- 1State Key Laboratory of Marine Environmental Science, Fujian Provincial Key Laboratory of Coastal Ecology and Environmental Studies, Xiamen University, Xiamen, China
- 2Institute of Oceanography, Minjiang University, Fuzhou, China
- 3Southern Marine Science and Engineering Guangdong Laboratory (Zhuhai), Zhuhai, China
Nano- and pico-eukaryotes play important roles in the diversity and functions of marine ecosystems. Warm, saline, and nutrient-depleted water that originates in the Kuroshio Current seasonally intrudes into the northern South China Sea (NSCS) from autumn to spring. To clarify the mechanisms in shaping the community structure of nano- and pico-eukaryotes as well as impacts of the Kuroshio intrusion on the NSCS ecosystem, genomic DNA and RNA were co-extracted from samples collected at two depths from nine stations, and then the V9 region of 18S rDNA and rRNA was sequenced with high-throughput sequencing. Our results showed that Dinophyceae was the most diverse and abundant nanoeukaryotic group during the study period revealed by both DNA and RNA surveys. In contrast, the relative read abundance of MAST, Pelagophyceae, and Dinophyceae in the size fraction of picoeukaryotes might be largely underestimated by the DNA survey. The RNA survey was the more reliable method to investigate the eukaryotic community structure. Environmental filtering played an important role in shaping the community structure, and the sampling depth became the governing factor of the beta diversity under the environmental setting of stratification during the study period. The spatial variations in the diversity of nanoeukaryotes were subject to the dispersal limitation under the size rule. The effects of the Kuroshio intrusion on the nanoeukaryotic community structure might also be explained by the dispersal limitation. Overall, neutral processes are critical in shaping the community structure of nanoeukaryotes. The relative metabolic activities of nanoeukaryotes were relatively stable in accordance with the high similarity of community structure between sampling sites. The responses of the relative metabolic activities of picoeukaryotes to environmental factors displayed two distinct patterns: positive correlations with salinity and nutrients and negative with temperature for Dinophyceae, MAST, and Pelagophyceae, while reversed patterns for Mamiellophyceae and Radiolaria. Our findings improve the understanding of the nano- and pico-eukaryotic communities in the NSCS and the mechanisms of their assembly.
Introduction
Nano- and pico-eukaryotes (size fractions of 3–20 and 0.2–3 μm, respectively) are present worldwide, abundantly, and persistently in various marine ecosystems (Massana et al., 2015). Much attention has been paid to their astonishing diversity (Caron et al., 2012; Piwosz et al., 2015; de Vargas et al., 2015; Caron et al., 2016) and more recently to their trophic modes and interactions (Piwosz et al., 2018; Adl et al., 2019; Sassenhagen et al., 2019). The photosynthetic groups of nano- and pico-eukaryotes are important contributors to primary production (Grob et al., 2007; Jardillier et al., 2010; Hartmann et al., 2012), which link trophic levels in the microbial food web (Massana et al., 2009), and mixotrophic groups are important bacterial grazers and have the potential to dominate the primary production and bacterivory in marine ecosystems (Unrein et al., 2014). Understanding the diversity, composition, and functions of these groups is therefore important to unveil critical biogeochemical cycles in the sea.
The main goal of microbial ecology is to reveal the mechanisms shaping and maintaining community assembly (Zhou and Ning, 2017). The framework described by Sloan et al. (2006) proposed four main processes for regulating the distribution patterns of microbial communities, including: selection, ecological drift, dispersal, and speciation. These four processes can be further grouped into two categories: deterministic processes (or selective processes) and neutral processes (or stochastic processes) (Sloan et al., 2006; Logares et al., 2013). The deterministic process refers to the governing of the microbial community structure by environmental conditions (e.g., temperature, salinity, and nutrient concentration) and biotic interactions (e.g., predation, competition, and mutualism) (Stegen et al., 2012; Yu et al., 2015; Zhou and Ning, 2017). The neutral process refers to the governing of the microbial community structure by the stochastic process of birth, death, extinction, colonization, and speciation (Hubbell, 2011). The neutral community model (NCM) is an adaptation of the neutral theory, which is very useful in quantifying the importance of neutral process in diverse environments (Logares et al., 2013; Chen et al., 2017). Normalized stochasticity ratio (NST), proposed by Ning et al. (2019), is also powerful in evaluating the community assembly processes (Li et al., 2020). The relative importance of deterministic and neutral processes varies among regions, taxa, and ecosystems (Bahram et al., 2016; Wu et al., 2017b).
Eukaryotic diversity in the ocean has been extensively studied. Most studies targeted one specific size fraction, while a few studies explored diversity across size, usually in four size classes: picoplankton (0.2–3 μm), nanoplankton (3–20 μm), microplankton (20–200 μm), and mesoplankton (200–2000 μm) (de Vargas et al., 2015; Massana et al., 2015; Ramond et al., 2019). These studies disclosed cryptic diversity across oceans with many parasitic and phagotrophic species unseen by traditional methods. The deeply sequenced samples help reacquaint the trophic modes of eukaryotes in the ocean (Ramond et al., 2019). In spite of the dominance of heterotrophs in every size fraction, the ratio between heterotrophs and autotrophs increases with the nominal size of fractions (de Vargas et al., 2015), and cell size constrains fundamental requirements and physiological rates (e.g., growth rate) of plankton as well as biotic interactions (e.g., grazing and competition) between them (Barton et al., 2013). In contrast with picoplankton studied by most research, nanoplankton have higher requirements for nutrients and food, in turn, nanoplankton contribute more to carbon export than picoplankton (Ward and Follows, 2016; Leblanc et al., 2018). Cell size is also an important factor for dispersal limitation to shape community structure. Dispersal limitation was reported to increase with cell size (de Vargas et al., 2015).
High-throughput sequencing (HTS) has revealed the astonishing diversity of protist (microbial eukaryotes) and greatly improved our understanding of their community composition in environmental samples (Edgcomb et al., 2011; Parris et al., 2014; Massana et al., 2015; de Vargas et al., 2015; Piwosz et al., 2018; Liu et al., 2019). Moreover, protistologists have generally accepted that protist assemblages inferred from 18S rRNA gene sequencing (hereafter DNA sequencing) represented the species present. Compared to DNA, RNA is unstable in extracellular conditions, microbial community structures inferred from rRNA sequencing (hereafter RNA sequencing) can exclude sequences from dissolved extracellular pool, dormant cells and dead materials (Not et al., 2009). Thus, the RNA-based approach was used to identify the active species or groups in environmental samples (Massana et al., 2015; Hu et al., 2016; Wang et al., 2019). The RNA: DNA ratio (calculated by relative sequence abundance) has been used as an index for the relative metabolic activity in protist (Hu et al., 2016; Wu and Liu, 2018). However, sequences information derived from the rRNA indicates the possible ribosomal activity (i.e., protein synthesis), which is not a direct indicator of cell activity (Blazewicz et al., 2013). In addition, gene copy numbers, life strategies and histories are very different among species. The RNA: DNA ratio should be used with caution (Blazewicz et al., 2013). Comparison of RNA: DNA ratios usually used in the same species or group among different samples, but not for the comparison between different species or groups (Hu et al., 2016). A previous study showed that the RNA: DNA ratio could be a potential indicator of the upcoming Phaeocystis globosa bloom in the eastern English Chanel (Rachik et al., 2018). Xu et al. (2017) observed the varied metabolic activities of protist community at different sampling depths in the South China Sea. Wang et al. (2019) found the varied metabolic activities of picoeukaryotes at spatial scale in the Pacific Ocean.
The South China Sea is the largest marginal sea of the North Pacific (from the equator to 23°N, from 99 to 121°E), and its surface circulation is driven by monsoons (Liu et al., 2002). The North South China Sea (NSCS) exchanges water with the North Pacific through only the Luzon Strait channel. Kuroshio, the western boundary current of the North Pacific, is characterized by warm and saline waters and intrudes seasonally into the NSCS by passing through the Luzon Strait (Nan et al., 2015). Intrusion also generates mesoscale eddies in the NSCS (Wang et al., 2018). The mix of Kuroshio and NSCS waters dilutes nutrients (Du et al., 2013), but has been found to stimulate the activities of ammonia-oxidizing bacteria (Xu et al., 2018; Lu et al., 2019) as well as primary productivity (Huang et al., 2019). The diversity and composition of picoeukaryotes in the NSCS have been previously explored by high-performance liquid chromatography (Xiao et al., 2018), fluorescence in situ hybridization (Wu et al., 2017c), and DNA sequencing (Wu et al., 2014). However, little is known about the diversity and composition of nano-eukaryotes in this area. Moreover, neutral processes have been overlooked in the past, but these processes could be important for Kuroshio-water-influenced areas because ocean currents are always related to the dispersal and colonization of species. This study examines the diversity and community composition of nano- and pico-eukaryotes in the NSCS using size-fractionated filtration and DNA/RNA high-throughput sequencing. The NSCS was sampled in late spring, when a significant proportion of the water mass is of Kuroshio origin, in order to evaluate the relative importance of environmental filtering and neutral processes in shaping communities. In particular, we assess how diversity and community composition of nano- and pico-eukaryotes are affected by deterministic processes by relating metabolic activity and environmental gradients.
Materials and Methods
Cruise, Sampling, and Environmental Parameters
The research cruise was conducted in the NSCS from 114 to 119°E and 18 to 22.5°N from May 15th to June 5th, 2016 (Supplementary Figure 1). A total of 36 samples (18 picoeukaryotes and 18 nanoeukaryotes) were collected from the surface layer (Sur, 5 m) and deep chlorophyll maximum (DCM) layers at nine stations (Supplementary Figure 1). For each sample, 10–20 L of seawater was collected using Niskin bottles (12-L PVC) mounted on a conductivity, temperature, and depth (CTD) rosette (Sea-Bird Electronics, United States). The seawater was pre-filtered through a 20 μm Bolting Cloth to remove large organisms. Then the pre-filtered seawater was sequentially filtered through 3- and 0.2 μm pore size polycarbonate membranes (142 mm diameter, Millipore, United States) using a peristaltic pump. To reduce the loss of RNA samples, the filtration rate in our experiment was controlled at 0.5–1 L/min. The filters (nanoeukaryotic samples: 3–20 μm, picoeukaryotic samples: 0.2–3 μm) were stored in 5 mL cryotubes containing 4.6 mL RNAlater (Thermo Fisher, Lithuania). The tubes were immediately frozen in liquid nitrogen. In this study, samples were named by the combination of sampling station and depth, for example, D2Sur means the sample was collected at the surface layer at Station D2. Seawater temperature and salinity at each sampling site were recorded using the SBE-911 CTD. Water samples (50 mL) were also collected at the sampling sites and preserved at −20°C for nutrient analysis. The nutrient concentrations (NO2–, NO2– + NO3–, PO43–, and SiO32–) were measured with an AutoAnalyzer using the SkalarSANplus model (Technicon AA3, Bran-Lube, GmbH). The analytical precision of NO2–, NO2– + NO3–, PO43–, and SiO32– were 0.1, 0.1, 0.05, and 0.2 μM. For chlorophyll a (Chl a) measurement, 1 L of seawater from each sampling depth were filtered onto 25 mm GF/F glass fiber filters (Whatman, United States) under 200 mm Hg pressure. The filters were stored in liquid nitrogen on board. In the laboratory, the filters were submersed in 90% acetone in the dark at −20°C for 24 h. The concentrations of Chl a extracted into the acetone were measured with a Trilogy fluorometer (Turner Design, United States) (Welschmeyer, 1994). A geostrophic current is the result of the horizontal pressure gradient and the Coriolis force. The direction of the geostrophic current is parallel to the contour of the sea surface height. To generate a synoptic view of how Kuroshio intrusion physically influences the SCS, sea level anomalies and geostrophic current vectors for the study area were downloaded from the US NOAA National Environmental Satellite, Data, and Information Service.1
Kuroshio Fraction at the HTS Sampling Stations
An isopycnal mixing model described by Du et al. (2013) was used to quantify the impact of the Kuroshio intrusion (KI) at our sampling stations. This model assumes that the different water masses are dominated by isopycnal mixing, and diapycnal mixing is comparatively negligible (Du et al., 2013). In this model, two end-members (representing the Kuroshio water and SCS water, respectively, in this study) are required for index estimation. The P4 station (122.959°E, 20.003°N) was used to represent the Kuroshio water mass end-member, and the South East Asian time-series (SEATS) station (116°E, 18°N) was selected to represent the proper South China Sea (SCS) water mass end-member (Figure 1A). Notably, the fractional contribution of the two end-members varies depending on the end-member selection. The proportion of the Kuroshio water (RK) indicated the relative contribution from P4 instead of the “typical Kuroshio” water. Our HTS samples were all collected at the surface (5 m) and DCM layers (less than 100 m), and the average RK values of the upper 100 m (RKave–100, 1 m interval) were used to represent the KI fraction. Hence, an index was used to indicate the degree of KI influence (KI index) at each sampling station (Supplementary Table 1). According to the assumption of this model, Xu et al. (2018) who participated in the same cruise have calculated and published the KI index at all sampling stations. In this study, KI index ≥0.35 was considered to indicate more KI (MKI) affected stations, and the D2, D6, A3, A7, and K4 stations belonged to this category. KI index <0.35, was considered to indicate less KI (LKI), and 2A1, A10, SEATS, and K8 stations belonged to this category (Figure 1A).
