- 1School of Marine Science and Ocean Engineering, University of New Hampshire, Durham, NH, United States
- 2Center for Acoustics Research and Education, Institute for the Study of Earth, Oceans, and Space, University of New Hampshire, Durham, NH, United States
- 3Center for Coastal and Ocean Mapping, University of New Hampshire, Durham, CA, United States
- 4JASCO Applied Sciences (Canada) Ltd., Victoria, BC, Canada
- 5Department of Biological Sciences, College of Life Sciences and Agriculture, University of New Hampshire, Durham, NH, United States
A methodology for the analysis of soundscapes was developed in an attempt to facilitate efficient and accurate soundscape comparisons across time and space. The methodology consists of a collection of traditional soundscape metrics, statistical measures, and acoustic indices that were selected to quantify several salient properties of marine soundscapes: amplitude, impulsiveness, periodicity, and uniformity. The metrics were calculated over approximately 30 h of semi-continuous passive acoustic data gathered in seven unique acoustic environments. The resultant metric values were compared to a priori descriptions and cross-examined statistically to determine which combination most effectively captured the characteristics of the representative soundscapes. The best measures of amplitude, impulsiveness, periodicity, and uniformity were determined to be SPLrms and SPLpk for amplitude, kurtosis for impulsiveness, an autocorrelation based metric for periodicity, and the Dissimilarity index for uniformity. The metrics were combined to form the proposed “Soundscape Code,” which allows for rapid multidimensional and direct comparisons of salient soundscape properties across time and space. This initial characterization will aid in directing further analyses and guiding subsequent assessments to understand soundscape dynamics.
Introduction
Ocean sound conveys a wealth of information due to the highly efficient manner in which acoustic energy travels through the water. Studying ambient ocean sound provides information on vocalizing marine life, ocean dynamics, and human use of the ocean (Hildebrand, 2009; Pijanowski et al., 2011; Howe et al., 2019). In recognition of its inherent value, ocean sound has been recently accepted as an Essential Ocean Variable (EOV) by the Global Ocean Observing System (GOOS) Biology and Ecosystem Panel (Ocean Sound EOV, 2018). EOVs are approved based on three considerations: (1) relevance in helping solve scientific questions and addressing societal needs, (2) contributions to improving marine resource management, and (3) feasibility for global observation regarding cost effectiveness, technology, and human capabilities1. In the context of ocean sound, inclusion in the GOOS framework provides a formal structure for recording ocean sound. Implementation of the ocean sound EOV will help to guide scientific data collection to ensure consistency and appropriate comparisons in soundscape analysis and ocean sound studies.
A soundscape is an acoustic environment tied to the function of a given landscape or marine habitat, and it is the sum of all sounds present; ISO 18405 defines soundscape as the characterization of the ambient sound in terms of its spatial, temporal, frequency attributes, and the types of sources contributing to the sound field. Defining and characterizing the soundscape is an important step in the task of assessing, monitoring, and comparing global acoustic environments. By utilizing soundscape analysis and ocean sound, researchers can better understand ocean dynamics (Radford et al., 2010; McWilliam and Hawkins, 2013; Miksis-Olds et al., 2013; Staaterman et al., 2014), biodiversity and ecosystem health (Parks et al., 2014; Staaterman et al., 2014), and the risk of anthropogenic impacts on marine life (Weilgart, 2007; Carroll et al., 2017).
Traditionally, sound is analyzed by measuring the sound pressure level (Sound Pressure Level; SPL), and other source- and amplitude-related parameters such as the number of sources detected, source classification, localization of detectable sources, or sound exposure level (SEL) (Martin et al., 2019). Recently, researchers have developed and applied metrics mathematically summarizing acoustic properties and comparing them with independent ecological data to understand the types of sources present in a soundscape. For example, the Acoustic Complexity Index (ACI) was proposed as a proxy for biodiversity Sueur et al. (2008), and (Pieretti et al., 2011) demonstrated the efficacy of the Entropy Index (H-index) and the Dissimilarity Index (D-index) at highlighting biodiversity of a terrestrial environment. Application of acoustic biodiversity indices in a marine environment have yielded mixed results (Parks et al., 2014; Staaterman et al., 2017; Bohnenstiehl et al., 2018; Bolgan et al., 2018). Further investigation into the utility of acoustic indices in marine applications is needed to assess their efficacy.
Even though ocean ambient sound and soundscape research has been conducted for decades, the ocean community has still not reached a consensus on the optimal way to accurately report and compare important aspects of ocean sound. Ocean sound studies are not trivial endeavors, and the complexity of ocean sound dynamics, combined with a lack of formal standards, guidelines, and consistent methods, make soundscape analyses and meaningful comparisons difficult. The methodologies utilized by researchers are often tailored to a specific study, which focuses on answering the question at hand, but contributes little to the understanding of soundscape dynamics on a large regional or global scale if the results cannot be easily interpreted or compared to data from other areas. Studies often fail to clearly report metric input parameters critical to the determination of the final metric value; ambiguities in reporting can make replicating study methodologies difficult, and can lead to erroneous comparisons. For example, different methods of averaging have yielded differences in final metric results of over 10 dB in previous works (Merchant et al., 2012; Hawkins et al., 2014). Some methodology descriptions are so vague it is nearly impossible to determine averaging times, integration windows, and exactly which metric is being calculated (McKenna et al., 2016). To accurately report important soundscape information, efforts must be made to standardize the way in which researchers acquire, process, analyze, and report acoustic metrics.
Many analysis methods produce graphical outputs, which are assessed visually but can become cumbersome when many comparisons need to be quantitative across time or space. Graphical information, supplemented with standardized quantitative analysis of the multidimensional soundscape within an accepted framework would produce thorough, accurate, and easily comparable results for acoustic recordings. A method that resembles this type of standardized quantitative analysis was adopted by The World Meteorology Organization (WMO) for comparing and reporting the state of sea ice that encompasses multidimensional information: ice coverage, stage of development, age, thickness and form. The WMO system for reporting sea ice is commonly referred to as the “egg code” (JCOMM Expert Team on Sea Ice, 2014). This egg code presents standard ice characteristics in a clear and succinct manner, and the multidimensional nature of the egg code reports a variety of relevant ice properties; “one size fits all” measures are rarely adequate in describing dynamic environments (Figure 1). The idea of a “measure” like the egg code that captures and reports salient information about an environment is the inspiration for the proposed soundscape code. While the egg code reports multiple dimensions of the environmental “feature” ice, the proposed soundscape code reports multiple dimensions of the environmental “feature,” sound pressure.
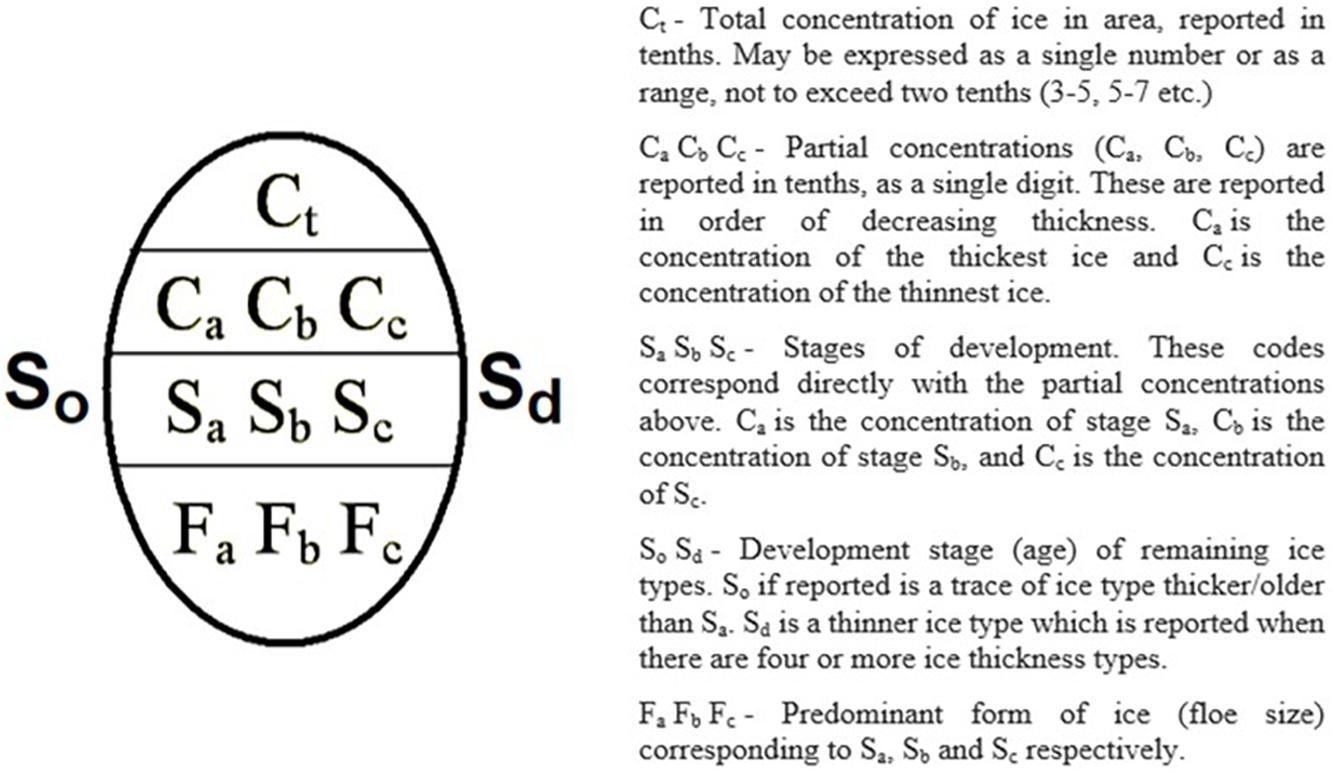
Figure 1. WMO egg code (JCOMM Expert Team on Sea Ice, 2014). Contained in the simple oval are data regarding concentrations, stages of development, and form of ice. Code conforms to an international convention.
Amplitude, periodicity, impulsiveness, and uniformity are physical soundscape properties that are important to understanding soundscapes and the distribution of sound energy across time, space, and frequency (Table 1). The objective of this study was to identify the optimal suite of metrics across the general soundscape properties (amplitude, impulsiveness, periodicity, and uniformity) to create a soundscape code infrastructure for comparing soundscapes. Multiple metrics within each soundscape property (Table 2) were selected and applied to a diverse set of soundscapes to identify the metric that best captured the salient aspects of the acoustic recordings. Comparing the acoustic properties of soundscapes is not meant to be an exhaustive assessment, but rather an initial analysis to understand some of the dynamics of acoustic environments and guide subsequent analysis for more targeted assessments. The resulting product forms the proposed soundscape code, which provides a framework for comparing soundscape properties across space and time utilizing metrics that capture spectral and temporal properties of acoustic environments; characterizing acoustic environments in terms of spatial, spectral, and temporal acoustic properties directly relates to the ISO 18405 definition of a soundscape.
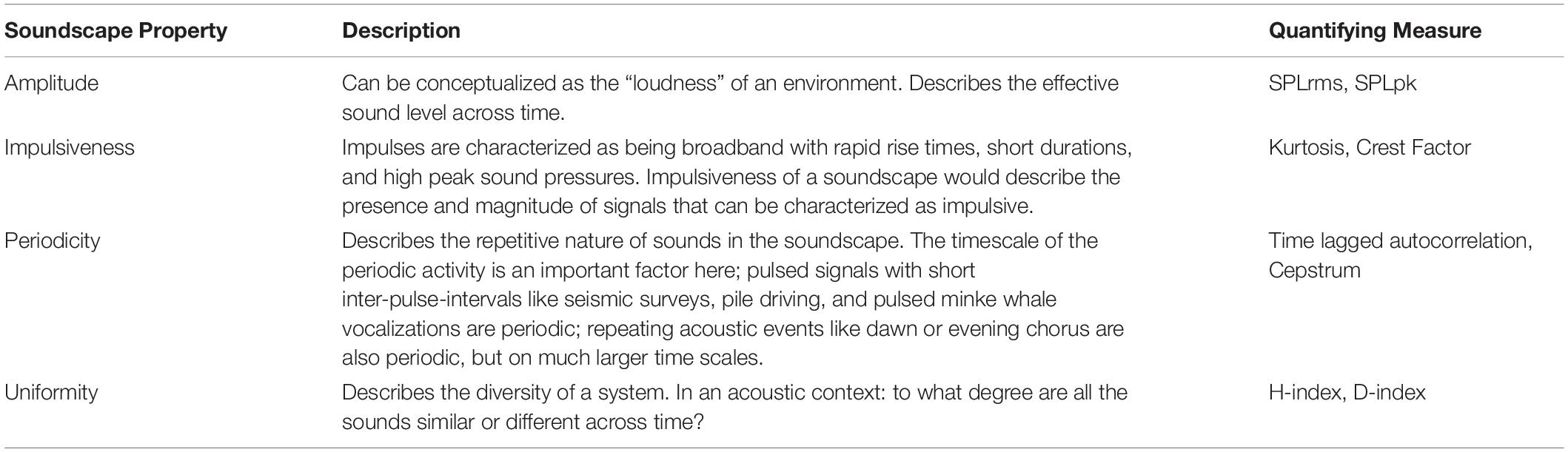
Table 2. Soundscape properties and corresponding metrics, statistical measures, and indices investigated for inclusion in the soundscape code.
