- 1Institut des Sciences de la Mer, Université du Québec à Rimouski, Rimouski, QC, Canada
- 2Québec-Océan, Université Laval, Québec, QC, Canada
- 3Takuvik Joint Université Laval/Centre National de la Recherche Scientifique Laboratory, Université Laval, Québec, QC, Canada
- 4Laboratoire Environnement Ressources Bretagne Nord, Institut Français pour la Recherche et l’Exploitation de la Mer, Dinard, France
- 5Institut Nordique de Recherche en Environnement et en Santé au Travail, Sept-Îles, QC, Canada
- 6Département de Biologie, Université Laval, Québec, QC, Canada
- 7Maurice Lamontagne Institute, Fisheries and Oceans Canada, Mont-Joli, QC, Canada
With the widespread influence of human activities on marine ecosystems, evaluation of ecological status provides valuable information for conservation initiatives and sustainable development. To this end, many environmental indicators have been developed worldwide and there is a growing need to evaluate their performance by calculating ecological status in a wide range of ecosystems at multiple spatial and temporal scales. This study calculated and contrasted sixteen indicators of ecological status from three methodological categories: abundance measures, diversity parameters and characteristic species. This selection was applied to coastal benthic ecosystems at Sept-Îles (Québec, Canada), an important industrial harbor area in the Gulf of St. Lawrence, and related to habitat parameters (organic matter, grain size fractions, and heavy metal concentrations). Nearly all indicators highlighted a generally good ecological status in the study area, where communities presented an unperturbed profile with high taxa and functional diversities and without the dominance of opportunistic taxa. Some correlations with habitat parameters were detected, especially with heavy metals, and bootstrap analyses indicated quite robust results. This study provides valuable information on the application of environmental indicators in Canadian coastal ecosystems, along with insights on their use for environmental assessments.
Introduction
Anthropogenic influences on marine ecosystems occur globally, with possible perturbation of habitats and communities (Halpern et al., 2007, 2019). Many international organizations have recognized the importance of biologically diverse ecosystems for humanity and have established objectives and targets for their protection and sustainable use (United Nations, 1992; Secretariat of the CBD, 2010; SDG, 2015). The management of ecosystems requires an understanding of how habitats and communities respond to drivers of change, i.e., forces that affect environmental processes and modify ecosystem state from equilibrium (Boonstra et al., 2015; Beauchesne et al., 2020; Orr et al., 2020). In addition to natural drivers (e.g., temperature anomalies, freshwater inputs, hypoxic events), influences from human activities (e.g., fisheries, chemical pollution, species introductions) are also considered as ecosystem drivers. As natural and anthropogenic drivers may affect ecosystems concomitantly, it is important to understand how both relate to observed effects (Brown et al., 2014). To tackle these questions, environmental assessments rely on the best available knowledge, acquired through ecological groundwork in ecosystems of interest (such as biodiversity surveys, time series monitoring or experimental studies), and on the communication of results to a wide range of stakeholders (Borja et al., 2012; Borja, 2014; Chapman, 2016; Teixeira et al., 2016). Because such assessments are important foundations for decision makers, it is essential to properly account for the inherent complexity and variability of ecological data.
The use of integrative methods, such as indicators, is particularly relevant in this context. An indicator of ecological status is defined as a quantitative measure that synthesizes ecosystem information to infer ecosystem status (Rice, 2003; Rees et al., 2008). Many holistic frameworks, such as ecosystem-based management, marine spatial planning and DPSIR (Driver Pressure State Impact Responses) models, have included indicators in their methodology (Smeets and Weterings, 1999; Niemi and McDonald, 2004; European Commission, 2008; Rees et al., 2008; Levin et al., 2009; Atkins et al., 2011; Borja et al., 2013, 2015, 2016; Santos et al., 2019). However, environmental indicators evaluate specific ecosystem components, perturbations and/or spatiotemporal scales, potentially limiting their applicability in other systems, thus leading to the development of many indicators worldwide (Niemi and McDonald, 2004; Pinto et al., 2009; Teixeira et al., 2016).
One of the ecosystem components most frequently selected for environmental indicators are macrobenthic invertebrates, as they play an important role in the structure and functioning of benthic marine ecosystems (Dauvin and Ruellet, 2007; Pratt et al., 2014). Examples of this include engineering species (e.g., structural features for other species, bioturbation) and interactions with nutrient cycles (e.g., nutrient sequestration in sediments, remineralization, benthic-pelagic coupling) (Largaespada et al., 2012; Link et al., 2013; Belley et al., 2016; Bourque and Demopoulos, 2018). Many macrobenthic species are characterized by a sedentary lifestyle and a relatively long life span, which is particularly interesting when studying human influence as communities will reflect medium-term conditions, resulting in adaptation or local extinction (e.g., Dauer, 1993; Borja et al., 2000; Wei et al., 2020).
As pointed out by Rice (2003) and Salas et al. (2006), environmental indicators may be classed into categories according to their methodological basis, including three main categories used in environmental assessments. Category 1 regroups indicators based on measures of abundance—such as density and biomass of individuals—to infer community status. Relationships between abundance and a community status have frequently been discussed, as species do not have the same tolerance to disturbance (Pearson and Rosenberg, 1978). As such, the use of abundance-biomass curves has been proposed to detect if communities are in a balanced state, where K-selected taxa are dominant, compared to a disturbed state, with a dominance of r-selected taxa (Pearson and Rosenberg, 1978; Gray, 1979; Warwick and Clarke, 1994). Category 2 indicators are biodiversity parameters, i.e., community characteristics such as taxa identity and prevalence, which allow complex information to be aggregated into a unique metric. Finally, indicators in Category 3 are computed based on variations of responses of taxa to disturbance. Pioneer works by Pearson and Rosenberg (1978) proposed a model of benthic community evolution along a gradient of organic enrichment, laying the path toward a set of indicators that relate community structure and ecological status.
Environmental indicators, such as the AZTI Marine Biotic Index or the Infaunal Trophic index, have been applied in a number of North American ecosystems, including Chesapeake Bay, Willapa Bay and the Southern California coast (United States), but efficiency to detect perturbation has been mixed (Word, 1978; Maurer et al., 1999; Ferraro and Cole, 2004; Borja et al., 2008b; Pelletier et al., 2018). Less commonly, studies on the Pacific and Atlantic coasts of Canada have also evaluated the utility of existing indicators, although these studies have most often found poor performance (Sutherland et al., 2007; Burd et al., 2008; Callier et al., 2008; Robert et al., 2013). There is thus a need to test and validate indicators for Canadian ecosystems, in particular by comparing outcomes and efficiency of existing methods, which will greatly benefit to tackle ecosystem management objectives within Canada’s Ocean Act and the Oceans Strategy (Government of Canada, 1996; Department of Fisheries and Oceans, 2002).
To this end, we evaluated various indicators of ecological status in a coastal industrial harbor area, where human activities may significantly impact local benthic ecosystems. Industrial harbor areas are regions regrouping significant industrial activities coupled with harbor platforms linking production with commercial shipping routes worldwide. We selected the region of Sept-Îles (Québec, Canada) for this study. Located in the Gulf of St. Lawrence, one of the management areas designated by Fisheries and Oceans Canada and a major strategic region for Québec (Department of Fisheries and Oceans, 2009; Daigle et al., 2017; Schloss et al., 2017; Ferrario and Archambault, in preparation), Sept-Îles is the fourth largest Canadian port in 2019 in terms of total exchanged goods and the second largest in Québec (Statistics Canada, 2011; Binkley, 2020). Industrial activities at Sept-Îles are largely focused on international shipping of iron ore mined in northern Québec and Labrador, the production of aluminum and various fisheries operate in the bay (Department of Fisheries and Oceans, 2019).
The objectives of this study are to (i) compare outcomes of various environmental indicators on benthic ecosystems of the Sept-Îles region and (ii) understand how these indicators relate to habitat parameters for validation and to select appropriate applications.
Materials and Methods
Study Area
We targeted ecosystems with a sandy-silty sediment in the industrial harbor area of Sept-Îles (Côte-Nord region of Québec, Canada), which considers ecosystems in the Baie des Sept Îles and the archipelago at its entrance (Figure 1A; Dreujou et al., 2018, 2020). Coasts are characterized by sandy beaches, tidal marshes and anthropogenic structures. Mean depth is 35 m in the bay and can reach up to 150 m in the archipelago (Dutil et al., 2012). It is influenced by freshwater inputs from multiple streams and strong tidal currents resulting in a mixed water column and an estuarine circulation (Shaw, 2019). Ecosystems in the Sept-Îles region are considered sub-Arctic due to the formation of ice on the shore in November/December and in the bay in January/February, along with an important freshwater run-off due to snowmelt in April (Demers et al., 2018).
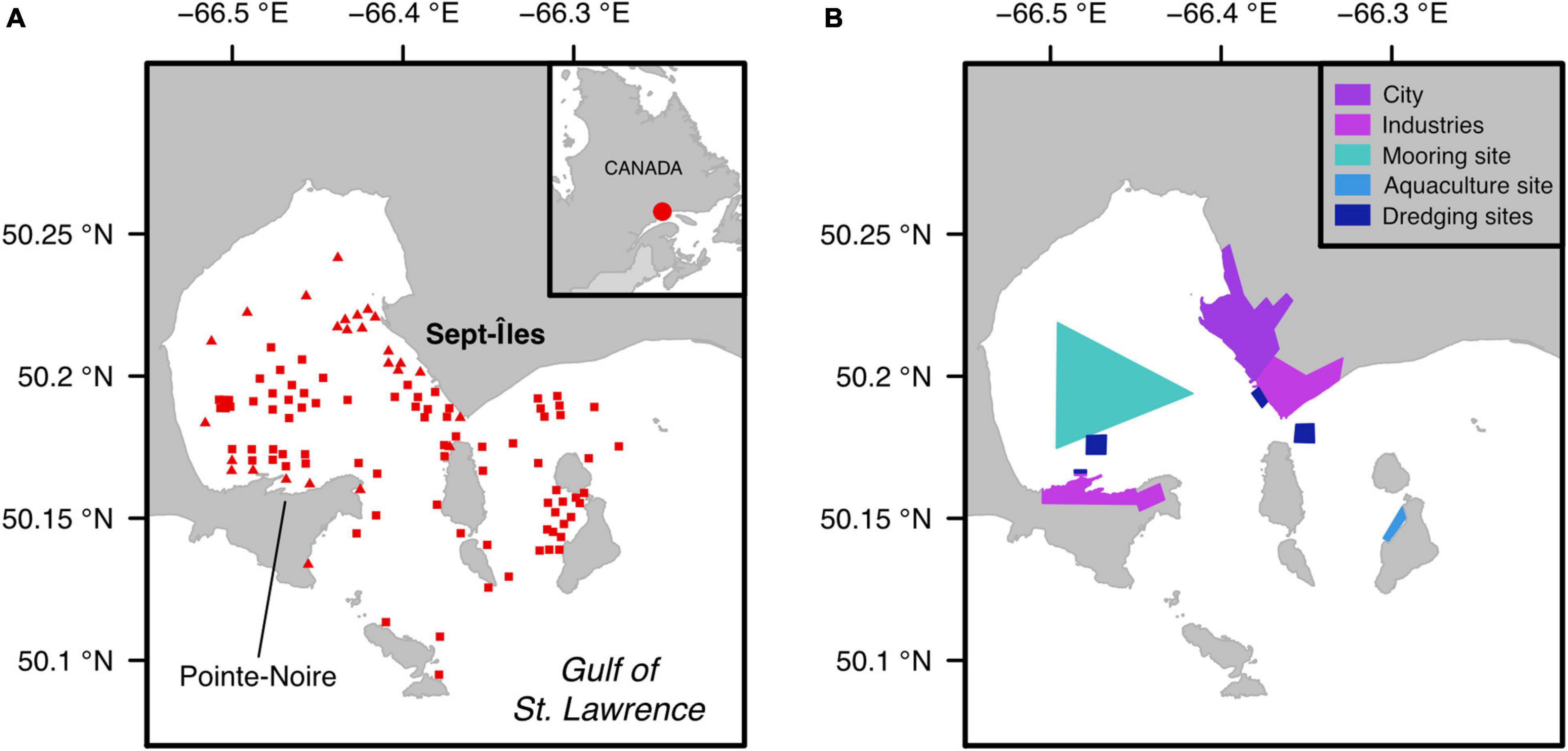
Figure 1. Map of the study area. (A) Location of the sampled stations, with light blue triangles and dark blue squares representing shallow (< 15 m) and deep (> 15 m) stations, respectively, (B) Location and identity of human activities present in the area.
