- 1Institute of Oceanography, National Taiwan University, Taipei, Taiwan
- 2Ecology and Evolutionary Biology, University of Michigan, Ann Arbor, MI, United States
- 3Department of Biotechnology and Bioindustry Sciences, National Cheng Kung University, Tainan, Taiwan
- 4Institute of Marine Environment and Ecology, National Taiwan Ocean University, Keelung, Taiwan
- 5Center of Excellence for the Oceans, National Taiwan Ocean University, Keelung, Taiwan
- 6Research Center for Environmental Changes, Academia Sinica, Taipei, Taiwan
- 7Institute of Ecology and Evolutionary Biology and Department of Life Science, National Taiwan University, Taipei, Taiwan
- 8National Center for Theoretical Sciences, Taipei, Taiwan
Predator and prey α-diversities are often positively associated; yet, understandings of the underlying mechanisms require manipulative experiments and thus remain unclear. We attempt to address this issue by deciphering how α-diversity of predator and prey influences each other’s community assembly processes, which subsequently determine their α-diversity. The occurrence of assembly processes was indicated by the mean pairwise taxonomic index within a community (αMPTI), assuming assembly processes left traceable imprints on species’ phylogeny. Specifically, αMPTI quantifies deviations of observed phylogenetic distances from that of random, so that it can be used to hint at the occurrence of non-random/deterministic assembly processes. Larger αMPTI of a community implies the occurrence of weaker homogenizing deterministic assembly processes, which suggests that this community might be comprised of less similar species and thus has higher α-diversity. We hypothesize that higher predator and prey α-diversity would be positively associated with each other’s αMPTI, which would then be positively associated with their α-diversity. To test the hypothesis, we calculated Shannon diversity and αMPTI for heterotrophic nanoflagellates (HNF; predator) and bacteria (prey) communities in the East China Sea (ECS). The HNF Shannon diversity was found to be positively associated with αMPTI of bacteria, which was then positively associated with bacterial Shannon diversity. In contrast, bacterial Shannon diversity did not correlate with HNF’s αMPTI. We argue that top-down control is one of the explanations to the positive α-diversity association among trophic levels in microbes of the ECS.
Introduction
The α-diversities of predator and prey communities are often found to be positively associated in natural systems (Haddad et al., 2009). To explain this positive association, studies have been conducted, including observational (Yang et al., 2018) and experimental (Haddad et al., 2009) studies as well as meta-analyses (Castagneyrol et al., 2012). The mechanisms proposed by these studies can be classified into three categories. The first one is that predator and prey α-diversities respond to the same external forces in the same direction so that they are positively correlated (e.g., Hawkins and Porter, 2003; Axmacher et al., 2009). The other two are related to trophic interactions between the predators and prey (Hunter and Price, 1992; Power, 1992) and can be further categorized into top-down or bottom-up control mechanisms. The top-down control mechanisms occur when predators either directly suppress the dominant prey and prevent competitive exclusion (Leibold, 1996; Proulx and Mazumder, 1998) or indirectly create an environment that fosters the diversity of prey (Borer et al., 2014). In contrast, bottom-up control mechanisms take place, while more diverse prey community provides greater diversity (Hutchinson, 1959; Southwood et al., 1979) or greater amount (Siemann, 1998; Srivastava and Lawton, 1998) of resources to promote predator diversity. These three mechanisms are not easy to disentangle because they can act jointly to determine diversity associations between predator and prey in nature.
To unveil the causes underlying the positive association between predator and prey α-diversities, manipulative experiments that include multiple trophic levels are often required (e.g., Scherber et al., 2010; Hertzog et al., 2016). Conducting this kind of complex and long-term experiments often costs large amount of labor and resources. Consequently, despite many efforts, identifying and disentangling the mechanisms remains challenging.
To address this issue, we propose an analytical framework that quantifies community assembly processes of predators and prey to decipher the bi-trophic diversity association. The community assembly processes are inferred from the degree of relatedness among species, i.e., phylogenetic similarity, within a community (Webb et al., 2002; Cavender-Bares et al., 2009) because phylogenetic similarity is considered as an imprint left by evolutionary and ecological processes (Mouquet et al., 2012; Gerhold et al., 2015). These community assembly processes have been synthesized in Vellend’s conceptual synthesis of community ecology (Vellend, 2010; Götzenberger et al., 2012), including four fundamental processes – ecological drift, selection, speciation, and dispersal. We propose to use phylogenetic similarity within a community as a hint of the occurrence of these processes that collectively determine the species composition and thus the diversity of a community (Logares et al., 2018).
Specifically, the analytical framework, we proposed here consists of two steps (Figure 1) to investigate mechanisms underlying the α-diversity association between predator and prey. First, we investigate how predator’s (or prey’s) α-diversity affects assembly processes of the other community. Then, we examine how the assembly processes of prey (or predator) community are associated with their own α-diversity. When we find significant effect of predator’s α-diversity on prey’s assembly processes, which are then significantly associated with the α-diversity of prey, we infer that top-down control mechanisms are likely to be responsible for the bi-trophic diversity association. On the other hand, significant association of prey’s α-diversity and predator’s assembly processes along with significant association between predator’s assembly processes and α-diversity would evidence bottom-up control mechanisms. When none of the above is found, the predator-prey diversity association may be a result of their congruent responses to the same external forces.
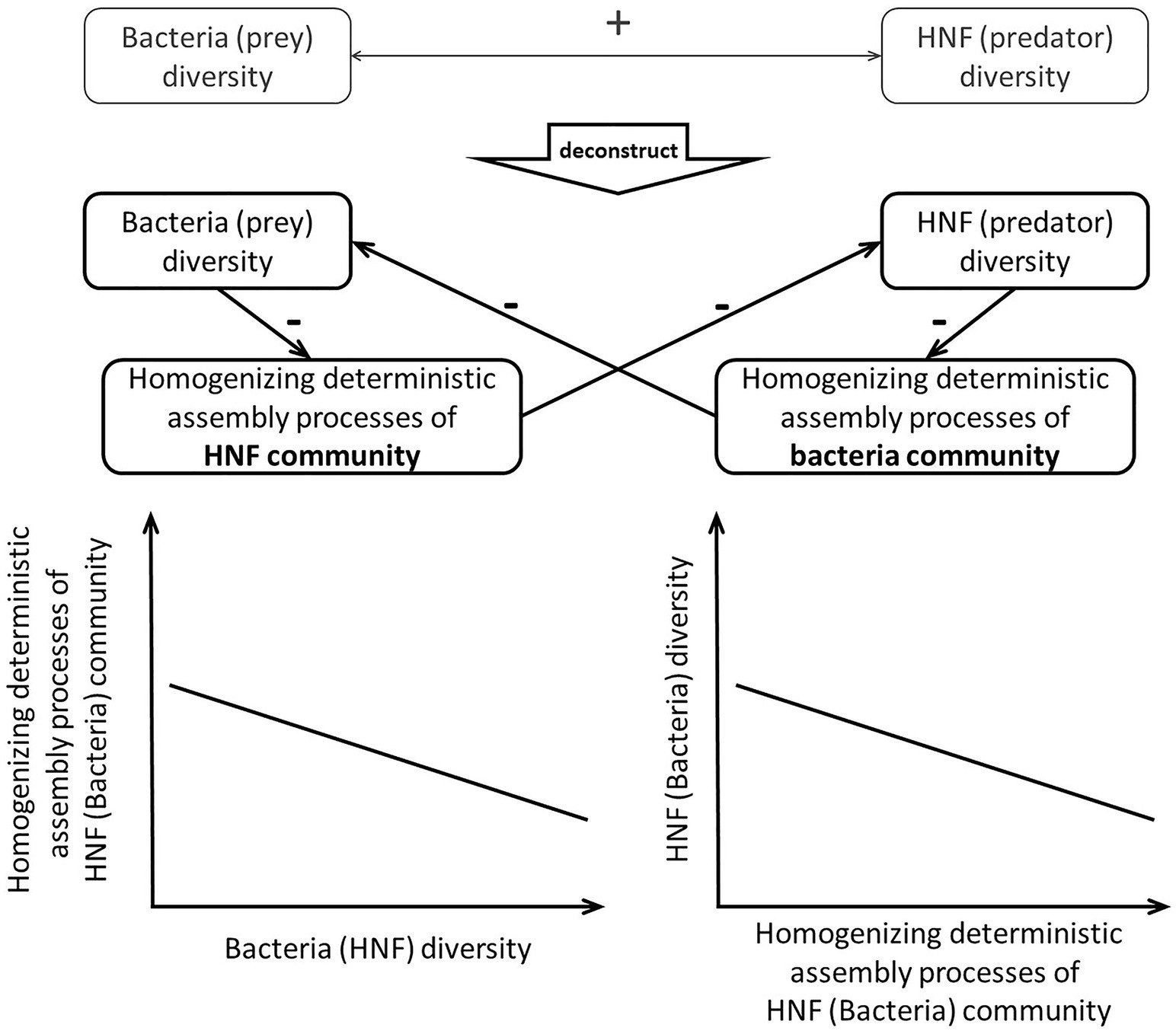
Figure 1. Schematic plots visualizing the two-step analytical framework and the ensuing expectations. We argue that the positive HNF (predator)-bacteria (prey) diversity association can be caused by top-down and/or bottom-up control mechanisms. The top-down control mechanisms should cause the HNF α-diversity to weaken the homogenizing deterministic assembly processes of bacteria community and thus lead to higher bacteria α-diversity. On the other hand, the bottom-up control mechanisms should cause the bacteria α-diversity to weaken the homogenizing deterministic assembly processes of bacteria community and thus lead to higher HNF α-diversity.
