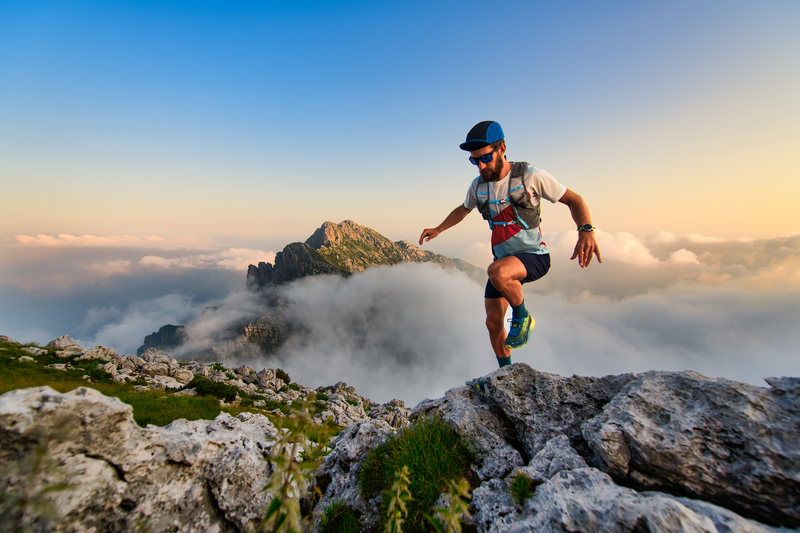
95% of researchers rate our articles as excellent or good
Learn more about the work of our research integrity team to safeguard the quality of each article we publish.
Find out more
ORIGINAL RESEARCH article
Front. Mar. Sci. , 23 August 2021
Sec. Marine Conservation and Sustainability
Volume 8 - 2021 | https://doi.org/10.3389/fmars.2021.650241
This article is part of the Research Topic Working Towards a Blue Future: Promoting Sustainability, Environmental Protection and Marine Management: Examples from the UK Government Blue Belt Programme and Current International Initiatives View all 23 articles
The sub-Antarctic South Sandwich Islands forms part of one of the largest marine protected areas (MPAs) in the world. Whilst the neighbouring island of South Georgia is known to be a biodiversity hotspot, very little was known about the benthic biodiversity or biogeography of the South Sandwich Islands. Here we present findings from the first biophysical assessment of this polar archipelago. Using open-access datasets, alongside results from a recent UK Government-funder Blue Belt expedition to the region, we assess how the island’s biodiversity is structured spatially and taxonomically and how this is driven by environmental factors. The South Sandwich Islands are shown to be both biologically rich, and biogeographically distinct from their neighbouring provinces. A gradient forest approach was used to map the archipelago’s benthic habitats which, based on the functional composition of benthic fauna and environmental characterisation of the benthic environment, demonstrated a distinct biogeographical north-south divide. This faunal and environmental discontinuity between the South Sandwich Islands and the rest of the MPA and between the different islands of the archipelago itself, highlights the importance of the zoned protection across the South Georgia and South Sandwich Islands Marine Protected Area.
The South Sandwich Islands (SSI) archipelago forms part of the Scotia Arc, a predominantly submarine ridge that extends from the Terra del Fuego region of South America to the Antarctic Peninsula (Figure 1). The archipelago consists of seven main volcanic islands stretching in a 390 km long island arc on a broadly north–south axis. The water surrounding the islands form part of the South Georgia and South Sandwich Islands Marine Protected Area (SGSSI MPA), covering over 1 million km2 and representing one of the largest MPAs in the world (Collins et al., 2012; Trathan et al., 2014).
Figure 1. (A) The geographical and oceanographic setting of South Georgia and South Sandwich Islands marine protected area (SGSSI MPA) (orange polygon) relative to the Polar Front (white line) and the Southern Antarctic Circumpolar Current Front (SACCF) (blue line). (B) The geographical setting of the South Sandwich Islands archipelago using bathymetry data from Leat et al. (2016), classified using 1,000 m depth contours.
Both the SSI and neighbouring South Georgia are internationally important sites for higher predators, hosting some of the largest populations of seabirds and marine mammals on Earth (Convey et al., 1999; Murphy et al., 2007; Clarke et al., 2012; Trathan et al., 2014; Rogers et al., 2015). Furthermore, South Georgia is recognised to be of global importance due to its abundant, diverse and unique benthic fauna (Barnes et al., 2011; Hogg et al., 2011). This diversity is thought to be driven by its proximity to powerful, nutrient rich currents, the age of the continental shelf (45-20 ma), its geographical isolation and large shelf area (Hogg et al., 2011). The SSI zone constitutes over half of the total area of the MPA, yet in contrast to South Georgia, relatively little is known about the benthic biodiversity of the island’s waters. Limited scientific sampling has been carried out as part of wider Antarctic or Scotia research expeditions (e.g., Ramos, 1999, Ramos-Esplá et al., 2005; Howe et al., 2004; Malyutina, 2004; Lockhart and Jones, 2008). Others sampling campaigns have been geographically restricted to individual islands, notably Southern Thule (e.g., Kaiser et al., 2008; Griffiths et al., 2009), or have had a focus on specific benthic communities, for example the chemosynthetic fauna of deep-sea hydrothermal vents (Marsh et al., 2012; Linse et al., 2019). Data from fisheries bycatch data (Collins et al., 2010; Roberts, 2012) has also contributed to the current understanding of the region’s benthic ecosystems. The logistical difficulty in carrying out in situ work at the islands, notably due to the archipelago’s geographical isolation, inhospitable climate and lack of natural harbours, has however resulted in significant gaps in our understanding of the region, and especially the benthic environment.
At less than 5 million years old (Hawkesworth et al., 1977; Thomson, 2004), the islands are, in geological terms, relatively young. The volcanoes that form the SSI developed in situ through the subduction of the South American tectonic plate under the South Sandwich plate (Leat et al., 2016). The continental shelf area around the islands is limited, with steep-sided volcanic slopes resulting in a very narrow band of shallow coastal water. The region is geographically remote; South Georgia is located 550 km to the north-west and the South Orkney Islands, 800 km to the west-south-west. Small, young, and remote, the SSI do not appear predisposed to high benthic diversity. The SSI marine environment is, however, one of extremes. Volcanic activity creates both disturbed environments resulting from volcanic eruptions and lava flows (e.g., Southern Thule; Griffiths et al., 2008; Rogers et al., 2015) and highly productive chemosynthetic environments such as the high-temperature black smokers at Kemp Caldera (Linse et al., 2019). Bathymetrically the waters range from these shallow coastal environments to deep-sea trench (i.e., Meteor Deep–8,266 m). The deep sea is punctuated by seamounts rising relatively near the surface and providing a major source of topographic complexity to the region. The archipelago exhibits pronounced environmental gradients with strong latitudinal trends in seasonal pack ice cover (and thickness), sea-surface temperatures and productivity. Furthermore, the southern boundary of the powerful Antarctic Circumpolar Current (ACC) which flows close to the islands represents a region of ecological importance centred around the upward flux and entrainment of high concentrations of macronutrients (Tynan, 1998). Conversely therefore, this spatial and temporal heterogeneity in habitat across the archipelago coupled with regions of high productivity could act as catalysts of a diverse and distinct fauna.
Understanding the structure and function of biodiversity at this potentially important, yet poorly understood sub-Antarctic archipelago is crucial to understanding the potential ramifications for future environmental change and to ensure the representative nature of protection afforded by the MPA. Furthermore, understanding the differences in communities across the MPA can highlight important or distinctive biogeographical zones, providing a framework to effectively manage the region’s benthic environment.
In this paper we adopt a macroecological approach to spatially analysing all available benthic biodiversity data for the SSI. This is combined with data recently collected as part of UK Government Blue Belt programme (Darby et al., 2021) to create a baseline measure of marine biodiversity at the SSI and thus provide a framework from which to identify ecologically sensitive areas and species, identify conservation priorities and monitor future biogeographical changes. This paper proposes to address five key questions: (1) How is SSI biodiversity structured spatially and taxonomically? (2) What environmental factors drive this structuring? (3) Can we identify priority areas around the SSI that are anomalously rich, vulnerable, or important to investigate due to paucity of knowledge? (4) How does the archipelago fit into its broader biogeographical setting (i.e., is it a distinct biogeographical realm or is its benthos a subset of that of one of its neighbouring regions)? (5) How can this information contribute to marine spatial planning in the region?
The greater SSI region, which forms the focus of this paper, is defined here by a bounding box of ∼510 km (32°W to 24°W) by ∼790 km (55°S to 62°S), covering an area of 405,270 km2. The region covers the South Sandwich volcanic arc which includes the SSI and associated seamounts extending from Protector Shoal in the north to the waters south of Herdman and Tyrell Banks in the south (Figure 1). To the east, the region includes the South Sandwich Trench and to the west, the East Scotia Ridge back-arc spreading centre. The study area covers a bathymetric range from the sublittoral to >8,000 m. The rationale for this geographical delimitation was based on the spatial extent of bathymetric datasets compiled for the region (i.e., Leat et al., 2013, 2016).
Biodiversity data were collected as part of the 2019 Blue Belt Programme Expedition on board RRS Discovery (DY99; Darby et al., 2021; see also Downie et al., 2021), which assessed the diversity and distributions of epibenthic macrofaunal communities at the SSI and their vulnerability to fishing impact. During DY99, 28 drop-camera transects, and 12 sampling deployments were undertaken at Zavodovski Island, Saunders Island, Montagu Island, Montagu Bank, and Southern Thule. Camera transects ranged in depth from 200 to 2,150 m and sample deployments at depths ranging from 182 to 843 m. Four sampling deployments used a benthic dredge, and eight employed an Agassiz trawl. For the purposes of this publication, DY99 data from both sampling deployments and camera surveys is presented as presence-only data with taxonomic identifications made conservatively at the highest possible taxonomic resolution. DY99 data were assimilated with data collated from the Global Biodiversity Information Facility open-access database1 (accessed 11th April 2019). This data included materials collected during the Discovery expeditions (1925–1938), Islas Orcadas 575 (1975) and USNS Eltanin (1968–1982), along with more recent research in the region led by the British Antarctic Survey (e.g., BIOPEARL expedition, 2006). This paper reports these data in a standardised format classifying all samples, where reconcilable, to species level, depth at which specimens were recorded, and the geo-reference at which the specimens were found. For gear deployments in which a precise position of collection was unavailable (e.g., trawl samples), the midpoint of latitude and longitude were calculated from the start and end position of the deployment. Inconsistencies in species classification were reconciled using the World Register of Marine Species match taxa tool2 thus avoiding synonymies, which were especially prevalent in some of the older collections.
Twenty-one environmental data layers were included in the analysis, to characterise physical geomorphology, surface productivity, physical oceanography, and sea ice extent. All input variables are summarised in Supplementary Table 1.
