- 1NOAA Fisheries Service – Alaska Fisheries Science Center, Seattle, WA, United States
- 2Institute for Marine and Antarctic Studies and Centre for Marine Socioecology, University of Tasmania, Hobart, TAS, Australia
- 3NOAA Fisheries Service – Office of Science and Technology, Silver Spring, MD, United States
- 4NOAA Fisheries Service – Northeast Fisheries Science Center, Woods Hole, MA, United States
- 5NOAA Fisheries Service – Southeast Fisheries Science Center, Miami, FL, United States
- 6NOAA Fisheries Service – Northwest Fisheries Science Center, Seattle, WA, United States
- 7National Institute of Water and Atmospheric Research, Wellington, New Zealand
As ecosystem-based fisheries management becomes more ingrained into the way fisheries agencies do business, a need for ecosystem and multispecies models arises. Yet ecosystems are complex, and model uncertainty can be large. Model ensembles have historically been used in other disciplines to address model uncertainty. To understand the benefits and limitations of multispecies model ensembles (MMEs), cases where they have been used in the United States to address fisheries management issues are reviewed. The cases include: (1) development of ecological reference points for Atlantic Menhaden, (2) the creation of time series to relate harmful algal blooms to grouper mortality in the Gulf of Mexico, and (3) fostering understanding of the role of forage fish in the California Current. Each case study briefly reviews the management issue, the models used and model synthesis approach taken, and the outcomes and lessons learned from the application of MMEs. Major conclusions drawn from these studies highlight how the act of developing an ensemble model suite can improve the credibility of multispecies models, how qualitative synthesis of projections can advance system understanding and build confidence in the absence of quantitative treatments, and how involving a diverse set of stakeholders early is useful for ensuring the utility of the models and ensemble. Procedures for review and uptake of information from single-species stock assessment models are well established, but the absence of well-defined procedures for MMEs in many fishery management decision-making bodies poses a major obstacle. The benefits and issues identified here should help accelerate the design, implementation, and utility of MMEs in applied fisheries contexts.
Introduction
Ecosystem-based fisheries management (EBFM) and an ecosystem approach to fisheries (EAF)—hereafter collectively referred to as EBFM—are now firmly embedded in modern fisheries science (Link, 2010; Dolan et al., 2016). At its core, EBFM emphasizes the need to consider the broader ecological and social contexts of fisheries to better inform policy and decision-making. In practice, this requires conceptualizing the larger systems fisheries operate within and the use of models to formalize and represent important processes. The complexity of the models used to support EBFM reflects the different scales of its implementation, ranging widely from inclusion of environmental forcings in simple population models to community, food web, and coupled social–ecological models for evaluating system-level tradeoffs (O’Farrell et al., 2017; Geary et al., 2020). However, ecological systems are often only partially understood, and multiple models differing in structure or parameterization may provide plausible alternative representations (Gardmark et al., 2013; Geary et al., 2020). As the relevance of multispecies models has increased, so have calls for explicit consideration of model uncertainty (Hill et al., 2007; Addison et al., 2013; Geary et al., 2020), but efforts to develop sets of multispecies models to inform EBFM problems remain limited.
A set of distinct, plausible models may permit multimodel inference and be treated as an ensemble. Model ensembles are used for analysis and operational forecasting in many fields including weather (Tracton and Kalnay, 1993; Zhou and Du, 2010) and long-term climate prediction (Tebaldi and Knutti, 2007; Semenov and Stratonovitch, 2010), and ecological applications of multispecies model ensembles (MMEs) include projection of impacts due to climate change (Gardmark et al., 2013; Cheung et al., 2016; Reum et al., 2020), fishing (Spence et al., 2018), and species eradications and invasions (Baker et al., 2017). Methods for combining quantitative ensemble projections are diverse: from unweighted methods (e.g., “democracy of models”) to more complex approaches that weight models based on various criteria including level of data support, e.g., Bayesian posterior model probabilities (Burnham and Anderson, 2002; King et al., 2009; Ianelli et al., 2016; Spence et al., 2018). In general, model ensembles address structural uncertainty with the added benefit that ensemble forecast quantities of interest can be more accurate than estimates from individual ensemble members (Hagedorn et al., 2005; Zhou and Du, 2010).
However, the benefits of adopting multi-model approaches in an EBFM context may extend beyond statistical and predictive advantages. With regard to policy making, a set of models explicitly acknowledges model uncertainty, which promotes transparency (Addison et al., 2013), and inclusion of distinct models increases avenues for representing diverse hypotheses, incorporating different knowledge sources, and engaging with stakeholders, all of which may help to legitimize EBFM policies and decisions (Fulton et al., 2015; Francis et al., 2018). From a research perspective, assembling a diverse candidate model set often also means recruiting researchers with different perspectives and areas of technical expertise onto a modeling team. Doing so may foster an environment favorable to knowledge exchange and the cross-pollination of ideas. Moreover, qualitative syntheses, rigorous comparisons of model behavior, and evaluation of the role key assumptions have on predictions can yield deeper insight into a system and guide future data collection and modeling efforts (Gardmark et al., 2013; Cheung et al., 2016; Hollowed et al., 2020; Reum et al., 2020). These benefits are valuable in their own right and can be attainable even when quantitative, statistical treatment of ensemble outputs remains out of reach.
As noted, some examples of multispecies model ensembles (MMEs) for fisheries research have been published (Gardmark et al., 2013; Cheung et al., 2016; Reum et al., 2020). However, MMEs for fisheries management applications are limited. The few instances of management application can partly be attributed to the novelty of MMEs in fisheries modeling (Townsend et al., 2014), but other challenges may exist. Understanding the benefits and limitations of MMEs is important so that the approach can be more fully leveraged in fisheries management. Here, we sought to identify how ensemble modeling and multispecies applications, in particular, are applied in practice to address objectives and issues related to EBFM.
We focus our review on three representative case studies in the United States that have developed MMEs in response to specific management objectives and goals, and presented outcomes to decision-makers, managers, or stakeholders. For each case study, we ask the general question: what do we get from considering an ensemble of multispecies models within a management context? In particular, we evaluated how multispecies model suites (1) were synthesized and utilized, (2) facilitated engagement with stakeholders, management plan teams, and researchers, and (3) influenced the credibility of the output or advice derived from the modeling exercises. We highlight lessons from the case studies that should accelerate adoption and implementation of multispecies model ensembles in support of EBFM.
Case Studies
Atlantic Menhaden
To address the potential effects of fishing mortality on a forage fish and its predators, the Atlantic States Marine Fisheries Commission used an MME approach. A lead model from the MME was used with a stock assessment model to help set ecologically based reference points for fishing mortality. Synthesis of the model outputs from the set was qualitative because time constraints prevented quantitative synthesis. Open, transparent development of the models in the MME enabled stakeholder engagement and benefited the decision-making process.
Management Issue
Atlantic menhaden (Brevoortia tyrannus) is a small-bodied forage fish found in estuarine and nearshore habitats along the eastern coastline of the United States and Canada. Since the development of the first fishery management plan for Atlantic menhaden (herein “menhaden”) in 1981, fisheries managers have acknowledged the potentially significant role of menhaden as a prey base for other fish stocks managed by the Atlantic States Marine Fisheries Commission (ASMFC,, 1998). To better resolve predation on menhaden, an ecosystem model—Multispecies Virtual Population Analysis—Extended, MSVPA-X (Garrison et al., 2010), was developed in the early 2000s to generate mortality rates that were then used in a statistical catch-at-age stock assessment model. The paired modeling approach was routinely used for the two subsequent stock assessments, but its use was discontinued.
Concurrently, the Atlantic Menhaden Management Board began to consider the larger ecological role of menhaden in their decision-making and requested additional information on how menhaden removals by the fishery might affect predator populations. The original MSVPA-X, while resolving predation impacts on menhaden, did not relate predator productivity to menhaden abundance, and could not directly address the issue. In 2015, the ASMFC convened an Ecosystem Management Objectives Workshop to explicitly delineate the desired objectives for the menhaden fishery and tasked a workgroup to develop ecological reference points based on alternative multispecies models identified by a technical committee. The broad objectives were to (1) sustain the menhaden stock and provide for the fishery, (2) sustain menhaden to provide for predators, (3) provide stability for a variety of fisheries, and (4) minimize risk to sustainable yield for menhaden management (and management of menhaden predators) attributable to a changing environment. Additional detail on the performance measures associated with these objectives is available in ASMFC, (2015). A newly developed Ecological Reference Point Workgroup (herein “workgroup”) was convened following the Ecosystem Management Objectives Workshop to identify a modeling framework that would begin to address these objectives and performance measures. We highlight the multiple model aspects of the larger modeling and management processes, and direct interested readers to Anstead et al. (2020) for additional details regarding the history of the menhaden fishery, its management, and the modeling used to inform its management.
Model Set and Synthesis Approach
The workgroup sought a model framework that would address as many of the Ecosystem Management Objectives as possible as well as closely replicate the population abundance and fishing mortality rate patterns produced by the menhaden single species stock assessment model (SEDAR, 2020). Considerable uncertainty in predator responses to changes in the menhaden stock existed. To partly account for this, the workgroup identified a set of multispecies food web models that ranged in taxonomic complexity. Food web interactions in the models reflected the degree to which predator groupings were taxonomically resolved. In more complex food web models, the ability to represent age structure for all populations becomes difficult, so the workgroup also wanted to address uncertainty in age structure. Finally, as understanding environmental variability was an important objective, the workgroup preferred models that could address uncertainty in natural processes. Overall, the selected models reflected an emphasis on addressing uncertainty attributable to structural complexity and, to a lesser extent, to natural variability and parameter uncertainty.