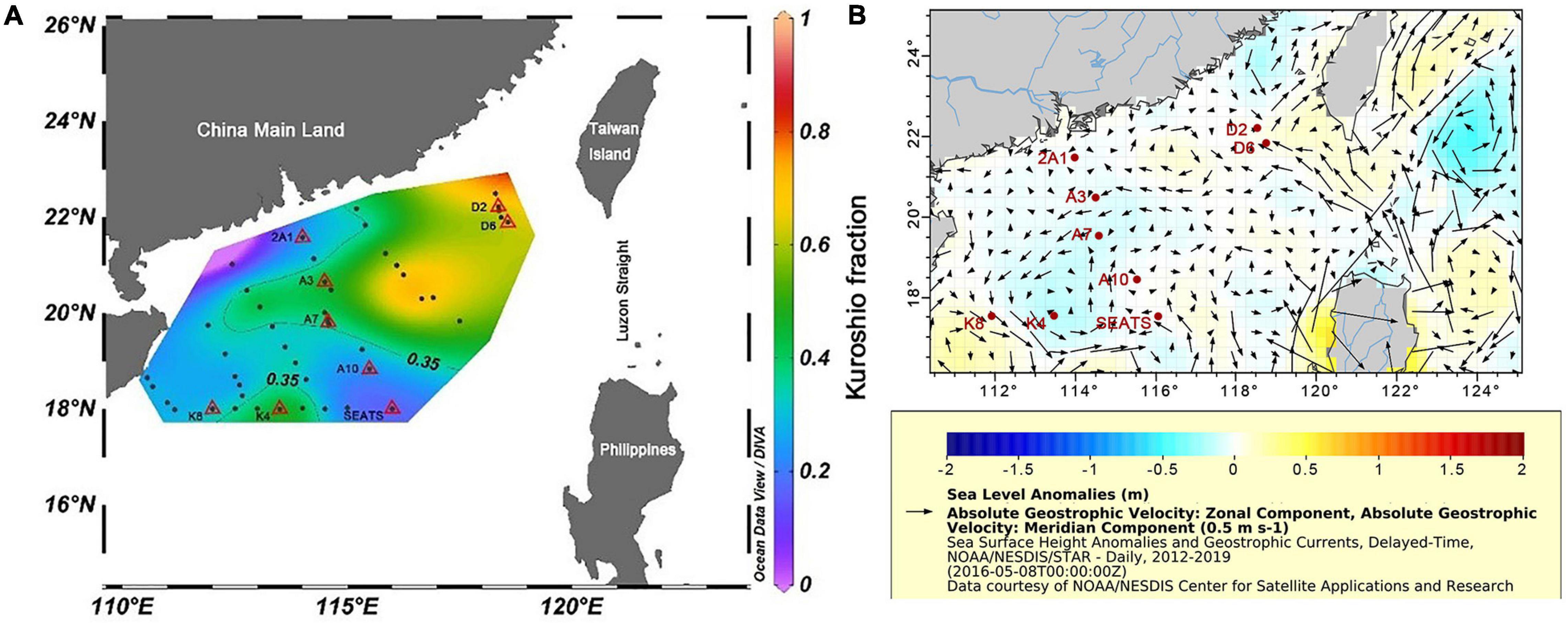
Figure 1. The spatial distribution of (A) averaged Kuroshio fraction for upper 100 m (1 m resolution) at our HTS sampling sites (triangles) in the NSCS during the summer cruise conducted in 2016 and (B) geostrophic currents and sea level anomalies in a week before the cruise. The P4 station was the end-member used in our study for the Kuroshio water. The thin line stands for the isopleth of RK (KI index) of 0.35. The color bar indicates the value Rkave–100 (from 0 to 1).
DNA and RNA Co-extraction, PCR Amplification, and Illumina High-Throughput Sequencing
For each sample, total DNA and RNA were extracted simultaneously using the AllPrep DNA/RNA Min Kit (Qiagen, #80204, Germany). The RNA extracts were purified using the gDNA wipeout buffer (Qiagen, #205311) to remove possible genomic DNA contamination, and then the purified RNA was reverse transcribed into cDNA using the QuantiTect Reverse Transcription Kit (Qiagen, #205311, Germany). The V9 hypervariable region of the eukaryotic 18S rRNA gene was amplified using the 1380F (5-CCCTGCCHTTTGTACACAC-3) and 1510R (5-CCTTCYGCAGGTTCACCTAC-3) primers (Amaral-Zettler et al., 2009). For each polymerase chain reaction (PCR) reaction, 25 μL mixture contained 12.5 μL 2 × Taq PCR mix (Takara, China), ≤1 μg DNA or cDNA template, 0.1 μM forward primer, and 0.1 μM reverse primer. The PCR program consisted of a 5-min initial denaturation step at 95°C, followed by 34 cycles of denaturation at 94°C for 1 min, annealing at 57°C for 45 s, and elongation at 72°C for 1 min, and a final extension at 72°C for 10 min. Each PCR was performed in duplicates for each DNA and cDNA sample. The duplicate PCR products were pooled and purified using the QIAquick Gel Extraction Kit (Qiagen, #28704, Germany). Amplicons were sent with dry ice to the Novogene sequencing company (Beijing, China) where sequencing library, constructed using the TruSeq DNA PCR-Free Sample Preparation Kit, was prepared and sequenced on a single run using the Illumina HiSeq 2500 platform. High-throughput sequencing data from this study were deposited in the GenBank Sequence Read Archive database under the accession number PRJNA684778.
Sequencing Processing and Statistical Analysis
The raw tags were processed and checked using the Quantitative Insights Into Microbial Ecology (v.1.70) pipeline (Caporaso et al., 2010). Chimeras were identified and discarded after checking with the chimera search module (USEARCH, version 4.2) (Edgar et al., 2011) using the Silva 123 release (Quast et al., 2013) as a reference. The remaining filtered sequences were grouped into operational taxonomic units (OTUs) with 0.95 similarities using UPARSE (v. 7.0.100; Edgar, 2013). The most abundant sequence of each OTU was chosen as the representative sequence, and then taxonomically annotated against the V9_PR2∗ database (de Vargas et al., 2015) using BLAST (E value = 10–6 and minimum percent query coverage = 0.9) (Altschul et al., 1990). The OTU taxonomy was determined from the top hit based on the BLAST score. OTUs occurring in a single sample, OTUs with only one read (singleton), and OTUs assigned to Metazoan, Bacteria, Archaea, and organelles were also discarded from the downstream analyses. To normalize the sampling effort, the nano- and pico-eukaryotic OTU tables were rarefied to 29,799 and 28,698 reads per sample, respectively (the lowest sequence reads numbers in these tables).
All statistical analyses in our study were performed using the R software (version 3.3.2) (R Core Team, 2014). To investigate whether the community structure of nano- and pico-eukaryotes in the NSCS was affected by the Kuroshio intrusion, principal coordinate analysis (PCoA) was conducted based on the Bray-Curtis distance matrix. Variation-partitioning analysis (VPA, Borcard et al., 1992) was performed to disentangle the relative effects of spatial and environmental factors on variation in nano- and pico-eukaryotic DNA/RNA-based communities using a 3-way PERMANOVA (McArdle and Anderson, 2001). One of the environmental factors was the data matrix consisting of water temperature, NO2– + NO3–, PO43–, SiO32–, and Chl a, and the other was the KI index. It might represent some unmeasured environmental variables that were related to the fraction of Kuroshio water or salinity. The spatial factor was computed as the principal coordinates of neighborhood matrix (PCNM) by the pcnm function of the “vegan” package in R, the second PCNM was used for the VPA analysis in this study. The neutral community model (NCM) was used to determine the potential contribution of neutral processes to the nano- and pico-eukaryotic communities by predicting the relationship between OTUs occurrence and their relative read abundance (Sloan et al., 2006). The normalized stochasticity test (NST) was used to further confirm the importance of neutral process during nano- and pico-eukaryotic community assembly. The NST was conducted using the function of the “NST” R package, which is based on the Ruzicka index (NSTruzicka). This index ranges from 0 to 100%, indicating an increase from more deterministic to more stochastic assembly (Ning et al., 2019). Principal component analysis (PCA) was performed using the “vegan” package in R to access the distribution pattern of eukaryotic assemblages among sampling sites. OTUs only occur in both DNA and RNA sequencing results and appeared in all samples were selected, and the RNA: DNA ratios of the selected OTUs were calculated based on their relative read abundance in the RNA and DNA results (Wu and Liu, 2018).
Results
Environmental Setting
Stations D2 and D6 were close to the Luzon Strait and characterized by higher salinity (34.44–34.81) than other sampling stations. The two stations were greatly influenced by the Kuroshio intrusion, as indicated by KI indexes larger than 0.6 (Figure 1A). Kuroshio water affected stations A3 and A7 in the middle of the transect from 2A1 to SEATS (Figure 1B). Although station 2A1 is geographically close to the Pearl River Estuary, it was not affected by fresh water during our sampling period. Salinity at surface and DCM layers of 2A1 station were 34.28 and 34.33, respectively, which was close to those of SEATS station. The distinct temperature between surface and DCM (paired Mann-Whitney U-test, p < 0.01) indicated stratification in water columns (Supplementary Table 2). Nutrients at the surface were mostly below the detection limits but were abundant in the DCM. The lower concentrations of Chl a at the surface (average 0.08 mg m–3) than at the DCM (average 0.37 mg m–3) (paired Mann-Whitney U-test, p < 0.01) was consistent with the different nutrient regimes.
Richness of Nano- and Pico-Eukaryotes in the NSCS
In our study, 852,873 high-quality DNA sequences and 997,272 high-quality RNA sequences were obtained from the nanoeukaryotic samples (Supplementary Tables 5, 6), whereas 798,801 high-quality DNA sequences, and 787,383 high-quality RNA sequences were obtained from the picoeukaryotic samples (Supplementary Tables 7, 8). Note that picoeukaryotic DNA amplicons in A7Sur, SEATS-Sur, and cDNA amplicons in A7Sur and 2A1Sur were too low concentration for library preparation during high-throughput sequencing, and thus these samples were not included. In this study, 3,198 and 3,233 nanoeukaryotic and picoeukaryotic OTUs, respectively, were generated. All the OTUs were affiliated to ten super-groups: Alveolata, Stramenopiles, Hacrobia, Archaeplastida, Rhizaria, Opisthokonta, Picozoa, Amoebozoa, Excavata, and Apusozoa (Figures 2A,B).