The first step of the analytical framework is to investigate the roles of predator and prey α-diversities in each other’s assembly processes. Here, we mainly focus on the two unambiguously deterministic processes, i.e., selection and speciation. We chose to make inferences based on deterministic processes because they result from species’ different responses given a set of biotic and/or abiotic environment, including predator-prey interactions (Vellend et al., 2014). The deterministic processes could thus be used to represent how those biotic and/or abiotic environments affect each other’s community diversity. In contrast, ecological drift is not our focus because it represents the random/stochastic events governing the presence of species in a community rather than predator-prey interactions (Hubbell, 2001). We also restrict ourselves from making inferences based on dispersal because dispersal could be deterministic or stochastic depending on whether species differ in dispersal ability or respond idiosyncratically to dispersal force (Nemergut et al., 2013; Lowe and McPeek, 2014). Consequently, in this study, we only investigate how the deterministic assembly processes of predator and prey community are affected by each other’s α-diversity.
After clarifying the effects of predator and prey α-diversity on each other’s deterministic assembly processes, we proceed to examine how the deterministic processes are associated with their own community diversity. Deterministic processes are positively or negatively associated with community diversity because those processes can weakly or strongly homogenize the community, respectively (MacArthur and Wilson, 1967; Zhou and Ning, 2017). When the deterministic processes weakly homogenize the community via selecting for different species and/or promoting speciation, species that are less similar to each other comprise a community. Such weak homogenizing deterministic processes should be positively associated with community diversity. On the other hand, when the deterministic processes impose consistent selection pressure to select for similar species or prevent speciation in a community (e.g., Dini-Andreote et al., 2015), species that are more similar to each other comprise a community. Such strong homogenizing processes should be negatively associated with community diversity. This second step in our analytical framework examines how the deterministic assembly processes (i.e., weak or strong homogenizing the community) is associated with community diversity. This framework can be used to deconstruct the positive α-diversity association in order to discern whether the positive association stems from the top-down control mechanisms or the bottom-up control mechanisms (Figure 1).
We apply this two-step analytical framework to deconstruct the α-diversity association between heterotrophic nanoflagellates (HNF) as the predators and bacteria as prey in the marine planktonic system of southern East China Sea (ECS). A previous study in the ECS has found a positive association between the α-diversity of HNF and bacteria, even after accounting for confounding environmental factors (Yang et al., 2018). According to our analytical framework, the positive α-diversity can result from the occurrence of either top-down control or bottom-up control, or both, mechanisms. When either mechanism occurs, the diversity of one trophic level is expected to make the deterministic processes weakly homogenizing the other community and thus lead to higher diversity of the other community. We thus hypothesize that the positive α-diversity association between bacteria and HNF results from the occurrence of either top-down control or bottom-up control, or both mechanisms.
Materials and Methods
Microbial Samples and Environmental Variables
Heterotrophic nanoflagellate and bacterial communities were revealed by using sequence data collected in the southern ECS from 14 cruises in the period of April 2014 to July 2017. In each sampling cruise, we visited the same six stations along a transect in the southern ECS (Supplementary Figure 1). Thus, a total of 84 samples each for HNF and bacteria were included in analysis. At each sampling, GoFlo bottles (General Oceanics) mounted on a conductivity, temperature, and depth profiler (CTD profiler, Sea-Bird Electronics, Bellevue, WA, United States) were used to collect 20 L of seawater from 5 m beneath surface. The seawater was first pre-filtered through a screen mesh with 20 μm openings to remove large organisms like zooplankton. The pre-filtered seawater was sequentially filtered onto 1.2 μm-pore-size filters (Millipore IsoporeTM hydrophilic polycarbonate membrane) to collect nanoflagellates and then onto 0.2 μm-pore-size filters (Millipore IsoporeTM hydrophilic polycarbonate membrane) to collect bacteria (Yang et al., 2018). After filtration, both the 0.2 and 1.2 μm-pore size filters were stored in liquid nitrogen and then at −20°C until molecular analysis (Yang et al., 2018).
Environmental variables, including temperature, salinity, and photosynthetic active radiation, were recorded by the CTD profiler. In addition, seawater samples for chlorophyll-a concentration as well as nutrient variables, including nitrite, nitrate, and phosphate concentrations, were collected and measured according to the standard methods developed by Gong et al. (2003).
DNA Extraction, Sequencing and Sequence Processing
We briefly introduced how we extracted DNA and analyzed sequences in this section (detailed methods are explained in Supplementary Material 1). Total DNA was extracted separately from the 0.2 and 1.2 μm-pore size filters with the PowerWater DNA Extraction Kit (PowerWater, Qiagen) according to the manufacturer’s instructions. DNA extracts from the 0.2 to 1.2-μm-pore size filters were used as templates of PCR to amplify the 16S rRNA gene for prey and 18S rRNA gene for predators, respectively. PCR was performed in two steps to gain better reproducibility and consistent results (Berry et al., 2011); see Supplementary Material 1 for details of the two-step PCR procedures. After obtaining 16S and 18S rRNA gene sequences, the DADA2 pipeline was used for quality filtering and assembling sequences into amplicon sequence variant (ASV; Callahan et al., 2016a; see Supplementary Material 1.2 for sequence merging procedures). Taxonomy assignment was performed on ASVs to recognize and select for predators from 18S rRNA gene and prey from 16S rRNA gene. From 18S rRNA gene, the Protist Ribosomal Reference database (PR2) database (Guillou et al., 2012; Vaulot and Guillou, 2019) was used to recognize taxonomy. Those belonging to the HNF taxonomy were further selected as the predators (see Supplementary Table 1 from Yang et al. (2018) for the list of HNF taxonomy). From 16S rRNA gene, the Silva 132 database (Quast et al., 2013) was used and only those classified under the bacteria kingdom were selected as prey. Finally, in order to obtain the phylogeny, we used maximum likelihood method (with negative edges length = 0; Callahan et al., 2016a) to build phylogenetic trees for bacteria and HNF from 16S rRNA gene and 18S rRNA gene of all cruises, respectively.
Quantifying Diversity of Predator (HNF) and Prey (Bacteria)
Before calculating the Shannon diversity index of HNF (predators) and bacteria (prey), each community was resampled once to achieve the same number of reads among stations, which were 331 for HNF and 13,129 for bacterial communities. The rarefaction yielded 93.76 ± 3.81 and 97.91 ± 0.69% sample coverage for HNF and bacterial communities, respectively (Supplementary Figure 2). Although this procedure addresses the disparity issue, i.e., unequal reads among stations, it is not appropriate when comparing the relative abundance of AVSs across stations (McMurdie and Holmes, 2014). Therefore, in order to have a fair among-station comparison, we applied method of Chao et al. (2014) to rarefy and estimate the expected Shannon diversity index of both HNF and bacteria communities for the following analyses (Chao and Chiu, 2016; Hsieh et al., 2016).
Inferring Community Assembly Processes
To hint the community assembly processes experienced by each HNF and bacteria community, we calculated the α mean pairwise taxonomic index (αMPTI). The αMPTI is akin to Webb’s net relatedness index (NRI; Webb, 2000; Webb et al., 2002), which quantifies the deviation of the observed mean pairwise phylogenetic distance (MPDobs) from a null distribution of mean pairwise phylogenetic distance (MPD). The MPDobs was the mean branch length among all pairs of species within each community. The null distribution of MPD was generated by random processes so that the distribution represented the values of MPD if a community is assembled by completely random processes, e.g., ecological drift. The deviation of MPDobs from the null thus represents the non-random/deterministic processes by which a community is assembled. To generate a null distribution of MPD by random processes, we first randomly shuffled the phylogeny (i.e., shuffled the tips of the phylogenetic tree) of all species within a community (i.e., each cruise-station). With the randomized phylogeny, we calculated MPD of each community. Repeating this randomization technique 999 times, we generated the null distribution of MPD. Finally, αMPTI = (MPDobs−meanMPDnull)/sdMPDnull, where meanMPDnull represents the mean of the null distribution of MPD, and sdMPDnull represents the SD of the null distribution of MPD.
When calculating αMPTI, we did not multiply −1 as in the NRI calculation (Webb, 2000; Webb et al., 2002). Therefore, the sign of αMPTI should more intuitively represent whether the observed phylogenetic distance is more different or less different from random. A positive value of αMPTI of a community means that species are phylogenetically more different than expected by random. Accordingly, positive αMPTI hints the occurrence of weak homogenizing assembly processes following the same logic, negative αMPTI hints the occurrence of strong homogenizing assembly processes.
