- 1Laboratório de Biologia Recifal, Instituto Oceanográfico, Universidade de São Paulo, São Paulo, Brazil
- 2Instituto de Estudos Avançados, Universidade de São Paulo, São Paulo, Brazil
Many species drive the diversity of ecosystems by adding structural complexity to the environment. In coral reefs, stony corals act as habitat-forming species, increasing niche availability for other organisms. Some coral species play key roles as reef builders due to their abundance or morpho-functional characteristics. Thus, changes in the distributions of these species can entail cascading effects in entire ecosystems. With climate change, many coral species are experiencing shifts in their distributions, threatening the preservation of coral reefs. Here, we projected the current and future distributions of three key reef builders of the Atlantic (Mussismilia hispida, Montastraea cavernosa, and the Siderastrea complex) under three relative concentration pathway scenarios: the most optimistic, the most pessimistic and one moderate scenario (RCP2.6, 4.5, and 8.5). Our models revealed that all the above species will undergo habitat loss in the future (2100) in the most pessimistic scenario, although new areas could become suitable, including regions in the eastern Atlantic Ocean. Additionally, when considering only its actual range of occurrence, M. hispida will lose habitats under all future scenarios. Moreover, in some regions of both the Tropical Northwestern Atlantic (TNA) and the Brazilian coast, these three species could disappear, with detrimental consequences for the associated communities. We highlight the need for an urgent change of course to guarantee functional reefs in the Atlantic in the future.
Introduction
In terrestrial and aquatic environments, the diversity and functioning of communities may be driven by several species, from trees to fiddler crabs, that provide the structural complexity of their environments (Tews et al., 2004; Warfe and Barmuta, 2006; Kristensen, 2008; Alvarez-Filip et al., 2011b; Graham and Nash, 2013). By creating three-dimensional space, these species provide shelter, increase niche availability, and directly or indirectly provide resources for other species (Jones et al., 1994, 1997). As species that provide structural complexity are lost, the ecosystem integrity is eroded with cascading effects in the community (Cattano et al., 2020; Richardson et al., 2020). In addition to reductions in the abundances of habitat-forming species, changes in the composition of these components can also boost the loss of diversity in ecosystems (Teagle and Smale, 2018). In coral reefs, stony corals play a key role in increasing structural complexity (Alvarez-Filip et al., 2011b; Darling et al., 2013, 2017). These organisms are considered bioengineers, contributing to the structural formation of reefs through the long-term deposition of carbonate (Perry et al., 2008).
Despite their importance, coral reefs are among the most threatened ecosystems in the world (Hoegh-Guldberg et al., 2007; Hughes et al., 2017). Indeed, in recent centuries, the Anthropocene has imposed challenges never before experienced by corals (Hughes et al., 2017; Williams and Graham, 2019). Coral reefs are directly impacted by pollution, fisheries (Zaneveld et al., 2016), tourism, urbanization (Sánchez-Quinto et al., 2020), ocean acidification (Hoegh-Guldberg et al., 2007; Anthony et al., 2008), and climate warming (Hughes et al., 2018; Kerry et al., 2018). Worldwide, climate change is impacting coral reef integrity, with coral bleaching events becoming more intense, frequent and long-lasting (Hughes et al., 2018; Skirving et al., 2019), leading to the loss of coral cover (Anthony et al., 2008; Eakin et al., 2010; Courtney et al., 2020; Duarte et al., 2020). As structural species are lost, many coral reefs experience phase shifts to alternative states [e.g., from the coral- to macroalgae-dominated state; see Norström et al. (2009)], a problem that has already been assessed in several reefs around the world (Hughes, 1994; Morais et al., 2020; Richardson et al., 2020). Declines in coral cover may compromise coral reef persistence in the future, also impacting the provision of essential ecosystem services (Hughes et al., 2017; Woodhead et al., 2019).
In tandem with coral cover reductions, climate change is already causing distributional changes to several coral species (Hoegh-Guldberg, 2011; Baird et al., 2012), with a general pattern of poleward increases in distribution ranges and losses of suitable habitats in the tropics (Hoegh-Guldberg, 2011; Yamano et al., 2011; Takao et al., 2015). This may lead to the tropicalization of temperate reefs with unprecedented impacts on entire communities (Vergés et al., 2019). Besides, as shown in recent projections, some species may also expand its ranges within the tropics, changing the local species composition, and richness (Rodriguez et al., 2019). Although several species may contribute to the structural complexity of a reef, some species play a key role either by their high abundances or morpho-functional traits (Alvarez-Filip et al., 2011a). Changes in the distributions of these key organisms may elicit negative effects on their community, even if other species with similar functions are able to colonize the vacant space (Teagle and Smale, 2018). Thus, understanding the possible changes in the distributions of key reef builders is essential for planning management actions, with consequences for entire ecosystems.
Projecting future changes in the composition of species in reef ecosystems, albeit essential, is also challenging. Species distribution modeling (SDM) has become an important tool for ecologists [see Elith and Leathwick (2009) for a complete review], enabling predictions of current and future habitat suitability for a certain species (Cacciapaglia and van Woesik, 2018; Durante et al., 2018; Morato et al., 2020). These models incorporate the current empirical distribution of a given species (gathered from geolocated presence and absence data from surveys and global databases) and environmental data to establish habitats with the necessary conditions for that species to survive (Pearson and Dawson, 2003; Pearson et al., 2006; Wiens et al., 2009). Despite the limitations of SDMs [see Pearson and Dawson (2003)], such as their failure to consider competition or dispersion, SDMs can help to overcome data deficiencies and provide fast and reliable tools to predict the impacts of climate change on marine organisms (e.g., Morato et al., 2020).
In the Atlantic Ocean, coral reefs are predominantly distributed along the Caribbean region and Brazilian coast, with Caribbean reefs being characterized by higher diversity (Porter, 1972). The Amazon and Orinoco rivers constitute the most important biogeographic barrier between those two regions. Stony corals are present on the West African coast (Laborel, 1974; Polidoro et al., 2017), but no coral reefs are found in this region due to a set of oceanographic features, such as low temperatures and major river discharges. Even though coral reefs along the Brazilian coast are less prevalent than those in the Caribbean region, they are characterized by a high level of endemism (Leão et al., 2003). Of the 15 zooxanthellate Scleractinia occurring in Brazilian shallow waters, four are endemic (Castro and Pires, 2001; Nunes et al., 2008). Brazilian coral species are also unique due to their adaptation to the turbid waters that prevail throughout the shelf.
Despite their ecological relevance, reefs in the Atlantic are in critical situations (Eakin et al., 2010; Duarte et al., 2020). Key species of corals are experiencing reductions in their areas of occurrence (e.g., Miller et al., 2002), leading to declines in the structural complexity of Atlantic reefs (Alvarez-Filip et al., 2009). Additionally, although South Atlantic reefs may be more resilient to the impacts of climate warming (Mies et al., 2020), increases in the number of bleaching events due to heat waves on Brazilian reefs also raise concerns, as bleaching events may lead to higher coral mortality (Duarte et al., 2020). Indeed, heat stress episodes threaten coral reefs over all Western Atlantic (e.g., Eakin et al., 2010; Duarte et al., 2020). Thus, understanding how climate change will affect foundation coral species is essential for enabling conservation actions on Atlantic reefs. Here, we modeled the current habitat suitability of three important reef builders of the southwestern Atlantic: Mussismilia hispida, Montastraea cavernosa, and the Siderastrea complex (considering two species: Siderastrea stellata and Siderastrea radians). We chose these species based on their high contribution to the structure of Southwestern Atlantic reefs, although some may also be important for the overall structure of reefs in other parts of the Atlantic (e.g., M. cavernosa). In addition to the current habitat suitability, we also projected potential changes in species habitat suitability considering three different climate scenarios (relative concentration pathways 2.6, 4.5, and 8.5; IPCC, 2014) estimated for the 2100s. Hence, we were able to detect and map the current extent of suitable habitats for coral species and estimate the future dimensions of these habitats under the effects of the estimated climate change scenarios. The projected changes in habitat suitability for reef builders demand the attention of researchers and managers in future years to preserve coral reef integrity and the provision of essential ecosystem services.
Materials and Methods
Study Area
We modeled the current and future suitable habitats of stony coral species in the western and eastern Atlantic Ocean, covering the areas between the latitudes of −42.5° and 42.5° and restricted to altitudes of 2 m and depths of 200 m. This region is characterized by hotspots of diversity, such as the West Caribbean (Roberts et al., 2002) and Northeast Brazil (Vila-Nova et al., 2014). This region is also impacted by local and regional scale detrimental anthropogenic activities, such as urban development, unregulated marine tourism, and overfishing, all of which impose risks to coastal ecosystems, including coral reefs (Leão et al., 2003, 2016; Leão and Kikuchi, 2005; Halpern et al., 2015; Polidoro et al., 2017; Shantz et al., 2020).
The Amazon and Orinoco River mouths constitute major geographic barriers on the western Atlantic Ocean, contributing to the differences in diversity between the Tropical Northwestern Atlantic (TNA) and South Atlantic (Floeter et al., 2008). This region is influenced by the south equatorial current, which bifurcates into the Brazil Current (which moves southward) and the North Brazil Current (Johns et al., 1998; Silveira et al., 2000). The Western and Eastern Atlantic are separated by the Mid-Atlantic Barrier that contributes to the differences in species composition between those two areas, with the Mid-Atlantic ridge islands (Ascension and Saint Helena) sharing species of both biogeographic regions (Floeter et al., 2008). The oceanographic features of the eastern Atlantic produce a narrow tropical region that is mainly influenced by three oceanic currents: the Benguela Current, which flows northward from the South African coast up to approximately 25°S, transporting cold waters (Garzoli et al., 1996); the Guinean Current, which transports warm waters from 3°N up to the Guinean Gulf; and the Canary Current, which transports cold waters from the north (Arístegui et al., 2004).
Selection of Species and Occurrence Data
We mapped the suitable habitats of three major reef builder species of the southwest Atlantic: M. hispida, M. cavernosa, and the Siderastrea complex. M. hispida is a massive coral endemic to Brazil, with a broad distribution that ranges from Maranhão State (∼2°S) to São Paulo State (∼25°S) (Castro and Pires, 2001; Leão et al., 2016). M. hispida can be one of the most abundant species in Brazilian reefs, contributing to the overall reef structure (Oigman-Pszczol and Creed, 2004). Montastraea cavernosa presents a wider distribution being very common in the Caribbean (Horta-Puga, 2003) and is also abundant on the Brazilian coast in reefs from northeastern to southeastern Brazil (Laborel, 1970). In the eastern Atlantic, it occurs off the African coast in São Tomé Island and is the dominant coral in some areas (Laborel, 1974; Maia et al., 2018). Montastraea cavernosa can occur in both shallow and mesophotic areas (Goreau and Wells, 1967), where it can thrive and become one of the main stony coral components (Goodbody-Gringley et al., 2015).
Three species of Siderastrea occur in the Atlantic (Siderastrea siderea, Siderastrea stellata, and Siderastrea radians) and constitute the “Atlantic Siderastrea complex” (Menezes et al., 2014). Siderastrea stellata was believed to be endemic to Brazil but was recently found in the Gulf of Mexico (Leão and Kikuchi, 2005; García et al., 2017). On the Brazilian coast, S. radians occurs simultaneously with S. stellata, and are hard to distinguish in the field due to their similar morphology (Menezes et al., 2014). Thus, considering the coinciding environmental requirements shared by these two species, we decided to model S. stellata and S. radians as a single entity (hereafter, Siderastrea sp.), as S. stellata occurrence points may be in fact S. radians. Although Siderastrea sp. may be considered a weedy species (following classification of Darling et al., 2012), in the Southwestern Atlantic it has a great importance in providing structural complexity to reefs. This species may be abundant in shallow waters and intertidal areas (Leão and Kikuchi, 2005). It may be dominant in several areas along the northeastern Brazilian shelf (Castro and Pires, 2001), also being one of the most common species in the emerged portions of reefs (Soares et al., 2011). Remarkably, Siderastrea sp. can also cover large portions of rocky reefs (Oigman-Pszczol and Creed, 2004).
The occurrence data of the studied species were retrieved from the GBIF (Global Biodiversity Facility) and OBIS (Ocean Biodiversity Information System) databases in the period from March 2019 to December 2020 using the packages “rgbif” and “robis.” We also obtained occurrence and absence data from published works included in the SCOPUS and Web of Science databases, searching for articles containing the keywords “Mussismilia hispida,” “Montastraea cavernosa,” and “Siderastrea + Atlantic.” Works that presented georeferenced occurrence data were thus selected (Supplementary Table 1). Extensive data cleaning was performed to ensure data quality. We removed points from unknown sources and from citizen science sources, as some species selected here are difficult to identify in the field. We also removed points falling on land using the “obistools” package. To avoid the negative effect of sampling bias, a single point per cell (in the same resolution as that of the environmental layers) was maintained (Elith and Leathwick, 2009). Additionally, for M. cavernosa and Siderastrea sp., a thinning procedure was adopted to avoid bias produced by densely sampled areas using OccurrenceThinner v1.04 (Verbruggen et al., 2013), with thresholds of 0.5 and 1. This was not necessary for M. hispida, as the geographic distribution of the occurrence points were not indicating sampling bias. A total of 95, 310 and 138 presence points were used for M. hispida, M. cavernosa, and Siderastrea sp., respectively.
The SDM algorithms selected for this work require both presence and absence data. When absence data is lacking (or there is not enough absence data), pseudoabsences are generated (Lobo et al., 2010). Several works have discussed the best alternative methods to generate pseudoabsences (Barbet-Massin et al., 2012; Golicher et al., 2012; Senay et al., 2013; Liu et al., 2019), but there is no consensus. Thus, considering our obtained data and analytical demands, we adopted a three-step approach to generate pseudoabsences based on methods used in recent works with positive results (e.g., Chefaoui et al., 2017; Morato et al., 2020). First, a 40-km buffer (i.e., approximately four times the size of the cell of the environmental layers) was generated around each presence point to avoid generating pseudoabsences in the same or proximal cells. Second, we obtained a presence-only model of the species niches based on Mahalanobis distances, as in Chefaoui et al. (2017). This model is based solely on presence points and environmental variables and can draw the niche of a species effectively (Farber and Kadmon, 2003). Finally, pseudoabsence points in a quantity of 10x the number of presence points were sampled in the area of study (Barbet-Massin et al., 2012), excluding the area selected by the Mahalanobis distance model (but not considering areas with less than 1% probability). In the case of M. hispida, we used 2x the number of presence points (i.e., a low number strategy; Liu et al., 2019), as this number yielded better predictions. Additionally, for M. hispida, points were sampled in a limited region to avoid sampling pseudoabsences in areas where the species could occur but is not present due to the existence of well-known biogeographical barriers. Thus, we limited this region using areas delimited in the Marine Ecoregions of the World classification that are in the same provinces where M. hispida occurs and further to the south (Spalding et al., 2007).
Selection of Environmental Variables
We initially selected a set of 13 candidate environmental variables (each with estimated parameters: mean, maximum or minimum) that are linked to coral species physiology. Calcite concentration, chlorophyll-a concentration, cloud cover (%), diffuse attenuation (KD490), nitrate concentration, photosynthetically active radiation (PAR), pH, phosphate concentration, salinity, silicate concentration, sea surface temperature (SST) and current velocity layers were obtained through Bio-ORACLE 2.0 (Assis et al., 2018) with a 5-arcmin resolution (cell size of approximately 9.2 km). Note that, although we included calcite and pH to consider the effects of ocean acidification, omega (the calcium carbonate saturation state) was not included, as data was unavailable. Bio-ORACLE layers were downloaded using the “sdmpredictors” package (Assis et al., 2018). Wind speed data were downloaded from the COPERNICUS database with a 0.25° resolution and adjusted to the resolution of Bio-ORACLE layers by kriging interpolation using the ‘‘gstat’’ and ‘‘raster’’ packages. The layers were cropped to include areas within an altitude of 2 m and a depth of 100 m, as obtained from the GEBCO bathymetry layer1 (Weatherall et al., 2015), using the “raster” package after correcting the GEBCO resolution (15 arcseconds) to match 5- arcmin.