The initial set of candidate models was assembled based on the expertise of the workgroup and other known existing models or models in development. One workgroup member had worked previously with a Surplus Production Model with time-varying r (SPM TVr), which can be useful for dealing with uncertainty in natural processes that drive stocks in production or mortality (Nesslage and Wilberg, 2019). The Steele-Henderson Surplus Production Model (SPM S-H) can help determine the importance of predators on menhaden population dynamics based on relative fits to data (Uphoff and Sharov, 2018). A multispecies statistical catch-at-age model (VADER—Virtual Assessment for the Description of Ecosystem Responses) included the age structure of prey and key predator stocks, but unlike MSVPA-X, modeled predation based on the consumption demand of predators (McNamee, 2018). Two Ecopath with Ecosim (EwE) models were also included in the model set. The first model included relatively fine taxonomic resolution (NWACS-Full, Northwest Atlantic Coastal Shelf) and was developed and parameterized by an academic partner (Buchheister et al., 2017a,b). The second model consisted of a scaled-down version of the first model (NWACS-MICE, Northwest Atlantic Coastal Shelf—Model of Intermediate Complexity for Ecosystem Assessment) and was composed of coarser taxonomic groupings. The latter model was easier to update with new data on an operational basis and was more computationally efficient and therefore amenable to parameter sensitivity analyses (Chagaris et al., 2020).
Initially, the workgroup intended to consider the proposed candidate models, review their structures, and select a single model to fully develop for use in setting ecological reference points (ERPs). Additional meetings were convened, so lead modelers could present an interim version of each candidate model and allow time for workgroup members to have hands-on experience with the models. Ultimately, the workgroup elected to move forward with fully developing all models, which would then be presented for review and potential use in setting ERPs individually or as an ensemble average. This decision was made because the members found that each model was useful for informing some subset of the ecosystem management objectives and provided some useful insights about the ecosystem. The fully developed models were parameterized or tuned using standardized, current data sets of biomass indices, harvests, and environmental variables.
All models were presented for Management Review (SEDAR, 2020). However, because of limited time for preparation and review, the working group opted to forego calculation of ensemble averages and instead focused on selecting a single model, NWACS-MICE, as the preferred model for setting ERPs (Figure 1). Since model review was scheduled for fall of 2019, the availability of the final data in late summer complicated updating data inputs for model development. Translating outputs (abundance/biomass and fishing mortality/exploitation rate) between model types (surplus production/biomass pool and age-structured) was a complex task that needed more time and consideration to complete. This additional layer would have also complicated the model review and would require more time than had been allotted for reviewers.
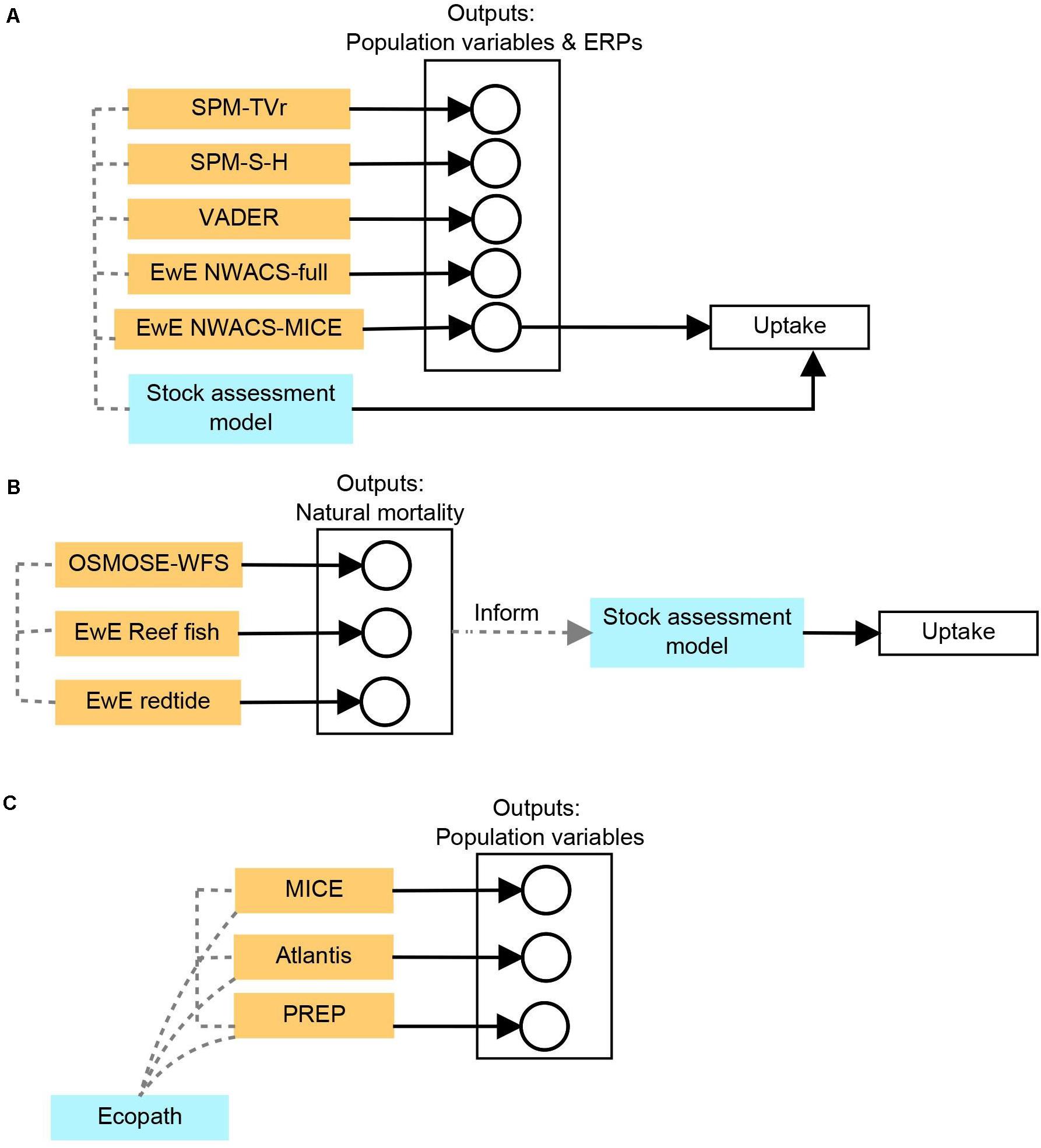
Figure 1. A visual summary of the model sets and qualitative synthesis approach used in each case study. (A) Atlantic menhaden, (B) Gulf of Mexico gag and red grouper, and (C) sardine and anchovy in the California Current. In all case studies, data sets used to calibrate and parameterize ensemble models were shared across models depending on applicability, and Information derived from models within the set were shared between models. Solid black arrows represent unidirectional information flow of outputs and towards management uptake. Dashed lines information sharing between models in the ensemble set (e.g., predation rates in one model inform natural mortality rates in another).
Ultimately, the NWACS-MICE model was chosen because it addressed most of the management objectives (and with further development could address virtually all). The NWACS-Full model had a similar benefit, but because of its increased structural complexity, future updates of that model may have been onerous and difficult to perform on an operational management timeline. VADER had a similar structural complexity and was able to address similar objectives as NWACS-MICE, but effects of prey on predators had not been developed at the time of the review. Both surplus production models were useful for understanding potential factors influencing Atlantic menhaden mortality and were amenable to rapid updating, but they did not address as many ecosystem management objectives as the other models.
In the future, the workgroup plans to continue using this set of models (or other models with a similar range of complexity). During the model development process, the members found that insight gained from one model helped inform other models and the overall ERP development (Figure 1). For example, the NWACS-Full model informed the structure and development of NWACS-MICE. The SPM-TVr model pointed to the potential of environmental processes that should be considered in future model/ERP development. Often multiple data sources were available for model parameterization and validation. Testing multiple models with multiple data sets led to discussions about the data and ecosystem dynamics. Ultimately, the models produced similar patterns in key indicators (e.g., menhaden biomass and fishing mortality rates), which led the workgroup to greater confidence that key ecological processes were captured in the models. More details on the models and model selection process for this example case are in Cieri et al. (2020).
Outcomes and Lessons Learned
Overall, the objective of the ERP workgroup was achieved. The NWACS-MICE model was accepted for use by the Menhaden Management Board to be used in combination with the single species stock assessment model for setting ERPs. The use of multiple models helped reassure the reviewers that key components of the ecosystem had been considered and that the NWACS-MICE model captured their dynamics sufficiently for providing management advice (SEDAR, 2020).
In this example, several benefits can be noted. Clear management objectives are helpful for understanding the principal ecosystem components that need to be included in a model ensemble. Developing these objectives helped managers and modelers focus on the key management issues. Using multiple models was helpful for understanding the level of model complexity needed to capture key components of an ecosystem and key management issues. Thorough and open discussion and presentation of models among modelers and stakeholders was beneficial. The open process enabled the ensemble team to address concerns about the models and guide stakeholders through ensemble development, with the end goal being stakeholder buy-in. In addition, this builds a collaborative and cooperative atmosphere among team members, which is important because an ensemble team requires many people who have to work together intensely and often.