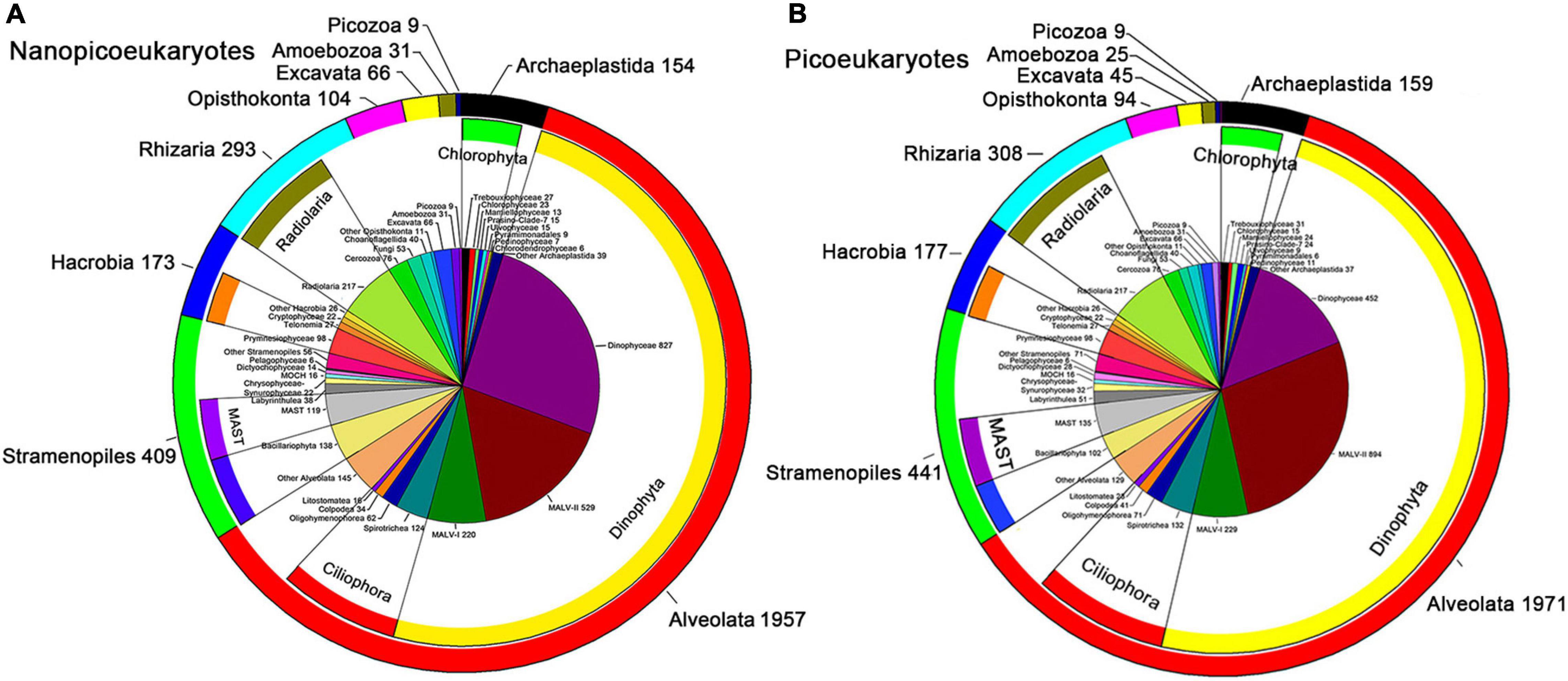
Figure 2. Number of OTUs of nano- (A) and pico-eukaryotes (B) in the NSCS. The pie chart represents the numbers of OTUs mainly at class level; the inner ring indicates these classes affiliated to the phylum; the outer ring represented the OTUs numbers at super-group level.
Alveolata was the richest super-group (in terms of the number of OTUs) in both nano- and picoeukaryotes, accounting for 61.2 and 61.0% of total richness (observed OTU number), respectively (Figure 2); followed by Stramenopiles (12.8 and 13.6%) and Rhizaria (9.2 and 9.5%). The other seven super-groups contributed only a small proportion of total richness. Dinophyta and Ciliophora were the top two phyla of Alveolata, Dinophyceae was the richest class of nanoeukaryotes while it was MALV-II for picoeukaryotes.
Among the 3,198 nanoeukaryotic OTUs (3,233 picoeukaryotic OTUs), there were 292 (319) unique DNA OTUs in the MKI stations and 215 (215) in the LKI stations (Supplementary Figures 4A,C); similar numbers were displayed for the RNA samples (Supplementary Figures 4B,D). In addition, there were more unique nanoeukaryotic DNA OTUs at the DCM than at the surface (466 vs. 192), similar to the unique picoeukaryotic DNA OTUs (397 vs. 228).
Relative Read Abundance of Nano- and Pico-Eukaryotes
Dinophyceae was the most sequenced nanoeukaryotic class (Figures 3A,B and Table 1). The most sequenced OTU was Dinophyceae Gymnodinium_03 aureolum, representing an average relative read abundance of 12.0 and 10.0% in DNA and RNA samples, respectively, and there was no significant difference between surface and DCM or between LKI and MKI stations. In contrast, MALV-II and MAST were the most sequenced picoeukaryotic classes in DNA and RNA samples, respectively (Figures 3C,D and Table 1). Radiolaria Astrosphaera hexagonalis (OTU5) which favored the DCM environments (Kruskal-Wallis test, p < 0.05) in MKI stations (Kruskal-Wallis test, p < 0.05) was the most sequenced OTU in picoeukaryotic DNA samples (averagely 8.2%), while Pelagophyceae Pelagomonas calceolata (OTU1) was the most sequenced in RNA samples (averagely 6.7%). The relative read abundance of the picoeukaryotic OTU1 was not significantly different between MKI and LKI stations (Kruskal-Wallis test, p = 0.79) but higher in DCM than in surface for some stations (Kruskal-Wallis test, p = 0.06).
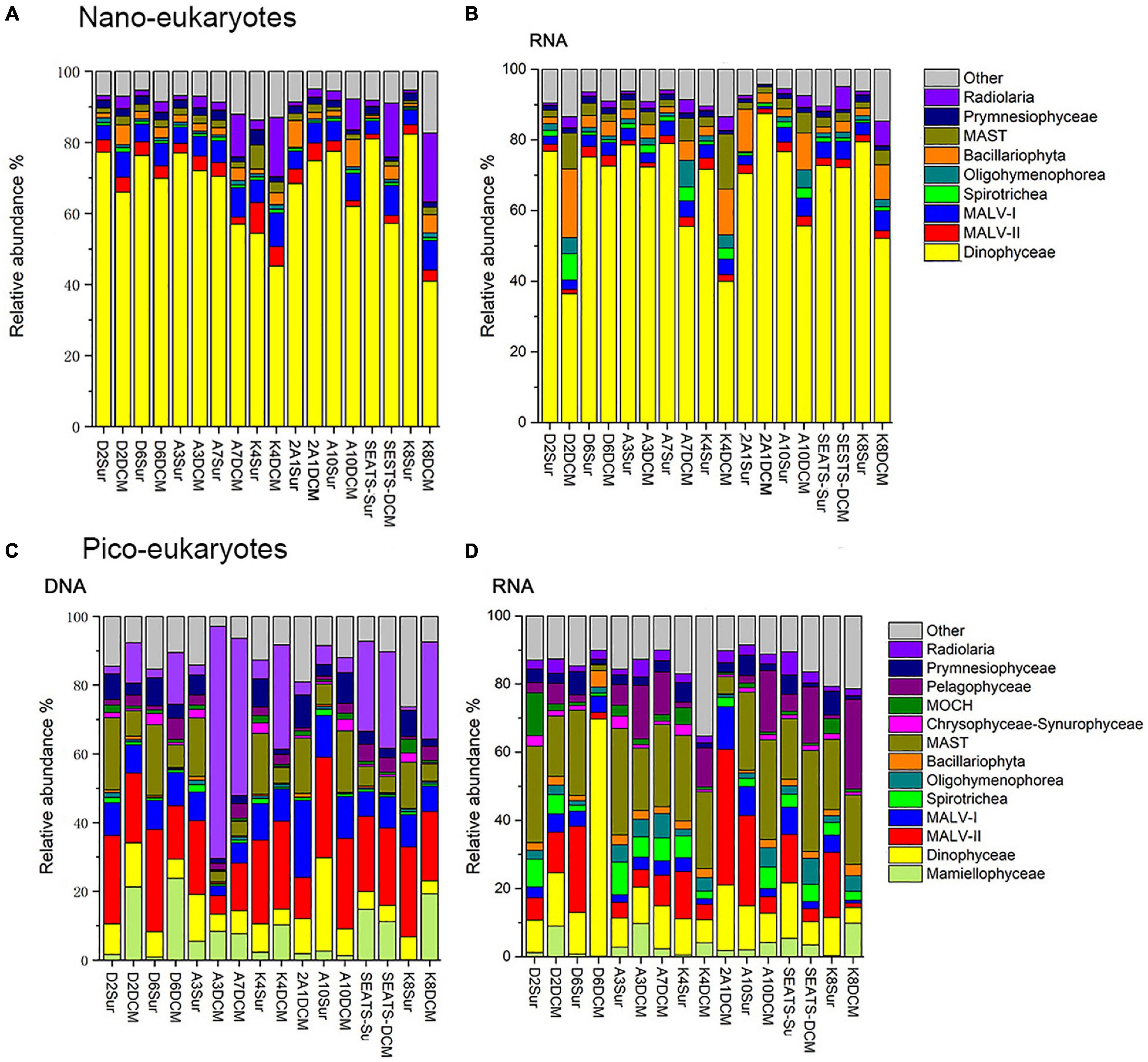
Figure 3. Relative read abundance of nanoeukaryotes (A,B) and picoeukaryotes (C,D) mainly at class level based on the DNA-derived (A,C) and RNA-derived (B,D) approaches for each sample. The other category refers to groups with relatively lower sequence abundance.
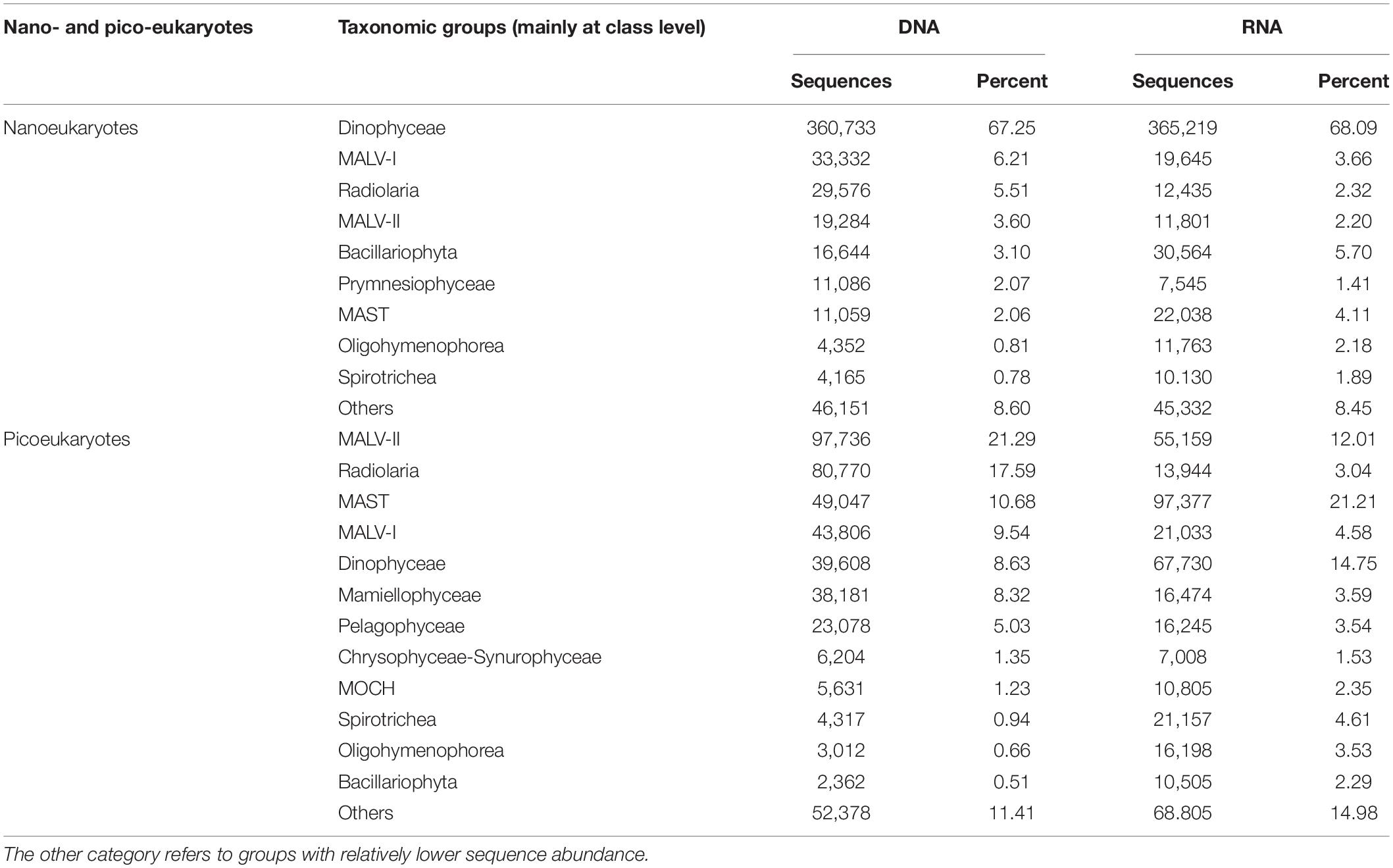
Table 1. Nano- and pico-eukaryotic taxonomic groups (mainly at class level) sequences and percentages across samples based on the DNA and RNA surveys.
