- 1South China Sea Fisheries Research Institute, Chinese Academy of Fishery Sciences, Guangzhou, China
- 2Southern Marine Science and Engineering Guangdong Laboratory, Guangzhou, China
- 3College of Marine Sciences, Shanghai Ocean University, Shanghai, China
- 4National Fishery Resources and Environment Dapeng Observation and Experimental Station, Shenzhen, China
Yellowfin seabream, Acanthopagrus latus, is one of the most important species in terms of stock enhancement in China. However, using metagenomic techniques to explore the feeding habits and stomach microbiome of yellowfin seabream is still rare. The objective of this work was to study the feeding habits and stomach microbiome of yellowfin sea bream from Daya Bay through metagenomic analysis of different weight classes (≤50, 50–100, and >100 g). Whole-metagenome shotgun sequencing and morphological observation were used to investigate the stomach contents. The dietary composition and the community composition of the stomach microbiome of A. latus were examined. In this study, 153 species were detected in the eukaryotic composition of the stomach contents of yellowfin sea bream. At the species level, Mytilus edulis was the only species identified by both metagenomic analysis and morphological observation. The proportion of fish and bivalves was over 98%, but the diet changed little with body size. Larimichthys crocea, Scophthalmus maximus, and Seriola dumerili were the most abundant species among all samples. In total, 285 species were identified in the stomach microbiome of yellowfin sea bream. Bacterium 2013Ark19i, bacterium 2013Arg42i and Acinetobacter baumannii, first reported in the stomach contents of yellowfin sea bream, were the most abundant species of the stomach microbiomes. There was no difference in the biodiversity of the stomach microbiomes among the different body sizes. Overall, the composition of the yellowfin sea bream diet mainly consists of fish and bivalves. The use of metagenomics techniques is a promising approach for assessing the feeding habits of yellowfin sea bream. The results derived from this study can provide important information for evaluating the feeding ecology of yellowfin sea bream in Daya Bay.
Introduction
The feeding behavior of fish regulates the material and energy flow of the ecosystem and is also an important way to link ecological composition with ecological function. Related research in this area has focused on marine food webs (Hong et al., 2012; Xi et al., 2015). To assess the nutritional structure of fish communities and the trophic levels of each fish in a community, as well as the interaction between various trophic levels, a comprehensive and in-depth study of the food chain and the material cycle of the food net must first focus on the feeding habits of fish (Dou, 1992, 1996; Xi et al., 2015; Qin et al., 2020).
Identification of the stomach contents of fish is an important part of determining the feeding habits of fish and food web construction (Xi et al., 2015). At present, the main methods of food analysis include stomach content analysis, the stable isotope method, the indoor feeding method and the direct observation method (Hong et al., 2012; Xi et al., 2015; Mo et al., 2017b; Liu et al., 2020). Stomach content analysis is used to understand food information by morphologically identifying the food composition; however, a large amount of work is still needed, and quickly digested prey items cannot be detected (Xue et al., 2004; Xi et al., 2015). Compared to morphological identification, DNA-based assessment has a range of advantages: (a) higher specificity and sensitivity at which prey DNA can be detected and identified; (b) the ability to standardize the methodology; (c) the ability to verify the food and parasites detected via DNA sequences; and (d) the possibility of employing high-throughput analyses (Traugott et al., 2020). With the development of molecular technology and the reduction of costs, an increasing number of scholars have used metagenomic techniques to explore the feeding habits of fish (Barnett et al., 2010; Hong et al., 2012; Leray et al., 2013, 2015, 2019; Xi et al., 2017; Yoon et al., 2017; Lin et al., 2018; Kodama et al., 2020). Barnett et al. (2010) performed a genetic analysis on the stomach contents of the broadnose sevengill shark (Notorynchus cepedianus) and collected gastric content samples by gastric lavage, which not only protected the research subjects but also allowed analysis of the diet to produce a more accurate understanding. Leray et al. (2015) used the metabarcoding approach to initially understand the diet and function of coral-predatory fish that feed on small invertebrates. Kodama et al. (2020) explored the diet of Pacific bluefin tuna (Thunnus orientalis) juveniles in two nursery farms by combining 16S amplicon sequencing and morphological observation. However, there are still some challenges and limitations when using metagenomic techniques to study feeding habits. The first challenge is related to technical settings. To process a large number of samples, it is necessary to provide high-quality diagnostic laboratories, high-throughput technologies, and laboratory procedures. Standardized sample processing is also required to avoid variability caused by different sample processing (Xi et al., 2015, 2017; Traugott et al., 2020). In addition to technical issues, the lack of detailed information on the methods provided in molecular dietary studies is another challenge for wet and dry laboratory procedures, greatly hindering the comparability of data throughout the study, which constitutes a major flaw in the use of the rapidly growing nutritional information generated by DNA technology (Stephen et al., 2009; Traugott et al., 2020).
The gastrointestinal (GI) tract of animals is composed of a very complex and dynamic microbial ecosystem, which is very important from the perspective of nutrition, physiology and pathology (Nayak, 2010). The GI microbiotas exert a variety of functions in the host. They play an important role in the nutrition and health of the host by promoting nutrient supply, preventing the colonization of infectious agents, participating in energy homeostasis, and maintaining normal mucosal immunity (Nayak, 2010; Klára et al., 2016; Dabrowski et al., 2020; Lukiw, 2020). The gut microbes of fish are considered to have an important impact on the life activities of the host, and the dominant microorganisms are usually bacteria (Deng et al., 2019). The bacterial community in the gut of fish, especially the colonized inherent microflora, is an indispensable and important part of the host fish (Chen et al., 2018). The bacterial community plays an important role in improving the digestion, nutrient absorption efficiency and immune defense function of the host (Austin, 2006; Round and Mazmanian, 2009; Nayak, 2010; Wei et al., 2010). A considerable part of the bacteria in the fish GI tract comes from the surrounding water environment, soil or sediment and food eaten (Nayak, 2010). The structure and diversity of gut flora of the same fish species will also change due to different growth stages, water environment and feeding habits (McDonald et al., 2012; Wong and Rawls, 2012; Bolnick et al., 2014; Miyake et al., 2015; Chen H. et al., 2019). The structural composition of intestinal microbes of a host fish can reflect their nutritional health level, habitat and diet (Chen et al., 2018). Currently, an increasing number of scholars are using metagenomic technology to explore the relationship between fish feeding habits and intestinal microorganisms to provide basic theoretical knowledge for the study of fish feeding ecology (Ghanbari et al., 2015; Chen et al., 2018; Chen H. et al., 2019). The community composition may be different between segments of the GI tract in fish (Llewellyn et al., 2014; Egerton et al., 2018). It has been suggested that the autochthonous microbiota may be different, considering the differences in physiological environments between the different parts of the digestive tract (Clements et al., 2014; Egerton et al., 2018). The stomach is often omitted from gut microbial composition analyses (Egerton et al., 2018). However, there have also been some studies using culture-independent techniques to compare the microbial communities in different gut segments, including the stomach (Paula et al., 2011; Estruch et al., 2015).
The yellowfin sea bream Acanthopagrus latus is a warm-water bottom fish that lives in shallow waters. It commonly inhabits coastal waters and estuaries and prefers rocky areas. Generally, yellowfin sea bream does not travel long distances (Xu, 1983). It has strong adaptability, as it can live at a water temperatures of 4–35°C and adapt to rapid changes in salinity, living normally in freshwater, brackish water and sea water (Wang, 2012). It is widely distributed in the Red Sea, the Arabian Sea, India, Indonesia, North Korea, Japan, the Philippines, and the south-eastern coast of China (Xu, 1983). Yellowfin sea bream is considered a marine fish species of economic importance and the most important species for stock enhancement on the coast of Guangdong Province in China (Xu, 1983; Chen et al., 2015; Liu et al., 2019; Wang et al., 2019). Generally, A. latus attains maturity ca. 24.5 cm, and the maximum body length and weight of A. latus can reach 35.0 cm and 350 g (Li et al., 1985; Zhang et al., 1991; Platell et al., 2007; Shi et al., 2012).
Many scholars have studied the feeding habits of yellowfin sea bream via morphological observation. Zhang et al. (1991) reported that yellowfin sea bream mainly fed on Macrura, Lamellibranchia and fish in Xipu Bay in China. Platell et al. (2007) found yellowfin sea bream preferred to feed on mangrove material, crabs, small gastropods and mytilid Brachidontes ustulatus in Shark Bay in Western Australia. Some studies of the intestinal tract of yellowfin sea bream have been conducted through culture-based approaches and culture-independent techniques (Zhou et al., 1996; Wang et al., 2018; Lin et al., 2020). However, using metagenomic techniques to explore the feeding habits and the stomach microbiome of yellowfin sea bream is still rare.
The present study was conducted in Daya Bay, which is a semi-enclosed subtropical bay surrounded by mountains on three sides located north of the South China Sea and east of the Pearl River Estuary and includes a variety of natural habitats, such as coral reefs, mangroves, rocks and other reefs (Xu, 1989; Yu et al., 2015; Qin et al., 2019). Yellowfin sea bream is an important species for stock enhancement in Daya Bay; however, the recovery of resources is still not satisfactory, even though stock enhancement activities have been carried out many times (Liu et al., 2019; Wang et al., 2019).
This study aimed to detect whether the metagenomic technique is an effective approach to provide the first detailed information on the feeding habits and stomach microbiome of A. latus using a small number of specimens. The dietary composition and the community composition of the stomach microbiome of A. latus were examined. The results derived from this study can provide important information for evaluating the feeding ecology of yellowfin sea bream in Daya Bay.
Materials and Methods
Stomach Content Sample Collection
Stomach content samples from nine yellowfin sea bream caught in gill nets were collected from Daya Bay, China (22°30′∼22°51′N, 114°30′∼114°50′E) in February 2019 (Figure 1). The stomach content samples were divided into three groups: small (S), medium (M), and large (L), i.e., ≤50, 50–100, and >100 g, according to the size fish from which they were derived (Table 1).
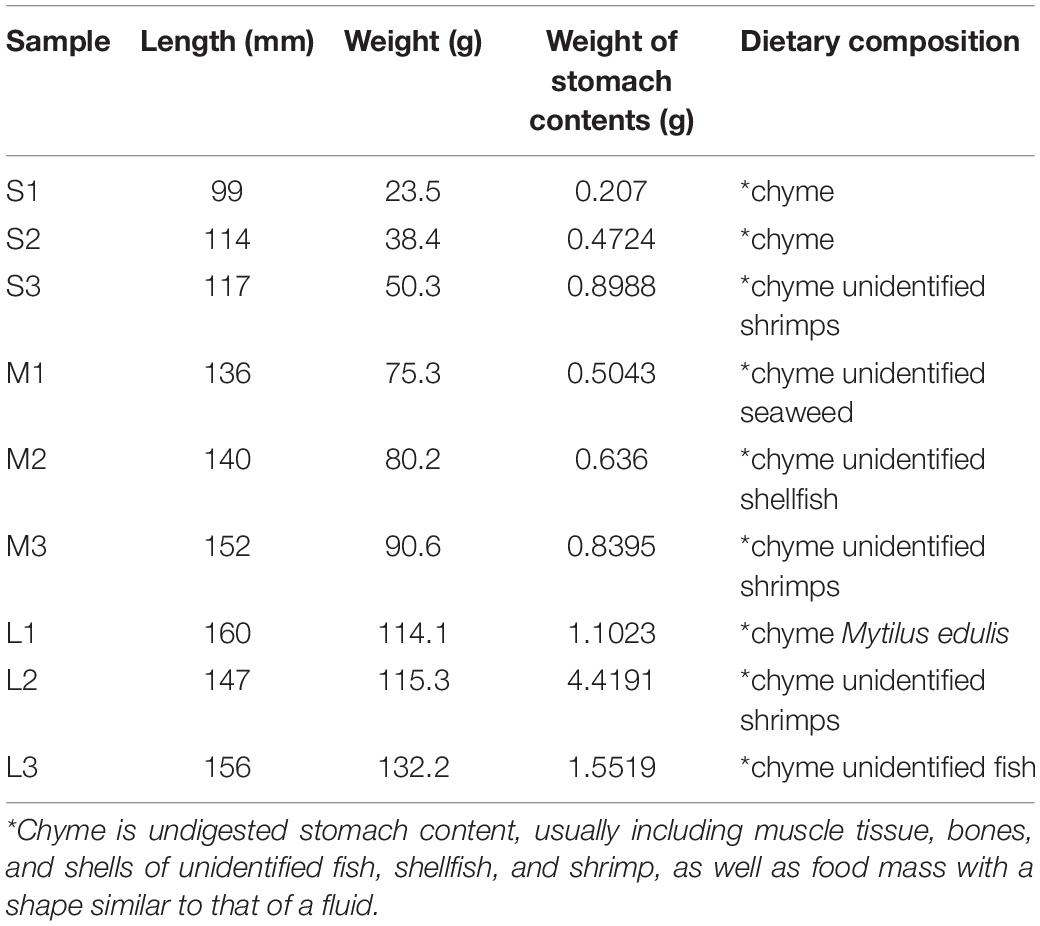
Table 1. The information on the collected fish individuals and the results of the morphological observation.