α-Diversity Association and Impacts of Community Assembly Processes
Before testing our hypotheses, we first conducted univariate regression modeling to show that HNF and bacteria α-diversity were positively associated. To do so, we performed generalized linear mixed effect model (GLMM) to regress the HNF Shannon diversity on bacteria Shannon diversity, with cruise as the random effect. Here, making cruise as a random effect should account for the temporal autocorrelations among cruises. After confirming the positive association, we proceed to test the hypothesis that HNF and bacteria α-diversity negatively affects each other’s deterministic assembly processes and in turn increases each other’s α-diversity (Figure 1). To do so, we performed two sets of univariate GLMMs to separately test for top-down control mechanisms and bottom-up control mechanisms. Each set of univariate GLMM consisted of two steps corresponding to the two steps of our analytical framework. First, we regressed the αMPTI of bacteria (or HNF) community on the Shannon diversity of HNF (or bacteria) to test how the Shannon diversity of predator (or prey) influenced prey’s (or predator’s) αMPTI, i.e., community assembly processes. We then regressed the Shannon diversity of bacteria (or HNF) on its own αMPTI to test how the community assembly processes of bacteria (or HNF) determined its Shannon diversity.
The objective of this study is to detect the effects of ecological interactions by statistically accounting for other potential confounding environmental factors. Therefore, when conducting the two sets of GLMMs, we used backward selections to identify the variables that also have significant effects but did not attempt to make inference from those variables. To conduct backward selection, we first included all available environmental variables in the GLMMs and then step-wisely removed variables that are not significant based on p-values. The environmental variables include temperature, salinity, total dissolved inorganic nitrogen (TN), total dissolved inorganic phosphorous (TP), chlorophyll a (Chla), and photosynthetically active radiation (PAR). In addition, we built Moran’s Eigenvector Maps (MEM; Dray et al., 2006, 2012) that are orthogonal vectors to account for the spatial autocorrelations among stations. These spatial eigen-vectors were always included in the backward selection processes in order to account for the effects of dispersal because bacteria and HNF are passive dispersers (Declerck et al., 2013). In all above GLMM analyses, cruise was set as a random effect to avoid spurious correlations simply due to seasonal variation among cruises.
Computation
We used the “phyloseq” package to perform sequence subsampling to achieve parity in total number of reads (McMurdie and Holmes, 2013), the “iNEXT” package to perform rarefaction and calculate the Shannon diversity index (Hsieh et al., 2016), the “phangorn” package to build phylogenetic trees (Callahan et al., 2016b), the “picante” package to calculate phylogenetic distances to derive αMPTI (Kembel et al., 2010) and the “nlme” package to perform GLMMs (Pinheiro et al., 2019). All packages were built and computation was carried out in R version 4.0.4 (R Core Team, 2020).
Results
Hydrology, HNF (Predator) and Bacteria (Prey) α-Diversities in Southern East China Sea
In the southern Each China Sea, temperature and salinity were significantly lower at the most nearshore station than other stations, especially in the spring time (p < 0.01; Supplementary Figure 3). This reflects the influences of river runoff from the Min River. The river runoff caused the concentrations of total dissolved inorganic nitrogen and phosphorous as well as chlorophyll a to be significantly higher at the most nearshore station than others (p < 0.01; Supplementary Figure 3), except for the station northeast of Taiwan (station 9; Supplementary Figure 3). The region northeast of Taiwan has long been reported to be influenced by the upwelling of subsurface Kuroshio waters that provide a large amount of nutrients to the East China Sea (Wong et al., 2000). Although the hydrology varied in the southern East China Sea, the HNF (p = 0.8) and bacteria (p = 0.08) α-diversity did not exhibit such clear spatial variation (Supplementary Figure 3). Rather, we found a negative, but weak (R2 = 0.08), association between HNF Shannon diversity and chlorophyll a concentration (Supplementary Figure 4). This finding suggests that HNF communities might not be strongly affected by abiotic environmental factors (Yang et al., 2018). On the other hand, bacteria Shannon diversity was positively associated with salinity (Supplementary Figure 4), which is consistent with a previous study (Fuhrman et al., 2008).
α-Diversity Association Between HNF (Predator) and Bacteria (Prey) Community
After accounting for the aforementioned hydrological processes, we found a positive association between the Shannon diversity of HNF and bacteria in the southern ECS (Figure 2). The positive association was still significant after either setting station as a random effect (p = 0.02), or setting station as a random effect nested within cruise to account for spatial autocorrelation (p = 0.02).
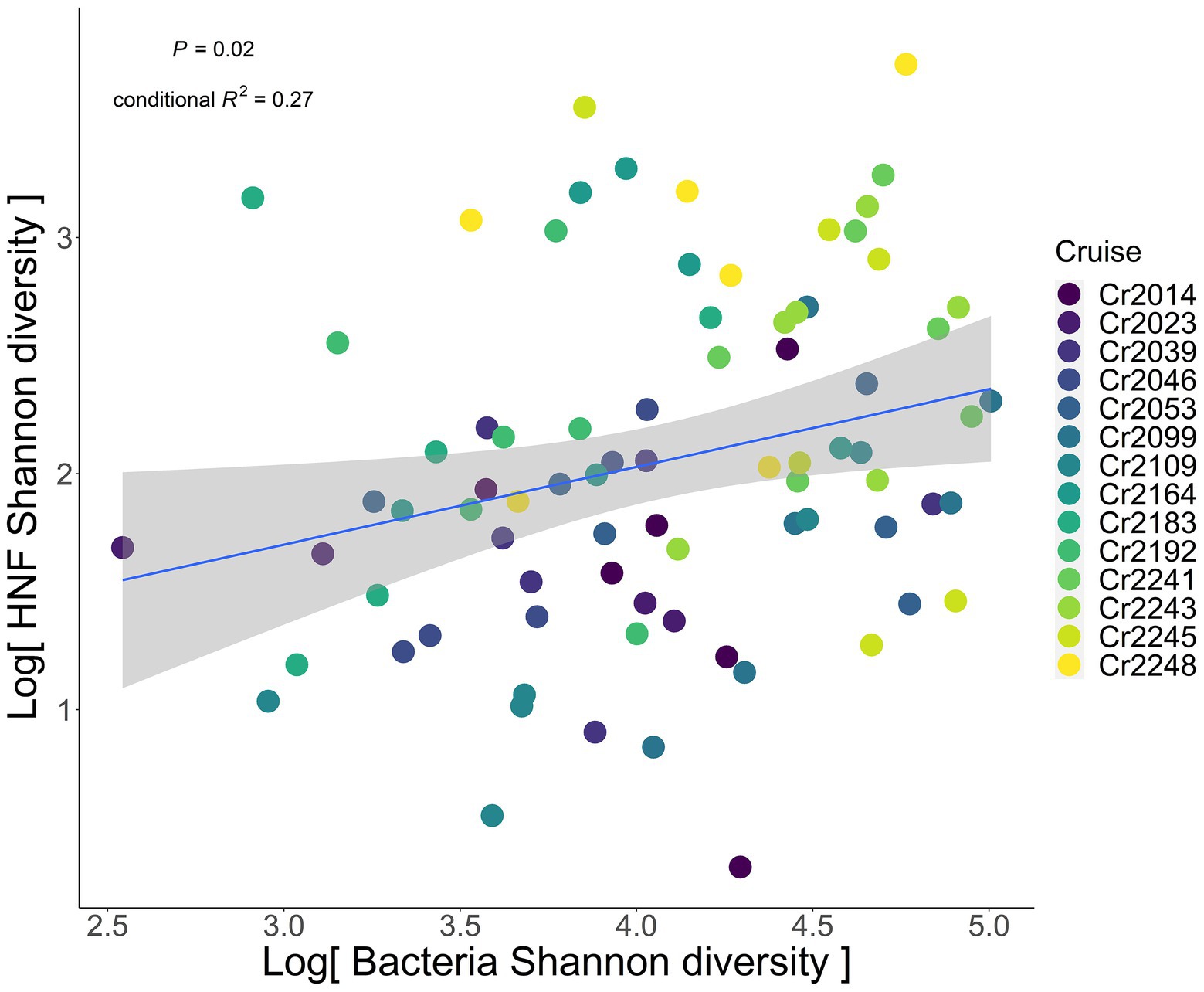
Figure 2. Relationship between heterotrophic nanoflagellate (predator) and bacteria (prey) Shannon diversity. Blue solid line indicates the significant regression line fitted by the GLMM, with cruise as the random effect. Gray shaded area represents the 95% confidence interval of the regression line. The colors of dots represent different sampling cruises.
In order to reveal whether the underlying mechanisms are top-down control and/or bottom-up control, we then applied the two-step analytical framework to deconstruct this positive association. From the top-down control perspective, we found that the αMPTI of bacteria community was not significantly associated with the Shannon diversity of HNF community with p = 0.1 (univariate regression coefficient = 0.27; Figure 3A). However, after accounting for other environmental variables via backward selections (Supplementary Tables 1 and 2), the αMPTI of bacteria community became significantly less negative with the increase of HNF Shannon diversity (Table 1). Less negative αMPTI of bacteria community was in turn associated with higher bacterial Shannon diversity (univariate regression coefficient = 0.23; p < 0.01; Figure 3B). These findings suggest that, after statistically accounting for the environmental factors, the α-diversity of HNF community possibly made the deterministic processes weakly homogenizing the bacteria community, which then led to higher diversity of bacteria community.
