- 1Tvärminne Zoological Station, Helsinki University, Hangö, Finland
- 2Department of Aquatic Resources, Institute of Coastal Research, Swedish University of Agricultural Sciences, Öregrund, Sweden
- 3Department of Information Technology, Metropolia University of Applied Sciences, Espoo, Finland
Advancing our understanding of how environmental variability affects the distribution of organisms is crucial for ecology and conservation. The exploration of changes in demographic patterns close to species distribution margins is important as populations here may provide a window into future population changes also elsewhere. However, the knowledge of factors causing recruitment variation is still inadequate in many systems and this deficiency is particularly evident close to species’ distribution borders. We studied the spatiotemporal variability in recruit-adult dynamics in a blue mussel, Mytilus trossulus, population to get insights into how environmental variables drive variation in recruitment and how this variability affects adult population growth. Thirty sites along a wave exposure gradient were monitored during four consecutive years. From each site, mussels were collected both from artificial recruitment units and from natural mussel beds. Our results showed high year-to-year variation in recruitment strength with high spatial variation. Mussel recruitment to artificial units and later recruitment to the benthos correlated highly. Juvenile abundances 1 year later paralleled prior recruitment strengths and caused synchronous but time-lagged changes in adult cohorts. Seawater salinity was the strongest predictor for recruitment variation, whereas sea temperature and wave exposure had low predictive power for this early life stage. For juveniles and for adults in the benthos, wave exposure explained the variation best, whereas temperature and especially salinity explained less. The results indicate that (a) the studied blue mussel population is strongly driven by variation in recruitment strength that (b) drives the size of the later cohorts, and the population is possibly even (c) recruitment limited in some years. Our study predicts a challenging future for this range population, resulting from a higher frequency of recruitment failure caused by a deteriorating sea climate. Knowledge about factors underlying variation in recruitment is thus essential for forecasting the future of this range population and for conserving its future state.
Introduction
Questions of why population sizes fluctuate through space and time and how organisms and the environment interact to produce patterns in natural systems are central for population ecology. At the heart of providing an answer to these questions lies the understanding of the factors that shape the supply and establishment of offspring to populations (Sutherland, 1987). In open populations with complex life cycles, recruitment is the closest equivalent to local reproduction as there is often only a loose link between local fecundity and subsequent establishment of offspring (Kinlan and Gaines, 2003). Therefore, in open populations, such as among several tree species (Carnicer et al., 2014), insects (Encalada and Peckarsky, 2012), fish (Doherty and Fowler, 1994; Kimmerling et al., 2018), and marine invertebrates (Sutherland, 1987; Karlson and Levitan, 1990), recruitment supply may limit population growth and may even be more important than density-dependent factors (e.g., Okamoto et al., 2020).
More than two decades ago, Caley et al. (1996) argued that a major weakness of most contemporary studies was a lack of attention to processes affecting survival of recruits over appropriate scales of time and space. More specifically, Caley et al. (1996) suggested a deeper focus on unraveling (1) the extent to which temporal and spatial variations in recruitment underlie variations in adult populations and (2) the underlying causes behind recruitment variation. Two decades later, the understanding of these mechanisms and how they modulate populations is still poorly known in many systems (Beukema et al., 2010; Shanks et al., 2017; Okamoto et al., 2020). This indeed also concerns blue mussel research in the Baltic Sea (Liénart et al., 2021) where there has been a call for studies on how different life stages of blue mussels respond to changes in the environment (Jaatinen et al., 2021). In light of pending ecosystem change, most evidently caused by a changing climate, the understanding of population dynamics in marginal areas is becoming increasingly important. In this context, marginal areas may be especially important for predicting changes also occurring elsewhere as responses to changes in the environment are generally seen earlier at species distribution limits (e.g., Gaston, 2003).
This paper addresses three main objectives: (1) it quantifies the spatiotemporal variation in recruitment among sites and years, (2) it tests the main causes of this variation, and (3) it examines the implications of recruitment variability for population replenishment. We hypothesize that recruitment strength: (a) is positively affected by seawater salinity and wave exposure, and negatively by temperature (Westerbom et al., 2019b), and (b) is the main factor determining juvenile and adult abundance. In the lack of major predation pressure on, and low interspecific competition for blue mussels in the Baltic Sea (Kautsky, 1982), density-dependent population regulation is mainly driven by intra-specific competition for food and space (Kautsky, 1982). However, the strength of the link between early recruitment variation and population growth and structure has not been studied in this system. If the hypothesis that recruitment strength affects the abundances of later cohorts is valid, abundance patterns of juvenile and adult cohorts should reflect previous recruitment abundances to the benthos (e.g., Sutherland, 1987). In contrast, if benthic density-dependent regulation is strong, peaks in recruitment should be smoothed out by density-dependent mechanisms and the predictability of adult population size from earlier recruitment statistics should in these cases be low (Doherty and Fowler, 1994). It therefore follows; in a recruitment-limited population, population density should vary with colonization success, rather than only being structured by internal density-dependent processes in the benthos (Doherty and Fowler, 1994).
To assess the spatiotemporal variability in recruitment and maintenance of a sublittoral mussel population, and to understand the primary drivers structuring it, we conducted a 4-year survey of a blue mussel (Mytilus trossulus) population along an archipelago wave exposure gradient. Using 1,200 samples spread over 30 sites in space and 4 years in time, we monitored the establishment of recruits both on artificial substrates (600 samples) that offer comparable structures over years and sites and also through the use of benthic samples from the natural mussel bed (600 samples). We then followed up how recruit establishment subsequently influenced the natural benthic population. This allowed us to assess of how the yearly recruitment strength is transformed into benthic recruit establishment, juvenile development and finally to adult population size. The northern Baltic Proper is a well-suited testing ground for benthic recruitment biology as there are no significant benthic invertebrate predators on blue mussels modifying recruitment input into adult populations and because interspecific competition is very limited due to the low number of dominant species (Kautsky, 1982; Díaz et al., 2015; Westerbom et al., 2019a). Additionally, whereas in most systems it is practically impossible to morphologically distinguish sibling species of Mytilidae from each other in their early life stages (Rilov et al., 2008; Phillips, 2017; Sorte et al., 2017), this problem is absent in the northern Baltic Proper, where only one mussel, of the genus Mytilus, is found (e.g., Larsson et al., 2017; Kijewski et al., 2019). This is a substantial advantage as taxonomic uncertainty in many systems aggravates ecological conclusions, because species-environment interactions are species-specific (Kimmerling et al., 2018).
Materials and Methods
Study Area and Study Species
The northern Baltic Sea is tideless and characterized by brackish water with a strong seasonality in water temperature and with salinity as an overriding factor controlling the distribution of species (Vuorinen et al., 2015). Since the 1970s, seawater salinity in the studied area has been declining from an annual mean of about 6.5 PSU to less than 6.0 PSU today (Westerbom et al., 2019b). The change may be considered small, but from the perspective of the blue mussel, it is a significant deterioration in sea climate conditions as mussels in the northern Baltic Sea live close to their lower salinity tolerance, making the system a border area for blue mussel distribution (for further reading, see Westerbom et al., 2002, 2019b). The consequences of this salinity decline for specifically blue mussel recruitment patterns have not been evaluated in this system (see however Westerbom et al., 2019b; Jaatinen et al., 2021). Rocky sublittoral communities within the northern Baltic Sea are completely dominated by small blue mussels, M. trossulus, that cover 50–100% of shallow seascapes and contribute with more than 90% of the animal biomass, providing ecosystem structure, stability, and a wealth of ecosystem services (Koivisto and Westerbom, 2012; Attard et al., 2020). Blue mussels are mainly found in the depth range of 3–20 m, but these mussels dominate the rocky shore animal community down to at least 30 m depth. M. trossulus has a biphasic life stage, with a dispersive planktotrophic larval stage and a sedentary adult stage. In the northern Baltic Proper, spawning occurs in June–July, whereas settlement occurs in July–September (Kautsky, 1982; Antsulevich et al., 1999). Like in many other cold water systems, reproduction is limited to only one annual peak period (Antsulevich et al., 1999; Sokolowski et al., 2017).
Site Selection
We examined the recruitment of M. trossulus and subsequent population succession during the spring and autumn of 2004–2008 on the south-eastern archipelago area of the Hanko peninsula, Finland (59°55′ N, 23°15′ E, Figure 1). The Hanko peninsula comprises a significant archipelago area at the entrance to the Gulf of Finland and is therefore ideal for studying spatiotemporal dynamics in systems with a complex geomorphology of the coastal zone. In order to understand the drivers for recruitment in this complex archipelago area, we selected 30 rocky sites along a local wave exposure gradient that is distributed over ca 65 km2 with a perimeter of the area of ca 35 km (Westerbom and Jattu, 2006).
