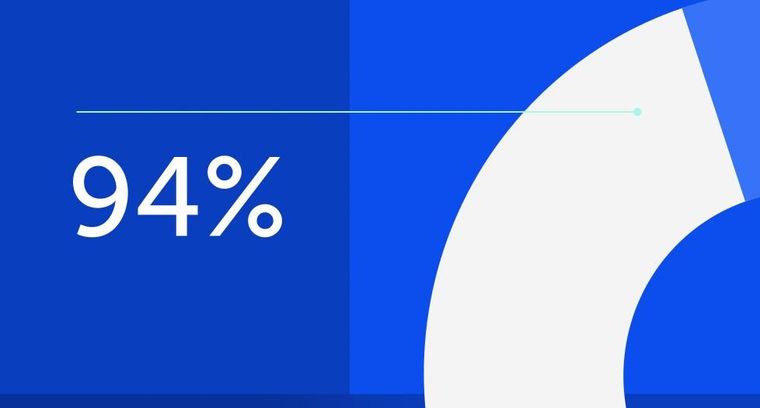
94% of researchers rate our articles as excellent or good
Learn more about the work of our research integrity team to safeguard the quality of each article we publish.
Find out more
BRIEF RESEARCH REPORT article
Front. Mar. Sci., 25 August 2021
Sec. Ocean Solutions
Volume 8 - 2021 | https://doi.org/10.3389/fmars.2021.644056
The sustainable use of common pool resources (CPRs) such as fisheries constitutes a major challenge for society. A large body of empirical studies conducted in discrete time indicates that resource users are able to prevent the ‘tragedy of the commons' under institutional arrangements that can promote cooperation. However, the variability exhibited by the human behaviour and the dynamic nature of renewable resources require continuous time experiments to fully explain the mechanisms underpinning the sustainable use of resources. We conducted CPR experiments in continuous time to investigate how the extraction behaviour of resource users changes in real-time in response to changes in resource availability under communication and no communication. We find that when communication is allowed, users adjust their extraction efforts based on knowledge of previous resource availability. In contrast, when communication is not allowed, users do not incorporate resource availability into their utility function. These results suggest that communication does not merely provide a forum for coordination but mediates a causal relationship between resource levels and extraction behaviour. Our findings may help the development of effective resource management policies.
Many common pool renewable resources are exploited beyond their regenerative capacities. For example, more than 33% of fish stocks around the world, which constitute a critical source of food, nutrition and employment for millions of people, are fished at biologically unsustainable levels (FAO, 2018). Despite a great variety of management tools (e.g., Marine Protected Areas with many different characteristics in relation to restrictions, which can range from fishing only specific species, or allowing limited recreational activities, to no-take, or even no-use) and policies (e.g., fishing quotas, incentives, and different forms of participation of resource users to fishery management decisions) are being adopted in many different contexts since decades, the state of marine fisheries has continued to decline (Pauly and Zeller, 2016).
Management procedures (Butterworth and Punt, 1999) and, more specifically, Management Strategy Evaluation (MSE) frameworks (Smith et al., 1999) have become widely used in fishery management because they help to evaluate the effectiveness of different policies at the ecosystem level and based on multiple and conflicting objectives. With the integration of observational data and simulation models, these frameworks upgrade traditional management approaches by systematically dealing with uncertainties and by accounting for the dynamic nature of resource systems (Punt et al., 2016). Simulative tools such as Agent-Based Models are also gaining popularity in fishery management (e.g., Bailey et al., 2019; Owusu et al., 2020). Agent-Based Models constitute a bottom-up modelling approach that incorporates the behaviour of many heterogeneous actors dynamically responding to changes in resource availability and other social and economic aspects and are also used in MSE frameworks (Burgess et al., 2020). These evaluation frameworks are thus decision support tools for natural resource managment, in which many components of the resource system are modelled simultaneously to analyse the performance of given management policies.