The limitations to this MME approach were largely based on time and the existing model review process. Initial development and review of multiple models (and eventually model-averaged ensembles) take considerably more time than using a single model. However, after the initial development of the model set, efficiencies can be identified to enable more rapid model production and operationalization. Reviewing multiple models (and eventually model-averaged ensembles) for fisheries management requires considerably more time (and expertise covering a range of disciplines) than a typical single model review. This additional effort should be taken into account when establishing review timelines.
In addition, the clear and open process for developing Ecosystem Management Objectives, developing the ecosystem models, and establishing the process for setting ERPs helped to ensure that this approach could be used for setting ERPs. Moreover, the open process provided a higher level of satisfaction among stakeholders. Previous Atlantic Menhaden Board meetings (in the 2000s) had been contentious. After the August 2020 Atlantic Menhaden Board meeting where the process for setting ERPs was approved, multiple stakeholders sent out press releases indicating their satisfaction with the process.
Gulf of Mexico Gag and Red Grouper
Severe red tides in this region frequently cause mass mortality events for fisheries. To aid in quantifying the effects of these events on reef fish population dynamics, multiple ecosystem models were developed. Outputs from these models were used to inform the variability in natural mortality for stock assessment models. In the process of developing the MME, stakeholders learned more about multispecies model approaches and the need for more data/research on red tides. Additional stakeholder involvement in model review and development should help build support for more robust use of the MME approach for this issue.
Management Issue
Harmful algal blooms in the Gulf of Mexico caused by the dinoflagellate Karenia brevis have been linked to massive fish kills (Flaherty and Landsberg, 2011), mass mortalities of marine mammals, and increased sea turtle strandings (Landsberg et al., 2009). One of the most severe events occurred in 2005, when the West Florida Shelf experienced an extensive and persistent K. brevis bloom event (also known as red tide) covering more than 500 square nautical miles and lasting from January 2005 through February 2006 (FWRI, 2020). While the Florida Fish and Wildlife Conservation Commission Fish and Wildlife Research Institute Harmful Algal Bloom database1 provides a comprehensive record of species identified in fish kills (Sagarese et al., 2017; DiLeone and Ainsworth, 2019), much of the data are collected opportunistically from beachcombers who report observations of dead, stranded fishes. Anecdotal evidence suggests that shallow-water groupers including Goliath grouper (Epinephelus itajara), red grouper (Epinephelus morio), gag grouper (Mycteroperca microlepis), and scamp (Mycteroperca phenax) may succumb to severe red tide events, although the mechanism of mortality remains unknown (Smith, 1975; Walter et al., 2013; Driggers et al., 2016). The 2009 update stock assessments for both gag and red groupers were the first assessments to explicitly incorporate additional natural mortality attributed to the 2005 red tide event (SEDAR, 2009a,b). However, both assessments highlighted the need for red tide research to develop quantitative estimates of red tide mortality for consideration and incorporation into stock assessments.
An effort led by the National Oceanographic and Atmospheric Administration’s Gulf of Mexico Integrated Ecosystem Assessment Program to estimate red tide natural mortality solidified following unanimous passage of two motions by the Gulf of Mexico Fishery Management Council’s Standing and Ecosystem Scientific and Statistical Committees to (1) expand the integration of ecosystem components into the assessment and management of fishery resources and (2) develop products that integrate ecosystem analyses into stock assessments (GMFMC,, 2013). Both motions were passed as part of the 2013–2014 Gulf of Mexico Gag Grouper Assessment and again as part of the 2014–2015 Gulf of Mexico Red Grouper Assessment.
Model Set and Synthesis Approach
To address calls for evaluating and integrating the ecosystem effects of red tides into gag and red grouper management, a set of existing ecosystem models was assembled. The models were developed as part of a larger Integrated Ecosystem Assessment initiative to integrate environmental and ecosystem considerations into the fisheries management decision-making process (Grüss et al., 2016a). In total, three ecosystem models were identified and used to estimate gag and red grouper natural mortality and partitioned natural mortality into a minimum of two categories: predation and all sources other than predation (non-predation). The model set was developed with the initial goal of generating time series estimates of grouper natural mortality to identify the potential magnitude of red tide impacts and provide red tide mortality estimates to force stock assessment models and thus account for environmental conditions in grouper management decision-making.
The ecosystem models included two EwE models, each representing the West Florida Shelf ecosystem and developed by academic researchers in partnership with NOAA. The first EwE model (WFS Reef fish EwE) emphasized managed reef fish dynamics, resolved multi-stanza age classes (e.g., juveniles and adults) for multiple reef fishes including gag and red grouper, and estimated predation and non-predation natural mortality (Chagaris et al., 2015). The second EwE model (WFS Red tide EwE) was similar to the first but differed in two key regards: a third category of natural mortality, mortality due to red tide events, was explicitly represented, and age structure was limited to gag and red grouper and a subset of other coastal and reef fishes known to also be vulnerable to red tide (Gray, 2014; Sagarese et al., 2015; Gray DiLeone and Ainsworth, 2019). Representation of red tide mortality in WFS Red tide EwE was accomplished by repurposing size- and age-specific mortality functions used to implement fleet-specific fishing mortality in Gray (2014) and Gray DiLeone and Ainsworth (2019).
The third ecosystem model, an Object-oriented Simulator of Marine ecOSystEms model for the West Florida Shelf (OSMOSE-WFS), is a two-dimensional, individual-based, multispecies model. In OSMOSE, predation mortality rates and diet compositions emerge as a function of predator–prey overlap in the horizontal dimension, predator to prey size ratios, and accessibility coefficients reflecting the degree of accessibility of prey to the predators due to implicit, underlying factors such as prey morphology or distribution in the water column (Grüss et al., 2015b, 2016b). Similar to the EwE models, OSMOSE-WFS estimated age- and size-specific predation and non-predation mortality and care was taken to ensure that OSMOSE-WFS shared a number of features with WFS Reef fish EwE (spatial domain, study period, reference period, and reference biomasses) to improve comparability of model outputs. Predicted age-specific total natural mortality estimates for red grouper from OSMOSE-WFS were used in sensitivity analyses in the 2014–2015 Gulf of Mexico Red Grouper assessment (Grüss et al., 2015b, 2016b).
Both OSMOSE-WFS and WFS Reef Fish EwE were presented to the Gulf of Mexico Fishery Management Council’s Standing and Ecosystem Scientific and Statistical Committees after the 2014 gag grouper and 2015 red grouper assessments. Presentations were demonstrative in nature, with the overall aim being to familiarize the committee with the data sources and inputs, model structures, assumptions, and predictions. Procedures for formally reviewing stock assessments are well established by the Office of Science and Technology through the Center of Independent Experts (Brown et al., 2006), but there are currently no analogous procedures for multispecies and ecosystem models, and no terms of reference were developed to guide a technical review. While lacking formal review, presentations to the committee on the models were highly interactive, and the modelers sought feedback and incorporated requested modifications in updates to their models. Further, the modelers worked collaboratively throughout the project to ensure outputs were comparable to facilitate cross-model analysis (Grüss et al., 2015a, 2016a,b).
Model developers on this project had planned to use a quantitative ensemble approach for estimating the natural mortality rates employed in the 2014 gag grouper and 2015 red grouper assessment models. However, this did not happen because: (1) the three ecosystem models had started being developed before the opportunity to serve the 2014 gag grouper and 2015 red grouper assessments emerged and, therefore, had not benefited from enough exposure to and feedback from stakeholders before the base assessment models were finalized; and (2) assessments in the Gulf of Mexico and other marine regions rely on a specific technical review process of assessment model inputs and outputs, which, at the time, were lacking for ecosystem model inputs and the products that ecosystem models deliver to assessments.
Outcomes and Lessons Learned
This case study highlights the utility of multiple ecosystem models for advancing integration of ecosystem considerations into single-species management and was recognized as an important tool through the Southeast Data Assessment and Review (SEDAR) process by both assessment participants and fishery managers. The WFS Reef fish EwE model (Chagaris, 2013; Chagaris et al., 2015) was deemed useful for informing the upcoming SEDAR stock assessments, WFS Red tide EwE was recognized as useful for informing the grouper assessments (Gray et al., 2013; Gray, 2014; Gray DiLeone and Ainsworth, 2019), and OSMOSE-WFS was found to be useful as a complementary tool for the grouper assessments because its structure and assumptions differed markedly from the two EwE models while sharing reference conditions (Grüss et al., 2013, 2015a,b, 2016a,b, 2017; Gruss et al., 2017). Ultimately, committee members were interested in applying multispecies models to fisheries management questions, but did voice some concerns over the representativeness of the data inputs, such as those used to parameterize trophic interactions and the spatial representation of the red tide mortality.
Both the 2014 gag grouper and 2015 red grouper assessments represented some of the first assessments in the Southeast United States to consider external effects due to environmental drivers, in this case red tide. The age-specific mortality rates estimated by OSMOSE-WFS and an index of red tide mortality derived from WFS Red tide EwE were explicitly tested in sensitivity analyses for red grouper (Sagarese et al., 2015). One sensitivity run replaced the Lorenzen age-specific natural mortality vector with the age-specific natural mortality (predation + non-predation mortality) vector estimated within OSMOSE-WFS. Another sensitivity run used the index of red tide mortality produced by the WFS Red Tide EwE model to drive red tide mortality. Mortality due to red tide was estimated within the stock assessment via a bycatch fleet, which is a customization available in the Stock Synthesis modeling framework to account for extra removals not due to directed fishing mortality (Methot et al., 2018). Within the stock assessment model, the index produced by WFS Red Tide EwE was input as a time series of effort data for the red tide bycatch fleet, where the effort index was essentially treated as a survey of red tide mortality. The assessment model, in turn, estimated the dead biomass due to red tide. While these sensitivity runs allowed valuable discussions of how ecosystem model outputs could be used in an assessment framework, they did not become the base assessment model. Ultimately, the base assessment models for both gag and red grouper estimated extra natural mortality due to red tide solely in 2005, which was a severe red tide event as supported by the ecosystem model outputs (e.g., WFS Red Tide EwE).