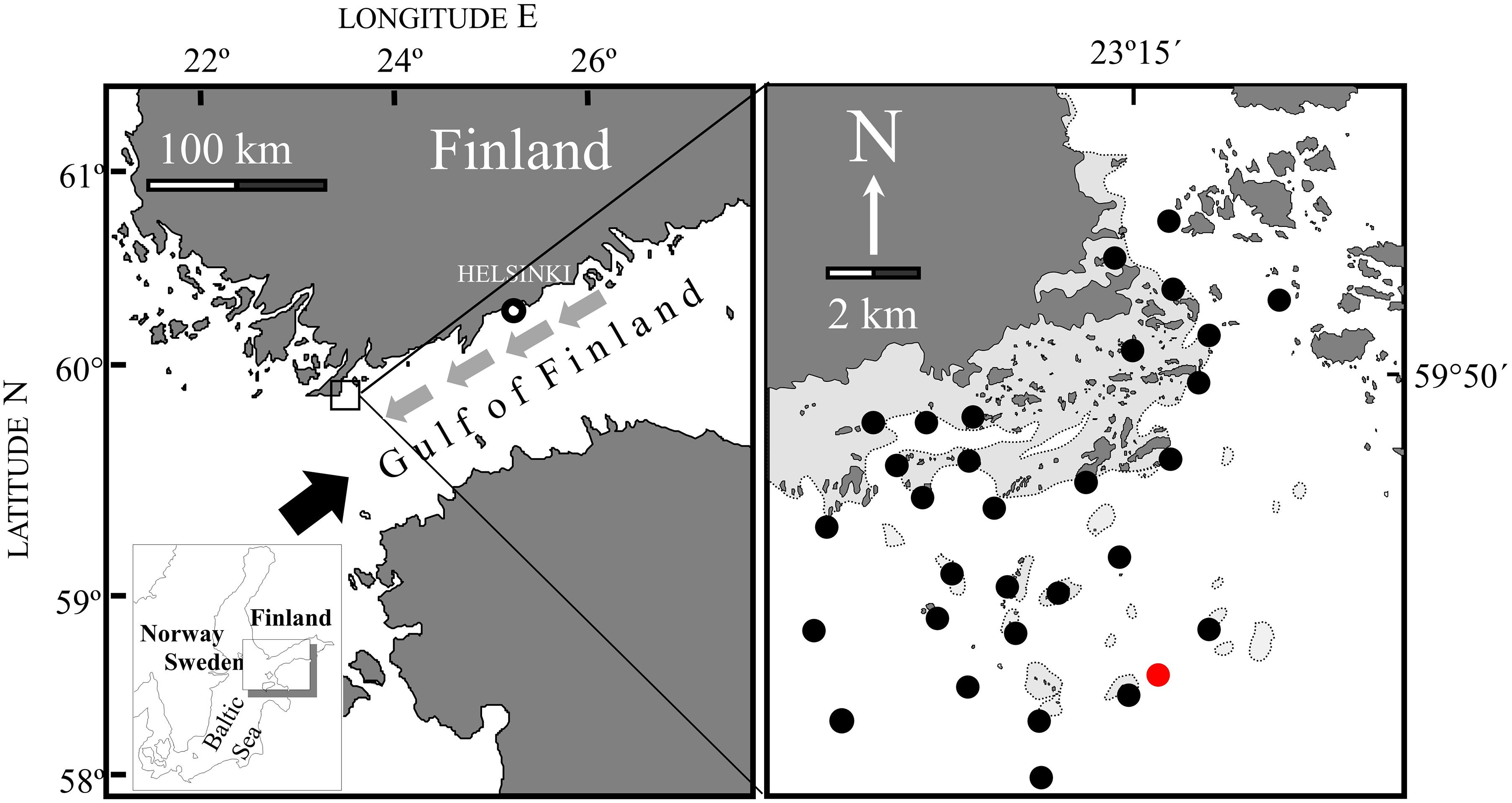
Figure 1. Study area with sampling stations. Red dot shows the position of the water monitoring station Längden. Black arrow in the left panel shows the average wind direction, whereas smaller + gray arrows indicate the direction of the average sea currents. Flanked by stations with the coordinates (WGS84) N: 59.864005445°/23.264735324°, S: 59.757547032°/23.218394607°, W: 59.791964318°/23.137171110°, and E: 59.847815912°/23.303082314°.
Sampling
Artificial Recruitment Units
We conducted two annual sampling campaigns, one at the beginning and one at the end of the reproductive season. In May 2004–2007, we placed out five spat collectors, consisting of roughed rope protruding from anchoring bricks, at 8 m depth at each site. We had roughened the polyester ropes (Ø 8 mm) on the bricks with a grinding machine to obtain a soft and hairy surface. To each brick, we attached three pieces of ropes, each 11 cm in length. Using SCUBA, we installed bricks with new ropes on the seafloor each year in May. We deployed replicates > 10 m apart at each site, making each brick an independent sample unit (all ropes on each brick were later pooled). In mid-September, we retrieved the bricks by SCUBA and we cut off three 10 cm pieces of rope and dried them for 5 days in 60°C. We then gently brushed attached recruits with a mean size of 0.6 mm off the ropes and counted these under a dissecting microscope. Occasionally, there were also mussels on the ropes that in terms of size were clear outliers. These are not young of the year recruits (Littorin and Gilek, 1999) that we therefore omitted from the counts. Finally, we thoroughly examined brushed ropes under a dissecting microscope to ensure that there were no mussels left. Mussel recruits from the collectors provide a standardized estimate of the recruitment potential in the benthic boundary layer. Similar artificial recruitment substrates have therefore routinely been used to correct for variation in benthic structures allowing for comparison among sites and years (e.g., Littorin and Gilek, 1999; Toupoint et al., 2012; Wilcox et al., 2020). To follow the timing of the recruitment process, we used control ropes at two sites. These showed that recruitment to the ropes started in August and was over by October as no significant increases or decreases were seen from September to October (see also Littorin and Gilek, 1999).
Benthic Samples
In order to provide an estimate of benthic recruitment success and recruit establishment, we collected five benthic samples per site in May 2005–2008, from 8 m depth, spaced at 10–20 m distance between replicates. At this time of the year, recruits are 8–10 months old and they have survived 6 months of winter conditions. For sampling, we used a 20 × 20 cm quadrat from which we collected all material into a bag. In the laboratory, we washed the samples through a series of successively finer mesh sieves (9.5, 4, 2, 1, and 0.5 mm) to separate size classes from each other upon which the mussels were counted. As we later pooled counts in 9.5 and 4 mm mesh sieves, this procedure provided us with four size classes of benthic mussels that regarding the two smallest sieves also differentiate year classes.
Definition of Recruits, Juveniles, and Adults
Recruits
In this study, we call mussels found on recruitment collectors (on ropes) and in the 0.5 mm mesh (from bottom samples) recruits. Recruits on the rope had an average size of 0.6 mm, whereas benthic mussels found in the smallest mesh sieve are less than 2 mm long. Benthic recruits are here equivalent in age to those defined by e.g., Beukema et al. (2010) and they are 8–10 months old.
Juveniles
We operationally define juveniles as benthic mussels found within mesh sieve 1 mm. These juveniles have survived two winters in the benthos during which they have reached a size of ca 2–4 mm in length.
Adults
We operationally call benthic mussels in mesh sieve 2 mm as small adults, whereas we call mussels found in the two larger mesh sieves large adults. Mussels in mesh sieve 2 mm varied in length between 4–8 mm, whereas mussels in mesh sieve 4 and 9.5 mm are larger than 8 mm (the blue mussels can reach a maximum length of ca 35 mm in the study area).
Environmental Data
To account for spatial variability and the lack of environmental monitoring data from each of the 30 stations, we extracted data interpolated from coastal data models, hosted by the Finnish Environment Institute. These data included sea surface salinity and sea surface temperature. To account for annual differences among years and stations, we used actual and frequent monitoring data from the Längden water monitoring station, which is located within the study area (Figure 1) and adjusted annual values for the individual stations according to the proportional differences among stations from the models. Environmental data are gathered 2–3 times per month at the Längden monitoring station. For salinity, we used June–August values as this period encompasses the time for blue mussel breeding, dispersing, settlement and recruitment. The processes of metamorphosis and settlement constitute a dramatic ecological shift in mussels when they are sensitive to abnormal environmental conditions (e.g., Jenewein and Gosselin, 2013). For temperature, we used only mean values from August as it was hypothesized that only summer temperature highs would limit propagule survivorship (Fly and Hilbish, 2013; Westerbom et al., 2019b) and August coincides with the upper yearly temperature maximum in this area. For station-specific wave exposure, we used the Isaeus wave exposure index modified by Bekkby et al. (2008). The wave exposure model is a GIS based model that includes shoreline topography, fetch, wind data and depth in estimating the wave exposure for each site and depth.