In nanoeukaryotic samples, some of minor classes showed large variations in relative read abundance. For example, Radiolaria was much more sequenced in DCM than in surface (Kruskal-Wallis test, p < 0.001) in DNA samples, increases of one order of magnitude occurred in DCM in stations such as A7, K4, SEATS, and K8 (Figure 3A). Increases of one order of magnitude in DCM relative to surface also occurred to RNA samples of Bacillariophyta and MAST in a few stations (Figure 3B). In picoeukaryotic samples, the distributions of relative read abundance among classes were not as uneven as in nanoeukaryotic samples, therefore some minor classes with large variations could dominate in a few stations. For example, the relative DNA abundance of Radiolaria was up to 67.6 and 45.8% in DCM of MKI stations A3 and A7; and the exceptional case for Dinophyceae was that it contributed 69.5% of RNA samples in DCM of the MKI station D6.
Beta Diversity of Nano- and Pico-Eukaryotic Communities and Controlling Factors
PCoA analysis showed that the beta diversity of both nano- and pico-eukaryotic communities was mainly shaped by sampling depths (Figure 4), and the surface and DCM samples were clearly separated on axis 1 while there was no apparent clustering on axis 2. Moreover, a 3-way PERMANOVA was used to assess the relative importance of environmental and spatial factors in shaping eukaryotic communities (Table 2). The combined effect of three components could explain 27.3-41.5% of variances in community structure. The unique environmental component [E| KI + S] (by excluding the effects of KI and spatial components) explained the largest proportions of variance for both nano- and pico-eukaryotic communities (p < 0.05) with numerically larger proportions for picoeukaryotes. The effect of the unique KI component [KI| E + S] was only significant for nanoeukaryotes (p < 0.05), and the effect of the unique spatial component [S| E + KI] was only significant for RNA samples of nanoeukaryotes (p < 0.05).
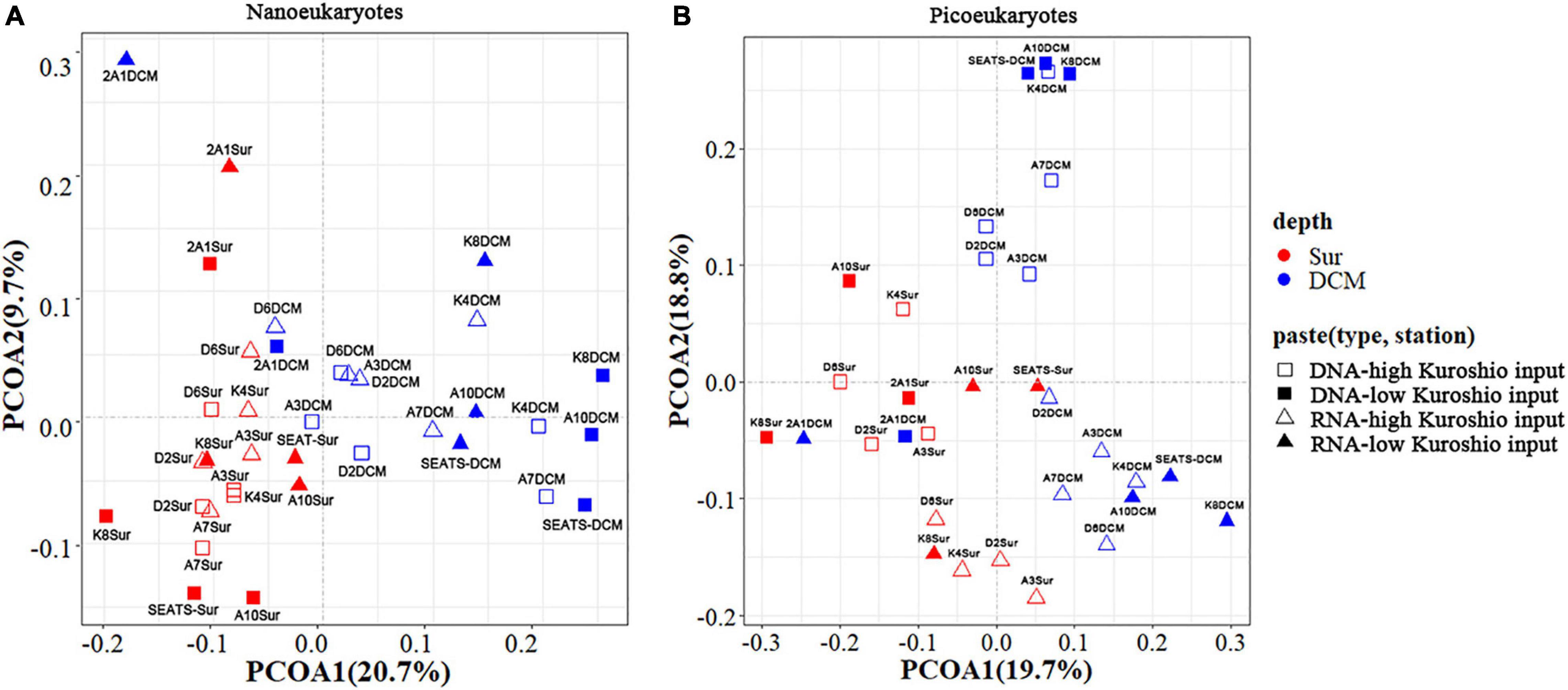
Figure 4. Principal coordinate analysis (PCoA) of nanoeukaryotes (A) and picoeukaryotes (B) in all samples based on the Bray-Curtis distance matrix. Red color: the surface samples; Blue color: the DCM samples.
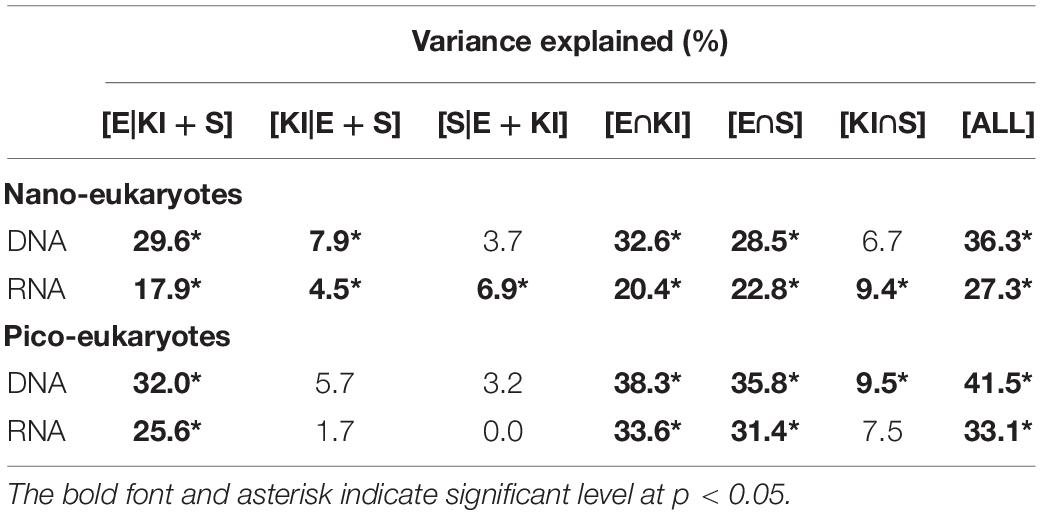
Table 2. Variation partitioning results showing the contribution of unique environmental [E| KI + S], unique KI [KI| E + S], and unique spatial [S| E + KI], as well as combined environmental and KI [E∩KI], environmental and spatial [E∩S], KI and spatial [KI∩S], and all [ALL] component to the components of the nano- and pico-eukaryotic communities inferred from DNA and RNA approaches.
In contrast, NCM explained large fractions (71–81%) of variabilities in the occurrence of nanoeukaryotes and picoeukaryotes during the study period (Figure 5). The m value (immigration rate) was higher for nanoeukaryotes than for picoeukaryotes. The NSTruzicka index showed that both DNA and RNA samples of nanoeukaryotes were primarily controlled by stochastic processes (average NSTruzicka = 59 and 62%, respectively; there was no significant difference between DNA and RNA samples, Mann-Whitney U-test, p = 0.17), while the value of 38% for DNA samples of picoeukayotes indicated the dominance of deterministic processes (Figure 6; difference between nano- and pico-eukaryotes was significant, Mann-Whitney U-test, p < 0.001). However, for RNA samples of picoeukaryotes in the DCM, deterministic processes were only marginally stronger than stochastic processes (average NSTruzicka = 50.1%; difference between DNA and RNA samples was significant, Mann-Whitney U-test, p < 0.001). The above results indicated that neutral processes (stochastic processes) played a critical role in shaping the eukaryotic communities during the study period.
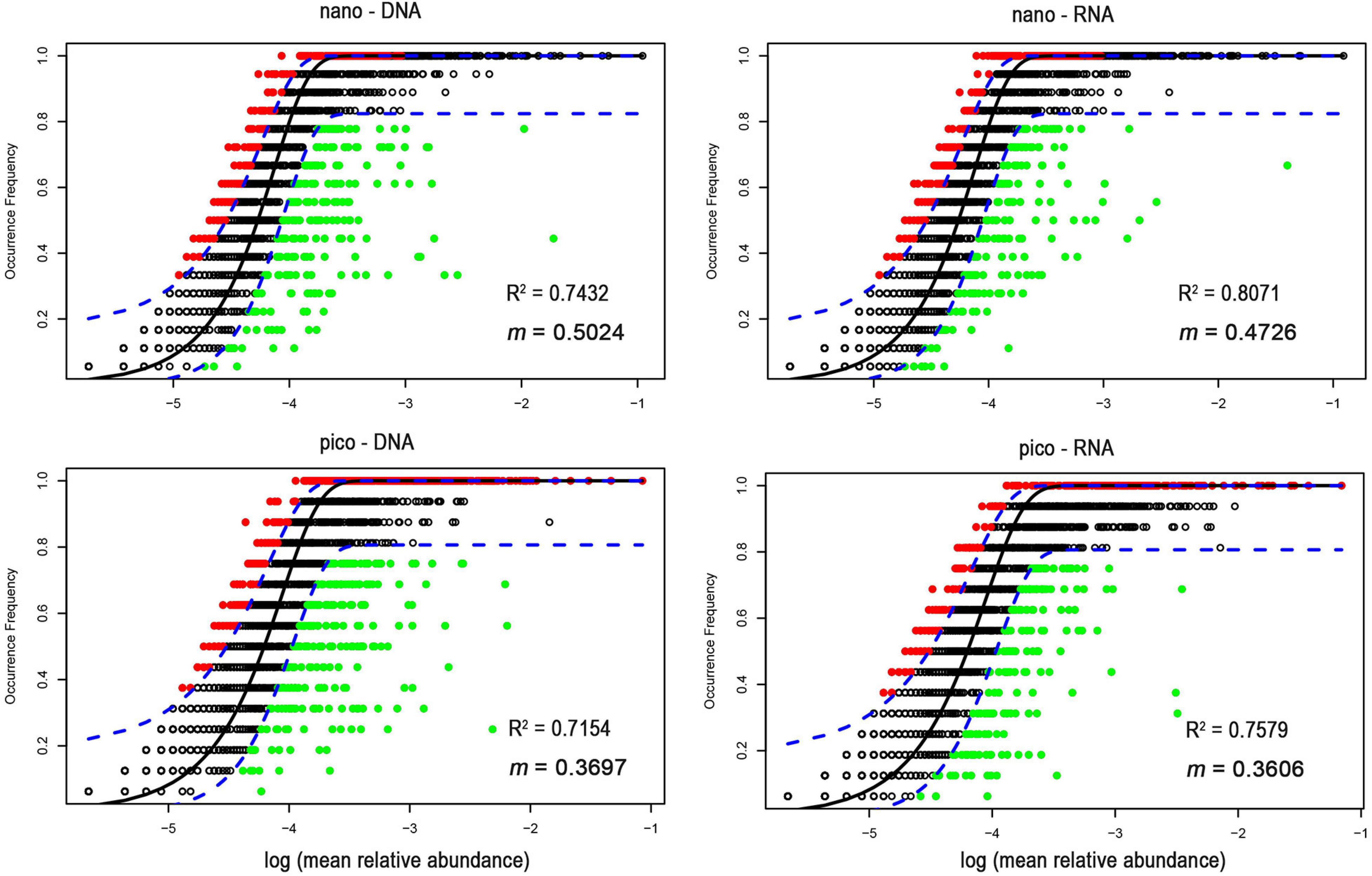
Figure 5. The neutral community model based on the relationship between OTU occurrence and relative read abundance. OTUs with higher frequency than predicted by the model are shown in red, while those with lower frequency are shown in green. OTUs within the predicted range are displayed in black. The dotted line represents the 95% confidence interval (blue dotted line) around the model prediction.