What was learned from the Gulf of Mexico gag and red grouper experiences was that the development of ecosystem models and their ultimate use in fisheries management need to place more emphasis on stakeholder engagement from the moment ecosystem models start being developed (Gruss et al., 2017; Chagaris et al., 2019) and throughout the review process, which can be iterative in nature. Increased buy-in and support from stakeholders, as well as incorporation of their knowledge, could help increase data quality and increase understanding of project objectives (e.g., bringing in stakeholder knowledge when parameterizing different diet matrix constructs could improve ecosystem model realism) (Bentley et al., 2019). Furthermore, substantial time needs to be allocated to a thorough technical review of ecosystem model inputs and their products for stock assessments to help address data concerns by stakeholders (e.g., diet matrices in EwE) and advance the direct use of ecosystem model predictions and MME in fisheries assessments and management.
The development of ecosystem models and the potential use of these ecosystem models and MME in the Gulf of Mexico fisheries assessments were greatly facilitated by strong collaboration between NOAA and academic partners. Relying on academic agencies to develop ecosystem models helped relieve some of the burden on the assessment process and NOAA, although considerable effort was still needed to determine how to incorporate the information within the stock assessment (Sagarese et al., 2015).
Multiple ecosystem models were used to confirm the importance of red tide on the mortality rates of gag and red grouper. Agreement between the different models built support for allowing the stock assessment models to account for elevated mortality in years with strong red tide. Specifically, the model building and comparison process helped (1) educate stakeholders and managers on multispecies model assumptions and applications, (2) build robust support for addressing red tide mortality in stock assessments, and (3) spur additional conversations about the application of multispecies models to other problems.
Sardine and Anchovy in the California Current
Concerns about low abundances of key forage fish in the California Current led to requests from a Pacific Fishery Management Council workshop for a modeling exercise to improve understanding of the availability of forage fish to support predators and fisheries. Models were brought together through a partnership between academic and government research institutions. As the fisheries for these forage species were closed, the models were not ultimately used to make tactical management decisions; however, the models provided strategic management advice and provided a framework for informing future decision-making.
Management Issue
Pacific sardine (Sardinops sagax) and anchovy (Engraulis mordax) are key forage fish species in the California Current, supporting both fisheries and predators, but also show strong fluctuations in abundance on multi-decadal time scales (Baumgarner, 1992). In 2014–2015, sardine and anchovy were both at low abundance (Hill et al., 2015; MacCall et al., 2016; Thayer et al., 2017), raising concerns regarding the impacts of fishing, and the availability of forage for predators. To better understand these concerns and to address a call for modeling from a Pacific Fishery Management Council workshop (PFMC,, 2013), a suite of models was brought together by the Ocean Modeling Forum (OMF2). The OMF is a collaboration between academic, state, and federal research scientists, policy analysts, fishery managers, and industry, to facilitate the integration of modeling approaches into applied marine resource decision-making (Francis et al., 2018). The OMF applies a case study approach to help marine managers frame questions and learn about and apply modeling approaches, while also allowing collaborations and improvement across modeling groups.
The overarching goal of this case study was to provide fisheries management, including the Pacific Fishery Management Council, with better knowledge to improve EBFM of small pelagic fish in the California Current. Providing this knowledge required answering basic questions: What predators eat sardine or anchovy? In turn, what do sardine and anchovy eat? How large are the population cycles of these forage fish? What is the interaction between forage fish species? A range of model types was applied to investigate these questions. The utility of individual models was amplified by linking different model types, by incorporating knowledge from other models and from empirical studies, and by including expert opinion about ecosystem-level dynamics. Applicability of results was improved by including details of the actual fishery management procedures as implemented by Canada, the United States, and Mexico (Francis et al., 2018).
Model Set and Synthesis Approach
Understanding the role of forage fish in the California Current, and the potential impacts of periods of low forage fish abundance, required a diverse suite of models. A non-dynamic Ecopath model was an essential first step to handle the “accounting” exercise of weighing predator needs against forage fish stocks (Koehn et al., 2016). This accounting of diets and biomasses was used as input to a dynamic Model of Intermediate Complexity for Ecosystem assessment, MICE, as broadly defined by Plaganyi et al. (2014). Here we will call the model implementation (Punt et al., 2016) the California Current MICE (CC-MICE), to differentiate from the NWACS-MICE model described above. The CC-MICE included basic spatial representation and trophic interactions between sardine, anchovy, and key predators, but not the broader food web (Figure 1). Strengths of the CC-MICE model included that it was able to capture realistic harvest policies and that it was simple enough to allow full Monte Carlo testing of scenarios for recruitment and structural uncertainty in ecological relationships. The diet information and inputs were also compared to those for an end-to-end ecosystem model, an Atlantis model of the California Current (Kaplan et al., 2017), but the values were not forced to be identical (Figure 1). Moreover, the Ecopath model provided information on diets and biomass that was used to make statistical predictions of predator response to prey declines (PREP, Predator Response to the Exploration of Prey) based on Pikitch et al. (2012) and to quantify prey importance (SURF index, Supportive Role to Fishery ecosystems) (Essington and Plaganyi, 2014; Figure 1).
The California Current team opportunistically leveraged modeling frameworks that were in development (Ecopath, Atlantis), but tailored them to new questions (especially for Atlantis) and supplemented them with new model types (CC-MICE). Repurposing models can be problematic (Essington and Plaganyi, 2014) particularly if model taxonomic and spatial resolution are insufficient to capture the species of interest and related model skill. For the California Current, this was partially addressed by co-developing major parts of the Atlantis and Ecopath models (literally in the same small room), with an eye toward addressing ecosystem-based forage fish management, and then subsequently using those findings to inform CC-MICE model development centered around the same species and management questions.
Translating outputs between structurally dissimilar models can be challenging and time consuming. In this case, the Ecopath model used biomass-based accounting, the CC-MICE modeled numbers of individuals, and Atlantis modeled numbers of individual vertebrates and weight-at-age (Kaplan et al., 2019). Ultimately sardine biomass was used as the common currency for Atlantis and CC-MICE, such that Atlantis simulation output could be qualitatively compared to CC-MICE output that represented conditions of similar sardine abundance. In this way, abundance or biomass of predators could be evaluated at different levels of sardine abundance. One caveat was that the Atlantis model had coarser taxonomic resolution of bird groups that are dependent on forage fish. Approximate comparisons could be made to the PREP predictions, in terms of proportional declines of predators under different sardine abundance scenarios. The primary goal was to qualitatively compare the predictions between models, keeping in mind the differences in model structure, currencies, and taxonomic resolution. Though this allowed comparison of the models’ predictions, it was not intended as a way of forming a true ensemble. Overall, model comparison illustrated that agreement (in terms of response of predators such as sea lions and birds) hinged on the level of taxonomic resolution, assumptions of generalist versus specialist diets, and whether the models included age structure and other “dampening” aspects that slow perturbations in the models (Kaplan et al., 2019).
Though having different “currencies” across models necessitated some careful translation, model comparison was feasible, and the currencies and units that resonated with particular users were retained. For instance, the CC-MICE model allowed calculations of population size and probabilities of falling below thresholds (familiar to stock assessment audiences) and Atlantis tracked population size (as in stock assessment) but also weight-at-age (relevant to predator condition). Ecopath and PREP captured aspects of energy transfer and trophic demands and clearly visualized diet dependencies (relevant to predator bioenergetics and forage needs). Overall, the diverse set of models meant that expert participants who were not involved in the hands-on modeling were nonetheless able to contribute information to one or more models in ways that built credibility and improved the representation of ecology or management.
Outcomes and Lessons Learned
The suite of models assembled to study forage fish proved useful in a research context, but somewhat paradoxically the impetus for the study (low sardine and anchovy abundances) also kept the fisheries closed, and this meant that managers did not have a pressing need to incorporate the modeling into new harvest decisions. Moreover, revised sardine harvest policies (Hurtado-Ferro and Punt, 2014) had been recently evaluated and adopted by fishery managers and there was no new set of harvest policy options directly under consideration. Nonetheless, the work was presented to the Coastal Pelagic Fishery Management Team of the Pacific Fishery Management Council, and previous engagement with the Management Council (on the Atlantis model specifically) provides the framework for further review and applications, including those related to forage fish harvest (Kaplan and Marshall, 2016). A formal review process and related fast timetable were not part of the California Current OMF forage fish work; though this was a disadvantage in some ways, it also allowed a wider breadth of model types to be considered (i.e., wider than could be handled in a focused review process).
As management needs arise for forage fish in the California Current, we expect some future version of this MME to be valuable for testing new harvest policies or for evaluating impacts of future changes in the environment. In particular, the dynamic models such as the CC-MICE and Atlantis could test alternate harvest thresholds, or alternative maximum fishing rates; these thresholds and maxima are part of current harvest policy in the region (PFMC,, 2019). Siple et al. (2019) suggest that these harvest policies should be matched closely to each forage species’s life history characteristics and population dynamics and, furthermore, that rapid monitoring and detection of stock trends can mitigate some risks and tradeoffs. MMEs can further test these results across a broader range of models and structural assumptions and can test novel approaches such as aggregate or guild catch limits rather than only single-species limits (Gaichas et al., 2017), i.e., to preserve abundance of total forage. To truly develop management-ready results for the United States portion of the California Current will require engagement of the MME teams with the United States Pacific Fishery Management Council, states, and tribes.