This region hosts several human activities, including industrial, commercial and dredging operations located at the City of Sept-Îles and the Pointe-Noire sector (on the southern section of Baie des Sept Îles), along with an aquaculture site and various fisheries throughout the bay (Figure 1B). Many projects have been done in this region to characterize pelagic and benthic communities and habitats in relation to coastal stressors (Canadian Healthy Oceans Network, 2016; Carrière, 2018; Dreujou et al., 2020).
Benthic Ecosystems Sampling
The sampling design and methods used to collect and analyze ecological samples were similar to those presented in Dreujou et al. (2020), with the exception that only one region (Baie des Sept Îles) and one type of community (individuals higher than 0.5 mm) were considered.
A total of 108 stations were selected in the study area, using a randomization algorithm to cover the full extent of the sector, constrained between 0 and 80 m deep, and with increased sampling effort in areas with human activities (Figure 1A). Himmelman (1991) showed that benthic communities in the Northern Gulf of St. Lawrence above and below 15–20 m deep differ. Likewise, preliminary fieldwork in the study region detected a thermocline in the water column at ca. 15 m deep. Consequently, we discriminated two groups of stations in order to ensure habitat homogeneity within depth classes: shallow (<15 m, 26 stations) and deep habitats (>15 m, 82 stations). We sampled the benthic ecosystem in July 2017, using a Ponar grab (0.05 m2) deployed from a boat, with two independent casts at each station.
The first cast collected two subsamples—one for the analyses of organic matter content and another for sediment grain size—stored at -20°C until processing in the laboratory. The percentage of total organic matter (i.e., sum of organic carbon and organic nitrogen) in the sediment was determined using the Loss-on-Ignition method (Davies, 1974). Grain-size analysis was done on a sieving column for the fraction with particles larger than 2 mm and with a Laser Diffraction Particle Size Analyzer for the smaller fractions. Results from both techniques were combined to yield a unified size distribution range from 0.04 μm to 26.5 mm. From this, percentages of gravel, sand, silt and clay were calculated as defined by Wentworth (1922) and Folk (1980).
All sediment obtained from the second cast was sieved on a 0.5 mm mesh size and preserved in a solution of BORAX-buffered formalin (4%) solution for subsequent benthic macrofauna identification (Dreujou et al., 2020). The resulting samples were sorted using a stereomicroscope and taxa identified to the lowest taxonomic level possible with reference manuals and identification guides; names were validated according to the World Register of Marine Species (WoRMS Editorial Board, 2020). Taxon density and biomass per grab were recorded by counting and weighting (blotted wet mass) individuals in each sample, respectively.
In addition to these parameters, we considered estimates of heavy metal concentrations in the sediment. Concentrations at the sampled stations were calculated based on values obtained in the same area in 2014 and 2016, retrieved from a database hosted by Carrière (2018), using Inverse Distance Weighting interpolation (Dale and Fortin, 2014). We focused on metals for which toxicity criteria have been defined in the Biological Effects Database for Sediments (Environment Canada and Ministère du Développement Durable de l’environnement et des Parcs du Québec, 2007; Centre d’Expertise en Analyse Environnementale du Québec, 2014): arsenic, cadmium, chromium, copper, mercury, lead and zinc; we also included iron and manganese to account for possible contamination from local ore industries.
Environmental Indicator Calculation
Indicators of ecological status were selected from Pinto et al. (2009), DEVOTES (2012), and Teixeira et al. (2016), and grouped into three Categories according to their methodology (Table 1). We targeted indicators related to descriptors D1 (biological diversity), D6 (seafloor integrity), and D8 (contaminants) of Good Environmental Status (European Commission, 2008; Borja et al., 2013), choosing those that applied to benthic invertebrates in soft-bottom habitats. We considered each station separately, allowing an assessment of the spatial variability and mean for each indicator, and when possible we pooled all stations together to obtain an estimate for the bay-scale system. We used R v4.0 to perform data manipulations and calculations (R Core Team, 2020).
We included in Category 1 the total density (number of individuals collected per grab), total biomass (wet mass of individuals collected per grab), and the W-Statistic Index, calculated based on abundance-biomass curves for the community (Warwick and Clarke, 1994). Those indicators were computed using benthic taxa abundance sampled at each station.
For Category 2, we considered taxa richness (number of collected taxa) and related metrics to describe the community’s structure and the relative prevalence of taxa within it, such as the Shannon index, Margalef index, Simpson index, and Pielou evenness (Legendre and Legendre, 1998; Magurran and McGill, 2011). We also considered taxonomic and functional diversities, based on taxonomic relationships between taxa and information about biological traits, respectively (Warwick and Clarke, 1995; Clarke and Warwick, 1998; Mason et al., 2005; Villéger et al., 2008). Taxa richness, Shannon index, Margalef index, Simpson index, and Pielou evenness were calculated using the benthic community at each station. For taxonomic diversity, we gathered relatedness data for taxa using the WoRMS online database (WoRMS Editorial Board, 2020). To estimate functional diversity, we computed functional richness, functional evenness and functional divergence (Mason et al., 2005; Villéger et al., 2008) by considering five biological traits—body composition, body size, feeding type, mobility and lifestyle—with a total of 26 modalities (Table 2). Because taxa can present several modalities for a trait, we assigned a continuous value between 0 (absence of the modality) and 1 (presence of the modality) for each taxon and each trait (the sum of values for every modality within a trait equals 1). Biological trait data was extracted from WoRMS, SealifeBase, the Encyclopedia of Life, and Arctic Traits databases as well as dedicated articles (Degen and Faulwetter, 2019; EoL, 2020; Palomares and Pauly, 2020; WoRMS Editorial Board, 2020). R Packages vegan and FD were used to calculate indicators in this category (Laliberté and Legendre, 2010; Laliberté et al., 2014; Oksanen et al., 2019).
Finally, indicators in Category 3 included the AZTI Marine Biotic Index (AMBI) and its multivariate version (M-AMBI), which are based on the relative proportion of taxa classified into five ecological groups depending on their tolerance to perturbation (Grall and Glemarec, 1997; Borja et al., 2000; Muxika et al., 2007), BENTIX, where only two ecological groups are considered (Simboura and Zenetos, 2002), and the Benthic Opportunistic Polychaetes Amphipods Index (BOPA), which compares proportions of opportunistic polychaetes and amphipods (Dauvin and Ruellet, 2007). Sampled taxa were assigned to ecological groups, from group I to V, based on the list of Borja et al., version of May 2019 (AZTI, 2019; Supplementary Table S1). Because this list was developed for European taxa, we assigned groups to unregistered taxa based on species physiology studies and taxonomic relationships (Pelletier et al., 2018). We used this list to further regroup taxa to a “sensitive” (groups I and II) and a “tolerant” (groups III to V) metagroup to compute BENTIX (Simboura and Zenetos, 2002), and to obtain the proportion of opportunistic polychaetes (groups III to V) and sensitive amphipods (group I) to calculate BOPA (Dauvin and Ruellet, 2007; Supplementary Table S1). M-AMBI was calculated using the dedicated software AMBI v5.0 (AZTI, 2019), where “bad” and “high” status conditions are required for taxa richness, Shannon index and AMBI (Muxika et al., 2007). Because historical data on benthic invertebrates is scarce in our study area, we used the outcomes of our sampling to establish these values by selecting the 5 and 95 percentiles of the variable distribution (for “bad” and “high” status, respectively, Supplementary Table S2) (Buchet, 2010).
Integration and Statistical Analysis
Results for each indicator were reviewed qualitatively and compared to benthic ecosystem data in the Gulf of St. Lawrence, when available. Robustness for indicators in Categories 1 and 2 was calculated as the 95% confidence interval using a resampling routine (bootstrap, 1000 replicates), and the difference between averages of each indicator and the resampling averages (i.e., bootstrap bias).
We computed the Ecological Quality Ratios for Category 3 indicators. This ratio compares the value of an indicator to a reference, such as a targeted state or unperturbed/pristine ecosystem, so that an Ecological Quality Status can be assigned (five categories: “bad,” “low,” “moderate,” “good,” and “high” status). The formula to compute the Ecological Quality Ratio is the following (Bund and Solimini, 2007):
Vind is the value of an indicator, Rbad is the reference value for a “bad” status and Rhigh is the reference value for a “high” status. Limits between each Ecological Quality Status class are specific to the indicator used (Borja et al., 2000; Simboura and Zenetos, 2002; Muxika et al., 2005, 2007; Dauvin and Ruellet, 2007).
Finally, we explored covariation between indicators and habitat parameters (organic matter content, grain size distribution and heavy metal concentrations), using scatterplots for each pair of variables. Correlation was assessed with Spearman’s rank coefficients to understand the relevance of each indicator to the computation of ecological status (Quinn and Keough, 2002).
Results
Overview of Benthic Habitats and Communities
Sediment was mostly composed of sand and silt fractions, with concentrations of organic matter rarely surpassing 3% (Supplementary Table S3). Heavy metal concentrations did not reach high toxicity levels as defined by Environment Canada (Environment Canada and Ministère du Développement Durable de l’environnement et des Parcs du Québec, 2007; Centre d’Expertise en Analyse Environnementale du Québec, 2014; Dreujou et al., 2020; Supplementary Table S3). A total of 132 taxa were identified, belonging to eight phyla, with a dominance of arthropods, mollusks, and annelids (Supplementary Table S1). The most abundant taxa were the polychaete Micronephthys neotena, the cumacean Eudorellopsis integra, the amphipod Protomedeia grandimana, Nematoda (adults), and the bivalve Macoma calcarea (Supplementary Table S1). From this list, no species which can be considered as exotic to this region have been reported (Simard et al., 2013).