Data Analysis
We ran parametric one-way ANOVAs whenever possible to test for annual changes in recruitment on ropes, as well as for testing changes in different cohorts in the benthos. In most cases, we transformed variables by log (x + 1) or square root (x + 1) to meet assumptions of normal distribution and homogeneity of variances. In case these assumptions were not met, we used Kruskal–Wallis tests. We used the Pearson correlation to evaluate possible correlations between different life-history stages. All samples in this study were balanced and included a total of 600 natural benthic and 600 artificial/experimental rope samples distributed over 30 sites and 4 years.
We assessed the individual association between five different response variables, i.e., recruits on ropes in autumn, spring samples of benthic recruits, benthic juveniles, benthic small adults and benthic large adults, and three to four explanatory environmental variables (depending on context) using distance-based linear modeling, DISTLM (Legendre and Anderson, 1999; McArdle and Anderson, 2001). The DISTLM analyses are based on a resemblance matrix that shows the level of similarity in abundance among sampled stations. We entered the response variables untransformed as individual counts and we added a dummy variable of one in order to cope with empty samples. We normalized the environmental variables prior to analyses.
In evaluating the influence of the environmental variables, we used the “BEST” option (Clarke and Gorley, 2006). BEST identifies a series of alternative models including an increasing number of explanatory variables. Then, the most parsimonious model among these is identified by the Akaike information criterion (AIC). Alternative models differing from each other with less than 2 units are identified as potential parallel models, and in these cases, the models with the lower number of variables included are preferred (Burnham and Anderson, 2002). We identified the individual relationship of each environmental variable to the registered mussel densities in each size class based on marginal F tests. We performed the DISTLM analyses in PERMANOVA + as implemented in PRIMER software version 7 (Anderson, 2005; Anderson et al., 2008).
Spatial Analysis for Recruitment
In order to estimate and visualize the spatial variability in recruitment among islands and over the archipelago, we ran spatially explicit analyses based on INLA (Integrated Nested Laplacian Approximation) combined with GLMM modeling. We built spatial models for recruits on the rope (model I) and for recruits in the benthos (model II). We chose INLA as it is able to handle spatial dependency in the calculation of parameters explaining recruitment variation in space. For modeling, we used the Gaussian random field (GRF). GRF is a collection of spatial random variables where the samples occur in a spatially continuous domain and therefore exhibit distance-based relationships (Moraga, 2019). To deal with these relationships, we used the stochastic partial differential equation approach (SPDE) and implemented it in the Bayesian R-INLA package (for closer description of the model, see Supplementary Material).
Results
Recruitment Variability in Time
During the four study years, the average seawater salinity differed considerably, being high in 2004 (6.0 ± 0.3), low in 2005 (5.5 ± 0.1) and 2006 (5.4 ± 0.5), and again high in 2007 (5.9 ± 0.2, mean ± SD). Seawater temperature in August (mean ± SD) showed very small variations among years (16.6 ± 2.9, 16.9 ± 2.0, 16.9 ± 2.1, 16.8 ± 1.9, for the respective years 2004–2007). Wind patterns differed among years. In 2004, there were relatively calm W–SSE winds. In 2005, SW winds were common. In 2006, the wind direction was variable and characterized by strong eastern, moderately southern and calm winds from the west. Finally, in 2007, strong winds from WSW–SW alongside with strong eastern winds were common (Figure 2).
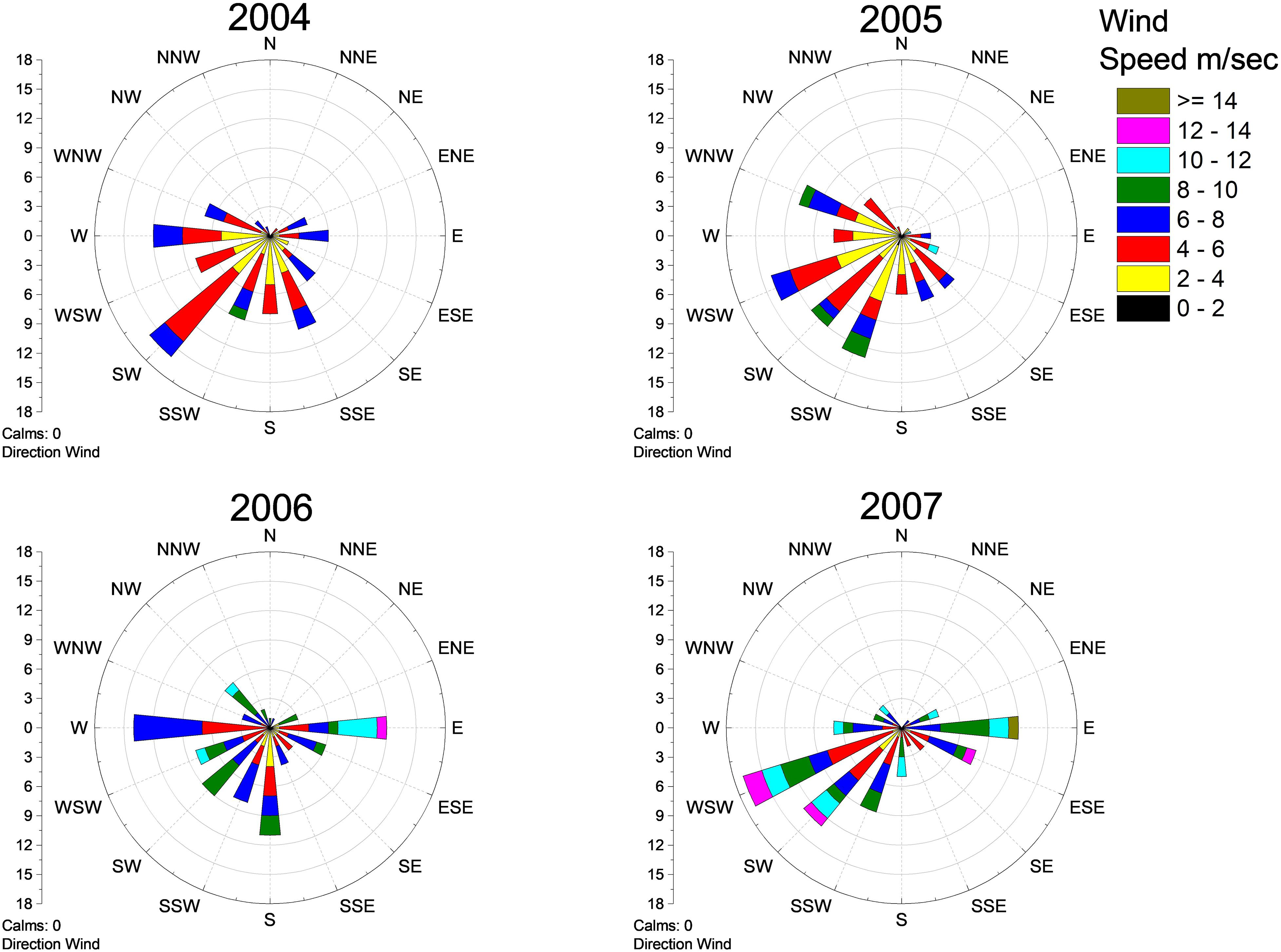
Figure 2. Wind roses from Hanko Russarö weather station (10 km west from the study area) showing wind patterns between June and August during the 4 years, 2004–2007.
In 2004, the recruitment rate of M. trossulus was extremely high to the artificial substrates throughout the archipelago area, whereas it was more than one order of magnitude weaker in 2005 and in 2006. In 2007, the recruitment rate was again high (Figure 3). These differences were highly significant among years (Kruskal–Wallis H3 = 435.8, P < 0.001) and the changes in recruitment to the artificial units reflected parallel changes in sea salinity (Figure 3).