The boom-and-bust nature of small pelagic fish in upwelling systems such as the California Current implies that fisheries, and perhaps therefore demand for MME-based advice, will be episodic. In these situations, modeling teams are likely to need to rapidly and periodically assemble suites of models. Another result of working within these highly variable systems is that it is important to ensure that the ensemble includes at least one model that captures stochasticity (e.g., in recruitment), such that results can be presented in terms of probability of catches or abundance of small pelagic fish and their predators falling below management reference levels. This is common in single-species models but is not ubiquitous in slower, more complex multispecies and ecosystem models. The CC-MICE model (Punt et al., 2016) was designed to offer this perspective regarding California Current forage fish, and similar approaches for other species and regions also offer this valuable probabilistic approach (Cochrane et al., 1998; Sanchez et al., 2019; Siple et al., 2019; Okamoto et al., 2020).
As discussed for the case studies above, the human collaboration involved in constructing, modifying, and applying the models strengthened each modeling effort and brought new insights and data to bear on the research questions. Francis et al. (2018) elaborate on how this process evolved within the Ocean Modeling Forum, and the benefits of this approach. For sardine and anchovy in the California Current, the very intentional set of structured meetings and dedicated funding facilitated this collaboration, rather than relying on ad hoc relationships between researchers. A benefit of the sardine and anchovy work and a related herring working group were new collaborations (co-authorship networks) across disciplines and between previously separated individuals (Francis et al., 2018). Intentional engagement with stakeholders in workshop settings has been a key component of similar efforts in other regions (Trenkel et al., 2015; Feeney et al., 2019).
Conclusion
The case studies presented here highlight advantages that come from developing MMEs in applied EBFM contexts as well as challenges that require forethought and planning. While the statistical advantages of ensembles are well understood, we show that in practice, estimation of ensemble-averaged quantities of interest remains an elusive goal. That said, the case studies reveal other benefits that provide strong support for pursuing MMEs and demonstrate a range of applications. Below, we summarize these benefits and discuss recommendations to avoid potential pitfalls.
The act of building ensemble modeling, and development of the ensemble model suite, can improve the credibility of multispecies/ecosystem models. In all case studies, development of MMEs required use of consistent data sets that could be employed across different modeling frameworks. This required a review and consideration of data sets that were likely more thorough than would be needed for a single model. Similarly, during the development process, a rigorous, impartial internal review of the models is needed to understand similarities and inconsistencies between the model outputs and with the data that models were fitted or tuned to. A single model would only be reviewed based on comparisons of its outputs to data. A thorough, rigorous internal review of models and data supports a modeling team in preparing for external review. A key characteristic of the fishery management world is rigorous external review processes. As multi-model approaches mature, external review processes can dictate what models are accepted/acceptable for use in an ensemble.
Ensembles can be qualitative in nature—that is, behavior and predictions can be compared and synthesized in a qualitative manner. At this time, MMEs for fisheries management applications have not been combined quantitatively to produce probability distributions of outputs. As ecosystem modeling is in the early stages of MME, methodologies for combining outputs from models with dissimilar output structures have not been fully developed. Similarly, time has been a limited resource when using multi-model approaches, so the additional time to apply model-averaging and other techniques for combining models has not been available. While formal quantitative ensembling might be desirable and among the goals for this discipline, other fields have emphasized that qualitative comparisons of models in an ensemble may be just as valuable as model-averaged ensembles (Townsend et al., 2014) (Beven, J. NOAA Weather Service, pers comm).
As noted by Townsend et al. (2014), applications of true ensembles in living marine resource management are rare (though there are some recent examples) (Gardmark et al., 2013; Reum et al., 2020). Instead, Townsend et al. (2014) discuss the “mingling of models,” and a need for at least a qualitative comparison of predictions from different models. This mingling of models and qualitative comparison was the approach used for California Current forage fish. In particular, this simple type of MME allowed static models (Ecopath) to inform different dynamic models (e.g., CC-MICE and Atlantis) and a comparison of their outputs. There was no natural way to create ensemble averages, and in this case, the dynamic models were so different in terms of structure and number of replicates (many replicates with CC-MICE, versus single tests of each fishing level with Atlantis), that true ensembles seemed infeasible, or at least a much longer term goal.
Involving a diverse set of stakeholders (plan teams—teams of people involved in the process—fisheries councils and fishers) is important for getting buy-in from the community. Especially noted in the menhaden case, a stakeholder process to set management objectives and an open model development process were beneficial in the ultimate application of ecosystem models for management. During the development phase, stakeholders should be in on the conversations of the modeling team, and they should have time to make statements or ask questions. This way, decisions about data and models can be seen to have a clear rationale based on science and practical concerns. As noted with the Gulf of Mexico grouper experience, to be efficient, stakeholder engagement needs to happen as soon as the ecosystem models start being developed.
Models with a range of different structures should be used. In all the cases, models with very different structures (e.g., biomass dynamic, age structured, food web, and individual-based) were used. Variety in model structure allows consideration of multiple hypotheses about key factors driving a system. A modeling team that has thoroughly considered the environmental and ecological mechanisms will be better prepared to answer questions from stakeholders and external reviewers as to why particular modeling decisions were made, thus improving the credibility of the advice given. In addition, as the relative importance of key system drivers may change over time, using models that keep track of drivers will improve awareness of potentially important changes.
Model of Intermediate Complexity for Ecosystem assessments are an important part of the model sets. In the menhaden and California Current cases, MICE were used in the model set. As noted above, a range of model structures is important, and similarly, models with a range of complexity are important. Often structure and complexity go hand-in-hand. Simple biomass dynamic models with just a few species and drivers can be quick to run and accommodate extensive sensitivity analysis, but they may not capture key drivers. Complex, end-to-end models will likely capture key drivers, but they can be unwieldy for sensitivity analyses and maintenance. MICEs strike a balance between these extremes, capture most of the key drivers (at least drivers deemed important during the modeled time frame), and are relatively easier to maintain and run sensitivity analyses on. This approach has been adopted for many systems worldwide and the benefits are further described in Plaganyi et al. (2014). While the Gulf of Mexico grouper case study served as a step toward EBFM in the southeast United States, jumping immediately into highly complex models likely complicated their uptake in the fisheries management process.
MME Recommendations
Based on three case studies analyzed in the present paper, MMEs for living marine resource management applications have some demonstrated benefits (e.g., added rigor in model data preparation, more thorough examination of key drivers of system dynamics, and improved ability to deal with uncertainty attributable to model structure). From these case studies, some clear recommendations for MMEs also emerge:
1. Multispecies model ensembles should consist of a range of models with different structures—MICE are a useful model type to be included in the ensemble.
2. Stakeholders should be included in the model development process to help with buy-in and transparency.
3. Qualitative syntheses of MME outputs are valuable in themselves and important for evaluating the potential utility of more involved quantitative approaches.
Beyond these general benefits and recommendations, there are practical matters to be considered before an MME is developed for an ecosystem or MME management application. The case studies analyzed in this paper demonstrate MME development for specific management questions, and, as a result, time for fully implementing MME (including exploration of quantitative synthesis approaches) was limited. With a bit of hindsight and reverse engineering, approaches for establishing MME processes for regional applications can be surmised and recommendations for planning future MME applications can be made and further benefits of MMEs can be achieved. Recommendations for implementing a regional MME program within a resource management agency issues include:
1. Establish automated data collation processes. After field data are collected, entered into electronic databases, and quality assurance processes are implemented, automated software or scripts should be used to synthesize and prepare the data for input into the MME. The input data types for each model in the MME may vary depending on the model structure. Documented automated processes for converting raw data to model input are necessary to ensure that models are using the same data, which will be important for synthesis of outputs. In addition, time saved on data wrangling allows more time for MME development and output synthesis.
2. Use a stakeholder-oriented process to clarify the key objectives and questions to address, the important processes within the system, and the potential universe of relevant models, e.g., Chagaris et al. (2019). Scientists can identify biophysical factors that drive and organize system dynamics. Social scientists can identify human activities that influence ecosystems. Conceptual models are a useful way to incorporate stakeholder input on important ecosystem components and drivers. In addition, they are useful for identifying important ecosystem indicators.
3. Depending on the aims and goals of the modeling effort, set up a range of models with a range of structural complexity. A minimal model set should include:
(a) One to three simpler models (e.g., extended stock assessment models and multispecies surplus production models).
(b) At least one MICE.
(c) One or two more complex models (e.g., end-to-end models, dynamic food web models, coupled biophysical models, and socioecological models).
4. Involve stakeholders in the development of each ecosystem model in the MME, ideally as early as ecosystem models start being developed. As ecosystem models keep being developed, regular presentations to stakeholder groups and management bodies will help with buy-in. Early and regular stakeholder engagement in model development can help to establish clear management objectives.
5. Develop long-term funding to support and maintain all models in the MME. Shorter term research funding can be used to adapt existing models in the MME to address novel management issues, to develop and incorporate new models into MME programs, and to develop approaches for quantitative synthesis of MMEs. Model development is iterative in nature and funding horizons should reflect that fact.
6. Develop model review procedures (or refine existing procedures) that can more readily deal with multiple models and models with increased ecological complexity relative to standard fisheries population dynamic models.