A high correlation between environmental layers can negatively affect regression models due to the effects of multicollinearity (Dormann et al., 2013). Thus, we analyzed the variation inflation factors (VIFs) of environmental layers prior to its use in the modeling fitting procedure using a threshold of VIF = 10 to remove variables with strong collinearity. This VIF threshold was adopted in recent works, with positive results (Burgos et al., 2020; Morato et al., 2020). VIF analysis was done using the “usdm” package (Naimi et al., 2014). If two variations of the same variable (e.g., mean and maximum) were selected in the VIF procedure, only one variation was retained based on which variation produced the stronger effect on the species biology. After the exclusion process, VIF values of the remaining variables were kept below five (VIF values are shown in Supplementary Table 2). The following layers were selected in the VIF procedure: mean calcite concentration, maximum chlorophyll-a concentration, maximum cloud cover (%), mean nitrate concentration, mean pH, maximum phosphate concentration, mean salinity, mean silicate concentration, maximum SST, mean current velocity, mean wind speed, maximum diffuse attenuation and maximum PAR. We further removed diffuse attenuation and PAR layers, as there was no future projection available for these variables, and the layers showed minor contributions in a first run of the models. We also added a static layer (in short geological terms) of bathymetry, as this can influence the suitability of the niches of benthic species. The remaining 12 environmental layers were then used in the modeling procedure.
The future climate layers were obtained from the Coupled Model Intercomparison Project (CMIP) five for the RCP2.6, RCP4.5, and RCP8.5 scenarios through the WRCP database2. We used data derived from the HADGEM2-ES model (r1i1p1 ensemble) from the Hadley Met Office (United Kingdom), except for phosphate and calcite, which were derived from the IPSL-CM5A-LR model from the Institut Pierre-Simon Laplace (r1i1p1 ensemble). Data for the HADGEM2-ES have an original resolution that varies from ∼0.3° (equator) to 1°, and IPSL-CM5A-LR resolution is approximately 2° (with an increased resolution of 0.5° near equator). Future changes in environmental variables were obtained by applying the change factor approach (Lima-Ribeiro, 2015), the same method adopted by Assis et al. (2018) for creating the BIO-Oracle v2.0 future dataset. For this, the future conditions of each variable were averaged from 2090 to 2100 and present conditions for 2000–2014. Then, we obtained the absolute difference between the future and present conditions for each environmental variable. All these steps were performed in the open source software Climate Data Operators3 (Schulzweida, 2019), which handles NetCDF files (the native for CMIP5 projections) well. Then, the change factor layers were interpolated to the final resolution using kriging interpolation through the “gstat” and “raster” packages. The interpolated change factors were then applied to the original environmental layers from Bio-ORACLE to obtain the final future layers. For the phosphate maximum, chlorophyll-a maximum, salinity mean and nitrate mean, we used the relative difference to avoid producing abnormal values; for the other variables, we used the absolute difference. A future layer for current velocity was obtained from Bio-ORACLE v2.0 (Assis et al., 2018), and the bathymetry layer was kept unaltered.
Modeling Procedure
Several SDM algorithms have been in use recently, with distinct performances (Hao et al., 2019). Here, we applied an ensemble multimodeling approach, generating models composed of individual models obtained through distinct algorithms with different rationales. This approach can reinforce the strength of each model and reduce their singular deficiencies [Marmion et al., 2009; but see Hao et al. (2020)]. We used the “BIOMOD2” package, which provides a framework for ensemble modeling (Thuiller et al., 2009) and has been extensively used for SDMs (e.g., Zanardo et al., 2017; Schickele et al., 2020). Each species was modeled individually using two machine learning algorithms and two regression algorithms: random forests (RFs, machine learning; Breiman, 2001), boosted regression trees (BRTs, machine learning; Elith et al., 2008), generalized linear models (GLMs, regression; McCullagh, 1984) and generalized additive models (GAMs, regression; Hastie and Tibshirani, 2014). All these algorithms showed good performances when modeling niches or species distributions in past similar applications (e.g., Chefaoui et al., 2017; Veazey et al., 2019; Morato et al., 2020) and are available on the “BIOMOD2” framework. Readers are referred to the cited original descriptions for more information about each algorithm.
The models were run with the default configurations, and the prevalence, which is the proportion of occupied locations to the number of absences, was set to 0.5 in all models (hence, presences and pseudo-absences have the same weight in the calibration of the model). Model selection for each algorithm is done by default by BIOMOD2 in each run, varying accordingly to the algorithm (for example, in GLM a stepwise procedure is done to get the lowest AIC). Validation was performed using a block cross-validation procedure (Roberts et al., 2017) using the “blockCV” package (Valavi et al., 2019). In this method, the study area is divided into blocks with a predefined size. The sizes of the blocks (approximately 274 km for M. cavernosa and Siderastrea sp. and 284 km for M. hispida) are established with an embedded function of the “blockCV” package that takes into account spatial autocorrelation. Each block is then assigned to one out of five groups. This assignment to groups is done randomly after 100 iterations, and the iteration that results in the most even proportion of presence and absence data in each group is selected. The models were run five times, with four groups of blocks selected for training the model and another group selected for testing each time. Finally, the model was trained with all the data available and used to build the ensemble if it scored well in the cross-validation (see below). Evaluation metrics were obtained through the mean of the fivefold cross-validation procedure. This method was chosen instead of the typical random data split because it is more sensitive to spatial variability and thus also provides better support for future condition projections (Wenger and Olden, 2012).
The ensembles were generated using the full models (i.e., using all data for training) of the algorithms that achieved mean TSS (true skill statistics) scores of at least 0.7, as assessed from the five block cross validations. This TSS score was considered above “good” in Morato et al. (2020). The ensembles were constructed using the committee averaging method (Araújo and New, 2007). In this method, models are first converted to binary maps using, as a threshold, the value that maximizes the balance between sensitivity and specificity (thus maximizing the TSS score). The models are then summed and divided by the number of models that were included in the ensemble. In this way, the values of the committee map are equivalent to the number of models that agree with the presence or absence reported in that cell. We thus produced final binary maps from the committee averaging results using, as a threshold, the value where at least three out of the four models agreed with the prediction. The importance of variables to the ensemble model was assessed through an embedded function of the “BIOMOD2” package based on the permutation of variables. In each permutation, one variable is removed, and a new model is generated. Then, a Pearson’s correlation between the original prediction and the “shuffled” prediction is generated, giving the importance of the variable to the model as 1-the Pearson’s correlation. Each variable was permutated 20 times.
To assess the spatial uncertainty associated with model predictions, we used a bootstrap approach, resampling the data with replacement and generating new models and predictions for the study area (Anderson et al., 2016). This procedure was repeated 100 times for each species. We thus computed two metrics for each cell of the map: the coefficient of variation (standard deviation divided by the mean) based on the raw models (non-binary) and the “unalikeability” for the binary ensembles. We opted for this metric, also called u2, because of its simplicity, easy interpretation and appropriateness for categorical outcomes (Kader and Perry, 2007). The “unalikeability” metric measures how often the outcomes vary; the metric ranges from 0 (no variation) to 0.5 (equal proportion of occurrence between outcomes). We also measured to what extent the localities in current and future environmental layers presented differences to the data used to train the models (presences/pseudo-absences in the current period) using the multivariate environmental similarity surface approach (MESS) (Elith et al., 2010). MESS measures the similarity between a point and a set of reference points, giving negatives values to those points that are dissimilar to the reference condition (with more extreme values associated to more dissimilar ones). This can inform where models are extrapolating to new conditions and thus special attention is needed when interpreting the model output. The final maps of the models were analyzed with the help of QGIS version 3.10.8 and plotted using the “ggplot2” package. All analyses were performed in R software version 3.6.2 (R Core Team, 2019). All data and codes are available at https://github.com/silasprincipe/reefbuilders, along with a reproducible version of the results section. The same data are available in the FigShare repository https://doi.org/10.6084/m9.figshare.14102825.
Results
Models Performance and Variables Importance
The models performed well for all three species (Table 1). All algorithms achieved TSS scores higher than the established threshold of 0.7 in the cross-validation procedure and were thus included in the final ensemble. The BRT and RF algorithms achieved the highest TSS scores, except when applied for Siderastrea sp., for which the GLMs outperformed RFs. The GAMs consistently yielded low TSS values among the algorithms. The AUC (area under the curve) values were higher than the TSS values for all models, but this metric can be inflated and should be used with caution as a model performance metric.
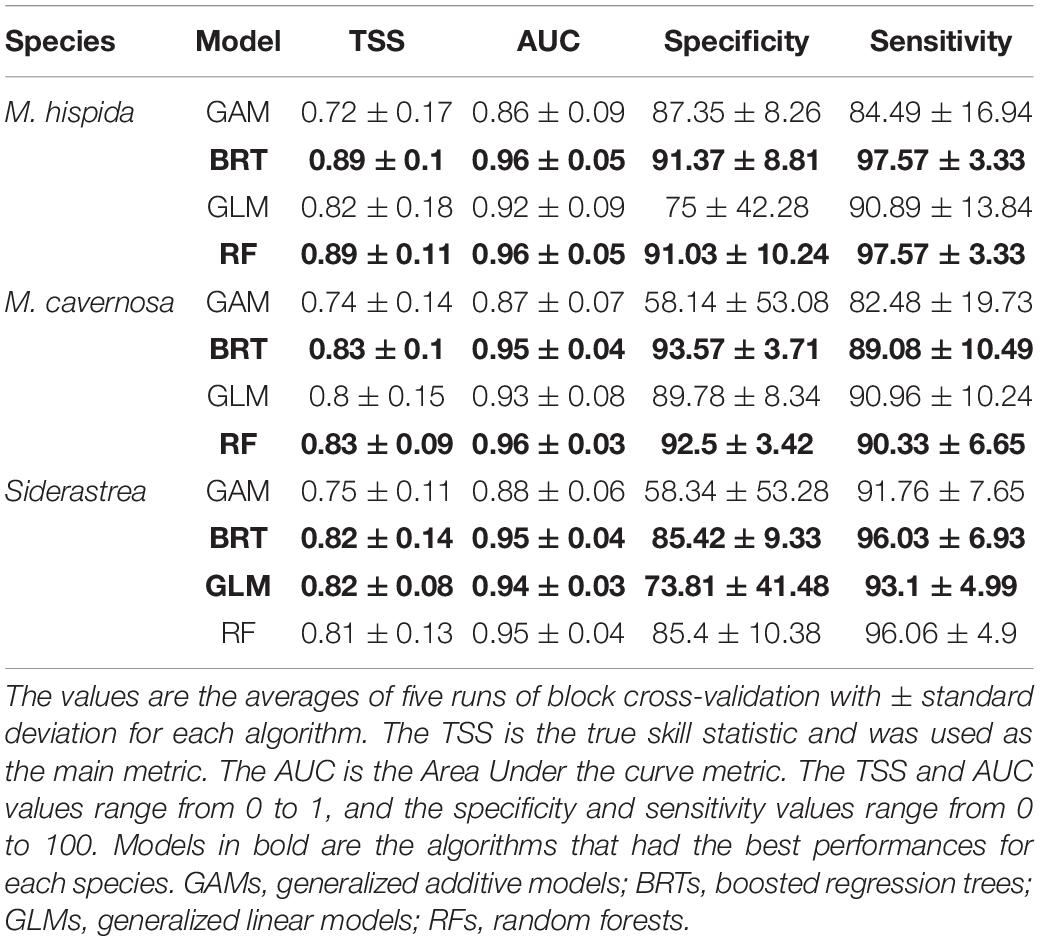
Table 1. Performance of species distribution models for Mussismilia hispida, Montastraea cavernosa and Siderastrea obtained through four distinct algorithms.
Our bootstrap analysis for detecting uncertainty in the predictions of models showed higher variations on the edges of the occurrence areas (Supplementary Figures 1–3). Thus, the predictions are thought to be more reliable in the current range of the species than elsewhere. The “unalikeability,” based on the binary ensembles, was less than 0.3 for all models/species. In general, the models were more often in disagreement on the edges of the presence points. As expected, the future predictions showed consistently higher uncertainty for all species when compared to the current period. Additionally, MESS analysis indicated that dissimilarities between the environmental data used for training the models and projected environmental conditions (including the current period) vary depending on the scenario considered. Smaller areas had dissimilar values in the current period and in the RCP 2.6 and 4.5 scenarios. In the RCP 8.5, areas in the Caribbean and in the coast of Africa (between 15°S and 15°N) were the ones which presented more dissimilar conditions. In most cases, no more than two variables were responsible for the extrapolation (although some small portions had values of up to five variables for M. hispida). These extrapolation zones were spatially explicit on the map (Supplementary Figures 4–6), and more attention was given to the interpretation of the results in these detected areas.
The most relevant variables for the models varied among species (Table 2). Salinity contributed the most to explaining the habitat suitability of M. hispida geographically expressed in the ensemble model. For M. cavernosa and Siderastrea sp., chlorophyll-a and temperature were the variables with the greatest importance. Calcite, the current velocity and the cloud cover showed minor importance for all species models.
Distributions of Reef Builders
All species studied here should experience a reduction in the suitability of their habitats in the future (2100) in at least one scenario; however, increases in suitable areas were also projected in some regions. As expected, M. hispida showed the smallest suitable area among the three species (even considering suitable areas beyond its current range), while Siderastrea sp. had the widest range of suitable areas. A summary of the current distributions and expected changes in the future scenarios is provided in Table 3. To better understand how the species are distributed in the present and in the future, we divided the Atlantic into three distinct regions, using the Marine Ecoregions of the World classification as a reference (Spalding et al., 2007). The first region was named the Southwestern Atlantic region and comprises the warm temperate southwestern Atlantic, tropical southwestern Atlantic, North Brazil Shelf and Magellanic provinces. The TNA region includes the province of the same name and all the areas to the north. Finally, the Eastern Atlantic region comprises all provinces in the eastern part of our study area, including the islands not contained in the other provinces.
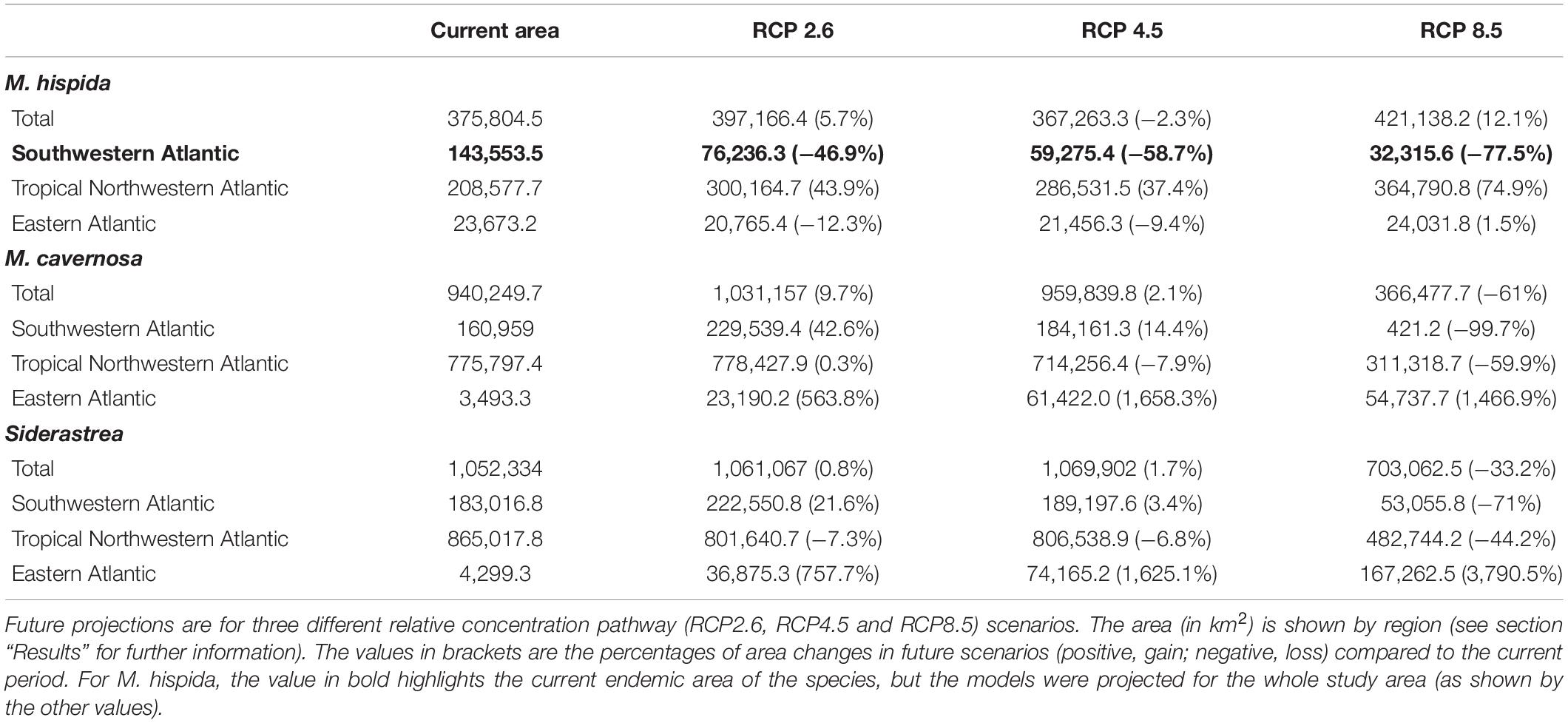
Table 3. Current and future projected areas for three reef builders of the Atlantic obtained through species distribution models.
