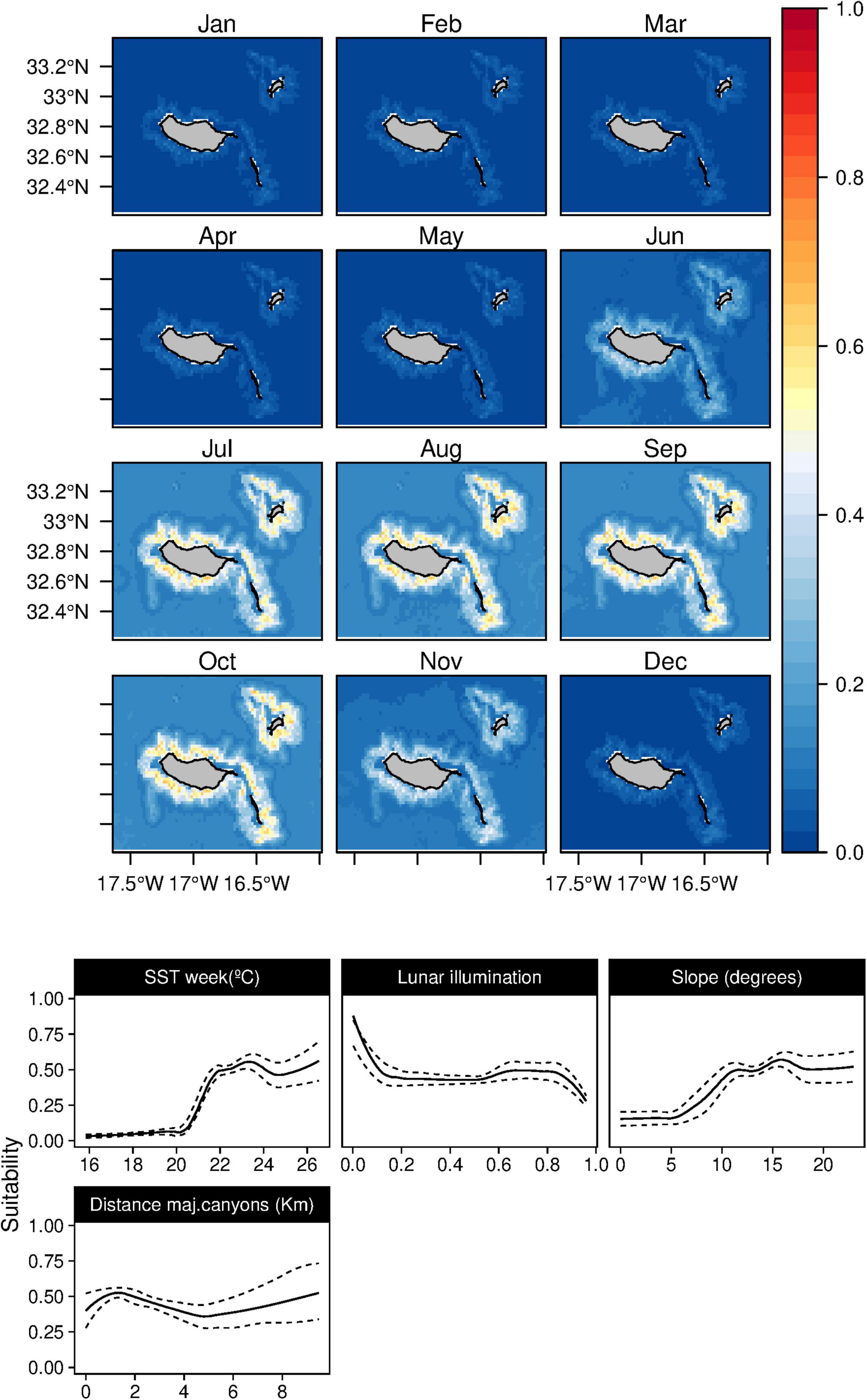
Figure 6. Mean monthly suitability maps (above) and smoothed response curves with standard deviation (below) in the Madeira archipelago for Steno bredanensis for the “8-days” models. In the maps, red represents more suitable and blue less suitable. Responses curves are ordered by percent contribution of the environmental variables.
In both of the modeling approaches used in this study, only one topographic variable was identified as an important predictor for the Atlantic spotted dolphins (S. frontalis), the bathymetry (Tables 3, 4). The species showed a preference for relatively deep-waters around the 1,000 m isoline. Moreover, both during the 8-day and in the previous month, the temperature was selected as the models’ relevant variables. Atlantic spotted dolphins niche was related with temperate/warm waters (over 18°C) with very low productivity values (Figure 7), leading to higher suitability values from June until October. However, high standard deviation values are found between February and May (Supplementary Figure 6), which denotes the high variability of the suitability indexes in those months.
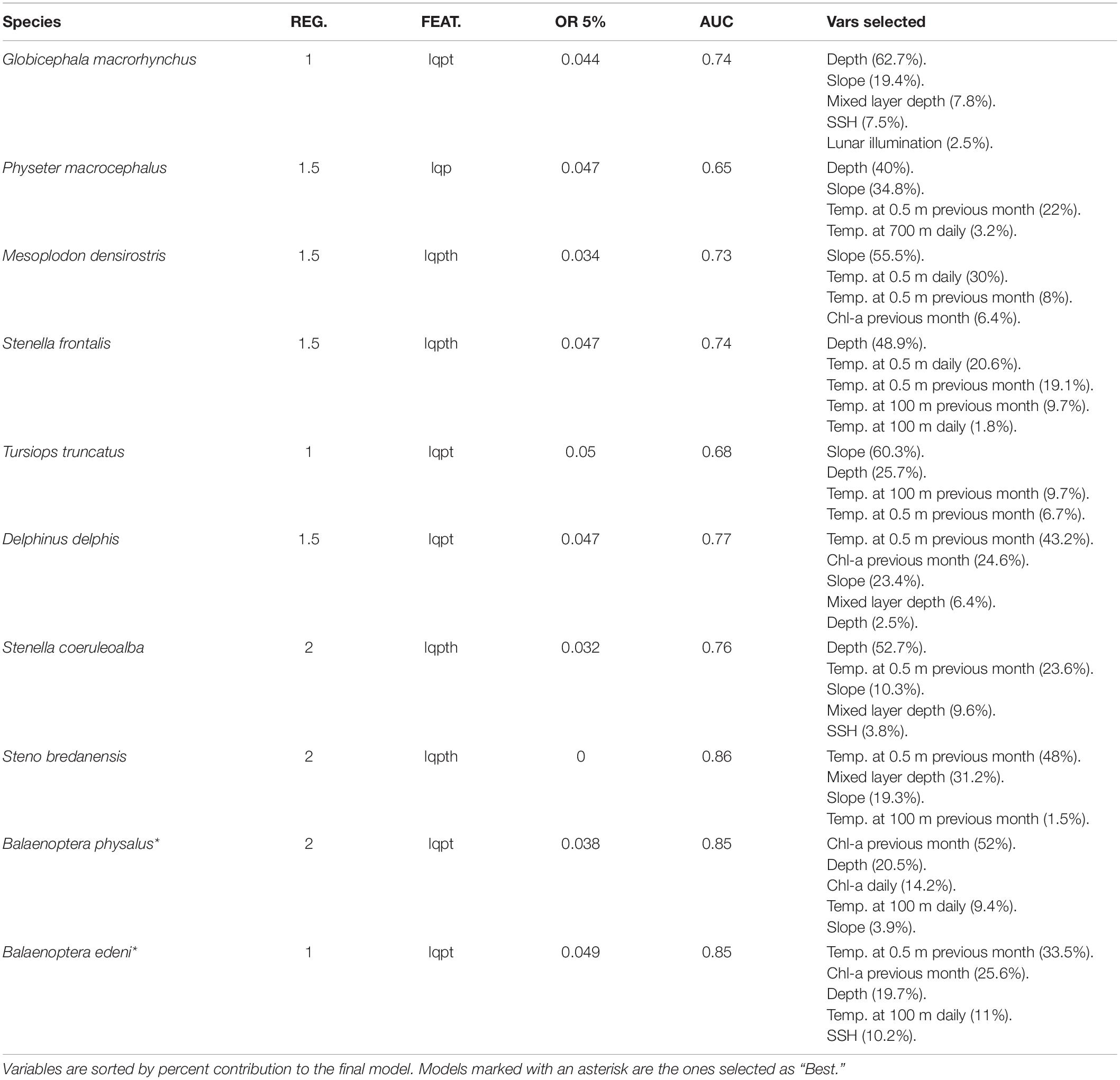
Table 4. Daily models regularization multiplier (REG.), feature classes (linear = l, quadratic = q, product = p, threshold = t, and hinge = h), omission rate at 5% (OR 5%), area under the curve (AUC), and variables selected (with its contribution) for each species.