Variations in Relative Metabolic Activity of Nano- and Pico-Eukaryotes
PCA analysis was conducted on both the nano- and pico-eukaryotic groups. For nanoeukaryotes, four groups that showed large contributions to the total variation and RNA: DNA ratios different from 1 were identified: Dinophyceae, Radiolaria, MAST, and Bacillariophyta (Figure 7A). The average RNA: DNA ratios of Bacillariophyta, MAST, and Radiolaria were significantly higher for the DCM samples than for the surface samples (Figure 7B, Mann-Whitney U-test, p < 0.05, Supplementary Table 4). Following the same procedure, five groups of picoeukaryotes were identified: Dinophyceae, Radiolaria, MAST, Mamiellophyceae, and Pelagophyceae (Figure 7C and Supplementary Table 3, Mann-Whitney U-test, p < 0.05). The RNA: DNA ratios of four groups showed significant differences between the surface and DCM (Figure 7D). The average RNA: DNA ratio of Radiolaria was slightly higher for the surface than for DCM (Mann-Whitney U-test, p < 0.05). In contrast, Dinophyceae, MAST, and Pelagophyceae showed significantly higher average RNA: DNA ratios at the DCM than at the surface (Mann-Whitney U-test, p < 0.05).
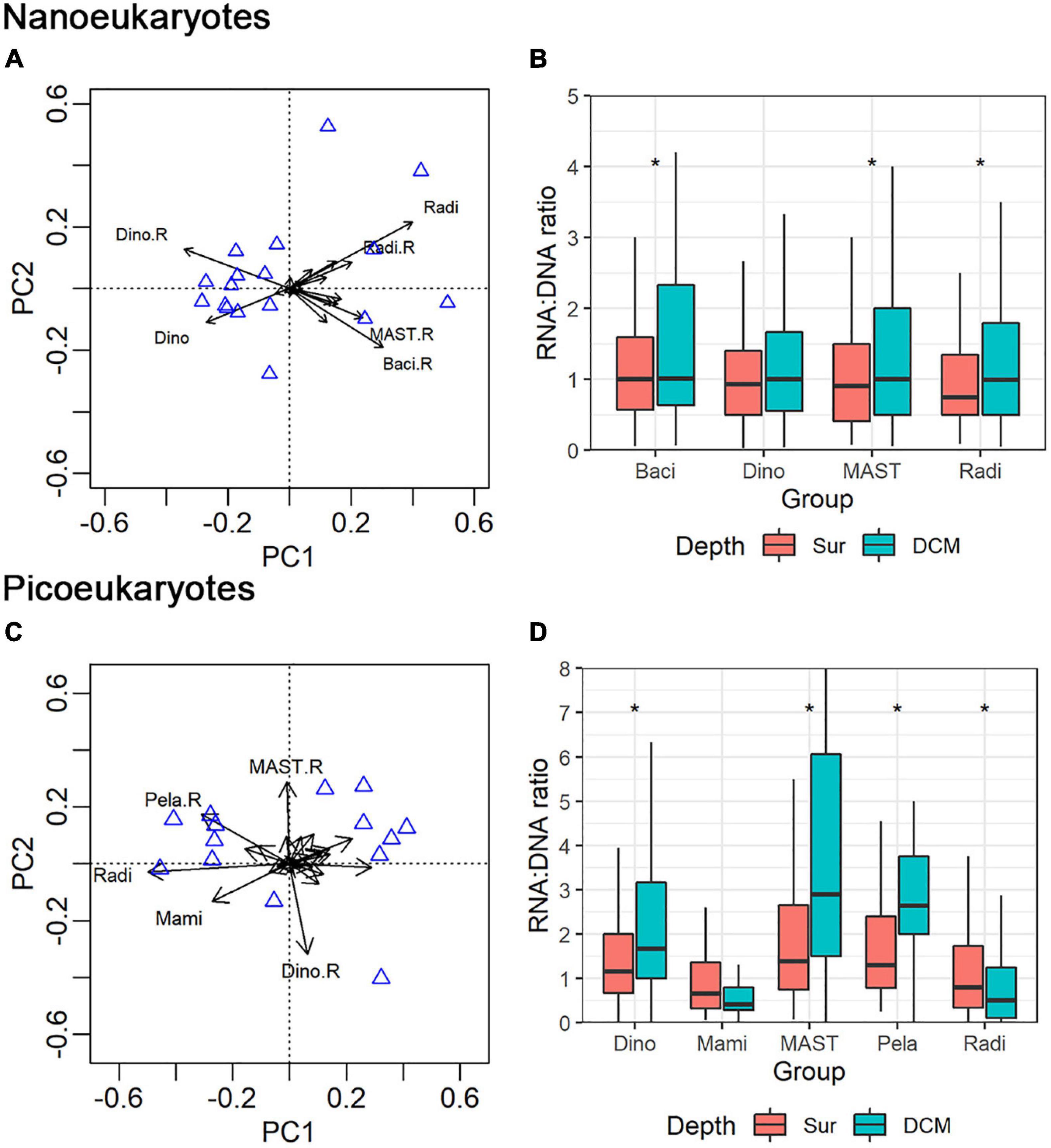
Figure 7. Principal component analysis (PCA) showed the distribution pattern of nano- (A) and pico-eukaryotic (C) major groups (mainly at class level) in combined DNA and RNA samples. Range of RNA: DNA ratios of the major groups of nano- (B) and pico-eukaryotes (D) at surface and DCM layers. Mam: Mamiellophyceae; Pel: Pelagophyceae; Rad: Radiolaria; Dino: Dionophyceae; Baci: Bacillariophyta. The asterisk indicates significant level at p < 0.05.
The relationships between the RNA: DNA ratios and the measured environmental factors (KI, salinity, water temperature, NO2– + NO3–, PO43–, SiO32–, and Chl a) were assessed. The RNA: DNA ratios of the nanoeukaryotic groups Dinophyceae and Radiolaria showed positive correlations with nutrients but negative correlations with the Chl a concentration (Figure 8A and Supplementary Figure 5), although the correlation coefficients were small. The metabolic responses of picoeukaryotes to environmental factors had roughly two patterns (Figure 8B and Supplementary Figure 6). Dinophyceae, MAST, and Pelagophyceae increased RNA: DNA ratios with salinity, nutrients, and Chl a concentration but decreased with water temperature. Meanwhile, RNA: DNA ratios of Mamiellophyceae and Radiolaria correlated negatively with nutrients and salinity but positively with water temperature.
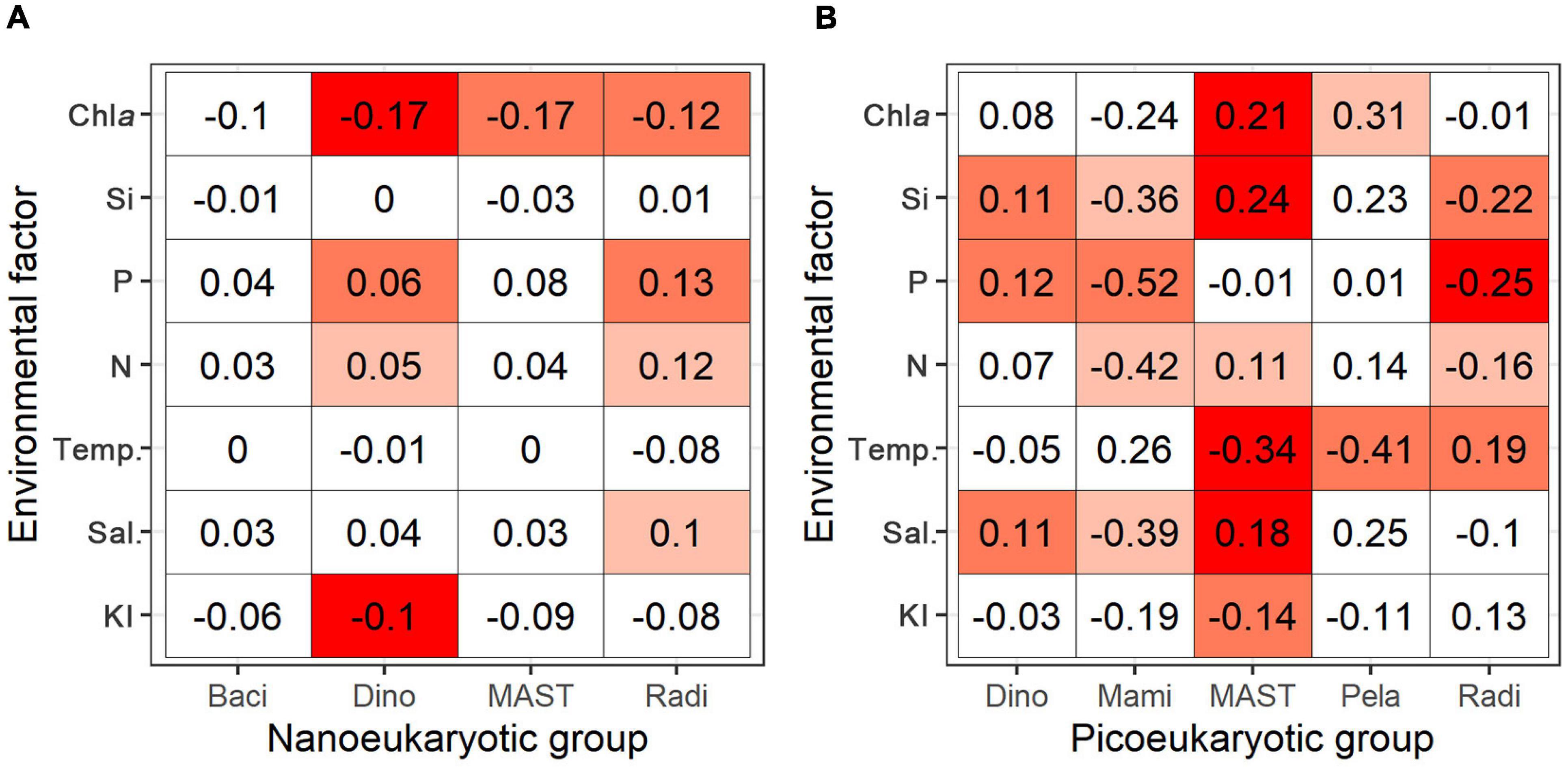
Figure 8. Heatmaps of correlation matrix (spearman correlation) of the RNA: DNA ratio versus environmental factors for major groups of nanoeukaryotes (A) and picoeukaryotes (B), including Bacillariophyta (Baci), Dinophyceae (Dino), Mamiellophyceae (Mami), MAST, Pelagophyceae (Pela), and Radiolaria (Radi). Environmental factors include Kuroshio intrusion (KI), salinity (Sal.), water temperature (Temp.), nitrate + nitrite (N), phosphate (P), silicate (Si), and chlorophyll a concentration (Chl a). Numbers are the correlation coefficients, background colors (from dark red to white) indicate significance levels associated to the p values (0.001, 0.01, 0.05) and no significance.
Discussion
Nano- and Pico-Eukaryotic Community Structure in the NSCS During the Study Period
In our study, the high rate of assignment for nano- and pico-eukaryotes could be that many assignments have low taxonomic ranks (e.g., only to “Eukaryota”). Dinophyceae was the dominant nanoeukaryotic group and the most diverse picoeukaryotic group at all sampling sites (Figures 2, 3 and Table 1). Previous studies have revealed that many dinoflagellates were mixotrophic (Stoecker, 1999; Jeong et al., 2004), and the mixotrophic species could consume a wide variety of prey items (Seong et al., 2006; Park et al., 2006; Berge et al., 2008; Glibert et al., 2009). The most sequenced nanoeukaryotic OTU was G. aureolum, a common bloom-forming species in temperate waters that was first described by Hulburt (1957). G. aureolum contains a horseshoe-shaped apical groove, a nuclear fibrous connective (NFC), and nuclear chambers (Jeong et al., 2010). Jeong et al. (2010) have shown that G. aureolum is a mixotrophic dinoflagellate that feeds on Synechococcus, heterotrophic bacteria, and some algal species. The Dinophyceae A. sanguinea (OTU2) was the dominant nanoeukaryotic species in the DCM layer of station 2A1, which was located close to the Pearl River Estuary. A. sanguinea is also a common bloom-forming species with a wide distribution, including the Black Sea (Gómez and Boicenco, 2004), the coast of Hong Kong (Lu and Hodgkiss, 2004), the South Sea off the coast of South Korea (Lim et al., 2008), and the Ariake Sea off the coast of Japan (Katano et al., 2011). Large-scale blooms of A. sanguinea have been reported to be a significant threat to seabirds, fish, and shellfish (Jessup et al., 2009; Reis-Filho et al., 2012).
