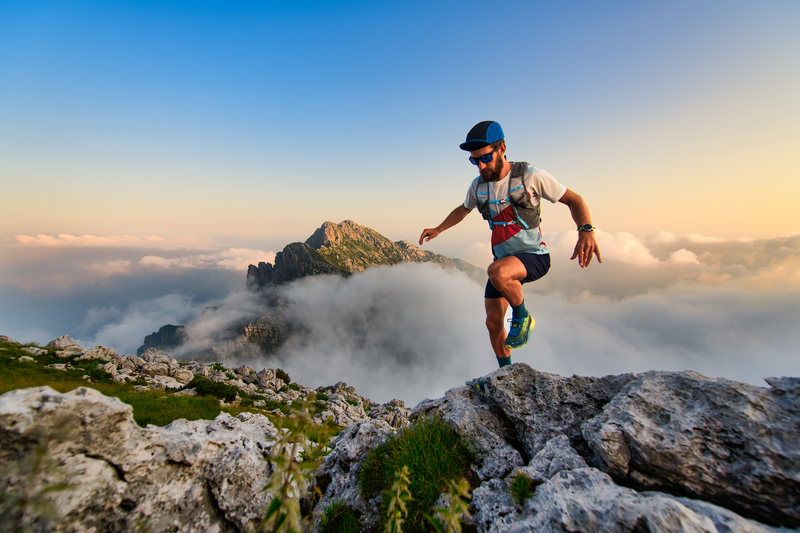
94% of researchers rate our articles as excellent or good
Learn more about the work of our research integrity team to safeguard the quality of each article we publish.
Find out more
ORIGINAL RESEARCH article
Front. Mar. Sci. , 24 May 2021
Sec. Coral Reef Research
Volume 8 - 2021 | https://doi.org/10.3389/fmars.2021.636902
This article is part of the Research Topic Advances in 3D Habitat Mapping of Marine Ecosystem Ecology and Conservation View all 15 articles
The choice of ecological monitoring methods and descriptors determines the effectiveness of a program designed to assess the state of coral reef ecosystems. Here, we comparer the relative performance of the traditional Line Intercept Transect (LIT) method with three methods derived from underwater photogrammetry: LIT on orthomosaics, photoquadrats from orthomosaics, and surface analyses on orthomosaics. The data were acquired at Reunion Island on five outer reef slopes and two coral communities on underwater lava-flows. Coral cover was estimated in situ using the LIT method and with LITs and photoquadrats digitized on orthomosaic. Surface analyses were done on the same orthomosaics. Structural complexity of the surveyed sites was calculated from digital elevation models using three physical descriptors (fractal dimension, slope, surface complexity), and used to explore their possible influence in coral cover estimates. We also compared the methods in terms of scientific outputs, the human expertise and time required. Coral cover estimates obtained with in situ LITs were higher than those obtained with digitized LITs and photoquadrats. Surfaces analyses on orthomosaics yielded the lowest but most the precise cover estimates (i.e., lowest sample dispersion). Sites with the highest coral cover also had the highest structural complexity. Finally, when we added scientific outputs, and requirements for human expertise and time to our comparisons between methods, we found that surface analysis on the orthomosaics was the most efficient method. Photoquadrats were more time-consuming than both in situ and digitized LITs, even though they provided coral cover estimates similar to those of digitized LITs and yielded more than one descriptor. The LIT in situ method remains the least time-consuming and most effective for species-level taxonomic identifications but is the most limited method in terms of data outputs and representativeness of the ecosystem.
The advent of SCUBA diving in the second part of the twentieth century facilitated direct observations of underwater marine ecosystems and prompted the development of coral reef survey techniques (Goreau, 1959; Loya, 1972; Riedl, 1980; Dahl, 1981). Quantitative methods [i.e., Line Intercept Transect Method (LIT), Point Intercept Transect Method (PIT), photoquadrats, video transect] and semi-quantitative methods [i.e., Dahl quotation, Medium Scale Approach (MSA)] were developed in what became reference studies in benthic reef ecology (Loya, 1978; English et al., 1997). These methods mostly focused on assessing 2D descriptors (e.g., percent coral cover), while few attempted to estimate 3D parameters such as “reef rugosity,” with the chain and tape method (Risk, 1972) or to combine 2D and 3D assessments as with the Chain Intercept Transect Method (see Hill and Wilkinson, 2004) and habitat complexity assessment (see Friedlander and Parrish, 1998; Wilson et al., 2007). The LIT method became widespread as it is well suited to acquire long-term monitoring data, as well as relatively easy and cheap (e.g., Global Coral Reef Monitoring Network). This method is one of more adopted worldwide to monitor benthic communities and specially to assess coral cover and provided historical records over the last 60 years (English et al., 1997; Hill and Wilkinson, 2004). Yet, over past two decades, photoquadrats, as a method, was increasingly recommended to standardize regional data as was done by the GCRMN Caribbean node (see Vallès et al., 2019).
Recently, new coral reef descriptors and innovative operational tools for monitoring reefs were developed and made more accessible (e.g., Burns et al., 2015; Hedley et al., 2016; Madin et al., 2016; Elise et al., 2019a). Compared to older, simpler traditional survey methods, these tools aim to enhance the quantity and quality of data (e.g., Lechene et al., 2019) which in turn lead to better understanding reef communities and their ecological functioning (e.g., Elise et al., 2019b; Zawada et al., 2019; Urbina-Barreto et al., 2020). In a recent study, Obura et al. (2019) reviewed and analyzed coral reef monitoring methods, assessment technologies, and short-term management perspectives. Obura et al. (2019) found that while hard coral cover is the single most reported reef parameter, alone it cannot reflect the status of a reef or be the basis for conservation measures. Other essential data needed include functional characteristics upon which coral communities rely, such as the relative abundance of different coral colony structures and descriptors of structural complexity of reefs (Pendleton et al., 2016; González-Barrios and Álvarez-Filip, 2018). Structural complexity supports the ecological functioning of coral reefs, as well as the resilience and the long-term stability of associated biodiversity (Peterson et al., 1998; Alvarez-Filip et al., 2013; Darling et al., 2017; Magel et al., 2019). However, a global review of coral benthic community monitoring methods shows that only three out of 15 methods included a descriptor of structural complexity, namely reef rugosity (Hill and Wilkinson, 2004).