In the Southwestern Atlantic, which is the current range of distribution of M. hispida, suitable areas were projected from the north coast of Santa Catarina (27°S) to Maranhão state (0.8°S), with some gaps associated with river discharges (Figure 1). A major gap is associated with the discharge of the São Francisco River (10°S), one of the major Brazilian rivers. This gap was also evident in the ranges of M. cavernosa and Siderastrea sp. (Figures 2, 3, respectively). To the south, another gap is located near the areas influenced by the Doce River along the coast of Espírito Santo state (approximately 19–20°S). Additionally, there is a discontinuity in the distribution associated with Guanabara Bay on the coast of Rio de Janeiro state (23°S). In northern Brazil, only two patches of suitable areas were projected, with the area farther north associated with the Parcel do Manuel Luis reef. Beyond the current distribution of the species, the model also identified suitable areas in the Caribbean region and on the coast of Morocco. Although the model projected suitable areas for M. hispida at depths over 100 m, the majority of suitable areas were shallower than 40 m (median = 21.2 m; Q1 = 42.4 m; Q3 = 8.2 m), which is in agreement with the actual biology of the species.
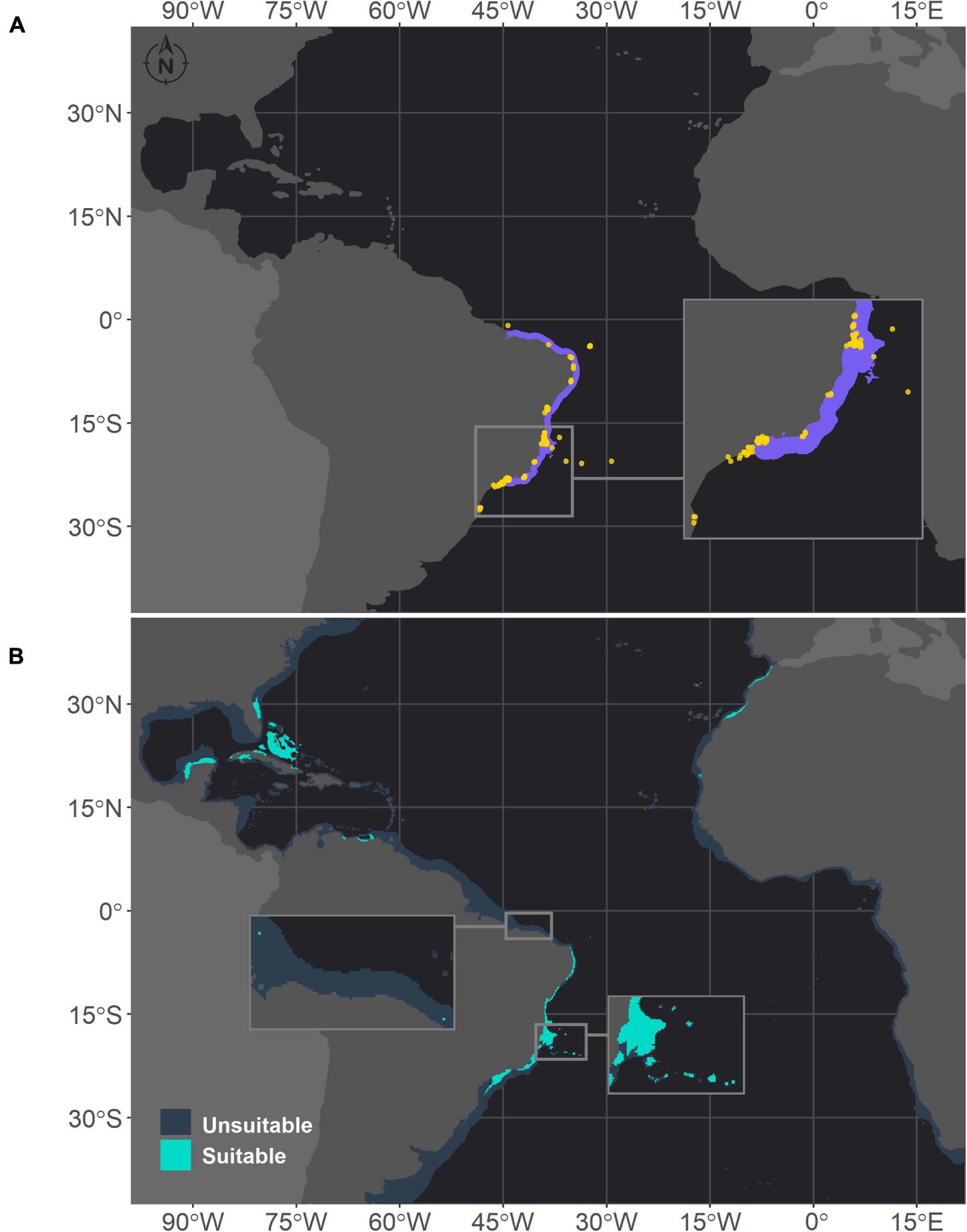
Figure 1. Projected habitat suitability of Mussismilia hispida in the Atlantic obtained through an ensemble modeling approach. Map (A) shows the presence points (in orange) used for training the model and shows (in purple) the expected range of M. hispida according to the IUCN. Map (B) presents the predicted habitat suitability for the current period. In both maps, inset maps show the Abrolhos region, where M. hispida plays a key role as a habitat-forming species. In map (B), the top inset map highlights the patches of suitable areas in northern Brazil.
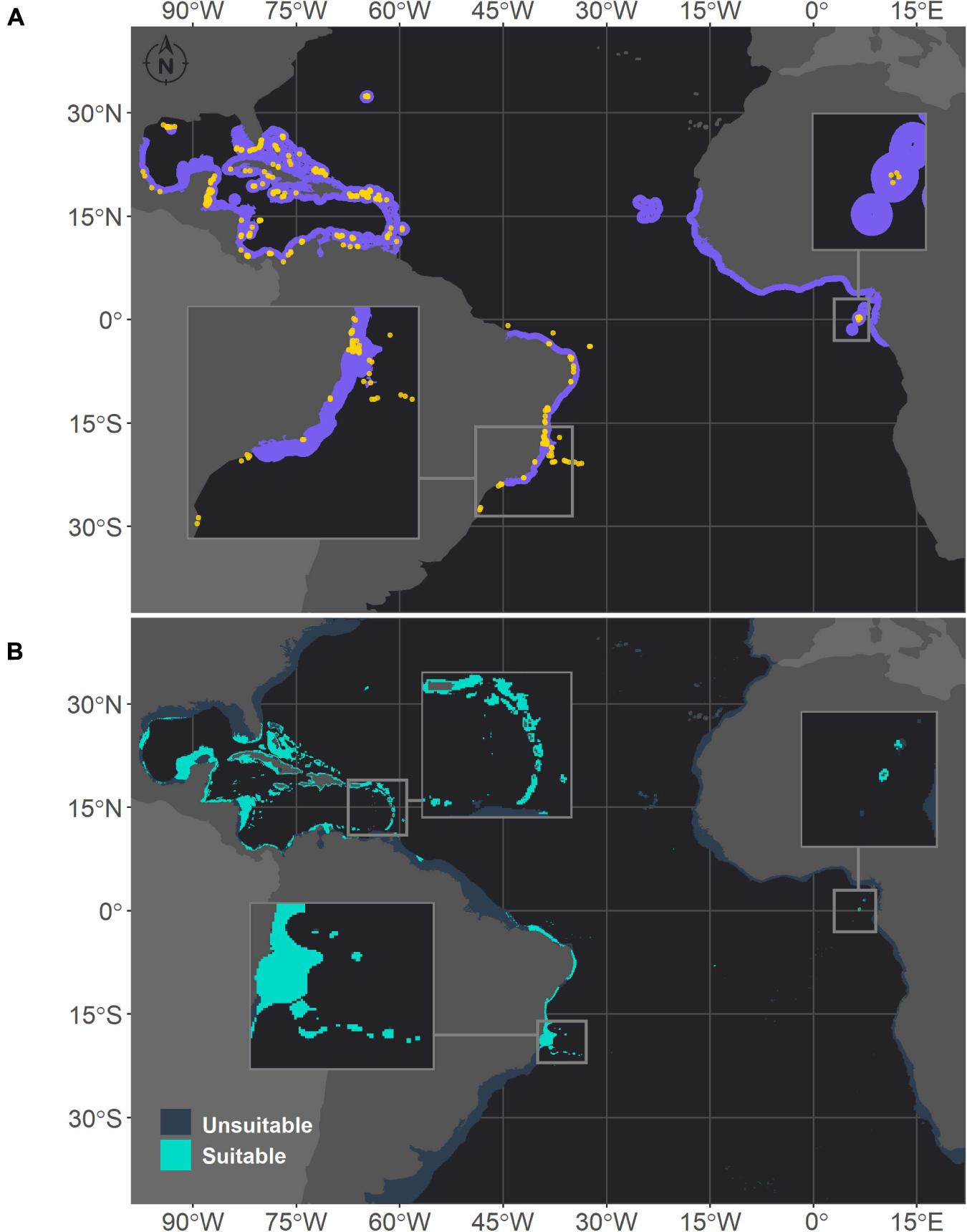
Figure 2. Projected habitat suitability for Montastraea cavernosa in the Atlantic obtained through an ensemble modeling approach. Map (A) shows the presence points (in orange) used for training the model and shows (in purple) the expected range of M. cavernosa according to the IUCN. Map (B) shows the predicted habitat suitability for the current period. In both maps, inset maps highlight the Abrolhos region (left) and São Tomé islands (right), and in map (B), the top inset highlights the Caribbean islands.
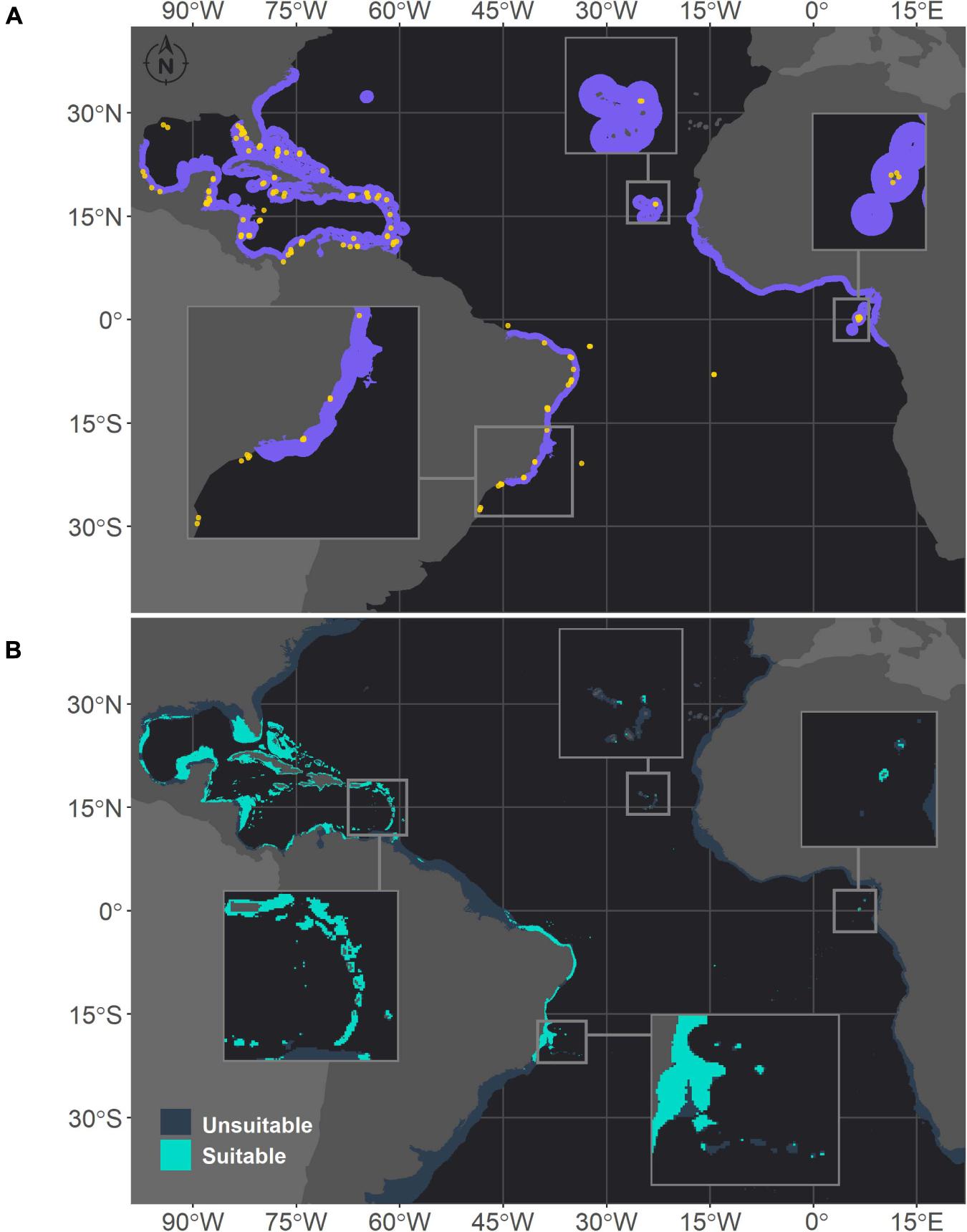
Figure 3. Projected habitat suitability for Siderastrea sp. in the Atlantic, as obtained through an ensemble modeling approach. Map (A) shows the presence points (in orange) used for training the model and shows (in purple) the expected range of Siderastrea according to the IUCN. Map (B) shows the predicted habitat suitability for the current period. In both maps, inset maps highlight the Abrolhos region (left), Cape Verde (top) and São Tomé islands (right), and in map (B), the top left inset highlights the Caribbean islands.
There were increases in the projected suitable areas for M. hispida in two future scenarios (RCP2.6 and RCP4.5; Figure 4). However, when considering only areas in the Southwestern Atlantic region, decreases are expected in all scenarios (Table 3 and Figure 4). Suitable areas for M. hispida will be lost all along the northeastern coast of Brazil and along its southern limit, regardless of the scenario. Additionally, the two patches on the coast of the Maranhão (0.8°S) and Ceará (3.6°S) states will be lost. Major losses of suitable areas will also occur in the Vitória-Trindade seamount chain and in the Abrolhos region; these losses would be critical in the RCP4.5 and RCP8.5 scenarios, with all suitable areas being lost.