Indicator Outcomes
Category 1 Indicators
Indicators in this category presented greater mean values in deep than shallow stations, with the exception of total density (Table 3). Shallow stations showed a higher total density than deep stations, but this may be an outlier effect due to a single station close to the City of Sept-Îles (Supplementary Figures S1A–C), where density was 899 individuals.grab–1 with a dominance of P. grandimana. Overall, shallow and deep stations presented low total biomass, except for a couple of stations due to the presence of the echinoderms Echinarachnius parma and Strongylocentrotus sp. (Supplementary Figures S1A–C). The W-Statistic Index was positive and close to zero at nearly all shallow and deep stations (Supplementary Figures S1A–C) and the abundance-biomass curve presented higher abundance than biomass values when species were ranked (Figure 2).
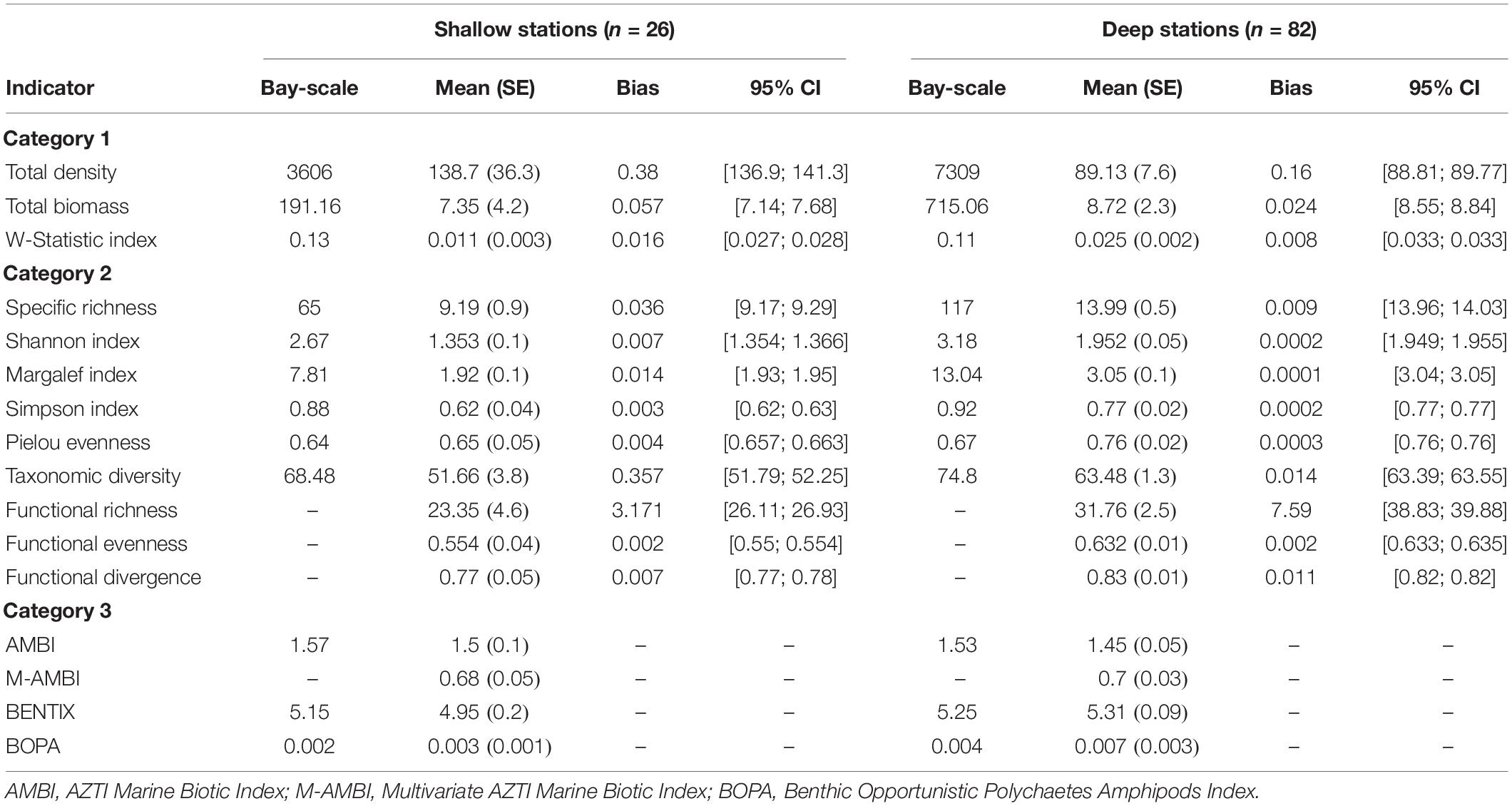
Table 3. Values of the mean and standard error (SE) for each indicator, the difference between bootstrapped mean and the true mean (bias) and the 95% confidence interval (CI), for shallow and deep stations.
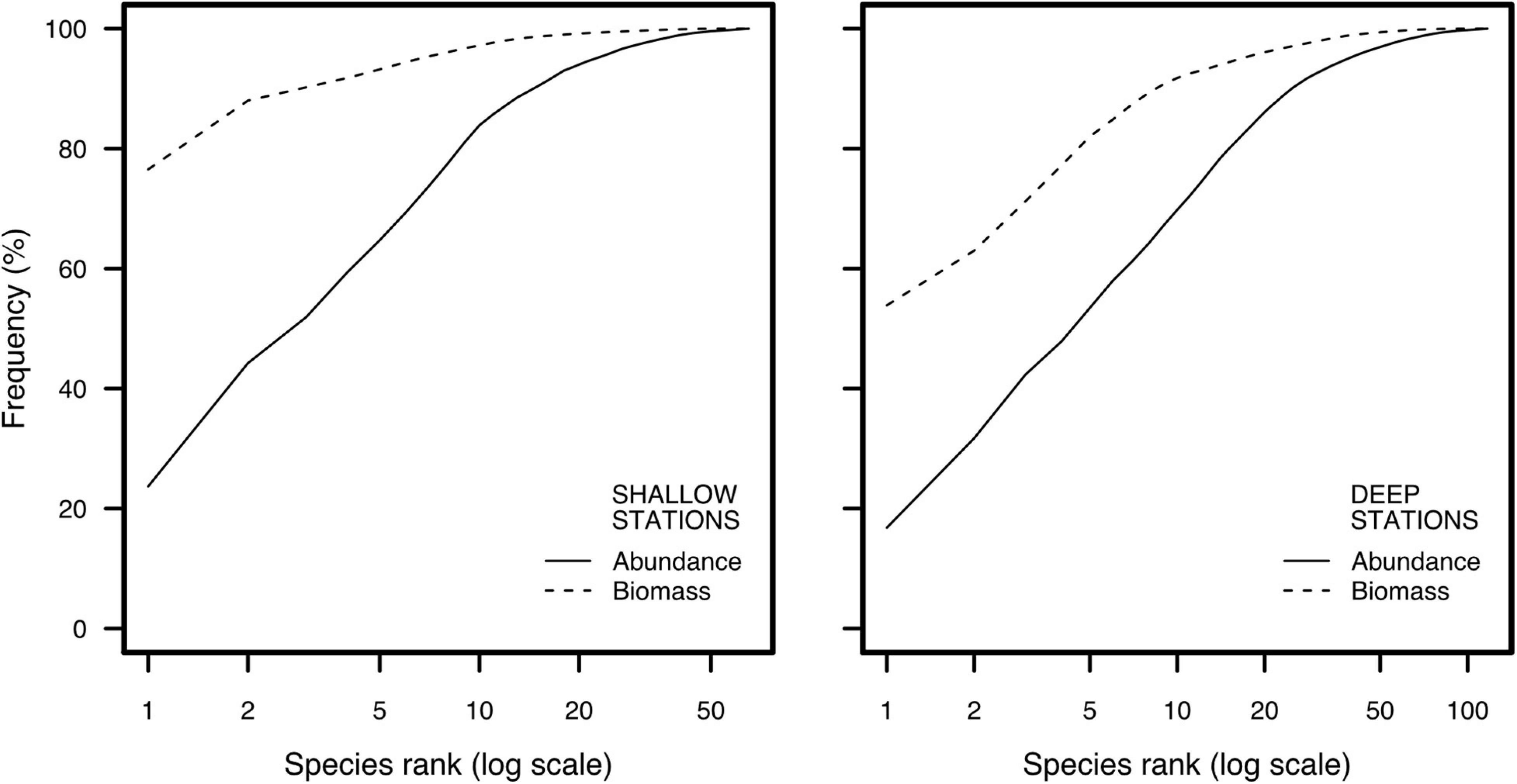
Figure 2. Values of abundance and biomass for ranked species (logarithm scale), for shallow stations and deep stations.
Category 2 Indicators
Category 2 indicators showed similar trends for shallow and deep stations, while being generally higher for the latter (Table 3). In particular, there is a close similarity between the spatial distributions of taxa richness, Shannon and Margalef indices and taxonomic diversity (Supplementary Figures S1D–L). Variability for shallow stations is quite low, except for a station in front of Pointe-Noire where only one taxon was present, while deep stations tend to display the highest values in the archipelago compared to the center of the bay (Supplementary Figures S1D–L). Mean values for the Simpson index and Pielou evenness reached 0.62 (standard error of 0.04) and 0.77 (0.02), respectively, for shallow stations and 0.66 (0.05) and 0.76 (0.02) for deep stations (Table 3). The same relationship between shallow and deep stations is observed for these metrics, even though the distribution for both is skewed with some stations closer to coasts presenting very low values (Supplementary Figures S1D–L). Concerning functional diversity, deep stations presented higher mean functional richness, functional evenness and functional divergence relative to those at shallow stations (Table 3). The most abundant modality for each biological trait was non-calcified tissue for body composition, small individuals for body size, surface deposit-feeders for feeding type, mobile organisms for mobility and burrowers for lifestyle, at both shallow and deep stations.
Category 3 Indicators
Classification of taxa into ecological groups to compute Category 3 indicators yielded 51 taxa in group I (sensitive to disturbance, 38.6% of the taxa), 63 in group II (indifferent to disturbance, 47.7%), 11 in group III (tolerant to disturbance, 8.3%), 1 in each of groups IV and V (second- and first-order opportunists, respectively, 0.8%) and 5 were not assigned due to a too broad taxonomic resolution (Supplementary Table S1). This classified 114 taxa in the “sensitive” group and 13 in the “tolerant” group (Supplementary Table S1). Concerning polychaetes and amphipods, we observed four opportunistic polychaetes (Cossura longocirrata, Eteone sp., Hediste diversicolor, Praxillella praetermissa) and nine sensitive amphipods (Ameroculodes edwardsi, Ampelisca vadorum, Byblis gaimardii, Lysianassidae, Maera danae, Phoxocephalus holbolli, Pontoporeia femorata, Quasimelita formosa, Quasimelita quadrispinosa).
