- 1Alaska Fisheries Science Center, NOAA Fisheries, Seattle, WA, United States
- 2Northeast Fisheries Science Center, NOAA Fisheries, Woods Hole, MA, United States
- 3Cooperative Institute for Marine and Atmospheric Studies, Rosenstiel School of Marine and Atmospheric Science, University of Miami, Coral Gables, FL, United States
- 4Northeast Fisheries Science Center, NOAA Fisheries, Narragansett, RI, United States
- 5National Centers for Coastal Ocean Science, NOAA, Oxford, MD, United States
- 6Southeast Regional Office, NOAA Fisheries, St. Petersburg, FL, United States
- 7Southeast Fisheries Science Center, NOAA Fisheries, Miami, FL, United States
- 8Pacific Islands Fisheries Science Center, NOAA Fisheries, Honolulu, HI, United States
- 9Office of Science and Technology, NOAA Fisheries, Silver Spring, MD, United States
- 10Southeast Fisheries Science Center, NOAA Fisheries, Galveston, TX, United States
- 11Southwest Fisheries Science Center, NOAA Fisheries, Santa Cruz, CA, United States
There has been a proliferation of coupled social-ecological systems (SES) models created and published in recent years. However, the degree of coupling between natural and social systems varies widely across the different coupled models and is often a function of the disciplinary background of the team conducting the research. This manuscript examines models developed for and used by NOAA Fisheries in support of Ecosystem Based Fisheries Management (EBFM) in the United States. It provides resource managers and interdisciplinary scientists insights on the strengths and weaknesses of the most commonly used SES models: end-to-end models, conceptual models, bioeconomic models, management strategy evaluations (MSEs), fisher behavior models, integrated social vulnerability models, and regional economic impact models. These model types are not unique to the literature, but allow us to differentiate between one-way coupled models – where outputs from one model are inputs into a second model of another discipline with no feedback to the first model, and two-way coupled models – where there are linkages between the natural and social system models. For a model to provide useful strategic or tactical advice, it should only be coupled to the degree necessary to understand the important dynamics/responses of the system and to create management-relevant performance metrics or potential risks from an (in)action. However, one key finding is to not wait to integrate! This paper highlights the importance of “when” the coupling happens, as timing affects the ability to fully address management questions and multi-sectoral usage conflicts that consider the full SES for EBFM or ecosystem based management (EBM) more generally.
Introduction
The concept of social-ecological systems (SES) was applied and popularized by Berkes and Folke (1998) who argued that the solution to resource management problems was not in increasing the complexity or performance of environmental and economic models, but rather in recognizing the feedbacks that occur between the two disciplines. Since their initial applications, the SES concept has been used in a wide range of fields and examples have proliferated, and yet the term remains poorly defined (Colding and Barthel, 2019). Here, we define a social-ecological system similar to Anderies et al. (2004) as: an ecological system of interdependent organisms or biological units interacting with a social system of interdependent humans deriving benefits from uses of the ecosystem as well as from the state of the ecosystem. These SESs can be represented by quantitative or qualitative models, however, in all cases, models are an abstraction from reality, and the direction of abstraction has strong bearing on the questions which can be answered with the model. Further, the exact manner in which the coupling between social and ecological systems is conceptualized has implications on the kind and variety of management questions that can be addressed by each coupled SES model. This manuscript discusses a number of approaches to creating coupled SES models used in the United States and provides resource managers and interdisciplinary scientists a guide for choosing when, how, and why to couple SES models. We discuss trade-offs between modeling approaches, including data requirements, the speed and scale at which the coupled model can be integrated across disciplines, model development stage by which the coupling is no longer possible/fruitful, and other issues which affect model utility in a management and scientific context. By considering each model’s ability to answer management-relevant questions as well as its development costs, we aim to provide interdisciplinary scientists and resource managers with a better understanding of not only why coupled models are important, but also what options are available for coupling depending on where in the development process they stand, the relevant research and management questions, and the time horizon in which answers are needed.
The backdrop for this manuscript is the United States National Oceanic and Atmospheric Administration’s (NOAA) Ecosystem Based Fisheries Management (EBFM) roadmap, published in 2016 (NOAA Fisheries, 2016). NOAA Fisheries defines EBFM as “a systematic approach to fisheries management in a geographically specified area that contributes to the resilience and sustainability of the ecosystem; recognizes the physical, biological, economic, and social interactions among the affected fishery- related components of the ecosystem, including humans; and seeks to optimize benefits among a diverse set of societal goals.” The EBFM roadmap was introduced into a well-established system in the United States where regional fishery management council harvest regulations rely on fishery reference points established in an analysis and review process based principally on individual species stock assessments. A recent review compares how current United States, Canadian, and European Union management approaches incorporate changing environmental conditions (ICES, 2021). EBFM provides a more effective and holistic approach to fisheries management than single species management by accounting for species interactions and environmental effects into the management process (Pikitch et al., 2004; Marshall et al., 2018), and the roadmap is the set of incremental steps to achieve that end.
The EBFM roadmap makes clear that modeling efforts should be coupled social-ecological endeavors to allow for effective trade-off analysis, and that they can run the gamut from qualitative conceptual models through quantitative end-to-end (i.e., from nutrients to apex predators to human uses) models. This manuscript describes the current state of coupled SES modeling within NOAA Fisheries and focuses on how these coupled models are used in support of management decision- making. Thus our focus is on fishery management-centric representations of the SES, but the framework and approach would be relevant to other sectors as well as more broadly for Ecosystem Based Management (EBM). This manuscript is not a survey of all the relevant scientific literature on coupled SES models. Rather, it is focused specifically on coupled models most frequently used to assess trade-offs within and across United States fisheries, although some discussion of the importance of coupled models in assessing trade-offs across ocean use sectors is provided in the discussion that follows. Many of the models included will contribute to NOAA Fisheries Integrated Toolbox, an ongoing effort to increase the ease of utilizing and integrating diverse models in fisheries management. This fisheries-specific focus allows us to assess model uptake by a specific clientele, the eight United States Regional Fishery Management Councils, and to identify where resource managers supported uptake across these case studies.