The recommendations for establishing MME programs may seem daunting at first; however, many regional management agencies have begun to implement some of these recommendations. Fisheries agencies have a number of multispecies/ecosystem models being used for management (ICES, 2019; Townsend et al., 2020). For example, multispecies interactions are considered in the management of multiple North Sea and Baltic Sea stocks by applying time-varying predation mortality estimated by multispecies models within single-species stock assessments (Lewy and Vinther, 2004; Bauer et al., 2019). Multispecies interactions in the Barents Sea are explicitly considered in the management of both capelin and Northeast Arctic cod. Capelin assessment and management explicitly considers forage for cod (Gjosaeter et al., 2002), and the cod harvest control rule has an upper B threshold where F increases (ICES, 2020), which may mediate capelin predation. In addition to the menhaden example reviewed here, ICES has used an ecosystem model to enhance single species advice in the Irish Sea (Howell et al., 2021). The NOAA-Alaska Fisheries Science Center has developed an MME for climate considerations (Hollowed et al., 2020). The NOAA-Northwest and Southwest Fisheries Science Centers have developed approaches for engaging with stakeholders to align models with management needs and identify where new models are needed (Tommasi et al., 2020). The NOAA-Northeast Fisheries Science Center is developing automation approaches for producing standardized model input data sets. The NOAA-Southeast Fisheries Science Center, in collaboration with academic partners and through additional funding via the NOAA Restore Science Program, is building ecosystem models for use as decision support tools for fisheries managers. Further, SEFSC is investing in a formal peer-review of the Gulf of Mexico Atlantis model for application to Gulf shrimp fisheries.
These steps toward making ecosystem modeling operational are part of an evolution. Historically, these models were used for research, and they have been used increasingly for management applications. The models applied for California Current forage fish illustrate two tensions: the need to both apply existing models as well as to develop new approaches with added capabilities; and the desire to delve deep into ecological complexity while also including an array of models that capture very different aspects of the fishery system. Development of MME programs will push government agencies to operationalize modeling, but care should be taken to not divest from the development of new models when needed nor divert resources from stock assessments, which themselves benefit from MME products. Recognizing the significant funding requirements of MME efforts, cost–benefit analyses could be performed to identify where resources should best be allocated within management systems. As was demonstrated in all of these case studies, collaborations between academic and government researchers can help to ensure that research and new model development are ongoing and potentially distribute and reduce overall costs.
Ultimately, the development of MME programs will not necessarily address all EBFM questions. However, a directed evolution of resource management modeling programs toward an MME program will enable more rapid response to EBFM questions as they arise.
Author Contributions
All authors listed have made a substantial, direct and intellectual contribution to the work, and approved it for publication.
Conflict of Interest
The authors declare that the research was conducted in the absence of any commercial or financial relationships that could be construed as a potential conflict of interest.
Footnotes
References
Addison, P. F. E., Rumpff, L., Bau, S. S., Carey, J. M., Chee, Y. E., Jarrad, F. C., et al. (2013). Practical solutions for making models indispensable in conservation decision-making. Divers. Distrib. 19, 490–502. doi: 10.1111/ddi.12054
Anstead, K., Drew, K., Chagaris, D., Cieri, M. D., Schueller, A., McNamee, J., et al. (2020). The past, present, and future of forage fish management: a case study of Atlantic Menhaden. Front. Mar. Sci. 10:20.
ASMFC (1998). American Shad Stock Assessment Peer Review Report. Arlington Mason, VA: Atlantic States Marine Fisheries Commission.
ASMFC (2015). Ecosystem Management Objectives Workshop Report. Arlington Mason, VA: Atlantic States Marine Fisheries Commission.
Baker, C. M., Gordon, A., and Bode, M. (2017). Ensemble ecosystem modeling for predicting ecosystem response to predator reintroduction. Conserv. Biol. 31, 376–384. doi: 10.1111/cobi.12798
Bauer, B., Horbowy, J., Rahikainen, M., Kulatska, N., Muller-Karulis, B., Tomczak, M. T., et al. (2019). Model uncertainty and simulated multispecies fisheries management advice in the Baltic Sea. PLoS One 14:ee0211320. doi: 10.1371/journal.pone.0211320
Baumgarner, T. S. (1992). Reconstruction of the history of the Pacific sardine and northern anchovy populations over the past two millenia from sediments of the Santa Barbara basin. Calif. Cooperative Ocean. Fish. Investig. Rep. 33, 24–40.
Bentley, J. W., Hines, D. E., Borrett, S. R., Serpetti, N., Hernandez-Milian, G., Fox, C., et al. (2019). Combining scientific and fishers’ knowledge to co-create indicators of food web structure and function. ICES J. Mar. Sci. 76, 2218–2234. doi: 10.1093/icesjms/fsz121
Brown, S. K., Shivlani, M., Die, D., Sampson, D. B., and Ting, T. A. (2006). The center for independent experts: the national external peer review program of NOAA’s national marine fisheries service. Fisheries 31, 590–600. doi: 10.1577/1548-8446(2006)31[590:tcfie]2.0.co;2
Buchheister, A., Miller, T. J., and Houde, E. D. (2017a). Evaluating ecosystem-based reference points for Atlantic Menhaden. Mar. Coast. Fish. 9, 457–478. doi: 10.1080/19425120.2017.1360420
Buchheister, A., Miller, T. J., Houde, E. D., and Lowensteiner, D. A. (2017b). Technical Documentation of the Northwest Atlantic Continental Shelf (NWACS) Ecosystem Model. Report to the Lenfest Ocean Program. Washington, DC: University of Maryland Center for Environmental Sciences Report.
Burnham, K. P., and Anderson, D. R. (2002). Model Selection and Multimodel Inference: A Practical Information-Theoretic Approach. New York: Springer-Verlag.
Chagaris, D. (2013). Ecosystem-based evaluation of fishery policies and tradeoffs on the West Florida Shelf. Ph.D., University of Florida, Florida.
Chagaris, D., Drew, K., Schueller, A., Cieri, M. D., Brito, J., and Buchheister, A. (2020). Ecological reference points for Atlantic Menhaden established using an ecosystem model of intermediate complexity. Front. Mar. Sci. 7:606417. doi: 10.3389/fmars.2020.606417
Chagaris, D., Sagarese, S., Farmer, N., Mahmoudi, B., de Mutsert, K., VanderKooy, S., et al. (2019). Management challenges are opportunities for fisheries ecosystem models in the Gulf of Mexico. Mar. Policy 101, 1–7. doi: 10.1016/j.marpol.2018.11.033
Chagaris, D. D., Mahmoudi, B., Walters, C. J., and Allen, M. S. (2015). Simulating the trophic impacts of fishery policy options on the west florida shelf using ecopath with ecosim. Mar. Coast. Fish. 7, 44–58. doi: 10.1080/19425120.2014.966216
Cheung, W. W. L., Frolicher, T. L., Asch, R. G., Jones, M. C., Pinsky, M. L., Reygondeau, G., et al. (2016). Building confidence in projections of the responses of living marine resources to climate change. ICES J. Mar. Sci. 73, 1283–1296. doi: 10.1093/icesjms/fsv250
Cieri, M. D., Drew, K., Schueller, A., Buchheister, A., Chagaris, D., Nesslage, G. M., et al. (2020). Balancing model complexity, data requirements, and management objectives in developing ecological reference points for Atlantic Menhaden. Front. Mar. Sci. 8:608059. doi: 10.3389/fmars.2021.608059
Cochrane, K. L., Butterworth, D. S., de Oliveira, J. A. A., and Roel, B. A. (1998). Management procedures in a fishery based on highly variable stocks and with conflicting objectives: experiences in the South African pelagic fishery. Rev. Fish Biol. Fish. 8, 177–214. doi: 10.1023/A:1008894011847
DiLeone, A. M. G., and Ainsworth, C. H. (2019). Effects of Karenia brevis harmful algal blooms on fish community structure on the West Florida Shelf. Ecol. Model. 392, 250–267. doi: 10.1016/j.ecolmodel.2018.11.022
Dolan, T. E., Patrick, W. S., and Link, J. S. (2016). Delineating the continuum of marine ecosystem-based management: a US fisheries reference point perspective. ICES J. Mar. Sci. 73, 1042–1050. doi: 10.1093/icesjms/fsv242
Driggers, W. B., Campbell, M. D., Debose, A. J., Hannan, K. M., Hendon, M. D., Martin, T. L., et al. (2016). Environmental conditions and catch rates of predatory fishes associated with a mass mortality on the West Florida Shelf. Estuar. Coast. Shelf Sci. 168, 40–49. doi: 10.1016/j.ecss.2015.11.009
Essington, T. E., and Plaganyi, E. E. (2014). Pitfalls and guidelines for “recycling” models for ecosystem-based fisheries management: evaluating model suitability for forage fish fisheries. ICES J. Mar. Sci. 71, 118–127. doi: 10.1093/icesjms/fst047
Feeney, R. G., Boelke, D. V., Deroba, J. J., Gaichas, S., Irwin, B. J., and Lee, M. (2019). Integrating management strategy evaluation into fisheries management: advancing best practices for stakeholder inclusion based on an MSE for Northeast US Atlantic herring. Can. J. Fish. Aquat. Sci. 76, 1103–1111. doi: 10.1139/cjfas-2018-0125
Flaherty, K. E., and Landsberg, J. H. (2011). Effects of a Persistent Red Tide (Karenia brevis) Bloom on Community Structure and Species-Specific Relative Abundance of Nekton in a Gulf of Mexico Estuary. Estuar. Coasts 34, 417–439. doi: 10.1007/s12237-010-9350-x
Francis, T. B., Levin, P. S., Punt, A. E., Kaplan, I. C., Varney, A., and Norman, K. (2018). Linking knowledge to action in ocean ecosystem management: the Ocean Modeling Forum. Elementa Sci. Anthropocene 6:83. doi: 10.1525/elementa.338
Fulton, E. A., Boschetti, F., Sporcic, M., Jones, T., Little, L. R., Dambacher, J. M., et al. (2015). A multi-model approach to engaging stakeholder and modellers in complex environmental problems. Environ. Sci. Policy 48, 44–56. doi: 10.1016/j.envsci.2014.12.006
Gaichas, S. K., Fogarty, M., Fay, G., Gamble, R., Lucey, S., and Smith, L. (2017). Combining stock, multispecies, and ecosystem level fishery objectives within an operational management procedure: simulations to start the conversation. ICES J. Mar. Sci. 74, 552–565. doi: 10.1093/icesjms/fsw119
Gardmark, A., Lindegren, M., Neuenfeldt, S., Blenckner, T., Heikinheimo, O., Muller-Karulis, B., et al. (2013). Biological ensemble modeling to evaluate potential futures of living marine resources. Ecol. Appl. 23, 742–754. doi: 10.1890/12-0267.1
Garrison, L. P., Link, J. S., Kilduff, D. P., Cieri, M. D., Maffley, B., Vaughan, D. S., et al. (2010). An expansion of the MSVPA approach for quantifying predator-prey interactions in exploited fish communities. ICES J. Mar. Sci. 67, 856–870. doi: 10.1093/icesjms/fsq005
Geary, W. L., Bode, M., Doherty, T. S., Fulton, E. A., Nimmo, D. G., Tulloch, A. I. T., et al. (2020). A guide to ecosystem models and their environmental applications. Nat. Ecol. Evol. 4, 1459–1471. doi: 10.1038/s41559-020-01298-8
Gjosaeter, H., Dalpadado, P., and Hassel, A. (2002). Growth of Barents Sea capelin (Mallotus villosus) in relation to zooplankton abundance. ICES J. Mar. Sci. 59, 959–967. doi: 10.1006/jmsc.2002.1240
GMFMC (2013). Standing and Ecosystem SSC Meeting Summary 03-2013. Tampa, FL: Gulf of Mexico Fishery Management Council.