The collected yellowfin sea bream were stored in an incubator filled with ice for refrigerated storage and then transported to the laboratory for analysis. Before the experiment, we sterilized the scalpels, tweezers, and scissors. At the same time, we used 75% alcohol to wipe the surface of the fish, as well as the desktop and instruments used in the experiment, to disinfect the fish and the experimental supplies. Under aseptic conditions, the yellowfin sea bream were dissected, and their stomachs were taken out and dissected for morphological observation. The bodyweights of yellowfin sea bream and the weights of the stomach content samples were recorded. After taking pictures, all the stomach contents were collected in sterile tubes and immediately placed in liquid nitrogen. The samples were stored in the laboratory at −80°C for further metagenomic shotgun sequencing analysis. Based on the data collected by demersal trawls of previous fishery resource surveys in Daya Bay in 2015, 2017, and 2018 (Mo et al., 2017a, b; Wang et al., 2019; Zeng et al., 2019; Xu et al., 2020), the biological resource species list was obtained for species identification through morphological observation (Supplementary Table 1). Animal studies were carried out in the South China Sea Fisheries Research Institute, Chinese Academy of Fishery Sciences. All experimental procedures were approved by the Laboratory Animal Welfare and Ethics Committee of South China Sea Fisheries Research Institute, Chinese Academy of Fishery Sciences (nhdf2020-02).
DNA Extraction, Library Preparation and Sequencing
Metagenomic DNA was extracted from the stomach content samples of A. latus using the E.Z.N.A.® stool DNA Kit (Omega Bio-Tek, Norcross, GA, United States) according to the manufacturer’s protocols. Metagenomic shotgun sequencing libraries were constructed and sequenced at Mingke Biotechnology Co., Ltd. (Hangzhou, China). In brief, for each sample, 1 μg of genomic DNA was sheared by a Covaris S220 focused-ultrasonicator (Woburn, MA, United States), and sequencing libraries were prepared with a fragment length of approximately 450 bp. All samples were sequenced in the Illumina HiSeq X instrument in paired-end 150 bp (PE150) mode. Raw sequence reads underwent quality trimming using Trimmomatic1 to remove adaptor contaminants and low-quality reads (Li and Zhu, 2009). The reads with low-quality data removed were called clean reads and used for further analysis.
Metagenomic de novo Assembly, Gene Prediction and Annotation and Sequence Analysis
Clean sequence reads were generated from a set of contigs of each sample using MegaHit2 with “–min-contig-len 500” parameters (Li et al., 2008). The open reading frames (ORFs) of assembled contigs were predicted using Prodigal (v2.6.3) (Qin et al., 2010), and all ORFs were generated with a set of unique genes after clustering using CD-HIT (parameters: -n 9 -c 0.95 -G 0 -M 0 -d 0 -aS 0.9 -r 1) (Li and Godzik, 2006). The longest sequence of each cluster was considered the representative sequence of each gene in the unique-gene set. To calculate the gene abundance within the total samples, salmon software (Tatusov et al., 2003) was applied to obtain the read number for each gene. Finally, the gene abundance was calculated using the following formulas:
Ab(S) represents the gene abundance; Ab(U) represents the single-mapping read abundance; Ab(M) represents the multimapping read abundance; and l represents the length of the gene sequence (Li et al., 2008).
The unique-gene set was searched against the Non-Redundant Protein Sequence (NR) Database using Blastp (BLAST Version 2.2.28+, e-value = 1e-53), and the species annotation was obtained through the taxonomic information database corresponding to the NR database. All reads were classified into seven phylogenetic levels (domain, phylum, class, order, family, genus, and species) or unclassified. Then, we used custom Perl scripts to determine the abundance (TPM) of all samples (Hu et al., 2020). The abundance profile was constructed at the corresponding taxonomic level. The unique-gene set was searched against the KEGG database using Blastp to identify the proteins and retrieve their functional annotations (Kanehisa and Goto, 2000). Based on the KO results of nine samples, the specific functions and pathways of each sample were obtained using the pathway mapped by the annotated genes using the KEGG pathway database. Predicted genes were transformed to amino acid sequences to make comparisons with the carbohydrate-active enzymes (CAZy) database (Lombard et al., 2014) and eggNOG database (Jensen, 2008) using DIAMOND (Buchfink et al., 2014).
LEfSe analysis was conducted using the online tool LEfSe4 to identify communities or species of the stomach microbiome with significant differences among groups (Segata et al., 2011). Alpha diversity analysis was performed to investigate the compositional and functional variation in the microbial communities across samples using Quantitative Insights into Microbial Ecology (QIIME) software and visualized via the Shannon diversity index5. Based on the standardized Bray-Curtis dissimilarity matrix, principal coordinate analysis (PCoA) was conducted to compare community compositions among the samples. Permutational ANOVA (PERMANOVA) and one-way ANOVA were used to test whether the significant variation in the dietary compositions and microbial diversity and function were due to body weight. Statistically significant differences were established at P < 0.05.
Results
Composition of the Yellowfin Sea Bream Diet
Stomach Content Analysis
In total, nine stomachs from Daya Bay were dissected, and the average body weight of yellowfin sea bream and wet weight of the stomach contents were 79.99 ± 37.20 and 1.18 ± 1.28 g, respectively. In this study, most of the stomach content samples were determined to have unidentified dietary compositions via morphological observation. At the species level, only Mytilus edulis was identified by morphological observation, and the partially digested food composition could not be identified. Unidentified fish, shellfish, shrimp and seaweed were considered to constitute the dietary compositions of the yellowfin sea bream diet (Table 1 and Supplementary Figures 1–6).
Whole-Metagenome Shotgun Sequencing Analysis
The analysis resulted in 64.4 Gb of raw sequencing data obtained via whole-metagenome shotgun sequencing. The raw sequencing data for all samples have been deposited to Sequence Read Archive6 under BioProject accession PRJNA663846.
In the study, in total, seven phyla were identified among all the eukaryotes of the stomach content samples. Based on the number of reads, all seven phyla were designated major phyla (Figure 2A). The PERANOVA results showed that there was no significant difference in the diet of yellowfin sea bream according to the size groups (P > 0.05).
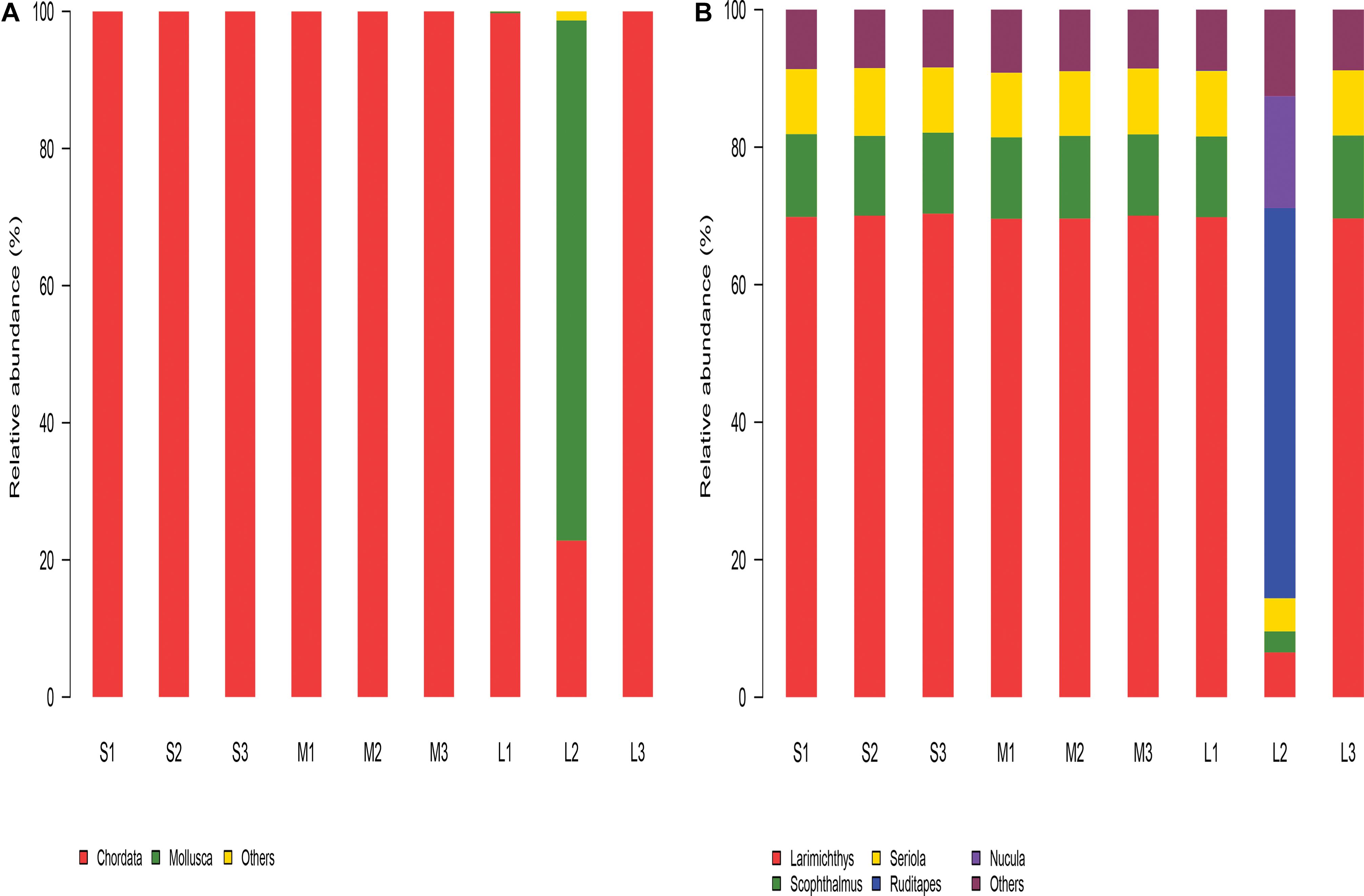
Figure 2. The relative abundance of the eukaryotic composition in the stomach content samples. (A) Relative abundance of the eukaryotic composition at the phylum level. (B) Relative abundance of the eukaryotic composition at the genus level.
The phyla, genera, or species that were most abundant in the samples were considered dominant. Among all nine samples, Chordata and Mollusca were the dominant phyla, accounting for over 98% of the stomach contents of A. latus. In groups S and M, Chordata was the most abundant phylum of all six samples, accounting for over 99%. In group L, Chordata was the most abundant phylum in all samples except sample L2, and Mollusca (75.79%) was the most abundant phylum in sample L2. The relative abundance of Chordata decreased and that of Mollusca increased significantly in group L compared with groups S and M.
At the genera level, Larimichthys, Scophthalmus, Seriola, and Oreochromis were the predominant genera in all nine samples. Ruditapes, Nucula, and Larimichthys were the predominant genera in sample L2 (Figure 2B).
The PCoA based on Bray-Curtis distances revealed the difference in the composition of the yellowfin sea bream stomach contents among the nine fish samples, but this difference was not statistically significant (R2 = 0.26185, P = 0.074). At the species level, PC1 explained 98.44% of the total variance, whereas PC2 explained 1.38% (Figure 3A). In this study, sample L2 was distinctly separated from the cluster of the other 8 samples.
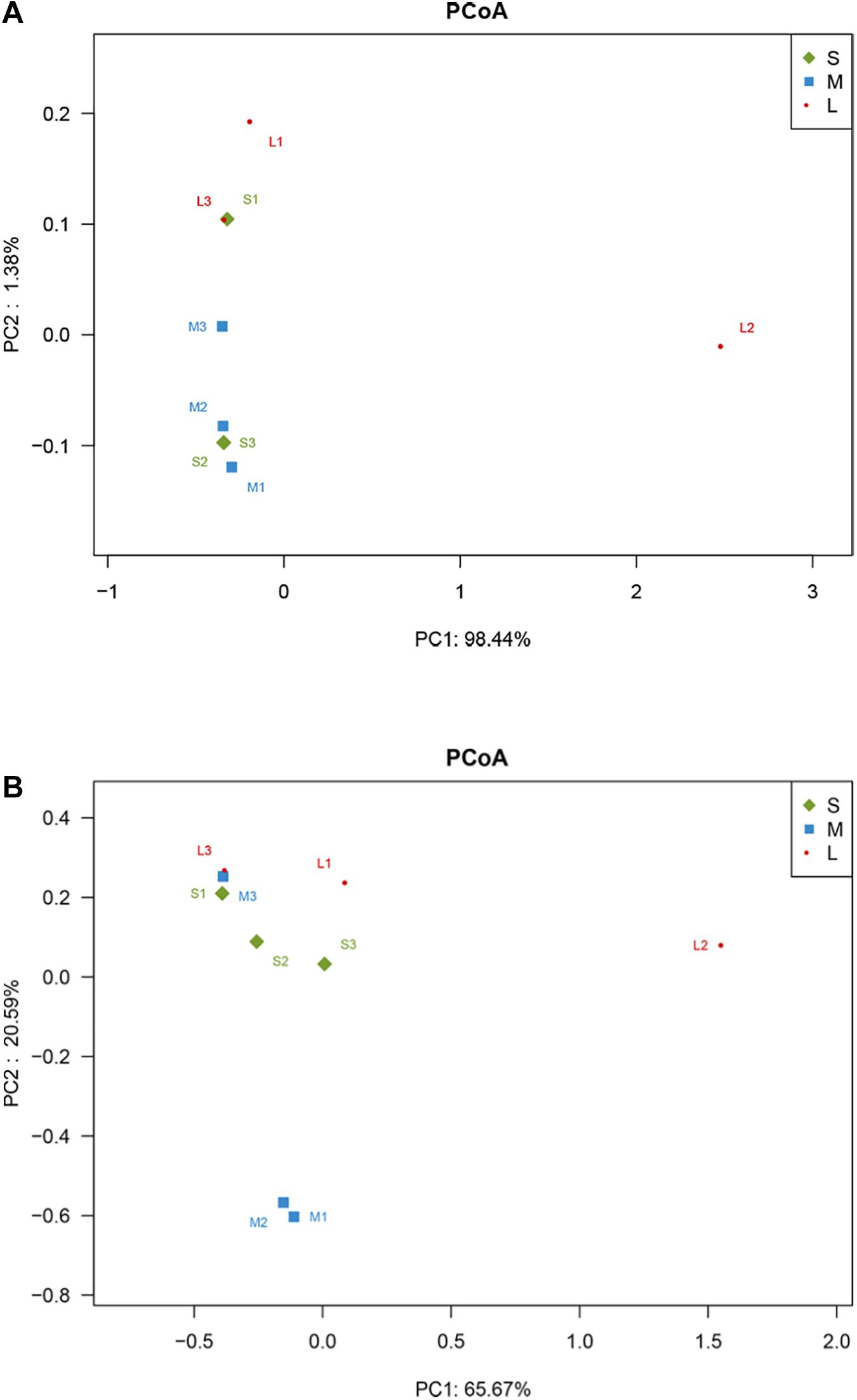
Figure 3. PCoA plot of Bray-Curtis distances between samples. (A) Eukaryotic composition of the yellowfin sea bream stomach contents. (B) Microbiome of the yellowfin sea bream stomach contents.