The selection of a given ecological monitoring method to assess the status of reefs should optimally be based on three main factors: (1) observer bias along with standardization of protocols and data (e.g., Caldwell et al., 2016; Flower et al., 2017; Rossi et al., 2021), (2) the ability to conduct assessment at varying spatial and temporal scales (e.g., González-Rivero et al., 2014, González-Rivero et al., 2020; Wedding et al., 2019), and (3) the technical, financial, and social feasibility of the method (e.g., Gilbert and Quod, 2018; Darling et al., 2019). Overly simple descriptors will not capture enough information while overly complicated ones will not be sustainable. The choice of survey methods should be guided by the objectives of the program and their ability to provide data appropriate for answering the question posed, in addition to being sustainable. Over the past 50 years, several studies compared the effectiveness and accuracy of ecological methods and descriptors for benthic reef surveys(e.g., Weinberg, 1981; Ohlhorst et al., 1988; Lam et al., 2006; Dumas et al., 2009; Facon et al., 2015; Storlazzi et al., 2016). Several handbooks were published to support both scientists and managers in selecting the best method corresponding to their specific objectives, including ecological, conservation, and management purposes (e.g., Dahl, 1981; Hill and Wilkinson, 2004; Obura and Grimsditch, 2009; Obura, 2014). Yet comparisons between traditional methods (e.g., LIT) and novel methods such as photogrammetric assessment are lacking.
Current technological advances provide novel tools and methods for reef surveys that can provide more descriptors of reef condition with a minimal increase in effort. Among them, photogrammetry by Structure from Motion (SfM) generates three-dimensional reconstructions of coral reefs as well as 3D models, Digital Elevation Models (DEM, i.e., digital representation of a continuous surface with terrain elevation data) and orthomosaics (i.e., geometrically corrected mosaicked images with a uniform scale). Photogrammetry enables the quantitative monitoring of physical (e.g., structural complexity: slope, fractal dimension, surface complexity) and biological features (e.g., cover of benthic communities, colonies size and abundance) of ecosystems over time (e.g., Storlazzi et al., 2016; Fukunaga et al., 2019; Price et al., 2019; Carlot et al., 2020). These new techniques and methods are likely to become new standards for reef surveying in the coming years (Obura et al., 2019; D’Urban et al., 2020) notably with new solutions helping to automate image analysis such as the widely used machine-learning CoralNet tool, which estimates of coral cover are highly comparable to those generated by reef experts (Williams et al., 2019) and other artificial intelligence applications (e.g., González-Rivero et al., 2016; Hopkinson et al., 2020; Mohamed et al., 2020).
Here, we compare four reef benthic survey methods to estimate coral cover: the traditional Line Intercept Transect method (LIT) and three methods derived from photogrammetric outputs (LIT on orthomosaics, photoquadrats from orthomosaics, and surface analyses at two different scales on orthomosaics. We also examine three physical descriptors of the structural complexity (fractal dimension, slope and surface complexity) in each study site. Our three main goals were to (1) compare estimates of percent coral cover between methods and explore the possible influence of structural complexity in these estimates; (2) examine whether the LIT and photoquadrats methods can be applied to orthomosaics (photogrammetric outputs); and (3) compare the scientific outputs, required resources (expertise and time), as well as the pros and cons of these methods. We then summarize our comparisons of practical aspects of traditional and novel reef assessment methods with regard to project objectives, available manpower and financial resources.
The study was conducted at seven coral reef sites around Reunion, an island of the Mascarene Archipelago in the Western Indian Ocean region. We sampled five outer reef slopes sites located on the West coast and two sites with coral communities on underwater lava-flows located on the East coast (Figure 1). This allowed us to compare the performance of survey methods in different reef environments. The fieldwork took place from March to August 2018 at depths varying from 8 to 15 m. Depending on the monitoring method and region considered, the size of coral reef study site can range from dozens to several hundreds square-meters (Hill and Wilkinson, 2004). For this study, “a site” was an area of 150 m2 for coral communities on lava flows and 500 m2 for those on outer reef slopes. All four reef survey methods were applied to each of these sites.
Figure 1. Map of the study sites. On the west coast: TRC, SAL, SBL, COR and GEN. On the east oast (lava-flow reefs): C77 and CAE.
We conducted the LIT in situ while using SCUBA and following the Global Coral Reef Monitoring Network protocol (Obura, 2014; Figure 2A). Three replicate transects were set up at each site. They were each 20 m long and spaced apart by at least 5 m. An experienced coral reef ecologist identified all benthic categories and estimated percent hard coral cover (scleractiniam corals) by dividing the total length of occurrence of hard corals by the total length of the transect.
Figure 2. Illustration of the traditional method: LIT in situ, sampling distance 20 m × 3 (A) and the photogrammetric methods (B): LIT on orthomosaic, sampling distance 20 m × 3 (B.1); photoquadrats from orthomosaic, sampling area 1 m2 × 10 × 3 (B.2); and surface analyses on orthomosaic: sampling area of 40 m2 × 3 (B.3) and sampling area of 150 m2 × 3 (B.4) colors show different benthic categories.
Reefscapes of the seven sites were modeled in 3D by using photogrammetry. Images collected using SCUBA covered 150 m2 area on underwater lava-flow sites (C77, CAE) and 500 m2 on outer reef slope sites (COR, GEN, SAL, SBL, and TRC). The mean overlap between images was ∼70%. For each site, we generated an orthomosaic and a DEM. The mean re projection error was 0.25 pixel, and the mean resolution (i.e., Ground Sampling Distance) was 0.13 cm pixel–1. Percent coral cover was estimated from the orthomosaics using digitized LITs (Figure 2B.1) and photoquadrats (Figure 2B.2). We also assessed percent cover by segmenting surfaces and manually classifying benthic communities on the same orthomosaics (Supplementary Figure 1), which were then clipped into two sampling units: 40 m2 × 3 (Figure 2B.3) and 150 m2 × 3 (Figure 2B.4). These methods applied the same benthic classification as for LITs done in-situ. Digital assessments were done using: (i) LITs on orthomosaics; (ii) Photoquadrats from orthomosaics; (iii) Surface analyses on orthomosaics; and analyses on DEMs of structural complexity descriptors.