An AMBI score of 1.57 and 1.53 was obtained for the bay-scale estimate at shallow and deep stations, respectively, which corresponds to a “slight imbalance” site classification (Borja et al., 2000). Overall, low AMBI values were obtained at each station, being 1.5 on average (standard error of 0.13) for shallow stations and 1.45 (0.05) for deep stations, and never exceeding 3, and no particular spatial trend can be observed (Table 3 and Supplementary Figures S1M–P). The bay-scale M-AMBI could not be computed with the percentile method, and at the station level, generally high mean values of 0.68 (0.05) and 0.7 (0.03) were observed for shallow and deep stations, respectively (Table 3). Stations outside of the bay tended to be characterized by higher values than those inside it, especially close to the coast and in the northern section of the bay, but this may be related to the spatial distribution of taxa richness and the Shannon index (Supplementary Figures S1M–P). The BENTIX bay-scale estimate was 5.15 for shallow stations and 5.25 for deep stations, while at the station-level mean values were 4.95 (0.23) and 5.31 (0.09), respectively (Table 3). These values correspond to a “normal/pristine” pollution classification for the majority of the area sampled, except for some stations close to coasts (Simboura and Zenetos, 2002). Finally, BOPA produced low scores of 0.002 and 0.004 for shallow and deep bay-scale estimates, respectively, similar to means of 0.0028 (0.0012) for shallow and 0.0067 (0.003) for deep stations, respectively (Table 3), denoting “high status” classifications. Only two stations had a score higher than 0.05, a trend that is not shared with neighboring stations, which may indicate localized low-intensity perturbations (Supplementary Figures S1M–P).
Calculation of Ecological Quality Ratios using Category 3 indicators produced similar results for AMBI, BENTIX and BOPA (Figure 3). The majority of stations (shallow and deep) presented a “high” or “good” ecological status except for a few stations with a “poor” status (Figure 3). In contrast, results for M-AMBI were less uniform, with a high variation among both shallow and deep stations, such that no general trends may be highlighted (Figure 3).
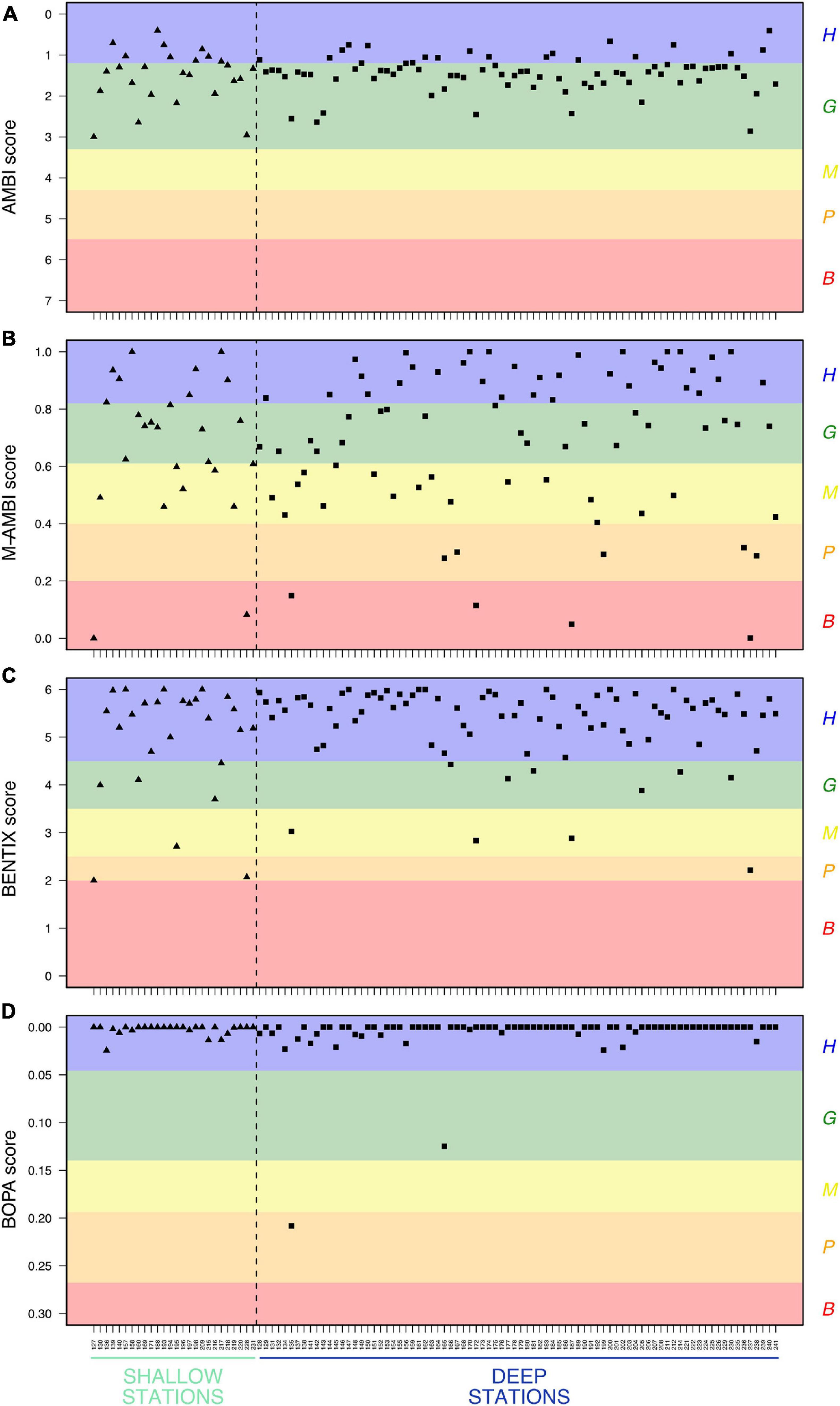
Figure 3. Values of Category 3 indicators ranked according to Ecological Quality Ratios, calculated for shallow and deep stations. (A) Calculated with the AZTI Marine Biotic Index (AMBI), (B) Calculated with the Multivariate AZTI Marine Biotic Index (M-AMBI), (C) Calculated with the BENTIX, (D) Calculated with the Benthic Opportunistic Polychaetes Amphipods Index (BOPA). B = “bad” status (red), P = “poor” status (orange), M = “moderate” status (yellow), G = “good” status (green), H = “high” status (blue).
Robustness and Covariation
For Category 1 and 2 indicators, bootstrap bias was low at both shallow and deep stations (less than 0.4), except for functional richness where it reached 3.17 and 7.59, respectively (Table 3), demonstrating a relatively high robustness of the indicators. The true mean was included in the 95% confidence interval for five indicators at shallow stations (taxa richness, total density, total biomass, functional evenness, functional divergence) and eight at deep stations (taxa richness, total density, total biomass, Shannon index, Margalef index, Simpson index, Pielou evenness, taxonomic diversity) (Table 3).
The analysis of covariation between indicators reported moderate to very high Spearman’s coefficients (0.22 < |ρ| < 0.96) (Table 4). Category 2 indicators presented the highest proportion of within-Category significant correlations at both shallow and deep stations (Table 4). The vast majority of these correlations were positive, with the strongest correlations between Shannon and Margalef indices, and were represented by linear proportionality between indicators on the scatterplots. Category 2 indicators were also frequently correlated to indicators from Categories 1 and 3, especially for the W-Statistic Index and the M-AMBI (Table 4). The latter Categories did not present high within-Category correlations, except between AMBI/BENTIX and M-AMBI/BOPA at shallow stations, and the W-Statistic Index and AMBI at deep stations.
Relationships With Habitat Parameters
Correlations between Category 1 indicators and abiotic parameters detected non-significant relationships with sediment parameters (except between the W-Statistic Index and gravel and sand contents at deep stations), while they were significant and negative between most heavy metals and total density and total biomass at shallow stations, and the W-Statistic Index at deep stations (Table 5). The absolute value of Spearman’s rank coefficients was high for total density and total biomass at shallow stations (between −0.4 and −0.61), highlighting relatively strong relationships, while they were less for the W-Statistic Index at deep stations (between −0.22 and −0.29).
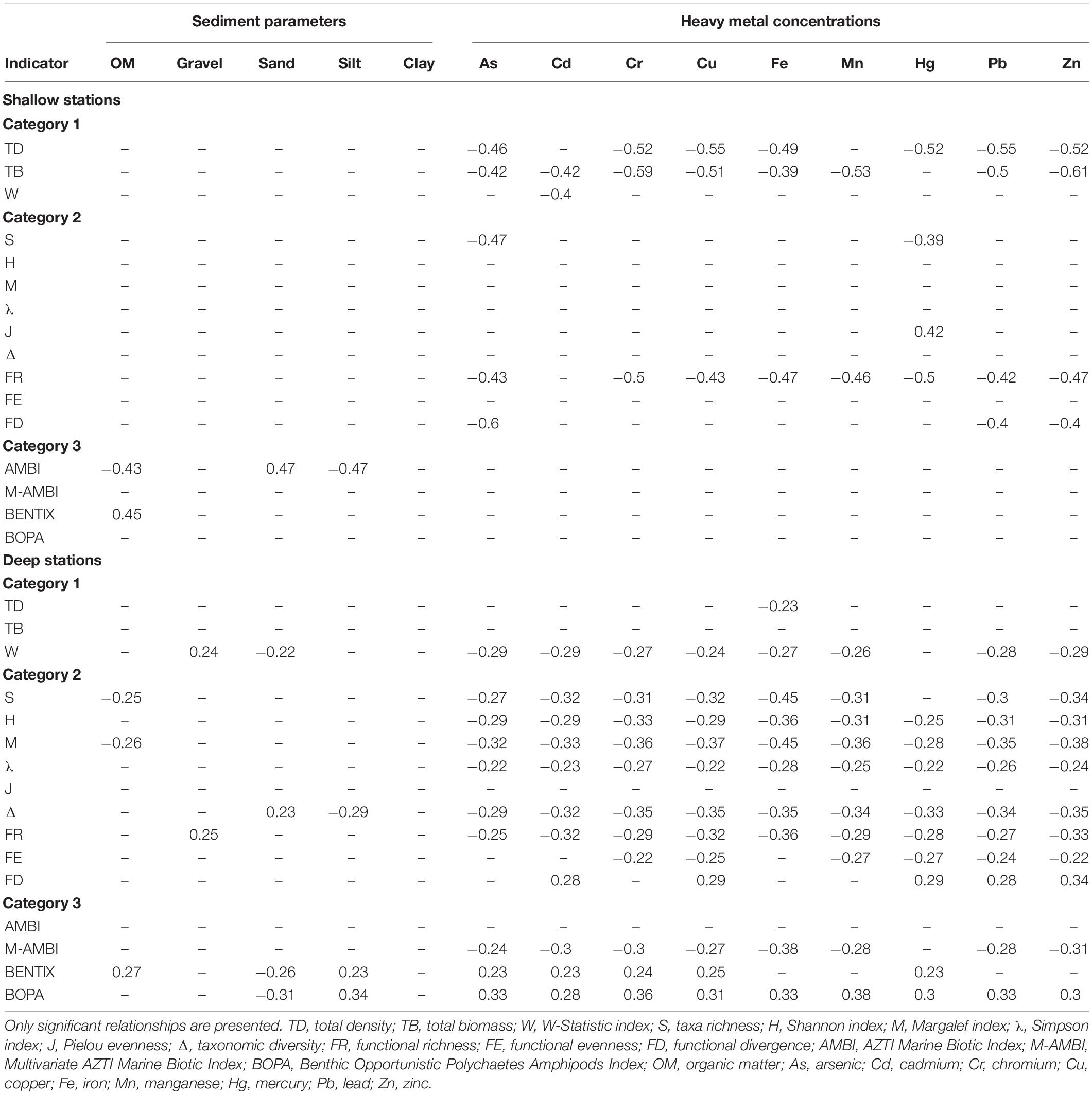
Table 5. Spearman rank correlation coefficients between environmental indicators and habitat parameters, for shallow and deep stations.