In this study, the dietary composition data from the sampled stomach contents were obtained via morphological identification and whole-metagenome shotgun sequencing. At the species level, 153 species were identified. Larimichthys crocea, Scophthalmus maximus, Seriola dumerili, Paralichthys olivaceus, Oreochromis niloticus, and Pagrus major were the predominant species among all nine samples (Supplementary Table 2). The main potential components of the yellowfin sea bream diet in Daya Bay were identified (Table 2). Fish, shellfish, shrimp, cephalopods and algae were the main components of the yellowfin sea bream diet. Animals accounted for a large proportion of the dietary composition. Yellowfin sea bream mainly fed on animals and rarely fed on plants.
Taxonomic Characterization of the Stomach Microbiome of Yellowfin Sea Bream
Whole genes from the stomach content samples were sequenced to study the bacterial community structure in the stomach contents of wild-caught A. latus.
In total, 20 phyla were identified via whole-metagenome shotgun sequencing of the stomach microbiota from these yellowfin sea bream.
Bacteria_norank (40.94%), Proteobacteria (34.55%), Firmicutes (18.37%), Chlamydiae (2.52%), Bacteroidetes (1.47%), and Actinobacteria (0.66%) were the main components of the stomach microbiome of the yellowfin sea bream in all samples, and the compositions showed differences, with increased Bacteria_norank and reduced Proteobacteria and Firmicutes levels in group M compared to those in group S and group L. Proteobacteria and Actinobacteria levels increased, and Bacteria_norank and Firmicutes levels decreased in group L compared to those in group S and group M (Figure 4A).
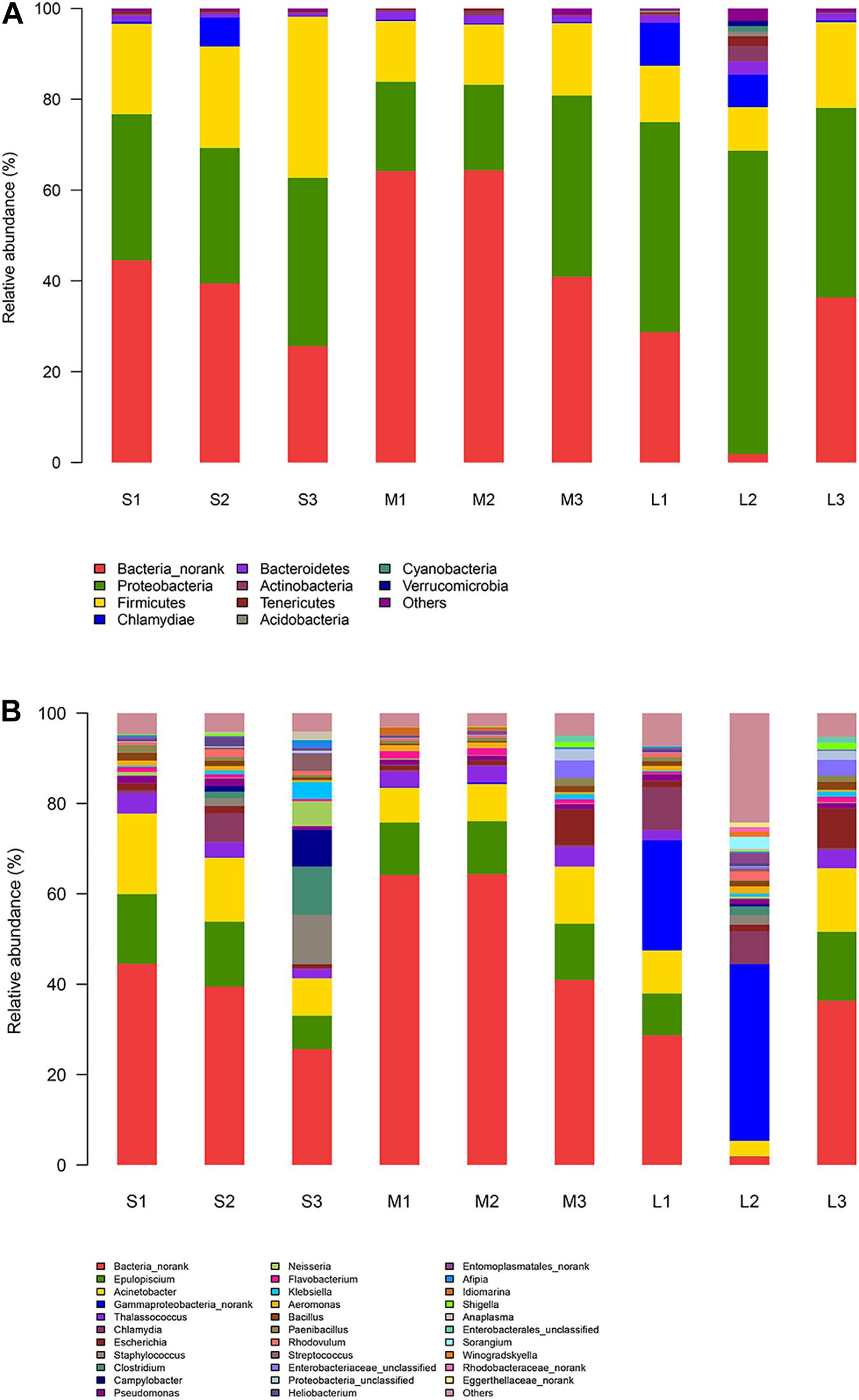
Figure 4. Relative abundance of the microbiota in the stomach content samples. (A) Relative abundance of the microbiota at the phylum level. (B) Relative abundance of the microbiomes at the genus level.
At the genus level, in total, 174 genera were identified. Bacteria_norank (40.94%), Epulopiscium (10.64%), Acinetobacter (10.00%), Gammaproteobacteria_norank (6.14%), Thalassococcus (3.00%), and Chlamydia (2.52%) were the predominant genera identified in next-generation sequencing of the stomach microbiota of the yellowfin sea bream. A total of two genera showed statistically significant differences among the three groups, i.e., Enterobacter and Pasteurella. The microbial composition changes in group S and group L compared to those in group M were as follows: Bacteria_norank and Wallemia decreased, and Brochothrix, Syntrophococcus, and Acetomicrobium increased (Figure 4B).
The phyla, genera, or species that were most abundant in the stomach microbiomes of all samples were considered dominant. At the species level, in total, 285 species were identified (Supplementary Table 3). Bacterium 2013Ark19i (Bacteria_norank) (25.39%), bacterium 2013Arg42i (Bacteria no_rank) (15.27%), Acinetobacter baumannii (9.79%), Epulopiscium sp. SCG-C07WGA-EpuloA2 (4.37%), Thalassococcus sp. WRAS1 (3.00%), and Solemya velum gill symbiont (2.83%) were the predominant species identified via whole-metagenome shotgun sequencing of the microbes from the yellowfin sea bream. A total of four species showed statistically significant differences among the three groups, i.e., Enterobacter cloacae, Enterococcus hirae, Pasteurella multocida, and Epulopiscium sp. AS2M-Bin002.
In group S, bacterium 2013Ark19i (17.53%), bacterium 2013Arg42i (16.38%), Acinetobacter baumannii (12.00%), Staphylococcus aureus (5.67%), and Epulopiscium sp. SCG-C07WGA-EpuloA2 (5.34%) were the dominant species. In group M, bacterium 2013Ark19i (40.90%), bacterium 2013Arg42i (18.45%), Acinetobacter baumannii (8.80%), Epulopiscium sp. SCG-C07WGA-EpuloA2 (4.17%) and Thalassococcus sp. WRAS1 (3.68%) were the dominant species. In group L, bacterium 2013Ark19i (12.41%), Solemya velum gill symbiont (9.99%), bacterium 2013Arg42i (9.40%), Bathymodiolus platifrons methanotrophic gill (8.73%), and Acinetobacter baumannii (8.61%) were the dominant species.
Interestingly, bacterium 2013Ark19i (27.78%), bacterium 2013Arg42i (16.69%), and Acinetobacter baumannii (10.46%) were the dominant species among the other eight samples, whereas the relative abundances of bacterium 2013Ark19i, bacterium 2013Arg42i and Acinetobacter baumannii accounted for 0.07, 0.19, and 2.73%, respectively, of sample L2. In sample L2, Solemya velum gill symbiont (18.79%), Bathymodiolus platifrons methanotrophic gill symbiont (10.62%), and Solemya pervernicosa gill symbiont (8.56%) were the dominant species.
The variation in the relative abundance of the top 50 species is shown by a heat map (Figure 5). Thirty-five species were significantly increased, and fifteen species were significantly decreased in the other eight samples compared with those in sample L2. Seven species were significantly increased in samples M1 and M2 compared with the remaining samples.
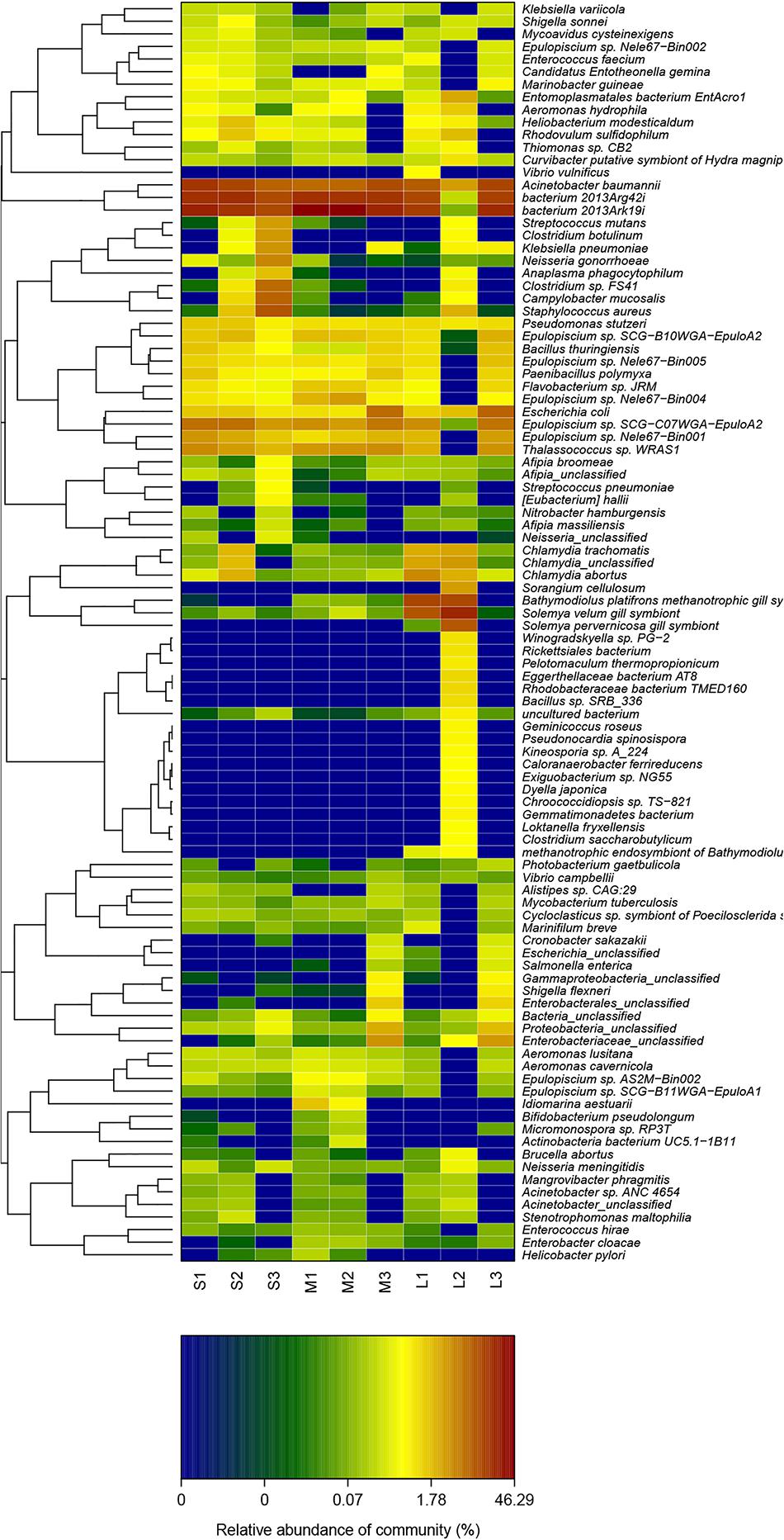
Figure 5. Heat map of the cluster analysis of the top 50 species in terms of relative abundance among the three groups.