The MALV-I and II groups accounted for a significant proportion of the picoeukaryotic relative read abundance in the DNA survey. However, their relative read abundance decreased largely in the RNA survey. Most species in the MALV-I and II groups have been characterized as parasites in a range of hosts (Bachvaroff et al., 2012). Similarly, the most sequenced OTU of picoeukaryotes was Radiolaria A. hexagonalis (OTU_5), accounting for 8.5% of total DNA sequences, but decreased sharply to 0.5% in the RNA survey. The different results between DNA and RNA surveys and the underlying reasons have been extensively discussed. Sample collection by the size-fractionated filtration may introduce artifacts, for example, cell breakage and fragments may go through filters and be retained in smaller size fractions (Massana et al., 2015). The Radiolaria A. hexagonalis is supposed to be an organism with the diameter of 150 μm (Haeckel, 1887). Its DNA sequences appearing in a smaller fraction may represent dead individuals or swarmer cells for reproduction (Li and Endo, 2020). In addition, high rDNA gene copy numbers of some species may cause them to be overrepresented in DNA samples. Massana et al. (2015) also reported that the MALV-I and II groups were overrepresented in the DNA survey for all size fractions of eukaryotes in European coastal waters and they attributed this phenomenon to higher rDNA gene copy numbers of these two groups than other eukaryotes. Overall, it is likely that the DNA survey overestimated the relative read abundance of Radiolaria, MALV-I, and MALV-II, while underestimated that of MAST, Pelagophyceae, and Dinophyceae (Figure 3D). MAST is a remarkably diverse group of uncultured species (Massana et al., 2014). MASTs are heterotrophic flagellates preying on bacteria (Lin et al., 2012). They are widely distributed in the temperate and tropical oceans and supposed to play an important role in the microbial food web (Seeleuthner et al., 2018). The RNA survey found the average relative read abundance of 21.4% belonged to the MAST group relative to only 10.3% by the DNA survey (Figure 3). This large bias reinforced the argument that the RNA survey is a more reliable method than the DNA survey to investigate the eukaryotic community structure (Massana et al., 2015). The RNA-based results showing the abundant MAST reflected active functions of the microbial food web in the NSCS.
In contrast, the DNA and RNA surveys reported roughly the same community structure for nanoeukaryotes in this study (Figure 3). However, we should not interpret this consistency as an indication of that the DNA survey did not obscure the results with artifacts. The consistency between the DNA and RNA samples happened at the group level where Dinophyceae dominated supremely. But differences emerged at the OTU level, for example, A. sanguinea (OTU2) at the DCM layer of station 2A1 occupied 68.2% of RNA sequences relative to 32.7% of DNA sequences. The findings emphasized again the necessity to conduct the RNA survey to tackle with the technical issues of the DNA survey (Massana et al., 2015).
Filters may collect material belonging to species from other size fractions. We found many pairs of OTUs with identical sequences between the nano- and pico-eukaryotes. This phenomenon has long been an issue for analyzing plankton samples (de Vargas et al., 2015; Massana et al., 2015). de Vargas et al. (2015) reported that 64% of all OTUs spanned two or more size classes and Massana et al. (2015) even found 32–55% of picoeukaryotic OTUs and 20–41% of nanoeukaryotic OTUs in filtrate passed through a 0.8 μm-pore-size membrane filter. We found a significant proportion of shared OTUs were MALV-II and MALV-I (also known as Syndiniales). Syndiniales are marine parasites that infect hosts from protists to fish, they could be detected in the pico-sized fraction because they produce small, flagellated cells (dinospores) to infect new hosts (Guillou et al., 2008; Siano et al., 2011). Moreover, identical sequences do not essentially indicate identical species because OTUs are clusters. In spite of deficiencies, filtration is the most feasible way to study diversity for size characteristics of plankton, and the method did reveal distinctness of size classes in terms of relative abundance of OTUs (Table 1 and Figure 3). Besides, BLAST identifies sequences with the top-hit taxon in the reference database which cannot guarantee the most accurate result because in fact there always have a series of hits with good scores; although BLAST is the common method, more robust methods for taxonomic annotation shall be further considered.
Differences in Mechanisms Shaping the Community Structure of Nano- and Pico-Eukaryotes in the NSCS
Environmental filtering played an important role in shaping the community structure of both nano- and pico-eukaryotes (Table 2). Phytoplankton growth in the NSCS is limited by nitrogen in the open water and phosphate and silicate in the river water influenced area (Yin et al., 2000, 2001). During the season when the study was conducted, the monsoon winds were too weak to break down water-column stratification, which prevents nutrients reentering the surface. Then, nutrients at the surface layer were depleted by light-saturated photosynthesis while nutrients at the DCM layer were abundant due to light limitation on nutrient uptake. Therefore, the community structure at the DCM layer was distinct from that at the surface layer because of the combined effects of photoadaptation and elevated nutrients, and decreased water temperature might play a role as well. Thus, it was not surprising that depth was the governing factor of the beta diversity (Countway et al., 2010; Wu et al., 2017a; Giner et al., 2020).
However, most of the variation in community structure were explained by neutral processes (Figures 5, 6), which was consistent with previous studies (Sloan et al., 2006). Furthermore, the NSTruzicka was higher for nanoeukaryotes than for picoeukaryotes, and the VPA results also indicated that nanoeukaryotes were subject to dispersal limitation while picoeukaryotes were not (Table 2). The latter was consistent with a previous study which suggested increasing dispersal limitation with cell size (de Vargas et al., 2015). Neutral community theory explains the species of a local community as a sample of the meta community regulated by immigration and extinction at the local level, therefore dissimilarities between local communities will be a function of the geographical distance (namely the dispersal limitation). The NCM displayed that the immigration rate for nanoeukaryotes was no less than that for picoeukaryotes (Figure 5). However, the number of individuals for the same amount of carbon biomass decreases with cell size, then the possibility of extinction at the local level will be higher for nanoeukaryotes than for picoeukaryotes, then the dispersal limitation might be explained. Ecological drift might be another mechanism accounting for the diversity of nanoeukaryotic community in this study. Ecological drift is stochastic changes in population resulted from equal chances of individuals to reproduce or die. Ecological drift drifts community composition from expectations of environmental filtering (Vellend, 2010). In this study, Dinophycease dominated the nanoeukaryotic communities by contributing two-thirds of the relative read abundance (Figures 3A,B), which might limit other taxa from establishing their population, leading to high similarity between local nanoeukaryotic communities.
The Kuroshio intrusion is supposed to mainly affect the eastern NSCS. However, Figure 1B shows the geostrophic currents in the NSCS in early May 2016 (a week before the cruise), which flowed into the NSCS through the northern Luzon Strait, and generated a westward branch at approximately 21°N. The current reached approximately 114°E and 20°N. Therefore, substantial fractions of Kuroshio water were found in the middle of the NSCS (Figure 1A). The VPA analysis showed that variations in the nanoeukaryotic community structure could be partially explained by the KI index. The reason could be different activities of archaea and bacteria that was reported by Xu et al. (2018). That study showed distinctly higher ammonia oxidation rates by archaea and bacteria in the MKI stations than in the LKI stations. Ammonia-oxidizing archaea and bacteria were dependent on dissolved organic matter, which was proportional to the faction of Kuroshio water.
Relative Metabolic Activities in Relation to Community Structure
Some argued that the discrepancy between the DNA and RNA surveys was mainly due to high rDNA copy numbers of some species or dead cells and did not reflect the metabolic activities, which, however, could be assessed by the relative changes of the RNA: DNA ratio between sampling sites (Hu et al., 2016). For example, a previous study in the northwestern Pacific Ocean reported different relative metabolic activities of Mamiellophyceae for different nutrient regimes (Wang et al., 2019). In this study, Mamiellophyceae also showed lower RNA: DNA ratios with increased nutrients (Figure 8B). Dinophyceae and MAST showed significantly higher RNA: DNA ratios at the DCM layers than at the surface layers (Figure 7B), which was consistent with a previous study in the South China Sea (Xu et al., 2017). That study reported that Dinophyceae, MALV-I, and MALV-II had the highest relative metabolic activities in middle layers (200, 300, and 500 m), followed by deep layers (1,000, 1,500, 2,000, and 3,900 m) and shallow layers (5, 25, and 75 m). Our analysis indicated that the relative metabolic activities of Dinophyceae and MAST increased with nutrients and the Chl a concentration (Figure 8).
In contrast, the correlations between the metabolic activities of nanoeukaryotes and environmental factors were not apparent (Figure 8). Nanophytoplankton were expected to have lower nutrient affinity than picophytoplankton, therefore nanophytoplankton would show larger changes of the relative metabolic activities than picophytoplankton for the ranges of nutrients in this study. However, our results contradicted this expectation. The metabolic activities of nanoeukaryotes might change parallelly, thus the changes were not reflected by the relative values. The relatively stable RNA: DNA ratios might account partially for the dominance of nanoeukaryotic Dinophyceae at all sampling sites (Figure 7B), but the underlying mechanism was not clear.
Conclusion
In the NSCS during the study period, Dinophyceae was the most diverse and sequenced nanoeukaryotic group revealed by both DNA and RNA surveys. In contrast, the relative read abundance of MAST, Pelagophyceae, and Dinophyceae in the size fraction of picoeukaryotes might be largely underestimated by the DNA survey. Environmental filtering played an important role in shaping the community structure, and the sampling depth became the governing factor of the beta diversity under the environmental setting of stratification during the study period. The spatial variations in the diversity of nanoeukaryotes were subject to the dispersal limitation under the size rule. The effects of the Kuroshio intrusion on the nanoeukaryotic community structure might also be explained by the dispersal limitation. Overall, neutral processes are critical in shaping the community structure of nanoeukaryotes. The relative metabolic activities of nanoeukaryotes were relatively stable in accordance with the high similarity of community structure between sampling sites. The responses of the relative metabolic activities of picoeukaryotes to environmental factors displayed two distinct patterns. Our findings improved the understanding of the nano- and pico-eukaryotic communities in the NSCS and the mechanisms of their assembly.
Data Availability Statement
The data presented in the study are deposited in the GenBank Sequence Read Archive repository, accession number PRJNA684778, https://www.ncbi.nlm.nih.gov/.
Author Contributions
FW, YX, and BH designed the experiments. FW carried out the experiments and wrote the manuscript. SC helped analyze the data. YX and BH provided critical feedback and revised the manuscript. XW and JM helped analyze the data and revised the manuscript. All authors contributed to the article and approved the submitted version.
Funding
This work was supported by grants from the National Natural Science Foundation of China (Nos. U1805241 and 41776146), Monitoring and Protection of Ecology and Environment in the East Pacific Ocean (No. DY135-E2-5-5), and the study was also partially supported by NSFC (No. 41890803) and Xiamen University Presidential Fund (20720180102).
Conflict of Interest
The authors declare that the research was conducted in the absence of any commercial or financial relationships that could be construed as a potential conflict of interest.
Publisher’s Note
All claims expressed in this article are solely those of the authors and do not necessarily represent those of their affiliated organizations, or those of the publisher, the editors and the reviewers. Any product that may be evaluated in this article, or claim that may be made by its manufacturer, is not guaranteed or endorsed by the publisher.
Acknowledgments
We thank JianYu Hu for providing the CTD data and Minhan Dai for proving the nutrient data. The nutrients concentration data could be acquired from the supporting information of Xu et al. (2018). This study also benefited from the discussion with Wenxue Wu at Sun Yat-sen University, and Lizhen Lin, Jixin Chen, and Heng Zhu at Xiamen University for their assistances in phytoplankton sample collections and analyses. We thank NSFC Shiptime Sharing Project for supporting the cruises by R/V Dongfanghong 2 (No. NORC2015-05), and we also acknowledge captains and crew for their cooperation during the cruise. We truly thank two reviewers whose comments helped to improve and clarify this manuscript.