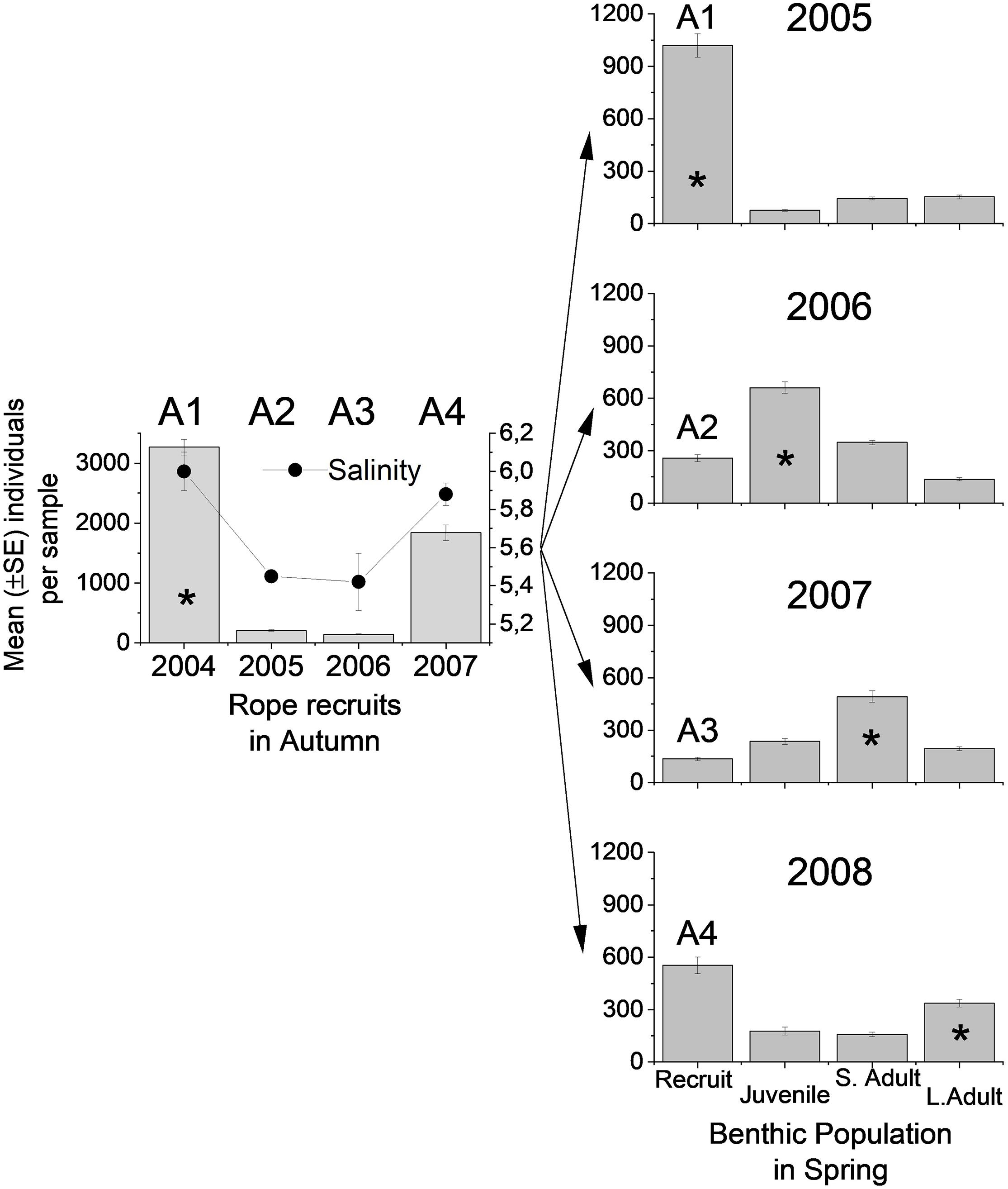
Figure 3. Mean abundance of different mussel size classes (±SE) during four years. Left panel shows recruit abundance on artificial units (ropes) in autumn 2004–2007 and mean (±SE) summer salinity. Right panel shows the structure of the benthic population during 4 years, sampled in spring each year 2005–2008. The star exemplifies how the recruits of 2004 have traveled through the different cohorts. A1–A4 combine the left and right panel and visualizes how similar the recruitment pulses were on the collectors and in the benthos.
Recruitment success to the benthos differed among years (ANOVA F3,596 = 114.3, P < 0.001) and showed a strong positive correlation with recruits on ropes 9 months earlier (Figure 3, Pearson r = 0.7, N = 600, P < 0.001). Juvenile abundances also varied strongly among years (Kruskal–Wallis H3 = 216.3, P < 0.001, Figure 3) and they correlated strongly with benthic recruits 12 months earlier (Pearson r = 0.81, N = 450, P < 0.001). In 2005, recruitment to the benthos was extremely high and caused a nearly nine-fold increase in juvenile abundance 1 year later. The pulse of new recruits had, however, wider consequences as the number of small adults correlated positively with the number of juveniles found in the benthos the previous year (Pearson r = 0.6, N = 450, P < 0.001). Also, small adults showed highly significant changes in abundance among years (Kruskal–Wallis H3 = 130, P < 0.001). Large adult mussels showed clear year effects (ANOVA F3,596 = 8.2, P < 0.001) with abundances in 2008 differing from the abundances in the previous years. In 2005–2007, there were no differences in adult abundance among years. Hence, the pulse of the new recruits in 2004 traveled through the population and manifested itself in the adult population of 2008 at the end of the study.
Factors Affecting Recruitment to Artificial Units in Autumn and to the Benthos in Spring
Using the explanatory variables salinity, seawater temperature, wave exposure, and number of adult mussels, we ran two DISTLMs to evaluate the roles of these factors for the recruitment to artificial units in autumn and for the recruitment to benthos in spring. The BEST model for both analyses included all four variables and marginal tests showed that all variables were highly significant (Table 1). For recruitment to ropes in autumn, 53.8% of the total variation in the data cloud was explained with salinity as the most influential factor, explaining 51.7% of the total variation. The other three variables explained less than 7% individually (Table 1).
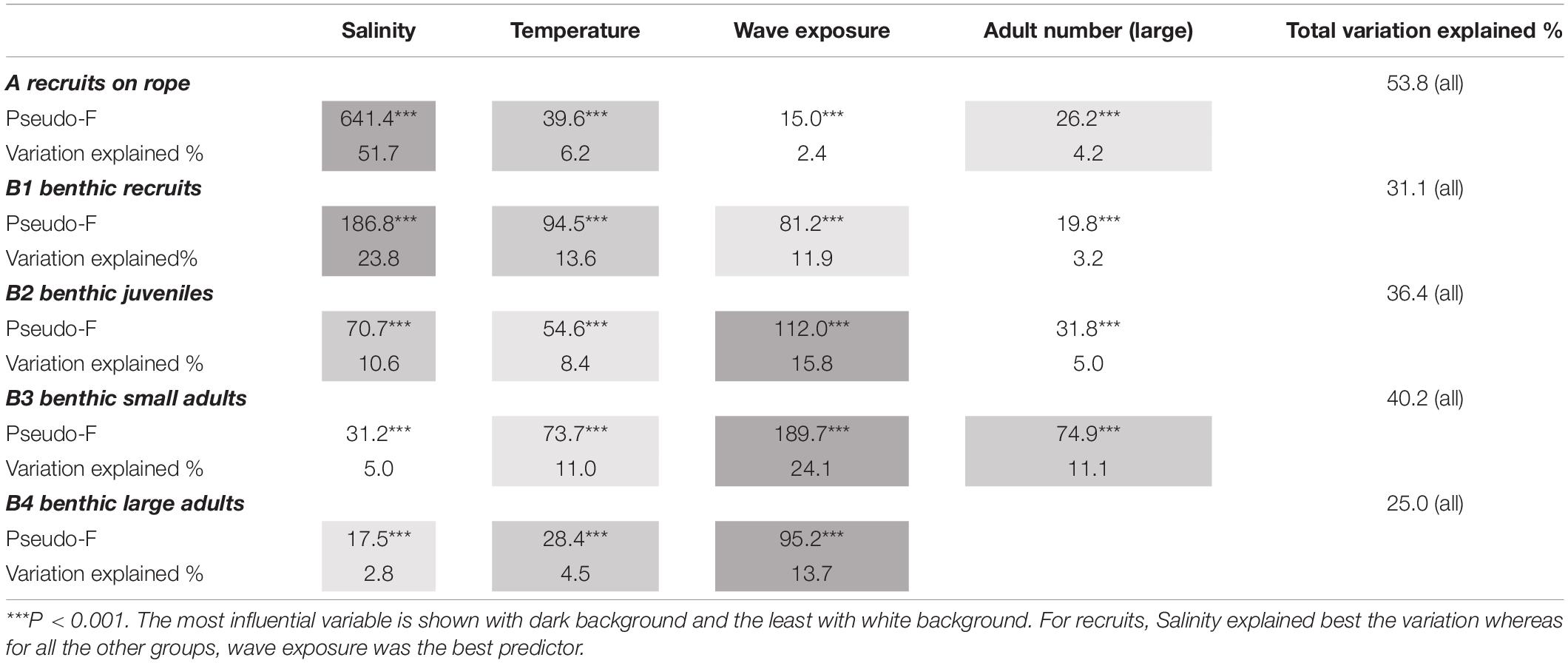
Table 1. Relationship between number of mussels within different groups and registered environmental variables according to five DISTLMs.