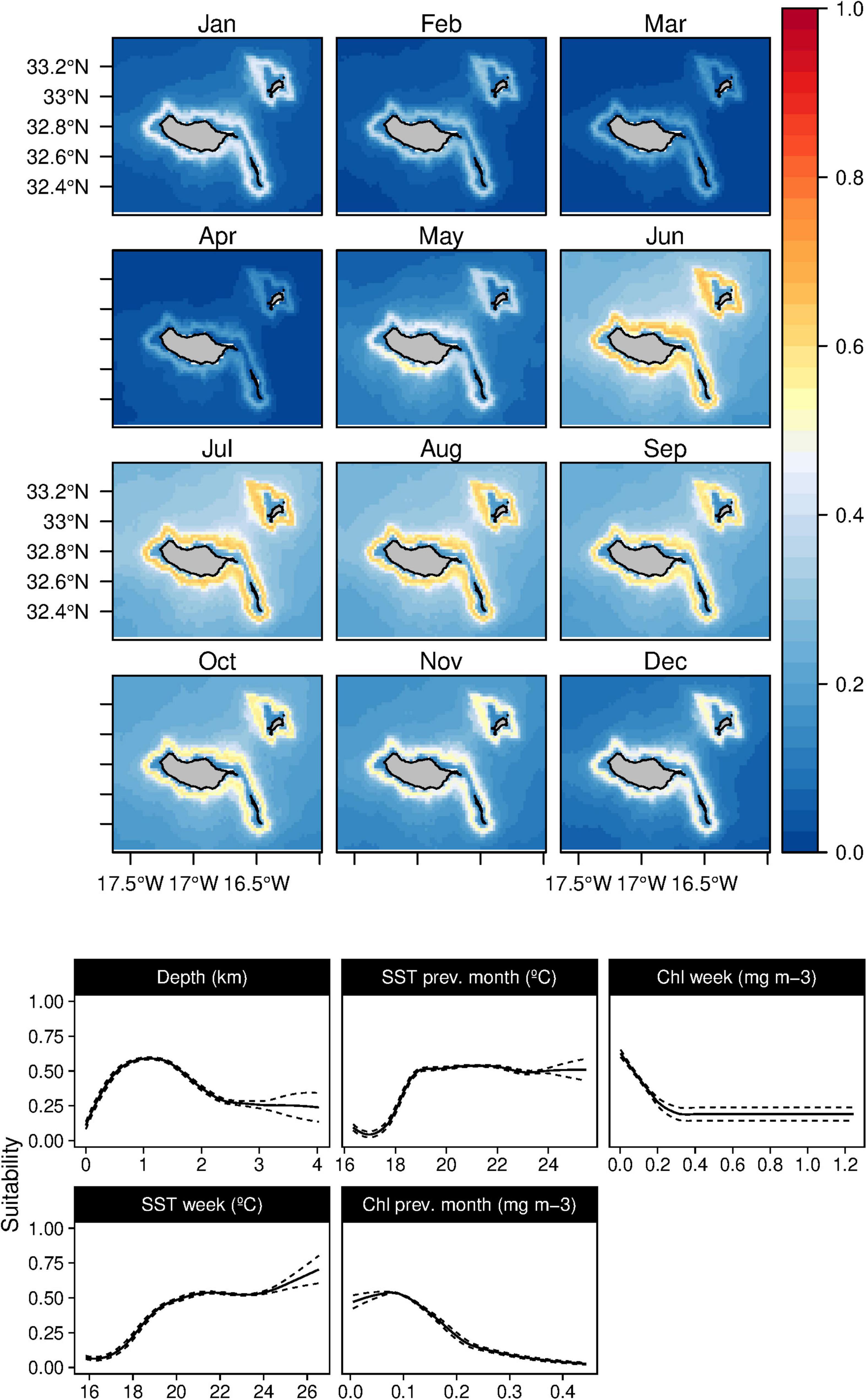
Figure 7. Mean monthly suitability maps (above) and smoothed response curves with standard deviation (below) in the Madeira archipelago for Stenella frontalis for the “8-days” models. In the maps, red represents more suitable and blue less suitable. Responses curves are ordered by percent contribution of the environmental variables.
In contrast, the short-beaked common dolphins (D. delphis) presented a strong relation with lower values of SST (both in the weekly and previous month) along with high values of Chl-a during the previous month (Figure 8). This delphinid niche was also related to relatively shallow waters, moderate to high slope regions, and areas closer to major canyons. Moreover, the daily model showed an influence of the mixed layer depth on the species niche. Common dolphins had higher suitability values in winter and spring (from December to June), with moderate standard deviation values in June (Supplementary Figure 7). The striped dolphins (S. coeruleoalba) were predominantly related to deep waters regions (over 1,000 m) and moderate slope values (Figure 9), alongside a preference for temperate waters (around 19–20°C). While the suitability values obtained indicate a year-round presence of these delphinids in the archipelago, higher suitability values were found from spring to early summer, with very low standard deviation values (Supplementary Figure 8).
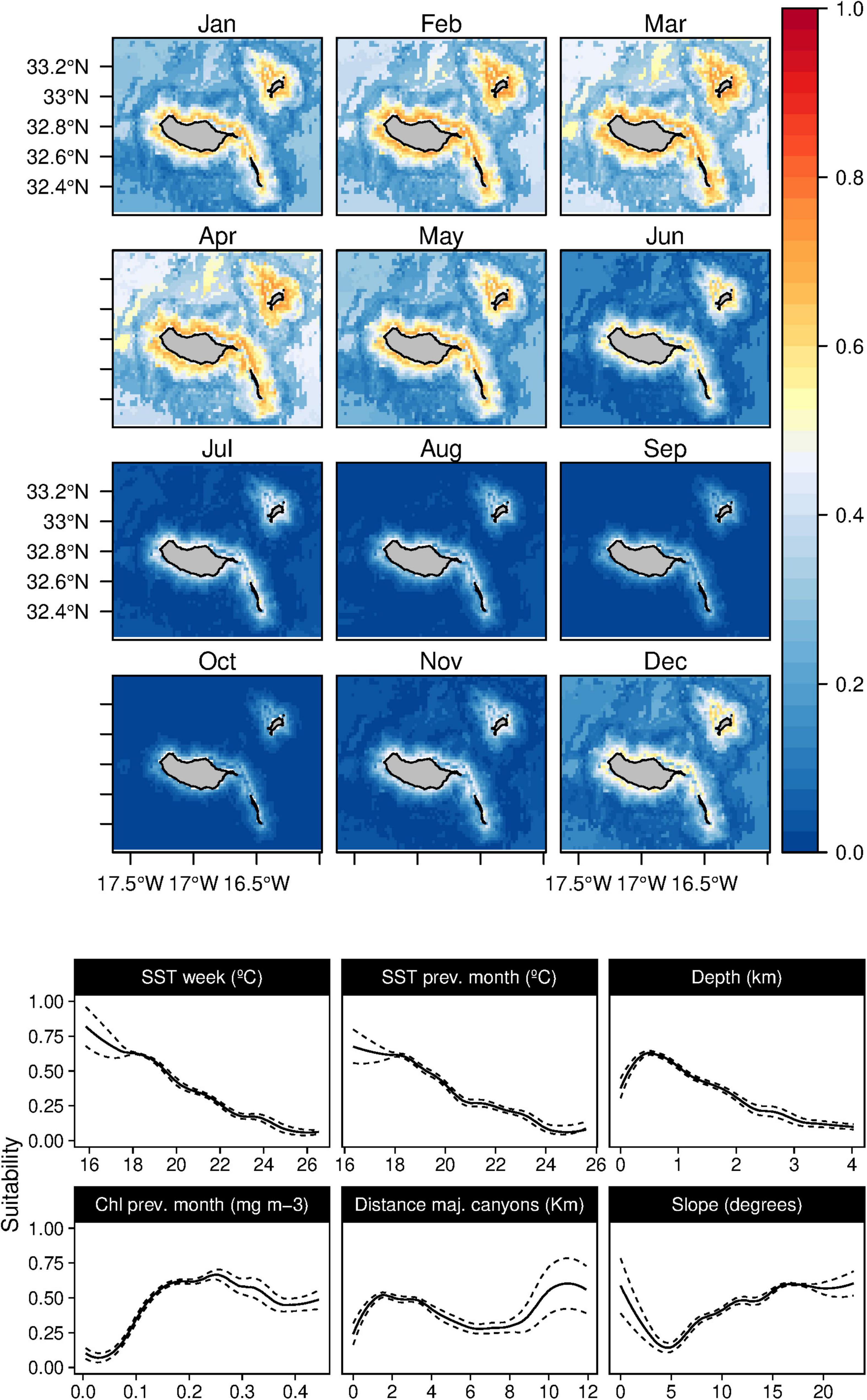
Figure 8. Mean monthly suitability maps (above) and smoothed response curves with standard deviation (below) in the Madeira archipelago for Delphinus delphis for the “8-days” models. In the maps, red represents more suitable and blue less suitable. Responses curves are ordered by percent contribution of the environmental variables.