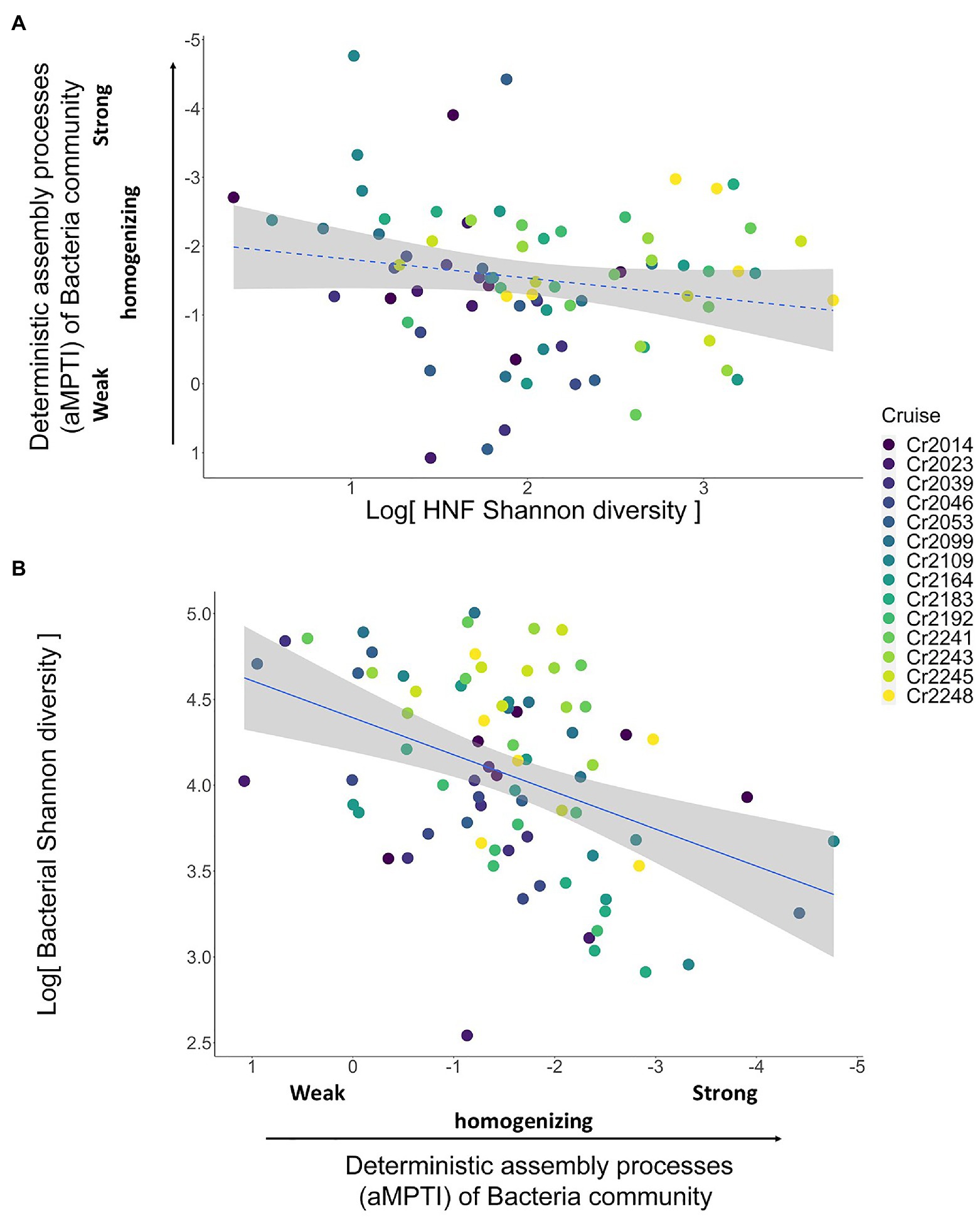
Figure 3. Scatterplots showing the test for top-down control mechanisms. Panel (A) shows the non-significant relationship with p = 0.1 (dashed blue line) between HNF Shannon diversity and the deterministic assembly processes (αMPTI) of bacteria community. This relationship becomes significant after including other environmental variables (Table 1). Panel (B) shows the significant relationship (solid blue line) between the αMPTI of bacteria community and the Shannon diversity of bacteria. Including other environmental variables does not qualitatively alter this result (Table 1). The p-value and R2 are derived from univariate GLMM. Note that the αMPTI of bacteria are mostly negative, so that, we reversed the y axis of panel (A) and x axis in panel (B) for visual intuition. The gray shaded area around the blue line indicates the 95% confidence interval of the regression line (GLMM). The colors of dots represent different sampling cruises.
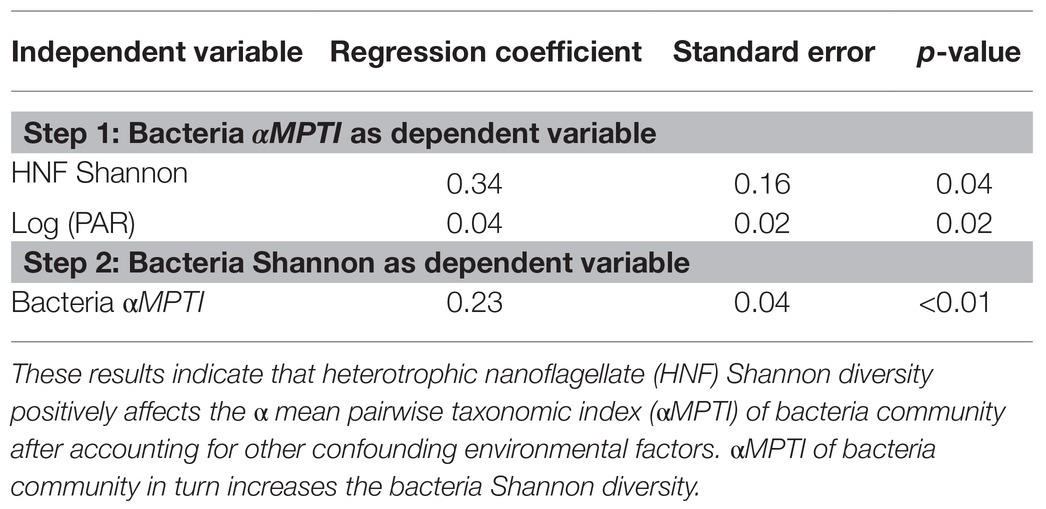
Table 1. Results of generalized linear mixed effect model (GLMM) after backward selection to test for top-down control mechanisms.
From the bottom-up control perspective, we first found that of HNF community was independent of the bacteria Shannon diversity (univariate regression p = 0.29; Figure 4A). Subsequently, we found that the HNF community did not significantly affect its Shannon diversity (p = 0.09; Figure 4B). Including other environmental variables did not alter these findings (Table 2). These results suggest that the bacteria α-diversity did not influence the assembly processes of HNF community, and these assembly processes did not determine the α-diversity of HNF community.