For Category 2 indicators, correlations with sediment parameters were significant only for some cases involving taxa richness, the Margalef index, taxonomic diversity and functional richness (Table 5). Relationships with heavy metals were detected mainly at deep stations, in particular for cadmium, copper, lead and zinc; at shallow stations, functional richness showed significant correlations with all heavy metals except cadmium, while functional divergence and taxa richness presented marginal correlations. The vast majority of these relationships were moderate to high (between −0.22 and −0.45), except at deep stations for gravel and sand contents and between functional divergence and some heavy metals.
Finally, several significant relationships were observed between Category 3 indicators and sediment parameters (organic matter, sand and silt contents), including at shallow stations for AMBI and BENTIX and at deep stations for BENTIX and BOPA (Table 5). Organic matter was negatively correlated with AMBI values (coefficient of −0.43) at shallow stations and positively with BENTIX values at shallow and deep stations (0.45 and 0.27, respectively); sand and silt contents had the opposite effect at shallow stations for AMBI (0.47 and −0.47, respectively) and at deep stations for BENTIX (−0.26 and 0.23, respectively) and BOPA (−0.31 and 0.34, respectively) values. Many relationships with heavy metals were detected at deep stations for all indicators except AMBI (Table 5). In particular, M-AMBI presented negative correlations with heavy metals (between −0.24 and −0.38), whereas correlations with BENTIX and BOPA were positive (between 0.23 and 0.36).
Discussion
Strengths, Limitations, and Ecological Considerations of Indicators
The analysis of benthic communities using Category 1 indicators relies on abundance relationships (either density or biomass of individuals) without consideration of taxonomic identity. Their calculation requires the least laboratory and analytical time relative to the other calculated indicators. Deep stations present a higher density of benthic organisms than shallow stations, as predicted by patterns of coastal marine biodiversity (Gray and Elliott, 2009; Levinton, 2013; Piacenza et al., 2015). The abundance-biomass curve for shallow and deep stations is characteristic of an unstressed profile (Pearson and Rosenberg, 1978; Warwick and Clarke, 1994), which is further supported by the W-Statistic Index being positive and close to 0 at nearly all stations (Clarke, 1990). Studying communities through abundance relationships thus provides interesting results concerning the status of the ecosystem, but the main assumption behind indicators in this Category is that all species are equivalent and have an identical role in the ecosystem structure and functioning. This, however is not necessarily true, as some species can be considered “key species” in ecosystems due to unique engineering or trophic roles (e.g., Bond, 1994; Lawton and Jones, 1995). Thus, Category 1 indicators should be coupled with ancillary methods focusing on biological characteristics of the species, such as life-history traits and physiological characteristics.
Category 2 indicators focus on community biodiversity, granting additional detail than that provided by Category 1 indicators. The notion of biodiversity can be interpreted along multiple points of view in an ecosystem, such as the diversity of species, genes, habitats or functions (United Nations, 1992; Wilson, 1992; Hooper et al., 2005; Stachowicz et al., 2007). While each targeted component has specific implications for the ecosystem, high richness and high diversity values have generally been interpreted as signs of good ecological status (Covich et al., 2004; Borja et al., 2013). This statement needs to be considered carefully, as it is necessary to discuss results with comparable ecosystems and historical data so that diversity trends are interpreted according to local background patterns (Covich et al., 2004).
Taxa richness indicated a quite diverse community, being nearly twice as great at deep stations, as expected given general trends (Gray and Elliott, 2009; Levinton, 2013; Piacenza et al., 2015). These numbers (132 taxa observed) are comparable to results from available benthic invertebrate surveys done in the study area, such as 27 taxa reported by OBIS (2020) in the Sept-Îles region and 148 taxa by Nozères et al. (2015) in the Gulf of St. Lawrence. Results from diversity indicators (Shannon index, Margalef index, Simpson index, Pielou evenness and taxonomic diversity) showed moderate to high benthic diversity, with no dominance by any taxa (even distribution) and great taxonomic breadth. Few stations differ from this general trend, and those that do are mostly close to coasts where diversity is low and there is no clear evidence of perturbation. Diversity indicators are frequently used in ecological studies to characterize communities and to detect disturbance, which allows discussing their results building on a vast corpus of studies worldwide (Magurran and McGill, 2011). These univariate estimates, however, may mask individual responses arising for example from adaptation or changes in biotic relationships, and they should be coupled with multivariate methods, such as ordination or similarity analysis, so that community-level effects are accurately described (Legendre and Legendre, 1998; Quinn and Keough, 2002; Magurran and McGill, 2011).
Concerning functional diversity, functional richness is generally lower at shallow stations and the two other indicators are in the same range, albeit being slightly greater at deep stations. These results suggest that taxa at shallow stations have more specialized niches, i.e., less diverse functional strategies (Villéger et al., 2008), indicating some redundancy of biological traits. This property is linked to an increased ecosystem stability and resilience, where possible extinctions due to perturbation will not modify the ecosystem structure even if some taxa disappear (Rosenfeld, 2002; Mouillot et al., 2013). However, bootstrap bias was very high for this indicator, making conclusions less robust. Moderate to high functional evenness and divergence denote that values for given biological traits are not evenly distributed and are skewed toward extremes (0 or 1). The consideration of biological traits in addition to species identity allows to study functions of the ecosystem, such as productivity, chemical elements cycling or energy transfers (Somerfield, 2008; Bellwood et al., 2019). This is an important addition to environmental assessments, as biological traits and adaptative responses to disturbance are highly related (Mouillot et al., 2013; Miatta et al., 2021). Indicators of functional diversity thus offer valuable information to characterize communities in complement to other Category 2 indicators, at the expense of increased analytical time to assemble a traits database, for which information may be lacking or difficult to obtain.
For Category 3 indicators, community ecological status is assessed by considering the tolerance of taxa to perturbation. Values for these indicators highlight an overall high status in the study area, where taxa sensitive to perturbation are present without a dominance of opportunists, as illustrated by the number of stations with a “high” Ecological Quality Status. M-AMBI detected greater variability between stations relative to the other indicators, particularly within the bay. A possible interpretation for this result may be the influence of the percentile method used to compute “bad” and “high” status conditions for this indicator, advocating for a careful description of comparison conditions and a fortiori references values (Borja et al., 2012). Furthermore, classification into ecological groups may introduce bias, as the list of Borja et al. (2000) were primarily designed for European coastal ecosystems. Inclusion of taxa found on Canadian coasts has been made based on taxonomic similarity with species already included in the list, by reviewing studies on perturbation tolerance and by expert opinion, but these choices need to be ground-truthed by dedicated ecological works. A wide spectrum of indicators may be included within Category 3, as compiled by Pinto et al. (2009) and Teixeira et al. (2016), and their use in environmental assessments is linked to scientific and management objectives. Such indicators have been used with success in a variety of ecosystems (e.g., Borja et al., 2008a; Gillett et al., 2015), but they require a high volume of data to accurately relate taxa and perturbation status, such as field observations, modeling of species distributions (native and non-indigenous), physiological studies and experimental work.
With these results, we can compare strengths and limitations of the calculated indicators. Category 1 indicators gather relevant baseline information on the ecosystem and requires the least time to be computed. The downside is that it is difficult to discriminate between anthropogenic perturbation and natural variability as other community characteristics may be impacted and, most importantly, they cannot be compared to reference conditions of ecological status. Category 2 indicators, such as the commonly used Shannon index or Pielou evenness, are easy to compute from well-built taxa lists, although taxonomic and functional diversity demand more time to gather complementary information about phylogenetic relationships and ecosystem functions. However, the latter indicators provide more information on the community structure and are backed by ecological literature to infer a certain ecological status (Magurran and McGill, 2011). Finally, Category 3 indicators demand the most time to be calculated, in particular with the classification of taxa relative to their response to disturbance, but they have been specifically designed to determine Ecological Quality Ratios and to consider reference conditions. Bias or uncertainty may be introduced during the classification process as extensive experimental groundwork is needed to properly assign taxa to groups, which is not always available. Many of these indicators are region-specific, with possible poor performance in other ecosystems (e.g., Callier et al., 2008; Robert et al., 2013), so that further research is needed to properly assess ecological status in sub-Arctic regions.
Implications for Sept-Îles and Canadian Ecosystems
Sept-Îles is an important industrial harbor area for Québec, with a variety of economic activities taking place in the bay and archipelago. All calculated indicators except M-AMBI pointed toward diverse benthic communities of generally good ecological status and no particular perturbation patterns have been detected in the study area, which is coherent with previous descriptions of benthic ecosystems in this region (Carrière, 2018; Dreujou et al., 2020). When applying Category 3 indicators on the data from these studies, we obtained similar conclusions with ecological status indicated as “good” to “high” for AMBI, BENTIX and BOPA.
No particular trend has been observed for stations identified as potentially impacted by Dreujou et al. (2020) in coastal regions close to the City of Sept-Îles and Pointe-Noire, further suggesting an overall limited effect of perturbations. Compared to the regions where many of the assayed indicators were developed, e.g., in Atlantic and Mediterranean European ecosystems, the magnitude of human activity is considerably lower at Sept-Îles. As such, it is possible the range of variation induced by anthropogenic perturbation is not sufficient to severely impact benthic ecosystems. This is even more relevant when we compare these results to other industrial harbor areas worldwide, where human influence is more pronounced (e.g., Hewitt et al., 2005; Borja et al., 2006; Chan et al., 2016; Birch et al., 2020). Other hypotheses may explain this, such as (i) high community resilience and resistance, (ii) limitation of effective impacts of activities by the dynamic of the ecosystem (e.g., flushing from tidal currents), and (iii) perturbation effects may be more pronounced on other components (such as phytoplankton or pelagic species).
Ecological indicators represent a valuable method to set conservation targets and to guide sustainable environmental projects. In light of initiatives such as the Marine Strategy Framework Directive, where indicators and descriptors have been identified to monitor the ecological status of European marine waters (European Commission, 2008; Borja et al., 2013, 2015, 2016), local stakeholders have the possibility to build on these works to establish ecosystem-based management adapted to Canadian ecosystems. Further research in other industrial coastal areas, including long-term monitoring, is needed to obtained coherent and robust environmental assessments.
Validation and Limitations
Assessing relationships between indicators highlighted correlations, especially among Category 2 indicators. While this does not necessarily imply causality in the interpretations, covariation indicates that information gathered by some indicators is similar. This was expected for indicators relying on specific ecosystem components to be computed, such as M-AMBI and the Shannon index, both of which being a function of taxa richness. With an environmental assessment perspective, these results show that calculation of some indicators will not provide additional information when the objective is to detect trends in a targeted area. Understanding these links will allow refining methodological protocols and to produce more efficient and accurate assessments.
The use of ecological status indicators requires a validation procedure to ensure that outcomes are relevant (Dauvin et al., 2010; Heink et al., 2016; Burgass et al., 2017; Moriarty et al., 2018). Because the region of Sept-Îles is not frequently represented in the scientific literature, it is then difficult to have baseline data to validate ecosystem assessments. The studies of Dreujou et al. (2018, 2020) represent the only campaigns describing benthic habitats and communities in the Baie des Sept Îles, and increased sampling would greatly improve indicator validation, in particular for Category 1 and 2 indicators where references conditions are currently not available. Inference of ecological status based on Category 3 indicators is relying on explicit reference conditions for “bad” and “high” status. Defining values for these conditions based on contemporary ecosystems will most certainly introduce bias, as most are likely to show some level of degradation (which cannot be assessed), and alternatives, such as historical datasets, are rare (Muxika et al., 2007; Borja et al., 2012).