Gray, A. (2014). Karenia Brevis Harmful Algal Blooms: Their Role in Structuring the Organismal Community on the West Florida Shelf. Master’s, University of South Florida, Florida.
Gray, A., Ainsworth, C. H., Chagaris, D., and Mahmoudi, B. (2013). Red tide mortality on gag grouper 1980-2009. North Charleston, SC: Southeast Data Assessment and Review.
Gray DiLeone, A. M., and Ainsworth, C. H. (2019). Effects of Karenia brevis harmful algal blooms on fish community structure on the West Florida Shelf. Ecol. Model. 392, 250–267.
Grüss, A., Harford, W. J., Schirripa, M. J., Velez, L., Sagarese, S. R., Shin, Y. J., et al. (2016a). Management strategy evaluation using the individual-based, multispecies modeling approach OSMOSE. Ecol. Model. 340, 86–105. doi: 10.1016/j.ecolmodel.2016.09.011
Gruss, A., Rose, K. A., Simons, J., Ainsworth, C. H., Babcock, E. A., Chagaris, D. D., et al. (2017). Recommendations on the use of ecosystem modeling for informing ecosystem-based fisheries management and restoration outcomes in the Gulf of Mexico. Mar. Coast. Fish. 9, 281–295. doi: 10.1080/19425120.2017.1330786
Grüss, A., Schirripa, M. J., Chagaris, D., Drexler, M., Simons, J., Verley, P., et al. (2013). Natural Mortality Rates and Diet Patterns of Gag Grouper (Mycteroperca microlepis) in the West Florida Shelf ecosystem in the 2000s: Insights from the Individual-Based, Multi-Species Model OSMOSE-WFS. North Charleston, SC: Southeast Data Assessment and Review.
Grüss, A., Schirripa, M. J., Chagaris, D., Drexler, M., Simons, J., Verley, P., et al. (2015a). Evaluation of the trophic structure of the West Florida Shelf in the 2000s using the ecosystem model OSMOSE. J. Mar. Syst. 144, 30–47. doi: 10.1016/j.jmarsys.2014.11.004
Grüss, A., Schirripa, M. J., Chagaris, D., Velez, L., Shin, Y. J., Verley, P., et al. (2016b). Estimating natural mortality rates and simulating fishing scenarios for Gulf of Mexico red grouper (Epinephelus morio) using the ecosystem model OSMOSE-WFS. J. Mar. Syst. 154, 264–279. doi: 10.1016/j.jmarsys.2015.10.014
Grüss, A., Schirripa, M. J., Chagaris, D., Verley, P., Shin, Y. J., Velez, L., et al. (2015b). Estimating Age- and Size-Specific Natural Mortality Rates for Gulf of Mexico Red Grouper (Epinephelus morio) Using the Ecosystem Model OSMOSEWFS. North Charleston, SC: Southeast Data Assessment and Review.
Grüss, A., Thorson, J. T., Sagarese, S. R., Babcock, E. A., Karnauskas, M., Walter, J. F., et al. (2017). Ontogenetic spatial distributions of red grouper (Epinephelus mono) and gag grouper (Mycteroperca microlepis) in the US Gulf of Mexico. Fish. Res. 193, 129–142. doi: 10.1016/j.fishres.2017.04.006
Hagedorn, R., Doblas-Reyes, F. J., and Palmer, T. N. (2005). The rationale behind the success of multi-model ensembles in seasonal forecasting - I. Basic concept. Tellus Ser. Dyn. Meteorol. Oceanogr. 57, 219–233. doi: 10.1111/j.1600-0870.2005.00103.x
Hill, K. T., Crone, P. R., Dorval, E., and Macewicz, B. J. (2015). Assessment of the Pacific sardine resource in 2015 for USA management in 2015–2016. Portland, OR: Pacific Fishery Management Council.
Hill, S. L., Watters, G. M., Punt, A. E., McAllister, M. K., Le Quere, C., and Turner, J. (2007). Model uncertainty in the ecosystem approach to fisheries. Fish Fish. 8, 315–336. doi: 10.1111/j.1467-2979.2007.00257.x
Hollowed, A. B., Holsman, K. K., Haynie, A. C., Hermann, A. J., Punt, A. E., Aydin, K., et al. (2020). Integrated modeling to evaluate climate change impacts on coupled social-ecological systems in Alaska. Front. Mar. Sci. 6:775. doi: 10.3389/fmars.2019.00775
Howell, D., Schueller, A. M., Bentley, J. W., Buchheister, A., Chagaris, D., Cieri, M., et al. (2021). Combining ecosystem and single-species modeling to provide ecosystem-based fisheries management advice within current management systems. Front. Mar. Sci. 7:607831. doi: 10.3389/fmars.2020.607831
Hurtado-Ferro, F., and Punt, A. E. (2014). Revised Analyses Related to Pacific Sardine Harvest Parameters. Portland, OR: Pacific Fishery Management Council.
Ianelli, J., Holsman, K. K., Punt, A. E., and Aydin, K. (2016). Multi-model inference for incorporating trophic and climate uncertainty into stock assessments. Deep Sea Res. II Top. Stud. Oceanogr. 134, 379–389. doi: 10.1016/j.dsr2.2015.04.002
ICES (2020). ICES Advice on Fishing Opportunities, Catch, and Effort Arctic Ocean, Barents Sea, and Norwegian Sea Ecoregions. Available online at: http://www.ices.dk/sites/pub/Publication%20Reports/Advice/2020/2020/cod.27.1-2.pdf (accessed June 16, 2020).
Kaplan, I. C., Francis, T. B., Punt, A. E., Koehn, L. E., Curchitser, E., Hurtado-Ferro, F., et al. (2019). A multi-model approach to understanding the role of Pacific sardine in the California Current food web. Mar. Ecol. Prog. Ser. 617, 307–321. doi: 10.3354/meps12504
Kaplan, I. C., Koehn, L. E., Hodgson, E. E., Marshall, K. N., and Essington, T. E. (2017). Modeling food web effects of low sardine and anchovy abundance in the California current. Ecol. Model. 359, 1–24. doi: 10.1016/j.ecolmodel.2017.05.007
Kaplan, I. C., and Marshall, K. N. (2016). A guinea pig’s tale: learning to review end-to-end marine ecosystem models for management applications. ICES J. Mar. Sci. 73, 1715–1724. doi: 10.1093/icesjms/fsw047
King, R., Morgan, B. J., Gimenez, O., and Brooks, S. P. (2009). Bayesian Analysis for Population Ecology. London: Chapman & Hall/CRC.
Koehn, L. E., Essington, T. E., Marshall, K. N., Kaplan, I. C., Sydeman, W. J., Szoboszlai, A. I., et al. (2016). Developing a high taxonomic resolution food web model to assess the functional role of forage fish in the California Current ecosystem. Ecol. Model. 335, 87–100. doi: 10.1016/j.ecolmodel.2016.05.010
Landsberg, J. H., Flewelling, L. J., and Naar, J. (2009). Karenia brevis red tides, brevetoxins in the food web, and impacts on natural resources: decadal advancements. Harmful Algae 8, 598–607. doi: 10.1016/j.hal.2008.11.010
Lewy, H., and Vinther, M. (2004). A Stochastic Age-Length-Structured Multispecies Model Applied to North Sea Stocks. Copenhagen: International Council For The Exploration of the Seas.