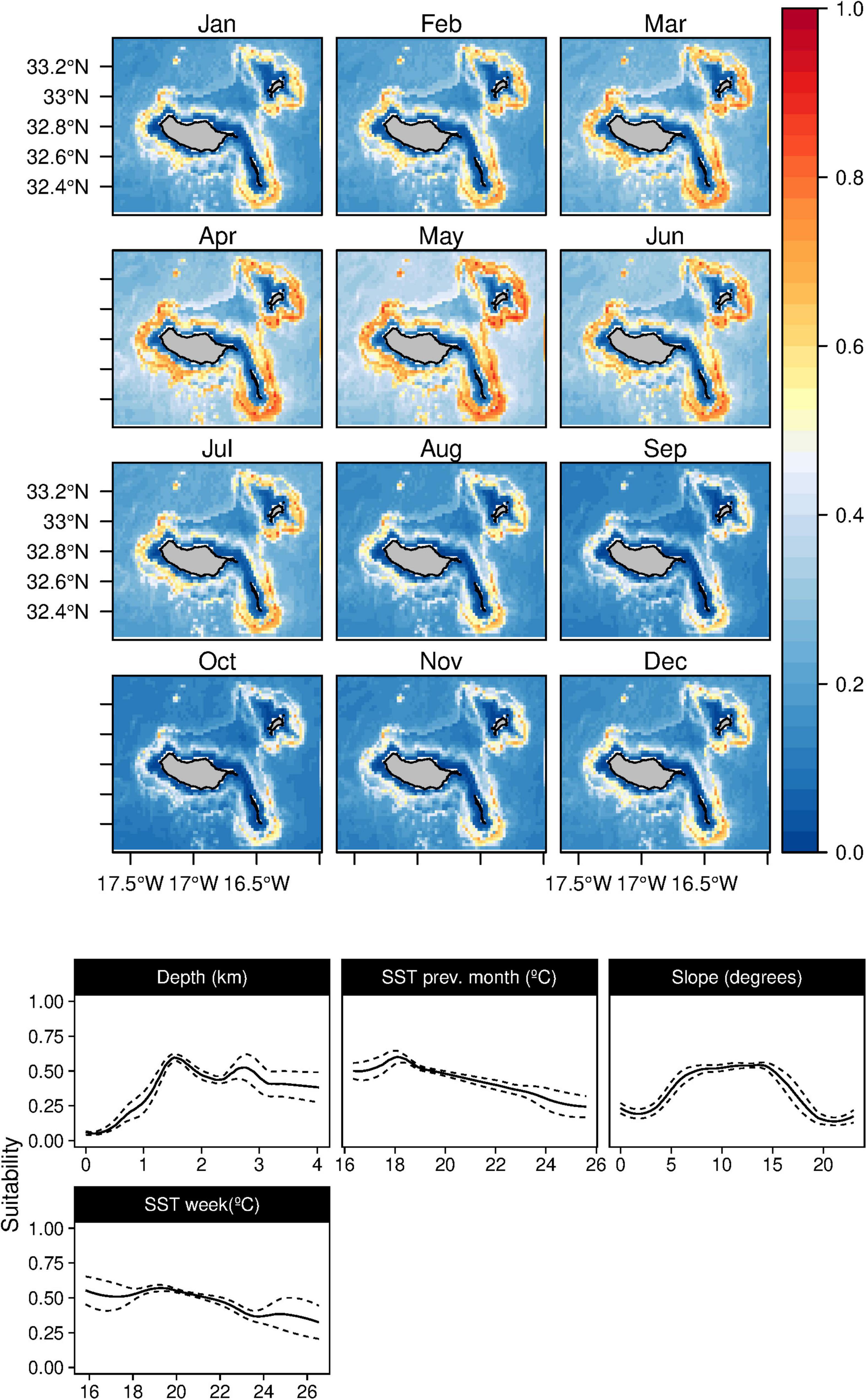
Figure 9. Mean monthly suitability maps (above) and smoothed response curves with standard deviation (below) in the Madeira archipelago for Stenella coeruleoalba for the “8-days” models. In the maps, red represents more suitable and blue less suitable. Responses curves are ordered by percent contribution of the environmental variables.
Balaenopterids
Oceanographic variables were constantly selected as the most influential variables for the baleen whales’ ecological niche in the present study. The fin whales suitability (B. physalus) was influenced mainly by moderate values of Chl-a in the previous month, together with high daily Chl-a values and low daily values of temperature in 100 m depth. Topographically, the species niche was related to intermediate bathymetry (with a peak at 2,000 m) and mid-slope values (Figure 10). Suitability maps exhibited elevated values from February to May, with high standard deviation values from February to August (Supplementary Figure 9).
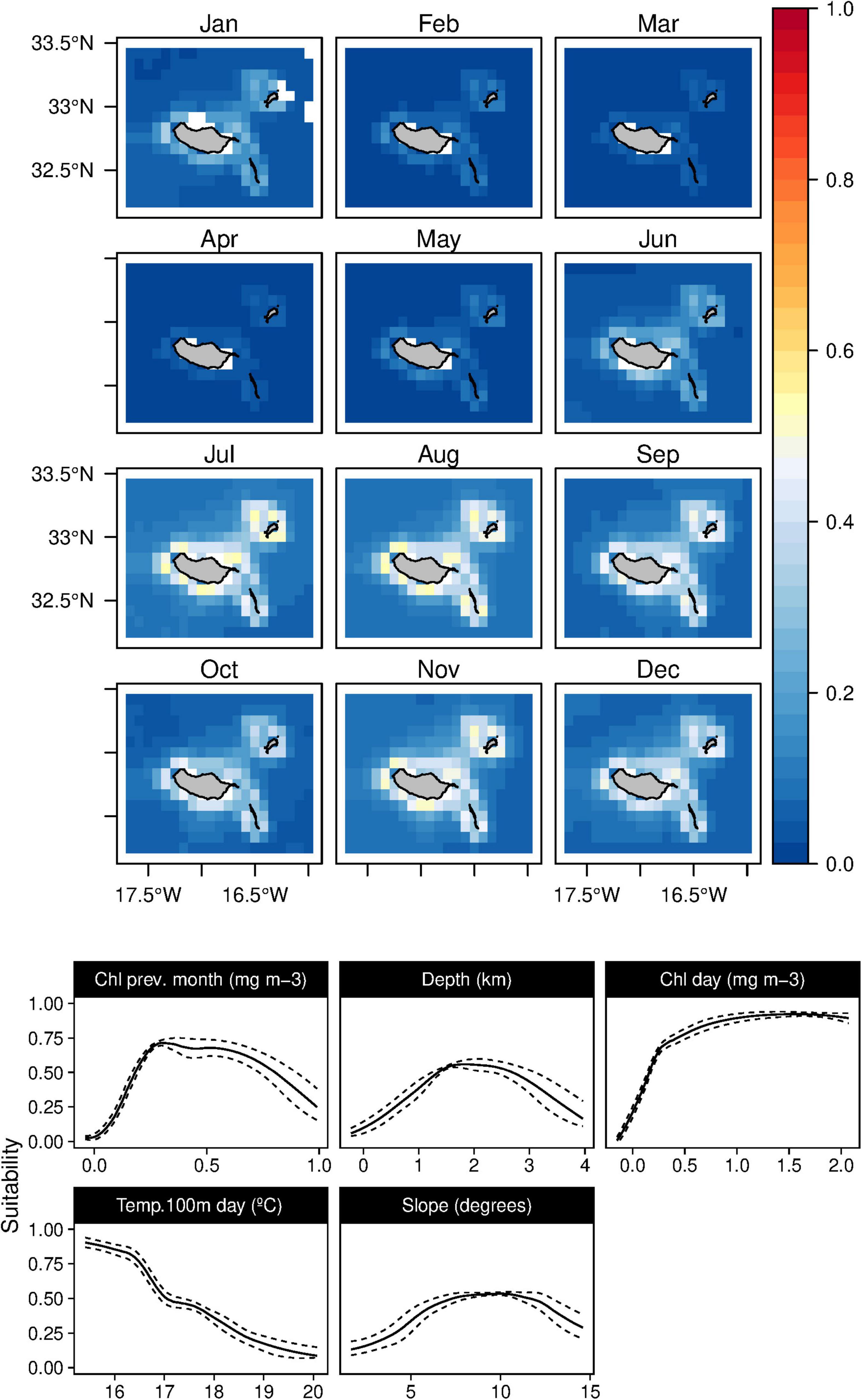
Figure 10. Mean monthly suitability maps (above) and smoothed response curves with standard deviation (below) in the Madeira archipelago for Balaenoptera physalus for the “daily” models. In the maps, red represents more suitable and blue less suitable. Responses curves are ordered by percent contribution of the environmental variables.