Sound level statistics and measures of the amplitude of acoustic power and energy are used frequently in ocean sound studies. The root-mean-square (rms) SPL captures the average sound pressure amplitude of the corresponding environment (SPLrms). Though susceptible to upward bias from loud, intermittent sounds, SPLrms is the most ubiquitous acoustic metric (Merchant et al., 2015). SPLrms does not capture all the important amplitude information of a soundscape such as maximum sound pressure levels (SPLpk), the sound floor (quietest periods in a soundscape), or sound exposure level. Reporting both the SPLrms and SPLpk provides detail on average sound amplitude as well as information on the range of sound amplitude.
Impulsive sounds are defined qualitatively as sounds that are of short duration, have rapid rise times, and high sound levels (NIOSH, 1998; NMFS, 2018). A wide variety of sound sources produce pulsed acoustic signatures which means that it is possible for the impulsiveness of a soundscape to be used as an indication of source presence/absence. Impulsive sounds can potentially have physiological impacts on fish (Halvorsen et al., 2012a, b; Casper et al., 2013a, b), and marine mammals (Lucke et al., 2009; Kastelein et al., 2015; Southall et al., 2019), so it is also a valuable property to consider from a regulatory perspective as well as a physical characteristic. Regulations lack quantitative definitions regarding the difference between impulsive and non-impulsive sounds, but several metrics for quantifying impulsiveness have been suggested including kurtosis, crest factor and Harris impulse factor (Erdreich, 1986; Starck and Pekkarinen, 1987; Kastelein et al., 2017). All three were initially considered candidate metrics to represent impulsiveness in the soundscape code, but Harris impulse factor was removed from consideration due to constraint in the range of the metric and the resulting implications for future use in comparative analysis.
The term periodicity is inherently general; periodicity can refer to a pattern that repeats over the course of a year, month, day, hour, or second. Seismic airgun signals (Greene and Richardson, 1988), echolocation clicks (Clarke et al., 2019), pulsed fish or whale vocalizations (Watkins et al., 1987; Lobel, 1992), and the rhythmic rasping of the California spiny lobster (Patek et al., 2009) are examples of real world ocean signals that are periodic. The proposed soundscape code focuses on periodicities that (1) impose physical characteristics to a soundscape over short time periods, (2) occur on time scales of less than a minute, and (3) can be captured by metrics calculated over a single minute of acoustic data. A metric for capturing larger scale periodicity related to diel, season, or annual cycles was not explored in this project but could be assessed using a time series of the individual soundscape code parameters. To our knowledge no metric designed specifically for quantifying the content of periodic signals in an acoustic environment exists, so metrics from other fields were repurposed as candidates to represent the periodicity property in the soundscape code. Cepstrum was first proposed as a tool for analyzing periodic seismological data (Bogert et al., 1963), where the arrival of various waves and phases could be considered as distorted echoes. Cepstrum is not widely used in marine soundscape studies, but has been used with efficacy in a variety of mechanical analyses, and it is considered underutilized by those that use it (Randall, 2017). Time lagged autocorrelation has been used to characterize soundscapes in terms of the dominant source types (Martin et al., 2019), and was repurposed in this study to quantify the content of periodic signals detected in a soundscape.
Soundscape uniformity is the degree to which the signals change over time in terms of temporal and frequency attributes of the soundscape. It answers the question “to what degree are the sounds similar or different?” and describes the dynamic nature of a given soundscape. The inclusion of the uniformity property in the soundscape code was motivated by the widespread interest in biodiversity, and the use of passive acoustic monitoring techniques to study biodiversity remotely (Peet, 1974; Pimm and Lawton, 1998; Sueur et al., 2014). A suite of quantitative indices has been developed and geared toward quantifying different properties of acoustic environments: Acoustic Complexity Index (ACI), H-index, D-index, and Acoustic Richness (AR). These indices have been widely used in terrestrial acoustic studies to measure biodiversity and species richness (Sueur et al., 2008, 2014; Pieretti et al., 2011, 2017). For the D-index, Sueur et al. (2008) utilized a measure that estimated the compositional dissimilarity between two communities. Within this work, the D-index is applied to two consecutive acoustic recordings in an effort to capture the acoustic differences and measure the acoustic uniformity. The Entropy Index (H) has been used as a proxy for biodiversity in the marine environment with mixed results (Parks et al., 2014; Harris et al., 2016). Harris et al. (2016) found that H values exhibited a dependence on the size of the fast Fourier transform (FFT) window, and at a window length of 512 showed little correlation to typical diversity measures, but correlation increased with spectral resolution. Parks et al. (2014) had to remove noise from a seismic survey before finding a significant connection between the H index and sampled biodiversity. Because H-index was designed to increase with signal diversity in time and frequency, it was repurposed in this study to represent acoustic uniformity, which shares similarities with the principle of acoustic diversity that the metric was built on.
Materials and Methods
The datasets used to assess the performance of the candidate metrics for use in the soundscape code were selected from a pool of passive acoustic data that had already been analyzed, and in some cases, used in publications (Martin et al., 2017, 2019, 2020; Martin and Barclay, 2019). Soundscape code datasets were picked based on previous knowledge of activity in the soundscape region. Digital passive acoustic data were converted to pressure data, and then metrics were calculated over each pressure time series. The metrics were analyzed to determine the optimal combination for capturing salient quantitative aspects of a soundscape. Each dataset was collected using Autonomous Multi-channel Acoustic Recorders (AMAR, JASCO Applied Sciences) that sampled at a variety of sample rates and durations (Table 3). Recorders were deployed intermittently between 2012 and 2016 at the seven different locations. While the sites may not all be unique in their location, the acoustic content of their recordings was unique; GB5 is actually about 70 km from GB4v35 and GB4v0, and while the latter two share the same site location designation, the datasets were recorded weeks apart. The seven data sets contain a variety of human-generated, natural biologic, and natural abiotic sounds including sounds from a seismic survey, impact pile driving, vessel passages, ice calving and icebergs, fin (Balaenoptera physalus) and humpback whale (Megaptera novaeangliae) vocalizations, northern bottlenose whale (Hyperoodon ampullatus) and common dolphin (Delphinus delphis) whistles and echolocation, and shallow-water reef sounds including foraging urchins, snapping shrimp, and fish grunts (Figure 2). The biological sounds present in the data sets are representative of the diversity of marine life and sounds produced ocean wide.
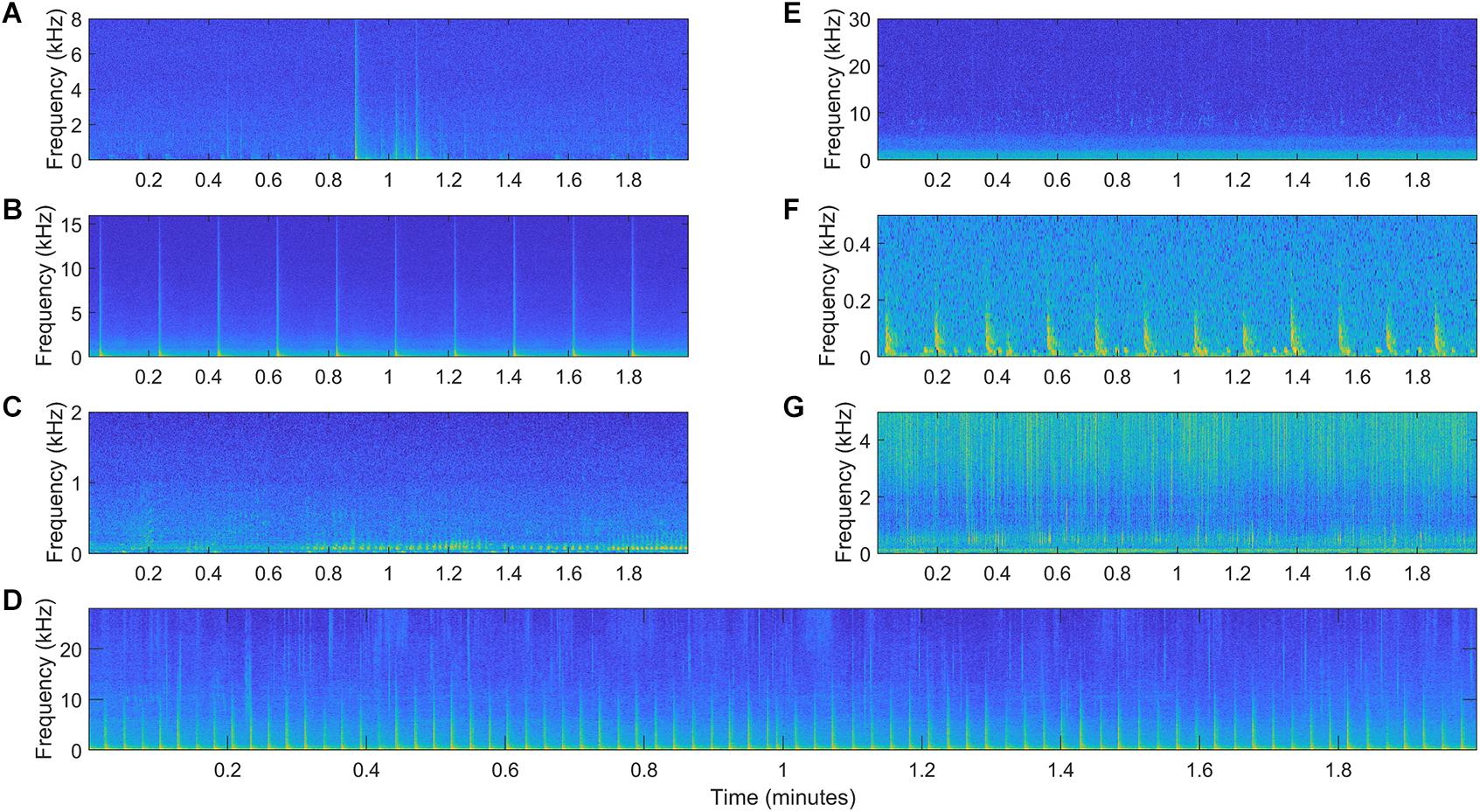
Figure 2. Signals detected at designated soundscape code dataset sites (A) Ice sounds, (B) seismic survey, (C) humpback and fin whale vocalizations, (D) impact pile driving, (E) northern bottlenose whale and common dolphin vocalizations in a quiet soundscape, (F) fin whale vocalizations, (G) reef sounds.
Data Processing
Five frequency bands were selected for soundscape code analysis: (1) 10–100 Hz (Low), (2) 100–1,000 Hz (Mid), (3) 1–10 kHz (High), (4) 10 kHz and above (Ultra-High), and (5) 10 Hz and above (broadband; BB). These frequency bands were chosen because the dominant frequencies of many signals can be isolated into a single soundscape code frequency band. Data from Biogully East (BGE) was low pass filtered with a passband out to 32 kHz to provide a uniform analysis in the Ultra-High band across Melville Bay (MB), Great Barrier Reef (GBR), Orsted (OR), and BGE. Sample rate restrictions precluded analysis of the Ultra-High band at the Grand Banks sites (GB4v0, GB4v35, and GB5). The high band at GB5 was included, even though the data could only be resolved up to 8 kHz due to the sample rate at this site (16 kHz) (Table 2).
The metrics assessed for the soundscape code were calculated over one-min time windows. The one-min time window is a standard time length in soundscape analysis and corresponds with the human auditory experience (Ainslie et al., 2018). All FFTs performed in calculating soundscape code metrics used 1-second time windows. The median and 95% confidence interval of each metric was reported for each site and analyzed. Acorr2, acorr3, SPLrms, SPLpk, kurtosis, crest factor, D-index, and H-index were calculated using custom code written in MATLAB (2019); The Mathworks Inc., Natick, MA, United States.
Sound pressure level (SPL), reported in logarithmic decibel (dB) units relative to a reference pressure of 1 μPa, is the most common amplitude metric reported in ocean sound studies (Equation 1)
where Pref is reference pressure, p(t) is the instantaneous pressure at time (t), and T is the analysis window duration (Madsen, 2005; Thompson et al., 2013; Merchant et al., 2015). The SPLpk has added value as an amplitude metric, as it is also a relevant measure in determining the risk of physical damage in auditory systems (Coles et al., 1968) (Equation 2).
Because the SPLpk and SPLrms metrics were identified from previously published work as well-established and effective measures of the amplitude of sound pressure, they were selected for use in the soundscape code without further analysis (Madsen, 2005; Thompson et al., 2013; Merchant et al., 2015).
The crest factor is defined as the difference, in dB, between the SPLpk and the time averaged sound pressure level. It describes the ratio of the SPLpk relative to the effective pressure level (Equation 3):
A crest factor of 1 indicates no peak, while large-valued crest factors indicate the presence of large peaks. This metric has been used in predicting auditory injury in industrial workers by utilizing A-weighted sound levels where a crest factor value of 15 dB or greater indicated dangerous impulse noises (Starck and Pekkarinen, 1987).
Kurtosis describes the shape of a probability distribution and is a measure of the “tailedness” of the probability distribution of a real valued random variable. Kurtosis is defined below for the pressure time series p(t) as (Equations 4-6):
where is the mean pressure. Proposed as an indicator of the impulsiveness of sounds by Erdreich (1986) for noise exposures with equal spectral energy, permanent threshold shift (PTS) was found to increase with kurtosis up to a value of 40 (Qiu et al., 2013); this value of 40 now represents the threshold above which signals are considered impulsive. Gaussian-distributed random noise produces kurtosis values of 3.0. Time series with strong sinusoidal signals have a kurtosis in the range of 0.0 to 3.0, and time series with transients produce kurtosis values above 3.0 (Martin et al., 2020).