Line Intercept Transects on orthomosaics were done by the same diver who conduct the LITs in situ did three 20 m transects (Figure 2B.1) using the open source QGIS software (version 3.1). Measurements of the lengths of the segments were done using the QGIS command: $length with the field calculator tool. Coral cover was calculated as described in section “Line Intercept Transects.”
Ten photoquadrats, each 1 m2 size and spaced 50 cm apart, were extracted along each of the three transects generated for the LIT orthomosaic method (Figure 2B.2). The 30 photoquadrats were exported from the orthomosaic and the CPCe software was applied to conduct a benthic classification (Kohler and Gill, 2006). Following the recommendation in Dumas et al. (2009), a stratified point sampling was done where each photoquadrat was divided into nine cells (three columns and three rows), with one point classified per cell (i.e., 9 total points were classified per photoquadrat). The software then directly calculated the mean total percent coral cover.
For the surface analyses done on orthomosaics, the benthic classification was done manually delineating each coral colony as a polygon in QGIS (Supplementary Figure 1). For all sites, the colony layer was clipped in three areas of 40 m2 (Figure 2B.3) and three sampling areas of 150 m2 for the five outer reef slopes (Figure 2B.4) to check for the possible differences between the two total sampling surfaces and optimize sampling (Lechene et al., 2019). The field calculator tool and the area($geometry) command in QGIS were used to measure the polygonal surfaces of hard corals. Percent coral cover was then estimated by dividing the sum of hard corals by the area of total sampling area (i.e., 40 or 150 m2). We used the total coral cover for a 150 m2 area as a possible reference value given that this area is typically used for benthic reef monitoring.
Finally, for the analyses of DEMs, at each site we estimated surface complexity (i.e., the ratio of 2D to 3D surface), slope, and fractal dimension from the DEMs (Supplementary Figure 2) running the R code developed by R Core Team (2019); Fukunaga et al. (2019). Importantly we assessed the influence of structural complexity on percent coral cover estimates by each method.
We performed two-way ANOVAs and Tukey multiple mean comparisons to test the effects of site, method, and their interaction on coral cover estimates.
The methods were compared in terms of data out-put, the human expertise and time required, advantages and limitations, and fields of application. Table 1 presents the comparison criteria. Importantly all authors contributed to these comparisons which therefore reflects varying experience or approaches to coral reef ecology including fundamental academic research, applied sciences, environmental consultancy, and research and development in coral reef ecology (research directors, senior lecture, PhD in marine ecology, environmental consultancy firm directors and managers, engineers in marine ecology, engineers in geography).
Estimates of percent coral cover were significantly different across sites and methods (two-way ANOVA method × site p < 0.05; Supplementary Table 1). The LITs done in situ resulted in the highest estimates of coral cover. In comparison LITs on orthomosaics and photoquadrats from orthomosaic methods yielded significantly lower coral cover and there was no significant difference in coral cover estimates between these two last methods. The surface analyses on orthomosaics (40 m2 × 3 and 150 m2 × 3) generated significantly lower coral cover estimates compared to the three other methods but without any significant differences between the two surfaces analyses (two-way ANOVA p < 0.001; Supplementary Table 1; Tukey tests p < 0.01; Supplementary Table 2; Figure 3). At each site, these two surface analyses provided the lowest coral cover estimates except for outer ref slope site SBL where photoquadrats and LITs on orthomosaics generated the lowest values, and for the underwater lava-flow site CAE, where LITs on orthomosaic also yielded a lower value compared to surface analyses. Coral cover estimated from surface analyses on orthomosaics was associated with a lower variance compared to the other methods (Figure 4). Estimates of single surface sampling (150 m2) on orthomosaics for each site showed similar trends at underwater lava-flow sites C77 and CAE. Coral cover estimates done at outer reef slope sites SAL, SBL and TRC were higher than the two sampling surfaces (40m2 × 3 and 150m2 × 3) but lowest than LIT in situ method. In contrast estimates for outer reef slopes sites GEN and COR were lower than all other methods (Figure 4 and Supplementary Table 3).
Figure 3. Method x Site interaction plot of percent coral cover estimations. Groups with the same Greek letters (α, β, γ) display no significant difference (two-way ANOVA and Tukey tests, p < 0.001). Colors represent survey methods: LIT in-situ (turquoise); LIT from orthomosaic (pink), Photoquadrats from orthomosaic (yellow); surface analyses on orthomosaic sampling 40 m2 × 3 (green); surface analyses on orthomosaic sampling 150 m2 × 3 (purple).
Figure 4. Percent coral cover by method across sites. Same letters (a, b, c, d or e) in top of boxplots/site means no significant differences between groups (two-way ANOVA and Tukey test p < 0.01).
With respect to sites, the percent coral cover was highest on underwater lava-flow sites C77, CAE and on the outer reef slope site GEN, with the lowest percent cover estimated on outer reef slope sites SBL and TRC (two-way ANOVA p < 0.001; Supplementary Table 1; Tukey tests p < 0.01; Figure 4; Supplementary Table 4).
With the DEMs we quantified structural complexity and examinated its possible influence on coral cover estimates (Table 2 and Figure 5). The differences between LITs done in situ and surface analyses on 40 m2 × 3 were significantly correlated with the slope (Pearson correlation R2 = 0.68; p < 0.05) and surface complexity (Pearson correlation R2 = 0.66; p < 0.05, Figure 5). The same trends were observed for LITs done on orthomosaics, although the slope and surface complexity effects were not significant (Supplementary Figure 3). Yet the fractal dimension showed no correlation when examining differences between LITs done in situ and photoquadrats from orthomosaics.
Table 2. Physical descriptors of the structural complexity: surface complexity, slope, and fractal dimension computed from each study site’s DEM.
Figure 5. Differences in percent coral cover obtained with LIT in situ and surface analysis on 40 m2 × 3 orthomosaics as a function of the structural complexity descriptors: for surface complexity (top) and slope (bottom).
Results of the method description and criteria evaluation are shown in Table 3 with three categories: Method description, Human expertise and Time required, and Review and Perspectives.