Overall, Category 1 and 2 indicators were relatively robust, with little difference between mean values calculated from the real and bootstrapped datasets (except for functional richness), indicating quite homogeneous results. The vast majority of significant correlations between indicators and environmental factors were found for heavy metal concentrations and most such correlations were negative. This implies that indicators would successfully detect perturbation due to heavy metal content, thus resulting in reduced ecological status, but fail to detect perturbations affecting other habitat parameters. AMBI and BENTIX were correlated to organic matter content, which was expected as original works of Borja et al. (2000) and Simboura and Zenetos (2002) were based on models predicting community changes in response to an organic enrichment (Pearson and Rosenberg, 1978; Grall and Glemarec, 1997). Concerning grain size variables, only sand and silt contents showed any significant correlations with indicators, mainly at deep stations, which may be due to very low amounts of gravel and clay in the sediments.
It is important to note that indicators summarize complex ecological data into unique values (univariate in a statistical point of view), which may be insufficient to correctly assess perturbation. Category 3 indicators were developed for specific types of disturbance, such as organic matter loading or oil-spill detection (Pearson and Rosenberg, 1978; Borja et al., 2000; Dauvin and Ruellet, 2007), and for specific ecosystems (e.g., European Commission, 2008). Even though we detected significant relationships with heavy metal concentrations, dedicated methods to monitor these types of perturbation would greatly benefit this portrait. Finally, the consideration of cumulative impacts from various sources of disturbance may be a good perspective for environmental assessments (Crain et al., 2008), in order to develop more holistic indicators.
Conclusion
This study provides insight on the use of environmental indicators in Canadian coastal ecosystems, in particular by applying and comparing indicators within an important industrial harbor area. An overall good status for benthic ecosystems was detected in our study area, which is a valuable addition to guide stakeholders and ecosystem management at the local scale. We were able to present strengths and caveats for each Categories of indicators, relating them to ecological implications and possible improvements. Finally, we were able to study the robustness of indicators and we highlighted improvements for ground-throughing and validation.
Further environmental assessments in sub-Arctic coastal areas in the Gulf of St. Lawrence and the Canadian Eastern Atlantic coast are needed to obtain a broader portrait of the region with a more diverse range of environmental conditions. Long-term monitoring will also produce reliable time series data to better understand variability of sub-Arctic benthic ecosystems.
Data Availability Statement
The datasets generated for this study can be found in the online repositories. The names of the repository/repositories and accession number(s) can be found below: https://dataverse.scholarsportal.info/dataverse/chone2, doi: 10.5683/SP2/WDDDMI.
Author Contributions
ED, JC, CM, and PA contributed to the conception and design of the study. ED gathered the data in the field. ED, ND, and LT organized the database. ED and ND performed the statistical analysis. ED wrote the first draft of the manuscript. All authors contributed to manuscript revision, read and approved the submitted version.
Funding
This research was sponsored by the NSERC Canadian Healthy Oceans Network and its partners: Fisheries and Oceans Canada and INREST (representing the Port of Sept-Îles and City of Sept-Îles) to PA (grant number: NETGP 468437-14), by the FRQNT Strategic Network Québec-Océan and by the Joint UL/CNRS Laboratory Takuvik.
Conflict of Interest
The authors declare that the research was conducted in the absence of any commercial or financial relationships that could be construed as a potential conflict of interest.
Acknowledgments
We would like to acknowledge and thank all the people that helped during the field campaigns, lab work, and data analysis: Claudy Dechêsne, Serge Gallienne, Dany Lévesque, Aurélie Foveau, Cindy Grant, Laure de Montety, Philippe-Olivier Dumais, and Raphaël Bouchard. This study is a contribution to the research program of FRQNT Strategic Network Québec-Océan, which also provided scientific support.
Supplementary Material
The Supplementary Material for this article can be found online at: https://www.frontiersin.org/articles/10.3389/fmars.2021.637546/full#supplementary-material
References
Atkins, J. P., Gregory, A. J., Burdon, D., and Elliott, M. (2011). Managing the marine environment: Is the DPSIR framework holistic enough? Syst. Res. Behav. Sci. 28, 497–508. doi: 10.1002/sres.1111
AZTI (2019). AZTI Marine Biotic Index Software. Available online at: https://ambi.azti.es/ (accessed February 26, 2021).
Beauchesne, D., Daigle, R. M., Vissault, S., Gravel, D., Bastien, A., Bélanger, S., et al. (2020). Characterizing exposure to and sharing knowledge of drivers of environmental change in the St. Lawrence system in Canada. Front. Mar. Sci. 7:383. doi: 10.3389/fmars.2020.00383
Belley, R., Snelgrove, P. V. R., Archambault, P., and Juniper, S. K. (2016). Environmental drivers of benthic flux variation and ecosystem functioning in Salish Sea and Northeast Pacific Sediments. PLoS One 11:e151110. doi: 10.1371/journal.pone.0151110
Bellwood, D. R., Streit, R. P., Brandl, S. J., and Tebbett, S. B. (2019). The meaning of the term ‘function’ in ecology: a coral reef perspective. Funct. Ecol. 33, 948–961. doi: 10.1111/1365-2435.13265
Binkley, A. (2020). Where is the federal port modernization review headed? Canad. Sail. 12–16. Available online at: https://www.canadiansailings.ca/documents/weekly_issue/feb_2020/feb_24_2020.pdf (accessed February 26, 2021).
Birch, G. F., Lee, J. H., Tanner, E., Fortune, J., Munksgaard, N., Whitehead, J., et al. (2020). Sediment metal enrichment and ecological risk assessment of ten ports and estuaries in the World Harbours Project. Mar. Pollut. Bull. 155:111129. doi: 10.1016/j.marpolbul.2020.111129
Bond, W. J. (1994). “Keystone species,” in Biodiversity and Ecosystem Function, eds E.-D. Schulze and H. A. Mooney (Berlin: Springer), 237–253. doi: 10.1007/978-3-642-58001-7_11
Boonstra, W. J., Ottosen, K. M., Ferreira, A. S. A., Richter, A., Rogers, L. A., Pedersen, M. W., et al. (2015). What are the major global threats and impacts in marine environments? Investigating the contours of a shared perception among marine scientists from the bottom-up. Mar. Policy 60, 197–201. doi: 10.1016/j.marpol.2015.06.007
Borja, Á, Bricker, S. B., Dauer, D. M., Demetriades, N. T., Ferreira, J. G., Forbes, A. T., et al. (2008a). Overview of integrative tools and methods in assessing ecological integrity in estuarine and coastal systems worldwide. Mar. Pollut. Bull. 56, 1519–1537. doi: 10.1016/j.marpolbul.2008.07.005
Borja, Á, Dauer, D. M., and Grémare, A. (2012). The importance of setting targets and reference conditions in assessing marine ecosystem quality. Ecol. Indicat. 12, 1–7. doi: 10.1016/j.ecolind.2011.06.018
Borja, Á, Muxika, I., and Franco, J. (2006). Long-term recovery of soft-bottom benthos following urban and industrial sewage treatment in the Nervión estuary (southern Bay of Biscay). Mar. Ecol. Progr. Ser. 313, 43–55. doi: 10.3354/meps313043
Borja, A. (2014). Grand challenges in marine ecosystems ecology. Front. Mar. Sci. 11:1. doi: 10.3389/fmars.2014.00001
Borja, A., Elliott, M., Andersen, J. H., Cardoso, A. C., Carstensen, J., Ferreira, J. G., et al. (2013). Good Environmental Status of marine ecosystems: what is it and how do we know when we have attained it? Mar. Pollut. Bull. 76, 16–27. doi: 10.1016/j.marpolbul.2013.08.042
Borja, A., Elliott, M., Andersen, J. H., Cardoso, A., Carstensen, J., Ferreira, J., et al. (2015). Report on Potential Definition of Good Environmental Status Deliverable 6.2, DEVOTES Project. 62.
Borja, A., Elliott, M., Snelgrove, P. V. R., Austen, M. C., Berg, T., Cochrane, S., et al. (2016). Bridging the gap between policy and science in assessing the health status of marine ecosystems. Front. Mar. Sci. 3:175. doi: 10.3389/fmars.2016.00175
Borja, A., Franco, J., and Pérez, V. (2000). A marine Biotic Index to establish the ecological quality of soft-bottom benthos within European estuarine and coastal environments. Mar. Pollut. Bull. 40, 1100–1114. doi: 10.1016/S0025-326X(00)00061-8
Borja, Á., Dauer, D. M., Díaz, R., Llansó, R. J., Muxika, I., Rodríguez, J. G., et al. (2008b). Assessing estuarine benthic quality conditions in Chesapeake Bay: a comparison of three indices. Ecol. Indicat. 8, 395–403. doi: 10.1016/j.ecolind.2007.05.003
Bourque, J. R., and Demopoulos, A. W. J. (2018). The influence of different deep-sea coral habitats on sediment macrofaunal community structure and function. PeerJ 2018:5276. doi: 10.7717/peerj.5276
Brown, C. J., Saunders, M. I., Possingham, H. P., and Richardson, A. J. (2014). Interactions between global and local stressors of ecosystems determine management effectiveness in cumulative impact mapping. Divers. Distribut. 20, 538–546. doi: 10.1111/ddi.12159
Buchet, R. (2010). Directive Cadre sur l’Eau: Consolidation des Conditions de Référence Pour les L’évaluation des Masses d’eau Biologiques Impliqués Dans Éléments de Qualité Littorales. Brest: Institut Français pour la Recherche et l’Exploitation de la Mer.
Bund, W. V. D., and Solimini, A. G. (2007). Ecological Quality Ratios for Ecological Quality Assessment in Inland and Marine Waters. Luxembourg: European Commission Joint Research Centre, Institute for Environment.
Burd, B. J., Barnes, P. A. G., Wright, C. A., and Thomson, R. E. (2008). A review of subtidal benthic habitats and invertebrate biota of the Strait of Georgia, British Columbia. Mar. Environ. Res. 66:4. doi: 10.1016/j.marenvres.2008.09.004
Burgass, M. J., Halpern, B. S., Nicholson, E., and Milner-Gulland, E. J. (2017). Navigating uncertainty in environmental composite indicators. Ecol. Indicat. 75, 268–278. doi: 10.1016/j.ecolind.2016.12.034
Callier, M. D., McKindsey, C. W., and Desrosiers, G. (2008). Evaluation of indicators used to detect mussel farm influence on the benthos: two case studies in the Magdalen Islands, Eastern Canada. Aquaculture 278, 77–88. doi: 10.1016/j.aquaculture.2008.03.026
Canadian Healthy Oceans Network (2016). To Evaluate and Model How Natural and Anthropogenic Stressors Interact to Imapct Pelagic and Benthic Communities Along a Sub-Arctic Coastline and Develop Bay-Scale Condition Indicators. Available online at: https://chone2.ca/find-research/coastal-stressors/ (accessed February 26, 2021).