The PERMANOVA results showed that there was no significant difference in the stomach microbiota of yellowfin sea bream among the three groups (P > 0.05).
The bacterial alpha diversity based on the Shannon index indicated that the diversity of the stomach microbiome in the stomach of group L was higher than that in the stomach of group S and group M. There was no significant difference in the biodiversity indices among the size groups (P = 0.05) (Figure 6).
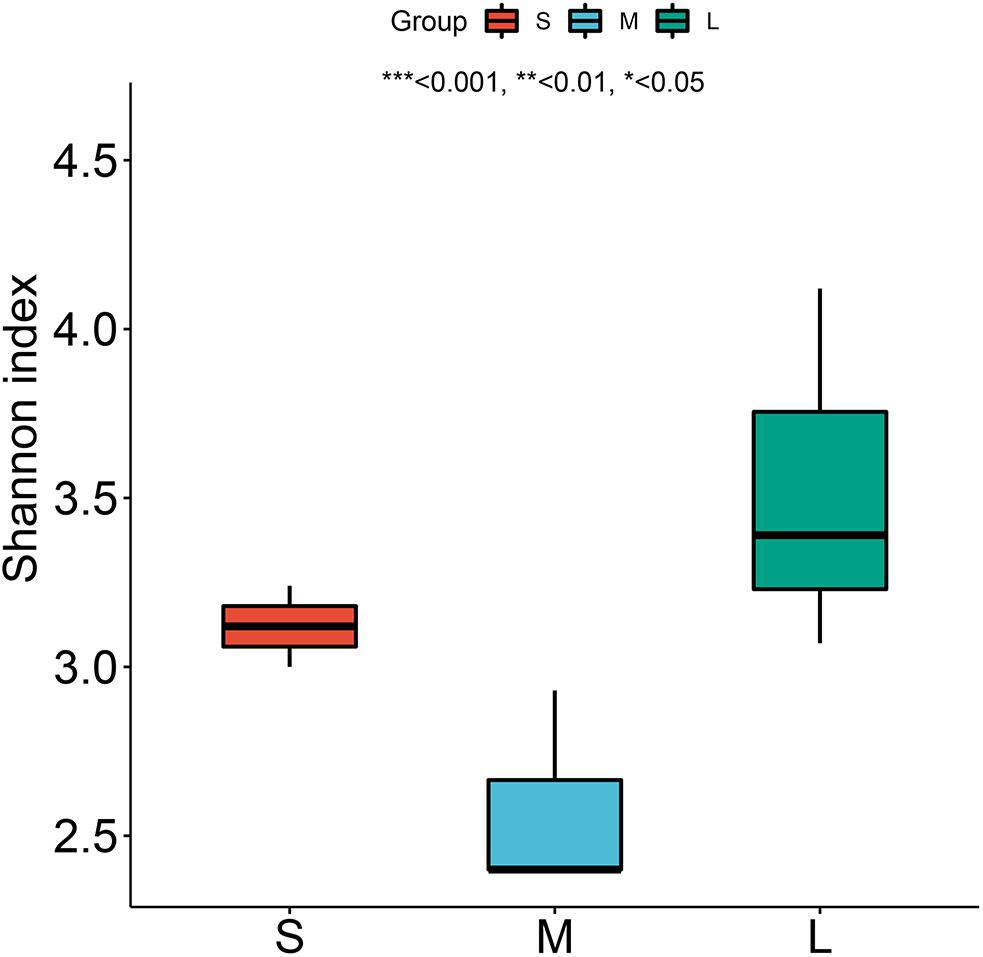
Figure 6. Shannon diversity index for yellowfin sea bream at the species level. * means P < 0.05, ** means P < 0.01, and *** means P < 0.001.
Principal coordinate analysis was used to compare the similarity in the microbial community compositions of nine specimens (Figure 3B). A scatter plot based on the PCoA scores showed the differences in the community composition among nine samples with different dietary compositions, but the differences were not statistically significant (R2 = 0.37728, P = 0.089). Sample L2 was distinctly separated from the cluster of the M1 and M2 samples, whereas other samples were clustered into one group. At the species level, PC1 explained 65.67% of the total variance, whereas PC2 explained 20.59% of the total variance.
In the study, we used linear discriminant analyses to identify the taxonomic biomarkers of the microbiomes of yellowfin sea bream among the three groups. The order Lactobacillales and the genera Pasteurella, Enterobacter, and Burkholderia were significantly different among the three groups. Within the phylum Firmicutes, the order Lactobacillales was abundant in group S. Within the phylum Proteobacteria, the genus Pasteurella was abundant in group S, the genus Enterobacter was abundant in group M, and the genus Burkholderia was abundant in group L (Figure 7).
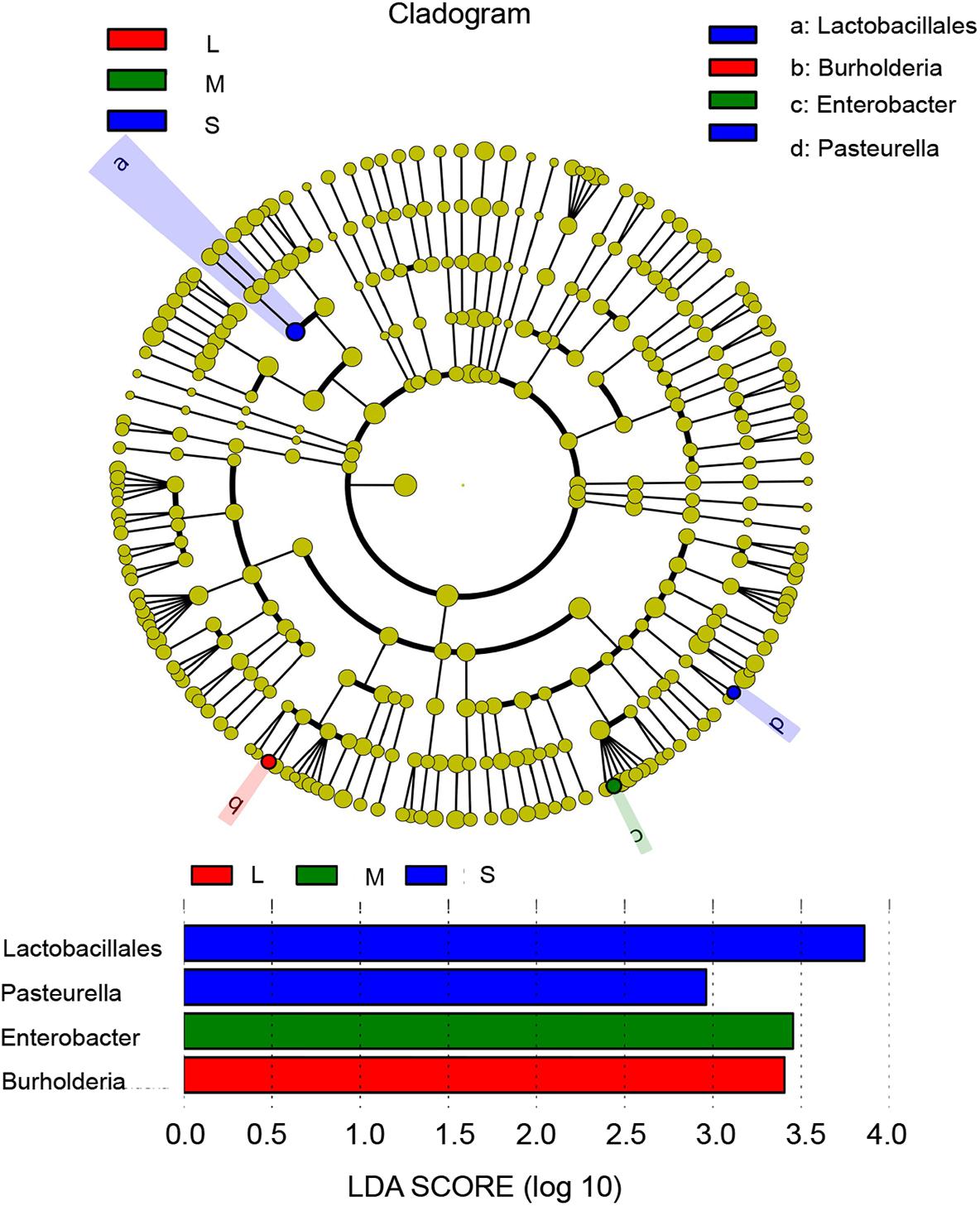
Figure 7. Differentially abundant taxa identified among samples of three sizes by linear discriminant analysis coupled with effect size (LEfSe) (LDA score > 2.0, P < 0.05). S, M, and L represent sizes. Blue box: enriched in S samples, green box: enriched in M samples, and red box: enriched in L samples.
Functional Characterization of the Stomach Microbiome of Yellowfin Sea Bream
Based on the KEGG pathway database, at level 2, global and overview mapping (22.65%), carbohydrate metabolism (6.39%), signal transduction (6.33%), immune system (5.51%), and amino acid metabolism (5.44%) were the predominant functions of the stomach microbiota of yellowfin sea bream identified via next-generation sequencing. The metabolism of other amino acids, signaling molecules and interactions, and the immune system were statistically significant among the three groups (P < 0.05).
The functional abundance changes in the three groups of yellowfin sea bream were as follows. Signal transduction, immune system, and infectious diseases from bacteria decreased, and global and overview mapping, carbohydrate metabolism, amino acid metabolism, and energy metabolism significantly increased in group L compared with group S and group M (Figure 8).
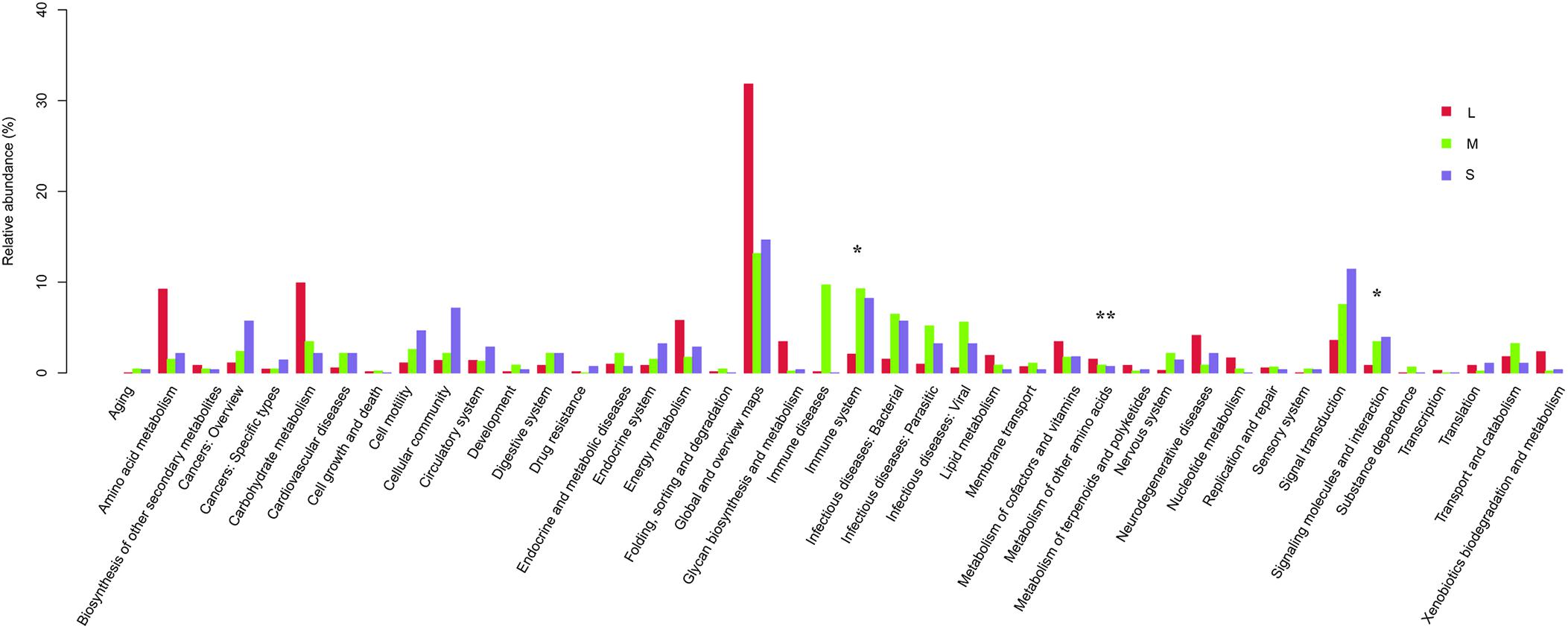
Figure 8. Comparison between the enriched KO markers at level 2 of the KEGG functional category for yellowfin sea bream. * means P < 0.05 and ** means P < 0.01.
At level 3, metabolic pathways (8.33%), biosynthesis of secondary metabolites (3.52%), microbial metabolism in diverse environments (3.50%), biosynthesis of antibiotics (2.48%), and regulation of actin cytoskeleton (2.15%) were the predominant functions identified.
Regarding general function prediction only, GO annotation of the unigenes obtained from sequencing revealed that replication, recombination and repair; posttranslational modification; protein turnover; chaperones; amino acid transport and metabolism; energy production and conversion; and carbohydrate transport and metabolism were the predominant gene functions in the stomach microbiota of yellowfin sea bream.
Based on the CAZy database, the main enzymes of the stomach microbiota of yellowfin sea bream were glycoside hydrolases (GHs), carbohydrate-binding molecules (CBMs), glycosyl transferases (GTs), and carbohydrate esterases (CEs).