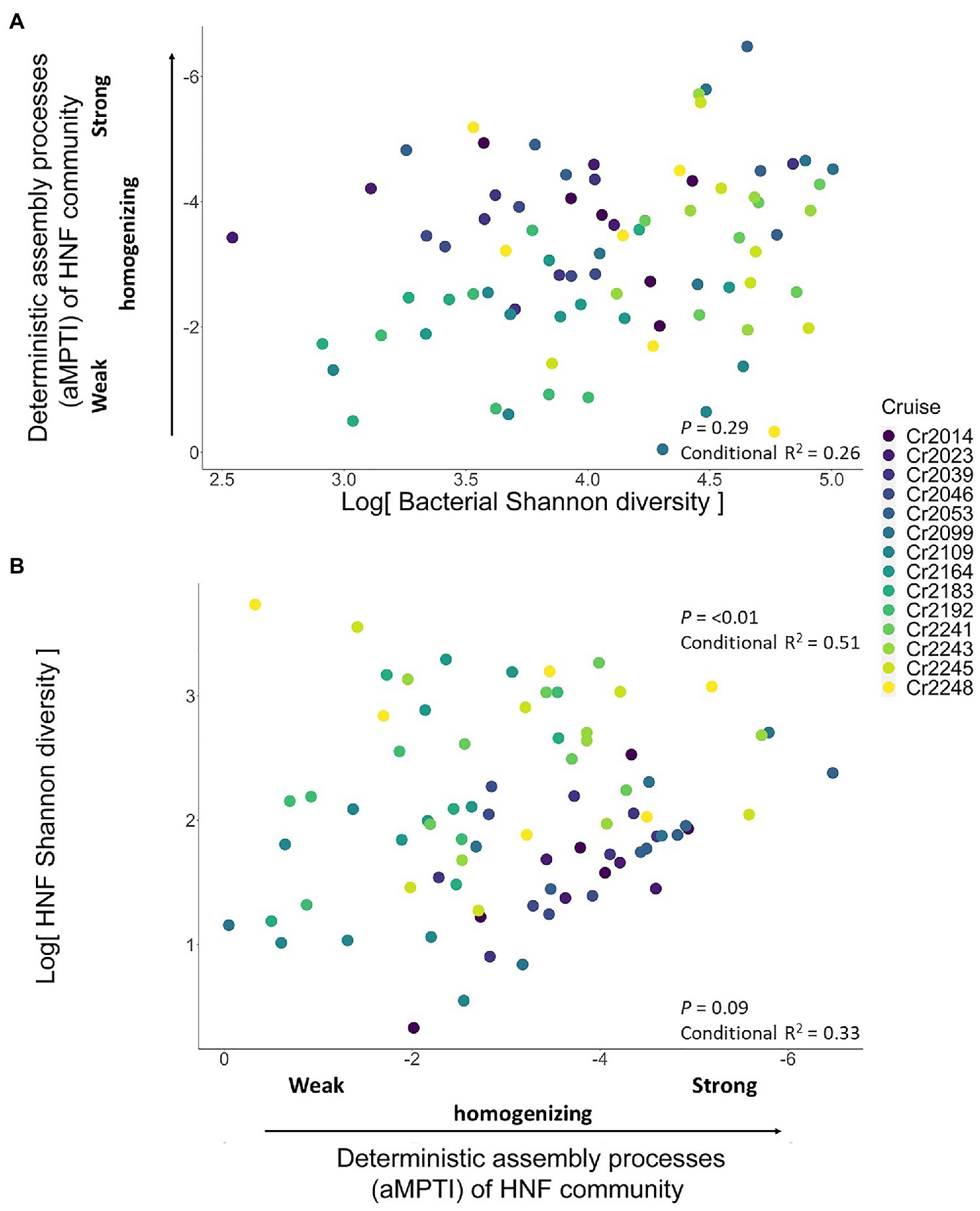
Figure 4. Scatterplots showing the test for bottom-up controlled mechanisms. Panel (A) shows the non-significant relationship (dashed blue line) between bacteria Shannon diversity and the deterministic assembly processes (αMPTI) of HNF community. Panel (B) shows the non-significant relationship (dashed blue line) between the αMPTI of HNF community and the Shannon diversity of HNF. The p-value and R2 are derived from univariate GLMM, whereas including other environmental variables does not qualitatively alter the conclusions (Table 1). Note that the αMPTI of bacteria are mostly negative, so that, we reversed the y axis of panel (A) and x axis in panel (B) for visual intuition. The gray shaded area around the blue line indicates the 95% confidence interval of the regression line (GLMM). The colors of dots represent different sampling cruises.
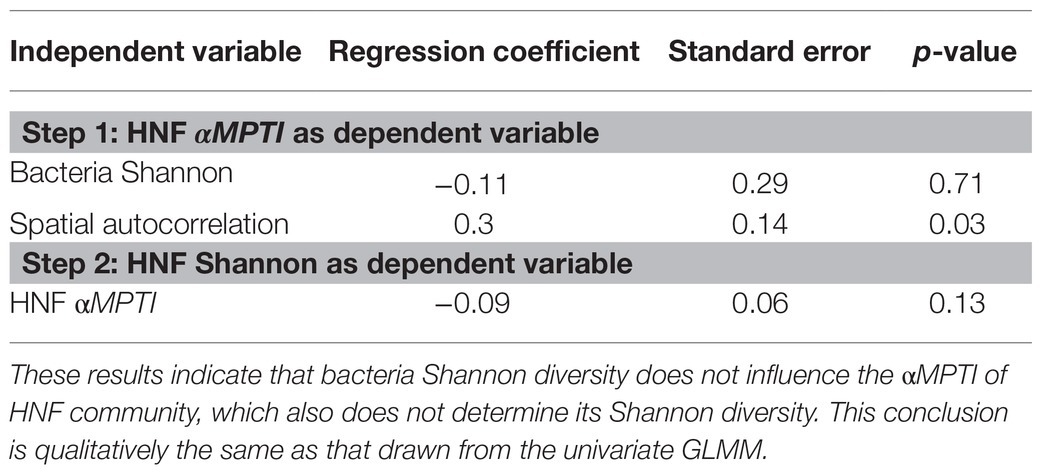
Table 2. Results of generalized linear mixed effect model (GLMM) after backward selection to test for top-down control mechanisms.
Discussion
In the southern ECS, the diversity of HNF (predator) and bacteria (prey) community are positively associated (Figure 2), which is consistent with a previous study that included data from the whole ECS (Yang et al., 2018). Positive association between predator and prey diversity is also observed in other aquatic systems (Aranguren-Riaño et al., 2011). More importantly, our novel analytical framework allows us to decipher how this positive association likely results from the associations between predator α-diversity and weak homogenizing deterministic assembly processes of prey community, which are then associated with higher prey α-diversity (Figure 3). Our findings echo the argument that top-down control mechanisms determine the α-diversity of prey community (Lovejoy et al., 2000; Vázquez-Domínguez et al., 2005a; Longnecker et al., 2010) and may propagate to affect energy flows in marine ecosystem (Vázquez-Domínguez et al., 2005b; Pradeep Ram et al., 2015).
The importance of top-down control mechanisms revealed in this study contradicts with many studies that emphasize the impacts of bottom-up control mechanisms (e.g., Scherber et al., 2010; Hertzog et al., 2016; Otero et al., 2020). One possible explanation to this disagreement is the fact that our data come from an aquatic system, whereas the majority studies demonstrating bottom-up control mechanisms is conducted in terrestrial systems (as reviewed in Haddad et al., 2009). In aquatic systems, predators have been demonstrated to impose larger impacts on prey than in terrestrial systems (Shurin and Borer, 2002; Shurin et al., 2006). Stronger predation pressures may act as a weak homogenizing deterministic processes for prey community, i.e., make the αMPTI of prey community less negative, and thus increase prey α-diversity. However, more detailed analyses are required to test this conjecture.
The prevalence of top-down control in aquatic systems implies that HNF community might also be controlled by their predators (Gasol, 1994; Segovia et al., 2014). Top-down control on HNF community is reportedly more prominent in productive regions because productive regions can sustain more diverse top predators, such as ciliates or other microzooplankton (Gasol et al., 2002; Pernthaler, 2005). Since the productivity in the southern East China Sea is not particularly low (Gong et al., 2000; Chen et al., 2004), it is possible that HNF diversity could be controlled by their predators instead of bacteria. Besides, lack of evidence for bottom-up control on HNF diversity could result from the fact that HNF have alternative food resources other than bacteria. It has been shown that HNF feed on picoeukaryotes with similar rate as that on prokaryotes (Parslow et al., 1986; Christaki et al., 2005). The α-diversity of HNF community could be affected not only by the diversity of bacteria but also picoeukaryotes. These speculations require empirical feeding experiments to be verified; these create some possibilities for future work.
The other possible explanation why the bottom-up control mechanisms are non-significant in our study is that the phylogeny of HNF in the southern ECS might not faithfully represents their ecological niche. Faithfully approximating species’ ecological niche using their phylogeny is a critical assumption that underpins the inferences based on species’ phylogeny (Losos, 2008). We did find significant phylogenetic signals, i.e., significant correlations phylogeny and habitat preferences, for both the HNF and bacteria community (Supplementary Figure 5). However, the phylogenetic signals of the HNF community were much weaker than that of bacteria (Supplementary Figure 5). For the HNF, the phylogenetic signal was only significant in a narrow range of phylogenetic distance (panel B of Supplementary Figure 5), meaning that the index based on phylogenetic distance (here, the αMPTI) might not completely represent the ecological processes experienced by the HNF community. Consequently, we do not completely rule out possible the occurrence of bottom-up control mechanisms in mediating bi-trophic diversity associations in the southern ECS.
We demonstrated that the top-down control mechanisms likely explain the positive association of predator and prey diversities; nevertheless, top-down control processes encompass a variety of detailed mechanisms (Hillebrand and Shurin, 2005). HNF have been shown to exhibit selective feeding behavior that can promote the diversity of their prey, including bacteria (Montagnes et al., 2008; Gerea et al., 2013). HNF have also been demonstrated to suppress dominant prey species and promote prey diversity, i.e., the kill the winner hypothesis (Winter et al., 2010). In addition, HNF, as predators, can indirectly increase prey diversity through regenerating resources (Attayde and Hansson, 1999), maintaining the diversity of resources for prey (Abrams, 2001), and/or trait-mediated indirect interactions (Werner and Peacor, 2003). For example, in a tri-trophic food web consisted of a basal resource (an isopod Jaera nordmanni), an intermediate consumer (an amphipod Echinogammarus marinus), and a top-predator fish (Lipophrys pholis), the presence of top-predator can reduce the feeding rate of an intermediate consumer (Alexander et al., 2013). Furthermore, both bacteria and HNF communities interact with other trophic groups, including marine viruses, phytoplankton, and zooplankton (Needham et al., 2017; Zeldis and Décima, 2020; Zimmerman et al., 2020). We cannot rule out the impacts from those organisms in this analysis. By including those trophic groups in the analysis could potentially resolve the puzzles. Clarifying the above mechanisms is a venue beyond the scope of this study but will render fruitful results.