Cepstrum treats the log spectrum of a time series as a waveform, and the spectrum of this log spectrum produces peaks when the original waveform contains echoes, or periodic components (Oppenheim and Schafer, 2004). Cepstrum is calculated by taking the real part of the inverse discrete Fourier transform (DFT) of the logarithm of the magnitude of the DFT of the signal (Equation 7):
where pts is the pressure time series. Cepstrum was calculated over averaged pressure time series using a built-in MATLAB function rceps. However, the graphical output of cepstrum needed to be further quantified for use in the soundscape code. To do this, a threshold set at c(n) = 0.1 was chosen, and any peaks above this threshold in the cepstrum output were used as proxies for periodicities with the number of peaks-per-minute (ppm) counted and reported in the soundscape code.
Inspired by Martin et al. (2019), time lagged autocorrelation used to highlight periodicities in acoustic data was considered as a periodicity metric candidate within the present study. Using an averaged pressure time series, the peaks above a selected threshold in autocorrelation plots can be counted and used as proxies for periodicity in a soundscape. Two averaging windows were assessed within this study to determine the best fit for the soundscape code: 1.0 s mean square (MS) sound pressure averages, and 0.1 s MS sound pressure averages. These nuanced autocorrelation metrics are referred to as “acorr2” (1.0 s average), and “acorr3” (0.1 s average). The threshold for periodicities using autocorrelation, a minimum peak prominence of ρyy(t, t + τ) = 0.5, was set using the MATLAB function findpeaks, and any autocorrelation coefficient peaks in the 1-min time window above this threshold were counted (ppm). For acorr2, 45 (75%) lags were considered. For acorr3, 420 lags (70%) were considered.
The H and D indices are calculated using the amplitude envelope which is given by the absolute value of the analytic signal ζ(t), defined as (Equation 8):
where: , and ph(t) is the Hilbert transform of the real valued signal p(t). Probability mass functions (PMF) give the probability that a discrete, random variable is exactly equal to some value, and the PMF of the amplitude envelope A(t) and PMF of the mean spectrum S(f) is given by (Equations 9 and 10):
and is used to quantify envelope dissimilarity where is the mean spectrum. Envelope dissimilarity is estimated between two signals by computing the difference between their PMFs (Equations 11 and 12):
where A(t) is the PMF of the amplitude envelope and S(f) is PMF of the mean spectrum. D-index (Equation 13) is the product of the temporal dissimilarity (Dt) and spectral dissimilarity(Df):
The D index is a between-group (β) index originally developed to measure differences between communities. In the context of this study, the D index is used to quantify differences in the soundscape across time by calculating it over consecutive acoustic recordings.
H-index (Equation 16) is the product of the spectral (Hf) and temporal (Ht) entropies (Equations 14 and 15):
where A(t) is the PMF of the amplitude envelope, and S(f) is the PMF of the mean spectrum. H is 0 for a single pure tone, increases with frequency bands and amplitude modulations, and approaches 1 for random noise.
Metric Performance Analysis
A qualitative analysis was done to determine the optimal representative metric for each of the three soundscape properties in the soundscape code. Visual analysis of spectrograms and waveforms, coupled with knowledge of the sound sources present at each site, helped to form a priori expectations for the candidate soundscape metrics (Figure 3). Metric statistics were compared against a priori expectations, identifying which metrics produced the strongest agreement across soundscape code properties. The SPLpk and SPLrms metrics have been well studied as quantitative metrics of amplitude (Madsen, 2005; Thompson et al., 2013; Merchant et al., 2015) and further comparison was not deemed necessary. A series of qualitative comparisons (Table 4) were used to inform the determination of which metric was optimal for each property. The qualitative comparisons shown in Table 4 do not represent an exhaustive review of the analysis completed using the soundscape code datasets, but rather represent the comparisons that produced definitive results in the analysis. Because amplitude metrics were already chosen, they are not featured among the list of comparisons.
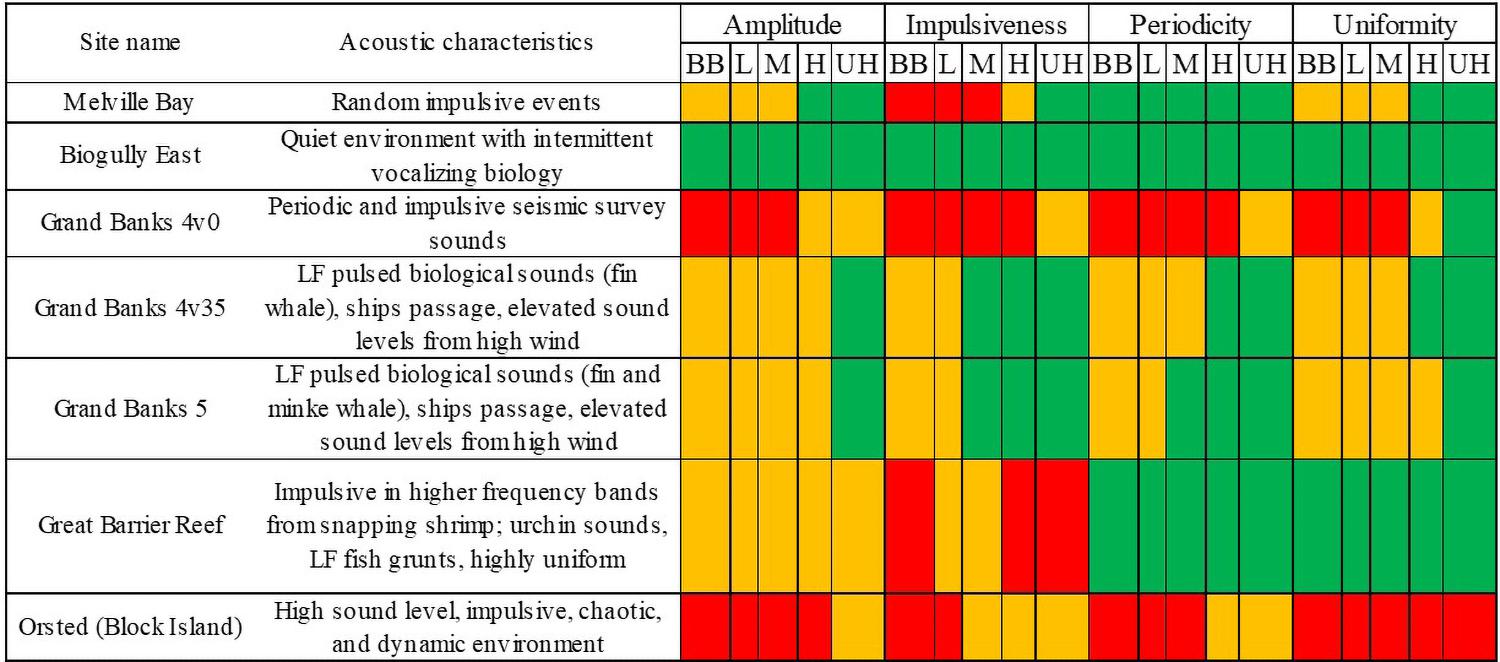
Figure 3. A priori metric response expectations for each data set. Expectations formed criteria to compare metrics and inform the metric selection. Green-Yellow-Red coloration represents relative expected metric response level where green indicates a low property level, yellow indicates a mid-level, and red indicates high-level responses. Low-level responses for the uniformity category indicate a uniform acoustic environment, and high-level responses indicate a lack of uniformity. Corresponding soundscape code frequency band is indicated by abbreviations for Broadband (BB), Low (L), Mid (M), High (H), Ultra-High (UH).
The qualitative comparisons and time series analysis of the candidate metrics informed most of the decision on which combination was optimal for use in the soundscape code. However, to explore how metric values could be used to distinguish or draw comparisons between sites, some statistical analysis of the metric values and distributions respective to each site and frequency band was desired. Because the ANOVA is an analysis specified for normally distributed Gaussian data, and distributions of kurtosis, D-index, and acorr3 violated this assumption of normality, a non-parametric multiple comparisons for all site pairs using the Dunn method for joint ranking was utilized (Dunn, 1964). Multiple comparisons tests were carried out using JMP ProTM 14.0.0 software and were repeated for the Broadband, Low, Mid, and High frequency bands; for each frequency band analyzed, 21 site pairs were assessed. For each metric, groupings in respective frequency bands were formed by sites whose metric distributions were determined by the multiple comparisons tests to not be significantly different. An assessment of how the candidate metrics “grouped” the soundscapes highlighted how the different metrics would compare or contrast the soundscapes of similar and different acoustic environments. Connected letters reports were created for the multiple comparisons test results to better visualize these groupings. Sites sharing common letters in the tables (within but not across frequency bands) have metric distributions that are not significantly different (according to the Dunn method for joint ranking).
Results
Results from a series of comparisons that led to the final choice of metrics are presented on a property-by-property basis. Results from several of the qualitative comparisons outlined in Table 4 are presented to highlight the responses that guided the metric selection. Metric comparisons were conducted for impulsiveness, periodicity, and uniformity properties. As stated previously, metrics for amplitude were already identified from previously published work as well-established and effective measures of the amplitude of sound pressure, so they were selected for the soundscape code without further analysis (Madsen, 2005; Thompson et al., 2013; Merchant et al., 2015). Calculated metric time series were compared to spectrograms, pressure waveforms, and a priori expectations to guide final metric selection.
Impulsiveness
Both kurtosis and crest factor were generally found to accurately report the presence of impulsive signals. However, kurtosis values more closely aligned with a priori expectations in characterizations of the impulsiveness of the soundscape code datasets. The superiority of kurtosis in indicating the presence of impulsive signals was suggested in qualitative comparison I1, which featured sound from only one dominant sound source: ice. Spectrograms showed that ice cracks, groans, and rumbling acoustic activity dominated the lower frequencies of the soundscape, but several instances of more broadband ice cracks exist in the dataset (Figure 2A). Impulsive metrics were expected to reflect the presence of impulsive signals in mostly BB, Low, or Mid soundscape code bands. Kurtosis reported many values exceeding the impulsive threshold in the BB, Low, and Mid soundscape code frequency bands indicating considerable impulsive acoustic activity in the expected frequency bands (Figure 4).
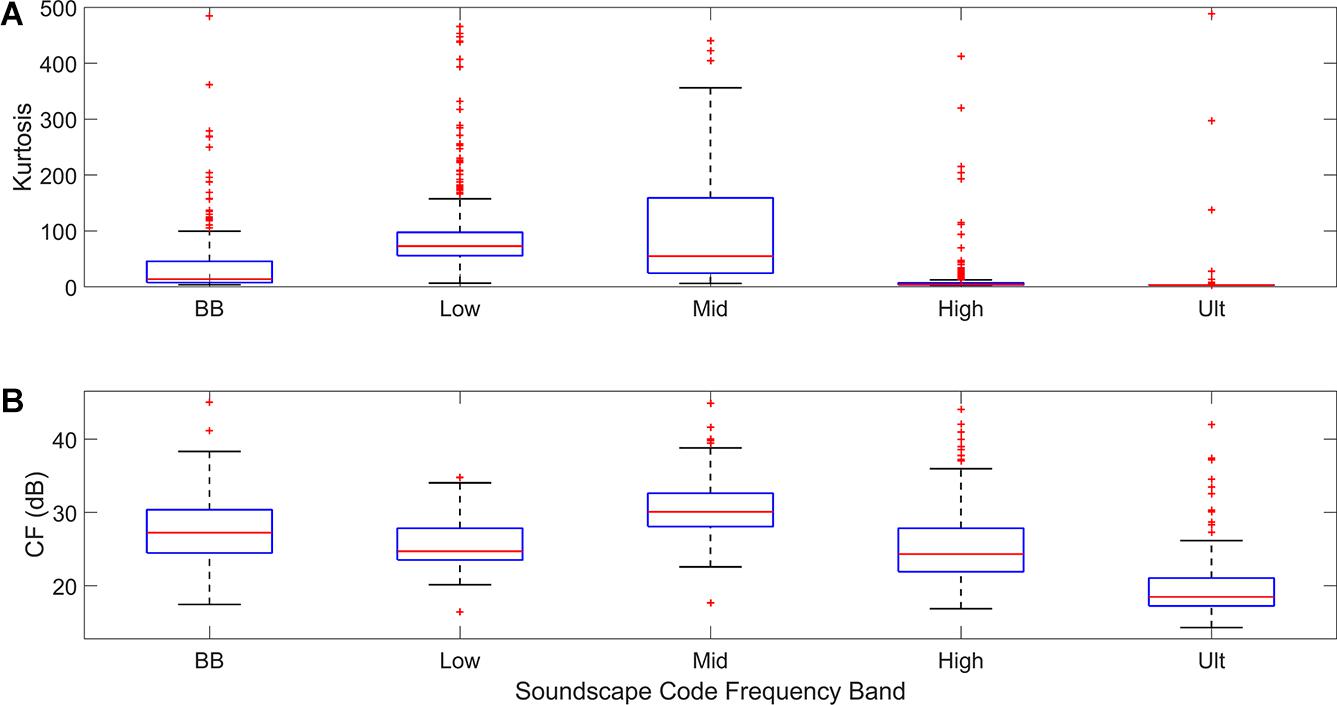
Figure 4. Impulsiveness comparison I1 results for (A) kurtosis and (B) crest factor. Wherein the boxplots red horizontal line indicates median value, outer edges of boxes represent 25th and 75th percentiles, whiskers mark boundary that contains approximately 99% of data values, and the red points are outliers.