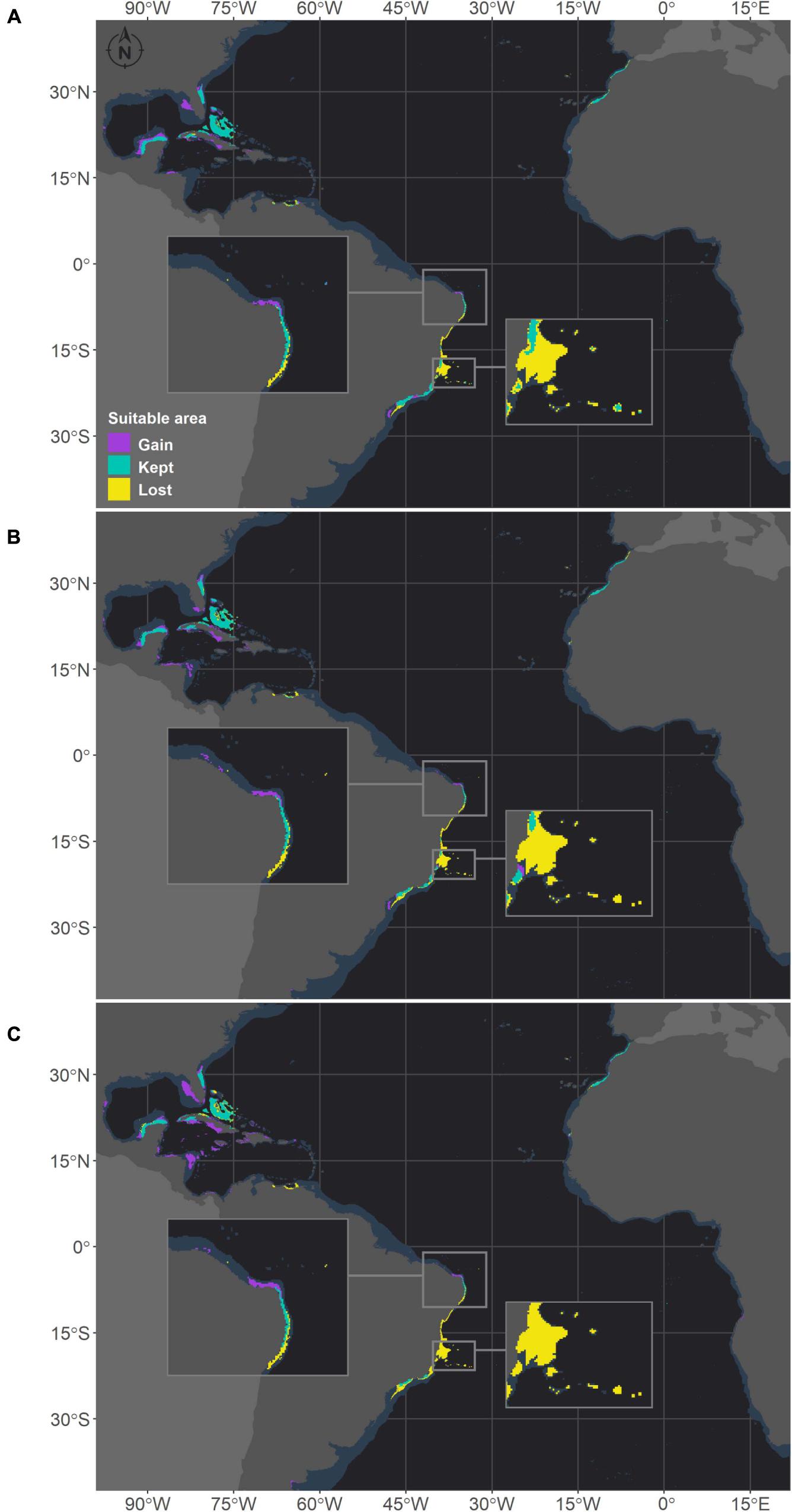
Figure 4. Projected habitat suitabilities for future relative concentration pathway (RCP) scenarios for Mussismilia hispida in the Atlantic Ocean, obtained through an ensemble modeling approach. The models were trained on present-day data of each species and projected for the (A) RCP2.6, (B) RCP4.5, and (C) RCP8.5 scenarios. Inset maps highlight changes in northern Brazil (top) and in the Abrolhos region (bottom).
New suitable areas are predicted in the southern and northern boundaries of the present distribution of M. hispida for all scenarios. In the RCP4.5 and RCP8.5 scenarios, the gain of new areas is diminished, but suitable areas are expected to occur far south of the current distribution region along the coast of Argentina (40–42°S). Interestingly, some areas that would become suitable in RCP2.6 scenario are in regions of river discharge. New suitable areas would also occur in the denominated TNA region. These projections do not consider the Amazon River mouth barrier, which currently limits the distribution of this species to the Brazilian coast. There was no evident change trend in the suitable area depth (RCP2.6: median = 20.1 m, RCP4.5: median = 16.8 m, RCP8.5: median = 17.3 m; Supplementary Table 3).
Montastraea cavernosa and Siderastrea sp. exhibited similar habitat suitability patterns in the current period, in agreement with their current distribution ranges (Figures 2, 3, respectively). The majority of suitable areas for both species were distributed in the northeastern and north coast of Brazil, up to the coast of Maranhão state and in the TNA. Suitable areas were also observed at similar depths, and as expected the maximum depth of M. cavernosa was deeper than that of Siderastrea sp. (M. cavernosa – median = 40.5 m, maximum = 194.6 m; Siderastrea sp. – median = 37.6 m, maximum = 185.8 m; Supplementary Table 3). Despite these similarities, some differences in habitat suitability are evident. The predicted southern limit of the suitable areas for M. cavernosa was 22.4°S, but for Siderastrea sp. the distribution extended farther south, reaching Rio de Janeiro state (23°S). Additionally, adequate areas for Siderastrea sp. were predicted on Ascension, Cape Verde, and São Tomé islands, but suitable areas for M. cavernosa were found only on São Tomé and Ascension (although the species is not currently found in the latter). Another evident difference is the larger extent of suitable areas for Siderastrea sp. in the Gulf of México and on the western side of Florida than that for M. cavernosa. However, M. cavernosa was the only species with suitable areas on Bermudas Island in the western Atlantic, extending farther north than Siderastrea sp. (approximately 32°N vs. approximately 28°N for Siderastrea sp.). Several areas that were considered within the ranges of both species according to the IUCN were not supported by our models as suitable areas.
Montastraea cavernosa and Siderastrea sp. are projected to experience net gains in suitable areas under the RCP2.6 and RCP4.5 scenarios, but the amounts vary between species, with M. cavernosa gaining more area than Siderastrea sp. under both scenarios (Table 3). Along the Brazilian coast, areas to the south would become suitable for M. cavernosa under the RCP2.6 and RCP4.5 scenarios, consistent with poleward increases in suitable areas (Figure 5). None of those areas are retained in the RCP8.5 scenario. Siderastrea sp. showed similar patterns of increases in suitable areas along the Brazilian coast (Figure 6); however, it showed a smaller southward expansion when compared to M. cavernosa. Additionally, more suitable areas were projected in the Abrolhos region under the RCP2.6 and RCP4.5 scenarios. In contrast to M. cavernosa, Siderastrea sp. would still broaden its distribution under the RCP8.5 scenario. In the Northern Hemisphere, the models projected new adequate areas for both M. cavernosa and Siderastrea sp. around their current occurrence ranges either under the RCP2.6 and RCP4.5 scenarios but not in the RCP8.5 scenario (Figures 5, 6, respectively). A poleward increase in suitable areas (to the north) was projected for M. cavernosa in the RCP4.5 and RCP8.5 scenarios and for Siderastrea sp. in all scenarios. Areas around Bermuda Island should be adequate for Siderastrea sp. under the RCP4.5 and RCP8.5 scenarios. In the eastern Atlantic, there were consistent increases in suitable areas for M. cavernosa and Siderastrea sp. considering all scenarios in the region of Guinea, in portions of the Gulf of Guinea and its islands, on Cape Verde islands and on seamounts from approximately 3–16°S. As also observed for M. hispida, no evident change in depth of suitable areas were identified for both species (Supplementary Table 3).
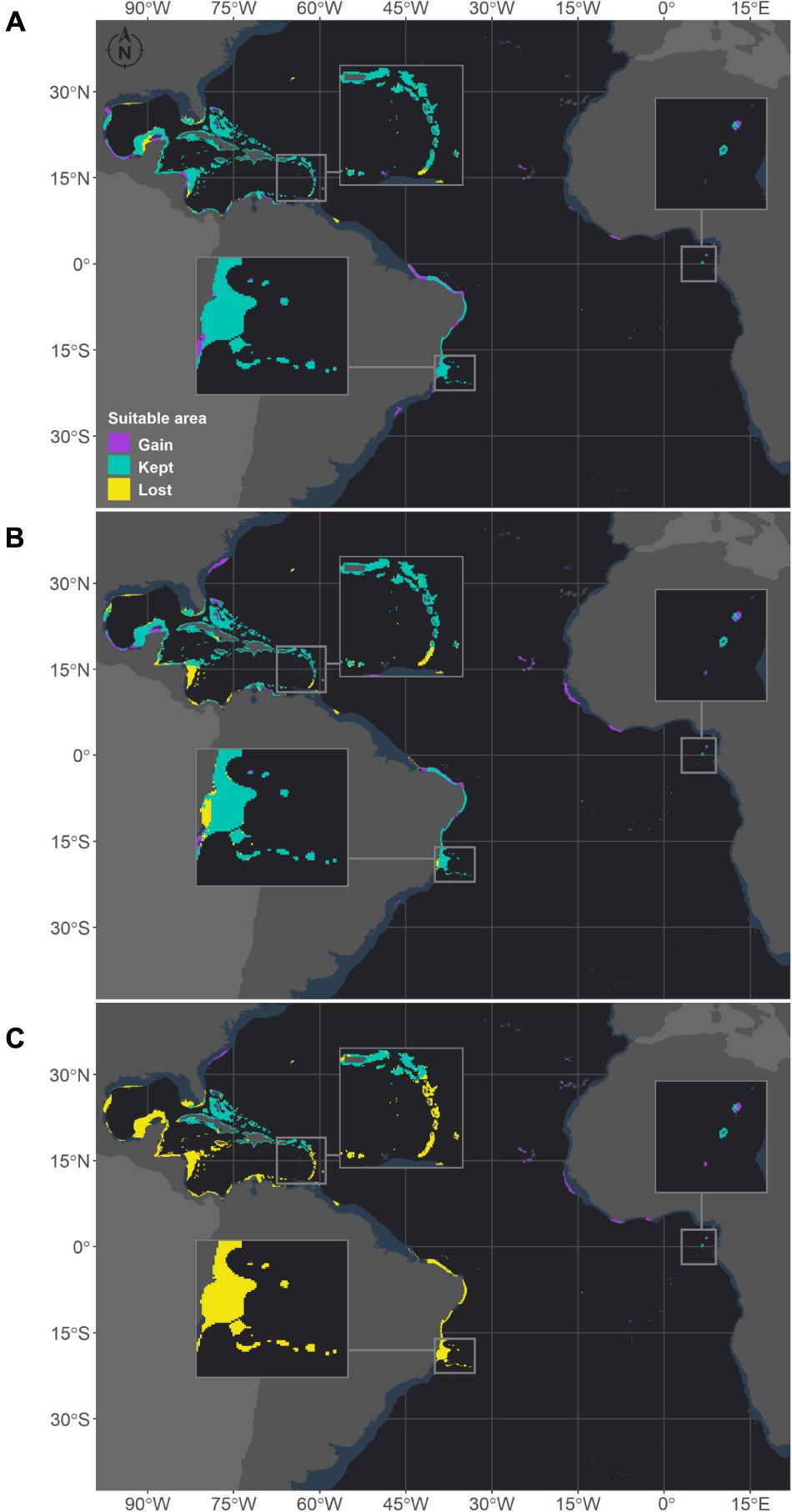
Figure 5. Projected habitat suitability for future relative concentration pathway (RCP) scenarios for Montastraea cavernosa in the Atlantic obtained through an ensemble modeling approach. Models were trained on present-day data of the species and projected for the (A) RCP2.6, (B) RCP4.5, and (C) RCP8.5 scenarios. Inset maps highlight the changes in the Caribbean region (top left), São Tomé islands (right) and in the Abrolhos region (bottom).
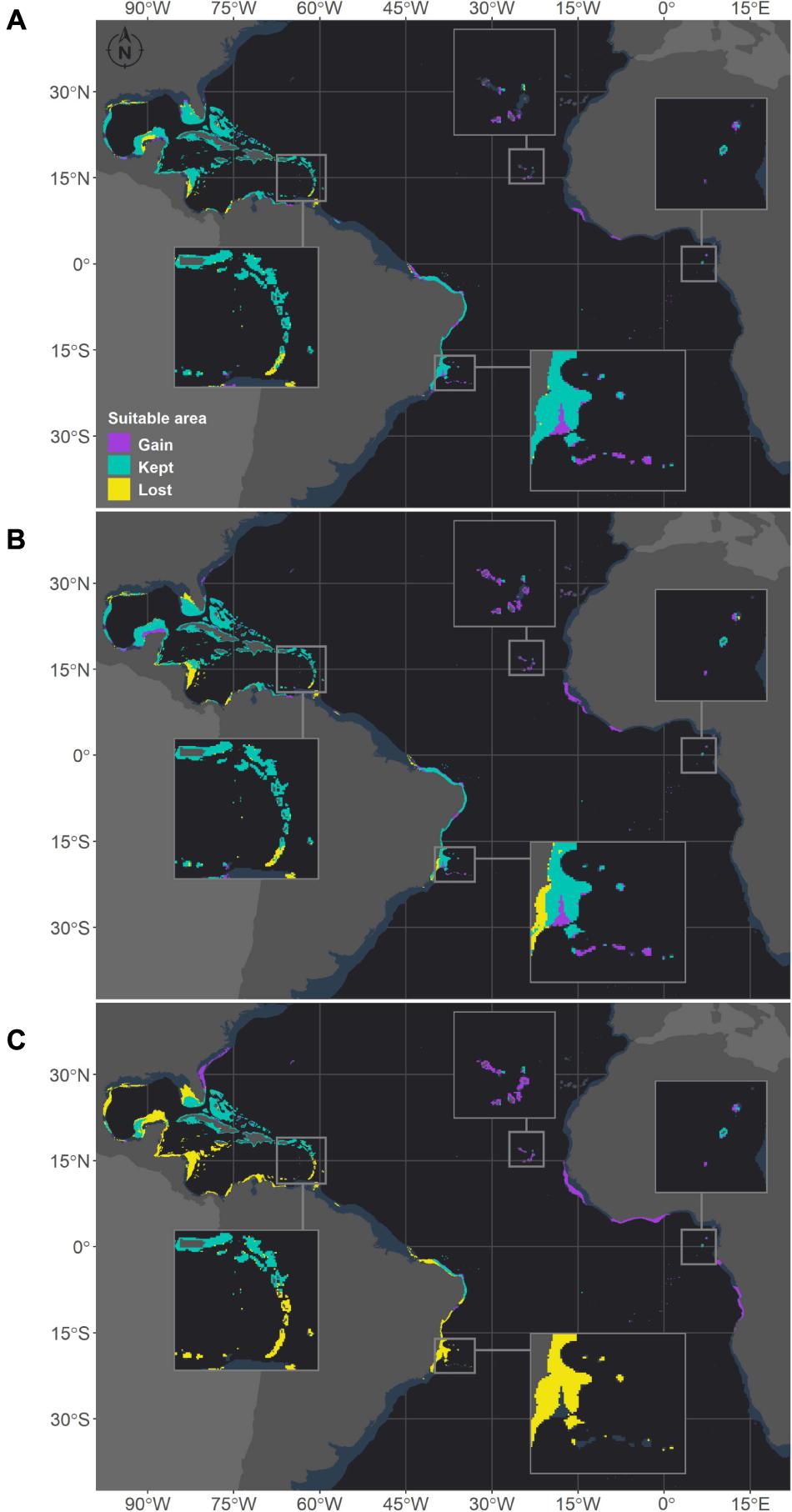
Figure 6. Projected habitat suitability under future relative concentration pathway (RCP) scenarios for Siderastrea sp. in the Atlantic Ocean obtained through an ensemble modeling approach. The models were trained on present-day data of the species and projected for the (A) RCP2.6, (B) RCP4.5, and (C) RCP8.5 scenarios. Inset maps highlight changes in the Caribbean region (top left), Cape Verde islands (top), São Tomé islands (right) and Abrolhos region (bottom).
Despite the net gains in suitable areas under RCP2.6 and RCP4.5, both M. cavernosa and Siderastrea sp. would lose suitable areas in regions that are currently adequate for them. As for the RCP8.5, both species would experience net losses (Table 3). Along the Brazilian coast, M. cavernosa would shrink its northern limit in the RCP2.6 and RCP4.5 and also lose areas in the Abrolhos region in this latter scenario (Figure 5). The RCP8.5 is more drastic than the other two scenarios; M. cavernosa is predicted to vanish from the entire Brazilian coast, with the exception of Fernando de Noronha Island and most of the Caribbean and Gulf of Mexico. For Siderastrea sp., the loss of suitable areas along the Brazilian coast includes both the northern and southern limits; the most critical loss is projected under RCP8.5, in which only the areas from 1.5 to 11°S are retained. None of the areas in the eastern Atlantic were lost in any projection for either species.