Conversely, very low values of Chl-a during the previous month were more related to the Bryde’s whales (B. edeni) niche, together with sea surface temperatures in the previous month between 20 and 24°C (Figure 11). This species’ results also indicated a relation with waters around 1,000 m depth and a positive correlation with the warmer daily temperature at 100 m. Suitability values for Bryde’s whales were relatively low, with a slight increase from June to January; however, high standard deviation values were found from June to November (Supplementary Figure 10).
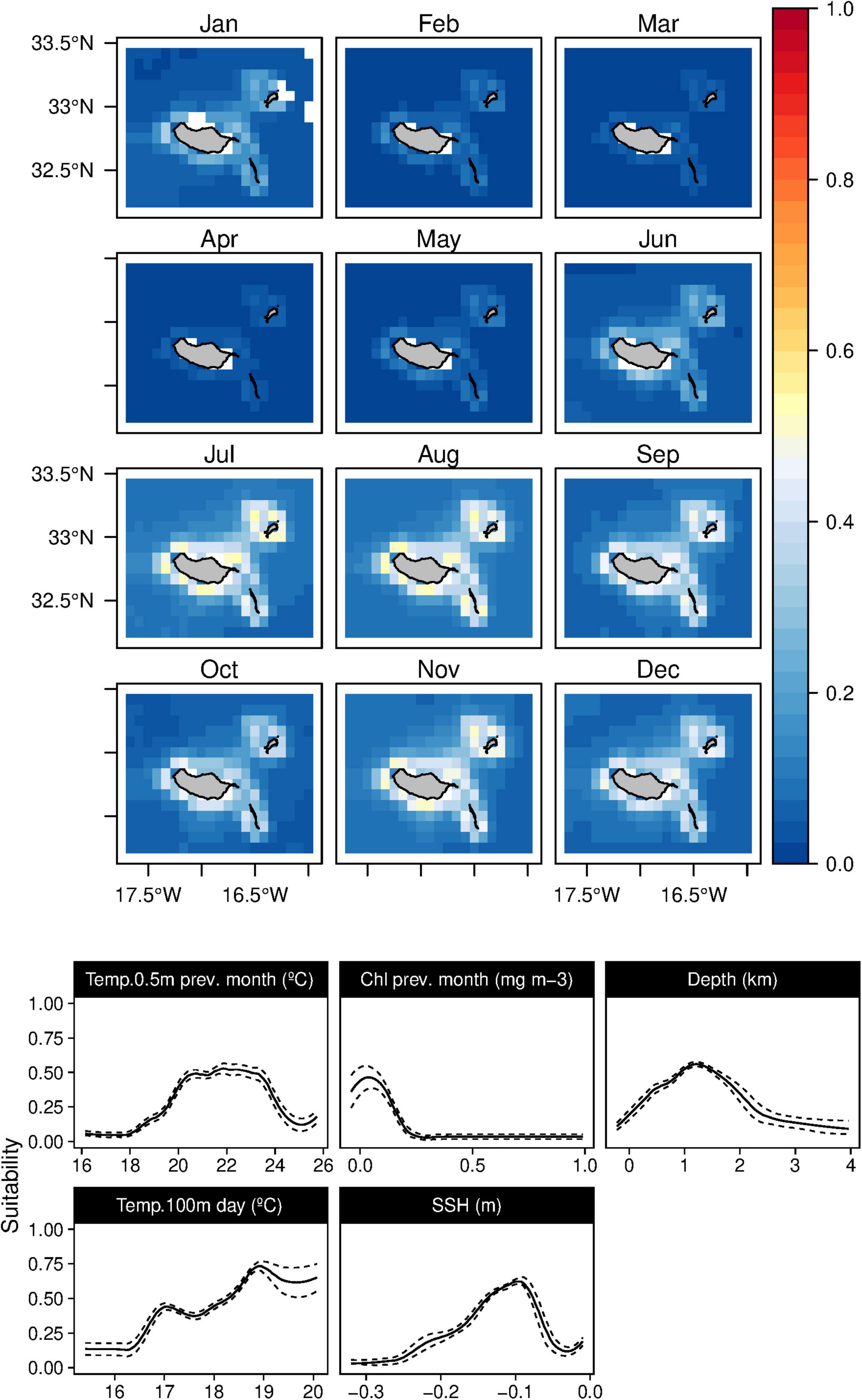
Figure 11. Mean monthly suitability maps (above) and smoothed response curves with standard deviation (below) in the Madeira archipelago for Balaenoptera edeni for the “daily” models. In the maps, red represents more suitable and blue less suitable. Responses curves are ordered by percent contribution of the environmental variables.
Discussion
The long-term opportunistic dataset used in this study provided an excellent opportunity to build reliable ecological niche models with various environmental conditions. Even if the AUC values alone might not be the best model performance indicator (Lobo et al., 2008), all selected models ranged between 0.7 and 0.9, which, together with the low omission rate values, indicates good overall predictive performance. This reinforces the potential of opportunistically collected datasets to produce reliable estimates of habitat suitability, as Henckel et al. (2020) found recently.
While a series of techniques to reduce overfitting and select the best models were applied (e.g., AICc index for model selection), few coincidental relationships between suitability predictions and environmental variables might still be present. Some of the relations found in the response curves (such as the high suitability predictions on low slope values found for the common dolphins; Figure 8) might look odd and spurious, affecting the explanatory power. Explanatory power and predictive accuracy are different qualities; a model will possess some level of each (Shmueli, 2010). Therefore, even if few coincidental relationships might be present, the validation techniques implemented assure good predictive performance for the models built.
Despite the small area where data was collected (limited mainly to the South coast of Madeira and West of Desertas islands), experts confirmed that extrapolated areas agree with their experience. However, using a reduced area to collect training data might result in a truncated response curve for some variables, missing fundamental environmental values to fully describe the niche (Thuiller et al., 2004; Williams and Jackson, 2007). This could be the case, especially for those more bottom-related species (such as the sperm whale, the short-finned pilot whale, or the bottlenose dolphin). The Maxent clamping implementation mitigates this issue (Phillips et al., 2006; Anderson and Raza, 2010); however, preferably, a wider sampling area with different topographic characteristics would be the best way to overcome this concern.