Bathymetry data was obtained from a 200 m resolution digital elevation model compiled for the region (Leat et al., 2013, 2016) and was used to calculate eight derivative datasets–slope angle, seabed rugosity, topographic position, valley depth, channel network base level, channel network distance, Slope Length and Steepness factor (LS-Factor) and curvature. Slope was calculated using LandSerf (version 2.3) multi-scale analysis. A window of 10 grid cells (2,000 m) was used with an inverse linear distance decay to give greater importance to cells closer to the target cell. The effect of this is to remove finer-scale variation in slope morphology but retain larger topographic features. Topographic position index (TPI) was calculated using Land Facet Corridor Tools extension for ArcGIS. TPI provides a measure of whether a cell is positioned on a peak, in a depression, or in a region of constant gradient (flat or constant slope) relative to the surrounding cells. It can account for local scale topography vs. broader-scale features by changing the size of the window of reference. For this analysis two separate window sizes (10 and 100) were used. Terrain ruggedness index (TRI) was calculated at broad (1,000 m) and fine (200 m) scale using SAGA GIS Terrain Analysis Morphometry tools as a measure of rugosity. TRI is calculated as the square root of the sum of squared difference between the bathymetric value of a cell and its 8 surrounding cells. Profile curvature was calculated using the spatial analyst toolbox in ArcGIS. It is a second derivative index of bathymetry that measures the surface shape of the seabed in the steepest downhill direction, quantifying the rate at which slope gradient changes. Regions with constant gradient return a value at or approaching zero, concave and convex slopes return large negative and positive values, respectively.
The Basic terrain analysis tool in SAGA GIS tools for QGIS (v. 3.2; Conrad et al., 2015) was used to calculate a set of regional terrain variables associated with hydrological properties (Olaya, 2009). They are based on the concept of the Channel Network Base Level, which is an interpolated elevation surface connecting the elevations at the bottom of a network of drainage channels derived from a digital elevation model (DEM) (Boehner and Selige, 2006). Channel Network Distance is calculated as the vertical distance between the DEM elevation and the Channel Network Base Level elevation, and higher values indicate topographic peaks. Valley Depth is calculated as the vertical distance to the lowest elevation of source flow, highlighting topographically enclosed terrain. The LS-factor is a combination of slope length and steepness. In the terrestrial environment it is used to predict erosion potential (Desmet and Govers, 1996) and can similarly be applied in the marine context to indicate potential stability of sediment deposits and hence the likelihood of exposed hard substrata (Kenchington et al., 2020).
Satellite derived primary productivity data (NPP) were accessed through Oregon State University3. Here net primary productivity is defined as a function of chlorophyll, available light, and photosynthetic efficiency. The data are derived from the Vertically Generalized Production Model (VGPM) (Behrenfeld and Falkowski, 1997), MODIS surface chlorophyll concentrations (Chlsat), MODIS 4-micron sea surface temperature data (SST4), and MODIS cloud-corrected incident daily photosynthetically active radiation (PAR). Euphotic depths are calculated from Chlsat. The data were extracted as monthly means over a 5-year period (2010–2014) with a grid cell resolution of 1/12 degree of latitude (∼9,275 m) by longitude (∼5,465 m). R (version 3.1.2) was used to define the geographic region of interest, create a data matrix of each month’s mean NPP, and transpose this into a raster dataset with the correct geographic projection and each grid cell pixel representing a mean and peak NPP for 5-year of monthly data.
Sea ice data were obtained from the National Snow and Ice Data Centre’s (NSIDC) online resource4. Sea ice cover was calculated as the mean number of days per year that an area is covered in sea ice based on the median extent of sea ice for each day between 1981 and 2010.
Oceanographic data on current velocity, seabed and sea surface temperature and salinity were obtained from the Operational Mercator global ocean analysis and forecast system based on the NEMO ocean model. Accessed through the E.U. Copernicus Marine Service Information. Data was extracted at a resolution of 1/12 degree of latitude (∼9,275 m) by longitude (∼5,465 m).
To standardise the spatial extent and resolution of each input variable, each raster was resampled using nearest neighbour analysis to the same spatial resolution. For oceanographic and satellite primary productivity data which had coarser spatial resolutions (see Supplementary Table 1), data were resampled to 200 m using ArcGIS spatial analyst spline (with barrier) interpolation. Spline with barrier interpolation was selected based on its suitability for environmental variables that change over gradients. The spline barrier used was a polygon of the coastline of the SSI to prevent values being interpolated across the physical boundary of the island.
Data management and analysis was undertaken using dplyr (Wickham et al., 2019) and vegan (Oksanen et al., 2019) R packages (R Core Team, 2019) and ArcMap version 10.5.
Species accumulation curves were constructed using the rarefaction method in vegan, calculated over 1,000 permutations. Differentials were calculated to quantify the rate at which novel species discovery was still occurring. To estimate total species richness at the SSI, the vegan package was used to extrapolate the current rate of species accumulation using Chao 1 and Jacknife 2 species richness estimators (Chao, 1987). For comparative analysis between islands, each island was delineated using a 1,500 m depth contour and the South Sandwich Trench was delineated using the 4,000 m depth contour. South Georgia data were obtained from a 2011 assessment of the region’s biodiversity (Hogg et al., 2011) combined with updated GBIF data for both South Georgia and the South Orkney Islands (see text footnote 1; data accessed 13 November 2019). Similarity matrices comparing species composition between each island were computed using the Jaccard similarity index.
Trends in the distribution of species richness throughout the archipelago were investigated over geographical and depth gradients. For geographical comparison, the region was split into 10 km2 grid squares. For depth comparison, the region was split into 250 m depth bins. For a comparative depth analysis residuals from a regression analysis of log-transformed species richness against sampling effort (defined by number of sampling locations) were used to adjust for the uneven sampling effort both over the depth gradient and highlight depth zones where species richness was either higher or lower than expected based on sampling effort.
The statistical approach to Gradient Forest analysis was used to (i) assess the key environmental drivers of faunal composition at the SSI and quantify their degree of influence; (ii) interpret the shape and magnitude of compositional changes in faunal groups along these key environmental gradients; (iii) apply this information to spatially cluster the benthic environment at the SSI; and (iv) characterise these clusters based on environmental and faunal descriptors.
Gradient Forest modelling was undertaken using the R package randomForest (Liaw and Wiener, 2002) and gradientForest (Ellis et al., 2012). The approach was based on mapping protocol discussed and described in detail in Ellis et al. (2012) and Pitcher et al. (2012). Gradient Forests are an extension of Random Forests, a machine learning method that builds a large number of classification or regression trees on random subsets of input data to predict values of a response variable based on a number of independent variables. Gradient Forest produces multiple Random Forests, one for each taxon in a community matrix and uses the multitude of splits derived from the component trees forming the Random Forests to identify where along the gradients of the environmental predictors more taxa than expected by chance show differences in their presence or abundance, hence identifying step changes in assemblage composition. Critical values identified along these gradients corresponding to changes in faunal composition are used to transform the gradients of environmental predictor layers to reflect the rate of taxonomic change. Principal components of the transformed predictors provide a multidimensional representation of variation in combined faunal and environmental composition. K-means clustering of the principal components further allows the apportioning of the variability into discrete classes with similar environmental and faunal attributes. The clusters can be plotted in map form and over the first two PCA axes, to present them in the biologically transformed environmental space. Each individual Random Forest model also assigns an importance statistic (how much the inclusion of the variable improves the model) to each predictor. The Gradient Forest finds the most influential predictor variables over all the Random Forests, weighted by the models’ internal R2 statistic (indicator of model fit), to identify the variables which most influence change in faunal communities along the environmental gradients.
The relative importance of environmental variables to individual functional groups was investigated using the boruta algorithm in the “Boruta” package in R (Kursa and Rudnicki, 2010). Collinearity in environmental variables that were identified as significant for at least one functional group by Boruta was checked using Spearman’s rank-order correlation. For any two variables with a correlation coefficient exceeding 0.7, the one with a higher Boruta importance to more functional groups was retained for analysis. The Variance Inflation Factor (VIF) was calculated for the final selection on predictor variables, to ensure it did not exceed 3 for any variable. The Gradient Forest analysis was done using this subset of the environmental variables and using functional groups, to ensure consistency across the different datasets and sufficient number of observations to train the component Random Forest models. To assign functional trait information to the taxa recorded at the SSI, data were collected on reproductive mode, feeding strategies, type of development (e.g., brooder vs. planktotrophic), mobility, lifestyle, affinity to specific substrata and reef-building capacity of each taxon, as well as whether they were considered habitat forming, whether they were associated with a certain stage of ecological succession and how fragile they are to physical impacts during sessile benthic life stages. A comprehensive description of this methodology is discussed in Hogg et al. (2018). Twenty-one functional groups were assigned based on these ten functional traits, in line with previous research on functional traits at South Georgia (Barnes and Sands, 2017). These groupings are summarised in Table 1.
Marine benthic biodiversity at SSI was rich across taxonomic levels and comparable to the neighbouring biodiversity hotspot of South Georgia in terms of richness in phyla (18), classes (45), and families (447). Species richness was lower (Tables 2, 3), though notably this was based on far fewer records with 2,670 recorded to species level (cf. South Georgia–17,732). In total 4,887 distinct georeferenced records were recorded at 773 sampling stations.
Table 2. Estimation of species richness for the South Sandwich Islands region extrapolated using Chao 1 and Jacknife 2.
Structured by depth, both species richness and sampling effort (Figure 2) were highest in shallower waters, with 58.3% of all records (2,849) collected between 0 and 500 m and 88% of SSI species (777) represented in this depth range. Whist record counts dropped at depths greater than 1,000 m, there was a secondary peak in record counts between 2,000 and 3,500 m which accounted for 22.2% of records. This depth band had an even more pronounced peak in species with 30.8% (272) represented in this bathymetric zone. A distinct shallow-deep discontinuity was observed in species composition, with only 69 species shared between the 0–500 m and 2,000–3,500 m depth ranges. Beyond 3,500 m, record counts, and to a slightly lesser degree species richness, tapered to a maximum sampling depth of ∼8,000 m.
Figure 2. Density plot of (A) record counts and (B) species richness by depth. Species richness is calculated as number of distinct species per 250 m depth bin. The species richness plot includes residuals for each 250 m depth bin derived from the linear regression of log transform species and station counts shown in panel (C). Blue bars in panel (B) denote negative residuals (relative species paucity), and red bars denote positive residuals (relative species richness). The light green shared zone between 700 and 2,250 m denote the depth range open to licenced long-line fishing. In panel (C) the regression line is shown in solid black (r2 = 0.84), the 95% regression confidence interval as grey ribbon and the 95% prediction line as a dashed black line. Log transformed data was tested for normality (Shapiro–Wilk P > 0.05).
Non-structured sampling by depth at the SSI makes it difficult to interpret depth trends in benthic diversity. Firstly, sampling effort, calculated in terms of number of distinct sample gear deployments, varies with depth. Secondly, sampling methodology is not standardised and often not explicitly recorded from historic surveys. Regression analysis on log-transformed species and station counts (Figure 2C) provided a crude means of adjusting for the confounding influence of variance in sampling effort across depth. Analysis of regression residuals calculated in 250 m depth bins between 0 and 8,000 m (Figure 2B) identified positive residuals in shallower water (<750 m) indicating high species richness relative to sampling effort. The licenced fishing zone (700–2,250 m) recorded largely negative residuals (i.e., low adjusted species richness). Over the entire 8,000 m depth range there were no other obvious discontinuities in standardised species richness.
Subdivision of the SSI region into 10 km2 grid squares demonstrates a geographically uneven distribution of sampling effort in terms of number of distinct sampling stations per grid cell (x̄ = 20.6; σ = 39.8). Sampling has focussed primarily around the seven main islands, with sampling much reduced in offshore, deep-water regions. Most sampling has occurred at the northern-most islands of Zavodovski and Candlemas and the southern-most island of Southern Thule. Sampling effort across the rest of the archipelago is notably lower, especially at Montagu Island, Montagu Bank and Bristol Island. Species richness was similarly uneven across the region (x̄ = 9.9; σ = 19.8). Higher species richness corresponded with higher sampling effort in the northerly islands, but notably not at Southern Thule, where despite high sampling effort, species richness was comparatively low. Family level richness was also shown to be uneven across the region (x̄ = 10.1; σ = 15.6).