We also stacked the future projections for all species to analyze how climate change may affect the composition of species (Figure 7). This projection reflects the composition of suitable niches for this group of species and not the composition of species in its realized niche, as a given species may not occur in the full extent of its suitable modeled niche. The areas where the number of species would be reduced were consistent among the three scenarios. However, for RCP8.5, the loss of species is higher in several areas and seems to be critical in the tropical TNA and on the Brazilian coast. Within the same conditions, areas in the Gulf of Mexico that are shared by Siderastrea sp. and M. cavernosa would retain only Siderastrea sp. Additionally, in all scenarios, areas in the western Caribbean and the Gulf of Mexico would completely lose suitability for both Siderastrea sp. and M. cavernosa. On the Brazilian coast, losses of species are expected along the coastal extension in all scenarios, and this loss is critical in the RCP8.5 scenario. This is mainly due to the loss of suitable areas for M. hispida and M. cavernosa in this region. Under this scenario, major losses are concentrated in the Abrolhos region and the Vitória-Trindade seamount chain, which mainly reflects the reduction of suitable areas for M. hispida. Also, all species would be lost in the Abrolhos region, while in northern Brazil only Siderastrea sp. would remain.
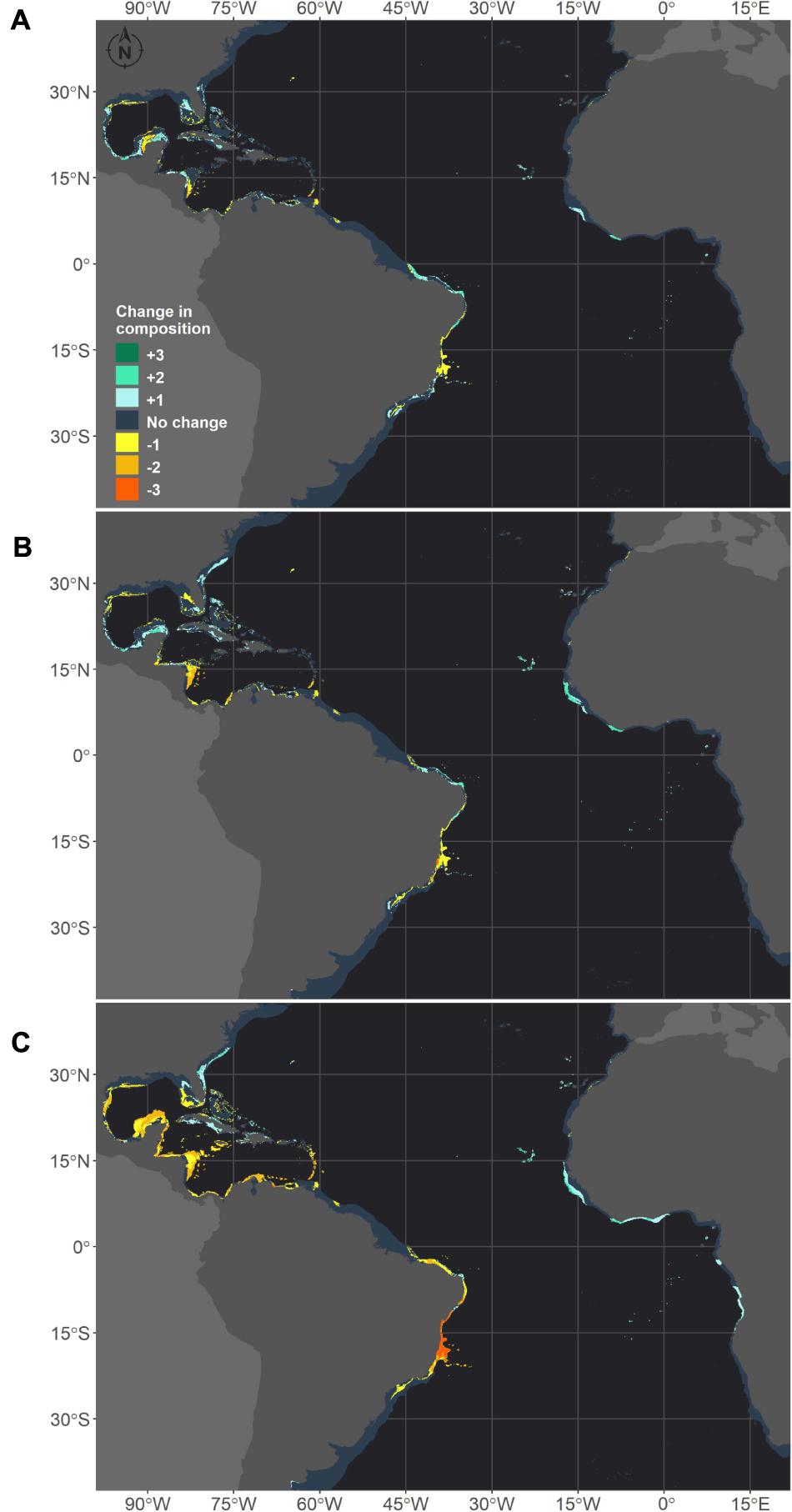
Figure 7. Future composition of adequate habitats for reef builder species in the western Atlantic obtained through species distribution models generated for three species: M. hispida, M. cavernosa and Siderastrea sp. Map (A) shows the number of species gained (positive values – green scale) or lost (negative values – yellow scale) in the RCP2.6 scenario in comparison to the current climate. The same is shown for the RCP4.5 (B) and RCP8.5 (C) scenarios. The dark gray depicts no change in the number of species.
The areas where the number of species would increase differed between the RCP8.5 and the other two scenarios, but there were consistent increases in the suitable habitat area in the Eastern Atlantic in all projections. In the RCP2.6 and RCP4.5, areas along Georgia and South Carolina and in the Gulf of Mexico and Caribbean Sea would become adequate for Siderastrea sp. and M. cavernosa. Some areas in the Yucatan Channel and in the Bahamas could also experience increases in the number of species due to the expansion of suitable areas for M. hispida. It is also important to note that some areas along the Caribbean, Gulf of México, and around Florida that are predicted to keep at least one species are, in fact, areas where there is suitable habitat for M. hispida; however, this species is currently restricted to Brazil. Along the Brazilian coast, increases in the number of species are expected in the southern limits of the corals studied here under the RCP2.6 and RCP4.5 scenarios and in Northeast/North Brazil under all scenarios (but reduced in the RCP8.5 scenario). Compared to the current period, changes were predicted for almost all regions where suitable areas were projected for the modeled species.
A map with the current composition of suitable niches for the three species is provided in Supplementary Figure 7. An interactive version of the results section is available at http://silasprincipe.github.io/reefbuilders.
Discussion
Climate change is impacting coral reefs worldwide (Hughes et al., 2017; Kubicek et al., 2019), increasing bleaching events (Duarte et al., 2020), changing distributional patterns (Yamano et al., 2011; Vergés et al., 2019) and decreasing ecosystem functioning (Williams and Graham, 2019; Morais et al., 2020). Understanding the composition and functioning of reefs in the future has become an urgent task and can help to target conservation actions (Williams and Graham, 2019). Here, we showed that the distributions of suitable areas for three major reef builders in the western Atlantic are expected to change under all considered RCP scenarios (2.6, 4.5, and 8.5). In some areas of the Gulf of Mexico and the Caribbean, suitable areas for M. cavernosa and Siderastrea sp. will disappear, with potential cascading effects in the communities. The same is expected for some areas on the Brazilian coast, where all species modeled here would lose suitable habitats under the considered scenarios. The loss of reef builders can potentially reduce habitat complexity (Graham and Nash, 2013), which is a major driver of coral reef richness (Darling et al., 2017). Although species may find new suitable areas in the future and even expand their distributions, for example, in the eastern Atlantic, the predicted changes in the distribution patterns of these three reef builders have implications for the persistence of Atlantic coral reefs. In the most extreme case, the RCP 8.5 scenario, drastic changes in the distribution of the species will occur, with all facing range reductions in the Western Atlantic. This critical scenario is unlikely considering the current trajectories of emissions, and we are probably closer to an intermediate scenario (RCP 4.5 or RCP 6.0) (Hausfather and Peters, 2020). However, those results are a clear warning of the need for urgent climate action in order to avoid an extreme scenario and for protecting Atlantic coral reefs.
Current Distributions of Reef Builders
Coral reefs in the Atlantic are present throughout the tropical realm but are concentrated in two very distinct provinces: the TNA and the Tropical Southwest Atlantic (TSA) (sensu Spalding et al., 2007). True coral reefs are not present in the eastern Atlantic, although some coral species thrive on the islands of the Gulf of Guinea and on Cape Verde. Diversity patterns are considerably different between the TNA and the TSA (Porter, 1972; Floeter et al., 2008), with Caribbean reefs having higher species richness (Floeter et al., 2008). However, Brazilian reefs have high endemism rates, despite representing a reduced percentage of marine ecosystems in the region (Castro and Pires, 2001). Among zooxanthellate Scleractinia, four species of Mussismilia are endemic to Brazil (Pires et al., 1999; Castro and Pires, 2001; Nunes et al., 2008). M. hispida is a major builder in Brazilian shallow water reef formations (Oigman-Pszczol and Creed, 2004; Leão et al., 2016). The areas in the TSA predicted to be suitable for M. hispida are partially in agreement with the current distribution range of the species, extending from Maranhão to São Paulo state (Leão et al., 2016), but we found some suitable areas up to Santa Catarina state (26.5°S). Our models revealed the strong effect that salinity has on the distribution of M. hispida. Indeed, salinity was found to be a significant variable in the global distributions of coral species (Couce et al., 2012). For M. hispida reduced salinity is a constraint (Laborel, 1970), as seen in the two gaps associated with major rivers. The same limitation is also observed for the hydrocoral genus Millepora (de Souza et al., 2017). In the same sense, the Amazon River discharge has historically restrained the dispersion of M. hispida further to the north and prevented the species from occupying other suitable areas on the coast of Venezuela that were revealed in our model. Interestingly, other variables that are closely linked to river discharges, such as silicate, had only a minor importance in the models for M. hispida. This marginal importance may reflect the higher tolerance of most Brazilian coral species to high-turbidity waters that are influenced by terrestrial runoff (Laborel, 1970; Mies et al., 2020).
Montastraea cavernosa and Siderastrea sp. showed similar distribution patterns in both the TNA and South Atlantic. M. cavernosa is one of the major contributors to reef structures in the Atlantic, including mesophotic areas (e.g., Goreau and Wells, 1967; Horta-Puga, 2003), and has a strong identitarian effect on the structural complexity of reefs (Alvarez-Filip et al., 2011a). In contrast, despite also being a depth generalist, Siderastrea sp. usually occupies shallower areas of Southwestern Atlantic reefs and is also important and abundant in the intertidal region (Oigman-Pszczol and Creed, 2004). In Brazilian reefs, Siderastrea sp. is considered an abundant species, with high resistance to stressors (Leão et al., 2003). In other regions, however, Siderastrea sp. may not be so conspicuous. In this sense, M. cavernosa can be classified as a stress-tolerant species, while Siderastrea sp. (S. radians) is generally a weedy species (Darling et al., 2012). Thus, despite showing similar patterns of distribution, these species contribute differently to the structures of the areas where they were predicted to co-occur. The variables with great contributions to the niche modeling of M. cavernosa and Siderastrea sp. were the SST and chlorophyll-a concentration, more remarkably the latter in the case of M. cavernosa. Both species were negatively affected by chlorophyll-a, which is a proxy for primary production and is known to adversely affect zooxanthellate Scleractinia (Déath and Fabricius, 2010). Primary production is linked to the phosphate and nitrate concentrations, which also influenced M. cavernosa and Siderastrea sp. models in this study. The influence of phosphate and nitrate on corals also agrees with previous findings (Kleypas et al., 1999; Rodriguez et al., 2019). Although some works point to direct negative effects on corals by high concentrations or imbalances of these nutrients (Fabricius, 2005), evidence shows that higher levels of nitrate and phosphate lead to increased phytoplankton loads, with more direct impacts on corals. High loads of phosphate and nitrate may result of continental influence, such as urban or agricultural runoffs (D’Angelo and Wiedenmann, 2014). It is important to highlight that high nutrient levels are already threatening Brazilian coral reefs (Costa et al., 2000). SST was the most important variable for Siderastrea sp. Temperature is an important limiting factor for the occurrence of coral reefs, with lower temperatures limiting coral growth (Kleypas et al., 1999), while high temperatures can lead to coral bleaching and mortality (e.g., Eakin et al., 2010).
Even though the predicted distributions are mostly within the current ranges of the modeled species, they certainly do not occupy all these areas. M. hispida, for example, usually inhabits shallow reefs, although it is able to reach deeper depths (Morais and Santos, 2018). Thus, some areas where the species is predicted to occur may not be adequate due to its depths. Siderastrea sp. can also exhibit the same constraints, as it seems to prefer even shallower areas (Oigman-Pszczol and Creed, 2004). Nevertheless, not all areas offer suitable substrates for the establishment of zooxanthellate corals. In that sense, the current niche of M. hispida is smaller than predicted, raising even further concern regarding this species. Other species of Mussismilia that are endemic to Brazil also have narrow suitable niche areas and are at risk of extinction (de Oliveira et al., 2019). Also, it’s important to note that even if areas are considered suitable for a reef builder species, this may not reflect in reef formation nor in optimal growth conditions. Indeed, many areas in the coast of Africa predicted as suitable for M. cavernosa and Siderastrea have historically a limited coral growth, with no true coral reefs being formed, most likely due to large river discharges and low temperatures resulting from oceanographic features in the region (Garzoli et al., 1996; Arístegui et al., 2004). Thus, species may be present in a certain locality but in marginal, sub-optimal conditions.
Predicted Changes in the Distribution of Reef Builders
Reef builders, like other ecosystem engineers or habitat-forming species, provide habitats for a wide array of species by increasing niche availability (Graham and Nash, 2013; Coker et al., 2014). These species are also key in maintaining reef structures (Perry and Alvarez-Filip, 2019). Even when not playing a major role as reef builders, coral species still contribute to the structural complexity of reefs. Thus, the loss of coral species constitutes a major harm to tropical reefs (Hughes et al., 2017; Morais et al., 2020). Several areas in the Caribbean and along the Brazilian coast will lose suitability for the species modeled here under any of the future scenarios according to our projections. As expected, RCP8.5 poses the greatest risks, especially for the western Caribbean region, as almost the entire area could become inadequate for M. cavernosa and Siderastrea sp. This contrasts with the eastern Caribbean, which keeps suitability for both species in that scenario.
The situation is particularly critical on the Brazilian coast, as fewer species contribute to the formation of coral reefs in this area and functional redundancy is even lower than for the Caribbean (Castro and Pires, 2001; Leão et al., 2003). One of the most important coral species in Brazil is M. hispida, that have a broad distribution and is abundant in shallow reefs, especially between 4 and 25 m depths (Castro and Pires, 2001). As for Siderastrea sp., it is one of the most common coral species in even shallower zones of northern Brazil (Soares et al., 2011). As an example, in Itacolomis reef it can reach up to 16% of benthic cover in areas between 1 and 3 m of depth (Castro et al., 2006). In the Abrolhos region, M. cavernosa is the dominant scleractinian and the fourth most abundant benthic organism (Francini-Filho et al., 2013). In this sense, the major loss of suitability for this species predicted in Abrolhos is of special concern. Moreover, loss of coral cover on this region will negatively affect the provision of many ecosystem services and impact associated communities, such as traditional populations that depend on artisanal fisheries (De Moura et al., 2009). It is also worthy of note that the reduction in suitable areas for M. cavernosa is particularly troublesome for both Brazilian and Caribbean reefs, as this species plays a key habitat-forming role in mesophotic reefs (Fricke and Meischner, 1985; Bongaerts et al., 2010).