For the recruitment to the benthos in spring, 31.1% of the total variation in the data cloud was explained and salinity was again the single variable that was the most influential factor. However, the explanatory power of salinity for the benthic recruits in spring was clearly lower (23.8%) than for the recruits in autumn whereas water temperature (13.6%) and wave exposure (11.9%), now played a greater role (Table 1).
Factors Affecting the Number of Juveniles and Adults in the Benthos in Spring
To examine which factors that were driving the number of benthic juveniles and small and large adults in spring, we ran three DISTLMs using the explanatory variables salinity, seawater temperature, wave exposure, and number of adult mussels (the latter factor only for juveniles and small adults). The BEST model for all three analyses included all applied variables and marginal tests showed that all variables were highly significant (Table 1). For benthic juveniles, 36.4% of the total variation in the data cloud was explained. Marginal tests identified wave exposure as the single variable that was most strongly attributed to changes in this data (15.8%) followed by salinity (10.6%) and temperature (8.4%) (Table 1).
For benthic small adults, 40.2% of the total variation in the data cloud was explained by the model. Marginal tests identified that wave exposure was clearly the most important variable (24.1%) followed by number of adult mussels (11.1%) and seawater temperature (11.0%), while salinity (5.0%) had a marginal effect (Table 1). For benthic large adults, 25.0% of the total variation in the data cloud was explained and marginal tests showed that wave exposure was clearly the most important variable (13.7%), while seawater temperature (4.5%) and salinity (2.8%) had very low explanatory power (Table 1).
Recruitment Variability in Space
The INLA models showed that recruits were not homogeneously spaced over the archipelago and there were strong year effects in their spatial distribution (Figure 4). In 2004, when salinity and recruitment were very high, we observed a peak recruitment on ropes in the inner archipelago. In contrast, during years with low recruitment (2005 and 2006), recruitment to the ropes was more evenly spaced over the entire archipelago gradient. In 2007, when seawater salinity was high again, we observed the highest recruitment in the outer archipelago. The model confirmed the DISTLM results and indicated that salinity had a positive effect on recruitment, whereas temperature and wave exposure showed weak negative effects (Supplementary Material). Benthic recruits also showed a patchy distribution, with clear year effects that mirrored the results from the artificial collectors. The model confirmed the DISTLM results, but in the model, the density of adult mussels did not explain variation in benthic recruitment.
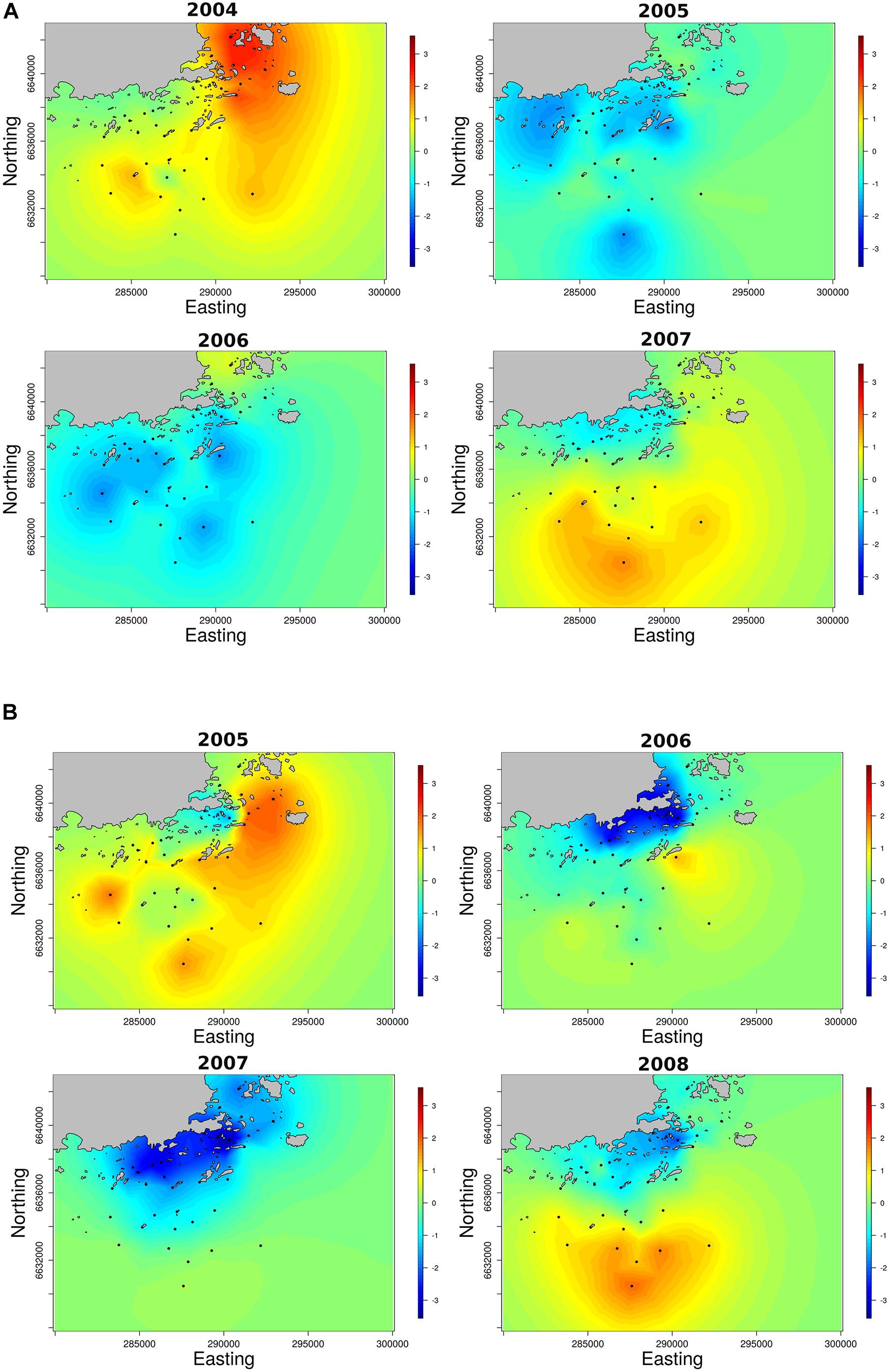
Figure 4. Spatiotemporal distribution of recruits collected from (A) experimental brick collectors in fall and from (B) the benthos in the following spring. Panels show the posterior Gaussian random fields (w) for each year in natural log scale. Positive and negative values indicate areas where the recruitment was strong or weak, respectively.
Discussion
In the larger context of identifying processes that structure blue mussel populations living at the lower limit of their salinity tolerance (e.g., Westerbom et al., 2019b; Jaatinen et al., 2021), this study contributes to the understanding of range ecology by providing insights into environmental factors that drive recruitment processes and by showing how recruitment pulses manifest into adult population structure. In line with the working hypotheses, (1) we demonstrate that fluctuations in ambient seawater salinity ultimately facilitates or obstructs the size of the blue mussel population in this range area by affecting the yearly recruitment strength. We also show that (2) peaks and dips in recruitment clearly affect the size of later cohorts and, thus, fluctuating recruitment causes synchronous but time-lagged changes in the overall population density and structure (van der Meer et al., 2001; Beukema et al., 2010; Beukema and Dekker, 2014). Finally, (3) the results suggest that recruit abundance is highly stochastic over the archipelago. This stochasticity is both temporal, showing large inter-annual variations, but it is also spatial, showing inconsistent patterns of highs and lows with regard to recruitment success over the archipelago area.
Ecological research often assumes that recruits are in plentiful supply and that population size is mainly determined by post-recruitment density-dependent interactions (c.f. Underwood and Keough, 2001). However, many studies have shown that variability in recruitment success is in fact a key driver for later population growth, and for the stability of populations (Roughgarden et al., 1988; Westerbom et al., 2019b; Okamoto et al., 2020; Wilcox et al., 2020). Nevertheless, the patterns and causes of spatial and temporal heterogeneity in recruitment processes have often remained elusive (Caley et al., 1996; Broitman et al., 2008; Rilov et al., 2008; Sokolowski et al., 2017). By using artificial recruitment units that are homogeneous in structure, we were able to record high temporal variation in early recruitment that adequately predicted later recruitment strength to the benthos. We further showed that this variation was reflected in the size of juvenile and adult cohorts 12–24 months later (Figure 3). At the same time, DISTLM showed that numbers of adult mussels only weakly explained recruitment (Table 1). These results suggest that regulation mechanisms are not strong enough to erase recruitment signals (this concerns both strong and weak signals) thus suggesting a recruitment driven system. Jaatinen et al. (2021) showed only weak effects of density-dependency in these mussel beds and corroborate this interpretation. The strong coherence between recruits in autumn on artificial units and later recruits in the benthos in spring across years and sites, as well as the clear relation between high and low recruitment on later life-history stages, suggest that recruitment is a key process per se for determining population size in this system. This notion does not preclude potential effects of inter- or intraspecific interactions in the plankton, or during the early post-recruitment, but it suggests that populations during some years are under-saturated with established recruits, possibly even recruitment limited (c.f. Karlson and Levitan, 1990; Palardy and Witman, 2014). In contrast to the “recruit–adult” hypothesis (Menge, 2000), which suggests that recruitment density is a good predictor of adult density when low, but not when high, early and later recruitment in this study predicted later cohorts well, also during high recruitment years. This raises two questions: (1) what are the causes behind recruitment fluctuation and the resulting variations in later life-history stages and (2) what are the consequences of this variation for the future population development in a changing Baltic Sea?