One major concern of our analytical framework is that interpretations of αMPTI, or phylogenetic similarity in general, are controversial. Using phylogenetic similarity to approximate species’ ecological difference is an assumption commonly made in the field of microbial ecology (Webb, 2000; Webb et al., 2002). Ever since Webb developed the net related index (NTI) and nearest taxon index (NRI), species’ phylogenetic similarity has been used to indicate whether a community is subject to environmental filtering (e.g., Horner-Devine and Bohannan, 2006) or competition (e.g., Cooper et al., 2008). More recently, species’ phylogeny has been used to decipher the structure or underlying processes of a meta-community (Stegen et al., 2013, 2015; Dini-Andreote et al., 2015; Wu et al., 2018). Based on this assumption of using phylogenetic similarity to approximate species’ ecological difference, we developed the analytical framework with the attempt to decipher the positive association between predator and prey diversity. However, as suggested in several studies, phylogenetic similarity is an imperfect proxy of species’ ecological difference because ecological interactions, e.g., trophic interactions, might not leave traceable footprints on species’ evolutionary history. Species’ phylogenetic similarity thus may not be used to faithfully represent community assembly processes (Mayfield and Levine, 2010; Pavoine and Bonsall, 2011). We are fully aware of this controversy and believe that quantifying ecological interactions, e.g., feeding rates, is the most direct way to decipher predator and prey diversity associations. However, without resorting to manipulative experiments, here, we assume that phylogeny could approximate species’ ecological niche. Verifying this assumption requires further investigations so that we mindfully regard the αMPTI as an implication of the occurrence of certain community assembly processes. Through our analyses, we offer a possible explanation to the diversity association but our findings critically hinge on the fidelity of phylogeny to ecological niche.
In spite of some caveats, we still provide a useful analytical framework to better decipher why the diversity of predator and prey are associated. This analytical framework can be an alternative to manipulative experiments that are often required to understand the mechanisms underpinning the association between the diversity of predator and prey (e.g., Scherber et al., 2010; Hertzog et al., 2016). Besides being costly and labor intensive, manipulative experiments could suffer from unsatisfying spatial or temporal scale (Briggs and Borer, 2005). Our analytical framework can potentially provide an alternative approach that can be applied in any predator-prey system.
In summary, our analyses support top-down control as the underlying mechanisms of positive α-diversity association between HNF and bacteria in the southern ECS. We showed a positive association between predator α-diversity and weak homogenizing deterministic assembly processes of prey community, which then are positively associated with the α-diversity of prey community. These results suggest that predators are likely the driver of the positive association between predator and prey α-diversity in the southern ECS microbes.
Data Availability Statement
The datasets generated and analyzed for this study can be found in the NCBI Sequence Read Archive (SRA) under the accession numbers: PRJNA662424 (https://www.ncbi.nlm.nih.gov/sra/?term=PRJNA662424).
Author Contributions
F-HC and C-hH developed the hypothesis, conducted the analyses, and composed the manuscript. All other authors contributed equally to collect data and help polish the manuscript.
Funding
This work was supported by the National Center for Theoretical Sciences, National Taiwan University, Foundation for the Advancement of Outstanding Scholarship, and the Ministry of Science and Technology, Taiwan.
Conflict of Interest
The authors declare that the research was conducted in the absence of any commercial or financial relationships that could be construed as a potential conflict of interest.
Acknowledgments
We thank Hon-Tsen Yu for providing facilities and advice on laboratory work.
Supplementary Material
The Supplementary Material for this article can be found online at: https://www.frontiersin.org/articles/10.3389/fmars.2021.651565/full#supplementary-material
References
Abrams, P. A. (2001). The effect of density-independent mortality on the coexistence of exploitative competitors for renewing resources. Am. Nat. 158, 459–470. doi: 10.1086/323113
Alexander, M. E., Dick, J. T. A., and O’Connor, N. E. (2013). Trait-mediated indirect interactions in a marine intertidal system as quantified by functional responses. Oikos 122, 1521–1531. doi: 10.1111/j.1600-0706.2013.00472.x
Aranguren-Riaño, N., Guisande, C., and Ospina, R. (2011). Factors controlling crustacean zooplankton species richness in neotropical lakes. J. Plankton Res. 33, 1295–1303. doi: 10.1093/plankt/fbr028
Attayde, J. L., and Hansson, L.-A. (1999). Effects of nutrient recycling by zooplankton and fish on phytoplankton communities. Oecologia 121, 47–54. doi: 10.1007/s004420050906
Axmacher, J. C., Brehm, G., Hemp, A., Tünte, H., Lyaruu, H. V. M., Müller-Hohenstein, K., et al. (2009). Determinants of diversity in afrotropical herbivorous insects (Lepidoptera: Geometridae): plant diversity, vegetation structure or abiotic factors? J. Biogeogr. 36, 337–349. doi: 10.1111/j.1365-2699.2008.01997.x
Berry, D., Mahfoudh, B. K., Wagner, M., and Loy, A. (2011). Barcoded primers used in multiplex amplicon pyrosequencing bias amplification. Appl. Environ. Microbiol. 77, 7846–7849. doi: 10.1128/AEM.05220-11
Borer, E. T., Seabloom, E. W., Gruner, D. S., Harpole, W. S., Hillebrand, H., Lind, E. M., et al. (2014). Herbivores and nutrients control grassland plant diversity via light limitation. Nature 508, 517–520. doi: 10.1038/nature13144
Briggs, C. J., and Borer, E. T. (2005). Why short-term experiments may not allow long-term predictions about intraguild predation. Ecol. Appl. 15, 1111–1117. doi: 10.1890/04-1776
Callahan, B. J., McMurdie, P. J., Rosen, M. J., Han, A. W., Johnson, A. J. A., and Holmes, S. P. (2016a). DADA2: high-resolution sample inference from Illumina amplicon data. Nat. Methods 13, 581–583. doi: 10.1038/nmeth.3869
Callahan, B. J., Sankaran, K., Fukuyama, J. A., McMurdie, P. J., and Holmes, S. P. (2016b). Bioconductor workflow for microbiome data analysis: from raw reads to community analyses. F1000Research 5:1492. doi: 10.12688/f1000research.8986.2
Castagneyrol, B., Jactel, H., and Cardinale, B. J. (2012). Unraveling plant-animal diversity relationships: a meta-regression analysis. Ecology 93, 2115–2124. doi: 10.1890/11-1300.1
Cavender-Bares, J., Kozak, K. H., Fine, P. V. A., and Kembel, S. W. (2009). The merging of community ecology and phylogenetic biology. Ecol. Lett. 12, 693–715. doi: 10.1111/j.1461-0248.2009.01314.x
Chao, A., and Chiu, C.-H. (2016). Species richness: estimation and comparison. Wiley Stats Ref Stat. Ref. Online, 1–26.