Taxonomically, arthropods were the dominant taxa constituting 27.5% of species and 31.9% of records (Table 3). 75.9% of species and 78.3% of records were recorded from the 4 most species rich phyla (arthropods, echinoderms, annelids, and molluscs). At species level no single species was dominant, with the top 25 species accounting for 17.3% of records and the top 100 species for 42.6%. Most species were rare, recorded only once or twice (46.4 and 23.3%, respectively). Only 49 species (<1%) were recorded more than ten times. The ten most common species represented ∼10% of total records.
General taxonomic trends were shared across the archipelago, with arthropods and echinoderms representing the dominant taxa. Notable exceptions to this were a dominance of molluscs and echinoderms on Bristol Island; a notably larger proportion of corals on Saunders and both corals and sponges (key VME taxa) on Montagu Bank. The South Sandwich Trench was a clear outlier to the group with arthropods being significantly more dominant constituent in catches. At Southern Thule, the proportion of annelid worms was significantly higher than elsewhere.
Rarefaction curves of all benthic species data (Figure 3) showed the rate of novel species discovery remains high across the region, with 0.75 new species recorded per new sample site. Novel species discovery was highest around the islands themselves (<1,500 m depth) with species differentials ranging from 2.57 new species per new site at Saunders Island to 1.68 at Bristol Island (Table 2). Assessment of individual islands in the archipelago demonstrated significant variance in sampling effort and species richness along the archipelago (Figure 3 and Table 2). At the most northernly islands of Zavodovski and Candlemas the curves did not reach an asymptote with novel species discovery remaining high. In contrast, the southerly island of Southern Thule recorded lower total species richness and a lower rate of novel species discovery, despite similar sampling effort. At Saunders Island sampling has been lower, corresponding to fewer species records, but species discovery was amongst the highest in the region. The islands and banks that form the centre of the island chain (Bristol Island, Montagu Island, and Montagu Bank) have few reported species but have also had very low sampling effort (at least sampling that has resolved specimens to species-level). The rarefaction curve for the South Sandwich Trench is shown to be levelling-off and reaching an asymptote (Figure 3). With lower recorded species richness and lower species differential (0.62), it is likely the Trench region is not as speciose as the shallower waters of the neighbouring islands.
Figure 3. Rarefaction curve showing (A) the rate of species accumulation with increasing sampling effort for each of the South Sandwich Islands, and (B) the rate of species accumulation with increasing sampling effort across nine major phyla (chordates excludes fish). Islands are delineated by 1,500 m depth contours. Zavodovski Island includes Protector shoal; Candlemas includes Visokoi Island and Southern Thule includes the Adventure and Kemp Caldera. Sample effort is defined by number of sampling sites. Green ribbons (for islands) and blue ribbons (for phyla) denote standard deviation over 1,000 permutations. The “All Islands” plot (red ribbon) shows species accumulation across the entire study region.
Species accumulation curves for key taxa across the region (Figure 3) demonstrated high rates of species discovery with no phylum reaching an asymptote. Novel species discovery was highest in byozoans (0.9) and as such represents the phyla for which current biodiversity estimates are poorest. There was little variance in novel species accumulation between all other key taxa (x̄ = 0.47; σ = 0.07). Two species richness extrapolations using Chao 1 and Jacknife 2 species richness estimators indicate that across the eight major phyla summarised in Table 3, 67.1 and 68.7% of probable species present at the SSI are currently represented in our sampling. Extrapolations based on these eight phyla (representative of 97.1% of total species) place total SSI species richness at between 1,317 (Chao 1) and 1,286 (Jacknife 2). Relative variance in biodiversity between the major phyla remained relatively constant between observed and predicted richness estimates with rank order species richness remaining broadly unchanged.
A large proportion of species (∼58.2%) at SSI were not shared across the other Scotia Arc islands (Table 4). SSI exhibits a stronger biogeographical link with South Georgia (sharing ∼16.7% of SSI Species) than the South Orkney Islands (8.9% of SSI species). 16.2% are considered cosmopolitan across the whole Scotia region.
Table 4. Faunal similarity between South Georgia (SG), South Orkney Islands (SOI) and the South Sandwich Islands (SSI).
The proportion of species recorded only from the SSI varied by phylum (x̄ = 56.2%; σ = 10.6%). Considering just the major phyla (here defined as those with >30 species), annelids, molluscs and ascidians had the highest proportion of species not recorded across the rest of the region (71.6, 62.5, and 60.6%, respectively). Bryozoan species, conversely, were least likely to be restricted just to the SSI (36.2%).
Subdivision of each of the SSI into distinct biogeographic zones (delineated by 1,500 m depth contours) demonstrated low species-level similarity between individual islands and between the continental shelf regions of South Georgia and the South Orkney Islands (Figure 4). Highest similarity was recorded between neighbouring Zavodovski and Candlemas/Visokoi Islands. This was followed by Saunders and Candlemas Islands and to a lesser degree Zavodovski Island and Saunders Island. There was low similarity between Saunders Island and any islands to the south. The South Sandwich Trench demonstrated high dissimilarity to all regions. Outside the SSI, the highest similarity (albeit still low) was seen between South Georgia and South Orkney Islands.
Figure 4. Jaccard dissimilarity matrix of the benthic faunal composition of (A) families and (B) species across the Scotia Arc. Islands are ordered from south (South Orkney Islands) to north (Shag Rocks). Islands are delineated by 1,500 m depth contours. Zavodovski Island includes Protector shoal; Candlemas includes Visokoi Island and Southern Thule includes the Adventure and Kemp Caldera. Montagu Bank and Bristol Island were excluded from the analysis due to limited samples. The South Sandwich trench was delineated by the 4,000 m depth contour. South Georgia data were obtained from a biogeographic analysis by Hogg et al. (2011). South Orkney Islands data were sourced from GBIF (GBIF.org; accessed 11th April 2019).
At family level there was very little similarity between South Georgia, Shag Rocks and the South Orkney Islands. At the SSI similarity appears a function of geographical proximity, with highest similarity between neighbouring islands (e.g., Southern Thule and Montagu or Zavodovski and Candlemas/Visokoi Islands). And similarity reducing between more distant islands (e.g., Zavodovski and Southern Thule).
The continental shelf around the SSI is limited, with steep-sided volcanic slopes dropping away to depths in excess of 3,000 m within 10 km of the coastline (Figure 5). As such the area of seabed under 1,000 m depth is restricted to 13,270 km2. Islands in the chain exhibit differing degrees of bathymetric connectivity with their neighbours. All are separated by regions of deeper water, typically between ∼1,000 and 1,500 m. Saunders Island is the most bathymetrically isolated of the archipelago, separated by Candlemas Island to the North and Montagu Island to the South by water depths of ∼2,500 m (See Supplementary Figure 1). Slope, rugosity and topographic position (i.e., whether a site is on a topographic peak or in a trough) were all associated with the volcanic slopes of the islands and the tectonic spreading zone in deep water to the west of the island chain. Slope angles generally peaked at ∼25° on steeper topographic features with gradients of 10° more generally associated with the flanks of the volcanoes.
Figure 5. Summary of regional environmental datasets: (A) depth, (B) slope, (C) sea ice, (D) mean primary productivity, (E) peak primary productivity, (F) current speed, (G) sea surface temperature, (H) seabed temperature, and (I) seabed salinity. Panels (D–I) show mean summer conditions. A full description of all the data including data sources are summarised in Supplementary Table 1.
Positioned broadly on a north–south axis, the SSI exhibit a strong latitudinal sea surface temperature gradient. During the summer months the region experiences a range of −1.4 to 2.2°C, dropping to between −1.85 and 0°C in Winter. The temperature range around the islands themselves is more constrained, ranging from 1°C at Protector Shoal to −0.8°C at Southern Thule in summer. In winter sea-surface temperatures are more homogenous with a range of −1.4 and −1.7°C across the island arc. Seabed temperature demonstrates collinearity with depth and little annual variance. The coldest region is the South Sandwich Trench with an annually stable temperature of ∼ 0.6°C which changes little with latitude. Seabed temperature around the islands ranges from 1°C (0.9°C winter) at Zavodovski to 0.6°C (0.45°C winter) at Southern Thule. Seabed salinity is also linked to depth with lower salinity in proximity to the islands (Figure 5).
Sea ice conditions varied considerably throughout the year and across the latitudinal gradient of the islands. In the north, Protector Shoal and Zavodovski Island experienced little or no sea ice, even during the height of winter. In contrast the southern-most island of Southern Thule had on average ∼ 200 sea-ice coverage days per year. Mean sea-surface productivity demonstrated a distinct east-west discontinuity. Waters at and to the east of the island chain demonstrate higher productivity with a marked drop-off west of the islands. Highest mean productivity was recorded in the water to the east of Zavodovski, Visokoi, and Candlemas Islands and on Montagu Bank. A second discontinuity was seen at Bristol Island, with all islands and Banks to the south demonstrating very low mean primary productivity. Peak primary productivity identified productivity hotspots at Zavodovski, Visokoi and the seamount chain extending to Leskov Island. A localised area of peak productivity occurred at Montagu Bank. All other islands showed no peaks in productivity. Regions of higher current velocities (peak average ∼ 0.2 m/s) appear linked to the topography of the lower flanks of the South Sandwich Trench (6,000 m depth). Areas of higher current velocity were also seen along the eastern flank of Zavodovski and Saunders Islands and in deeper water (∼2,500 m) bisecting between Saunders and Montagu Island. All other islands in the arc including Protector Shoal, Southern Thule, Bristol Island, and Montagu Island demonstrated low current velocities.
Spearman’s correlation was used to remove highly colinear environmental variables reducing predictor variables from 19 to 15. Those variables excluded were mean productivity (which correlated strongly with peak productivity), annual temperature range (which correlated with both bathymetry and bottom temperature), rugosity with a 5-cell neighbourhood (which correlated with slope angle and the standard measure of rugosity) and relative slope position (which correlated with channel network distance). The influence of each of the 15 environmental predictor variables on faunal communities along the environmental gradients was assessed using R2 statistic as an indicator of model fit.
The most important predictors for functional group distribution at SSI were depth, seabed temperature, channel network base level and latitude (see Supplementary Figure 2). Salinity and areas of peak primary productivity were of intermediate importance, as were measures of seabed topography such as slope angle, rugosity, and broad scale topographic position (i.e., location of topographic peaks and troughs). Fine-scale topographic position and current velocity were of least importance.
The frequency distributions of the locations of individual split nodes of all component decision trees over the range of values of predictor variables (Figure 6) show that changes in the composition of functional groups (summarised in Table 1) along environmental gradients were non-uniform. Along the depth gradient, a large number of splits occurred in the range 0–1,000 m, indicating that shallower waters were very heterogenous, with large changes in functional group composition. In contrast, across the rest of the bathymetric range (1,000–8,000 m) samples splits had low importance, indicating relatively little compositional faunal change. Sampling at the SSI has been higher in shallower waters (see Figure 6 red line). To account for this bias, the ratio of densities (blue line) plots the expected density of splits had depth been sampled with uniform density. The ratio of densities plots supports the assertion that large changes in functional group composition are seen between 0 and 1,000 m depth.
Figure 6. Kernel density plot of random forest tree splits across environmental gradients for the four most important predictors–depth, bottom temperature, channel network base level (CNBL), and latitude. A high density of splits indicates relatively large changes in functional group composition. Split density is denoted by the histogram and black line. Density of sampling is shown in red. The ratio of splits standardised by the sampling density is shown in blue, with ratios >1 (horizontal dashed line) indicating locations of relatively greater change in composition.