The reduction of suitable areas for foundation species can thus be critical for coral reef persistence in the Atlantic Ocean, affecting reef resilience through the loss of functions (Richardson et al., 2020), which in turn affects essential ecosystem services, such as coastal protection, fisheries and habitat provisioning (Woodhead et al., 2019; Rivera et al., 2020). The outcome cannot be predicted with certainty, but this new arrangement can affect organisms that depend on the high complexity of coral reefs. Reef complexity is directly linked to fish diversity and abundance (Graham and Nash, 2013; Coker et al., 2014) and is especially important for small-bodied and cryptobenthic fishes that depend on the niches created by such complexity (Alvarez-Filip et al., 2011b; Dalben and Floeter, 2012; Richardson et al., 2017). Other cryptobenthic organisms heavily depend on the structural complexity of their environment (Alexander, 2013) and may also be affected if the predicted reductions in habitat suitability for reef builders take place in the future. As biodiversity is directly linked to functioning in coral reefs, loss of species can impair the resilience of these ecosystems (Benkwitt et al., 2020). However, even higher biodiversity levels may not be enough to cope with the increase in frequency and severity of climate-related disturbances (Hughes et al., 2007; Benkwitt et al., 2020). Importantly, not only loss of foundation species but also changes in the composition of the reef building components can trigger substantial negative responses in coral reefs (Alvarez-Filip et al., 2013). Lastly, reefs with lower functional redundancy, as those of the Atlantic (and specially from Brazil), have an additional risk when there is loss or change in its species composition (Bellwood et al., 2004), adding to the importance in identifying how key species will respond to climate change.
Some areas may act as refuges for coral species, with no change in suitability predicted in the future. Portions of the Caribbean islands and part of the southern coast of Brazil (from approximately 22–24°S) are projected to maintain the same composition, at least under the RCP2.6 and RCP4.5. Additionally, Fernando de Noronha, Cape Verde, Ascension and São Tomé islands should still be adequate for these species in the future. Refuges are areas where climate change effects will be reduced and may provide certain stability to organisms; examples of refuges are high-latitude areas or locales with cool upwellings (Glynn, 1996). These areas can act as sources of larval supply and genetic variability to other areas (Bongaerts et al., 2010), constituting priority areas for conservation actions (e.g., the establishment of marine protected areas) that could guarantee protection from other anthropogenic threats (Beger et al., 2014). However, the conjecture that South Atlantic reefs may serve as refuges to global warming effects (Mies et al., 2020) is contrasted by our results, as indeed several areas are projected to lose suitability for reef builders regardless the scenario. Thus, it seems plausible that such resilience may not be enough to prevent the expected changes in the future. Deeper areas may also constitute refuges for some corals under climate change (Bongaerts et al., 2010), but these areas are certainly limited (Morais and Santos, 2018). As depth was included as a variable in our study, modeled response of species will be to some degree tied to the bathymetry layer, thus it is not possible to derive any conclusions regarding change of distribution patterns for different depths. The resolution employed here also hampers a more detailed analysis of the effects of depth on the studied species. Additionally, we found no evident trend of changes in the depths of suitable areas, even for M. cavernosa and M. hispida, for which depth showed minor importance in the model.
As expected, poleward increases in suitable areas were predicted for all species, and this result is in agreement with other recent findings of northward expansions of coral species in the Pacific (Yamano et al., 2011). Additionally, Baird et al. (2012) registered a poleward range expansion of Acropora spp. in the Pacific. This may lead to the tropicalization of previously subtropical or temperate ecosystems, impacting their dynamics and the persistence of temperate species (Vergés et al., 2019). Even if species are able to settle in new areas, local conditions may only be enough to support marginal reefs or no reefs at all. This is critical, as marginal reefs found in high latitudes usually have limited accretion and development rates (Camp et al., 2018). It is also important to point out that the establishment of coral species depends not solely on physical-chemical conditions but also on the availability of hard substrates. For instance, many places in the northern coast of the United States predicted as suitable in our study presents in general a muddy substrate. Thus, M. cavernosa and Siderastrea sp. may be unable to settle in some of the new projected areas, posing another concern for the future of Atlantic coral species. The same is valid for other areas in the Atlantic basin, such as the western coast of Africa, where species may expand their ranges. Today, the large number of drainages discharging sediments and freshwater, along with the lower temperatures of the Benguela Current, have limited the development of true coral reefs on the African Atlantic coast. A change in these conditions could certainly disrupt the functioning of marine communities in that region, enabling the establishment of coral species if adequate substrate is available. However, how those new coral communities will look like and function is still to be unveiled. Another cautionary note is necessary regarding the new suitable areas in future scenarios. The expansion of a given organism to new areas is not always possible and depends on a myriad of aspects, ranging from the reproductive strategy to the biophysical processes of the species (Yamano et al., 2011; Peluso et al., 2018). As an example, M. hispida is less prone to invade projected suitable areas in the Caribbean. Historically, the Amazon and Orinoco river discharges have acted as biogeographic filters that prevent the expansion of some South Atlantic species to the Caribbean (Floeter et al., 2008; Nunes et al., 2011). The settlement of M. hispida on the coast of Argentina, as predicted under the RCP4.5 and RCP8.5 scenarios, is also highly unlike due to the Plata River barrier, just to mention the more obvious. Understanding larval dynamics is important to be able to track the capacity of species to overcome these barriers, but a biophysical model for M. hispida larvae is lacking and deserves better investigation in the future.
In summary, although several areas targeted in our study remain suitable for at least one of the modeled species under the different scenarios, the scenarios are of concern, as the areas currently occupied by coral reefs may undergo compositional changes. Rodriguez et al. (2019) found the same trend of richness reductions in areas of the Caribbean and on the Brazilian coast for several stony coral species (but for a distinct scenario). Caribbean and Brazilian reefs are already experiencing negative climate change effects with the increase in frequency and intensity of heatwaves (Eakin et al., 2010; Duarte et al., 2020). This sums up to other climate change impacts that are imperiling coral reefs globally, as for example ocean acidification (Hoegh-Guldberg et al., 2007). Although Brazilian corals are thought to be more resilient than Caribbean corals (Banha et al., 2020; Mies et al., 2020), our results show that important species may face range reductions in the future. Based on our results, we suggest concentrating conservation actions in areas that should not undergo changes in their species compositions (portions of the Caribbean islands, southern coast of Brazil, Fernando de Noronha, Cape Verde, Ascension and São Tomé islands), as these areas can act as refuges. We also advise concentrating research efforts in areas with higher species loss predictions, namely, the Abrolhos region, northeast coast of Brazil, western Caribbean, Gulf of Mexico and areas on the coast of Florida, prioritizing actions for the monitoring and conservation of Western Atlantic coral reefs.
SDM Limitations
Although SDMs are an increasingly common tool used to predict habitat adequacy for species in current and future climates, it is important to highlight the possible limitations and caveats that are inherent to these modeling approaches. This analysis is not able to consider all environmental conditions that determine the realized niche of a given species, including its dispersion capacity or competition (Elith and Leathwick, 2009). Additionally, although the environmental variables selected here are deemed to be the ones that mostly affect corals, other variables (for example, terrain characteristics) also interact to produce the niches of benthic species (e.g., Chefaoui et al., 2017); however, data on many of these variables are not available for the whole studied basin. Climate models intrinsically also carry some uncertainty (IPCC, 2014). To reduce this uncertainty in SDM projections, we applied a block-cross validation procedure (Roberts et al., 2017; Valavi et al., 2019).
Presence data for a given species can also be incomplete due to limited/biased sampling and may carry a degree of positional uncertainty (Feeley and Silman, 2011). Here, we made an effort to obtain data from a large array of sources, applied strict quality control to the occurrence data, and limited the number of presence points to one per cell, a practice that was adopted in other studies to address oversampling effects (e.g., Durante et al., 2018). We also sampled pseudoabsences following robust techniques that can overcome the common problems associated with this procedure (e.g., Barbet-Massin et al., 2012). Regardless, the projected suitable areas for the current period are in agreement with the current range of the species and thus reflect the quality of our models. Despite these caveats, SDMs serve as important tools used to tackle the urgent demand for understanding the responses of species to climate change (Cheung et al., 2009; Guisan et al., 2013; Morato et al., 2020). Indeed, SDMs constitute indispensable tools for studies in areas where there is still limited knowledge of biodiversity, enabling extrapolative predictions with limited sets of data (e.g., Morato et al., 2020). The modeling approach developed and applied here proved to be robust in determining and mapping the habitat suitabilities of marine species, providing relevant results that can be used for conservation planning and to drive future research efforts (Robinson et al., 2017).
Conclusion
Three important reef builders of the western Atlantic could experience changes in their distributions regardless of the climate scenario. As expected, the RCP8.5 scenario reflected the highest proportion of niche suitability change. Even though M. hispida could expand to some new areas in the TNA, there is no guarantee that this species will be able to settle in those new regions, and the more probable scenario involves reductions in suitable areas along its current range under all scenarios. In this sense, the coral reefs of the Atlantic demand urgent conservation actions and better governance approaches to this rapidly changing Anthropocene condition.
Data Availability Statement
The datasets presented in this study can be found in online repositories. The names of the repository/repositories and accession number(s) can be found below: FigShare: https://doi.org/10.6084/m9.figshare.14102825 and GitHub: https://github.com/silasprincipe/reefbuilders.
Author Contributions
SP, AA, and TL contributed to the conception and design of the study. JA helped with data acquisition and filtering. SP created the codes, performed the modeling, and wrote the first draft of the manuscript. TL supervised the study. All authors contributed to manuscript revision, read, and approved the submitted version.
Funding
This work was funded by the National Council for Scientific and Technological Development (CNPq, Conselho Nacional de Desenvolvimento Científico e Tecnológico), National Institute of Science and Technology - INCT BioNat, grants # 465637/2014-0, and the State of São Paulo Research Foundation (FAPESP, Fundação de Amparo à Pesquisa do Estado de São Paulo), grants # 2014/50926-0, 2015/17177-6, and 2017/11948-6. JA received a grant from CNPq # 121118/2019-3 during this study. TL is a CNPq research fellow, grant # 302812/2017-2.
Conflict of Interest
The authors declare that the research was conducted in the absence of any commercial or financial relationships that could be construed as a potential conflict of interest.
Acknowledgments
We would like to thank Tianxiao Hao for his precious comments on SDM methods. We would also like to thank the BIOREC team for continuous support. SP was specially thankful to Muhammed Oyinlola for advice on modeling tools and to the Coding Club community for providing extensive high-quality material for R learning. We would also further like to thank the Open Source community.
Supplementary Material
The Supplementary Material for this article can be found online at: https://www.frontiersin.org/articles/10.3389/fmars.2021.673086/full#supplementary-material
Footnotes
- ^ https://www.gebco.net/
- ^ https://esgf-data.dkrz.de/search/cmip5-dkrz/
- ^ https://code.mpimet.mpg.de/projects/cdo
References
Alexander, T. J. (2013). Cryptic invertebrates on subtidal rocky reefs vary with microhabitat structure and protection from fishing. Mar. Ecol. Prog. Ser. 481, 93–104. doi: 10.3354/meps10263
Alvarez-Filip, L., Carricart-Ganivet, J. P., Horta-Puga, G., and Iglesias-Prieto, R. (2013). Shifts in coral-assemblage composition do not ensure persistence of reef functionality. Sci. Rep. 3:3486. doi: 10.1038/srep03486
Alvarez-Filip, L., Dulvy, N. K., Côteé, I. M., Watkinson, A. R., and Gill, J. A. (2011a). Coral identity underpins architectural complexity on Caribbean reefs. Ecol. Appl. 21, 2223–2231. doi: 10.1890/10-1563.1
Alvarez-Filip, L., Dulvy, N. K., Gill, J. A., Côté, I. M., and Watkinson, A. R. (2009). Flattening of Caribbean coral reefs: region-wide declines in architectural complexity. Proc. R. Soc. B Biol. Sci. 276, 3019–3025. doi: 10.1098/rspb.2009.0339
Alvarez-Filip, L., Gill, J. A., and Dulvy, N. K. (2011b). Complex reef architecture supports more small-bodied fishes and longer food chains on Caribbean reefs. Ecosphere 2:art118. doi: 10.1890/es11-00185.1
Anderson, O. F., Guinotte, J. M., Rowden, A. A., Tracey, D. M., Mackay, K. A., and Clark, M. R. (2016). Habitat suitability models for predicting the occurrence of vulnerable marine ecosystems in the seas around New Zealand. Deep Sea Res. I Oceanogr. Res. Pap. 115, 265–292. doi: 10.1016/j.dsr.2016.07.006
Anthony, K. R. N., Kline, D. I., Diaz-Pulido, G., Dove, S., and Hoegh-Guldberg, O. (2008). Ocean acidification causes bleaching and productivity loss in coral reef builders. Proc. Natl. Acad. Sci. U.S.A. 105, 17442–17446. doi: 10.1073/pnas.0804478105
Araújo, M. B., and New, M. (2007). Ensemble forecasting of species distributions. Trends Ecol. Evol. 22, 42–47. doi: 10.1016/j.tree.2006.09.010
Arístegui, J., Alvarez-Salgado, X. A., Barton, E. D., Figueras, F., Hernandez-Leon, S., Claude, R., et al. (2004). “Chapter 23. Oceanography and fisheries of the canary current / Iberian region of the eastern North Atlantic(18a, E),” in The Sea, eds K. H. Brink and A. R. Robinson (Harvard, MA: Harvard University Press), 877–932.
Assis, J., Tyberghein, L., Bosch, S., Verbruggen, H., Serrão, E. A., and De Clerck, O. (2018). Bio-ORACLE v2.0: extending marine data layers for bioclimatic modelling. Glob. Ecol. Biogeogr. 27, 277–284. doi: 10.1111/geb.12693
Baird, A. H., Sommer, B., and Madin, J. S. (2012). Pole-ward range expansion of Acropora spp. along the east coast of Australia. Coral Reefs 31, 1063–1063. doi: 10.1007/s00338-012-0928-6
Banha, T. N. S., Capel, K. C. C., Kitahara, M. V., Francini-Filho, R. B., Francini, C. L. B., Sumida, P. Y. G., et al. (2020). Low coral mortality during the most intense bleaching event ever recorded in subtropical Southwestern Atlantic reefs. Coral Reefs 39, 515–521. doi: 10.1007/s00338-019-01856-y
Barbet-Massin, M., Jiguet, F., Albert, C. H., and Thuiller, W. (2012). Selecting pseudo-absences for species distribution models: how, where and how many? Methods Ecol. Evol. 3, 327–338. doi: 10.1111/j.2041-210X.2011.00172.x
Beger, M., Sommer, B., Harrison, P. L., Smith, S. D. A., and Pandolfi, J. M. (2014). Conserving potential coral reef refuges at high latitudes. Divers. Distrib. 20, 245–257. doi: 10.1111/ddi.12140
Bellwood, D. R., Hughes, T. P., Folke, C., and Nyström, M. (2004). Confronting the coral reef crisis. Nature 429, 827–833. doi: 10.1038/nature02691
Benkwitt, C. E., Wilson, S. K., and Graham, N. A. J. (2020). Biodiversity increases ecosystem functions despite multiple stressors on coral reefs. Nat. Ecol. Evol. 4, 919–926. doi: 10.1038/s41559-020-1203-9
Bongaerts, P., Ridgway, T., Sampayo, E. M., and Hoegh-Guldberg, O. (2010). Assessing the “deep reef refugia” hypothesis: focus on Caribbean reefs. Coral Reefs 29, 309–327. doi: 10.1007/s00338-009-0581-x
Burgos, J. M., Buhl-Mortensen, L., Buhl-Mortensen, P., Ólafsdóttir, S. H., Steingrund, P., and Ragnarsson, S. Á, et al. (2020). Predicting the distribution of indicator taxa of vulnerable marine ecosystems in the arctic and sub-arctic waters of the Nordic Seas. Front. Mar. Sci. 7:131. doi: 10.3389/fmars.2020.00131
Cacciapaglia, C., and van Woesik, R. (2018). Marine species distribution modelling and the effects of genetic isolation under climate change. J. Biogeogr. 45, 154–163. doi: 10.1111/jbi.13115
Camp, E. F., Schoepf, V., Mumby, P. J., Hardtke, L. A., Rodolfo-Metalpa, R., Smith, D. J., et al. (2018). The future of coral reefs subject to rapid climate change: lessons from natural extreme environments. Front. Mar. Sci. 5:4. doi: 10.3389/fmars.2018.00004
Castro, C. B., de Amorim, L. C., Calderon, E. N., and Segal, B. (2006). Cobertura e recrutamento de corais recifais (Cnidaria: Scleractinia e Milleporidae) nos recifes Itacolomis, Brasil. Arq. Mus. Nac. 64, 29–40.