We begin by defining the organizing framework employed throughout the manuscript, including general data requirements and modeling complexities, before reviewing the management relevance with respect to questions each type of model can address and assessing management uptake. The discussion which follows looks to explicitly detail trade-offs across types of models, and highlight commonalities in case studies of management uptake, while the conclusion situates the current manuscript in the broader literature.
Materials and Methods
Natural and social science researchers met in St. Petersburg, FL, United States from December 9th to 11th, 2019 for the NOAA Fisheries National Ecosystem Modeling Workshop (NEMoW) to discuss a variety of ecosystem modeling approaches and challenges (Townsend et al., 2020). In preparation for NEMoW, participants were asked to fill out a questionnaire about the models either developed since 2012 or currently under development in their region of the United States, with particular interest in those which have been used to support fisheries management decision-making. This questionnaire identified the coupled SES models in each of the eight regions in which United States federal fisheries are managed, how frequently they are used or updated, a description of the model including the problem or question it addresses, the data requirements, model structure, and the coupled component, as well as whether the model has traction with managers and what factors contributed to or hindered management uptake. The aim of the questionnaire was not to develop a survey of the literature, as a number of examples are already available (e.g., Plagányi, 2007; Prellezo et al., 2012; Schluter et al., 2012; Stojanovic et al., 2016; Nielsen et al., 2018). Neither was it to develop a typology for coupled SES models. Rather, the aim was to delineate the capacity of commonly used coupled SES models to address management relevant questions and highlight the best uses for each through the reference to existing work. The accompanying discussion is aimed at briefly introducing these models to managers and scientists interested in interdisciplinary work, and highlight the importance and utility of coupling social and ecological systems. We identified over 30 individual models with some level of coupled SES components within the 11 United States large marine ecosystems (LMEs)1.
They represented seven commonly used types of models: end-to-end models, conceptual models, bioeconomic models, management strategy evaluations (MSEs), fisher behavior models, integrated social vulnerability models, and regional economic impact models, summarized in Table 1. A non-exhaustive list of coupled SES models considered for use by fisheries managers in the United States is summarized in Supplementary Appendix Table 1. During our workshop, we discussed commonalities across models, how they were developed, and how they were applied to management questions. We identified three core frameworks for further analysis: type of coupling, phase of project life cycle when the model was coupled, and category of management questions.
Here we define two types of SES coupled models which are distinguished by whether or not there are model linkages and feedbacks between the natural and social system (Figure 1). We distinguish between One-way coupled models, where outputs from one model are inputs into a second model with no connection back to the first model, and Two-way coupled models where there are feedbacks between the natural and social system. A One-way coupled model could begin by taking the output from a natural systems model and incorporating it into a social systems model to create a Natural-Social One-way Coupled Model or use output from a social systems model as input into a natural systems model, creating a Social-Natural One-way Coupled Model. Two-way coupled models require model linkages and feedback-loops between both the social system model and the natural system model.
One commonality across all model types and potential management questions was that “when” the coupling happened fundamentally affected the modeler’s ability to improve the model and the model’s ability to address complete management questions that consider the full SES. Based on model descriptions, we identified four key phases for project life cycle and entry point for social-ecological coupling: (1) Project Design and Scoping, (2) Model Development, (3) Model Assessment, and (4) Management Strategy Assessment (Figure 2). Next, we describe each project phase and the implications of coupling at this stage in terms of attainable degree of social-ecological Two-way coupling, from limited One-way coupling when integrated late in the project phase to complete Two-way coupling when integrated early. There is a continuum of degrees of coupling across both One-way and Two-way coupled models, and the level of attainable coupling is not always a function of the type of model, but rather when the natural and social system models are coupled.