Chao, A., Gotelli, N. J., Hsieh, T. C., Sander, E. L., Ma, K. H., Colwell, R. K., et al. (2014). Rarefaction and extrapolation with Hill numbers: a framework for sampling and estimation in species diversity studies. Ecol. Monogr. 84, 45–67. doi: 10.1890/13-0133.1
Chen, Y.-L. L., Chen, H.-Y., Gong, G.-C., Lin, Y.-H., Jan, S., and Takahashi, M. (2004). Phytoplankton production during a summer coastal upwelling in the East China Sea. Cont. Shelf Res. 24, 1321–1338. doi: 10.1016/j.csr.2004.04.002
Christaki, U., Vázquez-Domínguez, E., Courties, C., and Lebaron, P. (2005). Grazing impact of different heterotrophic nanoflagellates on eukaryotic (Ostreococcus tauri) and prokaryotic picoautotrophs (Prochlorococcus and Synechococcus). Environ. Microbiol. 7, 1200–1210. doi: 10.1111/j.1462-2920.2005.00800.x
Cooper, N., Rodríguez, J., and Purvis, A. (2008). A common tendency for phylogenetic overdispersion in mammalian assemblages. Proc. R. Soc. B Biol. Sci. 275, 2031–2037. doi: 10.1098/rspb.2008.0420
Declerck, S. A. J., Winter, C., Shurin, J. B., Suttle, C. A., and Matthews, B. (2013). Effects of patch connectivity and heterogeneity on metacommunity structure of planktonic bacteria and viruses. ISME J. 7, 533–542. doi: 10.1038/ismej.2012.138
Dini-Andreote, F., Stegen, J. C., van Elsas, J. D., and Salles, J. F. (2015). Disentangling mechanisms that mediate the balance between stochastic and deterministic processes in microbial succession. Proc. Natl. Acad. Sci. U. S. A. 112, E1326–E1332. doi: 10.1073/pnas.1414261112
Dray, S., Legendre, P., and Peres-Neto, P. R. (2006). Spatial modeling: a comprehensive framework for principal coordinate analysis of neighbour matrices (PCNM). Ecol. Model. 196, 483–493. doi: 10.1016/j.ecolmodel.2006.02.015
Dray, S., Pélissier, R., Couteron, P., Fortin, M.-J., Legendre, P., Peres-Neto, P. R., et al. (2012). Community ecology in the age of multivariate multiscale spatial analysis. Ecol. Monogr. 82, 257–275. doi: 10.1890/11-1183.1
Fuhrman, J. A., Steele, J. A., Hewson, I., Schwalbach, M. S., Brown, M. V., Green, J. L., et al. (2008). A latitudinal diversity gradient in planktonic marine bacteria. Proc. Natl. Acad. Sci. U. S. A. 105, 7774–7778. doi: 10.1073/pnas.0803070105
Gasol, J. M. (1994). A framework for the assessment of top-down vs bottom-up control of heterotrophic nanoflagellate abundance. Mar. Ecol. Prog. Ser. 113, 291–300. doi: 10.3354/meps113291
Gasol, J. M., Pedros-Alio, C., and Vaque, D. (2002). Regulation of bacterial assemblages in oligotrophic plankton systems results from experimental and emperi. Antonie Van Leeuwenhoek 81, 435–452. doi: 10.1023/A:1020578418898
Gerea, M., Queimaliños, C., Schiaffino, M. R., Izaguirre, I., Forn, I., Massana, R., et al. (2013). In situ prey selection of mixotrophic and heterotrophic flagellates in Antarctic oligotrophic lakes: an analysis of the digestive vacuole content. J. Plankton Res. 35, 201–212. doi: 10.1093/plankt/fbs085
Gerhold, P., Cahill, J. F., Winter, M., Bartish, I. V., and Prinzing, A. (2015). Phylogenetic patterns are not proxies of community assembly mechanisms (they are far better). Funct. Ecol. 29, 600–614. doi: 10.1111/1365-2435.12425
Gong, G. C., Shiah, F. K., Liu, K. K., Wen, Y. H., and Liang, M.-H. (2000). Spatial and temporal variation of chlorophyll a, primary productivity and chemical hydrography in the southern East China Sea. Cont. Shelf Res. 20, 411–436. doi: 10.1016/S0278-4343(99)00079-5
Gong, G., Wen, Y., Wang, B., and Liu, G. (2003). Seasonal variation of chlorophyll a concentration, primary production and environmental conditions in the subtropical East China Sea. Deep Sea Res. Part II Top. Stud. Oceanogr. 50, 1219–1236. doi: 10.1016/S0967-0645(03)00019-5
Götzenberger, L., de Bello, F., Bråthen, K. A., Davison, J., Dubuis, A., Guisan, A., et al. (2012). Ecological assembly rules in plant communities-approaches, patterns and prospects. Biol. Rev. Camb. Philos. Soc. 87, 111–127. doi: 10.1111/j.1469-185X.2011.00187.x
Guillou, L., Bachar, D., Audic, S., Bass, D., Berney, C., Bittner, L., et al. (2012). The Protist ribosomal reference database (PR2): a catalog of unicellular eukaryote small sub-unit rRNA sequences with curated taxonomy. Nucleic Acids Res. 41, D597–D604. doi: 10.1093/nar/gks1160
Haddad, N. M., Crutsinger, G. M., Gross, K., Haarstad, J., Knops, J. M. H., and Tilman, D. (2009). Plant species loss decreases arthropod diversity and shifts trophic structure. Ecol. Lett. 12, 1029–1039. doi: 10.1111/j.1461-0248.2009.01356.x
Hawkins, B. A., and Porter, E. E. (2003). Does herbivore diversity depend on plant diversity? The case of California butterflies. Am. Nat. 161, 40–49. doi: 10.1086/345479
Hertzog, L. R., Meyer, S. T., Weisser, W. W., and Ebeling, A. (2016). Experimental manipulation of grassland plant diversity induces complex shifts in aboveground arthropod diversity. PLoS One 11:e148768. doi: 10.1371/journal.pone.0148768
Hillebrand, H., and Shurin, J. B. (2005). “Biodiversity and aquatic food webs,” in Aquatic Food Webs – An Ecosystem Approach. eds. A. Belgrano, U. Scharler, J. Dunne, and R. Ulanowicz (Oxford, United Kingdom: Oxford University Press), 184–197.
Horner-Devine, M. C., and Bohannan, B. J. M. (2006). Phylogenetic clustering and Overdispersion in bacterial communities. Ecology 87, S100–S108. doi: 10.1890/0012-9658(2006)87[100:PCAOIB]2.0.CO;2
Hsieh, T. C., Ma, K. H., and Chao, A. (2016). iNEXT: an R package for rarefaction and extrapolation of species diversity (Hill numbers). Methods Ecol. Evol. 7, 1451–1456. doi: 10.1111/2041-210X.12613
Hubbell, S. P. (2001). The Unified Neutral Theory of Biodiversity and Biogeography (MPB-32). Princeton, New Jersey, United States: Princeton University Press.
Hunter, M. D., and Price, P. W. (1992). Playing chutes and ladders: heterogeneity and the relative roles of bottom-up and top-down forces in natural communities. Ecology 73, 724–732.
Hutchinson, G. E. (1959). Homage to Santa Rosalia or why are there so many kinds of animals? Am. Nat. 93, 145–159. doi: 10.1086/282070
Kembel, S. W., Cowan, P. D., Helmus, M. R., Cornwell, W. K., Morlon, H., Ackerly, D. D., et al. (2010). Picante: R tools for integrating phylogenies and ecology. Bioinformatics 26, 1463–1464. doi: 10.1093/bioinformatics/btq166
Leibold, M. A. (1996). A graphical model of keystone predators in food webs: trophic regulation of abundance, incidence, and diversity patterns in communities. Am. Nat. 147, 784–812. doi: 10.1086/285879
Logares, R., Tesson, S. V. M., Canbäck, B., Pontarp, M., Hedlund, K., and Rengefors, K. (2018). Contrasting prevalence of selection and drift in the community structuring of bacteria and microbial eukaryotes. Environ. Microbiol. 20, 2231–2240. doi: 10.1111/1462-2920.14265
Longnecker, K., Wilson, M. J., Sherr, E. B., and Sherr, B. F. (2010). Effect of top-down control on cell-specific activity and diversity of active marine bacterioplankton. Aquat. Microb. Ecol. 58, 153–165. doi: 10.3354/ame01366
Losos, J. B. (2008). Phylogenetic niche conservatism, phylogenetic signal and the relationship between phylogenetic relatedness and ecological similarity among species. Ecol. Lett. 11, 995–1003. doi: 10.1111/j.1461-0248.2008.01229.x
Lovejoy, C., Legendre, L., Therriault, J.-C., Tremblay, J.-E., Klein, B., and Grant Ingram, R. (2000). Growth and distribution of marine bacteria in relation to nanoplankton community structure. Deep Sea Res. Part II Top. Stud. Oceanogr. 47, 461–487. doi: 10.1016/S0967-0645(99)00115-0
Lowe, W. H., and McPeek, M. A. (2014). Is dispersal neutral? Trends Ecol. Evol. 29, 444–450. doi: 10.1016/j.tree.2014.05.009
MacArthur, R. H., and Wilson, E. O. (1967). The Theory of Island Biogeography. Princeton, New Jersey, United States: Princeton University Press.
Mayfield, M. M., and Levine, J. M. (2010). Opposing effects of competitive exclusion on the phylogenetic structure of communities. Ecol. Lett. 13, 1085–1093. doi: 10.1111/j.1461-0248.2010.01509.x
McMurdie, P. J., and Holmes, S. (2013). Phyloseq: An R package for reproducible interactive analysis and graphics of microbiome census data. PLoS One 8:e61217. doi: 10.1371/journal.pone.0061217
McMurdie, P. J., and Holmes, S. (2014). Waste not, want not: why rarefying microbiome data is inadmissible. PLoS Comput. Biol. 10:e1003531. doi: 10.1371/journal.pcbi.1003531
Montagnes, D. J. S., Barbosa, A. B., Boenigk, J., Davidson, K., Jürgens, K., Macek, M., et al. (2008). Selective feeding behaviour of key free-living protists: avenues for continued study. Aquat. Microb. Ecol. 53, 83–98. doi: 10.3354/ame01229
Mouquet, N., Devictor, V., Meynard, C. N., Munoz, F., Bersier, L. F., Chave, J., et al. (2012). Ecophylogenetics: advances and perspectives. Biol. Rev. 87, 769–785. doi: 10.1111/j.1469-185X.2012.00224.x
Needham, D. M., Sachdeva, R., and Fuhrman, J. A. (2017). Ecological dynamics and co-occurrence among marine phytoplankton, bacteria and myoviruses shows microdiversity matters. ISME J. 11, 1614–1629. doi: 10.1038/ismej.2017.29
Nemergut, D. R., Schmidt, S. K., Fukami, T., O’Neill, S. P., Bilinski, T. M., Stanish, L. F., et al. (2013). Patterns and processes of microbial community assembly. Microbiol. Mol. Biol. Rev. 77, 342–356. doi: 10.1128/MMBR.00051-12
Otero, J., Álvarez-Salgado, X. A., and Bode, A. (2020). Phytoplankton diversity effect on ecosystem functioning in a coastal upwelling system. Front. Mar. Sci. 7:592255. doi: 10.3389/fmars.2020.592255
Parslow, J. S., Doucette, G. J., Taylor, F. J. R., and Harrison, P. J. (1986). Feeding by the zooflagellate Pseudobodo sp. on the picoplanktonic prasinomonad Micromonas pusilla. Mar. Ecol. Prog. Ser. 29, 237–246. doi: 10.3354/meps029237
Pavoine, S., and Bonsall, M. B. (2011). Measuring biodiversity to explain community assembly: a unified approach. Biol. Rev. 86, 792–812. doi: 10.1111/j.1469-185X.2010.00171.x
Pernthaler, J. (2005). Predation on prokaryotes in the water column and its ecological implications. Nat. Rev. Microbiol. 3, 537–546. doi: 10.1038/nrmicro1180
Pinheiro, J., Bates, D., DebRoy, S., and Sarkar, D., R Core Team (2019). nlme: Linear and nonlinear mixed effects models. R-project. Available at: https://cran.r-project.org/package=nlme (Accessed January 10, 2021).