Our inferences on the drivers behind spatiotemporal changes in recruitment, and the subsequent changes in later life-history stages are correlative. Consequently, we did not design this study to provide mechanistic explanations of cause and effect relationships. Patterns were however clear, and they are corroborated by a number of recent studies. On a regional scale, shifts in population structure that follow geographical variations in salinity means have been shown (Westerbom et al., 2002, 2019a,b; Nyström Sandman et al., 2013; Kotta et al., 2015), but it has remained unclear which life stage that is the most sensitive one to such salinity changes (Jaatinen et al., 2021). The results of this study indicate that summer salinity, i.e., including the period of fertilization, pelagic drift, metamorphosis and settlement of blue mussels is a key driver that affects the recruitment potential in this system. Westerbom et al. (2019b) showed that an anomaly in sea salinity that remained high throughout the spring 1997 triggered a massive, homogeneous and synchronous recruitment event over the entire northern Gulf of Finland. This massive recruitment event elicited population growth in three distinct populations that persisted much longer at the high salinity end of the Gulf. Westerbom et al. (2019b) also identified a non-linear relationship between salinity on the one hand and population endurance, stability and biomass on the other. Over a short geographical distance where salinity declined by merely 0.5 PSU, there was a clear transition in Mytilus biomass that persisted through time. The existence of a tipping point in salinity is supported by findings in this study where we show that high recruitment to both the artificial units and to the benthos is associated with years when salinity conditions are high (closer or above 6 PSU). Completely the opposite patterns are shown during years when salinity levels are close to or below 5.5 PSU, which represent the lower boundary in long-term averaged salinity records. Hence, changes in recruitment are not driven by factors that operate predominantly on small scales, such as e.g., predation, competition bottom topography or structure, but they are driven by factors that operate on archipelago wide, or larger, scales. Another finding, revealed by the DISTLM was that salinity systematically affected younger cohorts more than older cohorts, whereas once established, wave exposure seemed to affect later cohorts more than the earlier ones, and salinity had a low effect for these mussels.
Recent studies by Westerbom et al. (2019b), Jaatinen et al. (2021), and Liénart et al. (2021) have indicated negative effects of gradually increasing seawater temperatures on population dynamics of Baltic Sea blue mussels. Even if temperature would be a general archipelago wide factor that should affect recruitment strength, we could only find a low negative effect of temperature on recruitment strength and later cohorts. This weak response is likely a result of very small yearly differences in seawater temperature during the years of this study and a lack of extreme highs in 2004–2008. Changes in phytoplankton composition have been shown to influence mussel dynamics over decadal scales (Liénart et al., 2021). A transition in phytoplankton composition or food amount would unlikely explain the stochastic changes in recruitment and population development that we documented here and that took place over a relatively short time-span and during a period when no apparent changes in inter-annual Chl-a concentrations could be seen (data from Finnish Environment Institute). Regional circulation patterns and upwelling events have been considered important for driving the supply of planktotrophic larvae and year class strengths in benthic populations (Porri et al., 2006; Palardy and Witman, 2014). Changes in hydrodynamics can affect the strength of yearly recruitment, but also their spatial patchiness. Since the production of offspring and delivery of recruits likely are decoupled from local population size (Kinlan and Gaines, 2003), the persistence of adult populations critically depends on external transport mechanisms that deliver larvae to adult populations (Caley et al., 1996; Wilcox et al., 2020). Elucidating the effects of transport mechanisms are beyond the scope of this study, but transport mechanisms and their seasonal frequency and stochasticity are possibly important when explaining year-to-year variability in recruitment success and their spatial patchiness that was seen in this study (e.g., Gaylord and Gaines, 2000; Porri et al., 2006; Rilov et al., 2008; Reaugh-Flower et al., 2011).
There is circumstantial evidence in the data suggesting that delivery of recruits may be an important factor determining spatial variation in recruitment in this system. The recruitment patterns in the archipelago showed strong year effects but no spatial consistency (Figure 4). Such chaotic distribution could be driven by erratic changes in surface and near-surface currents, whose direction and strength is driven primarily by wind (e.g., Delpeche-Ellmann et al., 2016). Meteorological forcing may therefore leave a chaotic fingerprint in recruitment patterns in the Baltic Sea, where tides are non-existent and where surface current direction and speed are determined by the wind. Upwelling events may also have contributed to the observed patterns. During upwelling, the water surface layer where pelagic mussels are entrained moves seaward in the Ekman layer (Delpeche-Ellmann et al., 2016), which may reduce larval availability nearshore. During moderate and low recruitment years, higher abundances of recruits were found in the seaward side of the study area, which may be an imprint of the effects of transport mechanisms at open sea driven by regional upwelling events or by general geographical (metapopulation) transport processes. Geographical transport, especially if the studied population is a sink-population (Pulliam, 1988) would explain the regional pattern with generally more recruits seaward and less landward (as seen in Jaatinen et al., 2021). The Tvärminne archipelago opens to the south-west and the predominating wind direction is from south-west (Figures 1, 2). Possibly, the complex geomorphology of the archipelago filters arriving larvae and causes higher settlement at sites closer to the geographical transport route at the open sea. In summer, global wind patterns are weaker and local wind patterns are stronger, causing a highly variable wind pattern (Delpeche-Ellmann et al., 2016 with references), which may explain the opposite recruitment patterns seen, e.g., in 2004 when wind speed was comparatively low. As shown by Jaatinen et al. (2021), blue mussel populations in the outer archipelago are much more stable over time compared to those closer to the mainland. While many factors may contribute to this pattern, the higher population stability offshore may mirror a higher temporal stability in recruitment, which may be a result of a more steady supply of larvae.
Conclusion
A commonly accepted view is that marginal populations living near the limit of their geographical range show higher sensitivity to stress, greater probability of local reproductive failure and a higher probability of recruitment limitation (e.g., Karlson and Levitan, 1990). We show here that recruitment strength varies highly in this marginal population and effects of recruitment variability is seen later in the adult cohorts. We also show that the number of recruits is affected by a fluctuating salinity. Hydrological changes in the Baltic Sea are among the fastest recorded globally (Belkin, 2009; Reusch et al., 2018) where negative trends in salinity development and positive trends in the evolution of water temperature have been shown (Westerbom et al., 2019b). As all evidence here indicates that fluctuating background conditions are the most important drivers for recruitment dynamics in this area, then alterations in climate may critically amend future patterns of mussel distribution through changes in recruitment success (Vuorinen et al., 2015; Westerbom et al., 2019b). Such climate driven changes in mussel abundance or distribution have been reported globally (e.g., Beukema and Dekker, 2014; Sorte et al., 2017) and those changes have been advocated to water temperature rise. This study emphasizes the importance of salinity changes as a key mechanism that through recruitment drives this marginal mussel population and predicts a challenging future enforced by ongoing climate change that is lowering the salinity of the Baltic Sea and raising its seawater temperature (see e.g., Westerbom et al., 2019b; Jaatinen et al., 2021). The present study provides an initial step for a better understanding of the implications of a decreased salinity caused by ongoing climate change on the recruitment biology of Baltic blue mussels and it provides insight into how marginal species respond to large-scale ecosystem change. Information from simplified systems and especially those from marginal areas, characteristics which both are true for the current study area, provide important insights into how climate change may reallocate species distributions or affect their abundance structure also in their current central areas of distribution that may become peripheral when conditions change (e.g., Sagarin et al., 2006). The conditions in the marginal areas may then serve as time machines for the study of larger-scale changes in an exercise of “space-for-time” substitution (Blois et al., 2013; Reusch et al., 2018).
Data Availability Statement
The raw data supporting the conclusions of this article will be made available by the authors, without undue reservation.
Ethics Statement
This study was carried out in accordance with the current laws in Finland. There are no legal or ethical restrictions involving benthic invertebrates.