Interestingly, 249 KO, 789 NOG, 76 COG, and five CAZy (family) showed statistically significant differences among the three groups. The variation tendencies in the relative abundances of the top 50 KO are shown in a heat map (Figure 9). Thirty-one KOs significantly increased, and 29 KOs significantly decreased in group L compared with those in groups S and M. Twenty-seven KOs significantly increased, and 23 KOs significantly decreased in the remaining eight samples compared with those in sample L2. Purine metabolism (K08041), vitamin digestion and absorption (K14619) and metabolic pathways (K05304) were the highest relatively abundant KOs associated with the stomach microbiota in yellowfin sea bream.
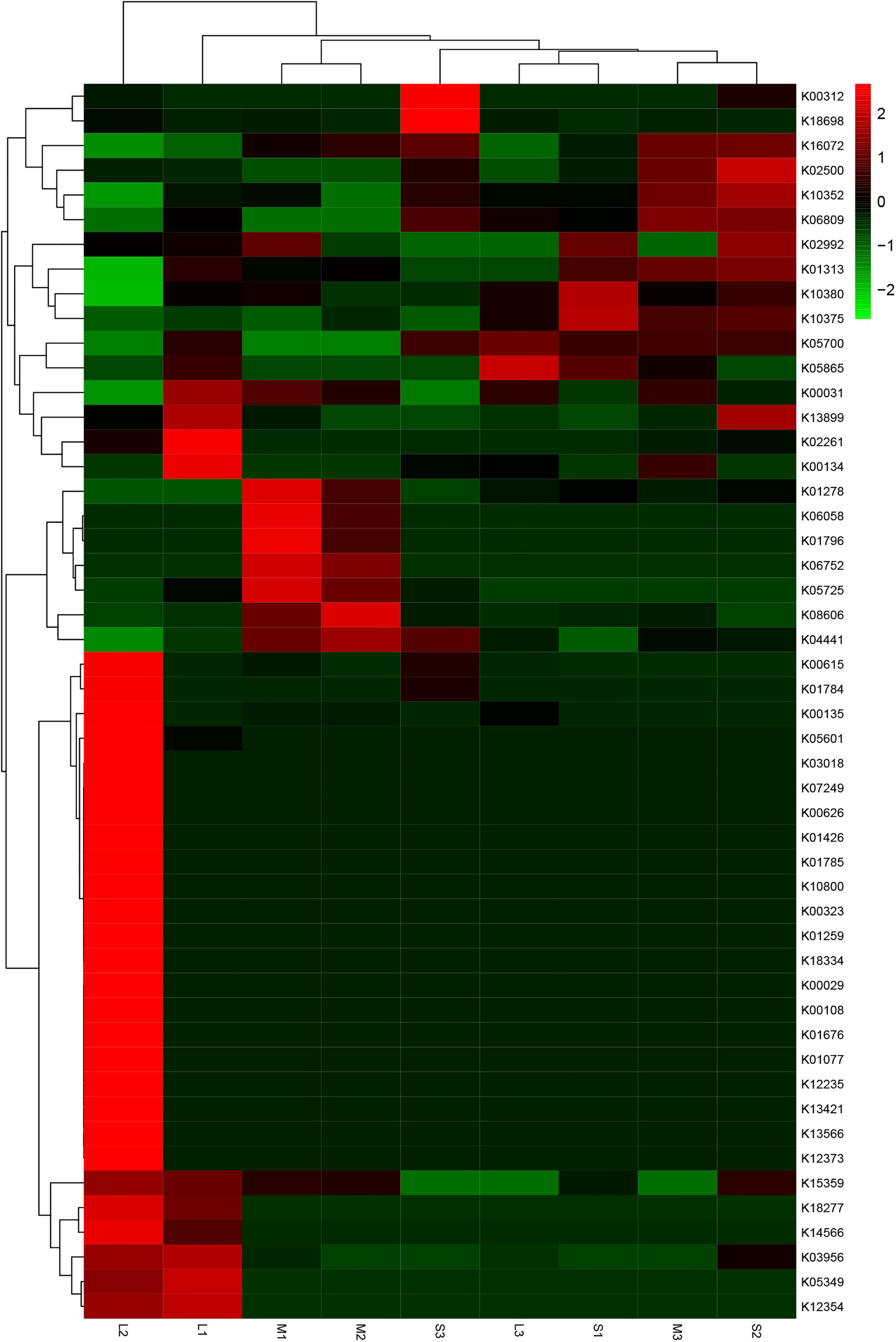
Figure 9. Heat map of clustering analysis of the top 50 KO in terms of relative abundance among the three groups. Red represents the species and KO with higher abundance in the corresponding sample, and green represents the species and KO with lower abundance.
Discussion
Is Metagenomic Analysis an Effective Way to Analyze Fish Food Habits?
In this study, morphological observation revealed unidentified dietary compositions for most of the stomach content samples. Fish, shellfish, shrimp and algae were identified as the main components of the yellowfin sea bream diet by morphological observation. However, at the species level, only Mytilus edulis was identified by both morphological identification and metagenomic whole-genome sequencing, and partially digested food could not be identified by morphological observation.
Metagenomic whole-genome sequencing technology was used to identify unidentifiable chyme. In total, 153 species were identified, which not only increased the dietary information about yellowfin seabream but also deepened the understanding of the resource situation in the sampled sea area, which is conducive to providing constructive suggestions for resource conservation and the proliferation of yellowfin sea bream in the future.
With a small number of specimens, in addition to Mytilus edulis, 152 new species were detected from the unidentifiable chyme through metagenomic whole-genome sequencing, outlining the diversity of the diet of yellowfin sea bream. Morphological identification is considered one of the traditional methods by which to explore the feeding habits but, still requires a large amount of work by skilled researchers, and a large number of samples and quickly digested prey items may not be identified (Xue et al., 2004; Xi et al., 2015). It is worth noting that all 153 detected species may not all be the prey of yellowfin sea bream. The food DNA detected in the dietary samples can either come directly from the food eaten by the consumer, the so-called primary prey, or stem from secondary prey, i.e., the food DNA contained in the diet of the primary prey (Sheppard et al., 2005; Traugott et al., 2020). As yellowfin sea bream take the prey into the stomach, the prey may bring the genetic material present in environmental samples, which is called environmental DNA (eDNA) (Taberlet et al., 2012; Bohmann et al., 2014; Barnes and Turner, 2015; Ficetola et al., 2016). eDNA may be obtained from the skin, mucous, blood, saliva, sperm, secretions, urine, eggs, roots, leaves, fruit, pollen, feces, and rotting bodies of larger organisms, and the microorganisms may be entirely obtained (Barnes and Turner, 2015; Ruppert et al., 2019). In the results of the identified species in the stomach contents of yellowfin sea bream, there are many species with extremely low abundance, e.g., seaweed (Supplementary Table 2). The tiny amounts of the seaweed detected in the stomach content may be considered eDNA from the environment (Ruppert et al., 2019; Traugott et al., 2020). The particularly broad diets of fish might be fraught with the problem of mixing eDNA and prey DNA, providing methodologically inflated food spectra (Traugott et al., 2020). Thus, dietary studies based on stomach samples of aquatic species should consider the interference of secondary predation and environmental contamination. If there is the possibility to have enough information on predators and their prey species within the examined food web, compiling the results twice – once excluding all potential secondary prey species and once including all prey detections – could be a practical solution (Traugott et al., 2020). Future studies would benefit from a consistent approach to this problem.
This article also explored the structure and diversity of the microbial flora of the stomach of yellowfin sea bream to improve information on the feeding habits of yellowfin sea bream. Abundant basic data on the microbial community of yellowfin sea bream were obtained using culture-independent techniques. The diet of the yellowfin sea bream mainly consisted of fish, bivalves and a tiny amount of seaweed. In the study, most of the identified genera in Proteobacteria, Firmicutes, and Bacteroidetes can produce proteinases, fatty acid enzymes, and chitinases that can help yellowfin sea bream digest prey (Zhou et al., 1996; Wu et al., 2015; Gong et al., 2017; Wu, 2017). In addition, pathogens, i.e., bacterium 2013Ark19i, bacterium 2013Arg42i and Acinetobacter baumannii, existed in the stomach of yellowfin sea bream and were the most abundant species. These pathogens were not reported in the previous studies of yellowfin sea bream (Xia et al., 2008; Seth-Smith et al., 2016).
Therefore, metagenomic sequencing analysis can be considered an effective method for analyzing the feeding habitats and stomach microbiomes of fish. The combination of metagenomic sequencing technology and other methods can provide technical support for better exploration of the dietary ecology of fish and enrich the theoretical basis of research on dietary ecology.
However, it is undeniable that there are some shortcomings in the use of metagenomic sequencing. For example, the use of DNA barcoding technology needs to be able to amplify the sequence information of the chyme without restriction of the identification object and assist in more accurate food analysis, a relatively complete database must be established in advance to meet the requirements of later comparative analysis. In addition, DNA barcoding technology can meet the requirements of only qualitative identification and cannot achieve quantitative analysis. Therefore, it is necessary to carry out quantitative analysis by morphological observation with a large number of specimens to obtain a more complete dietary analysis result. During the experiment, different primers need to be designed for different target genes when identifying different species. There may be genetic contamination or self-DNA interference (Leray et al., 2013), requiring the better design of primers. There are still errors in the use of high-throughput sequencing methods. On the other hand, the increasing detail of high-throughput sequencing and the increasing data output make it difficult for existing algorithms to fully utilize such a large amount of data (Wu et al., 2015).
Composition of the Yellowfin Sea Bream Diet in Daya Bay
In the present study, yellowfin sea breams in Daya Bay were partial to feeding on animal diets and only tiny amounts of benthic algae. The yellowfin sea bream diet mainly consisted of fish and bivalves.
The selectivity of fish for prey is mainly related to the types and numbers of feeders in the habitat (Hong et al., 2012). Daya Bay is rich in biological resources and diverse habitats (Qin et al., 2019). It is a spawning, feeding and rearing place for many economic fisheries with good biodiversity protection (Wang et al., 2019). Therefore, Daya Bay can provide a suitable habitat and rich fish prey for yellowfin sea bream to meet the needs of growth and development.
The PCoA based on Bray-Curtis distances revealed that there was no statistically significant difference in dietary composition for the nine yellowfin sea breams. In this study, sample L2 was distinctly separated from the cluster of the other eight samples—the volumetric contribution of bivalves to the diet of A. latus increased markedly, while that of fish clearly decreased. This may have been because yellowfin sea bream is highly opportunistic and depends on food availability in nature and selectivity (Platell et al., 2007; El-Naggar et al., 2019). Thus, there may have been abundant bivalves in the habitat of sample L2, while there were more abundant fish in the habitats of the other eight samples. In addition, as the samples were collected in the same season, the feeding habits of yellowfin sea bream may be relatively more affected by habitat (Platell et al., 2007). However, the study did not examine the feeding habits of yellowfin sea bream originating from different geographical areas and seasons. Related research will be conducted in the future. The feeding habits of yellowfin sea bream in Daya Bay are different from those in other areas. The prey of yellowfin sea bream in Xipu Bay, Fujian, China, mainly consists of Macrura and Lamellibranchia, followed by fish, benthic amphipods, posterior gills, polychaetes and benthic algae (Zhang et al., 1991). In Shark Bay in Western Australia, yellowfin sea bream predominantly feed on mangrove material, crabs and small gastropods in mangrove habitats and mainly feed on the mytilid Brachidontes ustulatus in rocky areas (Platell et al., 2007). The feeding habits of fish are affected by many factors, such as habitat, season, and size. In this study, the proportion of bivalves significantly increased, and the proportion of fish significantly decreased in group L compared with groups S and M, but there was no significant difference in the composition of the yellowfin sea bream diet at different body weights; thus, it is speculated that body weight may have no significant effect on their feeding habits. In the study of Platell et al. (2007), it was found that the feeding habits of yellowfin sea bream were not related to their body length but were more affected by habitat and season. In contrast, Sebastiscus marmoratus (<10, 10.0–13.9, and 14.0 cm length classes), Pagrus auratus, Pseudocaranx georgianus (<20, 20.0–40.0, and 40.0 cm length classes), and Acanthopagrus schlegelii (0.6–1.0, 1.1–1.5, 1.6–2.0, 2.1–2.5, 2.6–3.0, and 3.1–3.5 cm length classes) showed significant size-related dietary changes (Sarre et al., 2000; Nip et al., 2003; French et al., 2012; Wang K. et al., 2017). Larimichthys crocea, Scophthalmus maximus, Seriola dumerili, and Paralichthys olivaceus were the most abundant species identified in the stomach contents of yellowfin sea beam. These species were mostly abundant in the stomach contents of yellowfin sea bream and appeared more frequently. However, they were not abundant in the data from previous fishery resource surveys in this area due to the restriction from the gear selectivity and fishing methods, which are easily ignored in fishery resource investigations (Mo et al., 2017a, b; Wang et al., 2019; Zeng et al., 2019; Xu et al., 2020). Therefore, the species of food obtained in this study were more diverse, and fish was the main food component.
Larimichthys crocea was the dominant species in the bream diet in this study. They are seasonal migratory species that have multiple populations in China. After the autumn flood, fish schools enter the coast of Guangdong from the southern coast of Fujian and migrate from northeast to southwest. The fish reach the Raoping Offshore and the south-western coast of Nan’ao Island in September, appear in Shenquan and Jiazi in October, reach Shanwei in November, and arrive near Pinghai and Aotou Island (inside and outside of Daya Bay) in December. They begin to migrate to the sea in January (Qian, 2014). In Daya Bay, the large yellow croaker Larimichthys crocea spawn mainly in autumn (Zhang et al., 2011; Zheng et al., 2013), and the breeding period of yellowfin sea bream is from October to February of the following year (Zhang et al., 1991; Li and Ou, 2000; Shi et al., 2012). The seasonally migratory Larimichthys crocea provides seasonal food for yellowfin sea bream. During this period, the yellowfin sea bream is breeding, and the demand for food also increases. The sampling for this study occurred in February, and the collected yellowfin sea bream were all juveniles. The fry of the large yellow croaker hatched in autumn can provide seasonal food for the juveniles of yellowfin sea bream. Therefore, the large yellow croaker Larimichthys crocea is the most important prey species for yellowfin sea bream in this period.