Table 3. Comparison of the survey methods deployed in this study. Abbreviations: NA = not applicable.
The LITs conducted in situ generated higher estimates of coral cover compared to other methods which is consistent with findings of Leujak and Ormond (2007) who compared six methods of coral community surveys and found that LIT and Point Intercept Transect (PIT) methods overestimated principal benthic categories. Lam et al. (2006) also showed that the PIT method overestimates coral cover, particularly at sites with scarce coral colonies. However, Facon et al. (2015) show that the PIT method generates almost the same level of information as for the LIT method. Regarding digital assessments on orthomosaics, no differences were detected between LITs on orthomosaics and photoquadrats from orthomosaics suggesting that the linear and point sampling used (length and number) may be equivalent in terms of representativeness for coral cover and accuracy. Among all methods, surface analyses on orthomosaics resulted the lowest and least variable percent coral cover estimates, and is therefore probably the most accurate methodology. The sampling unit of surface analyses is a polygon (surface estimator) which is more representative than lines or points, despite errors associated with manual delineations on the orthomosaics. The low variances of percent coral cover estimates confirms that a surface analysis based on orthomosaics is comparatively the most accurate and reliable method. Molloy et al. (2013) showed that coral recovery could not be detected using even the most intensive photoquadrats protocols, and suggested a coral surface area as a more sensitive and powerful means to detect coral recovery. However, a weakness of photogrammetric methods relates to the orthographic projection used to generate orthomosaics. While this does not affect flat sites, sites whit high structural complexity or that steeply slope are more impacted by the orthographic projection resulting in an underrepresentation of the terrain when projected. Consequently, the surface area of colonies is likely underestimated in complex habitats on reef slopes (Urbina-Barreto et al., 2020). Here, the differences found between cover estimates from LITs done in situ and surface analyses on orthomosaics increased both with surface complexity and slope. This point to a methodological limitation which could be problematic when comparing sites with large differences in slope or complexity. Here, however, the studied sites had small differences in slope values and surface complexity. Further research is needed on assessing how habitat complexity descriptors affect estimates of coral cover (e.g., surface complexity, slope) when applying innovative photogrammetric methods.
The findings of our study could very well support coral reef conservation. Until now, coral reef studies applied different methods to map reefs as accurately as possible, aiming to improve the descriptors and determine the optimal level of sampling to monitor coral communities (e.g., Goreau, 1959; Weinberg, 1981; Leujak and Ormond, 2007; Casella et al., 2016; Fukunaga et al., 2019; Hernández-Landa et al., 2020). Scientists and managers often overlook the under or overestimation of coral cover, despite this being a crucial aspect when defining targets for resource conservation. Further such targets increasingly require to integrate the variable results of numerous surveys conducted at broad spatial scales (Edmunds and Bruno, 1996; Hoegh-Guldberg et al., 2018). Recently, Lechene et al. (2019) examined levels of sampling using large-area mosaics that optimize coral reef sampling strategies. They concluded that each benthic category has own optimal level of sampling. For instance they required a 60 m2 area to obtain representative cover estimates of 55% of all benthic features. This points to an essential need for a calibration between survey methods, in particular for LIT and PIT methods since they are widely used to support coral reef conservation. Vallès et al. (2019) studied the transition between the chain intercept transect (a method derived from LIT but using a chain instead of a taut measuring tape) and photoquadrat methods on Caribbean reefs and concluded that switching methods for coral surveys would be complicated, as almost all reef benthic categories would require different conversion procedures (i.e., specific mathematical adjustments). More such comparisons studies between traditional and photogrammetric methods for coral reef surveys are needed to prepare for a likely transition between methods, to ensure more accurate and long-term surveys of reef ecosystems at wide spatial scales without dismissing the fundamental historical data gathered over the last six decades.
The LIT in situ method generates significantly less data than photogrammetric methods (Table 3). Indeed surface analyses can deliver more than eight highly comprehensive and high-quality reef descriptors based on DEM and orthomosaic analyses, not to mention the analyses that could be performed on other photogrammetric outputs such as point clouds and meshes. The photoquadrats from orthomosaics method is a less comprehensive method, delivering only four descriptors, while LITs on orthomosaics only delivers a single descriptor (percent cover of benthic categories), as does LIT in situ method. Furthermore, photogrammetric raw field data (i.e., photographs) allow post-dive analysis by different operators or using new tools to automatically annotate underwater imagery (e.g., Beijbom et al., 2015; Williams et al., 2019; González-Rivero et al., 2020), whereas raw data collected with the LIT in situ method (or in any other in situ visually based method) relies on the diver’s in situ expertise. However, photogrammetric methods require more equipment (cameras, computer, and software), and are more constrained by environmental conditions. Despite limitations in terms of data output, the LIT in situ method allows for a finer taxonomic determination. Then again, compared to the LIT in situ method, a significant advantage of photogrammetric methods is the ability to calculate essential habitat descriptors on reefs, i.e., surface complexity, fractal dimension, slope, and rugosity profiles which are closely linked to reef biodiversity and productivity as well as ecological changes in seascapes (e.g., Graham and Nash, 2013; Rees et al., 2018; Price et al., 2019).
In terms of practicality, in contrast to the LIT in situ method, photogrammetric methods do not require expert biologists for planning and implementing survey operations and underwater data recording. Then again, an expert is not required for post-acquisition analyses of LIT in situ data whereas expertise is mandatory to generate 3D models and photogrammetric outputs, and to conduct ecological and spatial analyses.
As for the time required, the photogrammetric methods and ecological analyses comparatively need more preparation than the LIT in situ method, while the time needed for fieldwork is more or less the same. In decreasing order, the total time needed by method for the preparation, fieldwork and analyses was as follow: (1) Analyses on orthomosaics and DEM (5.8–10.3 man-days/site); (2) Photoquadrats from orthomosaics (5.6–6.1 man-days/site); (3) LIT on orthomosaics (5.4–5.9 man-days/site); (4) LIT in situ (2.4 man-days/site). As mentioned earlier, results are directly correlated with the quantity and quality of the information produced.