Whereas management procedures and evaluation frameworks represent important operational tools for evaluating different management measures against user- and resource-oriented objectives, at a more fundamental level, common-pool resource (CPR) game experiments have been used over the last decades to understand the basic factors driving human-resource interactions and have thus contributed to the definition of governance and management measures for specific resource systems (Ostrom et al., 1994). In particular, experimental research on the governance of common-pool resources (CPR) has enabled social scientists to disentagle the effects of multiple factors on specific aspects of common pool resources like, for example, user behaviour or resource levels (Ostrom, 2006), and to replicate results with diverse participants (Falk and Heckman, 2009). Experimental tools are particularly relevant when the object of investigation is the causal relationship between variables because in a laboratory setting the components of a theoretical situation can be carefully established and all factors can be controlled thus avoiding that they confound the analysis (Ostrom, 2006).
Common Pool Resource (CPR) experiments have shown, for example, that the "tragedy of the commons" (Hardin, 1968) can be avoided, and that under certain institutional arrangements (e.g., when participants can communicate) resource users can cooperate to maintain the CPR at sustainable levels (Ostrom et al., 1994). To account for resource dynamics, CPR experiments incorporate resource levels, whether as a static or as a dynamic externality. In the static case, the resource regenerates to its original level at the beginning of every round. In the dynamic case, the stock of the resource is taken over from one round to the next, including its growth rate (Bru et al., 2003). In these round-based experiments, resource users receive information on the resource levels at the beginning of each round and make decisions on the effort they want to exert during harvest. As resource levels and user efforts update only once per round, these experiments can be understood as discrete time experiments. Discrete time (or round based) experiments lack realism because in real-world user-resource interactions the resource continuously changes and users update their extraction behaviour repeatedly over time (Brandt et al., 2017).
Previous work shows that the sustainability of CPRs constitutes a long-standing challenge due to the highly dynamic nature of ecosystems (Schill et al., 2015). A recent study focused on the dynamic relationship between extraction effort and resource availability (Safarzynska, 2018) found that resource scarcity affects extraction behaviour. Besides this study and a few other exceptions (Janssen et al., 2010; Oprea et al., 2011; Bigoni et al., 2015), most of our experimental knowledge on CPR managament is based on experiments conducted in discrete time. Therefore, comparatively less attention has been devoted so far on the causal links between resource levels and efforts exerted by users under continuous time conditions. This aspect is of crucial importance because the dynamic nature of both human behaviours and ecological resources inherent to every CPR system involves feedback that are not accounted for in discrete time settings. While uncertainties related to resource dynamics are widely studied, their effects on the behaviour of users received less consideration (Fulton et al., 2012).
We conducted CPR experiments mimicking a fishery to gain insights on the way resource dynamics interact with effort dynamics. The experiments were conducted in continuous time using the open-source software OGUMI, specifically designed for this purpose (Brandt et al., 2017). In contrast to similar existing software tools (see Janssen, 2014, for a review), which focus on standard game theoretical situations (Pettit et al., 2014), are difficult to run in the field (Goldstone and Ashpole, 2004), or are not designed to capture real-time user responses to dynamically varying resources (Fischbacher, 2007; Chen et al., 2016), OGUMI produces high-frequency time-series that record the instantaneous adaptive reaction of humans to changing resource levels on mobile platforms (Brandt et al., 2017). In our experiments, we considered two classic institutional arrangements: communication and no communication (Balliet, 2010). We then used a stochastic process model to analyse the causality between the data on extraction behaviour and resource levels produced with our experiments. A fairly large body of literature shows that communication increases cooperation due to the beneficial effects of coordination (e.g., Ostrom, 2006; Balliet, 2010; Oprea et al., 2014; Mantilla, 2015). Here we find evidence that communication does not merely provide a forum for coordination (e.g., Turner et al., 2014) but mediates a causal relationship between resource levels and extraction behaviour that leads to sustainable harvest.