Author Contributions
MW designed and led the writing of this study. MW and OM executed the sampling. MW and PK did the non-spatial statistical analyses. ED did the Bayesian spatial modeling (INLA). All authors contributed critically to the drafts and gave final approval for publication
Funding
This study was provided by the EU-project AC 340165 (MW), the Walter and Andrée de Nottbecks Foundation (MW), the Svenska Kulturfonden (MW), and the Academy of Finland, project number 126831 (MW).
Conflict of Interest
The authors declare that the research was conducted in the absence of any commercial or financial relationships that could be construed as a potential conflict of interest.
Acknowledgments
Avi Rahman is thanked for assistance in the lab. We are grateful to Elina Virtanen at the Finnish Environment Institute for proving us with the wave exposure data.
Supplementary Material
The Supplementary Material for this article can be found online at: https://www.frontiersin.org/articles/10.3389/fmars.2021.659556/full#supplementary-material
References
Anderson, M. J. (2005). Permutational Multivariate Analysis of Variance. Auckland: Department of Statistics, University of Auckland.
Anderson, M. J., Gorley, R. N., and Clarke, R. K. (2008). PERMANOVA + for PRIMER: Guide to Software and Statistical Methods. Plymouth: PRIMER-E.
Antsulevich, A., Maximovich, N., and Vuorinen, I. (1999). Population structure growth and reproduction of the common mussel (Mytilus edulis L.) off the Island of Seili (SW Finland). Boreal Environ. Res. 4, 367–375.
Attard, K., Rodil, I., Berg, P., Mogg, A. O. M., Westerbom, M., Norkko, A., et al. (2020). Metabolism of a subtidal rocky mussel reef in a high-temperate setting: pathways of organic C flow. Mar. Ecol. Prog. Ser. 645, 41–54. doi: 10.3354/meps13372
Bekkby, T., Isachsen, P. E., Isæus, M., and Bakkestuen, V. (2008). GIS Modeling of wave exposure at thesSeabed: a depth-attenuated wave exposure model. Mar. Geodesy 31, 117–127. doi: 10.1080/01490410802053674
Belkin, I. M. (2009). Rapid warming of large marine ecosystems. Prog. Oceanogr. 8, 207–213. doi: 10.1016/j.pocean.2009.04.011
Beukema, J. J., and Dekker, R. (2014). Variability in predator abundance links winter temperatures and bivalve recruitment: correlative evidence from long-term data in a tidal flat. Mar. Ecol. Prog. Ser. 513, 1–15. doi: 10.3354/meps10978
Beukema, J. J., Dekker, R., and Philippart, C. J. M. (2010). Long-term variability in bivalve recruitment, mortality, and growth and their contribution to fluctuations in food stocks of shellfish-eating birds. Mar. Ecol. Prog. Ser. 414, 117–130. doi: 10.3354/meps08706
Blois, J. L., Williams, J. W., Fitzpatrick, M. C., Jackson, S. T., and Ferrier, S. (2013). Space can substitute for time in predicting climate-change effects on biodiversity. Proc. Natl. Acad. Sci. U.S.A. 110, 9374–9379. doi: 10.1073/pnas.1220228110
Broitman, B. R., Blanchette, C. A., Menge, B. A., Lubchenco, J., Krenz, C., Foley, M., et al. (2008). Spatial and temporal patterns of invertebrate recruitment along the West Coast of the United States. Ecol. Monogr. 78, 403–421. doi: 10.1890/06-1805.1
Burnham, K. P., and Anderson, D. R. (2002). Model Selection and Multi-Model Inference: a Practical Information-Theoretic Approach, 2nd Edn. New York, NY: Springer.
Caley, M. J., Carr, M. H., Hixon, M. A., Hughes, T. P., Jones, G. P., and Menge, B. A. (1996). Recruitment and the local dynamics of open marine populations. Annu. Rev. Ecol. Syst. 27, 477–500. doi: 10.1146/annurev.ecolsys.27.1.477
Carnicer, J., Coll, M., Pons, X., Ninyerola, M., Vayreda, J., and Penuelas, J. (2014). Large-scale recruitment limitation in Mediterranean pines: the role of Quercus ilex and forest successional advance as key regional drivers. Glob. Ecol. Biogeogr. 23, 371–384. doi: 10.1111/geb.12111
Delpeche-Ellmann, N., Torsvik, T., and Soomere, T. (2016). A comparison of the motions of surface drifters with offshore wind properties in the Gulf of Finland, the Baltic sea. Estuar. Coast. Shelf Sci. 172, 154–164. doi: 10.1016/j.ecss.2016.02.009
Díaz, E. R., Erlandsson, J., Westerbom, M., and Kraufvelin, P. (2015). Depth-related spatial patterns of sublittoral blue mussel beds and their associated macrofaunal diversity revealed by geostatistical analyses. Mar. Ecol. Prog. Ser. 540, 121–134. doi: 10.3354/meps11461
Doherty, P., and Fowler, T. (1994). An empirical test of recruitment limitation in a coral reef fish. Science 263, 935–939. doi: 10.1126/science.263.5149.935
Encalada, A. C., and Peckarsky, B. L. (2012). Large-scale manipulation of mayfly recruitment affects population size. Oecologia 168, 967–976. doi: 10.1007/s00442-011-2147-1
Fly, E. K., and Hilbish, T. J. (2013). Physiological energetics and biogeographic range limits of three congeneric mussel species. Oecologia 172, 35–46. doi: 10.1007/s00442-012-2486-6
Gaston, K. J. (2003). The Structure and Dynamics of Geographic Ranges. Oxford: Oxford University Press.
Gaylord, B., and Gaines, S. D. (2000). Temperature or transport? Range limits in marine species mediated solely by flow. Am. Nat. 155, 769–789. doi: 10.1086/303357
Jaatinen, K., Westerbom, M., Norkko, A., Mustonen, O., and Koons, D. N. (2021). Detrimental impacts of climate change may be exacerbated by density dependent population regulation in blue mussels. J. Anim. Ecol. 90, 562–573. doi: 10.1111/1365-2656.13377
Jenewein, B. T., and Gosselin, L. A. (2013). Ontogenetic shift in stress tolerance thresholds of Mytilus trossulus: effects of desiccation and heat on juvenile mortality. Mar. Ecol. Prog. Ser. 481, 147–159. doi: 10.3354/meps10221
Karlson, R. H., and Levitan, D. R. (1990). Recruitment-limitation in open populations of Diadema antillarum: an evaluation. Oecologia 82, 40–44. doi: 10.1007/BF00318531
Kautsky, N. (1982). Quantitative studies on the gonad cycle, fecundity, reproductive output and recruitment in a Baltic Mytilus edulis L. population. Mar. Biol. 68, 143–168. doi: 10.1007/BF00397601
Kijewski, T., Zbawicka, M., Strand, J., Kautsky, H., Kotta, J., Ratsep, M., et al. (2019). Random forest assessment of correlation between environmental factors and genetic differentiation of populations: case of marine mussels Mytilus. Oceanologia 61, 131–142. doi: 10.1016/j.oceano.2018.08.002
Kimmerling, N., Zuqert, O., Amitai, G., Gurevich, T., Armoza-Zvuloni, R., Kolesnikov, I., et al. (2018). Quantitative species-level ecology of reef fish larvae via metabarcoding. Nat. Ecol. Evol. 2, 306–316. doi: 10.1038/s41559-017-0413-2
Kinlan, B. P., and Gaines, S. D. (2003). Propagule dispersal in marine and terrestrial environments: a community perspective. Ecology 84, 2007–2020. doi: 10.1890/01-0622
Koivisto, M., and Westerbom, M. (2012). Invertebrate communities associated with blue mussel beds in a patchy environment: a landscape ecology approach. Mar. Ecol. Prog. Ser. 471, 101–110. doi: 10.3354/meps10010
Kotta, J., Oganjan, K., Lauringson, V., Pärnoja, M., Kaasik, A., Rohtla, L., et al. (2015). Establishing functional relationships between abiotic environment, macrophyte coverage, resource gradients and the distribution of Mytilus trossulus in a brackish non-tidal environment. PLoS One 10:e0136949. doi: 10.1371/journal.pone.0136949
Larsson, J., Lind, E. E., Corell, H., Grahn, M., Smolarz, K., and Lönn, M. (2017). Regional genetic differentiation in the blue mussel from the Baltic Sea area. Estuar. Coast. Shelf Sci. 195, 98–109. doi: 10.1016/j.ecss.2016.06.016
Legendre, P., and Anderson, M. J. (1999). Distance-based redundancy analysis: testing multispecies responses in multifactorial ecological experiments. Ecol. Monogr. 69, 1–24. doi: 10.1890/0012-9615(1999)069[0001:dbratm]2.0.co;2
Liénart, C., Garbaras, A., Qvarfordt, S., Sysoev, A. Ö, Höglander, H., Walve, J., et al. (2021). Long−term changes in trophic ecology of blue mussels in a rapidly changing ecosystem. Limnol. Oceanogr. 66, 694–710. doi: 10.1002/lno.11633
Littorin, B., and Gilek, M. (1999). A photographic study of the recolonization of cleared patches in a dense population of Mytilus edulis in the northern Baltic proper. Hydrobiologia 393, 211–219. doi: 10.1023/A:1003565602858
McArdle, B. H., and Anderson, M. J. (2001). Fitting multivariate models to community data: a comment on distance-based redundancy analysis. Ecology 82, 290–297. doi: 10.1890/0012-9658(2001)082[0290:fmmtcd]2.0.co;2
Menge, B. A. (2000). Recruitment vs. post-recruitment processes as determinants of barnacle population abundance. Ecol. Monogr. 70, 265–288. doi: 10.1890/0012-9615(2000)070[0265:rvppad]2.0.co;2
Moraga, P. (2019). Geospatial Health Data: Modeling and Visualization with R-INLA and Shiny. London: Chapman and Hall/CRC Biostatistics Series.