The daily models added some relevant information on species niches, as it considers a broader range of oceanographic variables in the three dimensions. However, its informative potential was primarily reduced due to the dataset’s coarse spatial resolution and the use of a broad-scale circulation oceanographic algorithm. Using models with a finer spatial resolution and including atmosphere-ocean interactions could improve the results, giving more insights on fine-scale features that can influence cetacean distributions. This fact is especially relevant when considering the fine-scale structures generated by the island in the archipelago (Caldeira et al., 2002).
Species Ecological Findings
Deep-Divers
The three deep-diving species (G. macrorhynchus, P. macrocephalus, and M. densirostris) showed differentiated niches, both in the spatial and temporal dimensions. The short-finned pilot whales’ ecological niche was clearly described by a preference for warmer waters (over 18°C) and low/moderate chlorophyll values. The species was found to be related to waters slightly deeper than 1,000 m, which agrees with the findings on the diving behavior for the species by Soto et al. (2008) in the Canary Islands and Alves et al. (2013a) in Madeira, with dives between 500 and 1,000 m. While major canyons played a role in the distribution of pilot whales, higher suitability values were found mostly related to moderate slopes. This agrees with Thorne et al. (2017) findings, where canyons and the shelf-break zones were found to be suitable habitat for tracked animals. The temporal occurrence pattern for the species in the archipelago seems to be shaped by the SST and Chl-a, with resultant higher suitability values in late-summer/autumn and winter (Figure 2).
These findings are in accordance with the known species’ temporal occurrence and might be related to some of the inter-archipelagic movements registered by Alves et al. (2019). Some animals might travel to other areas (such as the Azores or the Canary Islands) using oceanographic features, like the long-lived eddies in the Macaronesian region described by Sangrà et al. (2009) and Caldeira (2019), a behavior already observed for this species by Thorne et al. (2017) when following the Gulf stream meanders off the Atlantic Coast of the United States. Furthermore, Owen et al. (2019) recently found an influence of the lunar moon on the behavior and distribution of pilot whales in Hawaii, with a displacement toward offshore waters, together with deeper and longer dives during the full moon. These findings might be related to the subtle influence of the lunar illumination we found in the daily models (Table 3); results indicated that suitability around the island was lower with higher lunar illumination. Nevertheless, other approaches would be needed to understand this potential effect better.
Sperm whales are known to be a cosmopolitan species (Jefferson et al., 2011). Even if mostly feeding on cephalopods, they can be considered generalist foragers, consuming a wide variety of prey (e.g., Clarke, 1980; Evans and Hindell, 2004). Our results showed a broader niche of the species on the spatial dimension than the short-finned pilot whales, with a preference for waters deeper than 1 km (but not restricted to a specific range) in the proximity of submarine canyons areas. While the species seems to be present in the archipelago throughout the year, there is an apparent increase in suitability from June to November, linked to SST, with a peak around 23°C, similar to other areas, such as the Azores (Fernandez et al., 2018). In Madeiran waters, the group size for the species ranges between 1 and 30 animals, with calves present in 25% of the groups (Alves et al., 2018). Pirotta et al. (2020), found that solitary animals and groups used areas with different characteristics in the Balearic Islands, with groups preferring warmer waters. Therefore, the presence of groups with offspring in the archipelago might be related with the increase of suitability observed from June to December.
Finally, the Blainville beaked whale shows the most restricted ecological niche of the deep-diving species, mainly being linked to steep relief areas in the vicinity of major canyons (Table 3). This results in a very spatially constrained niche in some areas close to the island’s shoreline, which agrees with the findings in other regions, such as the Canary Islands (Ritter and Brederlau, 1999) and Hawaii (Schorr et al., 2009). The strong relation found with steep areas is probably related to the prey aggregation on these specific habitats, representing a reliable food resource for the species (Arranz et al., 2011). Finally, the explicit contribution of the SST to the models suggests a preference for warm waters, as suggested by Macleod (2000). All these results lead to relatively good suitability values almost all year round, peaking in summer and early autumn months, which explains the high site fidelity patterns previously found by Dinis et al. (2017) around Madeira.
Delphinids
Distinct habitat preferences characterized the two medium-sized delphinids (T. truncatus and S. bredanensis) distributions analyzed; nevertheless, both niches were linked to the island-induced features, reflected in the preference for mid-high slope values. The bottlenose dolphin niche was almost solely influenced by topographic variables (Table 3) with a clear preference for shallow coastal waters (<1,000 m), which agrees with the findings of Dinis et al. (2016b), with almost no temporal variation. This relation was also found in other oceanic archipelagos, such as the Azores (Fernandez et al., 2018). Previous studies found that this species might be present in the Madeira archipelago all year round, with no significant difference in the monthly encounter rate (Dinis et al., 2016a), reflected in the low variability of the suitability values during the year (Supplementary Figure 4). Interestingly, we found a marginal effect of the surface chlorophyll on their distribution, which could be related to fine-scale effects of primary productivity on the species distribution, as found for the species in shallow seas (Scott et al., 2010).
While the rough-toothed dolphin was also linked to areas with medium or high bottom inclination (usually associated with islands or seamounts), the temporal distribution for this species mainly was limited due to a preference for warm waters (over 21–22°C), such as those on the warm wake present mostly on summer months on the South Coast of Madeira (Caldeira et al., 2002; Alves et al., 2021).
When looking at the small delphinids an interesting pattern was found, with two species (D. delphis and S. frontalis) with differentiated ecological niches. Both species have a similar spatial distribution, with a widespread distribution around the islands, clearly demonstrated by the influence of depth. However, differences arise when looking at their temporal distribution; the common dolphin was closely related to lower SST values and high chlorophyll concentrations, while the Atlantic spotted dolphin preferred warm waters and low chlorophyll values (Figures 7, 8). As a result, higher suitability values were found in winter/spring for the former species and summer/autumn for the later species. Au and Perryman (1985) found that in the Eastern Tropical Pacific, common dolphins were found to be associated with upwelling modified waters, while tropical spotted dolphins (Stenella attenuata) were associated with tropical waters. In the present study, instead of S. attenuata, we have S. frontalis; however, a similar pattern seems to occur, with common dolphins preferring “cold” waters rich in nutrients and Atlantic spotted dolphins more associated with warm, and therefore, stratified areas. This is in agreement with the seasonal regimes found in the waters surrounding Madeira, reflected by the ocean static stability, with a much better mixed upper ocean in winter and a more stratified structure present in summer (Alves et al., 2021).
Our findings agree with the observations made by Freitas et al. (2004) for Madeira and are in harmony with the niche segregation hypothesis made by Silva et al. (2014) for the Azores. Nevertheless, it is true that when environmental conditions are suitable for both species, they might occupy the same areas. Furthermore, if we also add the high standard deviation values found in the suitability for the Atlantic spotted dolphins, it is possible that in some years both species might have high suitability values during some months, especially in spring and early summer. Finally, the striped dolphin was found to be an offshore species primarily occurring in deeper waters with a slight preference for lower temperatures and related to mid-slope areas, agreeing with the findings of Fernandez et al. (2018) for the Azores.
Balaenopterids
The two balaenopterids considered herein proved to have very differentiated niches. The fin whale mainly was related to high chlorophyll concentrations during the previous month and on the same day (Figure 10). The niche was shaped by the low water temperature preference at 100 m (<18°C), which might be related to the deep-diving foraging behavior previously documented in the Azores (Silva et al., 2013). The 8-day analysis also showed an influence of the previous month SST, peaking around 18–20°C, which agrees with findings by Fernandez et al. (2018) from the Azores. Conversely, in the present study, the chlorophyll levels were also relevant (with a preference of values higher than 0.5 mgm–3), which might be a limiting factor for the species in Madeira. As a result, higher suitability values (and high standard deviation) are found mostly during the spring months, compared to the Azores, where the species has been recorded during winter, spring, and summer (Silva et al., 2014). In the Azores, the combination of the complex topography with the highly energetic eddy field creates a confluence zone between the west and the east North Atlantic (Caldeira and Reis, 2017). Additionally, Caldeira (2019) found that the number of long-lived eddies (lifetime greater than 60 days) generated by the interaction of the oceanic flow with the islands is much larger in the Azores (n = 202) than in Madeira (n = 50). Considering that mesoscale eddies can modulate oceanic productivity in many ways (Dufois et al., 2016), together with the existence of a confluence area, might support the extended temporal presence of fin whales in the Azores compared to Madeira.