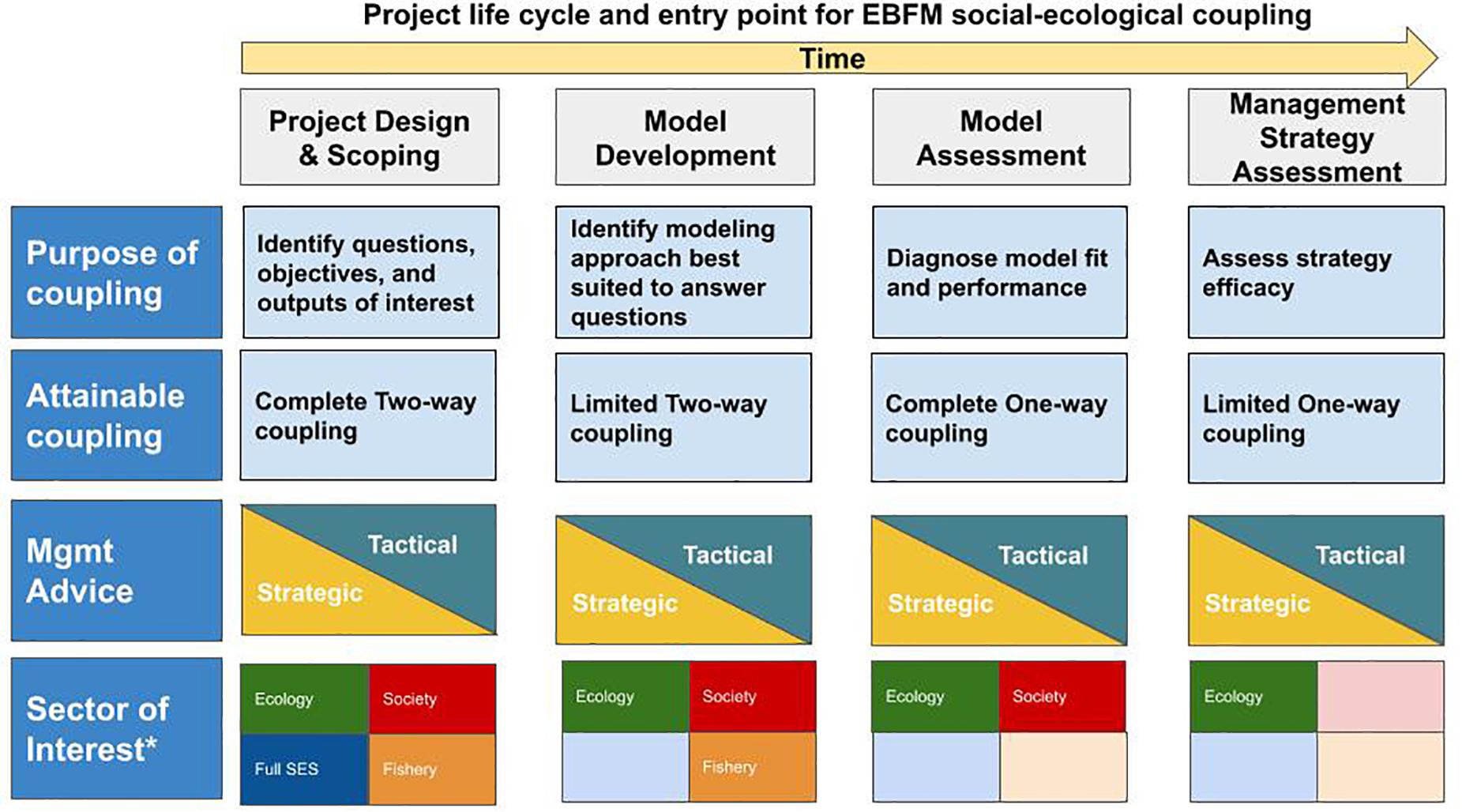
Figure 2. Project life cycle and entry point for EBFM social-ecological coupling. *Note: The four sectors of interest for management questions are Understanding Ecosystem Connections and Function (shorthand “Ecology”), Assessing the Impact of Environmental and Management Changes on Fisheries (shorthand “Fisheries”), Understanding the Distributional Impacts of Management Policies and Environmental changes on Society and the Economy (shorthand “Society”), and a Full SES Approach Integrating the other Three Sectors (shorthand “Full SES”).
Results: Don’t Wait to Integrate! Limitations Resulting From Delayed Coupling
For a model to be useful for a specific management question or issue, it should be coupled to the degree necessary to understand the system and have management-relevant performance metrics that can be used to assess the degree of management success or potential risks from an (in)action. Each model type described in Table 1 can be either minimally or fully coupled (Figure 1) and can also provide either strategic or tactical fisheries management advice.
Kaplan and Leonard (2012) and Fay et al. (2019) are two examples of Natural-Social One-way Coupled Models, where outputs from an Atlantis model were passed to an economic input-output model to estimate economic impacts on communities and regions caused by changes in seafood landings. One-way coupled models can also start from the model output from a social systems model and combine it with a natural systems model creating a Social-Natural One-way Coupled Model, such as Ruzicka et al. (2019). They combine the output of a series of fishery production models for nine fleets catching halibut and arrowtooth flounder in the Gulf of Alaska and uses those fleets in a (nutrients to fisheries) end-to-end model CGOA-ECOTRAN to evaluate the impact of alternate levels of fishing effort and large-scale changes in oceanographic conditions.
Examples of Two-way coupled models include bioeconomic models which incorporate a stage-structured population model of Bristol Bay Red King Crab (Punt et al., 2014b) and southern Tanner crab (Punt et al., 2016). By varying ocean acidification conditions they are able to estimate the long-term maximum economic yield (MEY) (as well as other reference points) in these fisheries as a result of having Economic Data Report (EDR) data available to parameterize the economic component of the model (Punt et al., 2014a, b).
We found that each type of model could be created to provide strategic (general and/or long-term) or tactical (action-specific and/or short-term) advice. The decision to create a One-or Two-way coupled model depended on the management objective and the timeframe in which results were needed. Not all One-way coupled models were simpler or less time consuming to create or run, nor were all Two-way coupled models necessarily more complex or did they take longer to develop and implement.
Project Design and Scoping
Project Design and Scoping is the very beginning stage of project formulation before hypotheses, objectives, and the full scope of research are defined. Developing a coupled model at this stage implies integration of both natural and social scientists in designing the project scope. This multi-disciplinary collaboration is necessary to create a fully Two-way coupled model of the SES that can meaningfully answer complex ecological and social questions that do not impact exclusively either the ecological or social system. The purpose of coupling at this stage is to better identify questions, objectives, and performance metrics of interest to the management and stakeholder communities. Only when social and natural scientists along with managers and resource users work together from the project design and scoping phase can truly integrated two-way coupled models be developed. An example of such a Two-way coupled model is an end-to-end model (Box 1). The development of integrated and coupled social and natural systems models can often span multiple years. It is very infrequent that two existing models can be fully integrated to produce Two-way coupled end-to-end models which address the relevant management priorities. Hence, this long timeframe to co-develop research questions and models should be reflected explicitly in funding priorities and approaches to enable this type of integrated research2. The utility of end-to-end models in a management context is the ability to quantify the impact(s) of dynamic system processes on the distribution and abundance of living marine resources and the overarching impact on long- term ecosystem resilience [e.g., the role of interspecies interactions on developing sustainable, harvest policies (Masi et al., 2018), the frequency of stock assessment updates (Hutniczak et al., 2019), and how ecosystem resilience impacts (conflicting) human use of the ecosystem (e.g., extractive vs. non-extractive use) (Weijerman et al., 2016)].