Along a gradient of sea bottom temperature, high relative rates of faunal assemblage change were seen in a bimodal distribution with peaks at ∼0.9 and 0.6°C. A series of distinct peaks in faunal assemblage change were shown along a latitudinal gradient, most notably at ∼ 56.2°S, where highest compositional change corresponded with Zavodovski Island. Smaller peaks were seen at Saunders Island (57.7°S), Montagu Island (58.4°S) and Southern Thule (59.5°S). In contrast, Candlemas Island (57°S) and Bristol Island (59.1°S) demonstrated little change in functional structure. A further two peaks were shown south of 60°S, these could correspond with sampling on and between Tyrell and Herdman Banks.
The Gradient Forest maps show these distinct environmental and faunal discontinuities (Figure 7) in geographical space. The most obvious divide was between shallow (Figure 7; Clusters 8 and 10) and deep environments (all other clusters). The two shallow clusters were then split along a latitudinal gradient with Cluster 8 covering the northern regions of Protector Shoal, Zavodovski Island, Candlemas and Visokoi Island and Cluster 10 covering the southern regions of Herdman and Tyrell Banks, Southern Thule, Bristol Island, Montagu Island and Bank and Saunders Island. The boundary between these two gradient forest clusters occurred at Candlemas Island. Cluster 8 was characterised by low latitude, low sea ice cover and high maximum productivity (see Supplementary Figure 3). Conversely Cluster 10 was characterised by high latitude and high sea ice cover. Functional group composition between clusters also differed (Supplementary Figure 4). The lower-latitude Cluster 8 representing the northernly islands of the archipelago demonstrated a higher dominance of soft-bodied sessile active and passive filter feeders such as Alcyonacea (soft corals), sea pens, anemones, and hydroids. The higher-latitude Cluster 10 was notable for its high ratio of presences across all functional groupings including the presence of climax communities.
Figure 7. Gradient forest mapping clusters combining transformed environmental variables with benthic functional group data. Clusters represent the expected continuous patterns of composition for seabed biodiversity at the South Sandwich Islands with similarity in composition denoted by similarity in colour. The biplot shows the first two principal dimensions of the biologically transformed environment space with vectors indicating the direction and magnitude of major environmental drivers and colours corresponding to mapping clusters. Mapping clusters are numbered and correspond with clustering classes shown in Supplementary Figures 3, 4.
This marked latitudinal discontinuity in fauna and environment was not restricted to shallow waters. The deep-sea environment, which covers much of the study region, was not a homogenous region but was sub-divided by the Gradient Forest analysis into ten environmentally and faunistically divergent clusters transitioning over both topographic and latitudinal gradients. In contrast to the shallow water clusters, the changes in the deep-water clusters are more graduated. This is demonstrated in Figure 7 by the similarity in colours between neighbouring clusters denoting relatedness. The deep-sea clusters transitioned over gradients of sea ice cover, latitude, and productivity. Faunistically, the northern-most deep-sea cluster (Cluster 5) was characterised by infaunal and epifaunal deposit feeders and mobile, jointed legged predators or scavengers such as sea spiders and decapod crustaceans. Further south Cluster 3 demonstrated representation across all functional groupings, though universally these were with low occurrences. In the deep sea surrounding Saunders Island, Cluster 3 transitioned to Cluster 4, which was characterised by grazing species, infaunal deposit feeders and mobile predators. The most southerly cluster (12) was similar in faunal composition to Cluster 4 with the addition of hard sessile species such as cup corals and whip corals. Other notable clusters included the South Sandwich Trench system (Clusters 1 and 2). Cluster 1 was dominated by mobile predators and infaunal species. In contrast the upper flanks of the trench (Cluster 2) had a higher proportion of climax communities (e.g., sponges) and sessile soft bodied active and passive filter feeders such as soft corals.
Here we present the SSI as a biogeographically distinct archipelago, linked by limited shared benthic species to South Georgia, and to an even lesser extent, the South Orkney Islands. Relatively few species were recorded as cosmopolitan across the Scotia Arc and there was near universal dissimilarity in the composition of benthic species and families between the three main island groups.
This analysis identified that the proportion of species recorded from the SSI, but not across the rest of the Scotia region, varies considerably across the eight major phyla. Previous research has suggested that amongst mobile fauna, biodiversity in known to be at least partially shared across the Scotia region (Roberts and Agnew, 2008). The highest levels of South Sandwich exclusivity were seen in annelid worms and molluscs, whilst bryozoans were shown to be the phyla most likely to be cosmopolitan in distribution. This biogeographical characterisation support previous descriptions of South Georgia’s fauna which reported 25–30% similarity in mollusc species between South Georgia, South Orkney Islands and the SSI (Linse et al., 2006) and high similarity in bryozoans (∼40%) between South Georgia and the SSI (Barnes and Griffiths, 2008). Where connectivity between regions is restricted (e.g., by deep water and strong currents), this is often reflected in the biogeography of the region’s benthic fauna. Some taxa, such as bryozoans for example, exhibit predominantly benthic larval stages. This limits their ability to proliferate into neighbouring regions and as such restricts their geographical distribution (Griffiths et al., 2009). There is also significant evidence to suggest however that even taxa with non-pelagic larvae such as bryozoans are known to disperse widely through biofouling of transport vectors such as macroalgae, plastics and volcanic pumice (e.g., Watts et al., 1998; Barnes, 2002; Smith, 2002; Bryan et al., 2012). Such dispersal has been shown to occur across major oceanographic boundaries such as the ACC (e.g., Fraser et al., 2016; Figuerola et al., 2017). The observation that bryozoans in the Scotia Arc seem to be amongst the most cosmopolitan of phyla implies that it may not be limited connectivity that restricts or fundamentally determines distribution, rather the suitability of habitats in geographically neighbouring, but environmentally dissimilar regions.
Comparing sea temperature as an example, South Georgia exhibits an annual range of ~5°C, with sea-surface temperatures ranging between 0 and −1°C in winter and between 3 and 4°C in summer. Seabed temperatures of South Georgia’s Circumpolar Deep Water (>150 m) range from 1 to 3°C. South Georgia is therefore amongst the warmest (Barnes et al., 2006), and most variable (Holeton et al., 2005) water masses south of the Polar Front. This wide range of environmental conditions, coupled with its geographical position as the most northerly continental shelf area within the Polar Front, result in an island characterised by strong biogeographical links between both Antarctica and South America (Hogg et al., 2011). In contrast, the South Orkney Islands and the SSI are both environmentally Antarctic in character with colder, more seasonally stable water masses and the presence of seasonal sea ice (Barnes et al., 2009; Rogers et al., 2015). The surface waters around the SSI themselves range from −1.7°C at the south of the archipelago in winter to ∼1°C at the north of the archipelago in summer, with a maximum local-annual range of ∼2.4°C. Seabed temperatures are even more constrained, ranging from a maximum of ∼1°C in the north during summer months to 0.45°C in the south during winter. At the South Orkney Islands water temperatures vary between −1.86 and 1.6°C at shallow depths (Clarke and Leakey, 1996).
If it is environment rather than connectivity that principally drives faunal discontinuity between the islands of the Scotia Arc, then based solely on temperature gradients, one might expect that in terms of benthic fauna, the higher latitude archipelagos of South Orkney and SSI to share more in common at the exclusion of the relatively warm waters of South Georgia. In contrast however, our analysis reports higher South Orkney Island–South Georgia species similarity (24.7% of South Orkney Island species shared) than South Orkney Island–SSI similarity (9.2% of South Orkney Island species shared).
The explanation for this is likely multifaceted, encompassing environmental suitability for species, oceanographic connectivity and geological factors such as island age, size and provenance. The SSI represents a steep-flanked, geologically young volcanic archipelago making it geomorphically distinct from any other landmass in the Southern Ocean (O’Brien et al., 2009). In contrast, the South Orkney Islands and South Georgia represent geologically older regions that once formed part of the same landmass (Livermore et al., 2007), and now represent topographically similar island groups with large continental shelf areas (Dickens et al., 2014; Hogg et al., 2016). In this context, South Georgia and the South Orkney Islands are more alike than either is to the SSI. Furthermore, the SSI are more oceanographically disconnected from the Scotia group with the clockwise flow of the ACC more directly connecting the South Orkney Islands to “down-stream” South Georgia, but with the SSI somewhat offset away from the currents northward flow (see Figure 1). Indeed, considering regional currents more broadly, it is possible that the SSI exhibits stronger links with the Weddell Sea province, linked through the outer edge of the Weddell Gyre. There is evidence for such as link, for example, in some fish species (Gon and Heemstra, 1990).
General taxonomic observations from prior research suggest invertebrate faunal composition at the northern end of the SSI archipelago closely resembled that seen in similar depths at South Georgia (Lockhart and Jones, 2008). Fauna on comparable soft sediments have also been reported as similar between the South Orkney Islands, South Georgia and the SSI (Lockhart and Jones, 2008; Brasier et al., 2018; Downie et al., 2021). In assessing similarity in species and family level composition between each of the SSI and the wider region, higher similarity was observed between neighbouring islands in the archipelago. This, however, this did not extend to the wider region. For example, northernmost Zavodovski Island was not notably more similar to its closest neighbour South Georgia than any of the other SSI. Similarly, the southern-most island, Southern Thule, was not more or less similar to the South Orkney Islands than any other island. Southern Thule was notably the most faunally similar island (at family level) to the South Sandwich Trench. This could be on account of the highly sediment-laden environment reported from Southern Thule (Downie et al., 2021) and lower seabed temperatures being environmental most similar to the trench environment.
The established paradigm of the Southern Ocean is one of an isolated system (Longhurst, 1998), with the ACC representing both a biogeographical discontinuity, greatly limiting the exchange of epipelagic and benthic fauna (Clarke et al., 2005), and acting as a homogenising influence on Antarctic marine fauna (Chown et al., 2015). With very few barriers to dispersal across a single Antarctic province (Griffiths et al., 2009), the SSI have typically been considered part of a “connected” High Antarctic or Extended Scotia Arc biogeographical region (Knox, 1960; Hedgpeth, 1969). South Georgia, with its high documented proportion of endemic species (Hogg et al., 2011), is often considered an exception to this, and classed as a distinct biogeographical province. Regional studies of littoral ascidians (Ramos-Esplá et al., 2005) supports a bioregional delineation between South Georgia, the South Orkney Islands and the Antarctic Peninsula. Where the SSI aligned with these regions was however unclear and appeared to be a transition zone between the sub-Antarctic islands and the Antarctic. Given the combination of distinct benthic fauna and distinct environmental conditions at the SSI, this bio-physical analysis supports the case for the archipelago to also be considered a distinct biogeographic province. This assessment is supported by previous attempts at ecoregionalisation in the Southern Ocean using environmental-based clustering, which have delineated South Georgia and SSI as separate biogeographical provinces. These ecoregions were shown to align strongly with the environmental structuring of the ACC (Longhurst, 1998; Grant et al., 2006). Furthermore, Spalding et al. (2007) using spatial analysis undertaken predominantly on molluscs (Linse et al., 2006), identified three distinct biogeographical sub-provinces in the Scotia Arc.
The SSI are a biologically rich archipelago. Here we report comparable biodiversity to the neighbouring biodiversity hotspot of South Georgia across taxonomic levels from phylum to family (Hogg et al., 2011). At species level, richness is lower than at South Georgia with 883 species currently recorded from the South Sandwich Archipelago (cf. 1,445 species from the South Georgia shelf region). SSI species richness is nonetheless notably high based on sampling effort, sampling focus and sampling area.