To investigate the dynamic relationship between resource and effort levels, we conducted a CPR experiment with two classic and well-studied treatments (see, for example, Ostrom, 2006), namely communication and no communication. In the first treatment, participants were invited to hold face-to-face discussions as the experiment progressed, thus as the effort decisions were taking place. In the second treatment, subjects were prohibited from communicating with one another, thus effort decisions were not affected by explicit group goals. Participants were seated in the same room at about 3 m from each other. Face-to-face communication involved continuous discussions on the effort levels to exert and on the consequences that increasing or decreasing efforts was having on the resource. Therefore, in the treatment that allowed communication, participants decided about their harvest in a cooperative manner.
We conducted the experiments at the Leibniz Centre for Tropical Marine Research in Bremen, Germany, in August 2015 and February 2016. We recruited 70 participants through an online platform for small jobs in Bremen. None of the subjects had participated in an economic experiment before. All subjects gave their informed consent for inclusion before they participated in the study, and the experiments were conducted anonymously. Participants were selected to reflect different demographic characteristics with respect to age, gender, and income (Table 1).
The experiments were conducted using OGUMI, a software designed to run CPR experiments in continuous time (Brandt et al., 2017). With OGUMI, the levels of a common fish pool are updated in real-time while participants extract from the common pool, according to the classic Schaefer's model (Schaefer, 1991):
Where R is the resource level, t is time, G is the resource gross growth, which is assumed to follow a logistic function with carrying capacity K and maximum growth rate μR, H is the harvest rate (i.e., the amount of fish extracted per time), N is the total number of resource users (i.e., the participants in our experiments), Ei is the fishing effort exerted by participant i, and q is the catchability coefficient (defined as the fraction of the resource fished per unit effort).
Three experimental sessions were run for each treatment (Table 2). In all sessions, participants constantly received (through the OGUMI screen) information on the status of the resource, their individual harvest, and their earnings (payoffs). Because resource uncertainty was beyond the scope of our investigation, we assumed that participants had perfect and continuous knowledge of the resource levels in both treatments. Final payoffs were determined by the individual harvests, which were converted into Euros using a fixed rate. Assuming a specific cost c per unit effort and a price p per fish unit, the payoff per unit time of participant i was calculated as follows:
With individual harvest Hi = qREi. Complete information on how the ecological and financial incentive models (Equations 1 and 2, respectively) are parameterised is provided in Supplementary Text. Fishing effort is defined in our system as a non-dimensional index varying from 0 (equivalent to no effort and thus to no harvest) to 10 (equivalent to maximum effort), see Supplementary Text for full details on model parameterisation. Each participant independently decided on how much fishing effort to exert, and could change this effort at any time during the experiment. All changes in effort and their effects on the resource level were updated instantaneously in real-time.
Table 2. Overview of the different experimental sessions per treatment and average payoffs the participants earned during each session.
Each experimental session involved the following steps: (1) each participant received an Android tablet upon arrival; (2) instructions were provided in writing and read aloud when all participants were in the room; (3) a test experiment was run for nearly 4 min to allow participants to familiarise with the software; (4) the actual CPR experiment was run for 10 min (600 s); (5) participants answered a series of questions, which allowed us to gather the demographic information mentioned above and reported in Table 1. All experiments were run with an initial resource level of 20 fish and a carrying capacity (K in Equation 1) of 100 fish.
From the time-series obtained in all sessions, we excluded the first 100 s to remove confounding effects due to an initial transient phase before average steady conditions are established and potential delays with which participants started the game. The time-series were then checked for stationarity, tested for co-integration, and examined with respect to the order of integration. We then applied the following vector autoregressive (VAR) model:
where R, E represents resource and effort, respectively; m is the lag-length; α1, α2 are constants; β1,i⋯1,m, γ1,i⋯1, m, β2,i⋯2,m, γ2,i⋯2,m, are coefficients; and u1,t, u2,t are white noise error terms.