In addition to feeding on fish, the yellowfin sea bream also fed on a large number of bivalves, mainly Ruditapes philippinarum, Nucula nucleus, Nucula sp. AL-2008 and Mytilus edulis. The A. latus individuals captured in this study were juveniles, and the structures of their digestive tracts were similar to those of adult fish (Platell et al., 2007; Wang, 2012). As the structure of its digestive organs adapts to its feeding habits, yellowfin sea bream can ingest hard or indigestible food that can be ground and digested in the stomach (Wang, 2012). In addition, a cannibalistic phenomenon of A. latus has been reported in previous studies (Xu, 1983; Shi et al., 2012), and in this study, DNA of yellowfin sea bream was detected (Supplementary Table 2). Therefore, there was no genetic contamination or self-DNA interference in this study (Leray et al., 2013).
Composition and Functional Structures of the Stomach Microbiome of Yellowfin Sea Bream
The intestinal microflora structure of aquatic animals is greatly influenced by the water quality and food (Li et al., 2017). The phylum Proteobacteria, in addition to Bacteroidetes and Firmicutes, comprise 90% of the intestinal microbiota of different marine fish species studied up to now (Ghanbari et al., 2015; Egerton et al., 2018).
In this study, Bacteria_norank (40.94%), Proteobacteria (34.55%), and Firmicutes (18.37%) were the dominant phyla of the stomach microbial composition of the yellowfin sea bream, which is consistent with the study of the intestinal microbial community of yellowfin seabream in Zhuhai, Guangdong Province (Lin et al., 2020). The dominant phyla and the contents of the microbial composition of the stomach of yellowfin sea bream were slightly different from the congeneric species black seabream, Acanthopagrus schlegelii, which prefer similar habitats and feeding habits to those of yellowfin sea bream (Chen D. et al., 2019; Deng et al., 2019). Firmicutes (57.3%), Proteobacteria (36.3%), Actinobacteria (2.4%) are the main phyla in black seabream Acanthopagrus schlegelii. Among them, the relatively abundant Firmicutes mainly includes 52.1% Bacilli and 5.1% Clostridia, and Proteobacteria mainly includes 26.3% Gammaproteobacteria and 5.6% Alphaproteobacteria (Deng et al., 2019).
Most of the genera in Proteobacteria, Firmicutes, and Bacteroidetes can produce proteinases and fatty acid enzymes (Wu, 2017). In this study, a large number of Proteobacteria, Firmicutes and Bacteroidetes existed, which can meet the digestion and absorption requirements of yellowfin sea bream. Most genera of Firmicutes and Bacteroidetes can produce enzymes that degrade plant cell walls and participate in the degradation and digestion of plant cell walls, enabling yellowfin sea bream to digest benthic algae (Wu et al., 2015). In addition, there are a large number of chitinase-producing microorganisms, such as Clostridium sp., Bacillus sp., Enterobacter sp., Aeromonas liquefaciens and Vibrio, in the stomach microflora of A. latus that can provide favorable conditions for A. latus to better digest the hard shell of shellfish (Zhou et al., 1996; Gong et al., 2017).
Ghanbari et al. (2015) used metagenomics to study the gut microbes of filter-feeding fish and compared them to the gut microbes of other fish-feeding fish. They found that filter-feeding fish and omnivorous fish have the highest types and number of intestinal flora and the most abundant structural compositions. In the gut of filter-feeding fish and omnivorous fish, there was not only the core microflora of Proteobacteria, Fusobacteria, and Firmicutes but also Actinobacteria and Cyanobacteria, which were rare in the gut of carnivorous and phytophagous fishes. This may be related to the fact that filter-feeding omnivorous fish can not only feed on common plant-insect larvae but can also filter individual tiny zooplankton, organic debris, bacterial aggregates, etc., greatly expanding the species on which they feed; thus, complex intestinal microorganisms are needed for decomposition and absorption (Zhai and Guo, 2016).
A large number of intestinal florae are distributed in the intestines of animals. After long-term evolution, these intestinal florae are closely related to the health and nutrition absorption of the host (Zhao and Tan, 2001; Li et al., 2017). Intestinal flora is affected by various factors, such as the host diet, genotype, age, disease, probiotics, drugs and living environment, which is a dynamic balance (Zheng et al., 2014; Li et al., 2017). According to the relationship with the host, the microbial flora in the intestinal tract of animals can be divided into three categories: commensal microbiota, pathogenic bacteria and opportunistic pathogenic bacteria. In general, the number of opportunistic pathogens is the highest, followed by that of commensal microbiota, and the number of pathogenic bacteria is the lowest (Zhou et al., 1996; Li et al., 2017). Environmental pressures, such as pollution, hypoxia, and sudden changes in temperature, can damage the immune system, causing pathogens to invade and change the intestinal microbial composition of the host (Chen et al., 2018).
In this study, a large number of pathogens identified in fish, such as bacterium 2013Ark19i (Bacteria_norank) (25.39%), bacterium 2013Arg42i (Bacteria no_rank) (15.27%), and Acinetobacter baumannii (9.79%) (Xia et al., 2008; Seth-Smith et al., 2016; Behera et al., 2017), existed in the stomach of yellowfin sea bream, and there were also many pathogenic microorganisms that affected fish health, such as Flavobacterium, Aeromonas, and Pseudomonas (Chen et al., 2018). However, the pathogenic bacteria reported in the intestinal tract of yellowfin sea bream, such as Streptococcus iniae, and Vibrio harveyi (Zhou et al., 1996; Wang et al., 2018), were not found in the stomach of yellowfin sea bream. Therefore, it is necessary to pay attention to the prevention and control of pathogenic diseases in the future in the process of seed selection, aquaculture and stock enhancement of yellowfin sea bream. The bacterial alpha diversity based on the Shannon index indicated that the biodiversity of the microbiota in the stomachs of group L was higher than that in the stomachs of group S and group M. There was no significant difference in the biodiversity indices between the fish samples (Figure 6). In group S and group M, bacterium 2013Ark19i, bacterium 2013Arg42i and Acinetobacter baumannii were the most abundant pathogens, while in group L, due to the ingestion of more bivalves, symbiotic bacteria such as Solemya velum gill symbiont, Bathymodiolus platifrons methanotrophic gill symbiont and Solemya pervernicosa gill symbiont, were obtained, reducing the abundance of pathogens and significantly increasing the bacterial diversity (Koito et al., 2010; Boutin et al., 2013; Dmytrenko et al., 2014; Reshma et al., 2018). Therefore, transient food may influence the structure of the microbial community of A. latus (Egerton et al., 2018; Reshma et al., 2018; Esmaeili et al., 2019).
Lactobacillales are common probiotics that can inhibit the growth of pathogenic bacteria by producing antibacterial substances such as organic acids, hydrogen peroxide and bacteriocin to strengthen the barrier function of host intestinal microorganisms and indirectly improve host non-specific immunity, thereby improving the health level and resistance (Wang T. et al., 2017). Enterobacter is a common pathogen in the gastrointestinal tract of marine fish that can produce cellulase and chitinase (Wang et al., 2014; Gong et al., 2017). Some bacteria of Burkholderia can produce a variety of metabolites with antibacterial activity, which have the functions of biological control, promoting plant growth and bioremediation (Gitaitis and Nischwitz, 2006). Lactobacillales and Pasteurella were significantly abundant in group S. Enterobacter was significantly abundant in group M, and Burkholderia was significantly abundant in group L (Figure 7).
In this experiment, there was no significant difference in the microbial community of A. latus with different body weights, which may have been due to the maturity level of the body and the gradual improvement in digestive function. In addition, the microbial population of fish gradually become enriched and stabilize (Palmer et al., 2007; Koenig et al., 2011; Wu et al., 2015). As the yellowfin sea bream samples captured in this study were at the same developmental stage and the stomach microbial community was relatively stable, the specifications had little effect on the microbial community of yellowfin sea bream (Platell et al., 2007; Wang, 2012; Zheng et al., 2013; Wu et al., 2015).
Principal coordinate analysis revealed individual fish variations in the stomach microbiome (Figure 3B). These variations may be attributable to the transient environmental effects (Sullam et al., 2012) or diet (Smith et al., 2015), although transient environmental effects were not examined in this study due to the wild-caught nature of the host and the similarity in the environmental variables between sampling sites of the present study. However, host genetics are also known to play a role in shaping the microbiota structure (Smith et al., 2015), and as a result, high variability between individuals is not uncommon in fish microbiota studies (Fjellheim et al., 2012; Larsen et al., 2015; Reshma et al., 2018). Sample L2 was separated from the left eight samples in the dietary composition. Furthermore, this fish also differed in its stomach microbial composition, separating it from the other samples on the PCoA plots. The results of the stomach microbiome should be treated with caution. The study used stomach contents for analysis, which are likely to be influenced by transient foods (Traugott et al., 2020).
Additionally, gut microbial communities have been demonstrated to play a large role in maintaining host health by increasing the digestion efficiency, boosting the immune system, and preventing the attachment and proliferation of opportunistic pathogens (Pérez et al., 2010). At the functional level, the functional structures of the stomach microbiomes of yellowfin sea bream among the three groups were significantly different. Signal transduction, immune system, and infectious diseases from bacteria significantly decreased, and global and overview mapping, carbohydrate metabolism, amino acid metabolism, and energy metabolism were increased in group L compared with group S and group M (Figure 8). Specifically, high relatively abundant KOs associated with the stomach microbiome of sample L2 compared with that of the left samples were as follows: dynein heavy chain, axonemal (K10408), alkaline phosphatase (K01077), trimethylamine monooxygenase (K18277), uridine monophosphate synthetase (K13421), and retinal dehydrogenase (K07249) were significantly increased, and myosin heavy chain (K10352), ankyrin (K10380) and cytochrome c oxidase subunit 2 (K02261) significantly decreased. These findings suggest that different dietary compositions may impact the composition and functional structures of the stomach microbiomes in yellowfin sea bream to a certain extent. However, we could not further explore the relationship between feeding habits and the stomach microbiome due to the characteristics of the samples, which were caught in a natural environment that was so complex and dynamic that we could not control the conditions. Therefore, related studies are needed to improve the experimental results.
Data Availability Statement
The datasets presented in this study can be found in online repositories. The names of the repository/repositories and accession number(s) can be found below: https://www.ncbi.nlm.nih.gov/, BioProject accession PRJNA663846.
Ethics Statement
The animal study was reviewed and approved by the Laboratory Animal Welfare and Ethics Committee of South China Sea Fisheries Research Institute, Chinese Academy of Fishery Sciences (nhdf2020-02).
Author Contributions
CQ, TZ, and GY conceived the study. TZ, WZ, HM, and SX led the collection the data. WP performed analyses and drafted the manuscript. CQ framed the manuscript and contributed to revisions. All authors gave final approval for publication.
Conflict of Interest
The authors declare that the research was conducted in the absence of any commercial or financial relationships that could be construed as a potential conflict of interest.
Acknowledgments
We would like to thank all colleagues for their generous support of the study. We also thank the editor and reviewers for their conductive comments on our present work.
Supplementary Material
The Supplementary Material for this article can be found online at: https://www.frontiersin.org/articles/10.3389/fmars.2021.634651/full#supplementary-material
Supplementary Figure 1 | The result of the morphological observation of sample L1.
Supplementary Figure 2 | The result of the morphological observation of sample M1.
Supplementary Figure 3 | The result of the morphological observation of sample M2.
Supplementary Figure 4 | The result of the morphological observation of sample L3.
Supplementary Figure 5 | The result of the morphological observation of sample S3.
Supplementary Figure 6 | The result of the morphological observation of sample S1.
Supplementary Table 1 | The species list of the aquatic organisms in Daya Bay.
Supplementary Table 2 | The abundance of identified species in the eukaryotic composition of the stomach contents.
Supplementary Table 3 | The abundance of identified species in the stomach microbiome of the stomach contents.
Footnotes
- ^ http://www.usadellab.org/cms/uploads/supplementary/Trimmomatic
- ^ https://github.com/voutcn/megahit
- ^ http://blast.ncbi.nlm.nih.gov/Blast.cgi
- ^ http://huttenhower.sph.harvard.edu/galaxy/root?Tool_id=lefse_upload
- ^ http://www.mothur.org/wiki/Shannon
- ^ http://www.ncbi.nlm.nih.gov/sra/
References
Austin, B. (2006). The bacterial microflora of fish, revised. Sci. World J. 6, 931–945. doi: 10.1100/tsw.2006.181
Barnes, M. A., and Turner, C. R. (2015). The ecology of environmental DNA and implications for conservation genetics. Conserv. Genet. 17, 1–17. doi: 10.1007/s10592-015-0775-4
Barnett, A., Redd, K. S., Frusher, S. D., Stevens, J. D., and Semmens, J. M. (2010). Non-lethal method to obtain stomach samples from a large marine predator and the use of DNA analysis to improve dietary information. J. Exp. Mar. Biol. Ecol. 393, 188–192. doi: 10.1016/j.jembe.2010.07.022
Behera, B. K., Paria, P., Das, A., Bhowmick, S., Sahoo, A. K., and Das, B. K. (2017). Molecular characterization and pathogenicity of a virulent Acinetobacter baumannii associated with mortality of farmed Indian Major Carp Labeo rohita (Hamilton 1822). Aquaculture 471, 157–162.