Carrière, J. (ed.) (2018). Observatoire de la Baie de Sept Îles. Sept-Îles, QC: Institut Nordique de Recherche en Environnement et en Santé au Travail.
Centre d’Expertise en Analyse Environnementale du Québec (2014). Détermination des Métaux : Méthode par Spectrométrie de Masse à Source Ionisante au Plasma D’argon, Vol. 9. Quebec, QC: Ministère du Développement Durable de I’environnement et de la Lutte contre les Changements Climatiques du Québec.
Chan, A. K. Y., Xu, W. Z., Liu, X. S., Cheung, S. G., and Shin, P. K. S. (2016). Sediment characteristics and benthic ecological status in contrasting marine environments of subtropical Hong Kong. Mar. Pollut. Bull. 103, 360–370. doi: 10.1016/j.marpolbul.2015.12.032
Chapman, P. M. (2016). Assessing and managing stressors in a changing marine environment. Mar. Pollut. Bull. 124, 587–590. doi: 10.1016/j.marpolbul.2016.10.039
Clarke, K. R. (1990). Comparisons of dominance curves. J. Exp. Mar. Biol. Ecol. 138, 143–157. doi: 10.1016/0022-0981(90)90181-B
Clarke, K. R., and Warwick, R. M. (1998). A taxonomic distinctness index and its statistical properties. J. Appl. Ecol. 35, 523–531. doi: 10.1046/j.1365-2664.1998.3540523.x
Covich, A. P., Austen, M. C., Bärlocher, F., Chauvet, E., Cardinale, B. J., Biles, C. L., et al. (2004). The role of biodiversity in the functioning of freshwater and marine benthic ecosystems. BioScience 54, 767–775.
Crain, C. M., Kroeker, K., and Halpern, B. S. (2008). Interactive and cumulative effects of multiple human stressors in marine systems. Ecol. Lett. 11, 1304–1315. doi: 10.1111/j.1461-0248.2008.01253.x
Daigle, R. M., Archambault, P., Halpern, B. S., Lowndes, J. S. S., and Côté, I. M. (2017). Incorporating public priorities in the Ocean Health Index: Canada as a case study. PLoS One 12:e0178044. doi: 10.1371/journal.pone.0178044
Dale, M. R. T., and Fortin, M.-J. (2014). Spatial Analysis: A Guide for Ecologists, 2nd Edn. Cambridge: Cambridge University Press. doi: 10.1017/CBO9780511978913
Dauer, D. M. (1993). Biological criteria, environmental health and estuarine macrobenthic community structure. Mar. Pollut. Bull. 26, 249–257. doi: 10.1016/0025-326X(93)90063-P
Dauvin, J. C., and Ruellet, T. (2007). Polychaete/amphipod ratio revisited. Mar. Pollut. Bull. 55, 215–224. doi: 10.1016/j.marpolbul.2006.08.045
Dauvin, J. C., Bellan, G., and Bellan-Santini, D. (2010). Benthic indicators: From subjectivity to objectivity – Where is the line? Mar. Pollut. Bull. 60, 947–953. doi: 10.1016/j.marpolbul.2010.03.028
Davies, B. E. (1974). Loss-on-ignition as an estimate of soil organic matter. Soil Sci. Soc. Am. J. 38:150. doi: 10.2136/sssaj1974.03615995003800010046x
Degen, R., and Faulwetter, S. (2019). The arctic traits database – a repository of arctic benthic invertebrate traits. Earth Syst. Sci. Data 11, 301–322. doi: 10.5194/essd-11-301-2019
Demers, K. A., Le Hénaff, A., and Carrière, J. (2018). “État des glaces,” in Observatoire Environnemental de la Baie de Sept-Îles, ed. J. Carrière (Sept-Îles, QC: INREST), 593–612.
Department of Fisheries and Oceans (2002). Canada’s Oceans Strategy: Our Oceans, Our Future. Ottawa, QC: Fisheries and Ocean Canada.
Department of Fisheries and Oceans (2009). Development of a Framework and Principles for the Biogeographic Classification of Canadian Marine Areas. Ottawa, QC: Canadian Science Advisory Secretariat.
Department of Fisheries and Oceans (2019). Update of Stock Status Indicators for Northern Shrimp in the Estuary and Gulf of St. Lawrence. Canadian Science Advisory Secretariat. Ottawa, ON: Department of Fisheries and Oceans.
DEVOTES (2012). DEVelopment Of Innovative Tools for Understanding Marine Biodiversity and Assessing Good Environmental Status (DEVOTES). Available online at: http://www.devotes-project.eu (accessed February 26, 2021).
Dreujou, E., McKindsey, C. W., Grant, C., Tréau de Coeli, L., St-Louis, R., and Archambault, P. (2020). Biodiversity and habitat assessment of coastal benthic communities in a Sub-Arctic Industrial Harbor Area. Water 12:2424. doi: 10.3390/w12092424
Dreujou, E., Paquette, L., Grant, C., Archambault, P., and Carrière, J. (2018). “Caractérisation de la faune benthique,” in Observatoire Environnemental de la Baie de Sept-Îles, ed. J. Carrière (Sept-Îles, QC: INREST), 377–452.
Dutil, J.-D., Proulx, S., Galbraith, P. S., Chasse, J., Lambert, N., and Laurian, C. (2012). Coastal and epipelagic habitats of the estuary and Gulf of St. Lawrence. Canad. Tech. Rep. Fisher. Aquat. Sci. 3009, 1–87.
Environment Canada and Ministère du Développement Durable de l’environnement et des Parcs du Québec (2007). Criteria for the Assessment of Sediment Quality in Quebec and Application Frameworks: Prevention, Dredging and Remediation. Criteria for the Assessment of Sediment Quality in Quebec and Application Frameworks: Prevention, Dredging and Remediation. Québec, QC: Environment Canada and Ministère du Développement Durable de l’environnement et des Parcs du Québec.
EoL (2020). Encyclopedia of Life (EoL). Available online at: https://eol.org (accessed February 26, 2021).
European Commission (2008). Directive 2008/56/EC of the European Parliament and the Council of 17 June 2008 Establishing a Framework for Community Action in the Field of Marine Environmental Policy (Marine Strategy Framework Directive). Luxembourg: European Commission.
Ferraro, S. P., and Cole, F. A. (2004). Optimal benthic macrofaunal sampling protocol for detecting differences among four habitats in Willapa Bay, Washington, USA. Estuaries 27, 1014–1025. doi: 10.1007/BF02803427
Folk, R. L. (1980). Petrology of Sedimentary Rocks, 2 Edn. Austin, TX: Hemphill Publishing Company. doi: 10.1017/cbo9780511626487
Gillett, D. J., Weisberg, S. B., Grayson, T., Hamilton, A., Hansen, V., Leppo, E. W., et al. (2015). Effect of ecological group classification schemes on performance of the AMBI benthic index in US coastal waters. Ecol. Indicat. 50, 99–107. doi: 10.1016/j.ecolind.2014.11.005
Grall, J., and Glemarec, M. (1997). Using biotic indices to estimate macrobenthic community perturbations in the Bay of Brest. Estuar. Coast. Shelf Sci. 44, 43–53. doi: 10.1016/S0272-7714(97)80006-6
Gray, J. S. (1979). Pollution-induced changes in populations. Philos. Transact. R. Soc. Lond. Ser. Biol. Sci. 286, 545–561. doi: 10.1098/rstb.1979.0045
Gray, J. S., and Elliott, M. (2009). Ecology of Marine Sediments: From Science to Management. Oxford: Oxford University Press.
Halpern, B. S., Frazier, M., Afflerbach, J., Lowndes, J. S., Micheli, F., O’Hara, C., et al. (2019). Recent pace of change in human impact on the world’s ocean. Sci. Rep. 9, 1–8. doi: 10.1038/s41598-019-47201-9
Halpern, B. S., Selkoe, K. A., Micheli, F., and Kappel, C. V. (2007). Evaluating and ranking the vulnerability of global marine ecosystems to anthropogenic threats. Conserv. Biol. 21, 1301–1315. doi: 10.1111/j.1523-1739.2007.00752.x
Heink, U., Hauck, J., Jax, K., and Sukopp, U. (2016). Requirements for the selection of ecosystem service indicators – the case of MAES indicators. Ecol. Indicat. 61, 18–26. doi: 10.1016/j.ecolind.2015.09.031
Hewitt, J. E., Anderson, M. J., and Thrush, S. F. (2005). Assessing and monitoring ecological community health in marine systems. Ecol. Appl. 15, 942–953. doi: 10.1890/04-0732
Himmelman, J. H. (1991). “Diving observations of subtidal communities in the Northern Gulf of St. Lawrence,” in The Gulf of St. Lawrence: Small Ocean or Big Estuary?, (Ottawa, ON: Department of Fisheries and Oceans), 319–332.
Hooper, D. U., Chapin, F. I., Ewel, J., Hector, A., Inchausti, P., Lavorel, S., et al. (2005). Effects of biodiversity on ecosystem functioning: a consensus of current knowledge. Ecol. Monogr. 75, 3–35. doi: 10.1890/04-0922
Laliberté, E., and Legendre, P. (2010). A distance-based framework for measuring functional diversity from multiple traits. Ecology 91, 299–305. doi: 10.1890/08-2244.1
Laliberté, E., Legendre, P., and Shipley, B. (2014). FD: Measuring Functional Diversity from Multiple Traits, and Other Tools for Functional Ecology. R Package Version 1.0-12.
Largaespada, C., Guichard, F., and Archambault, P. (2012). Meta-ecosystem engineering: Nutrient fluxes reveal intraspecific and interspecific feedbacks in fragmented mussel beds. Ecology 93, 324–333. doi: 10.1890/10-2359.1
Lawton, J. H., and Jones, C. G. (1995). “Linking species and ecosystems: organisms as ecosystem engineers,” in Linking Species & Ecosystems, eds C. G. Jones and J. H. Lawton (Boston, MA: Springer), 141–150. doi: 10.1007/978-1-4615-1773-3_14
Levin, P. S., Fogarty, M. J., Murawski, S. A., and Fluharty, D. (2009). Integrated ecosystem assessments: developing the scientific basis for ecosystem-based management of the ocean. PLoS Biol. 7:14. doi: 10.1371/journal.pbio.1000014
Levinton, J. S. (2013). Marine Biology: Function, Biodiversity, Ecology, 4th Edn. Oxford: Oxford University Press.
Link, H., Piepenburg, D., and Archambault, P. (2013). Are hotspots always hotspots? The Relationship between diversity, resource and ecosystem functions in the arctic. PLoS One 8:e0074077. doi: 10.1371/journal.pone.0074077
Magurran, A. E., and McGill, B. J. (2011). Biological Diversity: Frontiers in Measurement and Assessment. 1st Edn. Oxford: Oxford University Press.
Mason, N. W. H., Mouillot, D., Lee, W. G., and Wilson, J. B. (2005). Functional richness, functional evenness and functional divergence: the primary components of functional diversity. Oikos 111, 112–118. doi: 10.1111/j.0030-1299.2005.13886.x
Maurer, D., Nguyen, H., Robertson, G., and Gerlinger, T. (1999). The infaunal trophic index (ITI): its suitability for marine environmental monitoring. Ecol. Appl. 9, 699–713.