Box 1. End-to-end models.
An end-to-end ecosystem modeling platform encompasses parameters that allow for explicit representation of the marine environment (Link, 2010). In particular, an end-to-end model captures the realism and dynamics of the biological, physical, chemical and (social-) economical processes of the ecosystem across spatial scales, and the two-way interactions of those spatial and temporal processes across the food web (Rose et al., 2010). Tam et al. (2019) emphasize the need to continue to develop ecological and social and economic indicators of ecosystem health along with advances in end-to-end modeling, rather than being solely derived from model output. Typically, end-to-end models are driven with historical time series of ecosystem dynamics (e.g., physics from regional ocean models, fisheries catch time series, spatial and temporal nutrient inputs) and are projected forward in time based on assumptions of stationarity of input parameters (e.g., maximum individual growth remains constant over time). As an example, in the end-to-end Atlantis modeling platform, population dynamics of marine species are coupled to fishing fleet dynamics and an economics sub-model (Fulton et al., 2004, 2011). End-users can customize this sub-model to simulate a range of exploitation (e.g., changes in fleet behavior) and management scenarios (e.g., TACs, temporal and spatial closures), which are affected by revenue and quota limits (Audzijonyte et al., 2019). Recent applications of Atlantis incorporated calculation of metrics such as employment and wellbeing (Fay et al., 2019; Fulton et al., 2019).
Other examples of a two-way coupling modeling approach that can be particularly effective early in the project life cycle are conceptual and causal models (see Box 2). Conceptual and causal models are usually used to make sense of relationships and linkages within a system; these linkages are often developed in consultation with stakeholders to facilitate stakeholder participation and integrate diverse sources of knowledge of the system (Düspohl et al., 2012). This collaboration allows for a common understanding of all system aspects and gives individuals who are impacted by resource management decisions an opportunity to include relationships that are important to them. Furthermore, tapping into the collective knowledge of a large and diverse group of resource users can lead to a robust understanding of the SES and overcome scientific data gaps with regard to linkage (Aminpour et al., 2020). Thus, when projects are designed from the outset with important ecological and societal issues determining the outcomes of interest, these models can be fully coupled and address the entire SES, in addition to impacts on the ecology and outcomes on the fishery and society and vice versa.
Box 2. Conceptual and causal models.
Conceptual models are graphic representations of relationships among key components of an ecosystem including management options, e.g., factors (climate change, management scenarios) that influence the ecological state components, and how changes in ecological state components influence social state components and vice versa. These models can integrate social, economic, and ecological objectives through the identification of relationships among these aspects. Conceptual models can be operationalized into dynamic simulation frameworks through techniques such as the creation of Qualitative Network Models (Harvey et al., 2016), cognitive fuzzy mapping (Martin et al., 2019), and Bayesian Belief Networks (Little et al., 2004). When these models explicitly describe hypotheses about how cause and effect propagate throughout a SES, they are generally described as causal models and have been used across a variety of natural and social science disciplines (Cheng et al., 2020). Conceptual modeling can also be carried out in a participatory manner in order to engage individuals who are impacted by resource management decisions and capture their perspectives and knowledge of the systems being modeled.
Model Development
The Model Development phase typically occurs once a team has been established focused on a general research topic and a model is developed for a funding proposal. More often than not, coupling at this stage occurs in a limited two-way fashion between a very complicated social and/or economic model and a relatively simplistic representation of the ecology (see Box 3 on Bioeconomic models) or a similarly complicated ecological and/or biological model with a simple representation of the human system [see Box 4 on Management Strategy Evaluations (MSEs)].
Box 3. Bioeconomic models.
Bioeconomic models have a long history of use in fisheries management, including the foundational works of Gordon (1954), Schaeffer (1957), and Smith (1969) establishing the framework to explore the connections between the biology of a species and the economics of the harvesting sector. These early coupled bioeconomic models were fairly simplistic mathematical models of catch and fishing effort with surplus production stock dynamics and are well summarized in Clark (1990). These models typically have some type of objective function which is either optimized or simulated to explore the tradeoffs across different potential harvest strategies, and can be used to assess the impact of past or future management actions on the fishery and the fish stock. However, advances in the science of stock assessments, ecology, and fisheries economics have led to increasingly complex and data intensive models of stock dynamics, fisher behavior, and decision making often resulting in bioeconomic models with cutting-edge science in one discipline and a fairly simplistic representation of the other discipline (e.g., Kasperski, 2015; DePiper et al., 2017). This highlights the need to couple bioeconomic models so that these models represent the best available science to inform fisheries managers, not just advances within our own disciplines that others find implausible or highly speculative. There continue to be advances toward more fully coupled bioeconomic models such as Punt et al. (2014b) and Punt et al. (2016) with stock assessment and economic models that are both cutting-edge. Surveys of the literature include, Plagányi (2007), Prellezo et al. (2012), and Nielsen et al. (2018).
Box 4. Management strategy evaluation.