Power, M. E. (1992). Top-down and bottom-up forces in food webs: do plants have primacy. Ecology 73, 733–746. doi: 10.2307/1940153
Pradeep Ram, A. S., Colombet, J., Perriere, F., Thouvenot, A., and Sime-Ngando, T. (2015). Viral and grazer regulation of prokaryotic growth efficiency in temperate freshwater pelagic environments. FEMS Microbiol. Ecol. 91, 1–12. doi: 10.1093/femsec/fiv002
Proulx, M., and Mazumder, A. (1998). Reversal of grazing impact on plant species richness in nutrient-poor vs. nutrient-rich ecosystems. Ecology 79, 2581–2592. doi: 10.1890/0012-9658(1998)079[2581:ROGIOP]2.0.CO;2
Quast, C., Pruesse, E., Yilmaz, P., Gerken, J., Schweer, T., Yarza, P., et al. (2013). The SILVA ribosomal RNA gene database project: improved data processing and web-based tools. Nucleic Acids Res. 41, D590–D596. doi: 10.1093/nar/gks1219
R Core Team (2020). R: a language and environment for statistical computing. Available at: https://www.r-project.org/ (Accessed January 10, 2021).
Scherber, C., Eisenhauer, N., Weisser, W. W., Schmid, B., Voigt, W., Fischer, M., et al. (2010). Bottom-up effects of plant diversity on multitrophic interactions in a biodiversity experiment. Nature 468, 553–556. doi: 10.1038/nature09492
Segovia, B. T., Pereira, D. G., Bini, L. M., and Velho, L. F. M. (2014). Effects of bottom-up and top-down controls on the temporal distribution of planktonic heterotrophic nanoflagellates are dependent on water depth. Hydrobiologia 736, 155–164. doi: 10.1007/s10750-014-1904-7
Shurin, J., and Borer, E. (2002). A cross ecosystem comparison of the strength of trophic cascades. Ecol. Lett. 5, 785–791. doi: 10.1046/j.1461-0248.2002.00381.x
Shurin, J. B., Gruner, D. S., and Hillebrand, H. (2006). All wet or dried up? Real differences between aquatic and terrestrial food webs. Proc. R. Soc. B Biol. Sci. 273, 1–9. doi: 10.1098/rspb.2005.3377
Siemann, E. (1998). Experimental tests of effects of plant productivity and diversity on grassland arthropod diversity. Ecology 79, 2057–2070. doi: 10.1890/0012-9658(1998)079[2057:ETOEOP]2.0.CO;2
Southwood, T. R. E., Brown, V. K., and Reader, P. M. (1979). The relationships of plant and insect diversities in succession. Biol. J. Linn. Soc. 12, 327–348. doi: 10.1111/j.1095-8312.1979.tb00063.x
Srivastava, D. S., and Lawton, J. H. (1998). Why more productive sites have more species: an experimental test of theory using tree-hole communities. Am. Nat. 152, 510–529. doi: 10.1086/286187
Stegen, J. C., Lin, X., Fredrickson, J. K., Chen, X., Kennedy, D. W., Murray, C. J., et al. (2013). Quantifying community assembly processes and identifying features that impose them. ISME J. 7, 2069–2079. doi: 10.1038/ismej.2013.93
Stegen, J. C., Lin, X., Fredrickson, J. K., and Konopka, A. E. (2015). Estimating and mapping ecological processes influencing microbial community assembly. Front. Microbiol. 6, 1–15. doi: 10.3389/fmicb.2015.00370
Vaulot, D., and Guillou, L. (2019). Protist ribosomal reference database (PR2) - SSU rRNA gene database.
Vázquez-Domínguez, E., Casamayor, E. O., Català, P., and Lebaron, P. (2005a). Different marine heterotrophic nanoflagellates affect differentially the composition of enriched bacterial communities. Microb. Ecol. 49, 474–485. doi: 10.1007/s00248-004-0035-5
Vázquez-Domínguez, E., Gasol, J. M., Agustí, S., Duarte, C. M., and Vaqué, D. (2005b). Growth and grazing losses of prokaryotes in the Central Atlantic Ocean. J. Plankton Res. 27, 1055–1066. doi: 10.1093/plankt/fbi074
Vellend, M. (2010). Conceptual systhesis in community ecology. Q. Rev. Biol. 85, 183–206. doi: 10.1086/652373
Vellend, M., Srivastava, D. S., Anderson, K. M., Brown, C. D., Jankowski, J. E., Kleynhans, E. J., et al. (2014). Assessing the relative importance of neutral stochasticity in ecological communities. Oikos 123, 1420–1430. doi: 10.1111/oik.01493
Webb, C. O. (2000). Exploring the phylogenetic structure of ecological communities: an example for rain forest trees. Am. Nat. 156, 145–155. doi: 10.1086/303378
Webb, C. O., Ackerly, D. D., McPeek, M. A., and Donoghue, M. J. (2002). Phylogenies and community ecology. Annu. Rev. Ecol. Syst. 33, 475–505. doi: 10.1146/annurev.ecolsys.33.010802.150448
Werner, E. E., and Peacor, S. D. (2003). A review of trait-mediated indirect interactions in ecological communities. Ecology 84, 1083–1100. doi: 10.1890/0012-9658(2003)084[1083:AROTII]2.0.CO;2
Winter, C., Bouvier, T., Weinbauer, M. G., and Thingstad, T. F. (2010). Trade-offs between competition and defense specialists among unicellular planktonic organisms: the “killing the winner” hypothesis revisited. Microbiol. Mol. Biol. Rev. 74, 42–57. doi: 10.1128/MMBR.00034-09
Wong, G. T. F., Chao, S.-Y., Li, Y.-H., and Shiah, F.-K. (2000). The Kuroshio edge exchange processes (KEEP) study — an introduction to hypotheses and highlights. Cont. Shelf Res. 20, 335–347. doi: 10.1016/S0278-4343(99)00075-8
Wu, W., Lu, H.-P., Sastri, A., Yeh, Y.-C., Gong, G.-C., Chou, W.-C., et al. (2018). Contrasting the relative importance of species sorting and dispersal limitation in shaping marine bacterial versus protist communities. ISME J. 12, 485–494. doi: 10.1038/ismej.2017.183
Yang, J. W., Wu, W., Chung, C. C., Chiang, K. P., Gong, G. C., and Hsieh, C. H. (2018). Predator and prey biodiversity relationship and its consequences on marine ecosystem functioning - interplay between nanoflagellates and bacterioplankton. ISME J. 12, 1532–1542. doi: 10.1038/s41396-018-0111-3
Zeldis, J. R., and Décima, M. (2020). Mesozooplankton connect the microbial food web to higher trophic levels and vertical export in the New Zealand subtropical convergence zone. Deep Sea Res. Part I Oceanogr. Res. Pap. 155:103146. doi: 10.1016/j.dsr.2019.103146
Zhou, J., and Ning, D. (2017). Stochastic community assembly: does it matter in microbial ecology? Microbiol. Mol. Biol. Rev. 81, e00002–e00017. doi: 10.1128/MMBR.00002-17
Keywords: biodiversity, community assembly processes, homogeneous versus heterogeneous selection, predator-prey diversity relationship, phylogeny
Citation: Chang F-H, Yang JW, Liu AC-H, Lu H-P, Gong GC, Shiah F-K and Hsieh C-h (2021) Community Assembly Processes as a Mechanistic Explanation of the Predator-Prey Diversity Relationship in Marine Microbes. Front. Mar. Sci. 8:651565. doi: 10.3389/fmars.2021.651565
Edited by:
Chin-Chang Hung, National Sun Yat-sen University, TaiwanReviewed by:
Antonio Bode, Spanish Institute of Oceanography, SpainBangqin Huang, Xiamen University, China
Copyright © 2021 Chang, Yang, Liu, Lu, Gong, Shiah and Hsieh. This is an open-access article distributed under the terms of the Creative Commons Attribution License (CC BY). The use, distribution or reproduction in other forums is permitted, provided the original author(s) and the copyright owner(s) are credited and that the original publication in this journal is cited, in accordance with accepted academic practice. No use, distribution or reproduction is permitted which does not comply with these terms.
*Correspondence: Chih-hao Hsieh, Y2hzaWVoQG50dS5lZHUudHc=