Castro, C. B., and Pires, D. O. (2001). Brazilian coral reefs: what we already know and what is still missing. Bull. Mar. Sci. 69, 357–371.
Cattano, C., Agostini, S., Harvey, B. P., Wada, S., Quattrocchi, F., Turco, G., et al. (2020). Changes in fish communities due to benthic habitat shifts under ocean acidification conditions. Sci. Total Environ. 725:138501. doi: 10.1016/j.scitotenv.2020.138501
Chefaoui, R. M., Casado-Amezúa, P., and Templado, J. (2017). Environmental drivers of distribution and reef development of the Mediterranean coral Cladocora caespitosa. Coral Reefs 36, 1195–1209. doi: 10.1007/s00338-017-1611-8
Cheung, W. W. L., Lam, V. W. Y., Sarmiento, J. L., Kearney, K., Watson, R., and Pauly, D. (2009). Projecting global marine biodiversity impacts under climate change scenarios. Fish Fish. 10, 235–251. doi: 10.1111/j.1467-2979.2008.00315.x
Coker, D. J., Wilson, S. K., and Pratchett, M. S. (2014). Importance of live coral habitat for reef fishes. Rev. Fish Biol. Fish. 24, 89–126. doi: 10.1007/s11160-013-9319-5
Costa, O. S. Jr., Leão, Z. M. A. N., Nimmo, M., and Attrill, M. J. (2000). Nutrification impacts on coral reefs from northern Bahia, Brazil. Hydrobiologia 440, 307–315. doi: 10.1023/A:1004104118208
Couce, E., Ridgwell, A., and Hendy, E. J. (2012). Environmental controls on the global distribution of shallow-water coral reefs. J. Biogeogr. 39, 1508–1523. doi: 10.1111/j.1365-2699.2012.02706.x
Courtney, T. A., Barnes, B. B., Chollett, I., Elahi, R., Gross, K., Guest, J. R., et al. (2020). Disturbances drive changes in coral community assemblages and coral calcification capacity. Ecosphere 11:e03066. doi: 10.1002/ecs2.3066
D’Angelo, C., and Wiedenmann, J. (2014). Impacts of nutrient enrichment on coral reefs: new perspectives and implications for coastal management and reef survival. Curr. Opin. Environ. Sustain. 7, 82–93. doi: 10.1016/j.cosust.2013.11.029
Dalben, A., and Floeter, S. R. (2012). Cryptobenthic reef fishes: depth distribution and correlations with habitat complexity and sea urchins. J. Fish Biol. 80, 852–865. doi: 10.1111/j.1095-8649.2012.03231.x
Darling, E. S., Alvarez-Filip, L., Oliver, T. A., Mcclanahan, T. R., and Côté, I. M. (2012). Evaluating life-history strategies of reef corals from species traits. Ecol. Lett. 15, 1378–1386. doi: 10.1111/j.1461-0248.2012.01861.x
Darling, E. S., Graham, N. A. J., Januchowski-Hartley, F. A., Nash, K. L., Pratchett, M. S., and Wilson, S. K. (2017). Relationships between structural complexity, coral traits, and reef fish assemblages. Coral Reefs 36, 561–575. doi: 10.1007/s00338-017-1539-z
Darling, E. S., McClanahan, T. R., and Côté, I. M. (2013). Life histories predict coral community disassembly under multiple stressors. Glob. Chang. Biol. 19, 1930–1940. doi: 10.1111/gcb.12191
De Moura, R. L., Minte-Vera, C. V., Curado, I. B., Francini-Filho, R. B., Rodrigues, H. D. C. L., Dutra, G. F., et al. (2009). Challenges and prospects of fisheries co-management under a marine extractive reserve framework in northeastern Brazil. Coast. Manag. 37, 617–632. doi: 10.1080/08920750903194165
de Oliveira, U. D. R., Gomes, P. B., Silva Cordeiro, R. T., de Lima, G. V., and Pérez, C. D. (2019). Modeling impacts of climate change on the potential habitat of an endangered Brazilian endemic coral: discussion about deep sea refugia. PLoS One 14:e0211171. doi: 10.1371/journal.pone.0211171
de Souza, J. N., Nunes, F. L. D., Zilberberg, C., Sanchez, J. A., Migotto, A. E., Hoeksema, B. W., et al. (2017). Contrasting patterns of connectivity among endemic and widespread fire coral species (Millepora spp.) in the tropical Southwestern Atlantic. Coral Reefs 36, 701–716. doi: 10.1007/s00338-017-1562-0
Déath, G., and Fabricius, K. (2010). Water quality as a regional driver of coral biodiversity and macroalgae on the great barrier reef. Ecol. Appl. 20, 840–850. doi: 10.1890/08-2023.1
Dormann, C. F., Elith, J., Bacher, S., Buchmann, C., Carl, G., Carré, G., et al. (2013). Collinearity: a review of methods to deal with it and a simulation study evaluating their performance. Ecography 36, 27–46. doi: 10.1111/j.1600-0587.2012.07348.x
Duarte, G. A. S., Villela, H. D. M., Deocleciano, M., Silva, D., Barno, A., Cardoso, P. M., et al. (2020). Heat waves are a major threat to turbid coral reefs in Brazil. Front. Mar. Sci. 7:179. doi: 10.3389/fmars.2020.00179
Durante, L. M., Cruz, I. C. S., and Lotufo, T. M. C. (2018). The effect of climate change on the distribution of a tropical zoanthid (Palythoa caribaeorum) and its ecological implications. PeerJ 6:e4777. doi: 10.7717/peerj.4777
Eakin, C. M., Morgan, J. A., Heron, S. F., Smith, T. B., Liu, G., Alvarez-Filip, L., et al. (2010). Caribbean corals in crisis: record thermal stress, bleaching, and mortality in 2005. PLoS One 5:e13969. doi: 10.1371/journal.pone.0013969
Elith, J., Kearney, M., and Phillips, S. (2010). The art of modelling range-shifting species. Methods Ecol. Evol. 1, 330–342. doi: 10.1111/j.2041-210x.2010.00036.x
Elith, J., and Leathwick, J. R. (2009). Species distribution models: ecological explanation and prediction across space and time. Annu. Rev. Ecol. Evol. Syst. 40, 677–697. doi: 10.1146/annurev.ecolsys.110308.120159
Elith, J., Leathwick, J. R., and Hastie, T. (2008). A working guide to boosted regression trees. J. Anim. Ecol. 77, 802–813. doi: 10.1111/j.1365-2656.2008.01390.x
Fabricius, K. E. (2005). Effects of terrestrial runoff on the ecology of corals and coral reefs: review and synthesis. Mar. Pollut. Bull. 50, 125–146. doi: 10.1016/j.marpolbul.2004.11.028
Farber, O., and Kadmon, R. (2003). Assessment of alternative approaches for bioclimatic modeling with special emphasis on the Mahalanobis distance. Ecol. Model. 160, 115–130. doi: 10.1016/S0304-3800(02)00327-7
Feeley, K. J., and Silman, M. R. (2011). Keep collecting: accurate species distribution modelling requires more collections than previously thought. Divers. Distrib. 17, 1132–1140. doi: 10.1111/j.1472-4642.2011.00813.x
Floeter, S. R., Rocha, L. A., Robertson, D. R., Joyeux, J. C., Smith-Vaniz, W. F., Wirtz, P., et al. (2008). Atlantic reef fish biogeography and evolution. J. Biogeogr. 35, 22–47. doi: 10.1111/j.1365-2699.2007.01790.x
Francini-Filho, R. B., Coni, E. O. C., Meirelles, P. M., Amado-Filho, G. M., Thompson, F. L., Pereira-Filho, G. H., et al. (2013). Dynamics of coral reef benthic assemblages of the Abrolhos Bank, eastern Brazil: inferences on natural and anthropogenic drivers. PLoS One 8:e54260. doi: 10.1371/journal.pone.0054260
Fricke, H., and Meischner, D. (1985). Depth limits of Bermudan scleractinian corals: a submersible survey. Mar. Biol. 88, 175–187. doi: 10.1007/BF00397165
García, N. A. C., Campos, J. E., Tello Musi, J. L., Forsman, Z. H., Montero Muñoz, J. L., Reyes, A. M., et al. (2017). Comparative molecular and morphological variation analysis of Siderastrea (Anthozoa, Scleractinia) reveals the presence of Siderastrea stellata in the Gulf of Mexico. Biol. Bull. 232, 58–70. doi: 10.1086/691788
Garzoli, S. L., Gordon, A. L., Kamenkovich, V., Pillsbury, D., and Duncombe-Rae, C. (1996). Variability and sources of the southeastern Atlantic circulation. J. Mar. Res. 54, 1039–1071. doi: 10.1357/0022240963213763
Glynn, P. W. (1996). Coral reef bleaching: facts, hypotheses and implications. Glob. Change Biol. 2, 495–509. doi: 10.1111/j.1365-2486.1996.tb00063.x
Golicher, D., Ford, A., Cayuela, L., and Newton, A. (2012). Pseudo-absences, pseudo-models and pseudo-niches: pitfalls of model selection based on the area under the curve. Int. J. Geogr. Inform. Sci. 26, 2049–2063. doi: 10.1080/13658816.2012.719626
Goodbody-Gringley, G., Marchini, C., Chequer, A. D., and Goffredo, S. (2015). Population structure of Montastraea cavernosa on shallow versus mesophotic reefs in Bermuda. PLoS One 10:e0142427. doi: 10.1371/journal.pone.0142427
Goreau, T. F., and Wells, J. (1967). The shallow-water scleractinia of Jamaica: revised list of species and their vertical distribution range. Bull. Mar. Sci. 17, 442–453.
Graham, N. A. J., and Nash, K. L. (2013). The importance of structural complexity in coral reef ecosystems. Coral Reefs 32, 315–326. doi: 10.1007/s00338-012-0984-y
Guisan, A., Tingley, R., Baumgartner, J. B., Naujokaitis-Lewis, I., Sutcliffe, P. R., Tulloch, A. I. T., et al. (2013). Predicting species distributions for conservation decisions. Ecol. Lett. 16, 1424–1435. doi: 10.1111/ele.12189
Halpern, B. S., Frazier, M., Potapenko, J., Casey, K. S., Koenig, K., Longo, C., et al. (2015). Spatial and temporal changes in cumulative human impacts on the world’s ocean. Nat. Commun. 6:7615. doi: 10.1038/ncomms8615
Hao, T., Elith, J., Guillera-Arroita, G., and Lahoz-Monfort, J. J. (2019). A review of evidence about use and performance of species distribution modelling ensembles like BIOMOD. Divers. Distrib. 25, 839–852. doi: 10.1111/ddi.12892
Hao, T., Elith, J., Lahoz-Monfort, J. J., and Guillera-Arroita, G. (2020). Testing whether ensemble modelling is advantageous for maximising predictive performance of species distribution models. Ecography 43, 549–558. doi: 10.1111/ecog.04890
Hastie, T., and Tibshirani, R. (2014). “Generalized additive models,” in Wiley StatsRef: Statistics Reference Online, eds N. Balakrishnan, T. Colton, B. Everitt, W. Piegorsch, F. Ruggeri, and J. L. Teugels (Chichester: John Wiley & Sons, Ltd). doi: 10.1002/9781118445112.stat03141
Hausfather, Z., and Peters, G. P. (2020). Emissions - the ‘business as usual’ story is misleading. Nature 577, 618–620. doi: 10.1038/d41586-020-00177-3
Hoegh-Guldberg, O. (2011). Coral reef ecosystems and anthropogenic climate change. Reg. Environ. Change 11, 215–227. doi: 10.1007/s10113-010-0189-2
Hoegh-Guldberg, O., Mumby, P. J., Hooten, A. J., Steneck, R. S., Greenfield, P., Gomez, E., et al. (2007). Coral reefs under rapid climate change and ocean acidification. Science 318, 1737–1742. doi: 10.1126/science.1152509
Horta-Puga, G. (2003). Condition of selected reef sites in the Veracruz reef system (stony corals and algae). Atoll Res. Bull. 496, 360–369. doi: 10.5479/si.00775630.20.360
Hughes, T. P. (1994). Catastrophes, phase shifts, and large-scale degradation of a Caribbean coral reef. Science 265, 1547–1551. doi: 10.1126/science.265.5178.1547
Hughes, T. P., Anderson, K. D., Connolly, S. R., Heron, S. F., Kerry, J. T., Lough, J. M., et al. (2018). Spatial and temporal patterns of mass bleaching of corals in the Anthropocene. Science 359, 80–83. doi: 10.1126/science.aan8048
Hughes, T. P., Barnes, M. L., Bellwood, D. R., Cinner, J. E., Cumming, G. S., Jackson, J. B. C. C., et al. (2017). Coral reefs in the Anthropocene. Nature 546, 82–90. doi: 10.1038/nature22901
Hughes, T. P., Rodrigues, M. J., Bellwood, D. R., Ceccarelli, D., Hoegh-Guldberg, O., McCook, L., et al. (2007). Phase shifts, herbivory, and the resilience of coral reefs to climate change. Curr. Biol. 17, 360–365. doi: 10.1016/j.cub.2006.12.049
IPCC (2014). Climate Change 2014: Synthesis Report. Contribution of Working Groups I, II and III to the Fifth Assessment Report of the Intergovernmental Panel on Climate Change. Geneva: IPCC.
Johns, W. E., Lee, T. N., Beardsley, R. C., Candela, J., Limeburner, R., and Castro, B. (1998). Annual cycle and variability of the North Brazil current. J. Phys. Oceanogr. 28, 103–128. doi: 10.1175/1520-04851998028<0103:ACAVOT<2.0.CO;2
Jones, C. G., Lawron, J. H., and Shachak, M. (1997). Positive and negative effects of organisms as physical ecosystem engineers. Ecology 78, 1946–1957.
Jones, C. G., Lawton, J. H., Shachak, M., and Organisms, M. (1994). Organisms as ecosystem engineers. Oikos 69, 373–386.
Kader, G. D., and Perry, M. (2007). Variability for categorical variables. J. Stat. Educ. 15:179. doi: 10.1080/10691898.2007.11889465
Kerry, J. T., Baird, A. H., Connolly, S. R., Dietzel, A., Eakin, C. M., Heron, S. F., et al. (2018). Global warming transforms coral reef assemblages. Nature 556, 492–496.
Kleypas, J. A., McManu, J. W., and Mene, L. A. B. (1999). Environmental limits to coral reef development: where do we draw the line? Am. Zool. 39, 146–159. doi: 10.1093/icb/39.1.146
Kristensen, E. (2008). Mangrove crabs as ecosystem engineers; with emphasis on sediment processes. J. Sea Res. 59, 30–43. doi: 10.1016/j.seares.2007.05.004
Kubicek, A., Breckling, B., Hoegh-Guldberg, O., and Reuter, H. (2019). Climate change drives trait-shifts in coral reef communities. Sci. Rep. 9:3721. doi: 10.1038/s41598-019-38962-4
Laborel, J. (1970). Les peuplements de madréporaires des côtes tropicales du Brésil. Ann. Univ. Abidjan (Ser. E) 2, 1–260.
Laborel, J. (1974). West African reef corals: an hypothesis on their origin. Proc. Second Int. Symp. Coral Reefs 1, 425–443.