A management strategy evaluation (MSE) is a simulation study used to evaluate the performance of one or more preferred management actions (e.g., a fishing harvest rate). The simulation study will often consider variation among a broad range of biological or economic uncertainties (Punt et al., 2014a). A MSE can be a tactical or strategic application, and is typically conducted as a “closed-loop” simulation. A tactical MSE is used to address a short-term, specific management action [e.g., increasing the acceptable catch limit (ACL) for a chosen species], whereas a strategic MSE would explore a range of “what-if” scenarios and thus may not focus on any one outcome (Punt et al., 2016). A “closed-loop” MSE is a dynamic simulation that initializes by acquiring information about the operating model based on an individual or set of predefined indicators. For example, an indicator may be the available biomass of a given species. This information is acquired from the operating model (typically with added observational error) and then fed into the sampling model, where management thresholds (e.g., an overfishing limit) will be implemented (typically implementation error is added here). For example, if the available biomass is below the established threshold then the fishing allocation(s) will be limited in the subsequent cycle of the MSE loop (or until the simulation ends). The closed-loop simulation continues to iterate on an annual cycle, where the duration of the full simulation is based on the management objective. The coupling of the MSE cycle to a bioeconomic model may occur following the closed-loop MSE simulation. For example, MSE outputs from an end-to-end model being input into a bioeconomic model would be considered a one-way coupling. Goethel et al. (2019) provide an example of a two-way coupled MSE model where they integrated stakeholder engagement iteratively throughout each stage of the MSE.
The intent of coupling the ecological component of the model with a social component during the model development phase of a project is to assist the team in identifying the best modeling approaches to answer the set of already defined questions. In this phase, two-way coupling of the models is possible, but the questions that the coupled model will be able to answer will necessarily be a subset of those the entire interdisciplinary research team would have developed had they been involved from the start. However, this may be the best approach to take in some circumstances with well-defined questions that are somewhat limited in scope and a complete picture of the impact on the full SES is unnecessary or not feasible given regulatory or time constraints. The Bio-economic Length Age Structured Tool or “BLAST” Model (Lee et al., 2017) is one such example. It is a bioeconomic model which combines a utility-theory consistent model of recreational fishing demand and an age-structured stock dynamics model to help provide harvest advice to fisheries managers, initially developed in the Northeast United States.
Often, the way social and economic coupling occurs in this stage in an effort at implementing EBFM policies are through models of fisher behavior to better understand the economic and social costs and benefits of specific management rules and regulations (Abbott and Haynie, 2012; Abbott et al., 2015; Reimer et al., 2017; see Box 5). These models can either be created prospectively (as a fishery management council, regional planning body, or other management body is considering the impact of multiple alternatives) or through a retrospective analysis of the economic and social impacts of a management or environmental change.
Box 5. Fisher behavior.
Fisheries economists have employed discrete choice models and several other spatial models since the 1980’s in a number of fisheries to better understand and statistically explain what factors influence the spatial and participation choices that fishers make across fisheries and fishing grounds (Bockstael and Opaluch, 1983; Smith and Wilen, 2003; Girardin et al., 2017). Two key characteristics that economists have identified from this research is that (1) fishers are drawn to higher catch rates and revenues; and (2) travel costs are reduced whenever possible (e.g., Eales and Wilen, 1986; Haynie and Layton, 2010). Researchers have also been able to estimate the costs of different hypothetical and actual spatial closures (e.g., Reimer and Haynie, 2018).
Fisheries managers are often faced with decisions that may close areas or limit catch of certain species to fisheries, and so wish to know how this will affect fishers and in turn how the fishers will respond to hypothetical closures or changes in the environment. While there has been a significant amount of research in the location choice sub-field of economics, only a very limited amount of this research has directly informed decision makers. The primary goal of the nearly complete NOAA Fisheries Spatial Economic Toolbox for Fisheries (FishSET) is to help managers and analysts better answer spatial management questions as they are making decisions and framing policy options (Haynie, 2015).
The class of models that are coupled at the model development stage, whether One-way coupled or limited Two-way coupled, tend to have moderate or moderate-high management traction, which is intuitive because these are often the type of models that are created to address specific management problems. As a result, these models tend to focus on modeling fishing fleet dynamics (Branch et al., 2006; Watson and Haynie, 2018) and the impact of regulations, such as spatial or temporal closures (Abbott and Haynie, 2012; Reimer and Haynie, 2018), on the fishing industry and on society at large (Sanchirico et al., 2013).
Model Assessment
This is the phase of a project where the modeling team has a model developed to explain some real world phenomenon and is trying to assess the degree to which their model reflects reality or to assess the potential implications of the model to society. This phase can happen during or after the initial publication of the basic natural science or social science model manuscript(s) which often form the basis for creating an integrated SES model where the coupling occurs during the Model Assessment phase. The Integrated Social Vulnerability class of models (see Box 6) often represent One-way coupling of natural and social science models via the integration of a model of the risk to a natural and/or man-made hazard [such as climate change (Hare et al., 2016)] and a model of social vulnerability and/or adaptive capacity (Jepson and Colburn, 2013) specifically in regards to the risk from climate change and sea-level rise to coastal communities, as in Colburn et al. (2016). That study is an example of both a qualitative one-way coupling in integrating the species and community diversity metrics as well as a quantitative one-way coupling between sea-level rise risk and the number of marine businesses affected.
Box 6. Integrated social vulnerability.