Overall, the comparisons made here of coral cover estimates, data outputs, and required technical and human resources point to the method that may more suitable for a given reef monitoring program or resource conservation purpose. When taking into account the results of this study and those by others who compared means, fieldwork and accuracy across different benthic survey methods (e.g., Weinberg, 1981; Ohlhorst et al., 1988; Beenaerts and Berghe, 2007; Dumas et al., 2009; Facon et al., 2015; Lechene et al., 2019), surface analysis on orthomosaics stands out as the most efficient method when considering the quantity and quality of data gathered and time expended. The photoquadrat from orthomosaics method is an intermediate solution. The traditional LIT in situ method remains the least time-consuming method, efficient for specific taxonomic identifications, while being the most limited in terms of reef descriptors and representativeness of the ecosystem. The reproduction of traditional methods from photogrammetric outputs (DEMs or orthomosaics), as proposed here, is an innovative alternative to traditional reef survey methods that can be adapted for any coral reef study.
Confronting the global coral reef crisis, challenging conservation targets require the optimization of reef survey methods. New technologies can promote rapid advancements in reef science and support management programs to solve key issues facing coral reefs (Bellwood et al., 2004; Hoegh-Guldberg et al., 2018; Madin et al., 2019. In this context, our study is a first step to launch the likely transition from traditional methods to novel and more efficient ones (Storlazzi et al., 2016). We also provide new information to complement and optimize reef surveys, and enhance the effectiveness of conservation programs. Photogrammetric tools can be of particular interest to coastal planners and decision-makers when it comes to avoidance, reduction, or compensation measures often required by local environmental laws for coastal and seascape works. Improving the efficiency of conservation is likely to promote the recovery of marine life (if major pressures are relieved) and as such is an ethical and smart economic objective to achieve a sustainable future (Kenchington, 2018; Duarte et al., 2020). To this end, new methods adopting the FAIR principles (findability, accessibility, interoperability, and reusability) for stewardship of reef data can improve the monitoring of these ecosystems (Rossi et al., 2021). Among them the development of artificial intelligence for automated analysis of images (Williams et al., 2019; González-Rivero et al., 2020) and photogrammetry outputs (e.g., Hopkinson et al., 2020; Mohamed et al., 2020), multispectral and hyperspectral imagery (e.g., Parsons et al., 2018; Bajjouk et al., 2019; Li et al., 2019), and the improvement of diver-based or automated data acquisition from Remotely Operated Vehicles and Autonomous Underwater Vehicles (Friedman et al., 2012; Obura et al., 2019; Hatcher et al., 2020; Rossi et al., 2021) will likely revolutionize this field. Finally the integration of all existing reef habitat mapping data in common and open source data bases could help to overcome the lack of transferability across systems/scale, provide more accurate and representative assessments of habitats, and generate conservation measures and habitat rehabilitation actions (Madin et al., 2019; Rossi et al., 2021). Together, these efforts will foster the application of these new methods in both research and coral reef conservation programs.
The original contributions presented in the study are included in the article/Supplementary Material, further inquiries can be directed to the corresponding author/s.
IU-B, RG, SE, RP, and MA conceived the ideas. IU-B, RG, RP, J-PQ, LP, ED, and MA designed the methodology. IU-B, VM, J-PQ, RG, and SB collected the data. IU-B and RP generated digital elevation models and orthomosaics. IU-B, PD, CP, and SE performed data handling and analyzed the data. IU-B, RG, RP, J-PQ, MF, PD, SE, ED, LP, and MA evaluated the criteria methods. IU-B led the writing of the manuscript with SE, LP, and MA. All authors contributed critically to the drafts and gave final approval for publication.
IU-B was supported by a CIFRE fellowship, from the French Association of Research and Technologies, under the agreement 2017/0322. The project was also supported by Agence de l’Eau Rhône-Méditerranée-Corse (Pierre Boissery).
IU-B, RG, MF, VM, and ED were employed by Crecoean OI; RP and VM were employed by Geolab S.A.S; J-PQ was employed by ARVAM-Pareto.
The remaining authors declare that the research was conducted in the absence of any commercial or financial relationships that could be construed as a potential conflict of interest.
We are thankful to Henrich J. Bruggemann for the valuable advice of this manuscript. Thank you to Ken Deslarzes and Jane Ballard for the English revision of the manuscript. We thank Ifigenia Urbina-Barreto and Julio A. Urbina for their inputs throughout writing of the manuscript. We also thank the reviewers for the comments that greatly improved this manuscript. Thank you to the Reserve Naturelle Marine de La Réunion for authorizing the work inside the reserve. We are grateful to know that this study was selected by the SCORE-REEF project workshops and reflections, which is supported by FRB/CESAB.
The Supplementary Material for this article can be found online at: https://www.frontiersin.org/articles/10.3389/fmars.2021.636902/full#supplementary-material
Alvarez-Filip, L., Carricart-Ganivet, J. P., Horta-Puga, G., and Iglesias-Prieto, R. (2013). Shifts in coral-assemblage composition do not ensure persistence of reef functionality. Scientific Rep. 3, 1–5. doi: 10.1038/srep03486
Bajjouk, T., Mouquet, P., Ropert, M., Quod, J. P., Hoarau, L., Bigot, L., et al. (2019). Detection of changes in shallow coral reefs status: towards a spatial approach using hyperspectral and multispectral data. Ecol. Indic. 96, 174–191. doi: 10.1016/j.ecolind.2018.08.052
Beenaerts, N., and Berghe, E. V. (2007). Comparative Study of Three Transect Methods to Assess Coral Cover, Richness and Diversity. West. Ind. Ocean J. Mar. Sci. 4, 29–38. doi: 10.4314/wiojms.v4i1.28471
Beijbom, O., Edmunds, P. J., Roelfsema, C., Smith, J., Kline, D. I., Neal, B. P., et al. (2015). Towards Automated Annotation of Benthic Survey Images: variability of Human Experts and Operational Modes of Automation. PLoS One 10:e0130312. doi: 10.1371/journal.pone.0130312
Bellwood, D. R., Hughes, T. P., Folke, C., and Nyström, M. (2004). Confronting the coral reef crisis. Nature 429, 827–833. doi: 10.1038/nature02691
Burns, J. H. R., Delparte, D., Gates, R. D., and Takabayashi, M. (2015). Utilizing underwater three-dimensional modeling to enhance ecological and biological studies of coral reefs. Int. Arch. Photogr. 5, 61–66. doi: 10.5194/isprsarchives-XL-5-W5-61-2015
Caldwell, Z. R., Zgliczynski, B. J., Williams, G. J., and Sandin, S. A. (2016). Reef fish survey techniques: assessing the potential for standardizing methodologies. PLoS One 11:e0153066. doi: 10.1371/journal.pone.0153066
Carlot, J., Rovère, A., Casella, E., Harris, D., Grellet-Muñoz, C., Chancerelle, Y., et al. (2020). Community composition predicts photogrammetry-based structural complexity on coral reefs. Coral Reefs 39, 967–975. doi: 10.1007/s00338-020-01916-8
Casella, E., Collin, A., Harris, D., Ferse, S., Bejarano, S., Parravicini, V., et al. (2016). Mapping coral reefs using consumer-grade drones and structure from motion photogrammetry techniques. Coral Reefs 36, 1–7. doi: 10.1007/s00338-016-1522-0
Dahl, A. L. (1981). Coral Reef Monitoring Handbook. South Pacific Comission 1981. Autralia: Bridge Printery Sidney.