For the treatment that showed co-integrated variables, we specified a VAR with an error-correction term (VECM) as follows:
where Δ is the difference operator; ECT is the error-correction term; and λ1, λ2 are the error-correction coefficients. The optimal lag length m of the model was chosen based on the Schwarz Information Criterion.
On the estimated VAR model, we conducted an impulse response analysis to study the dynamic interaction between the variables in both treatments. Finally, we tested a causal relationship between the variables. Specifically, we tested whether previous knowledge on resource levels showed a cause-and-effect relationship with current effort levels using the Toda-Yamamoto approach (Toda and Yamamoto, 1995):
where dmax is the maximal order of integration of the variables. Causality between resource and effort exists if the null hypothesis Ho: β2,1 = β2,2 = ⋯ = β2,m ≠ 0 is verified. The Toda-Yamamoto approach is a sophisticated and well-established econometric test that involves estimating a VAR model in levels of the variables irrespective of whether the time series is integrated or co-integrated. In contrast to conventional causality tests, such as the standard Granger's test, which estimates a VAR model in the first differences of the variables (Granger, 1969), the Toda-Yamamoto test has the potential to overcome the problem of invalid asymptotic critical values when causality tests are performed in the presence of non-stationary or even co-integrated variables.
Empirical evidence shows that in laboratory experiments non-standard subjects display similar behavioural patterns to resource users and that they are appropriate subjects for examining human behaviour in relation to public policy design (Janssen et al., 2011; Exadaktylos et al., 2013). Based on this evidence and following the recommendations of Harrison and List (2004), we undertook artefactual field experiments where subjects were drawn randomly from the streets. This approach (which is alternative to the more common and easier approach of using students) provided us with some variability in socio-demographic characteristics, such as age, gender, and income, thus allowing us to assemble a heterogeneous population also in terms of time preferences. In doing so, we created a "convenience sample" of subjects with a good degree of heterogeneity and, thus, some level of generalisability.
Participants were able to consistently maintain a mean resource level of about 44 fishes (i.e., 44% of carrying capacity) when communication was allowed (Figure 1A), and of about 18 fishes (i.e., 18% of carrying capacity) when communication was not allowed (Figure 1C). Also, average earnings per session were higher under communication than under no-communication (Table 2). By defining sustainable conditions those that, in relative terms, are characterised by (1) resource levels closer to carrying capacity and (2) higher earnings, we found that participants harvested more sustainably under communication than under no-communication. In the last few seconds of the experiments, participants did not see any need in preserving the resource because they knew the experiments were about to end (a well-known end-game effect; see, for example, Janssen et al., 2010), thus resource levels reached minimum values. The final values of the resource were 32 and 12 fishes for, respectively, the communication and no-communication treatments.
Figure 1. Summary of results. Time-series of resource and effort levels obtained with the CPR experiments under (A) communication and (C) no communication. Resource and effort blue and red lines, respectively, in (A,C) are averages of the three experimental sessions we run for each treatment, see Table 2. The carrying capacity is set to 100 fishes in all sessions. Impulse response analysis showing responses of effort to changes in resource levels for (B) communication and (D) no communication (red lines). The dashed lines represent the 95% confidence intervals and the continuous horizontal lines mark the zero.
On a qualitative ground, our experiments showed that when effort is high, resource levels are low and vice versa under both treatments (Figures 1A,C). From a quantitative point of view, the results of our experiments showed that resource levels were on average 2.5 times higher (t = −5.89, p < 0.02) and effort levels were on average 1.5 times lower (t = 5.75, p < 0.02) for the treatment allowing communication compared to the treatment not allowing communication (Supplementary Table 1). Under communication, both resource and effort were non-stationary at levels but became stationary after first difference, i.e., integrated of order one (Supplementary Tables 2, 3). However, under no communication, resource was non-stationary at levels but became stationary after first difference, i.e., integrated of order one, and effort was stationary at levels, i.e., integrated of order zero (Supplementary Tables 4, 5).