Nyström Sandman, A., Wikström, S. A., Blomqvist, M., Kautsky, H., and Isaeus, M. (2013). Scale-dependent influence of environmental variables on species distribution: a case study on five coastal benthic species in the Baltic Sea. Ecography 36, 354–363. doi: 10.1111/j.1600-0587.2012.07053.x
Okamoto, D. K., Schroeter, S. C., and Reed, D. C. (2020). Effects of ocean climate on spatiotemporal variation in sea urchin settlement and recruitment. Limnol. Oceanogr. 65, 2076–2091. doi: 10.1002/lno.11440
Palardy, J. E., and Witman, J. D. (2014). Flow, recruitment limitation, and the maintenance of diversity in marine benthic communities. Ecology 5, 286–297. doi: 10.1890/12-1612.1
Phillips, N. E. (2017). Variability in early post-settlement mortality in intertidal mussels and the role of size at settlement. Mar. Biol. Res. 13, 726–732. doi: 10.1080/17451000.2017.1298806
Porri, F., McQuaid, C. D., and Radloff, S. (2006). Spatio-temporal variability of larval abundance and settlement of Perna perna: differential delivery of mussels. Mar. Ecol. Prog. Ser. 315, 141–150. doi: 10.3354/meps315141
Pulliam, H. R. (1988). Sources, sinks and population regulation. Am. Nat. 132, 652–661. doi: 10.1086/284880
Reaugh-Flower, K., Branch, G. M., Harris, J. M., McQuaid, C. D., Currie, B., Dye, B., et al. (2011). Scale-dependent patterns and processes of intertidal mussel recruitment around southern Africa. Mar. Ecol. Prog. Ser. 434, 101–119. doi: 10.3354/meps09169
Reusch, T. B. H., Dierking, J., Andersson, H. C., Bonsdorff, E., Carstensen, J., Casini, M., et al. (2018). The Baltic Sea as a time machine for the future coastal ocean. Sci. Adv. 4:eaar8195.
Rilov, G., Dudas, S. E., Menge, B. A., Grantham, B. A., Lubchenco, J., and Schiel, D. R. (2008). The surf zone: a semi-permeable barrier to onshore recruitment of invertebrate larvae? J. Exp. Mar. Biol. Ecol. 361, 59–74. doi: 10.1016/j.jembe.2008.04.008
Roughgarden, J., Gaines, S., and Possingham, H. (1988). Recruitment dynamics in complex life-cycles. Science 241, 1460–1466. doi: 10.1126/science.11538249
Sagarin, R. D., Gaines, S. D., and Gaylord, B. (2006). Moving beyond assumptions to understand abundance distributions across the ranges of species. Trends. Ecol. Evol. 21, 524–530. doi: 10.1016/j.tree.2006.06.008
Shanks, A. L., Morgan, S. G., MacMahan, J., and Reniers, A. J. H. M. (2017). Alongshore variation in barnacle populations is determined by surfzone hydrodynamics. Ecol. Monogr. 87, 508–532. doi: 10.1002/ecm.1265
Sokolowski, A., Ziólkowska, M., Balazy, P., Plichta, I., Kukliński, P., and Mudrak-Cegiolka, S. (2017). Recruitment pattern of benthic fauna on artificial substrates in brackish low-diversity system (the Baltic Sea). Hydrobiologia 784, 125–141. doi: 10.1007/s10750-016-2862-z
Sorte, C. J. B., Davidson, V. E., Franklin, M. C., Benes, K. M., Doellman, M. M., Etter, R. J., et al. (2017). Long-term declines in an intertidal foundation species parallel shifts in community composition. Glob. Change Biol. 23, 341–352. doi: 10.1111/gcb.13425
Sutherland, J. P. (1987). Recruitment limitation in a tropical intertidal barnacle: Tetraclitapanamensis (Pilsbry) on the Pacific coast of Costa Rica. J. Exp. Mar. Biol. Ecol. 113, 267–282. doi: 10.1016/0022-0981(87)90105-5
Toupoint, N., Gilmore-Solomon, L., Bourque, F., Myrand, B., Pernet, F., Olivier, F., et al. (2012). Match/mismatch between the Mytilus edulis larval supply and seston quality: effect on recruitment. Ecology 93, 1922–1934. doi: 10.1890/11-1292.1
Underwood, A. J., and Keough, M. J. (2001). “Supply-side ecology: the nature and consequences of variation in recruitment of intertidal organisms,” in Marine Community Ecology, eds M. D. Bertness, S. D. Gaines, and M. E. Hay (Sunderland, MA: Sinauer Associates).
van der Meer, J., Beukema, J. J., and Dekker, R. (2001). Long-term variability in secondary production of an intertidal bivalve population is primarily a matter of recruitment variability. J. Anim. Ecol. 70, 159–169. doi: 10.1111/j.1365-2656.2001.00469.x
Vuorinen, I., Hänninen, J., Rajasilta, M., Laine, P., Eklund, J., Montesino-Pouzols, F., et al. (2015). Scenario simulations of future salinity and ecological consequences in the Baltic Sea and adjacent North Sea areas–implications for environmental monitoring. Ecol. Indic 50, 196–205. doi: 10.1016/j.ecolind.2014.10.019
Westerbom, M., and Jattu, S. (2006). Effects of wave exposure on the sublittoral distribution of blue mussels (Mytilus edulis) in a heterogeneous archipelago. Mar. Ecol. Prog. Ser. 306, 191–200. doi: 10.3354/meps306191
Westerbom, M., Kilpi, M., and Mustonen, O. (2002). Blue mussels, Mytilus edulis, at the edge of the range: population structure, growth and biomass along a salinity gradient in the north-eastern Baltic Sea. Mar. Biol. 140, 991–999. doi: 10.1007/s00227-001-0765-6
Westerbom, M., Kraufvelin, P., Erlandsson, J., Korpinen, S., Mustonen, O., and Díaz, E. (2019a). Wave stress and biotic facilitation drive community composition in a marginal hard-bottom ecosystem. Ecosphere 10:e02883. doi: 10.1002/ecs2.2883
Westerbom, M., Mustonen, O., Jaatinen, K., Kilpi, M., and Norkko, A. (2019b). Population dynamics at the range margin: implications of climate change on sublittoral blue mussels (Mytilus trossulus). Front. Mar. Sci. 6:292. doi: 10.3389/fmars.2019.00292
Wilcox, M., Kelly, S., and Jeffs, A. (2020). Patterns of settlement within a restored mussel bed site. Restor. Ecol. 28, 337–346. doi: 10.1111/rec.13075
Keywords: environmental gradients, Mytilus, recruitment, spatial stochasticity, Baltic Sea, climate change, population structure
Citation: Westerbom M, Kraufvelin P, Mustonen O and Díaz E (2021) Explaining Recruitment Stochasticity at a Species’ Range Margin. Front. Mar. Sci. 8:659556. doi: 10.3389/fmars.2021.659556
Received: 27 January 2021; Accepted: 11 June 2021;
Published: 12 July 2021.
Edited by:
Iñigo Muxika, Technological Center Expert in Marine and Food Innovation (AZTI), SpainReviewed by:
Roman Wenne, Institute of Oceanology (PAN), PolandJonathan Paul Aden Gardner, Victoria University of Wellington, New Zealand
Copyright © 2021 Westerbom, Kraufvelin, Mustonen and Díaz. This is an open-access article distributed under the terms of the Creative Commons Attribution License (CC BY). The use, distribution or reproduction in other forums is permitted, provided the original author(s) and the copyright owner(s) are credited and that the original publication in this journal is cited, in accordance with accepted academic practice. No use, distribution or reproduction is permitted which does not comply with these terms.
*Correspondence: Mats Westerbom, mats.westerbom@helsinki.fi; mwesterbom@gmail.com