Link, J. S. (2010). Ecosystem-Based Fisheries Management Confronting Tradeoffs. Cambridge: Cambridge University Press.
MacCall, A. D., Sydeman, W. J., Davison, P. C., and Thayer, J. A. (2016). Recent collapse of northern anchovy biomass off California. Fish. Res. 175, 87–94. doi: 10.1016/j.fishres.2015.11.013
McNamee, J. (2018). A multispecies Statistical Catch-at-Age Model for a Mid-Atlantic Species Complex. Ph. D. thesis, University of Rhode Island, Kingston, RI.
Methot, R. D., Wetzel, C. R., and Taylor, I. G. (2018). Stock Synthesis User Manual Version 3.30.12. NOAA Fisheries. Seattle, WA: NOAA Fisheries.
Nesslage, G. M., and Wilberg, M. J. (2019). A performance evaluation of surplus production models with time-varying intrinsic growth in dynamic ecosystems. Can. J. Fish. Aquat. Sci. 76, 2245–2255. doi: 10.1139/cjfas-2018-0292
O’Farrell, H., Gruss, A., Sagarese, S. R., Babcock, E. A., and Rose, K. A. (2017). Ecosystem modeling in the Gulf of Mexico: current status and future needs to address ecosystem-based fisheries management and restoration activities. Rev. Fish Biol. Fish. 27, 587–614. doi: 10.1007/s11160-017-9482-1
Okamoto, D. K., Poe, M. R., Francis, T. B., Punt, A. E., Levin, P. S., Shelton, A. O., et al. (2020). Attending to spatial social-ecological sensitivities to improve trade-off analysis in natural resource management. Fish Fish. 21, 1–12. doi: 10.1111/faf.12409
PFMC (2013). Terms of reference and report of the Pacific Sardine Harvest Parameters Workshop. Portland, OR: Pacific Fishery Management Council.
PFMC (2019). Coastal Pelagic Species Fishery Management Plan As Amended Through Amendment 17. Portland, OR: PFMC.
Pikitch, E., Boersma, P. D., Boyd, L., Conover, D., Cury, P., Essington, T. E., et al. (2012). Little Fish, Big Impact: Managing a Crucial Link in Ocean Food Webs. Washington, DC: Lenfest Ocean Program.
Plaganyi, E. E., Punt, A. E., Hillary, R., Morello, E. B., Thebaud, O., Hutton, T., et al. (2014). Multispecies fisheries management and conservation: tactical applications using models of intermediate complexity. Fish Fish. 15, 1–22. doi: 10.1111/j.1467-2979.2012.00488.x
Punt, A. E., MacCall, A. D., Essington, T. E., Francis, T. B., Hurtado-Ferro, F., Johnson, K. F., et al. (2016). Exploring the implications of the harvest control rule for Pacific sardine, accounting for predator dynamics: a MICE model. Ecol. Model. 337, 79–95. doi: 10.1016/j.ecolmodel.2016.06.004
Reum, J. C. P., Blanchard, J. L., Holsman, K. K., Aydin, K., Hollowed, A. B., Hermann, A. J., et al. (2020). Ensemble projections of future climate change impacts on the Eastern bering sea food web using a multispecies size spectrum model. Front. Mar. Sci. 7:124. doi: 10.3389/fmars.2020.00124
Sagarese, S., Bryan, M. D., Walter, J. F., Schirripa, M. J., Gruss, A., and Karnauskas, M. (2015). Incorporating Ecosystem Considerations within the Stock Synthesis Integrated Assessment Model for Gulf of Mexico Red Grouper (Epinephelus morio). North Charleston, SC: Southeast Data Assessment and Review.
Sagarese, S. R., Lauretta, M. V., and Walter, J. F. (2017). Progress towards a next-generation fisheries ecosystem model for the northern Gulf of Mexico. Ecol. Model. 345, 75–98. doi: 10.1016/j.ecolmodel.2016.11.001
Sanchez, S., Ibaibarriaga, L., Uriarte, A., Prellezo, R., Andres, M., Abaunza, P., et al. (2019). Challenges of management strategy evaluation for small pelagic fish: the Bay of Biscay anchovy case study. Mar. Ecol. Prog. Ser. 617, 245–263. doi: 10.3354/meps12602
SEDAR (2009a). SEDAR 10 Update: Gulf of Mexico Gag Grouper Stock Assessment Report. North Charleston, SC: Southeast Data Assessment and Review.
SEDAR (2009b). SEDAR 12 Update: Gulf of Mexico Gag Grouper Stock Assessment Report. North Charleston, SC: Southeast Data Assessment and Review.
SEDAR (2020). SEDAR 69: Atlantic Menhaden Ecological Reference Points Stock Assessment Report. North Charleston: Southeast Data Assessment and Review.
Semenov, M. A., and Stratonovitch, P. (2010). Use of multi-model ensembles from global climate models for assessment of climate change impacts. Clim. Res. 41, 1–14. doi: 10.3354/cr00836
Siple, M. C., Essington, T. E., and Plaganyi, E. E. (2019). Forage fish fisheries management requires a tailored approach to balance trade-offs. Fish Fish. 20, 110–124. doi: 10.1111/faf.12326
Smith, G. B. (1975). The 1971 red tide and its impact on certain reef communities in the mid-eastern Gulf of Mexico. Environ. Lett. 9, 141–152. doi: 10.1080/00139307509435843
Spence, M. A., Blanchard, J. L., Rossberg, A. G., Heath, M. R., Heymans, J. J., Mackinson, S., et al. (2018). A general framework for combining ecosystem models. Fish Fish. 19, 1031–1042. doi: 10.1111/faf.12310
Tebaldi, C., and Knutti, R. (2007). The use of the multi-model ensemble in probabilistic climate projections. Philos. Trans. R. Soc. Math. Phys. Eng. Sci. 365, 2053–2075. doi: 10.1098/rsta.2007.2076
Thayer, J. A., Maccall, A. D., Sydeman, W. J., and Davison, P. C. (2017). California anchovy population remains low, 2012-16. Calif. Cooperative Ocean. Fish. Investig. Rep. 58, 69–76.
Tommasi, D., deReynier, Y., Townsend, H., Harvey, C. J., Satterwhaite, W. H., Marshall, K. N., et al. (2020). Connecting fisheries management challenges with models and analysis to support ecosystem-based management in the california current ecosystem. Front. Mar. Sci. [Epub ahead of print].
Townsend, H., Aydin, K., Brodie, S., DePiper, G., deReynier, Y., Harvey, C. J., et al. (2020). Progress in Ecosystem Modeling For Living Marine Resource Management. Seattle, WA: NOAA Technical Memorandum.
Townsend, H., Harvey, C. J., Aydin, K., Gamble, R., Gruss, A., Levin, P. S., et al. (2014). Report of the 3rd National Ecosystem Modeling Workshop (NEMoW 3): Mingling Models for Marine Resource Management – Multiple Model Inference. Seattle, WA: NOAA Technical Memorandum.
Tracton, M. S., and Kalnay, E. (1993). Operational ensemble prediction at the national meteorological center - practical aspects. Weather Forecast. 8, 379–398. doi: 10.1175/1520-0434(1993)008<0379:oepatn>2.0.co;2
Trenkel, V. M., Hintzen, N. T., Farnsworth, K. D., Olesen, C., Reid, D., Rindorf, A., et al. (2015). Identifying marine pelagic ecosystem management objectives and indicators. Mar. Policy 55, 23–32. doi: 10.1016/j.marpol.2015.01.002
Uphoff, J. H., and Sharov, A. (2018). Striped bass and atlantic menhaden predator-prey dynamics: model choice makes the difference. Mar. Coast. Fish. 10, 370–385. doi: 10.1002/mcf2.10030
Walter, J. F., Christman, M. C., Landsberg, J. H., Linton, B., Steidinger, K., Stumpf, R., et al. (2013). Satellite Derived Indices of Red Tide Severity for Input for Gulf of Mexico Gag Grouper Stock Assessment. North Charleston, SC: Southeast Data Assessment and Review.
Keywords: model ensemble, ecosystem model, ecosystem-based management, uncertainty, ecosystem-based fisheries management, multispecies model
Citation: Reum JCP, Townsend H, Gaichas S, Sagarese S, Kaplan IC and Grüss A (2021) It’s Not the Destination, It’s the Journey: Multispecies Model Ensembles for Ecosystem Approaches to Fisheries Management. Front. Mar. Sci. 8:631839. doi: 10.3389/fmars.2021.631839
Received: 21 November 2020; Accepted: 18 January 2021;
Published: 26 February 2021.
Edited by:
Tomaso Fortibuoni, Higher Institute for Environmental Protection and Research (ISPRA), ItalyReviewed by:
Andrea Belgrano, Swedish University of Agricultural Sciences, SwedenDaniel Howell, Norwegian Institute of Marine Research (IMR), Norway
Copyright © 2021 Reum, Townsend, Gaichas, Sagarese, Kaplan and Grüss. This is an open-access article distributed under the terms of the Creative Commons Attribution License (CC BY). The use, distribution or reproduction in other forums is permitted, provided the original author(s) and the copyright owner(s) are credited and that the original publication in this journal is cited, in accordance with accepted academic practice. No use, distribution or reproduction is permitted which does not comply with these terms.
*Correspondence: Jonathan C. P. Reum, Sm9uYXRoYW4uUmV1bUBub2FhLmdvdg==