The results of the cointegration test (Supplementary Table 6) suggests that the null hypothesis of a single cointegration vector for the communication treatment cannot be rejected. Thus, we conducted a VECM for the communication treatment and a VAR for the no communication treatment (the model parameters and tests are presented in Supplementary Tables 7–10).
Figure 1B shows that for the communication treatment, an unanticipated change in resource level significantly and permanently lowers effort in the first 20 s. A different pattern emerged for the no communication treatment, whereby efforts responded only temporarily and with a slight (albeit significant) decline in the first 12 s (Figure 1D). In addition, the Toda-Yamamoto causality test indicated that the lagged values of the resource levels significantly predict effort levels for the treatment allowing communication (χ2 = 12.10, p = 0.01, Table 3). In contrast, the lagged resource level had no significant impact on effort when communication was not allowed (χ2 = 1.90, p = 0.59, Table 3).
The fact that changes in resource levels have permanent effects on effort levels only when communication is allowed constitute a novel result in the field of common pool resource experiments, indicating that as long as participants communicate among each other, the long-term relationship between resource and effort levels creates sustainable conditions.
Human behaviour is considered a key source of uncertainty in fisheries management because unexpected behaviours can lead to unintended management outcomes (Fulton et al., 2012). Over the past years, CPR experiments studying the impacts of different institutional arrangements on harvest behaviour have received a great deal of attention. However, besides a few exceptions (e.g., Janssen, 2010; Owusu et al., 2019), all these experiments are conducted in discrete time. Due to the dynamic nature of ecological resources and because the behaviour of users in continuous time is different from what is expected in discrete time (Bigoni et al., 2015), continuous time experiments are able to capture the coupled dynamics between resource levels and effort and should thus produce more realistic results.
By conducting continuous-time CPR experiments mimicking a fishery, we revealed a causal relationship between resource levels and effort under the communication treatment. The role of communication in promoting cooperation is well established (Balliet, 2010). Our results, however, add to this knowledge by showing that effort levels respond to dynamic changes in resource levels when communication is allowed. We suggest that the ubiquitous variation observed in cooperation (McClung et al., 2017) is not exclusively related to either the human sphere (e.g., harvest behaviour) or the ecological sphere (e.g., resource characteristics and variability) but is a result of the coupled dynamics of the user-resource system.
The relevance of our findings encompasses different aspects. First, in the specific context of common pool resource experiments, the outcome that resource levels are dynamically coupled to variability in effort substantiates the need to move away from the traditional approach of running experiments in discrete time. Second, the study also confirms that our open-software OGUMI is a useful tool for running common pool resource experiments in continuous time. OGUMI, moreover, can be further developed and expanded to include specific features of fisheries. For example, effort, which is now simply connected to the ammount of fish extracted from the common pool, can be related to effective costs associated to fuel consumption, crew, or licencing fees in order to run laboratory experiments under more realistic conditions. Also, the ecological model underpinning the common pool in OGUMI can be further developed to simulate local resource patchiness and multiple fish species or to focus on specific, high-value species (e.g., bluefin tuna) for which consumers are willing to pay high enough prices so that even if abundances decline fishing is still profitable. Third, although only a small piece in the much broader and more complex puzzle of fishery management, the mechanistic link we found, i.e., the fact that effort levels respond to resource levels under communication, can be used to constrain simulative tools, such as Agent-Based Models, that capture more realistically the complexities of fishery management. For example, our result can be used as a workbench to drive (or, else, as a reference for) Agent-Based Models in which communication is represented by sharing information (e.g., about the location or the abundance of the catch, see: Klein et al., 2017).