Sampling effort, in terms of the number of sampling sites and records has been lower at the SSI than neighbouring regions. This is largely on account of the absence of local research bases at the SSI, compared to King Edward Point and Bird Island at South Georgia, and Orcadas Base and Signy station at the South Orkney Islands, which act as regional logistical hubs for research vessel to operate from.
There has been far less focus on certain species-rich, yet typically underreported taxa such as nematodes which were the second richest phyla reported from South Georgia (Hogg et al., 2011), but for which only 21 observations (8 species) have been recorded at the SSI. Furthermore, in contrast to both South Georgia and the South Orkney Islands, there has been no known sampling undertaken in intertidal communities (see Barnes et al., 2009; Hogg et al., 2011). These taxonomic and spatial gaps in the SSI dataset considered, our analysis likely represents a significant under-reporting of regional diversity across the SSI Archipelago. For the regions and taxa that have been sampled, we projected regional species count of between 1,286 and 1,317 based on the current rate of novel species discovery (cf. between 1,627 and 1,760 species at South Georgia).
Finally, unlike its neighbouring islands, the South Sandwich archipelago does not have a broad continental shelf area. Instead, the benthic environment consists of the flanks of steep-sided volcanic islands and submarine seamounts with a limited, relatively shallow-water zone restricted to narrow bands around the islands and the tops of the seamounts. Based on a richness-area relationship, in which as shelf area increases, so to do the number of species (Rosenzweig, 1995), species richness is high at the SSI considering only 13,270 km2 of its seabed is shallower than 1,000 m. To place this in a regional context, this is roughly 25% the shelf area of South Georgia (Hogg et al., 2011) or the South Orkney Islands (Dickens et al., 2014).
Biogeographic distribution patterns are difficult to constrain at the SSI because–(i) historic sampling effort has been both non-uniform and patchy (see Figures 2, 3); and (ii) most SSI species were rare–67.7% of species recorded only once or twice. Whilst this is not necessarily problematic when considering broad-scale ecosystem level comparisons, such as between the SSI and other polar archipelagos, it does limit our understanding of how biodiversity is structured spatially across the South Sandwich region.
Biodiversity was shown to vary across the region. Sampling decreased as a function of distance from the archipelago’s islands. As such, paucity in sampling was too high away from islands to formulate an informative overview of distribution patterns. Around the islands, species rarefaction curves (Figure 3) and species richness estimators (Table 2) projected highest species-level diversity at Zavodovski and Candlemas Islands. Conversely, lower biodiversity was recorded predominantly toward the southern end of the island chain at Bristol Island, Montagu Island, and Southern Thule. Sampling at Bristol Island and Montagu Island has however been low (Figure 3), so there is a higher degree of uncertainty with this assessment. Southern Thule, in contrast, has received relatively higher rates of sampling and reports relatively low rates of species accumulation. This assessment of the southernmost island of the archipelago is supported by previous observations which report the island to be species poor (Griffiths et al., 2008).
Previous research has highlighted that abundances recorded in samples were heavily depth dependent, with a marked decline from 200 to 500 m in demersal trawl samples (Griffiths et al., 2008) and from 300 to 1,500 m in epibenthic sledge samples (Kaiser et al., 2008). Rogers et al. (2015) also reported seamount summits as covered in large abundances of brittlestars, with scattered sea pens, large anemones and the seastar Labidiaster sp. In deeper waters around the seamount Rogers et al. reported an abundance of octocorals and brachiopods. Our analysis also demonstrated a depth gradient in species diversity, though as with spatial assessment it was difficult to account for the confounding influence of non-structured sampling across depth. Highest levels of sampling have occurred in waters shallower than 500 m (58% of sampling). Within these waters, 88% of species recorded at SSI are represented. A secondary peak in species richness and sampling records between 2,000 and 3,500 m revealed a change in species composition over depth, with few shared species between samples collected in waters shallower than 500 m samples and samples collected deeper than 2,000 m. Peaks in species richness at shallow (<500 m) and intermediate depths (2,000–3,500 m) were also apparent when sampling effort was adjusted for Figure 2. The zone between 1,000 and 2,000 m demonstrated the lowest adjusted species richness.
The SSI are unique in a Southern Ocean context in that they represent a long chain of islands extending almost 400 km on a north–south axis. As such, they traverse a broad gradient of environmental conditions. Whilst Lockhart and Jones (2008) and Roberts (2012) reported differences in the dominant phyla between the islands, this analysis is the first to attempt to assess changes in faunal composition across the archipelago. As mentioned, most species recorded in the region are rare and subsequently species (and family) composition is highly dissimilar between islands (Figure 4). Similarity was, however, shown to correspond to geographical proximity with highest species and family level similarity recorded between neighbouring islands (e.g., Zavodovski and Visokoi and Candlemas Islands) and similarity reducing as a function of distance. A potential discontinuity in species-level faunal composition was identified at Saunders Island which demonstrated little similarity with any of the islands to the south (Figure 4). In contrast Zavodovski, Visokoi-Candlemas and Saunders Islands demonstrate relatively higher species similarities. This divide between north and south, centred on Saunders Island has also been reported in Toothfish species and bycatch invertebrate species (Roberts, 2012). Roberts demonstrated an abrupt transition between an “Antarctic” and “sub-Antarctic” bioregions around Saunders Island, characterising these two bioregions by differences in sea temperature with depth, hydrographic characteristics, and biological productivity.
Environmental conditions at the SSI are predominantly linked to latitudinal gradients (e.g., sea ice, temperature, and productivity) and depth gradients (e.g., temperature and salinity). Sea-surface productivity also demonstrated an east-west divide. Saunders Island was identified as the most bathymetrically isolated island of the archipelago (see Supplementary Figure 2), separated by deep water (2,500 m) with oceanographic currents bisecting the water between Saunders Island and Montagu Island to the south (Figure 5).
The topography of the archipelago’s islands shows evidence of mass- wasting and slope instability (Leat et al., 2010, Leat et al., 2016). Collapse scars, slump structures, embayments and erosional gully systems can be seen on all the volcanic island flanks. Wave-like structures, particularly on the eastern, trench-facing side of the islands, are thought to be formed by down-slope, mass-flow transport of volcanic sediments (Leat et al., 2010). The instability of the archipelago’s slopes with significant down-slope sediment flows creates what is likely a heavily perturbed benthic environment. This will influence fauna that colonises these environments potentially selecting against slow growing, sessile communities in favour of faster-growing, pioneer communities (e.g., encrusting bryozoans and ascidians) or mobile scavengers and predators. It also seems logical to expect a discontinuity in faunal composition between the more stable environment of the shallow shelves around the islands and the slope. To the west of the island chain is a large plain at a relatively uniform 3,000 m depth. Here large amounts of volcaniclastic sediment, transported down-slope, have formed a thick (up to 1 km) layer of turbidite overlaying the bedrock (Leat et al., 2016). Though there has been limited benthic sampling (204 records) of this environment, what has been recorded is limited to infaunal and mobile epifaunal deposit feeders or scavengers such as polychaete worms and holothurians. This region transitions into the tectonic spreading zone and East Scotia Ridge, an area characterised by tectonically uplifted regions of topographic complexity. These hard-rock islands in the deep sea may represent “oases” for sessile suspension feeding communities, though connectivity across a vast plain of turbidite mud may limit this diversity. Running 100 km to the east and parallel to the Islands, the South Sandwich Trench reaches depths of over 8,000 m, with water depths commonly >7,000 m.
Given most species were recorded rarely at the SSI, it is difficult to assess how environmental conditions drive species-level composition. In this analysis we categorised the regions benthic fauna into 21 functional groups based predominantly on mobility, feeding strategies and lifestyle and then subdivided again based on taxonomy (see Table 1). These functional grouping provided a means of categorising benthic fauna in an ecologically meaningful way, whilst retaining enough sampling records per grouping to allow statistical significance when assessing their correlation with environmental covariates.
Depth, bottom temperature, channel network base level (CNBL) and latitude were identified as the most important drivers of benthic functional group composition. Spatial variation in marine biodiversity over gradients of latitude, depth, and temperature are well documented (Gaston and Spicer, 2013), with depth and temperature commonly cited as environmental drivers in habitat mapping models (reviewed in Harris and Baker, 2012). The CNBL represents a derivative bathymetry layer with local-scale elevations removed. Its importance therefore, as a driver of benthic functional composition, highlights the significance of larger landscape units such as large basins, continental shelf areas and deep troughs in classifying functional-relevant spatial units. Seemingly of less importance in our study region were measures of geomorphology (e.g., slope angle, rugosity, and topography) and variance in oceanographic current velocities. Measure of slope and topography are often useful predictors of faunal composition, notably with measures of geomorphology often employed as surrogates of hard substrate communities (Harris and Baker, 2012). One explanation for their lack of importance here could be that the resolution at which they were gridded (200 m) did not correspond with faunal compositional change. This mismatch in spatial scale may be even more pronounced in modelled oceanographic data for the region, representing only very broad-scale oceanographic currents (∼9,275 m by ∼5,465 m).
Some features of the marine environment are characterised by sharp discontinuities in environmental conditions (e.g., coral reefs, canyons, and continental shelf breaks). Most marine habitats, however, depending on the spatial scale at which they are observed, are not clearly delineated by abrupt boundaries. Instead, the environmental conditions that define habitats, change over gradients, transitioning with a degree of biological turnover between regions. As such, there is a fundamental inconsistency between this environmental and faunal continuum and categorical habitat classification systems, which make analysis and mapping of marine habitats problematic (e.g., see Hogg et al., 2018).
Gradient forest provides a non-linear and flexible methodology in constraining, quantifying, and interpreting compositional changes in fauna along environmental gradients. It is particularly applicable to datasets such as at the SSI, because its use of dimensionless R2 to quantify change allows analysis of amalgamated datasets, even if such data include disparate sampling methodologies (Pitcher et al., 2012).
Of the four most important drivers of faunal composition, latitude and depth proved the most spatially informative (Figures 6, 7). The largest changes in functional group composition occurred along a latitudinal gradient and coincided with specific islands in the archipelago. These changes occurred independently of sampling effort (Figure 6). The most notable threshold changes in faunal composition happened at Zavodovski Island, and to a lesser extent Saunders Island, Montagu Island and Southern Thule. The maps based on Gradient forest cluster groups, representing environmentally and biologically consistent regions, demonstrated clear latitudinal delineations in environment and fauna. Most notable was a north-south divide in shallow-water environments. This bio-physical classification of the SSI supports previous interpretations of distinct northern and southern bioregions at the archipelago (Roberts, 2012). The analysis by Roberts identified a clear discontinuity in toothfish species with Patagonian toothfish (Dissostichus eleginoides) reported north of Saunders Island and Antarctic toothfish (Dissostichus mawsoni) reported to the south. This distinct biogeographical cut-off in finfish was suggested to relate to temperature as the key range-limiting factor (Roberts, 2012). In this case Saunders Island represented a discontinuity because it exhibited the most northerly location at which mean winter surface temperatures dropped below −1°C. This is of physiological significance in toothfish because it represents the freezing point of blood plasma and so tolerance requires physiological adaptations (i.e., antifreeze proteins) which are only present in certain specialist Antarctic species (Roberts, 2012).