Darling, E. S., Graham, N. A. J., Januchowski-Hartley, F. A., Nash, K. L., Pratchett, M. S., and Wilson, S. K. (2017). Relationships between structural complexity, coral traits, and reef fish assemblages. Coral Reefs 36, 561–575. doi: 10.1007/s00338-017-1539-z
Darling, E. S., McClanahan, T. R., Maina, J., Gurney, G. G., Graham, N. A. J., Januchowski-Hartley, F., et al. (2019). Social-environmental drivers inform strategic management of coral reefs in the Anthropocene. Nat. Ecol. Evol. 3, 1341–1350. doi: 10.1038/s41559-019-0953-8
Duarte, C. M., Agusti, S., Barbier, E., Britten, G. L., Castilla, J. C., Gattuso, J. P., et al. (2020). Rebuilding marine life. Nature 5807801, 39–51.
Dumas, P., Bertaud, A., Peignon, C., Léopold, M., and Pelletier, D. (2009). A ‘quick and clean’ photographic method for the description of coral reef habitats. J. Exp. Mar. Biol. Ecol. 368, 161–168. doi: 10.1016/j.jembe.2008.10.002
D’Urban, J. T., Williams, G. J., Walker-Springett, G., and Davies, A. J. (2020). Three-dimensional digital mapping of ecosystems: a new era in spatial ecology. Proc. Biol. Sci. 287:20192383. doi: 10.1098/rspb.2019.2383
Edmunds, P. J., and Bruno, J. F. (1996). The importance of sampling scale in ecology: kilometer-wide variation in cora reef communities. Mar. Ecol. Prog. Ser. 143, 165–171.
Elise, S., Bailly, A., Urbina-Barreto, I., Mou-Tham, G., Chiroleu, F., Vigliola, L., et al. (2019a). An optimised passive acoustic sampling scheme to discriminate among coral reefs’ ecological states. Ecol. Indic. 107:105623. doi: 10.1016/j.ecolind.2019.105627
Elise, S., Urbina-Barreto, I., Pinel, R., Mahamadaly, V., Bureau, S., Penin, L., et al. (2019b). Assessing key ecosystem functions through soundscapes: a new perspective from coral reefs. Ecol. Indic. 107:105623. doi: 10.1016/j.ecolind.2019.105623
English, S., Wilkinson, C., and Baker, V. (1997). Survey Manual for Tropical Marine Resources, II Edn. Australia: Australian Institute of Marine Science.
Facon, M., Pinault, M., Obura, D., Pioch, S., Pothin, K., Bigot, L., et al. (2015). A comparative study of the accuracy and effectiveness of Line and Point Intercept Transect methods for coral reef monitoring in the southwestern Indian Ocean islands. Ecol. Indic. 60, 1045–1055. doi: 10.1016/j.ecolind.2015.09.005
Flower, J., Ortiz, J. C., Chollett, I., Abdullah, S., Castro-Sanguino, C., Hock, K., et al. (2017). Interpreting coral reef monitoring data: a guide for improved management decisions. Ecol. Indic. 72, 848–869. doi: 10.1016/j.ecolind.2016.09.003
Friedlander, A. M., and Parrish, J. D. (1998). Habitat characteristics affecting fish assemblages on a Hawaiian coral reef. J. Exp. Mar. Biol. Ecol. 224, 1–30. doi: 10.1016/S0022-0981(97)00164-0
Friedman, A., Pizarro, O., Williams, S. B., and Johnson-Roberson, M. (2012). Multi-Scale Measures of Rugosity, Slope and Aspect from Benthic Stereo Image Reconstructions. PLoS One 7:e50440. doi: 10.1371/journal.pone.0050440
Fukunaga, A., Burns, J., Craig, B., and Kosaki, R. (2019). Integrating Three-Dimensional Benthic Habitat Characterization Techniques into Ecological Monitoring of Coral Reefs. J. Mar. Sci. Eng. 2:27. doi: 10.3390/jmse7020027
Gilbert, D., and Quod, J. P. (2018). Coral Reef Monotoring Coping with Climate Change, Toward a Social-ecological System Perspective. Communicating Climate Change Information Decision-Making. Cham: Springer Climate.
González-Barrios, F. J., and Álvarez-Filip, L. (2018). A framework for measuring coral species-specific contribution to reef functioning in the Caribbean. Ecol. Ind. 95, 877–886. doi: 10.1016/j.ecolind.2018.08.038
González-Rivero, M., Beijbom, O., Rodriguez-Ramirez, A., Bryant, D. E., Ganase, A., and Gonzalez-Marrero, et al. (2020). Monitoring of coral reefs using artificial intelligence: a feasible and cost-effective approach. Remote Sens. 12, 1–22. doi: 10.3390/rs12030489
González-Rivero, M., Beijbom, O., Rodriguez-Ramirez, A., Holtrop, T., González-Marrero, Y., Ganase, A., et al. (2016). Scaling up Ecological Measurements of Coral Reefs Using Semi-Automated Field Image Collection and Analysis. Remote Sens. 8:30. doi: 10.3390/rs8010030
González-Rivero, M., Bongaerts, P., Beijbom, O., Pizarro, O., Friedman, A., Rodriguez-Ramirez, A., et al. (2014). The Catlin Seaview Survey - kilometre-scale seascape assessment, and monitoring of coral reef ecosystems. Aquat. Conser 24, 184–198. doi: 10.1002/aqc.2505
Goreau, T. (1959). The ecology of Jamaican coral reefs. I. species composition and zonation. Ecology 40, 67–90.