The intersections of social vulnerability metrics and natural hazards vulnerabilities is a specific application of an integrative model to a particular scenario, but one that has been applied successfully around the world in a wide variety of contexts [e.g., based on Susan Cutter’s Social Vulnerability Index (SoVI) to environmental hazards (Cutter, 2003)]. Communities need to plan for and respond to natural disasters and human-made harmful events. Various factors influence the community’s ability to mitigate the impacts. These factors, such as poverty, access to transportation, number of people per household, are known as social vulnerability. In the United States, the socio-economic and demographic data for these factors is commonly derived from Census data. The SoVI is meant to be used to make sense of the social system in comparison to natural hazards and built infrastructure in order to determine where hazards will have the largest and longest impact (Cutter, 2009). Because of SoVI’s reliance on Census data, geographic comparisons and time series analysis are also possible in order to determine the dynamics of vulnerability in space and time (Cutter and Finch, 2008). NOAA’s National Centers for Coastal Ocean Science (NCCOS) has incorporated SoVI into an Integrated Vulnerability Framework to examine geographic variability in and overlaps between social vulnerability, natural resource vulnerability, and structural vulnerability to natural hazards such as sea level rise, storm surge, stormwater flooding, heat, drought, and wildfire (Messick et al., 2016; Fleming et al., 2017). The geospatial approach of the Integrated Vulnerability Framework is designed to help communities qualify for adaptation grants and programs by demonstrating need, contextualize relative vulnerability among neighboring communities, and prioritize areas where adaptation programs can deliver benefits to communities most in need.
With a slightly different focus on social impact assessment and satisfying United States Magnuson-Stevens Act National Standard 8 about sustaining fishing communities of place, NOAA Fisheries has developed a series of Community Social Vulnerability Indices (CSVIs) to identify fishing communities that may be susceptible to the adverse impacts of regulatory change (Jepson and Colburn, 2013). However, the CSVIs are grounded in a broader effort to gauge the ability of coastal communities to adapt to change, especially from climate change, and how that adaptation contributes to overall community well-being and natural resource use. The CSVIs were expanded to include measures of risk from both sea level rise (Colburn et al., 2016) and storm surge. Most recently, the CSVIs have been updated to include trend data from 2009 through 2018 to better understand how these communities are adapting to change over time and how vulnerabilities may play a role in that adaptation.
In addition to coupling to describe the social impacts of changes in the marine environment, coupling at the Model Assessment phase can also help describe the economic impacts of these changes to the society as a whole, often through the use of One-way coupled Regional Economic Impact Models (see Box 7). These models have been integrated similarly as quantitative one-way coupled models where a climate-informed stock assessment model is used to generate a series of projections of future stock biomass and catch of the projection period (Ianelli et al., 2011) and the changes in fisheries yield is then used as an input in a dynamic computable general equilibrium (CGE) model of the Alaska fisheries and non-fisheries economy (Seung and Ianelli, 2016, 2019).
Box 7. Regional economic impact models.
There are a number of models that can assess the broader economic activity associated with recreational and commercial fisheries, beyond the fishers themselves. Regional economic impact models estimate the difference in economic activity, expressed in terms of sales, income, value-added, or employment, with and without a policy or environmental change [see Loveridge (2004), Seung and Waters (2006), and Seung (2015) for good reviews of these models]. The predominant approach utilized in coupled socio-ecological modeling endeavors are Regional Input-Output models. Regional Input-Output models were originally developed by Leontief (1951), and assess direct and indirect impacts from changes in landings revenue. This means they trace the impact of revenue changes to not only the fishing businesses themselves, but also forward to sectors that use seafood produced by the fishing sector (e.g., seafood processors, dealers, restaurants) and backward to business that supply inputs to fishing (e.g., marinas, ice and bait suppliers, marine repair and supply shops). The major drawback to this modeling framework is that it is static, meaning behavioral responses to changes are not captured. The analyses resulting from these models are thus best viewed as identifying impacts in the economy due to changes in landings, but not estimating changes in welfare itself which would be a more meaningful metric for policy analysis. Other frameworks such as Input- Output Linear Programming (e.g., Kirkley et al., 2011), Computable General Equilibrium (CGE, e.g., Jin et al., 2012; Seung and Ianelli, 2016), and dynamic CGE (e.g., Seung et al., 2015) have been used for coupled socio-ecological modeling exercises to better assess how behavior is likely to change due to system changes. However, the complexity of these models means that the nuanced differences across fishing fleets that are key in a management context can be lost through aggregation. The trade-off between these approaches thus depends on the question being addressed and ultimate application.
Management Strategy Assessment
The human activity most commonly included in existing United States EBFM coupled modeling efforts is fishery catch. However, coupling at the Management Strategy Assessment stage usually implies that catches are not driven by any kind of behavioral model of fishers but rather based upon simple assumptions of fisheries mortality rates. Coupling at this stage is often a simple quantitative one-way relationship in which a series of alternative catch projections are multiplied by some fixed price to assess potential “economic impacts” of these different catch projections. These models can have some utility in fisheries and stocks in which the total TAC is caught nearly every year (full utilization) and catch projections are unlikely to change the relative prices across alternative target species. However, these models may perform poorly when the catch projections have an impact on the overall size of the catch over time through size-based targeting and production strategies among the fleet, as shown by Chen (2018) in the Bering Sea pollock fishery. These models may also perform poorly in situations where bycatch or quota constraints of other species jointly caught with the target species of interest may result in lower than full TAC utilization, as can happen in the New England and Bering Sea groundfish fisheries, more often prior to the implementation of catch shares (Brinson and Thunberg, 2013, 2016). As this coupling occurs so late in the process, it generally still only provides meaningful information about the ecological impacts of proposed management strategies or environmental changes but the impacts to society and the fishery are largely through narrative description. Thus these types of models may be useful to assess the management strategies across ecological objectives, but are unlikely to provide substantial useful information about the social or economic impacts of these ecological outcomes. Integrated models that account for management and fishing responses to changing physical and economic responses will provide more realistic projections and understanding of uncertainty (e.g., Hollowed et al., 2020; Reum et al., 2020).