In slight contrast to Roberts (2012), here we show a transition in bioregion at Candlemas Island, with Saunders Island representing the most northernly island solely within the southern bioregion. In our assessment, the latitudinal discontinuity is less clear as there are no obvious abrupt clines in environmental gradients at either Candlemas or Saunders Island (Figures 5, 6). Instead it seems likely to result from the composite influence of a variety of physical and biological factors. There is, for example, a clear general differentiation in environmental characterisation between the islands of the north of the archipelago and the south. North of Saunders island there is a much steeper drop-off in terms of number of sea ice days (reducing to almost zero at Zavodovski Island) than there in from Saunders southward (Figure 5). This is aligned with a change in seabed temperature, with the northerly Cluster 8 characterised by temperatures ∼1°C, and the southerly islands forming Cluster 10 characterised by temperatures ∼0.5°C (see the first two temperature peaks in Figure 6). Furthermore, maximum productivity and surface temperature, also demonstrated higher values at Candlemas, Visokoi and Zavodovski Island and notably lower values especially at Southern Thule and Bristol Island.
Higher surface temperature, productivity and shorter sea ice duration toward the north of the archipelago have been proposed as possible drivers of a north-south divide in fauna (Perissinotto et al., 1992) and are all likely to result in greater fluxes in food availability at the seabed. A relatively nutrient-rich seabed environment at the north of the archipelago could, in part, account for the high species richness and high novel species discovery recorded at Zavodovski and Candlemas, and the higher abundances in benthic fauna observed by Downie et al. (2021) at Zavodovski Island. Biodiversity patterns are likely to also be shaped by environmental variables not accounted for in this study, for example seafloor substrate (see Roberts, 2012). Substrate type is a key driver of both diversity and functional composition of benthic environments. The higher frequency of consolidated hard-rock environments reported from Zavodovski Island (Downie et al., 2021) compared to the considerably more sediment-laden and disturbed environment at Southern Thule (Griffiths et al., 2009; Rogers et al., 2015; Downie et al., 2021) corresponded with clear differences in faunal diversity and faunal classes (Downie et al., 2021).
Geographically, the deep sea represents the largest environment mapped in this analysis. With depth, most compositional changes in fauna were shown to occur in relatively shallow waters (depth <500 m). This corresponded with the restricted shelf areas of the islands. Beyond this depth, turnover in functional composition was not marked by definitive thresholds (Figure 6). Analysis of channel network base level, which picks out broader-scale bathymetric features, (CNBL–Figure 6) did however identify secondary peaks in composition change at ∼8,000 and ∼6,000 m, representing the bottom of the South Sandwich Trench (Cluster 1) and flank of the trench (Cluster 2), respectively. Given the Antarctic deep-sea environment is known to be highly distinct, reporting both rare and potentially endemic species (Brandt et al., 2007), distinct biogeographical clustering, driven in part by a distinct faunal composition, seems logical. Conversely however, the non-trench, abyssal environment, notably between ∼3,000 and 5,000 m, did not exhibit the same level of differentiation. This may be accounted for by greater uniformity in environmental conditions such as seabed temperature at this depth which allows for a more homogenous eurybathic fauna (as described in Brandt et al., 2007).
Understanding of faunal distribution patterns at the SSI, especially in the deep sea, is limited by both paucity in samples and the predominance of rare species. The creation of functional groups in this study was therefore limited by the formulation of groups with enough samples for which functional information was available. As such, the resulting groups were relatively broad and so were limited in their ability to resolve more subtle faunistic differences between clusters. This likely resulted in the observed degree of cross-over between the observed functional composition of each of the 12 gradient forest clusters (see Supplementary Figure 4). That said, there were broad functional trends in our analysis, particularly over depth gradients, that align with previous research across the Antarctic. This included a higher prevalence to sessile suspension feeding taxa on the shelf environment, likely because of the more frequent instances of hard substrate in the form of exposed bedrock, coarse marine sediments and glacial dropstones (Clarke, 1996). In the deep sea, observed prevalence of mobile scavengers such as pycnogonids, amphipods and other peracarid crustaceans in the South Sandwich abyssal and trench clusters has been observed in the Antarctic deep-sea more broadly (Brandt and Hilbig, 2004; Brandt et al., 2007). Furthermore, many of the deep-water clusters also recorded higher prevalence of soft-bodied infaunal and epifaunal scavengers guilds such as polychaetes, nemerteans, and holothurians which have previously been documented to be a dominant taxa on the Antarctic continental slope and at abyssal depths (Brandt et al., 2007).
With SSI fauna linked to latitudinal gradients in temperature, sea ice and productivity, temporal changes in the marine environment resulting from climate change are of fundamental importance in understanding possible ramifications for future trends in diversity and distribution. Positioned south of the South Antarctic Circumpolar Current Front (Figure 1), environmentally the SSI are distinctly Antarctic in character. Consequently, the regions benthic fauna is expected to be characterised by slow growth rates, increased longevity, and deferred sexual maturity (Peck, 2002). As such, many species may have poor ability to cope or adapt to warming oceans compared to species at lower latitudes (Convey and Peck, 2019).
The Southern Ocean has undergone substantial warming since the 1930s (Gille, 2008; Swart et al., 2018) and is forecast to continue this trend (Turner et al., 2016). Regionally, observations at South Georgia demonstrate an average sea-surface (0–150 m) warming of 2.3°C over 81 years (Whitehouse et al., 2008). This warming exhibited intra-annual variation, with warming twice as strong during the winter months. Furthermore, the waters of the ACC have, since the 1960s, also demonstrated more significant warming than the Southern Ocean as a whole (Turner et al., 2013). Most notably, the Circumpolar Deep Water (150–500 m) on the southern edge of the ACC has demonstrated decadal increases up to 0.17°C (Gille, 2002; Böning et al., 2008). Model projections suggest above average future changes near the ACC (Sokolov and Rintoul, 2009), with poleward displacement of the ACC frontal features combined with a strengthening and/or warming of the southernmost jet (Turner et al., 2013).
These changes have significant implications on the capacity of the SSI’s fauna to adapt to, or tolerate, a future marine environment. Sea ice is known to be important to the structure and dynamics of SSI marine ecosystems (Rogers et al., 2015). In spring, melting sea ice is associated with the release of algae and iron incorporated into ice as it formed (Massom and Stammerjohn, 2010). Ice-edge spring melting corresponds with seasonal phytoplankton blooms which form the basis of higher predator food chains (Murphy et al., 2007). Climate-driven changes to sea ice distribution and duration will likely impact the onset and duration of seasonal plankton blooms and productivity fluxes. Furthermore, it could lead to potentially greater seabed disturbance from earlier and more extensive sea ice breakup and iceberg scouring (Barnes, 2017), impinging on the survivorship of benthic communities (Barnes and Souster, 2011).
Given our assessment of latitudinal and temperature gradients in bioregions and a north-south discontinuity in environment and faunal character, changing climate is a key consideration in future-proofing MPA design through assessing potential range-shifts in benthic fauna under different climate scenarios. Furthermore, given the regions unique setting in the Southern Ocean (i.e., an island chain extending across latitude), the islands provide a good natural laboratory in which to assess biogeographical changes in relation to climate change in the Southern Ocean more broadly.
The analysis presented here represents the first attempt to bio-physically describe this region, providing biogeographical context to the South Georgia and South Sandwich Islands Marine Protected Area. The SSI is both diverse in benthic fauna and biogeographically distinct from neighbouring islands in the Scotia Arc. This is particularly notable given the islands small shelf area and its young geological age and the fact that novel species discovery remains high. The composition of benthic fauna changed from north to south across the archipelago. This was manifest in distinct north and south bioregions in both shallow and deep water.
The SSI are typical of many Southern Ocean locations where, due to their isolation and the associated logistical and financial limitations of access, there remains significant paucity in our understanding of the region. We recognise that compilations of diversity data, like reported here, are ultimately limited by the fact that they have been assimilated from different sources. As such, though the data may be presented in the same format, the means by which the data has been collected varies considerably. An improved understanding of the SSI biogeography must therefore ultimately come from the kind of structured sampling effort presented by the research undertaken during the 2019 Blue Belt DY99 sampling campaign.
In terms of spatial protection, our analysis supports the need to conserve the SSI marine environment as distinctly different in physical and faunistic character from the rest of the SGSSI MPA. Protection along the full latitudinal range is important as there is evidence for distinct north-south differences. Based on our current understanding of the region’s benthic environment, the MPA covers a “representative” range of benthic habitats.
Publicly available datasets were analysed in this study. This data can be found here: https://ramadda.data.bas.ac.uk/repository/entry/show/?entryid=b8143952-421c-4544-8437-58f339253d30; https://www.gbif.org/; http://www.science.oregonstate.edu/ocean.productivity/; https://nsidc.org/data/seaice_index/archives/; and https://marine.copernicus.eu/. The source material and datasets collected on DY99 and the summary report are in the process of being made publicly available. Queries regarding access can be directed to the corresponding author.
OH conducted the statistical analysis, wrote the manuscript text, and prepared the figures. A-LD led gradient forest analysis, provided conceptual and analytical assistance, and was involved in the DY99 research expedition data collection. RV provided conceptual assistance and was involved in the DY99 research expedition data collection. CD was scientific lead on the South Georgia and South Sandwich Islands Blue Belt Programme and led the DY99 research expedition. All authors reviewed the manuscript.
This work was funded by the UK Government through the Blue Belt Programme (https://www.gov.uk/government/publications/the-blue-belt-programme). RV was supported by a Darwin Plus Award (DPLUS089).
The authors declare that the research was conducted in the absence of any commercial or financial relationships that could be construed as a potential conflict of interest.
All claims expressed in this article are solely those of the authors and do not necessarily represent those of their affiliated organizations, or those of the publisher, the editors and the reviewers. Any product that may be evaluated in this article, or claim that may be made by its manufacturer, is not guaranteed or endorsed by the publisher.
This manuscript is based on content which first appeared in the UK Government report: Benthic biodiversity in the South Sandwich Islands: Results from the Blue Belt Programme RRS Discovery Expedition DY99 (Darby et al., 2021). For help cataloguing taxa specific functional traits the authors would like to thank David Barnes (BAS), Angelika Brandt (Senckenberg), Rachel Downey (Australian National University), Marc Eléaume (Muséum National d’Histoire Naturelle, Paris), Anne-Nina Lörz (University of Hamburg), Melanie Mackenzie (Museum Victoria), Camille Moreau (Université Libre de Bruxelles), Estefania Rodriguez (American Museum of Natural History), Chester Sands (BAS), and Thomas Saucède (Université de Bourgogne).
The Supplementary Material for this article can be found online at: https://www.frontiersin.org/articles/10.3389/fmars.2021.650241/full#supplementary-material
Supplementary Figure 1 | Bathymetric profile of the South Sandwich Islands from north (left) to south (right). Profile follows shallowest line between each island and is derived from the Leat et al. (2016) digital elevation model.
Supplementary Figure 2 | Importance of environmental variables for predicting distributions of 21 benthic functional groups (see Table 1). Importance was calculated by weighting the functional group-level predictor importance by the functional group R2 and then averaging. For descriptions of environmental variables see Supplementary Table 1.
Supplementary Figure 3 | Environmental characterisation of the 12 mapping clusters shown in Figure 7. In each box plot the middle line represents the median; the upper and lower extent of the box represent the first and third quartile.
Supplementary Figure 4 | Faunal characterisation of the 12 mapping clusters shown in Figure 7. Plots show the ratio of presences (black) to absences (grey) of 21 functional groups (Table 1) for each mapping cluster.
Barnes, D. K. (2002). Invasions by marine life on plastic debris. Nature 416, 808–809. doi: 10.1038/416808a
Barnes, D. K. (2017). Iceberg killing fields limit huge potential for benthic blue carbon in Antarctic shallows. Glob. Change Biol. 23, 2649–2659.