Graham, N. A. J., and Nash, K. L. (2013). The importance of structural complexity in coral reef ecosystems. Coral Reefs 32, 315–326. doi: 10.1007/s00338-012-0984-y
Hatcher, G. A., Warrick, J. A., Ritchie, A. C., Dailey, E. T., Zawada, D. G., Kranenburg, C., et al. (2020). Accurate Bathymetric Maps From Underwater Digital Imagery Without Ground Control. Front. Mar. Sci. 7, 1–20. doi: 10.3389/fmars.2020.00525
Hedley, J., Roelfsema, C., Chollett, I., Harborne, A., Heron, S., Weeks, S., et al. (2016). Remote Sensing of Coral Reefs for Monitoring and Management: a Review. Remote Sens. 8:118. doi: 10.3390/rs8020118
Hernández-Landa, R. C., Barrera-Falcon, E., and Rioja-Nieto, R. (2020). Size-frequency distribution of coral assemblages in insular shallow reefs of the Mexican Caribbean using underwater photogrammetry. PeerJ. 8:e8957. doi: 10.7717/peerj.8957
Hill, J., and Wilkinson, C. (2004). Methods for Ecological Monitoring of Coral Reefs. Townsville: Australian Institute of Marine Science, doi: 10.1017/CBO9781107415324.004
Hoegh-Guldberg, O., Kennedy, E. V., Beyer, H. L., McClennen, C., and Possingham, H. P. (2018). Securing a Long-term Future for Coral Reefs. Trends Ecol. Evol. 33, 936–944. doi: 10.1016/j.tree.2018.09.006
Hopkinson, B. M., King, A. C., Owen, D. P., Johnson-Roberson, M., Long, M. H., and Bhandarkar, S. M. (2020). Automated classification of three-dimensional reconstructions of coral reefs using convolutional neural networks. PLoS One 15:e0230671. doi: 10.1371/journal.pone.0230671
Kenchington, R. (2018). Science and the management of coral reefs. Mar. Poll. Bull. 136, 508–515. doi: 10.1016/j.marpolbul.2018.09.046
Kohler, K. E., and Gill, S. M. (2006). Coral Point Count with Excel extensions (CPCe): a Visual Basic program for the determination of coral and substrate coverage using random point count methodology. Comput. Geosci. 32, 1259–1269. doi: 10.1016/j.cageo.2005.11.009
Lam, K., Shin, P. K. S., Bradbeer, R., Randall, D., Ku, K. K. K., Hodgson, P., et al. (2006). A comparison of video and point intercept transect methods for monitoring subtropical coral communities. J. Exp. Mar. Biol. Ecol. 333, 115–128. doi: 10.1016/j.jembe.2005.12.009
Lechene, M. A. A., Haberstroh, A. J., Byrne, M., Figueira, W., and Ferrari, R. (2019). Optimising Sampling Strategies in Coral Rees Using Large-Areas Mosaics. Remote Sens. 11:2907. doi: 10.3390/rs11242907
Leujak, W., and Ormond, R. F. G. (2007). Comparative accuracy and efficiency of six coral community survey methods. J. Exp. Mar. Biol. Ecol. 351, 168–187. doi: 10.1016/j.jembe.2007.06.028
Li, J., Schill, S. R., Knapp, D. E., and Asner, G. P. (2019). Object-based mapping of coral reef habitats using planet dove satellites. Remote Sens. 11:1445. doi: 10.3390/rs11121445
Loya, Y. (1972). Community structure and species diversity of hermatypic corals at Eilat Red Sea. Mar. Biol. 13, 100–123. doi: 10.1007/BF00366561
Loya, Y. (1978). Plotless and transect methods. In D. R. S. and R. E. Johannes (Ed.), Monographs on Oceanic Methodology. Coral Reefs 5, 197–218.
Madin, E. M. P., Darling, E. S., and Hardt, M. J. (2019). Emerging Technologies and Coral Reef Conservation: opportunities. Challenges, and Moving Forward. Front. Mar. Sci. 6:727. doi: 10.3389/fmars.2019.00727
Madin, J. S., Hoogenboom, M. O., Connolly, S. R., Darling, E. S., Falster, D. S., Huang, D., et al. (2016). A Trait-Based Approach to Advance Coral Reef Science. Trends Ecol. Evol. 31, 419–428. doi: 10.1016/j.tree.2016.02.012
Magel, J. M. T., Burns, J. H. R., Gates, R. D., and Baum, J. K. (2019). Effects of bleaching-associated mass coral mortality on reef structural complexity across a gradient of local disturbance. Scientific Rep. 9, 1–12. doi: 10.1038/s41598-018-37713-1
Mohamed, H., Nadaoka, K., and Nakamura, T. (2020). Towards Benthic Habitat 3D Mapping Using Machine Learning Algorithms and Structures from Motion Photogrammetry. Remote Sens. 12:127. doi: 10.3390/rs12010127
Molloy, P. P., Evanson, M., Nellas, A. C., Rist, J. L., Marcus, J. E., Koldewey, H. J., et al. (2013). How much sampling does it take to detect trends in coral-reef habitat using photoquadrat surveys? Aquat. Conserv. Mar. Freshw. Ecosyst. 23, 820–837. doi: 10.1002/aqc.2372
Obura, D. (2014). Coral Reef Monitoring Manual South-West Indian Ocean islands. France: Indian Ocean Commission.