Based on spectrogram analysis and an analysis of the sound pressure levels at MB, it was understood that while potentially impulsive events occurred frequently throughout the recording, a handful of high intensity events dominated the soundscape. It was expected that the impulse metrics would reflect the sporadic and intermittent nature of the ice cracks in boxplots of impulsiveness metric values through greater variability (Figure 4). Kurtosis performed as expected by indicating a wide range of kurtosis values that accurately captured the sporadic nature of the ice sounds. While crest factor reflected the presence of impulsive signals, it reported very little distinction between the soundscape code frequency bands, and the crest factor values varied much less than the kurtosis values so that it was not as possible to detect that a handful of high amplitude events characterized the impulsive nature of the soundscape at MB.
At GB4v35, where the 20 Hz pulsed vocalizations formed the basis for qualitative comparison I2, kurtosis values indicated the presence of impulsive signals the in Low band for minutes 1, 2, 3, 5, 6, 7, 9, 10, 11 which corresponded closely to the minutes containing pulsed fin whale vocalizations. Mid and High band kurtosis values maintained values of 3 for the duration of the qualitative comparison I2. Crest factor peaks also aligned with the pulse trains, but unlike kurtosis, crest factor impulse detections were identified in all soundscape code frequency bands, and for every minute but the 8th. The crest factor values in the Mid (100–1000 Hz), High (1–10 kHz), and Ultra-High (>10 kHz) soundscape code bands did not align with content visualized in the spectrograms or a priori expectations made based on the knowledge that the dominant sound source at this site was fin whales. However, 3–10 dB fluctuations in the 1-second SPLpk in the Ultra-high band were detected, which could indicate the presence of an impulsive sound and justify the higher than expected crest factor values (see Supplementary Figure 1 related to qualitative comparison I2). Ten-min boxplots were used to explore how the metric values changed over time at GB4v35 (Figure 5). Crest factor (Figure 5 Right) remained high during the period of ship noise (box 10–11), so it was difficult to deduce from the crest factor values that a ship had contributed significantly to the soundscape. In contrast, kurtosis values (Figure 5 Left) dropped quickly after the introduction of vessel noise to the soundscape (box 10–11), and values only increased after the vessel noise had subsided and the soundscape returned to being dominated by the pulsed signals of the fin whales (boxes 14–21). Kurtosis also only showed a slightly elevated response to a different fin whale chorus that correspond roughly to boxes 22-32 and a different recording period. Crest factor indicated little difference between the impulsiveness of the two different fin whale choruses.
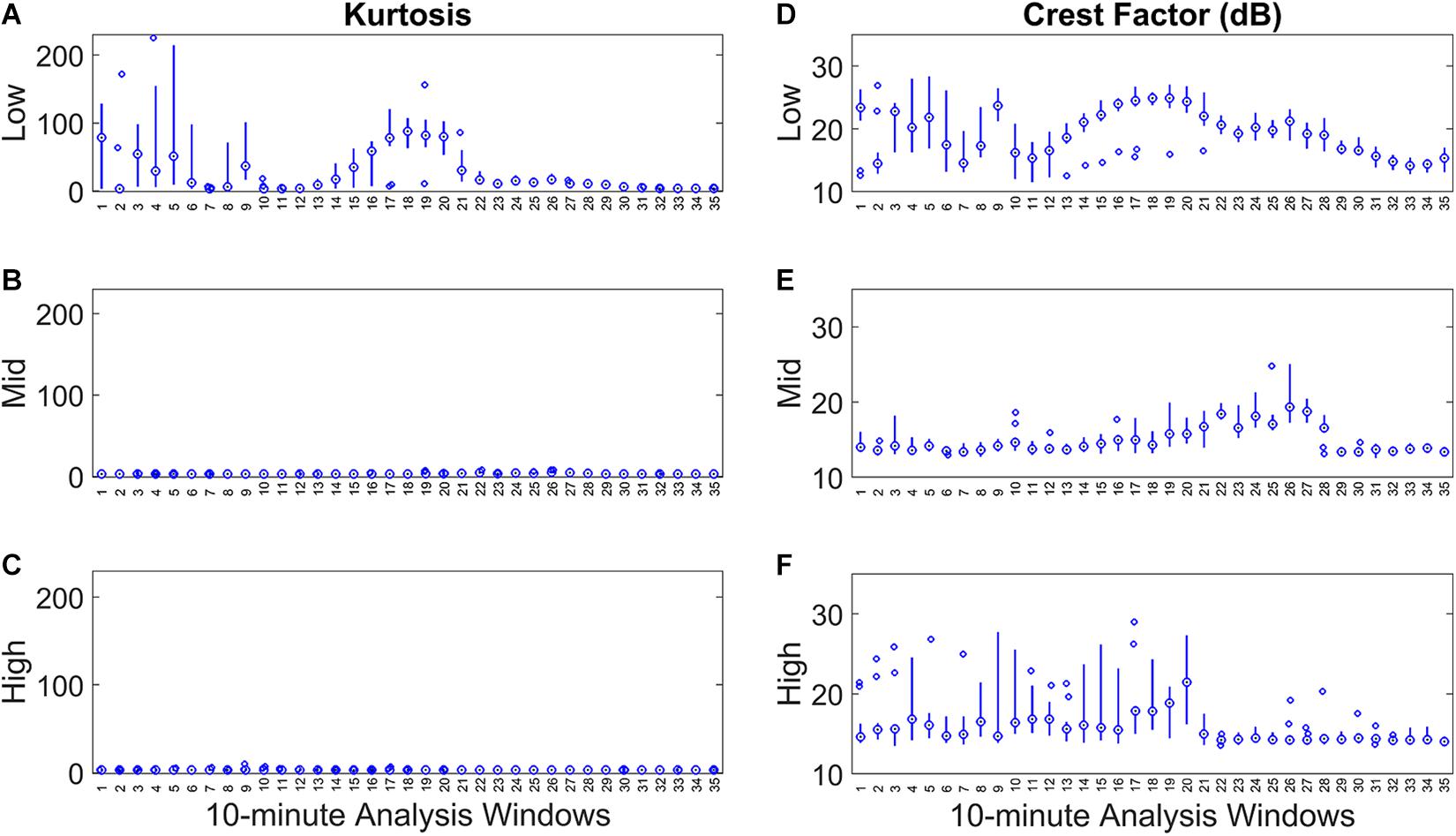
Figure 5. Boxplots of kurtosis values for the Low (A), Mid (B), and High (C) bands and crest factor values for the Low (D), Mid (E), and High (F) bands at GB4v35. Each box represents the range of metric values in a 10-min time window comprised of metrics calculated over 1-min time windows (each boxplot contains 10 metric values). Circled dots intersecting boxes indicate median values, thick boxes indicate 25th and 75th percentile range, narrower lines indicate range of 99% of data, and blue circles indicate outliers.
Qualitative comparison I3 which contained signals from a seismic survey (Figure 2B) yielded similar results in terms of the performance of the two impulsiveness metrics. Ultimately, both metrics adequately reported the nature of the impulsive seismic survey signals, but kurtosis again aligned more with the salient signals in the proper frequency bands (see Supplementary Figure 2 related to qualitative comparison I3). 10-min boxplots of both crest factor and kurtosis values adequately reflected the nature of the impulsive signals in the GB4v0 soundscape. However, kurtosis boxplots at GB4v0 highlighted the difference in seismic survey signals as the survey vessel approached, passed over the hydrophone, and departed. Crest factor, on the other hand, indicated little difference between the phases of the survey.
Periodicity
Periodicity metrics all reflected aspects of the periodic nature of each of the soundscapes and differences in metric responses were typically nuanced (Figure 6). Acorr3 was found to be more closely linked to the periodic nature of the soundscapes, and was more robust to mischaracterizations of the soundscapes that were observed with acorr2 and cepstrum.
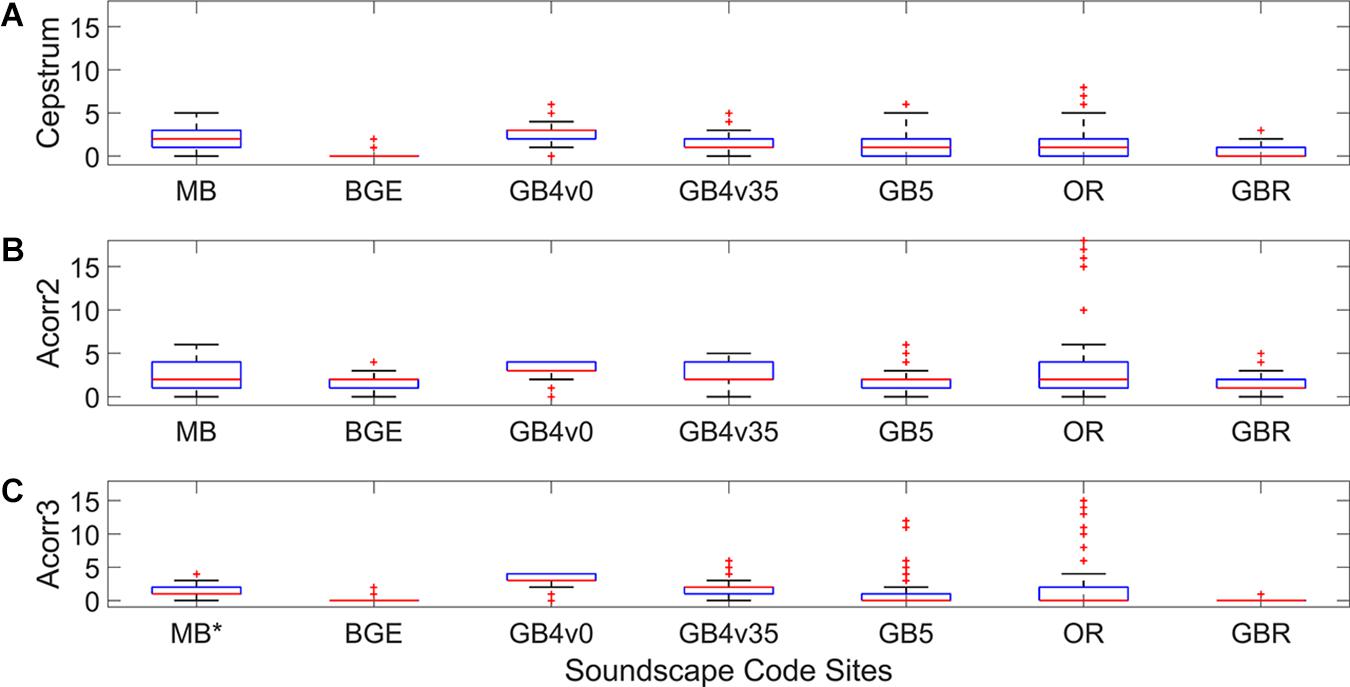
Figure 6. Broadband periodicity candidate metric results for all soundscape code datasets. Values represent peaks-per-minute as reported by (A) cepstrum, (B) acorr2, and (C) acorr3. The red horizontal line indicates the median value, outer edges of boxes represent 25th and 75th percentiles, whiskers mark the boundary that contains approximately 99% of data values, and the red points are outliers.
In comparison P1, subsets of the GB4v35 dataset contained unequal numbers of fin whale pulsed vocalizations, and this disparity was used to compare the responses of the periodicity measures to determine if the metrics would report more peaks in time window 1, which contained far more of the 20 Hz periodic fin whale vocalizations (Figure 7). Cepstrum reported 27 fewer peaks across frequency bands in time window 2, while acorr3 reported 11 fewer peaks. In a deviation from expectations, acorr2 reported six more peaks for the second time window.