Bohmann, K., Evans, A., Gilbert, M., Carvalho, G., Creer, S., Knapp, M., et al. (2014). Environmental DNA for wildlife biology and biodiversity monitoring. Trends Ecol. Evol. 29, 358–367. doi: 10.1016/j.tree.2014.04.003
Bolnick, D. I., Snowberg, L. K., Hirsch, P. E., Lauber, C. L., Knight, R., Caporaso, J. G., et al. (2014). Individuals’ diet diversity influences gut microbial diversity in two freshwater fish (threespine stickleback and Eurasian perch). Ecol. Lett. 17, 979–987.
Boutin, J., Martin, N., Reverdin, G., Xiaoming, Y., and Fabienne, G. (2013). Sea surface freshening inferred from SMOS and ARGO salinity: impact of rain. Ocean Sci. 9, 183–192.
Buchfink, B., Xie, C., and Huson, D. H. (2014). Fast and sensitive protein alignment using diamond. Nat. Methods 12, 59–60.
Chen, D., Wang, T., Liu, Y., Xiao, Y., Wang, J., and Li, C. (2019). Biological study of reproductive characteristics of Acanthopagrus schlegeli in Daya Bay. South China Fish. Sci. 15, 41–47.
Chen, H., Li, C., Liu, T., Chen, S., and Xiao, H. (2019). A metagenomic study of intestinal microbial diversity in relation to feeding habits of surface and cave-dwelling sinocyclocheilus species. Microb. Ecol. 79, 299–311. doi: 10.1007/s00248-019-01409-4
Chen, H., Yan, H., Zhao, Y., Xiao, H., and Chen, S. (2018). Metagenomics and its advances in research of fish gut microbiome. Fish. Sci. 37, 699–706.
Chen, P., Qin, C., Yu, J., Shu, L., Li, X., Zhou, Y., et al. (2015). Evaluation of the effect of stock enhancement in the coastal waters of Guangdong, China. Fish. Manag. Ecol. 22, 172–180. doi: 10.1111/fme.12113
Clements, K. D., Angert, E. R., Montgomery, W. L., and Choat, J. H. J. M. E. (2014). Intestinal microbiota in fishes: what’s known and what’s not. Mol. Ecol. 23, 1891–1898.
Dabrowski, W., Siwicka-Gieroba, D., Kotfis, K., Terpilowska, S., and Siwicki, A. K. J. C. N. (2020). The brain-gut axis – where are we now and how can we modulate these connections? Curr. Neuropharmacol. 18, 1–15. doi: 10.2174/1570159X18666201119155535
Deng, H., Liu, F., Li, G., Bao, W., Zhou, S., Zheng, P., et al. (2019). Response of gut bacterial community in black sea bream to different feather meal in feed. Oceanol. Limnol. Sin. 50, 1309–1317. doi: 10.11693/hyhz20190400072
Dmytrenko, O., Russell, S. L., Loo, W. T., Fontanez, K. M., Liao, L., Roeselers, G., et al. (2014). The genome of the intracellular bacterium of the coastal bivalve, Solemya velum: a blueprint for thriving in and out of symbiosis. BMC Genom. 15:924. doi: 10.1186/1471-2164-15-924
Dou, S. (1996). Feeding ecology of fish-a critical review on heories, methods and their application. Oceanol. Limnol. Sin. 27, 556–561.
Egerton, S., Culloty, S., Whooley, J., Stanton, C., and Ross, R. P. (2018). The gut microbiota of marine fish. Front. Microbiol. 9:873. doi: 10.3389/fmicb.2018.00873
El-Naggar, H. A., Khalaf Allah, H. M. M., Masood, M. F., Shaban, W. M., and Bashar, M. A. E. (2019). Food and feeding habits of some Nile River fish and their relationship to the availability of natural food resources. Egypt. J. Aquat. Res. 45, 273–280. doi: 10.1016/j.ejar.2019.08.004
Esmaeili, A., Sotoudeh, E., Morshedi, V., Bagheri, D., and Dorafshan, S. (2019). Corrigendum to “Effects of dietary supplementation of bovine lactoferrin on antioxidant status, immune response and disease resistance of yellowfin sea bream (Acanthopagrus latus) against Vibrio harveyi”. Fish Shellf. Immunol. 93, 917–923. doi: 10.1016/j.fsi.2019.10.008
Estruch, G., Collado, M. C., Peñaranda, D. S., Vidal, A. T., Cerdá, M. J., Martínez, G. P., et al. (2015). Impact of fishmeal replacement in diets for gilthead sea bream (Sparus aurata) on the gastrointestinal microbiota determined by pyrosequencing the 16S rRNA gene. PLoS One 10:e0136389. doi: 10.1371/journal.pone.0136389
Ficetola, G. F., Taberlet, P., and Coissac, E. (2016). How to limit false positives in environmental DNA and metabarcoding? Mol. Ecol. Resour. 16, 604–607. doi: 10.1111/1755-0998.12508
Fjellheim, A. J., Playfoot, K. J., Skjermo, J., and Vadstein, O. (2012). Inter−individual variation in the dominant intestinal microbiota of reared Atlantic cod (Gadus morhua L.) larvae. Aquacult. Res. 43, 1499–1508.
French, B., Platell, M. E., Clarke, K. R., and Potter, I. C. (2012). Ranking of length-class, seasonal and regional effects on dietary compositions of the co-occurring Pagrus auratus (Sparidae) and Pseudocaranx georgianus (Carangidae). Estuar. Coas. Shelf Sci. 115, 309–325. doi: 10.1016/j.ecss.2012.09.004
Ghanbari, M., Kneifel, W., and Domig, K. J. (2015). A new view of the fish gut microbiome: advances from next-generation sequencing. Aquaculture 448, 464–475. doi: 10.1016/j.aquaculture.2015.06.033
Gitaitis, R., and Nischwitz, C. (2006). “Burkholderia,” in Plant-Associated Bacteria, ed. S. S. Gnanamanickam (Dordrecht: Springer Netherlands), 645–670.
Gong, F., He, C., Tang, X., and Liu, C. (2017). Research progress and application status ofmicrobial chitinase. China Brewing 36, 20–25.
Hong, Q., Zhuang, P., Yang, G., Zhang, T., Hou, J., Zhang, L., et al. (2012). Feeding habits of lateolabrax maculatus in yangtze river estuary. Acta Ecol. Sin. 32, 4181–4190.
Hu, T., Dai, Q., Chen, H., Zhang, Z., Dai, Q., Gu, X., et al. (2020). Geographic pattern of antibioticresistance genes in the metagenomes of the giant panda. Microb. Biotechnol. 1–12. doi: 10.1111/1751-7915.13655
Jensen, L.J.e.a (2008). eggNOG: automated construction and annotation of orthologous groups of genes. Nucleic Acids Res. 36, D250–D254.
Kanehisa, M., and Goto, S. (2000). KEGG: kyoto encyclopedia of genes and genomes. Nucleic Acids Res. 28, 27–30.
Klára, V., Gomez, A., Petrželková, K. J., Whittier, C. A., Todd, A. F., Yeoman, C. J., et al. (2016). Effect of antibiotic treatment on the gastrointestinal microbiome of free-ranging western lowland Gorillas (Gorilla g. gorilla). Microb. Ecol. 72, 943–954.
Kodama, T., Hirai, J., Tawa, A., Ishihara, T., and Ohshimo, S. (2020). Feeding habits of the Pacific Bluefin tuna (Thunnus orientalis) larvae in two nursery grounds based on morphological and metagenomic analyses. Deep Sea Res. Pt. II 175:104745. doi: 10.1016/j.dsr2.2020.104745
Koenig, J. E., Spor, A., Scalfone, N., Fricker, A. D., Stombaugh, J., Knight, R., et al. (2011). Succession of microbial consortia in the developing infant gut microbiome. Proc. Natl. Acad. Sci. U.S.A. 108 (Suppl. 1), 4578–4585. doi: 10.1073/pnas.1000081107
Koito, T., Nakamura-Kusakabe, I., Yoshida, T., Maruyama, T., Omata, T., Miyazaki, N., et al. (2010). Effect of long-term exposure to sulfides on taurine transporter gene expression in the gill of the deep-sea mussel Bathymodiolus platifrons, which harbors a methanotrophic symbiont. Fish. Sci. 76, 381–388. doi: 10.1007/s12562-010-0219-5
Larsen, A. M., Bullard, S. A., Womble, M., and Arias, C. R. (2015). Community structure of skin microbiome of gulf killifish, fundulus grandis, is driven by seasonality and not exposure to oiled sediments in a louisiana salt marsh. Microb. Ecol. 70, 534–544. doi: 10.1007/s00248-015-0578-7
Leray, M., Agudelo, N., Mills, S. C., and Meyer, C. P. (2013). Effectiveness of annealing blocking primers versus restriction enzymes for characterization of generalist diets: unexpected prey revealed in the gut contents of two coral reef fish species. PLoS One 8:e58076. doi: 10.1371/journal.pone.0058076
Leray, M., Alldredge, A. L., Yang, J. Y., Meyer, C. P., Holbrook, S. J., Schmitt, R. J., et al. (2019). Dietarypartitioning promotes the coexistence of planktivorous species oncoral reefs. Mol. Ecol. 28, 2694–2710. doi: 10.1111/mec.15090
Leray, M., Meyer, C. P., and Mills, S. C. (2015). Metabarcoding dietary analysis of coral dwelling predatory fish demonstrates the minor contribution of coral mutualists to their highly partitioned, generalist diet. PeerJ 3:e1047. doi: 10.7717/peerj.1047
Li, J., and Ou, Y. (2000). Studies on the reproductive biology of the pond-cultured Sparus latus Houttuyn in the coast of Shenzhen Bay. J. Zhej. Ocean Univ. (Nat. Sci.) 19, 139–143.
Li, J., Zhou, H., Xu, B., and Zheng, J. (1985). A preliminary study on the growth of yellowfin sea bream, Sparus latus (Houttuyn). J. South China Norm. Univ. (Nat. Sci. Ed.) 1, 114–121.
Li, R., Li, Y., Kristiansen, K., and Wang, J. (2008). SOAP: short oligonucleotide alignment program. Bioinformatics 24, 713–714.
Li, R., and Zhu, H. (2009). De novo assembly of the human genomes with massively parallel short read sequencing. Genome Res. 20, 265–272. doi: 10.1101/gr.097261.109
Li, W., and Godzik, A. (2006). Cd-hit: a fast program for clustering and comparing large sets of protein or nucleotide sequences. Bioinformatics 22, 1658–1659.
Li, Y., Chen, X., and Song, T. (2017). Differences in intestinal flora of cultured large yellow croaker Pseudosciaena crocea with different growth rates. J. Dal. Ocean Univ. 32, 509–513.
Lin, G., Zheng, M., Li, S., Xie, J., Fang, W., Gao, D., et al. (2020). Response of gut microbiota and immune function to hypoosmotic stress in the yellowfin seabream (Acanthopagrus latus). Sci. Total Environ. 745:140976. doi: 10.1016/j.scitotenv.2020.140976
Lin, X., Hu, S., Liu, S., and Huang, H. (2018). Comparison between traditional sequencing and high-throughput sequencing on dietary analysis of juvenile fish. Chin. J. Appl. Ecol. 29, 3093–3101.
Liu, K.-M., Lin, Y.-H., Chang, S.-K., Joung, S.-J., and Su, K.-Y. (2020). Examining an ontogenetic shift in the diet of the whitespotted bamboo shark Chiloscyllium plagiosum in northern Taiwanese waters. Reg. Stud. Mar. Sci. 35:101234. doi: 10.1016/j.rsma.2020.101234
Liu, Y., Wu, Z., Yang, C., Shan, B., Liu, S., and Sun, D. (2019). Ecological carrying capacity of six species of stock enhancement in Pearl River estuary based on Ecopath model. South China Fish. Sci. 15, 19–28. doi: 10.12131/20180265
Llewellyn, M. S., Boutin, S., Hoseinifar, S. H., and Derome, N. (2014). Teleost microbiomes: the state of the art in their characterization, manipulation and importance in aquaculture and fisheries. Front. Microbiol. 5:207. doi: 10.3389/fmicb.2014.00207
Lombard, V., Ramulu, H. G., Drula, E., Coutinho, P. M., and Henrissat, B. (2014). The carbohydrate-active enzymes database (CAZy) in 2013. Nucleic Acids Res. 42, D490–D495.
Lukiw, W. (2020). Gastrointestinal (GI) tract microbiome-derived neurotoxins—potent neuro-inflammatory signals from the GI tract via the systemic circulation into the brain. Front. Cell. Infect. Microbiol. 10:22. doi: 10.3389/fcimb.2020.00022
McDonald, R., Schreier, H. J., and Watts, J. E. M. (2012). Phylogenetic analysis of microbial communities in different regions of the gastrointestinal tract in Panaque nigrolineatus, a wood-eating fish. PLoS One 7:e48018. doi: 10.1371/journal.pone.0048018
Miyake, S., Ngugi, D. K., and Stingl, U. (2015). Diet strongly influences the gut microbiota of surgeonfishes. Mol. Ecol. 24, 656–672.
Mo, B., Qin, C., Chen, P., Diao, Y., Yuan, H., Li, X., et al. (2017a). Preliminary analysis of structure and function of Daya Bay ecosystem based on Ecopath model. South China Fish. Sci. 13, 9–19.