Being affected by the typical constraints of laboratory contexts, our study is, of course, limited by the specific assumptions and simplifications adopted. For example, although we used a group of participants with varying socio-demographic traits, these traits may not be truly representative of the diversity of behaviours and skills that can be found across populations of real fishermen. We also assumed perfect knowledge in resource levels, although uncertainties in the amount of available resources can play a critical role in real-life situations. As summarised by Fulton et al. (2012), uncertainties also involve fishermen behaviours, which vary in space and time and are thus context-specific. Our findings, however, reveal the possibility to predict future effort levels based on the dynamics of past resource levels depending on the specific institutional arrangement considered and, if confirmed by experiments with real fishermen and under uncertainties in resource levels, can contribute to the development of resource management strategies.
The datasets generated and analysed in this study can be found in the DRYAD open-access repository (https://doi.org/10.5061/dryad.ncjsxksv0).
Ethical review and approval was not required for the study on human participants in accordance with the local legislation and institutional requirements. Written informed consent for participation was not required for this study in accordance with the national legislation and the institutional requirements.
MMK, KAO, and AM conceived the ideas, designed the study, contributed to the interpretation of the results, and to the writing of the manuscript. KAO and MMK generated and analysed the data. All authors gave final approval for publication.
This research was funded by the German Academic Exchange Service, DAAD, through the projects HARVEST (grant no. 57142704) and COMPLEX (grant no. 57384894).
The authors declare that the research was conducted in the absence of any commercial or financial relationships that could be construed as a potential conflict of interest.
All claims expressed in this article are solely those of the authors and do not necessarily represent those of their affiliated organizations, or those of the publisher, the editors and the reviewers. Any product that may be evaluated in this article, or claim that may be made by its manufacturer, is not guaranteed or endorsed by the publisher.
We gratefully acknowledge the German Academic Exchange Service, DAAD, for financing this research through the projects HARVEST (grant number 57142704) and COMPLEX (grant number 57384894). We are also grateful to Moustapha M. Fall, the Alexander von Humboldt research chair at AIMS Senegal, for the support provided on various aspects of this work.
The Supplementary Material for this article can be found online at: https://www.frontiersin.org/articles/10.3389/fmars.2021.644056/full#supplementary-material
Bailey, R. M., Carrella, E., Axtell, R., Burgess, M. G., Cabral, R. B., Drexler, M., et al. (2019). A computational approach to managing coupled human–environmental systems: the POSEIDON model of ocean fisheries. Sustain. Sci. 14, 259–275. doi: 10.1007/s11625-018-0579-9
Balliet, D. (2010). Communication and cooperation in social dilemmas: A meta-analytic review. J. Conflict Resolut. 54, 39–57. doi: 10.1177/0022002709352443
Bigoni, M., Casari, M., Skrzypacz, A., and Spagnolo, G. (2015). Time horizon and cooperation in continuous time. Econometrica 83, 587–616. doi: 10.3982/ECTA11380
Brandt, G., Kulesz, M. M., Nissen, D., and Merico, A. (2017). OGUMI – A new mobile application to conduct common-pool resource experiments in continuous time. PLoS ONE 12:e0178951. doi: 10.1371/journal.pone.0178951
Bru, L., Cabrera, S., Capra, C. M., and Gomez, R. (2003). A common pool resource game with sequential decisions and experimental evidence. Exp. Econ. 6, 91–114. doi: 10.1023/A:1024209010570
Burgess, M. G., Carrella, E., Drexler, M., Axtell, R. L., Bailey, R. M., Watson, J. R., et al. (2020). Opportunities for agent-based modelling in human dimensions of fisheries. Fish Fish. 21, 570–587. doi: 10.1111/faf.12447
Butterworth, D. S., and Punt, A. E. (1999). Experiences in the evaluation and implementation of management procedures. ICES J. Mar. Sci. 56, 985–998. doi: 10.1006/jmsc.1999.0532
Chen, D. L., Schonger, M., and Wickens, C. (2016). oTree–An open-source platform for laboratory, online, and field experiments. J. Behav. Exp. Finance, 9, 88–97. doi: 10.1016/j.jbef.2015.12.001
Exadaktylos, F., Espín, A., and Braãs Garza, P. (2013). Experimental subjects are not different. Sci. Rep. 3:1213. doi: 10.1038/srep01213
Falk, A., and Heckman, J. J. (2009). Lab experiments are a major source of knowledge in the social sciences. Science 326, 535–538. doi: 10.1126/science.1168244
FAO (2018). The State of World Fisheries and Aquaculture 2018 - Meeting the sustainable development goals. Technical Report Food and Agriculture Organization of the United Nations Rome.