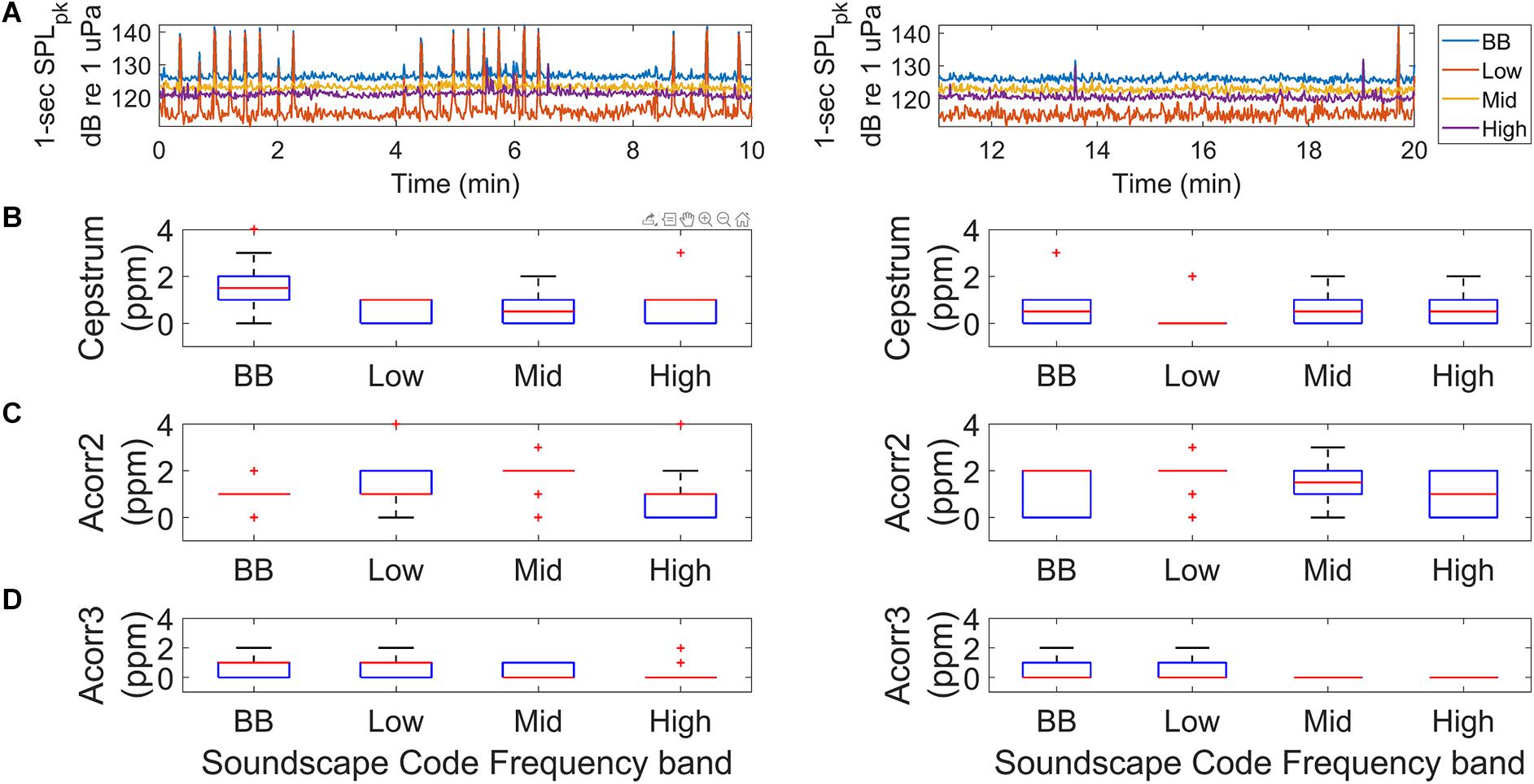
Figure 7. Periodicity comparison P1 results. 20-Hz fin whale vocalizations captured in (A) frequency filtered 1-SPLpk for time window 1 (left) and time window 2 (right). Boxplots show range of periodicity candidate metric values (ppm) corresponding to the two time windows for (B) cepstrum, (C) acorr2, and (D) acorr3. The red horizontal line indicates the median value, outer edges of boxes represent 25th and 75th percentiles, whiskers mark the boundary that contains approximately 99% of data values, and the red points are outliers.
Time series analysis using 10-min boxplots over the entirety of the GB4v35 dataset similar to the analysis presented in Figure 5 showed two main differences: (1) Acorr2 reported more peaks per minute than acorr3 in the High band for 69% of the minutes analyzed (n = 353). (2) Both acorr2 and acorr3 were highly consistent during the second period of fin whale vocalizations while cepstrum varied more (see Supplementary Figure 3 related to time series analysis of the periodicity metrics at GB4v35).
Qualitative comparisons P2 and P3 yielded results that were less conclusive than P1. Comparison P2 utilized sounds from a seismic survey (Figure 2B) and metrics were expected to report an increase in peaks-per-minute from time window 1 to time window 2. Time window 1 captured distant seismic survey signals, while time window 2 captured close proximity signals that were louder and had more consistent repetition. All metrics reported more peaks-per-minute across Soundscape Code frequency bands for the second time window of the GB4v0 dataset. Comparison P3 utilized the sounds from an impact pile driving operation (Figure 2D) and metrics were expected to report a decrease in peaks-per-minute from time window 1 to time window 2. Time window 1 featured intense pile driving sounds and time window 2 did not. The periodicity metrics in P3 reported a substantial decrease in peaks-per-minute across the two time windows. 10-min boxplots of periodicity metrics plotted over the duration of the datasets used in qualitative comparisons P2 and P3 did not indicate conclusive differences and all metrics responded appropriately to the different acoustic activity featured in the two datasets.
Uniformity
D-index values aligned with a priori expectations and outperformed the H-index in every analysis conducted using the soundscape code datasets. D-index values accurately captured the acoustic uniformity at all soundscape code datasets by indicating consistently high values at GB4v0 and OR, and the presence of high values in sites where dramatic changes in the acoustic environment occurred (Figure 8).
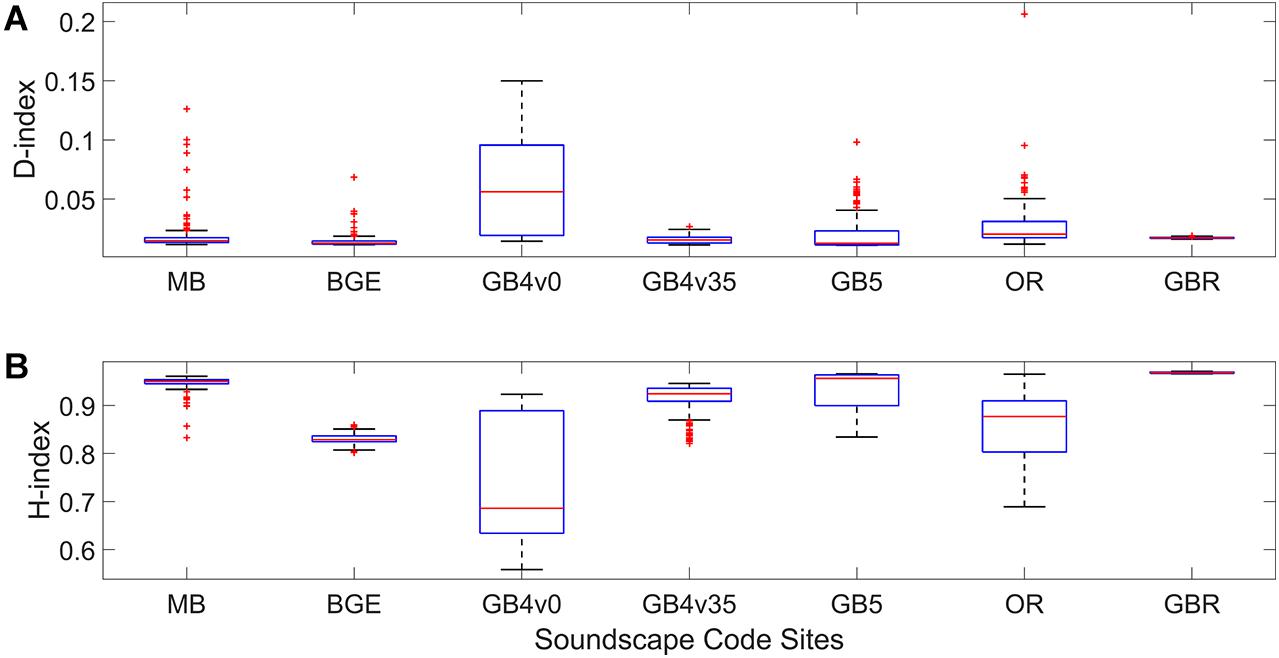
Figure 8. Broadband (A) D-index and (B) H-index metric values for all seven soundscape code sites. Red horizontal line indicates median value, outer edges of boxes represent 25th and 75th percentiles, whiskers mark boundary that contains approximately 99% of data values, and the red points are outliers.
Comparisons of uniformity metric results drew on ice sounds from MB (Figure 2A), pile driving and boat noise from OR (Figure 2D), reef sounds from GBR (Figure 2G), and sporadic echolocation and whistling activity from BGE (Figure 2E) to determine which metric would represent soundscape uniformity in the soundscape code (Table 4 rows 7 and 8). At MB, the D-index values in the low and mid bands reflected the sporadic and random ice noise (Figure 9). Compared to BGE, D-index values accurately characterized MB as more variable in these bands. In the High and Ultra-High bands, BGE D-index values were greater than MB, which again was an accurate representation of the acoustic activity of northern bottlenose. Disruption of acoustic uniformity from the northern bottlenose whales at BGE was reflected clearly in time series analysis of D-index values. H-index also reflected the decreased uniformity at MB, but the dependence of this metric on bandwidth made interpretation and comparison difficult, as H-index values increased from the Low to Ultra-High soundscape code band regardless of acoustic uniformity. D-index soundscape code values in Figure 9 reflect the substantial disparity in acoustic uniformity between the two sites in both magnitude and variability of the index. In contrast, the slightly larger range of the H-index values corresponding to the MB Low band indicated only a slight disparity in acoustic uniformity between the two sites, and the magnitude of the index was not representative of the recording content.
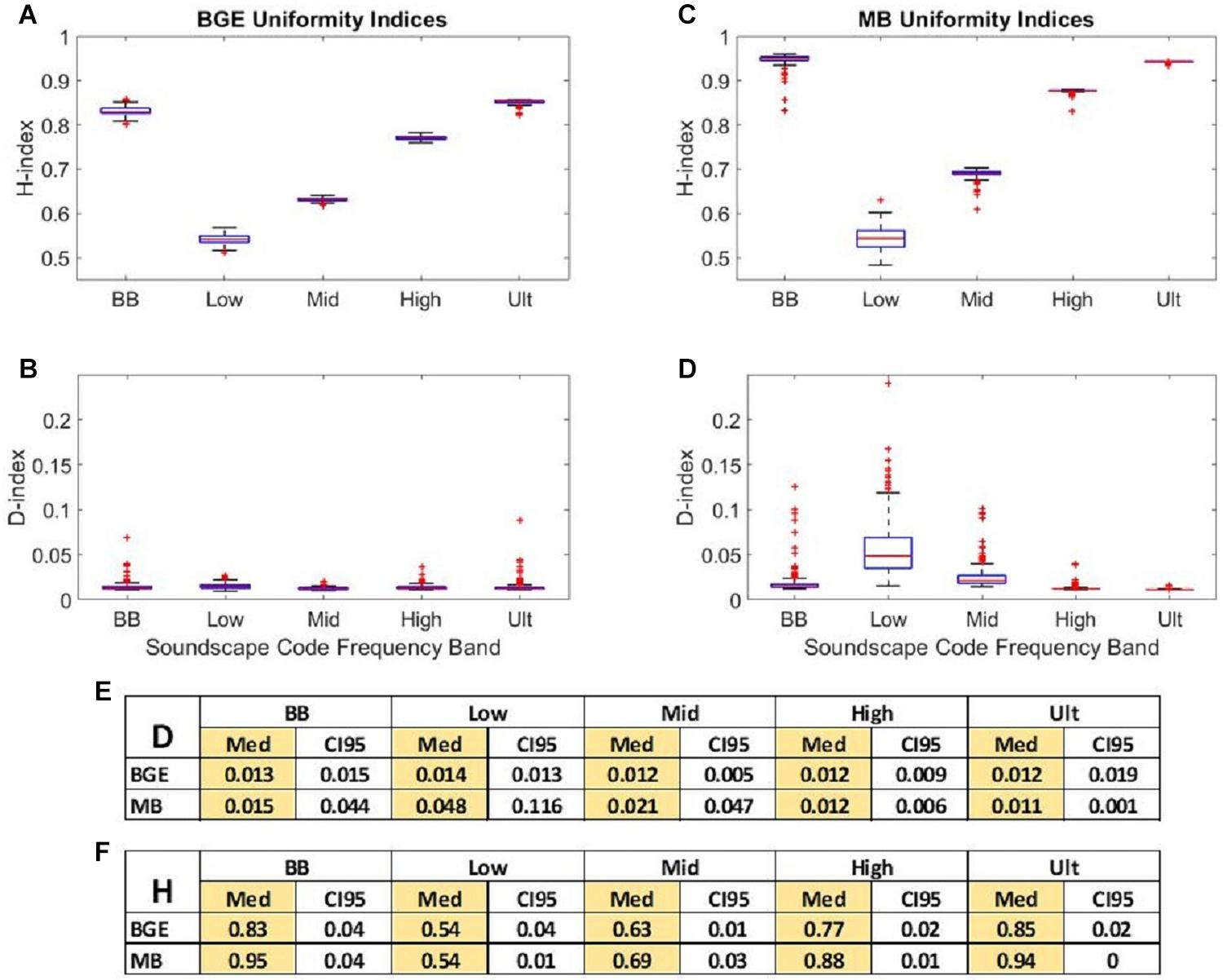
Figure 9. Uniformity comparison U1 results. H-index values for (A) BGE and (C) MB sites; D-index values for (B) BGE and (D) MB sites; Red horizontal line indicates median value, outer edges of boxes represent 25th and 75th percentiles, whiskers mark boundary that contains approximately 99% of data values, and the red points are outliers. Corresponding soundscape code results for (E) the D-index at BGE and MB, and (F) the H-index at BGE and MB report the median (med) and size of the 95% confidence interval (CI95).