Barnes, D. K. A., and Griffiths, H. J. (2008). Biodiversity and biogeography of southern temperate and polar bryozoans. Glob. Ecol. Biogeogr. 17, 84–99. doi: 10.1201/b14798-10
Barnes, D. K. A., and Souster, T. (2011). Reduced survival of Antarctic benthos linked to climate-induced iceberg scouring. Nat. Clim. Change 1, 365–368. doi: 10.1038/nclimate1232
Barnes, D. K. A., Collins, M. A., Brickle, P., Fretwell, P. T., Griffiths, H. J., Herbert, D., et al. (2011). The need to implement the convention on biological diversity at the high latitude site, South Georgia. Antarct. Sci. 23, 323–331. doi: 10.1017/s0954102011000253
Barnes, D. K. A., Kaiser, S., Griffiths, H. J., and Linse, K. (2009). Marine, intertidal, freshwater and terrestrial biodiversity of an isolated polar archipelago. J. Biogeogr. 36, 756–769. doi: 10.1111/j.1365-2699.2008.02030.x
Barnes, D. K. A., Linse, K., Waller, C., Morely, S., Enderlein, P., Fraser, K. P. P., et al. (2006). Shallow benthic fauna communities of South Georgia island. Polar Biol. 29, 223–228. doi: 10.1007/s00300-005-0042-0
Barnes, D. K., and Sands, C. J. (2017). Functional group diversity is key to Southern Ocean benthic carbon pathways. PLoS One 12:e0179735. doi: 10.1371/journal.pone.0179735
Behrenfeld, M. J., and Falkowski, P. G. (1997). Photosynthetic rates derived from satellite-based chlorophyll concentration. Limnol. Oceanogr. 42, 1–20. doi: 10.4319/lo.1997.42.1.0001
Boehner, J., and Selige, T. (2006). “Spatial prediction of soil attributes using terrain analysis and climate regionalisation,” in SAGA - Analysis and Modelling Applications, eds J. Boehner, K. R. McCloy, and J. Strobl (Goettingen: Goettinger Geographische Abhandlungen), 13–28.
Böning, C. W., Dispert, A., Visbeck, M., Rintoul, S. R., and Schwarzkopf, F. U. (2008). The response of the Antarctic circumpolar current to recent climate change. Nat. Geosci. 1, 864–869. doi: 10.1038/ngeo362
Brandt, A., and Hilbig, B. (2004). ANDEEP (ANtarctic benthic DEEP-sea biodiversity: colonization history and recent community patterns)—a tribute to Howard L. Sanders. Deep Sea Res. II Top. Stud. Oceanogr. 51, 1457–1465. doi: 10.1016/j.dsr2.2004.08.006
Brandt, A., De Broyer, C., De Mesel, I., Ellingsen, K. E., Gooday, A. J., Hilbig, B., et al. (2007). The biodiversity of the deep Southern Ocean benthos. Philos. Trans. R. Soc. B Biol. Sci. 362, 39–66. doi: 10.1098/rstb.2006.1952
Brasier, M. J., Grant, S. M., Trathan, P. N., Allcock, L., Ashford, O., Blagbrough, H., et al. (2018). Benthic biodiversity in the South Orkney Islands Southern shelf marine protected area. Biodiversity 19, 5–19.
Bryan, S. E., Cook, A. G., Evans, J. P., Hebden, K., Hurrey, L., Colls, P., et al. (2012). Rapid, long-distance dispersal by pumice rafting. PLoS One 7:e40583. doi: 10.1371/journal.pone.0040583
Chao, A. (1987). Estimating the population size for capture-recapture data with unequal catchability. Biometrics 43, 783–791. doi: 10.2307/2531532
Chown, S. L., Clarke, A., Fraser, C. I., Cary, S. C., Moon, K. L., and McGeoch, M. A. (2015). The changing form of Antarctic biodiversity. Nature 522:431. doi: 10.1038/nature14505
Clarke, A. (1996). “Benthic marine habitats in Antarctica,” in Origins and Evolution of the Antarctic Biota, eds R. M. Ross, E. E. Hoffman, and L. B. Quentin (London: The Geological Society), 253–268.
Clarke, A., and Leakey, R. J. G. (1996). The seasonal cycle of phytoplankton, macronutrients, and the microbial community in a nearshore Antarctic marine eco system. Limnol. Oceanogr. 41, 1281–1294. doi: 10.4319/lo.1996.41.6.1281
Clarke, A., Barnes, D. K. A., and Hodgson, D. A. (2005). How isolated is Antarctica? Trends Ecol. Evol. 20, 1–3. doi: 10.1016/j.tree.2004.10.004
Clarke, A., Croxall, J. P., Poncet, S., Martin, A. R., and Burton, R. (2012). Important bird areas: South Georgia. Br. Birds 105, 118–144.
Collins, M. A., Brickle, P., Brown, J., and Belchier, M. (2010). The Patagonian toothfish: biology, ecology and fishery. Adv. Mar. Biol. 58, 227–300.
Collins, M. A., Trathan, P., Grant, S., Davidson, D., and Ross, K. (2012). South Georgia and South Sandwich Islands Marine Protected Area Management Plan. Stanley: Government of South Georgia and South Sandwich Islands.
Conrad, O., Bechtel, B., Bock, M., Dietrich, H., Fischer, E., Gerlitz, L., et al. (2015). System for automated geoscientific analyses (SAGA) v. 2.1. 4. Geosci. Model Dev. 8, 1991–2007. doi: 10.5194/gmd-8-1991-2015
Convey, P., and Peck, L. S. (2019). Antarctic environmental change and biological responses. Sci. Adv. 5:eaaz0888. doi: 10.1126/sciadv.aaz0888
Convey, P., Morton, A., and Poncet, J. (1999). Survey of marine birds and mammals of the South Sandwich Islands. Polar Rec. 35, 107–124. doi: 10.1017/s0032247400026450
Darby, C. D., O, T., Hogg, Downie, A., Vieira, R., and Soeffker, M. (2021). Benthic Biodiversity of the South Sandwich Islands, Results from the RRS Discovery Expedition DY99, Report Number CR0124. U.K: Blue Belt Programme, 139.
Desmet, P. J. J., and Govers, G. (1996). A GIS procedure for automatically calculating the USLE LS factor on topographically complex landscape units. J. Soil Water Conserv. 51, 427–433.
Dickens, W. A., Graham, A. G. C., Smith, J. A., Dowdeswell, J. A., Larter, R. D., Hillenbrand, C. D., et al. (2014). A new bathymetric compilation for the South Orkney Islands, Antarctic Peninsula (49°–39°W to 64°–59°S): insights into the glacial development of the continental shelf. Geochem. Geophys. Geosyst. 15, 2494–2514. doi: 10.1002/2014GC005323
Downie, A. L., Vieira, R. P., Hogg, O. T., and Darby, C. (2021). Distribution of vulnerable marine ecosystems at the South Sandwich Islands: results from the Blue Belt Discovery Expedition 99 deep-water camera surveys. Front. Mar. Sci. 8:662285. doi: 10.3389/fmars.2021.662285
Ellis, N., Smith, S. J., and Pitcher, C. R. (2012). Gradient forests: calculating importance gradients on physical predictors. Ecology 93, 156–168. doi: 10.1890/11-0252.1
Figuerola, B., Barnes, D. K., Brickle, P., and Brewin, P. E. (2017). Bryozoan diversity around the Falkland and South Georgia Islands: overcoming Antarctic barriers. Mar. Environ. Res. 126, 81–94. doi: 10.1016/j.marenvres.2017.02.005
Fraser, C. I., Kay, G. M., Plessis, M. D., and Ryan, P. G. (2016). Breaking down the barrier: dispersal across the Antarctic Polar Front. Ecography 40, 235–237.
Gaston, K. J., and Spicer, J. I. (2013). Biodiversity: An Introduction. Hoboken, NJ: John Wiley & Sons.
Gille, S. T. (2002). Warming of the Southern Ocean since the 1950s. Science 295, 1275–1277. doi: 10.1126/science.1065863
Gille, S. T. (2008). Decadal-scale temperature trends in the Southern Hemisphere ocean. J. Clim. 21, 4749–4765. doi: 10.1175/2008jcli2131.1
Gon, O., and Heemstra, P. C. (eds) (1990). Fishes of the Southern Ocean, Vol. 1. Grahamstown: JLB: Smith Institute of Ichthyology.
Grant, S., Constable, A., Raymond, B., and Doust, S. (2006). Bioregionalisation of the Southern Ocean: Report of Experts Workshop, Hobart, September 2006. WWF-Australia and ACE CRC. Gland: WWF, 45.
Griffiths, H. J., Barnes, D. K., and Linse, K. (2009). Towards a generalized biogeography of the Southern Ocean benthos. J. Biogeogr. 36, 162–177. doi: 10.1111/j.1365-2699.2008.01979.x
Griffiths, H. J., Linse, K., and Barnes, D. K. (2008). Distribution of macrobenthic taxa across the Scotia Arc, Southern Ocean. Antarct. Sci. 20, 213–226. doi: 10.1017/s0954102008001168
Harris, P. T., and Baker, E. (2012). “Seafloor Geomorphology as Benthic Habitat,” in GeoHAB Atlas of Seafloor Geomorphic Features and Benthic Habitats, eds P. T. Harris and E. K. Baker (London: Elsevier).
Hawkesworth, C. J., O’nions, R. K., Pankhurst, R. J., Hamilton, P. J., and Evensen, N. M. (1977). A geochemical study of island-arc and back-arc tholeiites from the Scotia Sea. Earth Planet. Sci. Lett. 36, 253–262. doi: 10.1016/0012-821x(77)90207-2
Hedgpeth, J. W. (1969). Introduction to Antarctic Zoogeography. Distribution of Selected Groups of Marine Invertebrates in S. Antarctic Map Folio Series, Folio 11, Waters South of 35°, eds V. C. Bushnell and J. W. Hedgpeth (New York, NY: American Geographical Society), 1–29.
Hogg, O. T., Barnes, D. K. A., and Griffiths, H. J. (2011). Highly diverse, poorly studied and uniquely threatened by climate change: an assessment of marine biodiversity on South Georgia’s continental shelf. PLoS One 6:e19795. doi: 10.1371/journal.pone.0019795
Hogg, O. T., Huvenne, V. A., Griffiths, H. J., and Linse, K. (2018). On the ecological relevance of landscape mapping and its application in the spatial planning of very large marine protected areas. Sci. Tot. Environ. 626, 384–398. doi: 10.1016/j.scitotenv.2018.01.009
Hogg, O. T., Huvenne, V. A., Griffiths, H. J., Dorschel, B., and Linse, K. (2016). Landscape mapping at sub-Antarctic South Georgia provides a protocol for underpinning large-scale marine protected areas. Sci. Rep. 6:33163.
Holeton, C. L., Nedelec, F., Sanders, R., Brown, L., Moore, C. M., Stevens, D. P., et al. (2005). Physiological state of phytoplankton communities in the Southwest Atlantic sector of the Southern Ocean, as measured by fast repetition rate fluorometry. Polar Biol. 29, 44–52. doi: 10.1007/s00300-005-0028-y
Howe, J. A., Shimmield, T. M., and Diaz, R. (2004). Deep-water sedimentary environments of the northwestern Weddell Sea and South Sandwich Islands, Antarctica. Deep Sea Res. II Top. Stud. Oceanogr. 51, 1489–1514. doi: 10.1016/j.dsr2.2004.07.011
Kaiser, S., Barnes, D. K., Linse, K., and Brandt, A. (2008). Epibenthic macrofauna associated with the shelf and slope of a young and isolated Southern Ocean island. Antarct. Sci. 20, 281–290. doi: 10.1017/s0954102008001107
Kenchington, E., Lirette, C., Murillo, F. J., Beazley, L., and Downie, A.-L. (2020). Vulnerable Marine Ecosystems in the NAFO Regulatory Area: Updated Kernel Density Analyses of Vulnerable Marine Ecosystem Indicators. N7030. Report Number: NAFO SCR Doc. 19/058. Dartmouth, NS: Northwest Atlantic Fisheries Organization, 68.