Discussion
As shown in Table 1, there is overlap between potential model capabilities, depending on how the model is created and for what purpose. We further categorize these different types of coupled SES models by the EBFM sector they inform or for which management questions they are most appropriately designed to address. The four categories of management questions (depicted in Figure 2) are: Understanding Ecosystem Connections and Function (shorthand “Ecology”), Assessing the Impact of Environmental and Management Changes on Fisheries (shorthand “Fisheries”), Understanding the Distributional Impacts of Management Policies and Environmental changes on Society and the Economy (shorthand “Society”), and a Full SES Approach Integrating the other Three Sectors (shorthand “Full SES”). The section below provides examples by EBFM sector of applying models to management questions.
Understanding Ecosystem Connections and Function (Ecology)
The therMizer model was developed in order to better understand the effects of rising ocean temperature and changing plankton communities on fish size and abundance (Woodworth-Jefcoats et al., 2019). It is a size-based food web model with individual species represented, gear-specific fishery, and effects of temperature on metabolism and aerobic scope. It can incorporate dynamic fishing scenarios and the output can be used to estimate changes in catch value as a result of modeled climate and/or fishing scenarios, but is not intended to explain changes in fisheries behavior from any non-ecological basis.
Initial modeling results from the Alaska Climate Integrated Modeling (ACLIM) project are focused on key fisheries management areas of concern about climate change and species distribution shifts in the Eastern Bering Sea (Hermann et al., 2019; Holsman et al., 2020; Reum et al., 2020). The ACLIM project relies on repeated communication with stakeholders and managers to assess potential climate change effects as well as potential management and fleet responses to the changes they are currently experiencing (Hollowed et al., 2020). As the ACLIM project develops further, connections will be made to integrate impacts beyond commercial fisheries to create a series of integrated end-to-end models that explore a suite of climate scenarios with a variety of fisheries fleet dynamics models and potential management instruments.
Assessing the Impact of Environmental and Management Changes on Fisheries (Fisheries)
Although less prevalent than their commercial counterparts, recreational fishery bioeconomic models are also employed in assessing the impact of alternate regulations on fisheries and stocks. In the Northeast United States, a multispecies SES for cod and haddock is used to assess the impact of differing possession and size limits and seasonal closures on both stocks, as well as changes in the recreational welfare derived from this mixed recreational fishery due to the regulations (Lee et al., 2017). The model provides two-way coupling between an economic recreational demand module based on choice experiment survey data and an age-structured stock dynamics module. Recreational landings and discards are estimated based on fishing regulations, stock structure, and recreational effort derived from expected utility maximization. The resultant fishing mortality is passed to the stock dynamics module, which allows estimation of alternate stock trajectories based on variability around initial conditions, uncertainty in recruitment, and changes in regulations. These changes in stock conditions will, in turn, affect future recreational fishing behavior.
Researchers at the Northeast Fisheries Science Center developed the model and a joint Northeast Fishery Management Council (NEFMC)/Mid-Atlantic Fishery Management Council (MAFMC) panel reviewed the model in 2012. The authors note challenges to employing the model in support of resource management decision-making includes time lags in and uncertainty around the scientific information used, as well as inflexibilities in the management system, which results in a condensed period for model updating and an undermining of stakeholder trust in the management process due to the use of outdated information to assess current conditions. Results from the first implementation of the model in support of fisheries management indicate that although changes in regulations had substantial impacts on the recreational welfare generated, minimal long-run conservation value was derived from even the most draconian alternatives assessed. This result actually facilitated management uptake, as it was viewed favorably by stakeholders, minimizing concerns around the adoption of a novel approach for specification setting which might have otherwise hampered adoption. Since 2013, this model has been employed to support the selection of recreational groundfish measures for the Gulf of Maine in each round of specification setting. Revised versions of the choice experiment were conducted in 2014 and 2019. The simulation model has also since been further refined at the request of the NEFMC to allow for analysis of slot limits and regulations that vary within the year or by fishery mode.
Understanding the Distributional Impacts of Management Policies and Environmental Changes on Society and the Economy (Society)
To better understand ecosystem function and the deep connections local stakeholders have with the marine environment, conceptual models of the SES can be developed in partnership between scientists and resource users at a local scale, such as for the case of the community of Sitka, Alaska, and Sitka Sound (Rosellon-Druker et al., 2019), or at a regional-or ecosystem-scale (Harvey et al., 2016). These “place-based” SES models have been developed as part of an Integrated Ecosystem Assessment (IEA) in which the two-way coupled conceptual models are used to understand the multifaceted nature of well-being in local communities and to generate a set of feasible indicators of community well-being related to their interactions with the marine environment (Szymkowiak and Kasperski, 2021). The repeated interaction with the community of Sitka has helped generate trust between the researchers and community members, and they appreciate the availability of performance metrics that reflect how they interact with the marine environment, but these models and metrics have not been designed to support any specific management decision and are generally limited to providing local context for decision makers.
Full SES Approach Integrating the Other Three Sectors (Full SES)
Kaplan and Leonard (2012) offer one example of a simple One-way coupling from an end-to-end model to a regional input-output model. In this work, catch projections from Atlantis (Fulton et al., 2011) ecosystem model scenarios (Kaplan et al., 2012) were passed to the IOPAC input-output model (Leonard and Watson, 2011). This allowed the authors to evaluate the economic impact (in terms of jobs and income in the broader economy) stemming from changes in port-level landed revenue; landed revenue was assumed to be the product of catch and constant price per port. This coupling to IOPAC allowed outputs from the end-to-end model to be translated to direct effects (on the seafood sector), indirect effects (on suppliers to the seafood sector), and induced effects (related to broader household spending), rather than only reporting landed revenue as a modeling endpoint. The Atlantis ecosystem model scenarios tested effects of fishing gear shifts and spatial closures. Although the Atlantis model projection period was 20 years, the coupling to the input-output model was made only for years 1 and 15, largely due to the caveats described above related to the static nature of input-output models and their lack of behavioral responses.