Similar analysis carried out on data from the OR and GBR sites yielded slightly different results. In qualitative comparison (U2), comparisons of respective uniformity metrics across the sites highlights differences in acoustic uniformity. D-index values more clearly capture the disparity in acoustic uniformity between OR and GBR especially in the increased size of the boxplots of values at OR in the High and Ultra-High bands. H-index values used to compare the acoustically distinct OR and GBR sites failed to reflect the acoustic disparity by producing almost identical soundscape code medians, with only slightly more variability of the 1-min H-index values reported at OR. Similar to the H-index, the magnitudes of the D-index values at both OR and GBR were only slightly different. The variability measure of the D-index, however, did reflect the disparity in acoustic uniformity across OR and GBR (see Supplementary Figure 4 figure related the qualitative comparison U2). When metric values were analyzed using 10-min boxplots over the full OR recording, the D-index more effectively captured the dynamic nature of the soundscape, while the H-index values hardly indicated any changes in acoustic activity (see Supplementary Figures related time series analysis of the uniformity indices at OR and GBR). The intuitive nature of the D-index and much closer alignment to salient acoustic activity in the soundscapes of the soundscape code datasets than H-index suggested D-index was the optimal metric to represent acoustic uniformity in the soundscape code.
Statistical Groupings of Metric Values
The non-parametric multiple comparisons tests were used to form the statistical groupings of sites based on the medians of the metric values (Figure 10missing). Respective to each site, metric values that are not significantly different are “connected” by identical letters in the connected letter tables that report the multiple comparisons results. In key frequency bands, the uniquely impulsive soundscapes of MB, GBR, OR, and GB4v0 were all found to have kurtosis values that were significantly different than the sites where impulsive signals were either rare or only faint (BGE, GB4v35, and GB5) (Figure 10A). Kurtosis was observed to outperform crest factor in terms of robustness, sensitivity, and more informative soundscape grouping, which led to the selection of kurtosis to represent impulsiveness in the soundscape code. Periodicity metrics failed to produce intuitive groupings of the sites in terms of periodic content, and acorr3 was the only metric that produced significantly different values between the highly periodic site and the moderate-low periodic sites (MB, BGE, GB5, and GBR) (Figure 10B). In spite of less than ideal groupings for acorr3, optimal performance in qualitative comparisons and other analyses made it the only viable choice and acorr3 was selected as the metric to represent soundscape periodicity. Multiple comparisons results for the D-index were both adequate and less than ideal, depending on which frequency band was being considered (Figure 10C). However, considering the far more intuitive nature of the index and consistently better performance relative to the H-index, D-index was chosen to represent acoustic uniformity in the soundscape code.
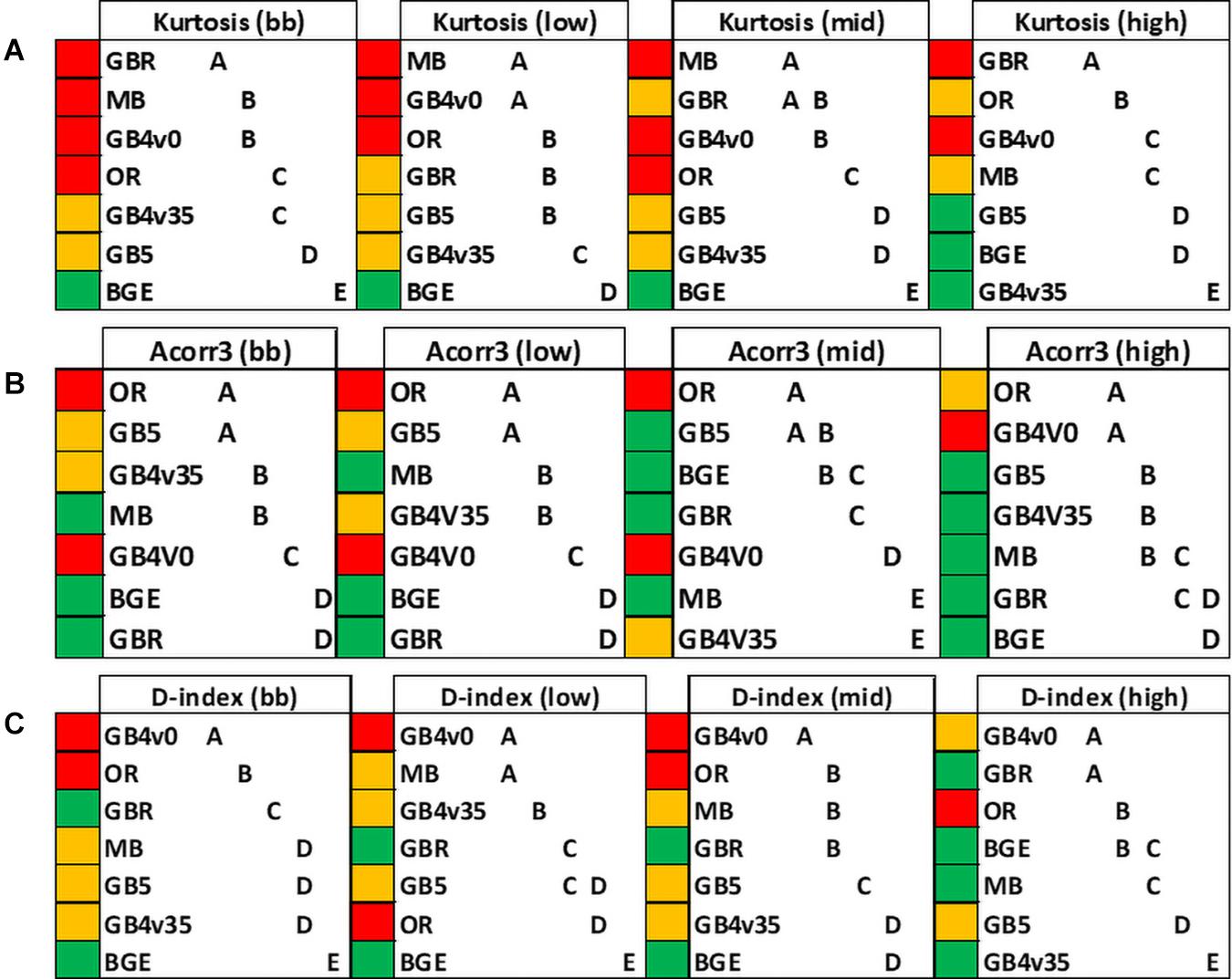
Figure 10. Connected letters plot showing results from multiple comparisons tests for (A) kurtosis, (B) acorr3, and (C) D-index. Sites connected by identical letters were determined by the multiple comparisons tests to have 1-min metric values that are not significantly different. Connected letters within but not across each frequency band form groupings of sites based on metric values. Color bars corresponding to the a priori expectations detailed in Figure 3 were provided to demonstrate how the quantitative site groupings of the multiple comparisons tests compared to the a priori expectations. The colors indicate the expected level of the metric response where green indicates a low property level, yellow indicates a mid-level, and red indicates high-level responses.
Discussion
A collection of metrics was applied to a series of unique soundscapes to identify the optimal suite of metrics for capturing the salient soundscape characteristics, which ultimately enables quick and simple quantitative comparisons of soundscapes. The final determination considered both the metric efficacy in quantifying the corresponding soundscape property, and how well the metric fit into the infrastructure of the soundscape code. SPLrms and SPLpk (amplitude), kurtosis (impulsiveness), D-index (uniformity), and acorr3 (periodicity) were determined to be the best metrics out of the candidate metrics for comparing soundscapes. Soundscape codes comprised of the optimal metrics indicated dominant signal frequencies and salient differences in acoustic environments (Figure 11). Figure 11 represents what an initial soundscape assessment using the soundscape code methodology might look like; tabulated soundscape information across frequency bands and metrics offers an initial “glimpse” into a marine acoustic environment and highlights areas of interest for further targeted analysis. The soundscape code is proposed here as a first step in the direction of a standardized soundscape analysis methodology that will ultimately facilitate quantitative comparison and assessment of soundscapes, and guide subsequent analysis.
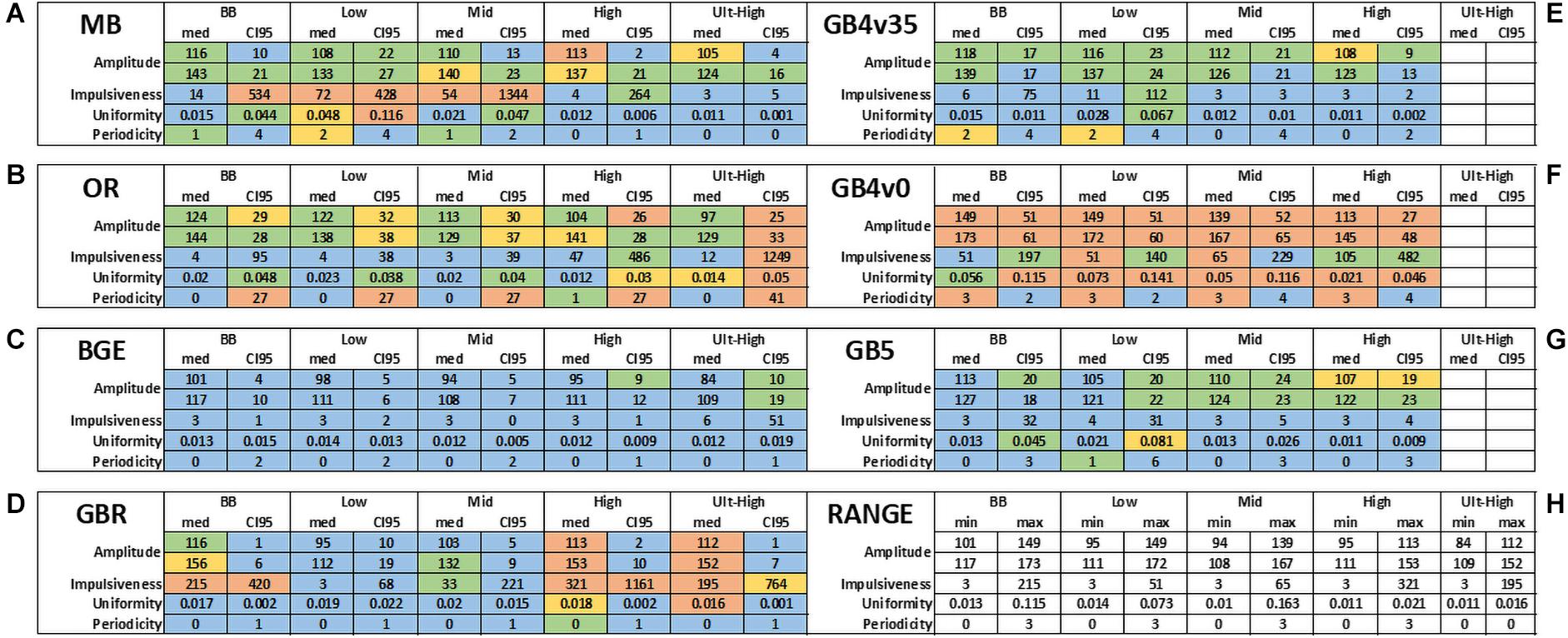
Figure 11. Soundscape code results for the seven soundscape code datasets: (A) MB, (B) OR, (C) BGE, (D) GBR, (E) GB4v35, (F) GB4v0, and (G) GB5. Columns indicate the frequency band, and for each band, the median (med) and 95% confidence intervals (CI95) are reported. (H) The minimum and maximum soundscape code median values observed across all sites in corresponding frequency bands. Metrics represented in each row of the soundscape codes are from top to bottom: SPLrms, SPLpk, kurtosis, D-index Index, acorr3. The total range of the soundscape code medians and 95% CIs was divided into quartiles (respectively), and the cell colors correspond to which quartile the value falls into from low to high: blue, green, yellow, red.
Traditionally, underwater soundscape studies focus mostly on quantifying fluctuations, central tendencies, or minimum/maximum observed levels of amplitude typically represented by sound pressure, intensity, or acoustic energy (Table 1). If metrics that quantify aspects of other soundscape properties are included in soundscape analysis, a more thorough assessment of soundscapes is possible. The soundscape properties outlined in Table 2 were quantified by the selected metrics, which allowed comparisons of the soundscape code datasets to be made in terms of sound amplitude, impulsiveness and transient events, content of repetitive signals, and spectral and temporal variability. For example, in a comparison of the impulsiveness of the soundscape code datasets GB5, GB4v35, and BGE, impulsiveness metric values indicate they are the least impulsive sites of the seven (Figure 11). This observation was made quickly, and demonstrates the ease with which one can compare and contrast different soundscapes when identical metrics are being compared. This assessment of across site impulsiveness can be taken a step further: The elevated 95% CI value in the Ultra-High (relative to BB, Low, Mid, and High) band at BGE indicates the presence of acoustically active northern bottlenose whales. At the same time, the median and 95% CI in the Low bands at GB4v35 and GB5, respectively, indicate the presence of chorusing fin whales. Martin et al. (2020) showed that 1-min kurtosis values increased as the amplitude of simulated impulses increased, so the slightly elevated impulsiveness metric values at GB4v35 relative to GB5 could be a manifestation of the higher amplitude of the fin whale chorus at GB4v35, and this coincides with increased SPLpk values at this site. This example highlights how a combination of multidimensional metrics can be used congruently to understand a soundscape and how nuanced differences in the metrics can indicate significant differences in soundscape composition. The collection of metrics captures temporal and frequency characteristics of acoustic environments and depending on application can be used to assess spatial temporal and variation in soundscapes directly corresponding to the soundscape components defined in ISO (2017) 18405.
The proposed soundscape code provides a valuable framework to simply covey complex ocean characteristics and is a “first step” in the direction of a standardized soundscape analysis and reporting structure. We recognize that the future use and potential improvement of the soundscape code will benefit from more thorough assessment of duty cycling, bandwidth definitions, and dataset durations, as only data sets of multiple hours and a majority of continuous sampling regimes were used to select the proposed soundscape code metrics. Further work assessing the impact and performance of different analysis windows (larger time scales), datasets with unique acoustic features not captured in this work, datasets with significant overlapping of source signals, and threshold selections is required to ensure the development of an effective, rapid, and robust quantitative soundscape framework.