While not much is known, the results presented here agree with the Bryde’s whales described literature. The species is known to move through tropical and warm-temperate waters, with a specific preferred thermal range (Kato and Perrin, 2018). We found that higher suitability values mainly were related to warm surface waters and low surface chlorophyll concentrations during the previous months (Figure 11). This agrees with the higher number of sightings for this species in summer/autumn in the archipelago (Alves et al., 2018). The relatively low suitability values and the high standard deviation we detected could be caused by the high variability of interannual occurrence rates. In the present study, the species was related to a specific SST (between 20 and 24°C). Nevertheless, in California, no significant effect of the temperature occurrence pattern was found, with observations of animals in waters as cold as 15°C (Kerosky et al., 2012). The same authors hypothesize that interannual climate oscillations and oceanographic indexes (such as the ENSO) could explain some of the variability observed, recognizing that other environmental variables rather than the SST might influence the whales’ movements. Therefore, depending on general circulation patterns, the animals might move to Madeira, to northern latitudes, up to the Azores (Steiner et al., 2008), or could remain in southern latitudes.
Conclusion
The distributional estimates presented here are the first attempt to better understand spatial-temporal patterns of cetaceans in the Madeira archipelago. Except for the fin whale, all the other nine species analyzed in the present study showed a clear relation with specific topographic features, which might be related to the island-mass effect and associated eddies. To better understand how the specific fine-scale island-related oceanographic features might affect cetaceans’ populations, we recommend using coupled atmospheric-ocean models in future studies. The use of fine-scale accurate data (both in the temporal and spatial dimension) is crucial to improve dynamic ocean management actions in cetaceans, as demonstrated recently by Hausner et al. (2021).
Moreover, we acknowledge that some models might greatly benefit from more data, especially from unsampled areas. Ideally, the next steps to obtain better distributional estimates will include data from different sources (such as dedicated surveys, telemetry data, or other platforms of opportunity like fishing boats, ferries, or cargo ships) to cover as much as possible the potential factors influencing the species distribution. However, different datasets might have different biases, and therefore data merging from different sources should be analyzed carefully (Fletcher et al., 2019).
Data Availability Statement
The original contributions generated for this study are included in the article/Supplementary Material, further inquiries can be directed to the corresponding author.
Author Contributions
MF, AD, and FA conceived the study design. FA, AD, RF, PT, and J-CF supported the data collection and organized the databases. MF analyzed the data and wrote the first draft of the manuscript. All authors actively contributed on the writing and editing of the manuscript.
Funding
This study was supported by: (i) INTERTAGUA, MAC2/1.1.a/385 funded by MAC INTERREG 2014-2020, (ii) Oceanic Observatory of Madeira throughout the project M1420-01-0145-FEDER-000001-OOM, and (iii) Fundação para a Ciência e Tecnologia (FCT), Portugal, through the strategic project UID/MAR/04292/2020 granted to MARE UI&I. AD and FA have grants funded by ARDITI—Madeira’s Regional Agency for the Development of Research, Technology and Innovation, throughout the project M1420-09-5369-FSE-000002. RF was partially supported by a FCT doctoral grant (SFRH/BD/147225/2019).
Conflict of Interest
J-CF and PT were employed by Lobosonda - Madeira Whale Watching.
The remaining authors declare that the research was conducted in the absence of any commercial or financial relationships that could be construed as a potential conflict of interest.
Acknowledgments
We wish to thank to all the people and organizations involved in the collection of data over the years. We thank the whale-watching operators Ventura | Nature Emotions, Lobosonda and Seaborn (especially to Miguel Fernandes).
Supplementary Material
The Supplementary Material for this article can be found online at: https://www.frontiersin.org/articles/10.3389/fmars.2021.688248/full#supplementary-material
Footnotes
References
Aiello-Lammens, M. E., Boria, R. A., Radosavljevic, A., Vilela, B., and Anderson, R. P. (2015). spThin: an R package for spatial thinning of species occurrence records for use in ecological niche models. Ecography 38, 541–545. doi: 10.1111/ecog.01132
Albouy, C., Delattre, V., Donati, G., Frölicher, T. L., Albouy-Boyer, S., Rufino, M., et al. (2020). Global vulnerability of marine mammals to global warming. Sci. Rep. 10, 1–12.
Alves, F., Alessandrini, A., Servidio, A., Mendonça, A. S., Hartman, K. L., Prieto, R., et al. (2019). Complex biogeographical patterns support an ecological connectivity network of a large marine predator in the north-east Atlantic. Divers. Distrib. 25, 269–284. doi: 10.1111/ddi.12848
Alves, F., Dinis, A., Ribeiro, C., Nicolau, C., Kaufmann, M., Fortuna, C. M., et al. (2013b). Daytime dive characteristics from six short-finned pilot whales Globicephala macrorhynchus off Madeira Island. Arquipélago Life Mar. Sci. 31, 1–8.
Alves, F., Quérouil, S., Dinis, A., Nicolau, C., Ribeiro, C., Freitas, L., et al. (2013a). Population structure of short-finned pilot whales in the oceanic archipelago of Madeira based on photo-identification and genetic analyses: implications for conservation. Aquat. Conserv. 23, 758–776. doi: 10.1002/aqc.2332
Alves, F., Ferreira, R., Fernandes, M., Halicka, Z., Dias, L., and Dinis, A. (2018). Analysis of occurrence patterns and biological factors of cetaceans based on long-term and fine-scale data from platforms of opportunity: Madeira Island as a case study. Mar. Ecol. 39:e12499. doi: 10.1111/maec.12499
Alves, J., Tomé, R., Caldeira, R., and Miranda, P. (2021). Asymmetric ocean response to atmospheric forcing in an island wake: a 35-year high-resolution Study. Front. Mar. Sci. 8:624392. doi: 10.3389/fmars.2021.624392
Anderson, R. P., Lew, D., and Peterson, A. T. (2003). Evaluating predictive models of species distributions: criteria for selecting optimal models. Ecol. Model. 162, 211–232. doi: 10.1016/s0304-3800(02)00349-6
Anderson, R. P., and Raza, A. (2010). The effect of the extent of the study region on GIS models of species geographic distributions and estimates of niche evolution: preliminary tests with montane rodents (genus Nephelomys) in Venezuela. J. Biogeogr. 37, 1378–1393. doi: 10.1111/j.1365-2699.2010.02290.x
Araújo, M. B., Anderson, R. P., Barbosa, A. M., Beale, C. M., Dormann, C. F., Early, R., et al. (2019). Standards for distribution models in biodiversity assessments. Sci. Adv. 5:eaat4858. doi: 10.1126/sciadv.aat4858
Aristegui, J., Sangra, P., Hernandez-Leon, S., Canton, M., Hernandez- Guerra, A., and Kerling, J. L. (1994). Island-induced eddies in the Canary Islands. Deep Sea Res. 41, 1509–1525. doi: 10.1016/0967-0637(94)90058-2
Arranz, P., De Soto, N. A., Madsen, P. T., Brito, A., Bordes, F., and Johnson, M. P. (2011). Following a foraging fish-finder: diel habitat use of Blainville’s beaked whales revealed by echolocation. PLoS One 6:e28353. doi: 10.1371/journal.pone.0028353
Au, D. W., and Perryman, W. L. (1985). Dolphin habitats in the eastern tropical Pacific. Fish. Bull. 83, 623–644.
Azzellino, A., Gaspari, S., Airoldi, S., and Nani, B. (2008). Habitat use and preferences of cetaceans along the continental slope and the adjacent pelagic waters in the western Ligurian Sea. Deep Sea Res. I 55, 296–323. doi: 10.1016/j.dsr.2007.11.006
Barton, E. D., Basterretxea, G., Flament, P., Mitchelson-Jacob, E. G., Jone, B., Aristegui, J., et al. (2000). Lee region of gran canaria. J. Geophys. Res. 105, 17173–17193. doi: 10.1029/2000jc900010
Boehner, J., and Selige, T. (2006). “Spatial prediction of soil attributes using terrain analysis and climate regionalisation,” in SAGA - Analysis and Modelling Applications, eds J. Boehner, K. R. McCloy, and J. Strobl (Goettingen: Göttinger Geographische Abhandlungen), 13–28.