Fischbacher, U. (2007). z-Tree: Zurich toolbox for ready-made economic experiments. Exp. Econ. 10, 171–178. doi: 10.1007/s10683-006-9159-4
Fulton, E., Smith, A., Smith, D., and van Putten, I. (2012). Human behaviour: the key source of uncertainty in fisheries management. Fish Fish. 12, 2–17. doi: 10.1111/j.1467-2979.2010.00371.x
Goldstone, R. L., and Ashpole, B. C. (2004). Human foraging behavior in a virtual environment. Psychon. Bull. Rev. 11, 508–514. doi: 10.3758/BF03196603
Granger, C. W. J. (1969). Investigating causal relations by econometric models and cross spectral methods. Econometrica 37, 424–438. doi: 10.2307/1912791
Hardin, G. (1968). The tragedy of the commons. Science 162, 1243–1248. doi: 10.1126/science.162.3859.1243
Harrison, G. W., and List, J. A. (2004). Field experiments. J. Econ. Lit. 42, 1009–1055. doi: 10.1257/0022051043004577
Janssen, M. (2010). Introducing ecological dynamics into common-pool resource experiments. Ecol. Soc. 15, 7. doi: 10.5751/ES-03296-150207
Janssen, M. (2014). Experimental platforms for behavioral experiments on social-ecological systems. Ecol. Soc. 19, 20. doi: 10.5751/ES-06895-190420
Janssen, M., Anderies, J., and Cardenas, J. C. (2011). Head-enders as stationary bandits in asymmetric commons: Comparing irrigation experiments in the laboratory and the field. Ecol. Econ. 70, 1590–1598. doi: 10.1016/j.ecolecon.2011.01.006
Janssen, M. A., Holahan, R., Lee, A., and Ostrom, E. (2010). Lab experiments for the study of social-ecological systems. Science 328, 613–617. doi: 10.1126/science.1183532
Klein, E. S., Barbier, M. R., and Watson, J. R. (2017). The dual impact of ecology and management on social incentives in marine common-pool resource systems. R. Soc. Open Sci. 4:170740. doi: 10.1098/rsos.170740
Mantilla, C. (2015). Communication networks in common-pool resource games: Field experimental evidence. J. Econ. Behav. Organ. 118, 215–226. doi: 10.1016/j.jebo.2015.06.003
McClung, J. S., Placì, S., Bangerter, A., Clément, F., and Bshary, R. (2017). The language of cooperation: shared intentionality drives variation in helping as a function of group membership. Proc. R. Soc. B 284:20171682. doi: 10.1098/rspb.2017.1682
Oprea, R., Charness, G., and Friedman, D. (2014). Continuous time communication in a public-goods experiment. J. Econ. Behav. Organ. 108, 212–223. doi: 10.1016/j.jebo.2014.09.012
Oprea, R., Henwood, K., and Friedman, D. (2011). Separating the Hawks from the Doves: Evidence from continuous time laboratory games. J. Econ. Theory 146 2206–2225. doi: 10.1016/j.jet.2011.10.014
Ostrom, E. (2006). The value-added of laboratory experiments for the study of institutions and common-pool resources. J. Econ. Behav. Organ. 61, 149–163. doi: 10.1016/j.jebo.2005.02.008
Ostrom, E., Gardner, R., and Walker, J. (1994). Rules, Games, and Common-Pool Resources. Ann Arbor, MI: University of Michigan Press.