Leão, Z. M. A. N., Kikuchi, R., and Testa, V. (2003). “Corals and coral reefs of Brazil,” in Latin American Coral Reefs, ed. J. Cortés (Amsterdam: Elsevier Science), 9–52. doi: 10.1016/B978-0-444-51388-5.50003-5
Leão, Z. M. A. N., and Kikuchi, R. K. P. (2005). A relic coral fauna threatened by global changes and human activities, Eastern Brazil. Mar. Pollut. Bull. 51, 599–611. doi: 10.1016/j.marpolbul.2005.04.024
Leão, Z. M. A. N., Kikuchi, R. K. P., Ferreira, B. P., Neves, E. G., Sovierzoski, H. H., Oliveira, M. D. M., et al. (2016). Brazilian coral reefs in a period of global change: a synthesis. Braz. J. Oceanogr. 64, 97–116. doi: 10.1590/S1679-875920160916064sp2
Lima-Ribeiro, M. S. (2015). EcoClimate: a database of climate data from multiple models for past, present, and future for macroecologists and biogeographers. Biodivers. Inform. 10, 1–21. doi: 10.17161/bi.v10i0.4955
Liu, C., Newell, G., and White, M. (2019). The effect of sample size on the accuracy of species distribution models: considering both presences and pseudo-absences or background sites. Ecography 42, 535–548. doi: 10.1111/ecog.03188
Lobo, J. M., Jiménez-Valverde, A., and Hortal, J. (2010). The uncertain nature of absences and their importance in species distribution modelling. Ecography 33, 103–114. doi: 10.1111/j.1600-0587.2009.06039.x
Maia, H. A., Morais, R. A., Quimbayo, J. P., Dias, M. S., Sampaio, C. L. S., Horta, P. A., et al. (2018). Spatial patterns and drivers of fish and benthic reef communities at São Tomé Island, Tropical Eastern Atlantic. Mar. Ecol. 39:e12520. doi: 10.1111/maec.12520
Marmion, M., Parviainen, M., Luoto, M., Heikkinen, R. K., and Thuiller, W. (2009). Evaluation of consensus methods in predictive species distribution modelling. Divers. Distrib. 15, 59–69. doi: 10.1111/j.1472-4642.2008.00491.x
McCullagh, P. (1984). Generalized linear models. Eur. J. Oper. Res. 16, 285–292. doi: 10.1016/0377-2217(84)90282-0
Menezes, N., Neves, E., Kikuchi, R. K. P., and Johnsson, R. (2014). Morphological variation in the atlantic genus Siderastrea (Anthozoa, Scleractinia). Pap. Avulsos Zool. 54, 199–208. doi: 10.1590/0031-1049.2014.54.16
Mies, M., Francini-Filho, R. B., Zilberberg, C., Garrido, A. G., Longo, G. O., Laurentino, E., et al. (2020). South Atlantic coral reefs are major global warming refugia and less susceptible to bleaching. Front. Mar. Sci. 7:514. doi: 10.3389/FMARS.2020.00514
Miller, M. W., Bourque, A. S., and Bohnsack, J. A. (2002). An analysis of the loss of acroporid corals at Looe Key, Florida, USA: 1983-2000. Coral Reefs 21, 179–182. doi: 10.1007/s00338-002-0228-7
Morais, J., and Santos, B. A. (2018). Limited potential of deep reefs to serve as refuges for tropical Southwestern Atlantic corals. Ecosphere 9:e02281. doi: 10.1002/ecs2.2281
Morais, R. A., Depczynski, M., Fulton, C., Marnane, M., Narvaez, P., Huertas, V., et al. (2020). Severe coral loss shifts energetic dynamics on a coral reef. Funct. Ecol. 34, 1507–1518. doi: 10.1111/1365-2435.13568
Morato, T., González-Irusta, J. M., Dominguez-Carrió, C., Wei, C. L., Davies, A., Sweetman, A. K., et al. (2020). Climate-induced changes in the suitable habitat of cold-water corals and commercially important deep-sea fishes in the North Atlantic. Glob. Chang. Biol. 26, 2181–2202. doi: 10.1111/gcb.14996
Naimi, B., Hamm, N. A. S., Groen, T. A., Skidmore, A. K., and Toxopeus, A. G. (2014). Where is positional uncertainty a problem for species distribution modelling? Ecography 37, 191–203. doi: 10.1111/j.1600-0587.2013.00205.x
Norström, A. V., Nyström, M., Lokrantz, J., and Folke, C. (2009). Alternative states on coral reefs: beyond coral-macroalgal phase shifts. Mar. Ecol. Prog. Ser. 376, 293–306. doi: 10.3354/meps07815
Nunes, F., Fukami, H., Vollmer, S. V., Norris, R. D., and Knowlton, N. (2008). Re-evaluation of the systematics of the endemic corals of Brazil by molecular data. Coral Reefs 27, 423–432. doi: 10.1007/s00338-007-0349-0
Nunes, F. L. D., Norris, R. D., and Knowlton, N. (2011). Long distance dispersal and connectivity in Amphi-Atlantic corals at regional and basin scales. PLoS One 6:e22298. doi: 10.1371/journal.pone.0022298
Oigman-Pszczol, S. S., and Creed, J. C. (2004). Size structure and spatial distribution of the corals Mussismilia hispida and Siderastrea stellata (Scleractinia) at Armação dos Búzios, Brazil. Bull. Mar. Sci. 74, 433–448.
Pearson, R. G., and Dawson, T. P. (2003). Predicting the impacts of climate change on the distribution of species: are bioclimate envelope models useful? Glob. Ecol. Biogeogr. 12, 361–371. doi: 10.1046/j.1466-822X.2003.00042.x
Pearson, R. G., Thuiller, W., Araújo, M. B., Martinez-Meyer, E., Brotons, L., McClean, C., et al. (2006). Model-based uncertainty in species range prediction. J. Biogeogr. 33, 1704–1711. doi: 10.1111/j.1365-2699.2006.01460.x
Peluso, L., Tascheri, V., Nunes, F. L. D., Castro, C. B., Pires, D. O., and Zilberberg, C. (2018). Contemporary and historical oceanographic processes explain genetic connectivity in a Southwestern Atlantic coral. Sci. Rep. 8:2684. doi: 10.1038/s41598-018-21010-y
Perry, C. T., and Alvarez-Filip, L. (2019). Changing geo-ecological functions of coral reefs in the Anthropocene. Funct. Ecol. 33, 976–988. doi: 10.1111/1365-2435.13247
Perry, C. T., Spencer, T., and Kench, P. S. (2008). Carbonate budgets and reef production states: a geomorphic perspective on the ecological phase-shift concept. Coral Reefs 27, 853–866. doi: 10.1007/s00338-008-0418-z
Pires, D. O., Castro, C. B., and Ratto, C. C. (1999). Reef coral reproduction in the Abrolhos Reef Complex, Brazil: the endemic genus Mussismilia. Mar. Biol. 135, 463–471. doi: 10.1007/s002270050646
Polidoro, B. A., Ralph, G. M., Strongin, K., Harvey, M., Carpenter, K. E., Arnold, R., et al. (2017). The status of marine biodiversity in the Eastern Central Atlantic (West and Central Africa). Aquat. Conserv. Mar. Freshw. Ecosyst. 27, 1021–1034. doi: 10.1002/aqc.2744
Porter, J. W. (1972). Patterns of species diversity in Caribbean reef corals. Ecology 53, 745–748. doi: 10.2307/1934796
R Core Team (2019). R: A Language and Environment for Statistical Com? Puting. Vienna: R Foundation for Statistical Computing.
Richardson, L. E., Graham, N. A. J., and Hoey, A. S. (2020). Coral species composition drives key ecosystem function on coral reefs. Proc. R. Soc. B Biol. Sci. 287:20192214. doi: 10.1098/rspb.2019.2214
Richardson, L. E., Graham, N. A. J., Pratchett, M. S., and Hoey, A. S. (2017). Structural complexity mediates functional structure of reef fish assemblages among coral habitats. Environ. Biol. Fishes 100, 193–207. doi: 10.1007/s10641-016-0571-0
Rivera, H., Chan, A., and Luu, V. (2020). Coral reefs are critical for our food supply, tourism, and ocean health. We can protect them from climate change. MIT Sci. Policy Rev. 1, 18–33. doi: 10.38105/spr.7vn798jnsk
Roberts, C. M., McClean, C. J., Veron, J. E. N., Hawkins, J. P., Allen, G. R., McAllister, D. E., et al. (2002). Marine biodiversity hotspots and conservation priorities for tropical reefs. Science 295, 1280–1284. doi: 10.1126/science.1067728
Roberts, D. R., Bahn, V., Ciuti, S., Boyce, M. S., Elith, J., Guillera-Arroita, G., et al. (2017). Cross-validation strategies for data with temporal, spatial, hierarchical, or phylogenetic structure. Ecography 40, 913–929. doi: 10.1111/ecog.02881
Robinson, N. M., Nelson, W. A., Costello, M. J., Sutherland, J. E., and Lundquist, C. J. (2017). A systematic review of marine-based species distribution models (SDMs) with recommendations for best practice. Front. Mar. Sci. 4:421. doi: 10.3389/fmars.2017.00421
Rodriguez, L., Martínez, B., and Tuya, F. (2019). Atlantic corals under climate change: modelling distribution shifts to predict richness, phylogenetic structure and trait-diversity changes. Biodivers. Conserv. 28, 3873–3890. doi: 10.1007/s10531-019-01855-z
Sánchez-Quinto, A., da Costa, J. C., Zamboni, N. S., Sanches, F. H. C., Principe, S. C., Viotto, E. V., et al. (2020). Development of a conceptual framework for the management of biodiversity and ecosystem services in the Mexican Caribbean. Biota Neotrop. 20 (Suppl. 1), 1–18. doi: 10.1590/1676-0611-bn-2019-0901
Schickele, A., Leroy, B., Beaugrand, G., Goberville, E., Hattab, T., Francour, P., et al. (2020). Modelling European small pelagic fish distribution: methodological insights. Ecol. Model. 416:108902. doi: 10.1016/j.ecolmodel.2019.108902
Senay, S. D., Worner, S. P., and Ikeda, T. (2013). Novel three-step pseudo-absence selection technique for improved species distribution modelling. PLoS One 8:e71218. doi: 10.1371/journal.pone.0071218
Shantz, A. A., Ladd, M. C., and Burkepile, D. E. (2020). Overfishing and the ecological impacts of extirpating large parrotfish from Caribbean coral reefs. Ecol. Monogr. 90:e01403. doi: 10.1002/ecm.1403
Silveira, I. C. A., Schmidt, A. C. K., Campos, E. J. D., de Godoi, S. S., and Ikeda, Y. (2000). A corrente do Brasil ao largo da costa leste brasileira. Rev. Bras. Oceanogr. 48, 171–183. doi: 10.1590/s1413-77392000000200008
Skirving, W. J., Heron, S. F., Marsh, B. L., Liu, G., De La Cour, J. L., Geiger, E. F., et al. (2019). The relentless march of mass coral bleaching: a global perspective of changing heat stress. Coral Reefs 38, 547–557. doi: 10.1007/s00338-019-01799-4
Soares, M. D. O., Rabelo, E. F., and Mathews-cascon, H. (2011). Intertidal anthozoans from the coast of Ceará (Brazil). Braz. J. Biosci. 9, 437–443.
Spalding, M. D., Fox, H. E., Allen, G. R., Davidson, N., Ferdaña, Z. A., Finlayson, M., et al. (2007). Marine ecoregions of the world: a bioregionalization of coastal and shelf areas. Bioscience 57, 573–583. doi: 10.1641/B570707
Takao, S., Yamano, H., Sugihara, K., Kumagai, N. H., Fujii, M., and Yamanaka, Y. (2015). An improved estimation of the poleward expansion of coral habitats based on the inter-annual variation of sea surface temperatures. Coral Reefs 34, 1125–1137. doi: 10.1007/s00338-015-1347-2
Teagle, H., and Smale, D. A. (2018). Climate-driven substitution of habitat-forming species leads to reduced biodiversity within a temperate marine community. Divers. Distrib. 24, 1367–1380. doi: 10.1111/ddi.12775
Tews, J., Brose, U., Grimm, V., Tielbörger, K., Wichmann, M. C., Schwager, M., et al. (2004). Animal species diversity driven by habitat heterogeneity/diversity: the importance of keystone structures. J. Biogeogr. 31, 79–92. doi: 10.1046/j.0305-0270.2003.00994.x
Thuiller, W., Lafourcade, B., Engler, R., and Araújo, M. B. (2009). BIOMOD - a platform for ensemble forecasting of species distributions. Ecography 32, 369–373. doi: 10.1111/j.1600-0587.2008.05742.x
Valavi, R., Elith, J., Lahoz-Monfort, J. J., and Guillera-Arroita, G. (2019). blockCV: an r package for generating spatially or environmentally separated folds for k-fold cross-validation of species distribution models. Methods Ecol. Evol. 10, 225–232. doi: 10.1111/2041-210X.13107
Veazey, L., Williams, O., Wade, R., Toonen, R., and Spalding, H. L. (2019). Present-day distribution and potential spread of the invasive green alga Avrainvillea amadelpha around the main Hawaiian Islands. Front. Mar. Sci. 6:402. doi: 10.3389/fmars.2019.00402
Verbruggen, H., Tyberghein, L., Belton, G. S., Mineur, F., Jueterbock, A., Hoarau, G., et al. (2013). Improving transferability of introduced species’ distribution models: new tools to forecast the spread of a highly invasive seaweed. PLoS One 8:e68337. doi: 10.1371/journal.pone.0068337
Vergés, A., McCosker, E., Mayer-Pinto, M., Coleman, M. A., Wernberg, T., Ainsworth, T., et al. (2019). Tropicalisation of temperate reefs: implications for ecosystem functions and management actions. Funct. Ecol. 33, 1000–1013. doi: 10.1111/1365-2435.13310
Vila-Nova, D. A., Ferreira, C. E. L., Barbosa, F. G., and Floeter, S. R. (2014). Reef fish hotspots as surrogates for marine conservation in the Brazilian coast. Ocean Coast. Manag. 102, 88–93. doi: 10.1016/j.ocecoaman.2014.09.005
Warfe, D. M., and Barmuta, L. A. (2006). Habitat structural complexity mediates food web dynamics in a freshwater macrophyte community. Oecologia 150, 141–154. doi: 10.1007/s00442-006-0505-1
Weatherall, P., Marks, K. M., Jakobsson, M., Schmitt, T., Tani, S., Arndt, J. E., et al. (2015). A new digital bathymetric model of the world’s oceans. Earth Space Sci. 2, 331–345. doi: 10.1002/2015EA000107
Wenger, S. J., and Olden, J. D. (2012). Assessing transferability of ecological models: an underappreciated aspect of statistical validation. Methods Ecol. Evol. 3, 260–267. doi: 10.1111/j.2041-210X.2011.00170.x
Wiens, J. A., Stralberg, D., Jongsomjit, D., Howell, C. A., and Snyder, M. A. (2009). Niches, models, and climate change: assessing the assumptions and uncertainties. Proc. Natl. Acad. Sci. U.S.A. 106, 19729–19736. doi: 10.1073/pnas.0901639106
Williams, G. J., and Graham, N. A. J. (2019). Rethinking coral reef functional futures. Funct. Ecol. 33, 942–947. doi: 10.1111/1365-2435.13374
Woodhead, A. J., Hicks, C. C., Norström, A. V., Williams, G. J., and Graham, N. A. J. (2019). Coral reef ecosystem services in the Anthropocene. Funct. Ecol. 33, 1023–1034. doi: 10.1111/1365-2435.13331
Yamano, H., Sugihara, K., and Nomura, K. (2011). Rapid poleward range expansion of tropical reef corals in response to rising sea surface temperatures. Geophys. Res. Lett. 38:L04601. doi: 10.1029/2010GL046474
Zanardo, N., Parra, G. J., Passadore, C., and Möller, L. M. (2017). Ensemble modelling of southern Australian bottlenose dolphin Tursiops sp. distribution reveals important habitats and their potential ecological function. Mar. Ecol. Prog. Ser. 569, 253–266. doi: 10.3354/meps12091
Keywords: corals, niche models, habitat complexity, global warming, Anthropocene, foundation species
Citation: Principe SC, Acosta AL, Andrade JE and Lotufo TMC (2021) Predicted Shifts in the Distributions of Atlantic Reef-Building Corals in the Face of Climate Change. Front. Mar. Sci. 8:673086. doi: 10.3389/fmars.2021.673086
Received: 26 February 2021; Accepted: 21 June 2021;
Published: 19 July 2021.
Edited by:
Verena Schoepf, University of Amsterdam, NetherlandsReviewed by:
Gengping Zhu, Tianjin Normal University, ChinaJustin H. Baumann, Bowdoin College, United States
Copyright © 2021 Principe, Acosta, Andrade and Lotufo. This is an open-access article distributed under the terms of the Creative Commons Attribution License (CC BY). The use, distribution or reproduction in other forums is permitted, provided the original author(s) and the copyright owner(s) are credited and that the original publication in this journal is cited, in accordance with accepted academic practice. No use, distribution or reproduction is permitted which does not comply with these terms.
*Correspondence: Silas C. Principe, silasprincipe@yahoo.com.br