Knox, G. A. (1960). Littoral ecology and biogeography of the southern oceans. Proc. R. Soc. Lond. Ser. B Biol. Sci. Sci. 152, 577–624.
Kursa, M. B., and Rudnicki, W. R. (2010). Feature selection with the boruta package. J. Stat. Softw. 36, 1–13.
Leat, P. T., Day, S. J., Tate, A. J., Martin, T. J., Owen, M. J., and Tappin, D. R. (2013). Volcanic evolution of the South Sandwich volcanic arc, South Atlantic, from multibeam bathymetry. J. Volcanol. Geothermal Res. 265, 60–77. doi: 10.1016/j.jvolgeores.2013.08.013
Leat, P. T., Fretwell, P. T., Tate, A. J., Larter, R. D., Martin, T. J., Smellie, J. L., et al. (2016). Bathymetry and geological setting of the South Sandwich Islands volcanic arc. Antarct. Sci. 28, 293–303. doi: 10.1017/s0954102016000043
Leat, P. T., Tate, A. J., Tappin, D. R., Day, S. J., and Owen, M. J. (2010). Growth and mass wasting of volcanic centers in the northern South Sandwich arc, South Atlantic, revealed by new multibeam mapping. Mar. Geol. 275, 110–126. doi: 10.1016/j.margeo.2010.05.001
Linse, K., Copley, J. T., Connelly, D. P., Larter, R. D., Pearce, D. A., Polunin, N. V., et al. (2019). Fauna of the kemp caldera and its upper bathyal hydrothermal vents (South Sandwich Arc, Antarctica). R. Soc. Open Sci. 6:191501. doi: 10.1098/rsos.191501
Linse, K., Griffiths, H. J., Barnes, D. K., and Clarke, A. (2006). Biodiversity and biogeography of Antarctic and sub-Antarctic mollusca. Deep Sea Res. Part II Top. Stud. Oceanogr. 53, 985–1008.
Livermore, R., Hillenbrand, C. D., Meredith, M., and Eagles, G. (2007). Drake passage and cenozoic climate: an open and shut case? Geochem. Geophys. Geosyst. 8, 1–11.
Lockhart, S. J., and Jones, C. D. (2008). Biogeographic patterns of benthic invertebrate megafauna on shelf areas within the Southern Ocean Atlantic sector. CCAMLR Sci. 15, 167–192.
Malyutina, M. (2004). Russian deep-sea investigations of Antarctic fauna. Deep Sea Res. II Top. Stud. Oceanogr. 51, 1551–1570. doi: 10.1016/j.dsr2.2004.07.012
Marsh, L., Copley, J. T., Huvenne, V. A., Linse, K., Reid, W. D., Rogers, A. D., et al. (2012). Microdistribution of faunal assemblages at deep-sea hydrothermal vents in the Southern Ocean. PLoS One 7:e48348. doi: 10.1371/journal.pone.0048348
Massom, R. A., and Stammerjohn, S. E. (2010). Antarctic Sea ice change and variability–physical and ecological implications. Polar Sci. 4, 149–186. doi: 10.1016/j.polar.2010.05.001
Murphy, E. J., Watkins, J. L., Trathan, P. N., Reid, K., Meredith, M. P., Thorpe, S. E., et al. (2007). Spatial and temporal operation of the Scotia Sea ecosystem: a review of large-scale links in a krill centred foodweb. Philos. Trans. R. Soc. B 362, 113–148. doi: 10.1098/rstb.2006.1957
O’Brien, P. E., Post, A. L., and Romeyn, R. (2009). “Antarctic-wide Geomorphology as an aid to habitat mapping and locating Vulnerable Marine Ecosystems,” in Proceedings of the Science Committee to the Commission of Antartctic Marine Living Resources (SC-CAMLR-XXVIII/10) Workshop on Vulnerable Marine Ecosystems. La Jolla, CA, USA 3–7th August 2009: GeoScience Australia. Conference Paper: WS-VME-09/10 (La Jolla, CA).
Oksanen, J., Blanchet, F. G., Friendly, M., Kindt, R., Legendre, P., McGlinn, D., et al. (2019). Vegan: Community Ecology Package. R Package Version 2.5-5. Available online at: https://CRAN.R-project.org/package=vegan
Olaya, V. (2009). “Basic land-surface parameters,” in Geomorphometry. Concepts, Software, Applications, eds T. Hengl and H. I. Reuter (Amsterdam: Elsevier), 141–169. doi: 10.1016/S0166-2481(08)00006-8
Peck, L. S. (2002). “Ecophysiology of Antarctic marine ectotherms: limits to life,” in Ecological Studies in the Antarctic Sea Ice Zone, eds W. Arntz and A. Clarke (Berlin: Springer), 221–230. doi: 10.1007/978-3-642-59419-9_29
Perissinotto, R., Laubscher, R., and Mcquaid, C. (1992). Marine productivity enhancement around Bouvet and the South Sandwich Islands (Southern-Ocean). Mar. Ecol. Prog. Ser. 88, 41–53. doi: 10.3354/meps088041
Pitcher, R. C., Lawton, P., Ellis, N., Smith, S. J., Incze, L. S., Wei, C. L., et al. (2012). Exploring the role of environmental variables in shaping patterns of seabed biodiversity composition in regional-scale ecosystems. J. Appl. Ecol. 49, 670–679. doi: 10.1111/j.1365-2664.2012.02148.x
R Core Team (2019). R: A Language and Environment for Statistical Computing. Vienna: R Foundation for Statistical Computing.
Ramos, A. (1999). The megazoobenthos of the Scotia Arc islands. Sci. Mar. 63, 171–182. doi: 10.3989/scimar.1999.63s1171
Ramos-Esplá, A. A., Cárcel, J. A., and Varela, M. (2005). Zoogeographical Relationships of the Littoral Ascidiofauna Around the Antarctic Peninsula in the Scotia Arc and in the Magellan Region. Spain: Scientia Marina.
Roberts, J. O. (2012). Ecology and Management of Range Edge Populations: The Case of Toothfish Species at the South Sandwich Islands. Doctoral Thesis. London: Imperial College London.
Roberts, J., and Agnew, D. A. (2008). “Proposal for an extension to the mark recapture experiment to estimate toothfish population size in Subarea 48.4,” in Proceedings of the Commission for the Conservation of Antarctic Marine Living Resources. Document WG-FSA-08/46 (Hobart, TAS).
Rogers, A. D., Yesson, C., and Gravestock, P. (2015). A biophysical and economic profile of South Georgia and the South Sandwich Islands as potential large-scale Antarctic protected areas. Adv. Mar. biol. 70, 1–286. doi: 10.1016/bs.amb.2015.06.001
Rosenzweig, M. L. (1995). Species Diversity in Space and Time. Cambridge: Cambridge University Press.
Smith, S. D. (2002). Kelp rafts in the Southern Ocean. Glob. Ecol. Biogeogr. 11, 67–69. doi: 10.1046/j.1466-822x.2001.00259.x
Sokolov, S., and Rintoul, S. R. (2009). Circumpolar structure and distribution of the Antarctic Circumpolar Current fronts: 1. Mean circumpolar paths. J. Geophys. Res. Oceans 114, 1–19.
Spalding, M. D., Fox, H. E., Allen, G. R., Davidson, N., Ferdana, Z. A., Finlayson, M. A. X., et al. (2007). Marine ecoregions of the world: a bioregionalization of coastal and shelf areas. BioScience 57, 573–583. doi: 10.1641/b570707
Swart, N. C., Gille, S. T., Fyfe, J. C., and Gillett, N. P. (2018). Recent Southern Ocean warming and freshening driven by greenhouse gas emissions and ozone depletion. Nat. Geosci. 11, 836–841.
Thomson, M. R. A. (2004). Geological and palaeoenvironmental history of the Scotia Sea region as a basis for biological interpretation. Deep Sea Res. II Top. Stud. Oceanogr. 51, 1467–1487.
Trathan, P. N., Collins, M. A., Grant, S. M., Belchier, M., Barnes, D. K., Brown, J., et al. (2014). The South Georgia and South Sandwich Islands MPA: protecting a biodiverse oceanic island chain situated in the flow of the Antarctic Circumpolar Current. Adv. Mar. Biol. 69, 15–77.
Turner, J., Lu, H., White, I., King, J. C., Phillips, T., Hosking, J. S., et al. (2016). Absence of 21st century warming on Antarctic Peninsula consistent with natural variability. Nature 535, 411–415.
Turner, J., Maksym, T., Phillips, T., Marshall, G. J., and Meredith, M. P. (2013). The impact of changes in sea ice advance on the large winter warming on the western Antarctic Peninsula. Int. J. Climatol. 33, 852–861.
Tynan, C. T. (1998). Ecological importance of the southern boundary of the Antarctic Circumpolar Current. Nature 392, 708–710.
Watts, P. C., Thorpe, J. P., and Taylor, P. D. (1998). Natural and anthropogenic dispersal mechanisms in the marine environment: a study using cheilostome Bryozoa. Philos. Trans. R. Soc. Lond. Ser. B Biol. Sci. 353, 453–464.
Whitehouse, M. J., Meredith, M. P., Rothery, P., Atkinson, A., Ward, P., and Korb, R. E. (2008). Rapid warming of the ocean around South Georgia, Southern Ocean, during the 20th century: forcings, characteristics and implications for lower trophic levels. Deep Sea Res. I Oceanogr. Res. Pap. 55, 1218–1228.
Wickham, H., François, R., Henry, L and Müller, K. (2019). dplyr: A Grammar of Data Manipulation. R package Version 0.8.0.1. Available online at: https://CRAN.R-project.org/package=dplyr
Keywords: benthic ecology, marine conservation, marine spatial planning, Southern Ocean, bioregionalisation, habitat mapping, functional trait analysis
Citation: Hogg OT, Downie A-L, Vieira RP and Darby C (2021) Macrobenthic Assessment of the South Sandwich Islands Reveals a Biogeographically Distinct Polar Archipelago. Front. Mar. Sci. 8:650241. doi: 10.3389/fmars.2021.650241
Received: 06 January 2021; Accepted: 29 July 2021;
Published: 23 August 2021.
Edited by:
David Andrew Feary, MRAG Ltd., United KingdomReviewed by:
Blanca Figuerola, Institute of Marine Sciences (ICM-CSIC), SpainCopyright © 2021 Hogg, Downie, Vieira and Darby. This is an open-access article distributed under the terms of the Creative Commons Attribution License (CC BY). The use, distribution or reproduction in other forums is permitted, provided the original author(s) and the copyright owner(s) are credited and that the original publication in this journal is cited, in accordance with accepted academic practice. No use, distribution or reproduction is permitted which does not comply with these terms.
*Correspondence: Oliver T. Hogg, b2xpdmVyLmhvZ2dAY2VmYXMuY28udWs=
Disclaimer: All claims expressed in this article are solely those of the authors and do not necessarily represent those of their affiliated organizations, or those of the publisher, the editors and the reviewers. Any product that may be evaluated in this article or claim that may be made by its manufacturer is not guaranteed or endorsed by the publisher.
Research integrity at Frontiers
Learn more about the work of our research integrity team to safeguard the quality of each article we publish.