Obura, D., Aeby, G., Amornthammarong, N., Appeltans, W., Bax, N., Bishop, J., et al. (2019). Coral Reef Monitoring, Reef Assessment Technologies, and Ecosystem-Based Management. Front. Mar. Sci. 6:580. doi: 10.3389/fmars.2019.00580
Obura, D., and Grimsditch, G. (2009). Resilience Assessment of Coral Reefs bleaching and thermal stress. IUCN Resil. Sci. Group Work. Paper Ser. 5:70.
Ohlhorst, S. L., Liddell, W. D., Taylor, R. J., and Taylor, J. M. (1988). “Evaluation of reef census techniques,” in Proceedings of the 6th International Coral Reef Symposium, Vol. 2, (Australia: Townsville), 319–324.
Parsons, M., Bratanov, D., Gaston, K. J., and Gonzalez, F. (2018). UAVs, hyperspectral remote sensing, and machine learning revolutionizing reef monitoring. Sensors 18, 1–20. doi: 10.3390/s18072026
Pendleton, L. H., Hoegh-Guldberg, O., Langdon, C., and Comte, A. (2016). Multiple stressors and ecological complexity require a new approach to coral reef research. Front. Mar. Sci. 3:36. doi: 10.3389/fmars.2016.00036
Peterson, G., Allen, C. R., and Holling, C. S. (1998). Ecological resilience, biodiversity, and scale. Ecosystems 1, 6–18.
Price, D. M., Robert, K., Callaway, A., Lo lacono, C., Hall, R. A., and Huvenne, V. A. I. (2019). Using 3D photogrammetry from ROV video to quantify cold-water coral reef structural complexity and investigate its influence on biodiversity and community assemblage. Coral Reefs 38, 1007–1021. doi: 10.1007/s00338-019-01827-3
R Core Team (2019). R?: A language and Statistical, Statistical Computing. Vienna: R Foundation for Computing.
Rees, M. J., Knott, N. A., Neilson, J., Linklater, M., Osterloh, I., Jordan, A., et al. (2018). Accounting for habitat structural complexity improves the assessment of performance in no-take marine reserves. Biol. Conserv. 224, 100–110. doi: 10.1016/j.biocon.2018.04.040
Rossi, P., Ponti, M., Righi, S., Castagnetti, C., Simonini, R., Mancini, F., et al. (2021). Needs and Gaps in Optical Underwater Technologies and Methods for the Investigation of Marine Animal Forest 3D-Structural Complexity. Front. Mar. Sci. 8, 1–9. doi: 10.3389/fmars.2021.591292
Storlazzi, C. D., Dartnell, P., Hatcher, G. A., and Gibbs, A. E. (2016). End of the chain? Rugosity and fine-scale bathymetry from existing underwater digital imagery using structure-from-motion (SfM) technology. Coral Reefs 35, 889–894. doi: 10.1007/s00338-016-1462-8
Urbina-Barreto, I., Chiroleu, F., Pinel, R., Fréchon, L., Mahamadaly, V., Elise, S., et al. (2020). Quantifying the shelter capacity of coral reefs using photogrammetric 3D modelling, from colonies to reefscapes. Ecol. Indicat. 121:107151. doi: 10.1016/j.ecolind.2020.107151
Vallès, H., Oxenford, H. A., and Henderson, A. (2019). Switching between standard coral reef benthic monitoring protocols is complicated: proof of concept. PeerJ. 2019, 1–24. doi: 10.7717/peerj.8167
Wedding, L. M., Jorgensen, S., Lepczyk, C. A., and Friedlander, A. M. (2019). Remote sensing of three-dimensional coral reef structure enhances predictive modeling of fish assemblages. Remote Sens. Ecol. Conser 5, 150–159. doi: 10.1002/rse2.115
Weinberg, S. (1981). A comparison of coral reef survey methods. Bijdrag. Tot. de Dierk. 51, 199–218.
Williams, I. D., Couch, C. S., Beijbom, O., Oliver, T. A., Vargas-Angel, B., Schumacher, B., et al. (2019). Leveraging automated image analysis tools to transform our capacity to assess status and trends on coral reefs. Front. Mar. Sci. 6, 1–14. doi: 10.3389/fmars.2019.00222
Wilson, S. K., Graham, N. A. J., and Polunin, N. V. C. (2007). Appraisal of visual assessments of habitat complexity and benthic composition on coral reefs. Mar. Biol. 151, 1069–1076. doi: 10.1007/s00227-006-0538-3
Keywords: coral cover, LIT, orthomosaic, reef survey methods, structural complexity, underwater photogrammetry
Citation: Urbina-Barreto I, Garnier R, Elise S, Pinel R, Dumas P, Mahamadaly V, Facon M, Bureau S, Peignon C, Quod J-P, Dutrieux E, Penin L and Adjeroud M (2021) Which Method for Which Purpose? A Comparison of Line Intercept Transect and Underwater Photogrammetry Methods for Coral Reef Surveys. Front. Mar. Sci. 8:636902. doi: 10.3389/fmars.2021.636902
Received: 02 December 2020; Accepted: 27 April 2021;
Published: 24 May 2021.
Edited by:
Manuel Gonzalez-Rivero, Australian Institute of Marine Science (AIMS), AustraliaReviewed by:
Chris M. Roelfsema, The University of Queensland, AustraliaCopyright © 2021 Urbina-Barreto, Garnier, Elise, Pinel, Dumas, Mahamadaly, Facon, Bureau, Peignon, Quod, Dutrieux, Penin and Adjeroud. This is an open-access article distributed under the terms of the Creative Commons Attribution License (CC BY). The use, distribution or reproduction in other forums is permitted, provided the original author(s) and the copyright owner(s) are credited and that the original publication in this journal is cited, in accordance with accepted academic practice. No use, distribution or reproduction is permitted which does not comply with these terms.
*Correspondence: Isabel Urbina-Barreto, aXNhYmVsLnVyYmluYS1iYXJyZXRvQHVuaXYtcmV1bmlvbi5mcg==
Disclaimer: All claims expressed in this article are solely those of the authors and do not necessarily represent those of their affiliated organizations, or those of the publisher, the editors and the reviewers. Any product that may be evaluated in this article or claim that may be made by its manufacturer is not guaranteed or endorsed by the publisher.
Research integrity at Frontiers
Learn more about the work of our research integrity team to safeguard the quality of each article we publish.