Duty cycle was found to have impacts on the D-index, as the D-index measures the difference between consecutive recordings, and consecutive recordings will have more in common than recordings spaced apart by longer periods of time. The selected frequency bandwidths worked for the purposes of this project, but other frequency banding should be explored to better represent evolving regulations and knowledge of marine life hearing. Similar to duty cycle concerns, dataset duration being represented in the soundscape code should be explored to understand how a comparison of soundscape code results from a small duration dataset (minutes to hours) compares to results from a large duration datasets (days to months). A final aspect to consider in future soundscape code performance and application is the duration of the integration and averaging windows for each of the property metrics. All soundscape code metrics were based on 1-min time windowing protocol to better align with what few standard soundscape analysis methods there are (Ainslie et al., 2018). Averaging of sound pressure for the periodicity metrics was done on 0.1-second and 1.0 s windows. Other window sizes should be explored to assess performance and use of the soundscape code. Exhaustive analysis of the impact that different analysis parameters have on the soundscape code metrics would have added to the value of this research, but it did not fit into the scope of the project. Thorough metric analysis did, however, identify several aspects of the selected soundscape code metrics that should be explored.
The selection of acorr3 as the periodicity metric is a prime candidate for additional assessment and development within the soundscape code structure. It was noticed that acorr3 produced “false positives” due to noise in the autocorrelation outputs. This was found with all of the candidate periodicity metrics, but in acorr3 it occurred at a much reduced and more manageable manner. In spite of the potential to falsely indicate the presence of periodicities, acorr3 accurately characterized the soundscape datasets in terms of periodicity, with the exception of MB where the repeated cracking of ice led to a mischaracterization of this site being more periodic than expected.
The candidate metric for uniformity, the H-index, exhibited a strong dependence on the bandwidth of the signal being analyzed, which made within site comparisons of the H-index across soundscape code frequency bands futile and would severely limit the utility of the uniformity metric in the soundscape code. Furthermore, the observed behavior of the H-index in response to anthropogenic activity is similar to findings in Parks et al. (2014): anthropogenic sounds confounded the metric. At OR and GB4v0, the sounds of a seismic survey and pile driving drove the H-index down, while the opposite was observed for the D-index. Ship noise at GB4v35 and GB5 had little effect on the H-index but drove D-index values down as biological signals from fin whales were masked. Elevated ambient sound levels from hurricane Nicole had a similar masking effect on the H and D indices, where the H-index was unaffected but the D-index dropped significantly. The D-index was found to more closely align with the real world signals in the soundscape code datasets and consistently reflected the acoustic uniformity of known sound sources in proper frequency bands. D-index demonstrated a sensitivity that allowed it to highlight nuanced differences in soundscape composition, and ultimately it was chosen as the metric to represent acoustic uniformity. However, extremely small values of the D-index make interpretation more difficult than the metrics that report the other soundscape properties and further scrutiny should be given to ensure metric efficacy.
Both impulsiveness metrics were closely tied to the content of impulsive signals in the soundscape but kurtosis outperformed crest factor in meeting a priori expectations and produced values that made assessments of impulsiveness easier and quicker. The constrained range of possible crest factor values means the variability it produced when characterizing sites in terms of impulsiveness can be narrow and hard to interpret. The larger range of possible kurtosis values meant it could more dramatically reflect differences in transient or impulsive acoustic activity between sites, which makes rapid assessments more feasible. Kurtosis is already well established in signal analysis and acoustics, so compared to the metrics representing periodicity and uniformity, it does not need to be as thoroughly assessed in terms of efficacy. Analysis of kurtosis time series to explain soundscape code metric values across properties led to a realization that time series analysis of the soundscape code metrics is also an informative method for exploring and assessing acoustic environments with implications for future applications.
Targeted analysis of large acoustic datasets could be made easier by analyzing time series data of the soundscape code metrics. D-index time series consistently indicated time periods of dynamic acoustic activity. Peaks in acorr3 metric time series regularly highlighted the presence of echolocation signals and transient periodic acoustic signatures. Time series analysis of kurtosis values demonstrated an impressive utility in the assessment of a variety of aspects of underwater sound by indicating the presence of transient acoustic activity and shifts in acoustic activity in general. Time series analysis of kurtosis suggests the metric could be used in a variety of applications beyond the scope of soundscape comparison using the soundscape code. The relationship between kurtosis and impulsive sounds, and resultant relevance in impact studies indicates it could be used in assessments of noise impacts and mitigation. For example, bubble curtain efficacy could be assessed using the soundscape code or time series analysis of soundscape code metrics as the change in signal across a bubble curtain would assuredly be captured by impulsiveness and amplitude metrics, if not uniformity and periodicity metrics as well. Noise studies sometimes analyze sound at different ranges from a sound source, and the soundscape code metrics could easily be applied to this type of assessment and would quickly and clearly highlight salient differences in the impacted soundscape.
The soundscape code methodology provides a structure for quick and easy quantitative comparisons meant to capture salient soundscape characteristics for directed assessments of sources, patterns, and trends. The utility of the soundscape code methodology lies in succinct, consistent, and transparent reporting of acoustic soundscape properties. If the combination of metrics is calculated and reported in a uniform manner, then direct comparisons are easily made. Ambiguity in reporting of metric calculation parameters makes interpretation of results time consuming and can result in erroneous conclusions; the uniform integration times and frequency bands of the soundscape code allows for accurate direct comparisons with immediate understanding of exactly what is being calculated. Furthermore, the multidimensional nature of the soundscape code helps to highlight similarities and differences in soundscapes that are sometimes overlooked in traditional soundscape analyses.
Data Availability Statement
The data analyzed in this study is subject to the following licenses/restrictions: Some of the soundscape code data sets are proprietary within the companies that collected them. Data access and availability will be considered upon request. Requests to access these datasets should be directed to DW, dcw1017@wildcats.unh.edu.
Author Contributions
JM-O, SM, DH, and AL contributed to the conceptual framework of the study. SM and MS both assisted in the design and practical implementation of the framework. KL guided statistical analysis and interpretation of statistical results. All authors contributed to manuscript revisions, read, and approved the submitted version.
Funding
This work was supported by the NOAA Grant No. NA15NOS4000200 provided to the Center for Coastal and Ocean Mapping at the University of New Hampshire and by the United States Department of the Interior, Bureau of Ocean Energy Management, Environmental Studies Program, Washington, DC contract No. M17PC00009, in partnership with NOAA and USGS, project titled DEEP Sea Exploration to Advance Research on Coral/Canyon/Cold seep Habitats (DEEPSEARCH).
Conflict of Interest
SM was employed by the company JASCO Applied Sciences (Canada) Ltd.
The remaining authors declare that the research was conducted in the absence of any commercial or financial relationships that could be construed as a potential conflict of interest.
Publisher’s Note
All claims expressed in this article are solely those of the authors and do not necessarily represent those of their affiliated organizations, or those of the publisher, the editors and the reviewers. Any product that may be evaluated in this article, or claim that may be made by its manufacturer, is not guaranteed or endorsed by the publisher.
Acknowledgments
This research would not have been possible without collaboration from Shell Global Solutions for the Baffin Bay data; Deepwater Wind/Block Island Wind Farm for the Block Island data; JASCO Applied Sciences for the Great Barrier Reef data (in collaboration with James Cook University), and the Orphan Basin data (part of a Contribution Agreement with the Environmental Studies Research Fund); and Department of Fisheries and Oceans Canada, Newfoundland, for the Carson Canyon data set. We would also like to thank the reviewers of this manuscript for their thorough comments and feedback.
Supplementary Material
The Supplementary Material for this article can be found online at: https://www.frontiersin.org/articles/10.3389/fmars.2021.672336/full#supplementary-material
Supplementary Figure 1 | Impulsiveness comparison I2 results. (A) 1-second SPLpk plot shows pulsed fin whale vocalizations that mostly occur in the first 10-minute time window, and corresponding 1-minute (B) kurtosis and (C) crest factor values.
Supplementary Figure 2 | Impulsiveness comparison I3 results. (A) 1-second SPLpk plot shows pulsed seismic survey signals that increase in repetition and amplitude from time window 1 (left) to time window 2 (right). Corresponding 1-minute (B) kurtosis and (C) crest factor values are shown for the two time windows.
Supplementary Figure 3 | Boxplots of (A) cepstrum, (B) acorr2, and (C) acorr3 values corresponding to the GB4v35 dataset. Each box represents the range of metric values in a 10-minute time window comprised of metrics calculated over 1-minute time windows (each boxplot contains 10 metrics values). Red horizontal line indicates median value, outer edges of boxes represent 25th and 75th percentiles, whiskers mark boundary that contains approximately 99% of data values, and the red points are outliers.
Supplementary Figure 4 | Uniformity comparison U2 results. H-index values for (A) OR and (C) GBR sites; D-index values for (B) OR and (D) GBR sites; Red horizontal line indicates median value, outer edges of boxes represent 25th and 75th percentiles, whiskers mark boundary that contains approximately 99% of data values, and the red points are outliers. Corresponding soundscape code results for (E) the D-index at ORand GBR, and (F) the H-index at OR and GBR report the median (med) and size of the 95% confidence interval (CI95).
Footnotes
References
Ainslie, M. A., Miksis-Olds, J., Martin, S. B., Heaney, K., de Jong, C. A. F., von Benda-Beckmann, A. M., et al. (2018). ADEON Underwater Soundscape and Modeling Metadata Standard, Version 1.0. Technical report by JASCO Applied Sciences for ADEON Prime Contract No. M16PC00003. Halifax: JASCO.
Bertucci, F., Parmentier, E., Berten, L., Brooker, R. M., and Lecchini, D. (2015). Temporal and spatial comparisons of underwater sound signatures of different reef habitats in Moorea Island, French Polynesia. PLoS One 10:e0135733. doi: 10.1371/journal.pone.0135733
Bogert, B. P., Healy, M. J. R., and Tukey, J. W. (1963). “Quefrency alanysis of time series for echoes: cepstrum, pseudo-autocovariance, cross-cepstrum, and saphe-cracking,” in Proceedings of the Symposium on Time Series Analysis, (New York, NY: Wiley).
Bohnenstiehl, D. R., Lyon, R. P., Caretti, O. N., Ricci, S. W., and Eggleston, D. B. (2018). Investigating the utility of ecoacoustic metrics in marine soundscapes. J. Ecoacoust. 2:12. doi: 10.22261/JEA.R1156L
Bolgan, M., Amorim, M. C. P., Fonseca, P. J., Di Iorio, L., and Parmentier, E. (2018). Acoustic complexity of vocal fish communities: a field and controlled validation. Sci. Rep. 8:10559. doi: 10.1038/s41598-018-28771-6
Buscaino, G., Ceraulo, M., Pieretti, N., Corrias, V., Farina, A., Filiciotto, F., et al. (2016). Temporal patterns in the soundscape of the shallow waters of a Mediterranean marine protected area. Sci. Rep. 6:34230. doi: 10.1038/srep34230
Carroll, A. G., Przeslawski, R., Duncan, A., Gunning, M., and Bruce, B. (2017). A critical review of the potential impacts of marine seismic surveys on fish & invertebrates. Mar. Pollut. Bull. 114, 9–24. doi: 10.1016/j.marpolbul.2016.11.038
Casper, B. M., Halvorsen, M. B., Matthews, F., Carlson, T. J., and Popper, A. N. (2013a). Recovery of barotrauma injuries resulting from exposure to pile driving sound in two sizes of hybrid striped bass. PLoS One 8:e73844. doi: 10.1371/journal.pone.0073844
Casper, B. M., Smith, M. E., Halvorsen, M. B., Sun, H., Carlson, T. J., and Popper, A. N. (2013b). Effects of exposure to pile driving sounds on fish inner ear tissues. Comp. Biochem. Physiol. Part A Mol. Integr. Physiol. 166, 352–360. doi: 10.1016/j.cbpa.2013.07.008
Clarke, E., Feyrer, L. J., Moors-Murphy, H., and Stanistreet, J. (2019). Click characteristics of northern bottlenose whales (Hyperoodon ampullatus) and Sowerby’s beaked whales (Mesoplodon bidens) off eastern Canada. J. Acoust. Soc. Am. 146, 307–315. doi: 10.1121/1.5111336
Coles, R. R. A., Garinther, G. R., Hodge, D. C., and Rice, C. G. (1968). Hazardous exposure to impulse noise. J. Acoust. Soc. Am. 43, 336–343.