Owusu, K. A., Acevedo-Trejos, E., Fall, M. M., and Merico, A. (2020). Effects of cooperation and different characteristics of Marine Protected Areas in a simulated small-scale fishery. Ecol. Complexity 44, 1–13. doi: 10.1016/j.ecocom.2020.100876
Owusu, K. A., Kulesz, M. M., and Merico, A. (2019). Extraction behaviour and income inequalities resulting from a common pool resource exploitation. Sustainability 11, 536. doi: 10.3390/su11020536
Pauly, D., and Zeller, D. (2016). Catch reconstructions reveal that global marine fisheries catches are higher than reported and declining. Nat. Commun., 7:10244. doi: 10.1038/ncomms10244
Pettit, J., Friedman, D., Kephart, C., and Oprea, R. (2014). Software for continuous game experiments. Exp. Econ. 17, 631–648. doi: 10.1007/s10683-013-9387-3
Punt, A. E., Butterworth, D. S., de Moor, C. L., De Oliveira, J. A. A., and Haddon, M. (2016). Management strategy evaluation: best practices. Fish Fish. 17, 303–334. doi: 10.1111/faf.12104
Safarzynska, K. (2018). The impact of resource uncertainty and intergroup conflict on harvesting in the common-pool resource experiment. Environ. Res. Econ. 71, 1001–1025. doi: 10.1007/s10640-017-0193-9
Schaefer, M. B. (1991). Some aspects of the dynamics of populations important to the management of the commercial marine fisheries. Bull. Math. Biol. 53, 253–279. doi: 10.1016/S0092-8240(05)80049-7
Schill, C., Lindahl, T., and Crépin, A.-S. (2015). Collective action and the risk of ecosystem regime shifts: insights from a laboratory experiment. Ecol. Soc. 20, 48. doi: 10.5751/ES-07318-200148
Smith, A. D. M, Sainsbury, K. J, and Stevens, R. A. (1999). Implementing effective fisheries-management systems – management strategy evaluation and the Australian partnership approach. ICES J. Mar. Sci. 56, 967–979. doi: 10.1006/jmsc.1999.0540
Toda, H. Y., and Yamamoto, T. (1995). Statistical inference in vector autoregressions with possibly integrated processes. J. Econom. 66, 225–250. doi: 10.1016/0304-4076(94)01616-8
Keywords: common pool resource, strategic behaviour, institutional arrangements, tragedy of the commons, fishery, sustainability
Citation: Owusu KA, Kulesz MM and Merico A (2021) Communication Among Harvesters Leads to Sustainable Fishing Behaviour in a Continuous Time Common Pool Resource Experiment. Front. Mar. Sci. 8:644056. doi: 10.3389/fmars.2021.644056
Received: 19 December 2020; Accepted: 26 July 2021;
Published: 25 August 2021.
Edited by:
Catherine Sarah Longo, Marine Stewardship Council (MSC), United KingdomReviewed by:
Athanasios Kampas, Agricultural University of Athens, GreeceCopyright © 2021 Owusu, Kulesz and Merico. This is an open-access article distributed under the terms of the Creative Commons Attribution License (CC BY). The use, distribution or reproduction in other forums is permitted, provided the original author(s) and the copyright owner(s) are credited and that the original publication in this journal is cited, in accordance with accepted academic practice. No use, distribution or reproduction is permitted which does not comply with these terms.
*Correspondence: Agostino Merico, YWdvc3Rpbm8ubWVyaWNvQGxlaWJuaXotem10LmRl
†Present Address: Micaela M. Kulesz, Facultad de Ciencias Económicas, Universidad de Buenos Aires, Buenos Aires, Argentina
Disclaimer: All claims expressed in this article are solely those of the authors and do not necessarily represent those of their affiliated organizations, or those of the publisher, the editors and the reviewers. Any product that may be evaluated in this article or claim that may be made by its manufacturer is not guaranteed or endorsed by the publisher.
Research integrity at Frontiers
Learn more about the work of our research integrity team to safeguard the quality of each article we publish.