Supplementary Material
The Supplementary Material for this article can be found online at: https://www.frontiersin.org/articles/10.3389/fmars.2021.658233/full#supplementary-material
Footnotes
References
Adl, S. M., Bass, D., Lane, C. E., Lukeš, J., Schoch, C. L., Smirnov, A., et al. (2019). Revisions to the classification, nomenclature, and diversity of eukaryotes. J. Eukaryot. Microbiol. 66, 4–119. doi: 10.1111/jeu.12691
Altschul, S. F., Gish, W., Miller, W., Myers, E. W., and Lipman, D. J. (1990). Basic local alignment search tool. J. Mol. Biol. 215, 403–410. doi: 10.1016/S0022-2836(05)80360-2
Amaral-Zettler, L. A., Mccliment, E. A., Ducklow, H. W., and Huse, S. M. (2009). A method for studying protistan diversity using massively parallel sequencing of v9 hypervariable regions of small-subunit ribosomal RNA genes. PLoS One 4:e6372. doi: 10.1371/journal.pone.0006372
Bachvaroff, T. R., Kim, S., Guillou, L., Delwiche, C. F., and Coats, D. W. (2012). Molecular diversity of the syndinean genus Euduboscquella based on single-cell PCR analysis. Appl. Environ. Microbiol. 78, 334–345. doi: 10.1128/aem.06678-11
Bahram, M., Kohout, P., Anslan, S., Harend, H., Abarenkov, K., and Tedersoo, L. (2016). Stochastic distribution of small soil eukaryotes resulting from high dispersal and drift in a local environment. ISME J. 10, 885–896. doi: 10.1038/ismej.2015.164
Barton, A. D., Pershing, A. J., Litchman, E., Record, N. R., Edwards, K. F., Kiørboe, T., et al. (2013). The biogeography of marine plankton traits. Ecol. Lett. 16, 522–534. doi: 10.1111/ele.12063
Berge, T., Hansen, P. J., and Moestrup, Ø (2008). Prey size spectrum and bioenergetics of the mixotrophic dinoflagellate Karlodinium armiger. Aquat. Microb. Ecol. 50, 289–299. doi: 10.3354/ame01166
Blazewicz, S. J., Barnard, R. L., Daly, R. A., and Firestone, M. K. (2013). Evaluating rRNA as an indicator of microbial activity in environmental communities: limitations and uses. ISME J. 7, 2061–2068. doi: 10.1038/ismej.2013.102
Borcard, D., Legendre, P., and Drapeau, P. (1992). Partialling out the spatial component of ecological variation. Ecology 73, 1045–1055. doi: 10.2307/1940179
Caporaso, J. G., Kuczynski, J., Stombaugh, J., Bittinger, K., Bushman, F. D., Costello, E. K., et al. (2010). QIIME allows analysis of high-throughput community sequencing data. Nat. Methods 7, 335–336. doi: 10.1038/nmeth.f.303
Caron, D. A., Alexander, H., Allen, A. E., Archibald, J. M., Armbrust, E. V., Bachy, C., et al. (2016). Probing the evolution, ecology and physiology of marine protists using transcriptomics. Nat. Rev. Microbiol. 15, 6–20. doi: 10.1038/nrmicro.2016.160
Caron, D. A., Countway, P. D., Jones, A. C., Kim, D. Y., and Schnetzer, A. (2012). Marine protistan diversity. Annu. Rev. Mar. Sci. 4, 467–493.
Chen, W. D., Pan, Y. B., Yu, L. Y., Yang, J., and Zhang, W. J. (2017). Patterns and processes in marine microeukaryotic community biogeography from Xiamen coastal waters and intertidal sediments, Southeast China. Front. Microbiol. 8:1912. doi: 10.3389/fmicb.2017.01912
Countway, P. D., Gast, R. J., Dennett, M. R., Savai, P., Rose, J. M., and Caron, D. A. (2010). Distinct protistan assemblages characterize the euphotic zone and deep sea (2500 m) of the western north Atlantic (Aargasso Sea and Gulf Stream). Environ. Microbiol. 9, 1219–1232. doi: 10.1111/j.1462-2920.2007.01243.x
de Vargas, C., Audic, S., Henry, N., Decelle, J., Mahé, F., Logares, R., et al. (2015). Eukaryotic plankton diversity in the sunlit ocean. Science 348:1261605. doi: 10.1126/science.1261605
Du, C., Liu, Z., Dai, M., Kao, S. J., and Li, Y. (2013). Impact of the Kuroshio intrusion on the nutrient inventory in the upper northern South China Sea: insights from an isopycnal mixing model. Biogeosciences 10, 6419–6432. doi: 10.5194/bg-10-6419-2013
Edgar, R. C. (2013). UPARSE: highly accurate OTU sequences from microbial amplicon reads. Nat. Methods 10, 996–998. doi: 10.1038/NMETH.2604
Edgar, R. C., Haas, B. J., Clemente, J. C., Quince, C., and Knight, R. (2011). UCHIME improves sensitivity and speed of chimera detection. Bioinformatics 27, 2194–2200. doi: 10.1093/bioinformatics/btr381
Edgcomb, V., Orsi, W., Bunge, J., Jeon, S., Christen, R., Leslin, C., et al. (2011). Protistan microbial observatory in the Cariaco Basin, Caribbean. I. Pyrosequencing vs Sanger insights into species richness. ISME J. 5, 1344–1356. doi: 10.1038/ismej.2011.6
Giner, C. R., Pernice, M. C., Balagué, V., Duarte, C. M., and Massana, R. (2020). Marked changes in diversity and relative activity of picoeukaryotes with depth in the world ocean. ISME J. 14, 437–449. doi: 10.1038/s41396-019-0506-9
Glibert, P. M., Burkholder, J. M., Kana, T. M., Alexander, J., Skelton, H., and Shilling, C. (2009). Grazing by Karenia brevis on Synechococcus enhances its growth rate and may help to sustain blooms. Aquat. Microb. Ecol. 55, 17–30. doi: 10.3354/ame01279
Gómez, F., and Boicenco, F. (2004). An annotated checklist of dinoflagellates in the Black Sea. Hydrobiologia 517, 43–59. doi: 10.1023/B:HYDR.0000027336.05452.07
Grob, C., Ulloa, O., Li, W., Alarcón, G., Fukasawa, M., and Watanabe, S. (2007). Picoplankton abundance and biomass across the eastern South Pacific Ocean along latitude 32.5∘S. Mar. Ecol. Prog. Ser. 332, 53–62. doi: 10.3354/meps332053
Guillou, L., Viprey, M., Chambouvet, A., Welsh, R. M., Kirkham, A. R., Massana, R., et al. (2008). Widespread occurrence and genetic diversity of marine parasitoids belonging to Syndiniales (Alveolata). Environ. Microbiol. 10, 3349–3365. doi: 10.1111/j.1462-2920.2008.01731.x
Haeckel, E. (1887). “Report on the radiolaria collected by H.M.S. Challenger during the years 1873–1876. pp. i–viii [=1–8], i–clxxxviii [=1–188], 1–1803,” in Report of the Scientific Results of the Voyage of H.M.S. Challenger During the Years 1873–1876 Under the Com-mand of Captain George S. Nares and Captain Frank Tourle Thomson, Zoology. vol. XVIII [=18]., eds C. W. Thomson and J. Murray (London, Edinburgh, Dublin), 1–140.
Hartmann, M., Grob, C., Tarran, G. A., Martin, A. P., Burkill, P. H., Scanlan, D. J., et al. (2012). Mixotrophic basis of Atlantic oligotrophic ecosystems. Proc. Natl. Acad. Sci. U. S. A. 109, 5756–5760. doi: 10.1073/pnas.1118179109
Hu, S. K., Campbell, V., Connell, P., Gellene, A. G., Liu, Z., Terrado, R., et al. (2016). Protistan diversity and activity inferred from RNA and DNA at a coastal site in the eastern North Pacific. FEMS Microbiol. Ecol. 92:fiw050. doi: 10.1093/femsec/fiw050
Huang, Y., Laws, E., Chen, B., and Huang, B. (2019). Stimulation of heterotrophic and autotrophic metabolism in the mixing zone of the Kuroshio Current and northern South China Sea: implications for export production. J. Geophys. Res. Biogeo. 124, 2645–2661. doi: 10.1029/2018JG004833
Hubbell, S. P. (2011). The Unified Neutral Theory of Biodiversity and Biogeography (MPB-32). Princeton: Princeton University Press.
Hulburt, E. M. (1957). The taxonomy of unarmored Dinophyceae of shallow embayments on Cape Cod, Massachusetts. Biol. Bull. 112, 196–219. doi: 10.2307/1539198
Jardillier, L., Zubkov, M. V., Pearman, J., and Scanlan, D. J. (2010). Significant CO2 fixation by small prymnesiophytes in the subtropical and tropical northeast Atlantic Ocean. ISME J. 4, 1180–1192. doi: 10.1038/ismej.2010.36
Jeong, H. J., Yoo, Y. D., Kang, N. S., Rho, J. R., Seong, K. A., Park, J. W., et al. (2010). Feeding by red-tide dinoflagellate Gymnodinium aureolum from the western Korean waters. Aquat. Microb. Ecol. 59, 239–255.
Jeong, H. J., Yoo, Y. D., Kim, J. S., Kim, T. H., Kim, J. H., Kang, N. S., et al. (2004). Mixotrophy in the phototrophic harmful alga Cochlodinium polykrikoides (Dinophycean): prey species, the effects of prey concentration and grazing impact. J. Eukaryot. Microbiol. 51, 563–569. doi: 10.1111/j.1550-7408.2004.tb00292.x
Jessup, D. A., Miller, M. A., Ryan, J. P., Nevins, H. M., Kerkering, H. A., Mekebri, A., et al. (2009). Mass stranding of marine birds caused by a surfactant-producing red tide. PLoS One 4:e4550. doi: 10.1371/journal.pone.0004550
Katano, T., Yoshida, M., Yamaguchi, S., Hamada, T., Yoshino, K., and Hayami, Y. (2011). Diel vertical migration and cell division of bloom-forming dinoflagellate Akashiwo sanguinea in the Ariake Sea, Japan. Plankton Benthos. Res. 6, 92–100. doi: 10.3800/pbr.6.92
Leblanc, K., Quéguiner, B., Diaz, F., Cornet, V., Michel-Rodriguez, M., Xavier, D. D. M., et al. (2018). Nanoplanktonic diatoms are globally overlooked but play a role in spring blooms and carbon export. Nat. Commun. 9:953.
Li, L., and Endo, K. (2020). Phylogenetic positions of “pico-sized” radiolarians from middle layer waters of the tropical Pacific. Prog. Earth. Planet. Sci. 7:70. doi: 10.1186/s40645-020-00384-6
Li, P., Li, W., Dumbrell, A. J., Liu, M., Li, G., Wu, M., et al. (2020). Spatial variation in soil fungal communities across paddy fields in subtropical China. mSystems 5, e00704–19. doi: 10.1128/mSystems.00704-19
Lim, W. A., Lee, Y. S., and Lee, S. G. (2008). Characteristic of environmental factors related to outbreak and decline of Cochlodinium polykrikoides bloom in the southeast coastal waters of Korea, 2007. J. Korean Soc. Oceanogr. 13, 325–332.