Breen, P., Pirotta, E., Allcock, L., Bennison, A., Boisseau, O., Bouch, P., et al. (2020). Insights into the habitat of deep diving odontocetes around a canyon system in the northeast Atlantic ocean from a short multidisciplinary survey. Deep Sea Res. Part I 159:103236. doi: 10.1016/j.dsr.2020.103236
Caldeira, R. (2019). “Island wakes,” in Encyclopedia of Ocean Sciences, 3rd Edn, Vol. 3, eds J. K. Cochran, J. H. Bokuniewicz, and L. P. Yager (Amsterdam: Elsevier), 83–91. doi: 10.1016/b978-0-12-409548-9.11614-8
Caldeira, R., Groom, S., Miller, P., Pilgrim, D., and Nezlin, N. P. (2002). Sea-surface signatures of the island mass effect phenomena around Madeira Island, Northeast Atlantic. Remote Sens. Environ. 80, 336–360. doi: 10.1016/s0034-4257(01)00316-9
Caldeira, R., and Reis, J. C. (2017). The Azores confluence zone. Front. Mar. Sci. 4:37. doi: 10.3389/fmars.2017.00037
Catlin-Groves, C. L. (2012). The citizen science landscape: from volunteers to citizen sensors and beyond. Int. J. Zool. 2012, 1687-8477. doi: 10.1155/2012/349630
Clarke, M. R. (1980). Cephalopods in the diet of sperm whales of the Southern Hemisphere and their bearing on sperm whale biology. Discov. Rep. 37, 1–324.
Cobos, M. E., Peterson, A. T., Barve, N., and Osorio-Olvera, L. (2019a). kuenm: an R package for detailed development of ecological niche models using Maxent. PeerJ 7:e6281. doi: 10.7717/peerj.6281
Cobos, M. E., Peterson, A. T., Osorio-Olvera, L., and Jiménez-García, D. (2019b). An exhaustive analysis of heuristic methods for variable selection in ecological niche modeling and species distribution modeling. Ecol. Informatics 53:100983. doi: 10.1016/j.ecoinf.2019.100983
Dinis, A., Alves, F., Nicolau, C., Ribeiro, C., Kaufmann, M., Cañadas, A., et al. (2016a). Bottlenose dolphin Tursiops truncatus group dynamics, site fidelity, residency and movement patterns in the Madeira Archipelago (North-East Atlantic). Afr. J. Mar. Sci. 38, 151–160. doi: 10.2989/1814232x.2016.1167780
Dinis, A., Carvalho, A., Alves, F., Nicolau, C., Ribeiro, C., Kaufmann, M., et al. (2016b). Spatial and temporal distribution of bottlenose dolphins, Tursiops truncatus, in the Madeira archipelago, NE Atlantic. Arquipélago. Life Mar. Sci. 33, 45–54.
Dinis, A., Marques, R., Dias, L., Sousa, D., Gomes, C., Abreu, N., et al. (2017). Site fidelity of Blainville’s beaked whale (Mesoplodon densirostris) off Madeira Island (Northeast Atlantic). Aquat. Mamm. 43:387. doi: 10.1578/am.43.4.2017.387
Dinis, A., Molina, C., Tobeña, M., Sambolino, A., Hartman, K., Fernandez, M., et al. (2021). Large-scale movements of common bottlenose dolphins in the Atlantic: dolphins with an international courtyard. PeerJ 9:e11069. doi: 10.7717/peerj.11069
Dufois, F., Hardman-Mountford, N. J., Greenwood, J., Richardson, A. J., Feng, M., and Matear, R. J. (2016). Anticyclonic eddies are more productive than cyclonic eddies in subtropical gyres because of winter mixing. Sci. Adv. 2:e1600282. doi: 10.1126/sciadv.1600282
Embling, C. B., Walters, A. E. M., and Dolman, S. J. (2015). How much effort is enough? The power of citizen science to monitor trends in coastal cetacean species. Glob. Ecol. Conserv. 3, 867–877. doi: 10.1016/j.gecco.2015.04.003
Evans, K., and Hindell, M. A. (2004). The diet of sperm whales (Physeter macrocephalus) in southern Australian waters. ICES J. Mar. Sci. 61, 1313–1329. doi: 10.1016/j.icesjms.2004.07.026
Fernandez, M., Yesson, C., Gannier, A., Miller, P. I., and Azevedo, J. M. N. (2017). The importance of temporal resolution for niche modelling in dynamic marine environments. J. Biogeogr. 44, 2816–2827. doi: 10.1111/jbi.13080
Fernandez, M., Yesson, C., Gannier, A., Miller, P. I., and Azevedo, J. M. N. (2018). A matter of timing: how temporal scale selection influences cetacean ecological niche modelling. Mar. Ecol. Prog. Ser. 595, 217–231. doi: 10.3354/meps12551
Fletcher, R. J. Jr., Hefley, T. J., Robertson, E. P., Zuckerberg, B., McCleery, R. A., and Dorazio, R. M. (2019). A practical guide for combining data to model species distributions. Ecology 100:e02710. doi: 10.1002/ecy.2710
Freitas, L., Dinis, A., Alves, F., and Nobrega, F. (2004). Relatório dos Resultados Científicos do Projecto Para a Conservação dos Cetáceos no Arquipélago da Madeira. Report to the European Commission. Madeira: Museu da Baleia da Madeira.
Freitas, L., Dinis, A., Nicolau, C., Ribeiro, C., and Alves, F. (2012). New records of cetacean species for Madeira Archipelago with an updated checklist. Bol. Mus. Mun. Funchal. 62, 25–43.
Guisan, A., Weiss, S. B., and Weiss, A. D. (1999). GLM versus CCA spatial modeling of plant species distribution. Plant Ecol. 143, 107–122.
Hausner, A., Samhouri, J. F., Hazen, E. L., Delgerjargal, D., and Abrahms, B. (2021). Dynamic strategies offer potential to reduce lethal ship collisions with large whales under changing climate conditions. Mar. Policy 130:104565. doi: 10.1016/j.marpol.2021.104565
Henckel, L., Bradter, U., Jönsson, M., Isaac, N. J., and Snäll, T. (2020). Assessing the usefulness of citizen science data for habitat suitability modelling: opportunistic reporting versus sampling based on a systematic protocol. Divers. Distrib. 26, 1276–1290. doi: 10.1111/ddi.13128
Jefferson, T. A., Webber, M. A., and Pitman, R. L. (2011). Marine Mammals of the World: A Comprehensive Guide to Their Identification. Amsterdam: Elsevier.
Kato, H., and Perrin, W. F. (2018). “Bryde’s whales Balaenoptera edeni,” in Encyclopedia of Marine Mammals, 3rd Edn, eds B. Würsig, J. G. M. Thewissen, and K. Kovacs (London: Academic Press), 143–145.
Kerosky, S. M., Širović, A., Roche, L. K., Baumann-Pickering, S., Wiggins, S. M., and Hildebrand, J. A. (2012). Bryde’s whale seasonal range expansion and increasing presence in the Southern California Bight from 2000 to 2010. Deep Sea Res. Pt. I 65, 125–132. doi: 10.1016/j.dsr.2012.03.013
Lobo, J. M., Jiménez-Valverde, A., and Real, R. (2008). AUC: a misleading measure of the performance of predictive distribution models. Glob. Ecol. Biogeogr. 17, 145–151. doi: 10.1111/j.1466-8238.2007.00358.x
Macleod, C. D. (2000). Review of the distribution of Mesoplodon species (order Cetacea, family Ziphiidae) in the North Atlantic. Mamm. Rev. 30, 1–8. doi: 10.1046/j.1365-2907.2000.00057.x
Mann, K., and Lazier, J. (1991). Dynamics of Marine Ecosystems: Biological–Physical Interactions in the Oceans. London: Blackwell.
Merow, C., Smith, M. J., and Silander, J. A. Jr. (2013). A practical guide to MaxEnt for modeling species’ distributions: what it does, and why inputs and settings matter. Ecography 36, 1058–1069. doi: 10.1111/j.1600-0587.2013.07872.x
Moore, S. E., Waite, J. M., Mazzuca, L. L., and Hobbs, R. C. (2000). Mysticete whale abundance and observations of prey associations on the central Bering Shelf. J. Cet. Res. Manage 2, 227–234.