Mo, B., Qin, C., Chen, P., Li, X., Feng, X., Tong, F., et al. (2017b). Feeding habits of the purple sea urchin Heliocidaris crassispina based on stable carbon and nitrogen isotope analysis. J. Fish. Sci. China 24:16278. doi: 10.3724/sp.J.1118.2017.16278
Nip, T. H. M., Ho, W.-Y., and Kim Wong, C. (2003). Feeding ecology of larval and juvenile black seabream (Acanthopagrus schlegeli) and Japanese seaperch (Lateolabrax japonicus) in Tolo Harbour, Hong Kong. Environ. Biol. Fishes 66, 197–209.
Palmer, C., Bik, E. M., DiGiulio, D. B., Relman, D. A., and Brown, P. O. (2007). Development of the human infant intestinal microbiota. PLoS Biol. 5:e177. doi: 10.1371/journal.pbio.0050177
Paula, S.F.C.d, Robert, N. J., Luiz, Z.-I. J., Sadasivam, K., and Ecology, G. F.-J. J. F. M. (2011). Influence of the diet on the microbial diversity of faecal and gastrointestinal contents in gilthead sea bream (Sparus aurata) and intestinal contents in goldfish (Carassius auratus). FEMS Microbiol. Ecol. 78, 285–296.
Pérez, T., Balcázar, J. L., Ruiz-Zarzuela, I., Halaihel, N., Vendrell, D., de Blas, I., et al. (2010). Host-microbiota interactions within the fish intestinal ecosystem. Mucosal. Immunol. 3, 355–360. doi: 10.1038/mi.2010.12
Platell, M. E., Ang, H. P., Hesp, S. A., and Potter, I. C. (2007). Comparisons between the influences of habitat, body size and season on the dietary composition of the sparid Acanthopagrus latus in a large marine embayment. Estuar. Coas. Shelf Sci. 72, 626–634. doi: 10.1016/j.ecss.2006.11.026
Qin, C., Chen, P., Sarà, G., Mo, B., Zhang, A., and Li, X. (2020). Ecological implications of purple sea urchin (Heliocidaris crassispina, Agassiz, 1864) enhancement on the coastal benthic food web: evidence from stable isotope analysis. Mar. Environ. Res. 158:104957. doi: 10.1016/j.marenvres.2020.104957
Qin, C., Zhu, W., Ma, H., Duan, D., Zuo, T., Xi, S., et al. (2019). Are habitat changes driving protist community shifts A case study in DayaBay, China. Estuar. Coast. Shelf Sci. 227:106356. doi: 10.1016/j.ecss.2019.106356
Qin, J., Li, R., Raes, J., Arumugam, M., Burgdorf, K. S., Manichanh, C., et al. (2010). A human gut microbial gene catalogue established by metagenomic sequencing. Nat. Rev. Immunol. 464, 59–65. doi: 10.1038/nature08821
Reshma, K. J., Sumithra, T. G., Nair, A. V., Stefi Raju, V., Kishor, T. G., Sreenath, K. R., et al. (2018). An insight into the gut microbiology of wild-caught Mangrove Red Snapper, Lutjanus argentimaculatus (Forsskal, 1775). Aquaculture 497, 320–330. doi: 10.1016/j.aquaculture.2018.08.008
Round, J. L., and Mazmanian, S. K. (2009). The gut microbiota shapesintestinal immune responses during health and disease. Nat. Rev. Immunol. 9, 313–323.
Ruppert, K. M., Kline, R. J., and Rahman, M. S. (2019). Past, present, and future perspectives of environmental DNA (eDNA) metabarcoding: a systematic review in methods, monitoring, and applications of global eDNA. Global Ecol. Conserv. 17:e00547. doi: 10.1016/j.gecco.2019.e00547
Sarre, G., Platell, M. E., and Potter, I. C. (2000). Do the dietary compositions of Acanthopagrus butcheri (Sparidae) in four estuaries and a coastal lake vary with body size and season and within and amongst these water bodies? J. Fish Biol. 56, 103–122.
Segata, N., Izard, J., Waldron, L., Gevers, D., Miropolsky, L., Garrett, W. S., et al. (2011). Metagenomic biomarker discovery and explanation. Genome Biol. 12, R60–R60.
Seth-Smith, H. M. B., Dourala, N., Fehr, A., Qi, W., Katharios, P., Ruetten, M., et al. (2016). Emerging pathogens of gilthead seabream: characterisation and genomic analysis of novel intracellular β-proteobacteria. ISME J. 10, 1791–1803. doi: 10.1038/ismej.2015.223
Sheppard, S. K., Bell, J., Sunderland, K. D., Fenlon, J., Skervin, D., and Symondson, W. O. C. (2005). Detection of secondary predation by PCR analyses of the gut contents of invertebrate generalist predators. Mol. Ecol. 14, 4461–4468. doi: 10.1111/j.1365-294X.2005.02742.x
Shi, X., Shi, H., Lou, B., and Mao, G. (2012). Biological characteristics and artificial propagation, culture technique for Sparus latus (Houttuyn). Hebei Fish. 12, 52–55.
Smith, C. C., Snowberg, L. K., Gregory Caporaso, J., Knight, R., and Bolnick, D. I. (2015). Dietary input of microbes and host genetic variation shape among-population differences in stickleback gut microbiota. Isme J. 9, 2515–2526. doi: 10.1038/ismej.2015.64
Stephen, A. B. V. B., Garson, J. A., Hellemans, J., Huggett, J., Kubista, M., Mueller, R., et al. (2009). TheMIQE guidelines: minimum information for publication of quantitativereal-time PCR experiments. J. Clin. Chem. 55, 611–622.
Sullam, K. E., Essinger, S. D., Lozupone, C. A., O’Connor, M. P., Rosen, G. L., Knight, R., et al. (2012). Environmental and ecological factors that shape the gut bacterial communities of fish: a meta-analysis. Mol. Ecol. 21, 3363–3378. doi: 10.1111/j.1365-294X.2012.05552.x
Taberlet, P., Coissac, É, Pompanon, F., Brochmann, C., and Willerslev, E. (2012). Towards next-generation biodiversity assessment using DNA metabarcoding. Mol. Ecol. 21, 2045–2050. doi: 10.1111/j.1365-294X.2012.05470.x
Tatusov, R. L., Fedorova, N. D., Jackson, J. D., Jacobs, A. R., Kiryutin, B., Koonin, E. V., et al. (2003). The COG database: an updated version includes eukaryotes. BMC Bioinform. 4:41. doi: 10.1186/1471-2105-4-41
Traugott, M., Thalinger, B., Wallinger, C., and Sint, D. (2020). Fish aspredators and prey: DNA-based assessment of their role in food webs. J. Fish. Biol. 1–16. doi: 10.1111/jfb.14400
Wang, H., Huang, Y., Lin, C., and Qi, D. (2018). Isolation, identification and drug sensitivity test of Streptococcus iniae from Sparus latus. J. Anhui Agricult. Sci. 46:112.
Wang, K., Li, C., Wang, Z., Zhao, J., and Zhang, S. (2017). Feeding habits of the marbled rockfish Sebastiscus marmoratus in the marine ranching off Ma’an Archipelago. Chinese J. Appl. Ecol. 28, 2321–2326.
Wang, T., Sun, T., Xiao, Y., Liu, Y., Li, C., Chen, D., et al. (2019). Nested distribution patterns of fish assemblages in daya bay. Chin. J. Zool. 54, 327–338. doi: 10.13859/j.cjz.201903003
Wang, T., Wei, X., Chang, C., Han, H., and Jiang, H. (2017). Identification and characteristics of a lactic acid bacteria associated from intestine of black rockfish Sebastes schlegelii. Oceanol. Limnol. Sin. 48, 94–100.
Wang, W., Wu, S.g, Zhou, H., Zheng, Y., Cheng, Y., and Wang, G. (2014). Characterization of cellulose-decomposing bacteria in the intestine of grass carp, Ctenopharyngodon idella (VAL.). Acta Hydrobiol. Sin. 38, 291–297.
Wang, Y. (2012). Growing Development Characteristics on the Digestive Tract and Histology and Histochemistry of Wild and Cultivated Sparus Latus. Master thesis, Shanghai Ocean University, Shanghai.
Wei, H., Dong, L., Wang, T., Zhang, M., Hua, W., Zhang, C., et al. (2010). Structural shifts of gut microbiota as surrogate endpoints for monitoring host health changes induced by carcinogen exposure. FEMS Microbiol. Ecol. 73, 577–586. doi: 10.1111/j.1574-6941.2010.00924.x
Wong, S., and Rawls, J. F. (2012). Intestinal microbiota composition in fishes is influenced by host ecology and environment. Mol. Ecol. 21, 3100–3102. doi: 10.1111/j.1365-294x.2012.05646.x
Wu, C. (2017). Diversity of Cultivable Protease-Producing Bacteria and Lipase-Producing Bacteria in the Sediments of Bohai Sea and the North Yellow Sea. Master thesis, Lanzhou Jiaotong University, Lanzhou.
Wu, S., Zhang, Y., and Zan, L. (2015). Progress on the metagenomic based on high-throughput sequencing technique applicated in animal gastrointestinal microorganisms. Curr. Biotechnol. 5, 77–84.
Xi, X., Bao, B., and Zhang, S. (2015). Application of DNA barcoding in species analysis of fish stomach content. J. Shang. Ocean Univ. 24, 203–210.
Xi, X., Bao, B., and Zhang, S. (2017). Application of DNA barcoding in analyzing food composition of Belanger’s croaker (Johnius belangerii) in Ma’an Archipelago. J. Fish. China 41, 1533–1541. doi: 10.11964/jfc.20160310329
Xia, L., Xiong, D., Gu, Z., Xu, Z., Chen, C., Xie, J., et al. (2008). Recovery of Acinetobacter baumannii from diseased channel catfish (Ictalurus punctatus) in China. Aquaculture 284:288. doi: 10.1016/j.aquaculture.2008.07.038
Xu, S., Guo, J., Fan, J., Xu, Y., Li, L., and Li, C. (2020). Annual variation in fish biomass size spectrum in Daya Bay, South China Sea in summer. South China Fish. Sci. 16, 28–38.
Xue, Y., Jin, X., Zhang, B., and Liang, Z. (2004). Diet composition and seasonal variation in feeding habits of small yellow croaker Pseudosciaena polyactis bleeker in the central Yellow Sea. J. Fish. Sci. China 11, 237–243.
Yoon, T.-H., Kang, H.-E., Lee, S. R., Lee, J.-B., Baeck, G. W., Park, H., et al. (2017). Metabarcoding analysis of the stomach contents of the Antarctic Toothfish (Dissostichus mawsoni) collected in the Antarctic Ocean. PeerJ 5:e3977. doi: 10.7717/peerj.3977
Yu, J., Chen, P., Tang, D., and Qin, C. (2015). Ecological effects of artificial reefs in Daya Bay of China observed from satellite and in situ measurements. Adv. Space Res. 55, 2315–2324. doi: 10.1016/j.asr.2015.02.001
Zeng, L., Chen, G., Li, C., and Yu, J. (2019). Seasonal heterogeneity of nekton community and its ecological effect at Daya Bay mouth. South China Fish. Sci. 15, 22–32.
Zhai, W., and Guo, A. (2016). Research progress on the fish intestinal microbiota. Henan Fish. 4, 18–21.
Zhang, Q., Hong, W., Chen, Z., Chen, X., and Huang, Z. (1991). On age, growth and feeding habits of yellowfin seabream (Sparus latus) in Xipu Bay, Fujian. J. Oceanogr. Taiwan Strait 10, 68–76.
Zhang, Q., Hong, W., Yang, S., and Liu, M. (2011). Discussion on the division of geographic populations for the large Yellow croaker (Larimichthys crocea). Mod. Fish. Inform. 26, 3–8.
Zhao, Q., and Tan, Y. (2001). Distributions of intestinal bacteria in cyprinidate and relationship of symbiosis between fishes and intestinal microorganisms. Chin. J. Prevent. Vet. Med. 23, 51–54.
Zheng, Y., Hong, W., and Zhang, Q. (2013). Review and prospects for resource biology of main marine demersal food fishes along the coastal waters of China. J. Fish. China 37, 151–160. doi: 10.3724/SP.J.1231.2013.38253
Zheng, Y., Zhang, J., Guo, Z., and Zhang, H. (2014). Research progress on high-throughput sequencing to analysis the diversity of gut microbiota and its influence factors. J. Chin. Instit. Food Sci. Technol. 14, 157–164.
Keywords: yellowfin sea bream, metagenomic analysis, feeding habits, stomach microbiome, stomach content
Citation: Pan W, Qin C, Zuo T, Yu G, Zhu W, Ma H and Xi S (2021) Is Metagenomic Analysis an Effective Way to Analyze Fish Feeding Habits? A Case of the Yellowfin Sea Bream Acanthopagrus latus (Houttuyn) in Daya Bay. Front. Mar. Sci. 8:634651. doi: 10.3389/fmars.2021.634651
Received: 28 November 2020; Accepted: 11 January 2021;
Published: 03 February 2021.
Edited by:
Hui Zhang, Institute of Oceanology (CAS), ChinaReviewed by:
Xiangli Tian, Ocean University of China, ChinaMing Liu, Morgan State University, United States
Zhi Huang, Temasek Polytechnic, Singapore
Copyright © 2021 Pan, Qin, Zuo, Yu, Zhu, Ma and Xi. This is an open-access article distributed under the terms of the Creative Commons Attribution License (CC BY). The use, distribution or reproduction in other forums is permitted, provided the original author(s) and the copyright owner(s) are credited and that the original publication in this journal is cited, in accordance with accepted academic practice. No use, distribution or reproduction is permitted which does not comply with these terms.
*Correspondence: Chuanxin Qin, qincx@scsfri.ac.cn; Gang Yu, gyu0928@163.com