Dunn, O. J. (1964). Multiple comparisons using rank sums. Technometrics 6, 241–252. doi: 10.2307/1266041
Erdreich, J. (1986). A distribution based definition of impulse noise. J. Acoust. Soc. Am. 79, 990–998. doi: 10.1121/1.393698
Greene, C. R., and Richardson, W. J. (1988). Characteristics of marine seismic survey sounds in the Beaufort Sea. J. Acoust. Soc. Am. 83, 2246–2254. doi: 10.1121/1.396354
Halvorsen, M. B., Casper, B. M., Matthews, F., Carlson, T. J., and Popper, A. N. (2012a). Effects of exposure to pile-driving sounds on the lake sturgeon, Nile tilapia and hogchoker. Proc. R. Soc. Lond. B Biol. Sci. 279, 4705–4714. doi: 10.1098/rspb.2012.1544
Halvorsen, M. B., Casper, B. M., Woodley, C. M., Carlson, T. J., and Popper, A. N. (2012b). Threshold for onset of injury in Chinook Salmon from exposure to impulsive pile driving sounds. PLoS One 7:e38968. doi: 10.1371/journal.pone.0038968
Harris, S. A., Shears, N. T., and Radford, C. A. (2016). Ecoacoustic indices as proxies for biodiversity on temperate reefs. Methods Ecol. Evol. 7, 713–724. doi: 10.1111/2041-210X.12527
Haver, S. M., Klinck, H., Nieukirk, S. L., Matsumoto, H., Dziak, R. P., and Miksis-Olds, J. L. (2017). The not-so-silent world: measuring Arctic, Equatorial, and Antarctic soundscapes in the Atlantic Ocean. Deep Sea Res. Part I. 122, 95–104. doi: 10.1016/j.dsr.2017.03.002
Hawkins, R. S., Miksis-Olds, J. L., and Smith, C. M. (2014). Variation in low-frequency estimates of sound levels based on different units of analysis. J. Acoust. Soc. Am. 135, 705–711. doi: 10.1121/1.4861252
Hildebrand, J. (2009). Anthropogenic and natural sources of ambient noise in the ocean. Mar. Ecol. Progr. Ser. 395, 5–20. doi: 10.3354/meps08353
Howe, B. M., Miksis-Olds, J., Rehm, E., Sagen, H., Worcester, P. F., and Haralabus, G. (2019). Observing the oceans acoustically. Front. Mar. Sci. 6:426. doi: 10.3389/fmars.2019.00426
ISO (2017). 18405.2. Underwater Acoustics—Terminology. Geneva: International Organization for Standardization.
Kastelein, R. A., Gransier, R., Marijt, M. A., and Hoek, L. (2015). Hearing frequency thresholds of harbor porpoises (Phocoena phocoena) temporarily affected by played back offshore pile driving sounds. J. Acoust. Soc. Am. 137, 556–564. doi: 10.1121/1.4906261
Kastelein, R. A., Helder-Hoek, L., Van de Voorde, S., von Benda-Beckmann, A. M., Lam, F. A., Jansen, E., et al. (2017). Temporary hearing threshold shift in a harbor porpoise (Phocoena phocoena) after exposure to multiple airgun sounds. J. Acoust. Soc. Am. 142, 2430–2442. doi: 10.1121/1.5007720
Kyhn, L. A., Wisniewska, D. M., Beedholm, K., Tougaard, J., Simon, M., Mosbech, A., et al. (2019). Basin-wide contributions to the underwater soundscape by multiple seismic surveys with implications for marine mammals in Baffin Bay, Greenland. Mar. Pollut. Bull. 138, 474–490. doi: 10.1016/j.marpolbul.2018.11.038
Lin, T.-H., Yang, H.-T., Huang, J.-M., Yao, C.-J., Lien, Y.-S., Wang, P.-J., et al. (2019). “Evaluating changes in the marine soundscape of an offshore wind farm via the machine learning-based source separation,” in Proceedings of the 2019 IEEE Underwater Technology (UT), (Kaohsiung: IEEE), 1–6. doi: 10.1109/UT.2019.8734295
Lobel, P. S. (1992). Sounds produced by spawning fishes. Environ. Biol. Fish. 33, 351–358. doi: 10.1007/BF00010947
Lucke, K., Siebert, U., Lepper, P. A., and Blanchet, M. A. (2009). Temporary shift in masked hearing thresholds in a harbor porpoise (Phocoena phocoena) after exposure to seismic airgun stimuli. J. Acoust. Soc. Am. 125, 4060–4070. doi: 10.1121/1.3117443
Madsen, P. T. (2005). Marine mammals and noise: problems with root mean square sound pressure levels for transients. J. Acoust. Soc. Am. 117, 3952–3957. doi: 10.1121/1.1921508
Martin, S. B., and Barclay, D. R. (2019). Determining the dependence of marine pile driving sound levels on strike energy, pile penetration, and propagation effects using a linear mixed model based on damped cylindrical spreading. J. Acoust. Soc. Am. 146, 109–121. doi: 10.1121/1.5114797
Martin, S. B., Lucke, K., and Barclay, D. R. (2020). Techniques for distinguishing between impulsive and non-impulsive sound in the context of regulating sound exposure for marine mammals. J. Acoust. Soc. Am. 147, 2159–2176. doi: 10.1121/10.0000971
Martin, S. B., Matthews, M. R., MacDonnell, J. T., and Bröker, K. (2017). Characteristics of seismic survey pulses and the ambient soundscape in Baffin Bay and Melville Bay, West Greenland. J. Acoust. Soc. Am. 142:3331.
Martin, S. B., Morris, C., Bröker, K., and O’Neill, C. (2019). Sound exposure level as a metric for analyzing and managing underwater soundscapes. J. Acoust. Soc. Am. 146, 135–149. doi: 10.1121/1.5113578
McDonald, M. A., Hildebrand, J. A., and Wiggins, S. M. (2006). Increases in deep ocean ambient noise in the Northeast Pacific west of San Nicolas Island, California. J. Acoust. Soc. Am. 120, 711–718. doi: 10.1121/1.2216565
McKenna, M., Shannon, G., and Fristrup, K. (2016). Characterizing anthropogenic noise to improve understanding and management of impacts to wildlife. Endang. Species Res. 31, 279–291. doi: 10.3354/esr00760
McWilliam, J. N., and Hawkins, A. D. (2013). A comparison of inshore marine soundscapes. J. Exp. Mar. Biol. Ecol. 446, 166–176. doi: 10.1016/j.jembe.2013.05.012
Merchant, N. D., Blondel, P., Dakin, D. T., and Dorocicz, J. (2012). Averaging underwater noise levels for environmental assessment of shipping. J. Acoust. Soc. Am. 132, EL343–EL349. doi: 10.1121/1.4754429
Merchant, N. D., Fristrup, K. M., Johnson, M. P., Tyack, P. L., Witt, M. J., Blondel, P., et al. (2015). Measuring acoustic habitats. Methods Ecol. Evol. 6, 257–265. doi: 10.1111/2041-210X.12330
Miksis-Olds, J. L., Stabeno, P. J., Napp, J. M., Pinchuk, A. I., Nystuen, J. A., Warren, J. D., et al. (2013). Ecosystem response to a temporary sea ice retreat in the Bering Sea: winter 2009. Progr. Oceanogr. 111, 38–51. doi: 10.1016/j.pocean.2012.10.010
NIOSH (1998). Criteria for a Recommended Standard: Occupational Noise Exposure. Revised Criteria. Washington, DC: US Department of Health and Human Services, 126.
NMFS (2018). 2018 Revisions to: Technical Guidance for Assessing the Effects of Anthropogenic Sound on Marine Mammal Hearing (Version 2.0). 178.
Ocean Sound EOV (2018). Available online at: http://www.goosocean.org/index.php?option=com_oe&task=viewDocumentRecord&docID=22567 (accessed July 11, 2019).
Oppenheim, A. V., and Schafer, R. W. (2004). DSP history – From frequency to quefrency: a history of the cepstrum. IEEE Signal Process. Magazine 21, 95–106. doi: 10.1109/MSP.2004.1328092
Parks, S. E., Miksis-Olds, J. L., and Denes, S. L. (2014). Assessing marine ecosystem acoustic diversity across ocean basins. Ecol. Inform. 21, 81–88. doi: 10.1016/j.ecoinf.2013.11.003
Patek, S. N., Shipp, L. E., and Staaterman, E. R. (2009). The acoustics and acoustic behavior of the California spiny lobster (Panulirus interruptus). J. Acoust. Soc. Am. 125, 3434–3443. doi: 10.1121/1.3097760
Pieretti, N., Farina, A., and Morri, D. (2011). A new methodology to infer the singing activity of an avian community: the Acoustic Complexity Index (ACI). Ecol. Indic. 11, 868–873. doi: 10.1016/j.ecolind.2010.11.005
Pieretti, N., Martire, M. L., Farinaa, A., and Danovaro, R. (2017). Marine soundscape as an additional biodiversity monitoring tool: a case study from the Adriatic Sea (Mediterranean Sea). Ecol. Indic. 83, 13–20. doi: 10.1016/j.ecolind.2017.07.011
Pijanowski, B. C., Villanueva-Rivera, L. J., Dumyahn, S. L., Farina, A., Krause, B. L., Napoletano, B. M., et al. (2011). Soundscape ecology: the science of sound in the landscape. Bioscience 61, 203–216. doi: 10.1525/bio.2011.61.3.6
Pimm, S. L., and Lawton, J. H. (1998). Planning for Biodiversity. Science 279, 2068–2069. doi: 10.1126/science.279.5359.2068
Qiu, W., Hamernik, R. P., and Davis, R. I. (2013). The value of a kurtosis metric in estimating the hazard to hearing of complex industrial noise exposures. J. Acoust. Soc. Am. 133, 2856–2866. doi: 10.1121/1.4799813
Radford, C., Stanley, J., and Jeffs, A. (2014). Adjacent coral reef habitats produce different underwater sound signatures. Mar. Ecol. Progr. Ser. 505, 19–28. doi: 10.3354/meps10782
Radford, C., Stanley, J. A., Tindle, C. T., Montgomery, J. C., and Jeffs, A. G. (2010). Localised coastal habitats have distinct underwater sound signatures. Mar. Ecol. Progr. Ser. 401, 21–29. doi: 10.3354/meps08451
Randall, R. B. (2017). A history of cepstrum analysis and its application to mechanical problems. Mech. Syst. Signal Process. 97, 3–19. doi: 10.1016/j.ymssp.2016.12.026
Southall, B. L., Finneran, J. J., Reichmuth, C., Nachtigall, P. E., Ketten, D. R., Bowles, A. E., et al. (2019). Marine mammal noise exposure criteria: updated scientific recommendations for residual hearing effects. Aquat. Mamm. 45, 125–232. doi: 10.1578/AM.45.2.2019.125
Staaterman, E., Ogburn, M. B., Altieri, A. H., Brandl, S. J., Whippo, R., Seemann, J., et al. (2017). Bioacoustic measurements complement visual biodiversity surveys: preliminary evidence from four shallow marine habitats. Mar. Ecol. Progr. Ser. 575, 207–215. doi: 10.3354/meps12188
Staaterman, E., Paris, C. B., DeFerrari, H. A., Mann, D. A., Rice, A. N., and D’Alessandro, E. K. (2014). Celestial patterns in marine soundscapes. Mar. Ecol. Progr. Ser. 508, 17–32. doi: 10.3354/meps10911
Staaterman, E., Rice, A. N., Mann, D. A., and Paris, C. B. (2013). Soundscapes from a Tropical Eastern Pacific reef and a Caribbean Sea reef. Coral Reefs 32, 553–557. doi: 10.1007/s00338-012-1007-8
Starck, J., and Pekkarinen, J. (1987). Industrial impulse noise: crest factor as an additional parameter in exposure measurements. Appl. Acoust. 20, 263–274. doi: 10.1016/0003-682X(87)90063-6
Sueur, J., Farina, A., Gasc, A., Pieretti, N., and Pavoine, S. (2014). Acoustic indices for biodiversity assessment and landscape investigation. Acta Acust. United Acust. 100, 772–781. doi: 10.3813/AAA.918757
Sueur, J., Pavoine, S., Hamerlynck, O., and Duvail, S. (2008). Rapid acoustic survey for biodiversity appraisal. PLoS One 3:e4065. doi: 10.1371/journal.pone.0004065
Thompson, P. M., Brookes, K. L., Graham, I. M., Barton, T. R., Needham, K., Bradbury, G., et al. (2013). Short-term disturbance by a commercial two-dimensional seismic survey does not lead to long-term displacement of harbour porpoises. Proc. R. Soc. Lond. B Biol. Sci. 280:20132001. doi: 10.1098/rspb.2013.2001
Watkins, W. A., Tyack, P., Moore, K. E., and Bird, J. E. (1987). The 20-Hz signals of finback whales (Balaenoptera physalus). J. Acoust. Soc. Am. 82, 1901–1912. doi: 10.1121/1.395685
Keywords: soundscape, kurtosis, Dissimilarity Index, ocean sound, metrics, marine acoustics
Citation: Wilford DC, Miksis-Olds JL, Martin SB, Howard DR, Lowell K, Lyons AP and Smith MJ (2021) Quantitative Soundscape Analysis to Understand Multidimensional Features. Front. Mar. Sci. 8:672336. doi: 10.3389/fmars.2021.672336
Received: 25 February 2021; Accepted: 25 June 2021;
Published: 05 August 2021.
Edited by:
Ana Sirovic, Texas A&M University at Galveston, United StatesReviewed by:
Craig Aaron Radford, The University of Auckland, New ZealandMegan F. McKenna, Stanford University, United States
Copyright © 2021 Wilford, Miksis-Olds, Martin, Howard, Lowell, Lyons and Smith. This is an open-access article distributed under the terms of the Creative Commons Attribution License (CC BY). The use, distribution or reproduction in other forums is permitted, provided the original author(s) and the copyright owner(s) are credited and that the original publication in this journal is cited, in accordance with accepted academic practice. No use, distribution or reproduction is permitted which does not comply with these terms.
*Correspondence: Dylan Charles Wilford, dcw1017@wildcats.unh.edu