Lin, Y., Campbell, T., Chung, C., Gong, G., Chiang, K., and Worden, A. (2012). Distribution patterns and phylogeny of marine stramenopiles in the north Pacific Ocean. Appl. Environ. Microbiol. 78, 3387–99. doi: 10.1128/AEM.06952-11
Liu, J., Meng, Z., Liu, X., and Zhang, X. (2019). Microbial assembly, interaction, functioning, activity and diversification: a review derived from community compositional data. Mar. Life Sci. Technol. 1, 112–128. doi: 10.1007/s42995-019-00004-3
Liu, K., Chao, S., Shaw, P., Gong, G., Chen, C., and Tang, T. (2002). Monsoon-forced chlorophyll distribution and primary production in the South China Sea: observations and a numerical study. Deep-Sea Res. I Oceanogr. 49, 1387–1412. doi: 10.1016/S0967-0637(02)00035-3
Logares, R., Lindström, E. S., Langenheder, S., Logue, J. B., Paterson, H., Laybourn-Parry, J., et al. (2013). Biogeography of bacterial communities exposed to progressive long-term environmental change. ISME J. 7, 937–948. doi: 10.1038/ismej.2012.168
Lu, S. H., and Hodgkiss, I. J. (2004). Harmful algal bloom causative collected from Hong Kong waters. Hydrobiologia 512, 231–238. doi: 10.1023/B:HYDR.0000020331.75003.18
Lu, Y., Wen, Z., Shi, D., Lin, W., Bonnet, S., Dai, M., et al. (2019). Biogeography of N2 fixation influenced by the western boundary current intrusion in the South China Sea. J Geophys. Res. Ocean 124, 6983–6996. doi: 10.1029/2018JC014781
Massana, R., del Campo, J., Sieracki, M. E., Audic, S., and Logares, R. (2014). Exploring the uncultured microeukaryote majority in the oceans: reevaluation of ribogroups within stramenopiles. ISME J. 8, 854–866. doi: 10.1038/ismej.2013.204
Massana, R., Gobet, A., Audic, S., Bass, D., Bittner, L., Boutte, C., et al. (2015). Marine protist diversity in European coastal waters and sediments as revealed by high-throughput sequencing. Environ. Microbiol. 17, 4035–4049. doi: 10.1111/1462-2920.12955
Massana, R., Unrein, F., Rodriguez-Martinez, R., Forn, I., Lefort, T., Pinhassi, J., et al. (2009). Grazing rates and functional diversity of uncultured heterotrophic flagellates. ISME. J. 3, 588–596. doi: 10.1038/ismej.2008.130
McArdle, B. H., and Anderson, M. J. (2001). Fitting multivariate models to community data: a comment on distance-based redundancy analysis. Ecology 82, 290–297. doi: 10.2307/2680104
Nan, F., Xue, H., and Yu, F. (2015). Kuroshio intrusion into the South China Sea: a review. Prog. Oceangr. 137, 314–333. doi: 10.1016/j.pocean.2014.05.012
Ning, D., Deng, Y., Tiedje, J. M., and Zhou, J. (2019). A general framework for quantitatively assessing ecological stochasticity. Proc. Natl. Acad. Sci. U. S. A. 116, 16892–16898. doi: 10.1073/pnas.1904623116
Not, F., del Campo, J., Balagué, V., de Vargas, C., and Massana, R. (2009). New insights into the diversity of marine picoeukaryotes. PLoS One 4:e7143. doi: 10.1371/journal.pone.0007143
Park, M. G., Kim, S. J., Kim, H. S., Myung, G. O., Kang, Y. G., and Yih, W. H. (2006). First successful culture of the marine dinoflagellate Dinophysis acuminata. Aquat. Microb. Ecol. 45, 101–106. doi: 10.1007/BF00407285
Parris, D. J., Ganesh, S., Edgcomb, V. P., DeLong, E. F., and Stewart, F. J. (2014). Microbial eukaryote diversity in the marine oxygen minimum zone off northern Chile. Front. Microbiol. 5:543. doi: 10.3389/fmicb.2014.00543
Piwosz, K., Całkiewicz, J., Gołębiewski, M., and Creer, S. (2018). Diversity and community composition of pico- and nanoplanktonic protists in the Vistula River estuary (Gulf of Gdańsk, Baltic Sea). Estuar. Coast. Shelf Sci. 207, 242–249. doi: 10.1016/j.ecss.2018.04.013
Piwosz, K., Spich, K., Całkiewicz, J., Weydmann, A., Kubiszyn, A. M., and Wiktor, J. M. (2015). Distribution of small phytoflagellates along an Arctic fjord transect. Environ. Microbiol. 17, 2393–2406. doi: 10.1111/1462-2920.12705
Quast, C., Pruesse, E., Yilmaz, P., Gerken, J., Schweer, T., Yarza, P., et al. (2013). The SILVA ribosomal RNA gene database project: improved data processing and web-based tools. Nucleic Acids Res. 41, D590–D596. doi: 10.1093/nar/gks1219
R Core Team. (2014). R: a Language and Environment For Statistical Computing. Vienna: R Foundation for Statistical Computing.
Rachik, S., Christaki, U., Li, L. L., Genitsaris, S., Breton, E., and Monchy, S. (2018). Diversity and potential activity patterns of planktonic eukaryotic microbes in a mesoeutrophic coastal area (eastern English Channel). PLoS One 13:e0196987. doi: 10.1371/journal.pone.0196987
Ramond, P., Sourisseau, M., Simon, N., Romac, S., Schmitt, S., Rigaut-Jalabert, F., et al. (2019). Coupling between taxonomic and functional diversity in protistan coastal communities: functional diversity of marine protists. Environ. Microbiol. 21, 730–749. doi: 10.1111/1462-2920.14537
Reis-Filho, J. A., da Silva, E. M., Nunes, J. A. C. C., and Barros, F. (2012). Effects of a red tide on the structure of estuarine fish assemblages in northeastern Brazil. Internat. Rev. Hydrobiol. 97, 389–404. doi: 10.1002/iroh.201101457
Sassenhagen, I., Irion, S., Jardillier, L., Moreira, D., and Christaki, U. (2019). Protist interactions and community structure during early autumn in the Kerguelen region (Southern Ocean). Protist 171:125709. doi: 10.1016/j.protis.2019.125709
Seeleuthner, Y., Mondy, S., Lombard, V., Carradec, Q., Pelletier, E., Wessner, M., et al. (2018). Single-cell genomics of multiple uncultured stramenopiles reveals underestimated functional diversity across oceans. Nat. Commun. 9:310. doi: 10.1038/s41467-017-02235-3
Seong, K. A., Jeong, H. J., Kim, S., Kim, G. H., and Kang, J. H. (2006). Bacterivory by co-occurring red-tide algae, heterotrophic nanoflagellates, and ciliates. Mar. Ecol. Prog. Ser. 322, 85–97. doi: 10.3354/meps322085
Siano, R., Alves-de-Souza, C., Foulon, E., Bendif, E. M., Simon, N., Guillou, L., et al. (2011). Distribution and host diversity of Amoebophryidae parasites across oligotrophic waters of the Mediterranean Sea. Biogeosciences 8, 267–278. doi: 10.5194/bg-8-267-2011
Sloan, W. T., Lunn, M., Woodcock, S., Head, I. M., Nee, S., and Curtis, T. P. (2006). Quantifying the roles of immigration and chance in shaping prokaryote community structure. Environ. Microbiol. 8, 732–740. doi: 10.1111/j.1462-2920.2005.00956.x
Stegen, J. C., Lin, X. J., Konopka, A. E., and Fredrickson, J. K. (2012). Stochastic and deterministic assembly processes in subsurface microbial communities. ISME J. 6, 1653–1664. doi: 10.1038/ismej.2012.22
Stoecker, D. K. (1999). Mixotrophy among dinoflagellates. J. Eukaryot. Microbiol. 46, 397–401. doi: 10.1111/j.1550-7408.1999.tb04619.x
Unrein, F., Gasol, J. M., Not, F., Forn, I., and Massana, R. (2014). Mixotrophic haptophytes are key bacterial grazers in oligotrophic coastal waters. ISME J. 8, 164–176. doi: 10.1038/ismej.2013.132
Vellend, M. (2010). Conceptual synthesis in community ecology. Q. Rev. Biol. 85, 183–206. doi: 10.1086/652373
Wang, F., Xie, Y., Wu, W., Sun, P., Wang, L., and Huang, B. (2019). Picoeukaryotic diversity and activity in the northwestern Pacific Ocean based on rDNA and rRNA high-throughput sequencing. Front. Microbiol. 9:3259. doi: 10.3389/fmicb.2018.03259
Wang, L., Huang, B., Laws, E. A., Zhou, K., Liu, X., Xie, Y., et al. (2018). Anticyclonic eddy edge effects on phytoplankton communities and particle export in the northern South China Sea. J. Geophys. Res. Oceans 123, 7632–7650. doi: 10.1029/2017JC013623
Ward, B. A., and Follows, M. J. (2016). Marine mixotrophy increases trophic transfer efficiency, mean organism size, and vertical carbon flux. Proc. Natl. Acad. Sci. U. S. A. 113, 2958–2963. doi: 10.1073/pnas.1517118113
Welschmeyer, N. A. (1994). Fluorometric analysis of chlorophyll a in the presence of chlorophyll b and pheopigments. Limnol. Oceanogr. 39, 1985–1992. doi: 10.4319/lo.1994.39.8.1985
Wu, W., Huang, B., and Zhong, C. (2014). Photosynthetic picoeukaryote assemblages in the South China Sea from the Pearl River estuary to the SEATS station. Aquat. Microb. Ecol. 71, 271–284. doi: 10.3354/ame01681
Wu, W., and Liu, H. (2018). Disentangling protist communities identified from DNA and RNA surveys in the Pearl River-South China Sea Continuum during the wet and dry seasons. Mol. Ecol. 27, 4627–4640. doi: 10.1111/mec.14867
Wu, W., Logares, R., Huang, B., and Hsieh, H. (2017a). Abundant and rare picoeukaryotic sub-communities present contrasting patterns in the epipelagic waters of marginal seas in the northwestern Pacific Ocean. Environ. Microbiol. 19, 287–300. doi: 10.1111/1462-2920.13606
Wu, W., Lu, H., Sastri, A., Yeh, Y., Gong, G., Chou, W., et al. (2017b). Contrasting the relative importance of species sorting and dispersal limitation in shaping marine bacterial versus protist communities. ISME J. 12, 485–494. doi: 10.1038/ismej.2017.183
Wu, W., Wang, L., Liao, Y., Xu, S., and Huang, B. (2017c). Spatial and seasonal distributions of photosynthetic picoeukaryotes along an estuary to basin transect in the northern South China Sea. J. Plankton Res. 3, 1–13. doi: 10.1093/plankt/fbx017
Xiao, W., Wang, L., Edward, L., Xie, Y., Chen, Y., Liu, X., et al. (2018). Realized niches explain spatial gradients in seasonal abundance of phytoplankton groups in the South China Sea. Prog. Oceanogr. 162, 223–239. doi: 10.1016/j.pocean.2018.03.008
Xu, D., Li, R., Hu, C., Sun, P., Jiao, N., and Warren, A. (2017). Microbial Eukaryote diversity and activity in the water column of the South China Sea based on DNA and RNA high-throughput sequencing. Front. Microbiol. 8:1121. doi: 10.3389/fmicb.2017.01121
Xu, M., Zhang, W., Zhu, Y., Liu, L., Zheng, Z., Wan, X. S., et al. (2018). Enhanced ammonia oxidation caused by lateral Kuroshio intrusion in the boundary zone of the northern South China Sea. Geophys. Res. Lett. 45, 6585–6593. doi: 10.1029/2018GL077896
Yin, K., Qian, P., Chen, J., Hsieh, D., and Harrison, P. J. (2000). Dynamics of nutrients and phytoplankton biomass in the pearl river estuary and adjacent waters of Hong Kong during summer: preliminary evidence for phosphorus and silicon limitation. Mar. Ecol. Prog. Ser. 194, 295–305. doi: 10.3354/meps194295
Yin, K., Qian, P., Wu, M., Chen, J., Huang, L., Song, X., et al. (2001). Shift from P to N limitation of phytoplankton growth across the pearl river estuarine plume during summer. Mar. Ecol. Prog. Ser. 221, 17–28. doi: 10.1038/s41467-018-03376-9
Yu, L., Zhang, W., Liu, L., and Yang, J. (2015). Determining microeukaryotic plankton community around Xiamen Island, southeast China, using Illumina MiSeq and PCR-DGGE techniques. PLoS One 10:e0127721. doi: 10.1371/journal.pone.0127721
Keywords: nano- and pico-eukaryotes, RNA/DNA comparison, neutral process, Kuroshio intrusion, northern South China Sea
Citation: Wang F, Huang B, Xie Y, Cai S, Wang X and Mu J (2021) Diversity, Composition, and Activities of Nano- and Pico-Eukaryotes in the Northern South China Sea With Influences of Kuroshio Intrusion. Front. Mar. Sci. 8:658233. doi: 10.3389/fmars.2021.658233
Received: 25 January 2021; Accepted: 10 August 2021;
Published: 31 August 2021.
Edited by:
Xavier Pochon, The University of Auckland, New ZealandReviewed by:
Margaret Anne Mars Brisbin, Okinawa Institute of Science and Technology Graduate University, JapanFranck Lejzerowicz, University of California, San Diego, United States
Copyright © 2021 Wang, Huang, Xie, Cai, Wang and Mu. This is an open-access article distributed under the terms of the Creative Commons Attribution License (CC BY). The use, distribution or reproduction in other forums is permitted, provided the original author(s) and the copyright owner(s) are credited and that the original publication in this journal is cited, in accordance with accepted academic practice. No use, distribution or reproduction is permitted which does not comply with these terms.
*Correspondence: Bangqin Huang, YnFodWFuZ0B4bXUuZWR1LmNu