Moors-Murphy, H. B. (2014). Submarine canyons as important habitat for cetaceans, with special reference to the Gully: a review. Deep Sea Res. II 104, 6–19. doi: 10.1016/j.dsr2.2013.12.016
Norris, K., Terry, A., Hansford, J. P., and Turvey, S. T. (2020). Biodiversity conservation and the earth system: mind the gap. Trends Ecol. Evol. 35, 919–926. doi: 10.1016/j.tree.2020.06.010
Owen, K., Andrews, R. D., Baird, R. W., Schorr, G. S., and Webster, D. L. (2019). Lunar cycles influence the diving behavior and habitat use of short-finned pilot whales around the main Hawaiian Islands. Mar. Ecol. Prog. Ser. 629, 193–206. doi: 10.3354/meps13123
Peterson, A. T., Papeş, M., and Soberón, J. (2008). Rethinking receiver operating characteristic analysis applications in ecological niche modeling. Ecol. Model. 213, 63–72. doi: 10.1016/j.ecolmodel.2007.11.008
Phillips, S. J., Anderson, R. P., and Schapire, R. E. (2006). Maximum entropy modeling of species geographic distributions. Ecol. Model. 190, 231–259. doi: 10.1016/j.ecolmodel.2005.03.026
Pirotta, E., Brotons, J. M., Cerdà, M., Bakkers, S., and Rendell, L. E. (2020). Multi-scale analysis reveals changing distribution patterns and the influence of social structure on the habitat use of an endangered marine predator, the sperm whale Physeter macrocephalus in the Western Mediterranean Sea. Deep Sea Res. Pt. I 155:103169. doi: 10.1016/j.dsr.2019.103169
Radosavljevic, A., and Anderson, R. P. (2014). Making better Maxent models of species distributions: complexity, overfitting and evaluation. J. Biogeogr. 41, 629–643. doi: 10.1111/jbi.12227
Redfern, J. V., Ferguson, M. C., Becker, E. A., Hyrenbach, K. D., Good, C., Barlow, J., et al. (2006). Techniques for cetacean–habitat modeling. Mar. Ecol. Prog. Ser. 310, 271–295. doi: 10.3354/meps310271
Ritter, F., and Brederlau, B. (1999). Behavioural observations of dense beaked whales (Mesoplodon densirostris) off La Gomera, Canary Islands (1995-1997). Aquat. Mamm. 25, 55–62.
Robbins, J. R., Babey, L., and Embling, C. B. (2019). Citizen science in the marine environment: a case-study estimating common dolphin densities in the north-east Atlantic. PeerJ 8:e8335. doi: 10.7717/peerj.8335
Rodríguez, J. P., Brotons, L., Bustamante, J., and Seoane, J. (2007). The application of predictive modelling of species distribution to biodiversity conservation. Divers. Distrib. 13, 243–251. doi: 10.1111/j.1472-4642.2007.00356.x
Roman, J., Estes, J. A., Morissette, L., Smith, C., Costa, D., McCarthy, J., et al. (2014). Whales as marine ecosystem engineers. Front. Ecol. Environ. 12, 377–385. doi: 10.1890/130220
Sangrà, P., Pascual, A., Rodríguez-Santana, Á., Machín, F., Mason, E., McWilliams, J. C., et al. (2009). The Canary Eddy Corridor: A major pathway for long-lived eddies in the subtropical North Atlantic. Deep Sea Res. Part I: Oceanogr. Res. Pap. 56, 2100–2114. doi: 10.1016/j.dsr.2009.08.008
Schorr, G. S., Baird, R. W., Hanson, M. B., Webster, D. L., McSweeney, D. J., and Andrews, R. D. (2009). Movements of satellite-tagged Blainville’s beaked whales off the island of Hawaii. Endanger. Species Res. 10, 203–213. doi: 10.3354/esr00229
Scott, B. E., Sharples, J., Ross, O. N., Wang, J., Pierce, G. J., and Camphuysen, C. J. (2010). Sub-surface hotspots in shallow seas: fine-scale limited locations of top predator foraging habitat indicated by tidal mixing and sub-surface chlorophyll. Mar. Ecol. Prog. Ser. 408, 207–226. doi: 10.3354/meps08552
Silva, M. A., Prieto, R., Cascão, I., Seabra, M. I., Machete, M., Baumgartner, M. F., et al. (2014). Spatial and temporal distribution of cetaceans in the mid-Atlantic waters around the Azores. Mar. Biol. Res. 10, 123–137. doi: 10.1080/17451000.2013.793814
Silva, M. A., Prieto, R., Jonsen, I., Baumgartner, M. F., and Santos, R. S. (2013). North Atlantic blue and fin whales suspend their spring migration to forage in middle latitudes: building up energy reserves for the journey? PLoS One 8:e76507. doi: 10.1371/journal.pone.0076507
Soto, N. A., Johnson, M. P., Madsen, P. T., Díaz, F., Domínguez, I., Brito, A., et al. (2008). Cheetahs of the deep sea: deep foraging sprints in short-finned pilot whales off Tenerife (Canary Islands). J. Anim. Ecol. 77, 936–947. doi: 10.1111/j.1365-2656.2008.01393.x
Steiner, L., Silva, M. A., Zereba, J., and Leal, M. J. (2008). Bryde’s whales, Balaenoptera edeni, observed in the Azores: a new species record for the region. Mar. Biodivers. Rec. 1:e66.
Thorne, L. H., Foley, H. J., Baird, R. W., Webster, D. L., Swaim, Z. T., and Read, A. J. (2017). Movement and foraging behavior of short-finned pilot whales in the Mid-Atlantic Bight: importance of bathymetric features and implications for management. Mar. Ecol. Prog. Ser. 584, 245–257. doi: 10.3354/meps12371
Thuiller, W., Brotons, L., Araújo, M. B., and Lavorel, S. (2004). Effects of restricting environmental range of data to project current and future species distributions. Ecography 27, 165–172. doi: 10.1111/j.0906-7590.2004.03673.x
Tyne, J. A., Loneragan, N. R., Johnston, D. W., Pollock, K. H., Williams, R., and Bejder, L. (2016). Evaluating monitoring methods for cetaceans. Biol. Conserv. 201, 252–260. doi: 10.1016/j.biocon.2016.07.024
Warren, D. L., and Seifert, S. N. (2011). Ecological niche modeling in Maxent: the importance of model complexity and the performance of model selection criteria. Ecol. Appl. 21, 335–342. doi: 10.1890/10-1171.1
Weiss, A. D. (2001). “Topographic positions and landforms analysis (Poster),” in Proceedings of the ESRI International User Conference, San Diego, CA.
Williams, J. W., and Jackson, S. T. (2007). Novel climates, no-analog communities, and ecological surprises. Front. Ecol. Environ., 475–482. doi: 10.1890/070037
Keywords: whales, dolphins, pelagic, ecological niche modeling, opportunistic data, whale watching
Citation: Fernandez M, Alves F, Ferreira R, Fischer J-C, Thake P, Nunes N, Caldeira R and Dinis A (2021) Modeling Fine-Scale Cetaceans’ Distributions in Oceanic Islands: Madeira Archipelago as a Case Study. Front. Mar. Sci. 8:688248. doi: 10.3389/fmars.2021.688248
Received: 30 March 2021; Accepted: 15 June 2021;
Published: 08 July 2021.
Edited by:
Matthew Lewis, Bangor University, United KingdomReviewed by:
Victoria Paige Van de Vuurst, Virginia Tech, United StatesJames Waggitt, Bangor University, United Kingdom
Copyright © 2021 Fernandez, Alves, Ferreira, Fischer, Thake, Nunes, Caldeira and Dinis. This is an open-access article distributed under the terms of the Creative Commons Attribution License (CC BY). The use, distribution or reproduction in other forums is permitted, provided the original author(s) and the copyright owner(s) are credited and that the original publication in this journal is cited, in accordance with accepted academic practice. No use, distribution or reproduction is permitted which does not comply with these terms.
*Correspondence: Marc Fernandez, bWFyYy5mZXJuQGdtYWlsLmNvbQ==