Miatta, M., Bates, A. E., and Snelgrove, P. V. R. (2021). Incorporating biological traits into conservation strategies. Annu. Rev. Mar. Sci. 13, 421–443. doi: 10.1146/annurev-marine-032320-094121
Moriarty, P. E., Hodgson, E. E., Froehlich, H. E., Hennessey, S. M., Marshall, K. N., Oken, K. L., et al. (2018). The need for validation of ecological indices. Ecol. Indicat. 84, 546–552. doi: 10.1016/j.ecolind.2017.09.028
Mouillot, D., Graham, N. A. J., Villéger, S., Mason, N. W. H., and Bellwood, D. R. (2013). A functional approach reveals community responses to disturbances. Trends Ecol. Evol. 28, 167–177. doi: 10.1016/j.tree.2012.10.004
Muxika, I., Borja, Á, and Bald, J. (2007). Using historical data, expert judgement and multivariate analysis in assessing reference conditions and benthic ecological status, according to the European Water Framework Directive. Mar. Pollut. Bull. 55, 16–29. doi: 10.1016/j.marpolbul.2006.05.025
Muxika, I., Borja, Á, and Bonne, W. (2005). The suitability of the marine biotic index (AMBI) to new impact sources along European coasts. Ecol. Indicat. 5, 19–31. doi: 10.1016/j.ecolind.2004.08.004
Niemi, G. J., and McDonald, M. E. (2004). Application of ecological indicators. Annu. Rev. Ecol. Evol. Syst. 35, 89–111. doi: 10.1146/annurev.ecolsys.35.112202.130132
Nozères, C., Bourassa, M.-N., Gendron, M.-H., Plourde, S., Savenkoff, C., Bourdages, H., et al. (2015). Using annual ecosystemic multispecies surveys to assess biodiversity in the Gulf of St. Lawrence. Canad. Techn. Rep. Fish. Aquat. Sci. 3149:126.
OBIS (2020). Ocean Biodiversity Information System (OBIS). Available online at: https://www.obis.org (accessed February 17, 2021).
Oksanen, J., Blanchet, F. G., Friendly, M., Kindt, R., Legendre, P., McGlinn, D., et al. (2019). vegan: Community Ecology Package.
Orr, J. A., Vinebrooke, R. D., Jackson, M. C., Kroeker, K. J., Kordas, R. L., Mantyka-pringle, C., et al. (2020). Towards a unified study of multiple stressors: divisions and common goals across research disciplines. Proc. R. Soc. B Biol. Sci. 20:20200421. doi: 10.1098/rspb.2020.0421
Palomares, M., and Pauly, D. (2020). SeaLifeBase. Available online at: https://sealifebase.ca (accessed February 17, 2021).
Pearson, T. H., and Rosenberg, R. (1978). Macrobenthic succession in relation to organic enrichment and pollution of the marine environment. Oceanogr. Mar. Biol. Annu. Rev. 16, 229–311.
Pelletier, M. C., Gillett, D. J., Hamilton, A., Grayson, T., Hansen, V., Leppo, E. W., et al. (2018). Adaptation and application of multivariate AMBI (M-AMBI) in US coastal waters. Ecol. Indicat. 89, 818–827. doi: 10.1016/j.ecolind.2017.08.067
Piacenza, S. E., Barner, A. K., Benkwitt, C. E., Boersma, K. S., Cerny-Chipman, E. B., Ingeman, K. E., et al. (2015). Patterns and variation in benthic biodiversity in a large marine ecosystem. PLoS One 10:135. doi: 10.1371/journal.pone.0135135
Pinto, R., Patrício, J., Baeta, A., Fath, B. D., Neto, J. M., and Marques, J. C. (2009). Review and evaluation of estuarine biotic indices to assess benthic condition. Ecol. Indicat. 9, 1–25. doi: 10.1016/j.ecolind.2008.01.005
Pratt, D. R., Lohrer, A. M., Pilditch, C. A., and Thrush, S. F. (2014). Changes in ecosystem function across sedimentary gradients in estuaries. Ecosystems 17, 182–194. doi: 10.1007/s10021-013-9716-6
Quinn, G. P., and Keough, M. J. (2002). Experimental Design and Data Analysis for Biologists. Cambridge: Cambridge University Press.
Rees, H. L., Hyland, J. L., Hylland, K., Mercer Clarke, C. S. L., Roff, J. C., and Ware, S. (2008). Environmental indicators: Utility in meeting regulatory needs. An overview. ICES J. Mar. Sci. 65, 1381–1386. doi: 10.1093/icesjms/fsn153
Rice, J. (2003). Environmental health indicators. Ocean Coast. Manag. 46, 235–259. doi: 10.1016/S0964-5691(03)00006-1
Robert, P., McKindsey, C. W., Chaillou, G., and Archambault, P. (2013). Dose-dependent response of a benthic system to biodeposition from suspended blue mussel (Mytilus edulis) culture. Mar. Pollut. Bull. 66, 92–104. doi: 10.1016/j.marpolbul.2012.11.003
Salas, F., Marcos, C., Neto, J. M., Patrício, J., Pérez-Ruzafa, A., and Marques, J. C. (2006). User-friendly guide for using benthic ecological indicators in coastal and marine quality assessment. Ocean Coast. Manag. 49, 308–331. doi: 10.1016/j.ocecoaman.2006.03.001
Santos, C., Ehler, C. N., Agardy, T., Andrade, F., Orbach, M. K., and Crowder, L. B. (2019). “Marine spatial planning,” in World Seas: An Environmental Evaluation, ed. C. Sheppard (Cambridge, CA: Academic Press), 571–592. doi: 10.1016/B978-0-12-805052-1.00033-4
Schloss, I., Archambault, P., Beauchesne, D., Bourgault, D., Cusson, M., Dumont, D., et al. (2017). “Impacts potentiels cumulés des facteurs de stress liés aux activités humaines sur l’écosystème marin du Saint-Laurent dans Les hydrocarbures dans le golfe du Saint-Laurent − Enjeux sociaux, économiques et environnementaux,” in Les Hydrocarbures Dans le Golfe du Saint-Laurent — Enjeux Sociaux, Économiques et Environnementaux, eds P. Archambault, I. Schloss, C. Grant, and S. Plante (Rimouski, QC: Notre Golfe), 132–165.
SDG (2015). Sustainable Development Goals Knowledge Platform (SDG). Available online at: http://www.un.org/sustainabledevelopment/news/communications-material/ (accessed February 26, 2021).
Secretariat of the CBD (2010). Aichi Biodiversity Targets. Available online at: https://www.cbd.int/sp/targets/ (accessed February 26, 2021).
Shaw, J.-L. (2019). Hydrodynamique de la Baie de Sept-Îles. Master’s thesis, Université du Québec à Rimouski, Rimouski.
Simard, N., Pereira, S., Estrada, R., and Nadeau, M. (2013). État de la situation des espèces envahissantes marines au Québec. Rapp. manus. Can. sci. halieut. aquat. 3020:61.
Simboura, N., and Zenetos, A. (2002). Benthic indicators to use in ecological quality classification of Mediterranean soft bottom marine ecosystems, including a new Biotic Index. Mediter. Mar. Sci. 3, 77–111.
Smeets, E., and Weterings, R. (1999). Environmental Indicators: Typology and Overview. Copenhagen: European Environment Agency.
Somerfield, P. J. (2008). Identification of the bray-curtis similarity index: comment on Yoshioka (2008). Mar. Ecol. Prog. Ser. 372, 303–306. doi: 10.3354/meps07841
Stachowicz, J. J., Bruno, J. F., and Duffy, J. E. (2007). Understanding the effects of marine biodiversity on communities and ecosystems. Annu. Rev. Ecol. Evol. System. 38, 739–766. doi: 10.1146/annurev.ecolsys.38.091206.095659
Sutherland, T. F., Levings, C. D., Petersen, S. A., Poon, P., and Piercey, B. (2007). The use of meiofauna as an indicator of benthic organic enrichment associated with salmonid aquaculture. Mar. Pollut. Bull. 54, 1249–1261. doi: 10.1016/j.marpolbul.2007.03.024
Teixeira, H., Berg, T., Uusitalo, L., Fürhaupter, K., Heiskanen, A. S., Mazik, K., et al. (2016). A catalogue of marine biodiversity indicators. Front. Mar. Sci. 3:207. doi: 10.3389/fmars.2016.00207
United Nations (1992). Convention on Biological Diversity (CBD). New York, NY: United Nations. doi: 10.1016/B978-0-12-384719-5.00418-4
Villéger, S., Mason, N. W. H., and Mouillot, D. (2008). New multidimensional functional diversity indices for a multifaceted framework in functional ecology. Ecology 89, 2290–2301. doi: 10.1890/07-1206.1
Warwick, R. M., and Clarke, K. R. (1994). Relearning the ABC: taxonomic changes and abundance/biomass relationships in disturbed benthic communities. Mar. Biol. 118, 739–744. doi: 10.1007/BF00347523
Warwick, R. M., and Clarke, K. R. (1995). New ‘biodiversity’ measures reveal a decrease in taxonomic distinctness with increasing stress. Mar. Ecol. Prog. Ser. 129, 301–305. doi: 10.3354/meps129301
Wei, C. L., Cusson, M., Archambault, P., Belley, R., Brown, T., Burd, B. J., et al. (2020). Seafloor biodiversity of Canada’s three oceans: patterns, hotspots and potential drivers. Divers. Distribut. 26, 226–241. doi: 10.1111/ddi.13013
Wentworth, C. K. (1922). A scale of grade and class terms for clastic sediments. J. Geol. 30, 377–392. doi: 10.1086/622910
Word, J. (1978). “The infaunal trophic index,” in Southern California Coastal Water Research Project, ed. W. Bascom (Los Angeles, CA: Wiley), 19–39.
WoRMS Editorial Board (2020). World Register of Marine Species (WoRMS). Available online at: http://marinespecies.org (accessed February 26, 2021).
Keywords: environmental indicators, ecological status, coastal benthos, macrofauna, Gulf of St. Lawrence
Citation: Dreujou E, Desroy N, Carrière J, Tréau de Coeli L, McKindsey CW and Archambault P (2021) Determining the Ecological Status of Benthic Coastal Communities: A Case in an Anthropized Sub-Arctic Area. Front. Mar. Sci. 8:637546. doi: 10.3389/fmars.2021.637546
Received: 03 December 2020; Accepted: 05 March 2021;
Published: 31 March 2021.
Edited by:
Macarena Ros, Seville University, SpainReviewed by:
Xiaoshou Liu, Ocean University of China, ChinaSarah Samadi, Muséum National d’Histoire Naturelle, France
Panagiotis D. Dimitriou, University of Crete, Greece
Copyright © 2021 Dreujou, Desroy, Carrière, Tréau de Coeli, McKindsey and Archambault. This is an open-access article distributed under the terms of the Creative Commons Attribution License (CC BY). The use, distribution or reproduction in other forums is permitted, provided the original author(s) and the copyright owner(s) are credited and that the original publication in this journal is cited, in accordance with accepted academic practice. No use, distribution or reproduction is permitted which does not comply with these terms.
*Correspondence: Elliot Dreujou, elliot.dreujou@uqar.ca