The coupled approach of Kaplan and Leonard (2012) has been replicated with other end-to-end models, and gained traction with fishery management audiences, but the approach is not “operational,” i.e., it is not routinely delivered as a management product. Fay et al. (2019) recently applied a similar coupled approach in the Northeast United States, coupling an Atlantis model to the NERIOCOM input-output model (Steinback and Thunberg, 2006). The Atlantis-IOPAC coupling of models has been presented to the Pacific Fishery Management Council, council subcommittees, and review panels (Kaplan and Marshall, 2016). A recent application by Hodgson et al. (2018) considered port-level effects of ocean acidification on revenue, income, and employment. IOPAC is routinely updated for use by the Pacific Fishery Management Council, so further coupling is possible and is likely a constructive way to frame ecosystem modeling results, in particular because input-output models are commonly used throughout the United States by policy makers (including outside fisheries).
Management Uptake
A review of key factors which facilitated management uptake, as detailed in Supplementary Appendix Table 1, indicates some commonalities across models successfully used in management support. Most obvious is when stakeholders and managers request answers to specific questions that necessitate the development of a coupled model, regardless of the types of models or coupling employed. Somewhat less apparent and equally necessary is the need to have models which function within management timelines and are able to test management-relevant policy instruments. For tactical advice, this reality usually translates into a need to develop relatively lightweight models which can iterate combinations of policies quickly to inform the development of management actions. For strategic advice, models need to realistically capture the dynamics of resources most closely associated with human actions under management, such that stakeholders and managers glimpse their perception of the system in model outputs, which can build trust in its function. Providing either tactical or strategic advice in this manner necessitates coupled SES models, in that it is the interplay of biology and human behavior which determines the success or failure of policy instruments. Timing plays a key role in management uptake, and good models are often left unused because they may not be completely developed in time for management actions.
In light of this, building models based on recurring demands helps to ensure the often long lead time necessary to develop models does not unduly interfere with their adoption. Many times uptake is as much a fortuitous confluence of events as careful planning. As such, having a developed model which can answer a scientifically interesting and seemingly policy-relevant question ready when it becomes important from a manager’s perspective can also bear fruit. Retrospective analyses of prior management or environmental shocks also provide valuable insights into probable human responses to future management alternatives and environmental shocks such as climate change.
Implications for General Modeling Support
One of the advantages of recognizing the need for coupled modeling is that generic modeling support activities can be applied to all disciplines. For example, the extensive use of a variety of models for stock assessments has led to NOAA support for the development of a modeling toolbox infrastructure, which includes the NOAA Fisheries Integrated Toolbox and current supports a number of the SES models described in this manuscript. This integrated toolbox infrastructure is being designed to support stock assessment, ecosystem, economic and human dimension models. Sharing a basic requirement for provision of model metadata, version control, model sharing, and other aspects will facilitate model coupling. Similarly, the need and investment for access to high-speed computing for coupled earth-system models will apply to coupled SES models.
Conclusion
In this manuscript we have provided an overview of a framework to describe coupled SES fishery models, including the trade-offs between approaches and management questions which they can address. We reviewed management uptake of these SES models to identify commonalities across case studies in terms of both successes and failures. The ultimate purpose of this manuscript is to provide interdisciplinary scientists and resource managers with guidance on how, when, and what to couple in order to provide actionable information for a suite of management-relevant questions. The main takeaway from this analysis is that timing plays a key role in management uptake and successful coupling. Early engagement between disciplines, and even across sub-disciplines, ensures the broadest range of questions can be addressed within a management timeline.
This manuscript focused on fishery management-centric SES models, but the framework is applicable within a broader EBM construct and the ideas outlined in this paper resonate into multi-sectoral modeling approaches, particularly the need to integrate early not only within a single sector (such as through EBFM) but also across sectors. Further, all the modeling approaches outlined here can inform decision-makers whenever fisheries and other ocean uses come into conflict. When shifting from a focus on fisheries toward broader interactions between fisheries, wind energy development, tourism, and other ocean uses, coupled SES models will continue to play an important role in understanding the breadth of trade-offs entailed. As always, the management questions and industry sectors under consideration will dictate the relative value of modeling approaches and metrics which can help inform resource managers.
Data Availability Statement
The original contributions presented in the study are included in the article/Supplementary Material, further inquiries can be directed to the corresponding author.
Author Contributions
SK, GD, and AH organized the original conception and design of the study. SK, GD, AH, SB, LC, AF, MJ, MK, KL, DL, MM, CS, HT, and MW participated in the NEMoW Workshop, contributed to the list of coupled SES models in Supplementary Appendix Table 1, which ultimately resulted in structure and organization of the manuscript, and wrote sections of the manuscript. SK wrote the first draft of the manuscript. All authors contributed to manuscript revision, read, and approved the submitted version.
Funding
This research was supported by NOAA Fisheries.
Conflict of Interest
The authors declare that the research was conducted in the absence of any commercial or financial relationships that could be construed as a potential conflict of interest.
Acknowledgments
We would like the thank all of the participants in the 5th National Ecosystem Modeling Workshop (NEMoW) in St. Petersburg, FL, United States December 9–11th, 2019 for their assistance in the discussion which helped shape this manuscript as well as Isaac Kaplan for contributing to earlier drafts of the manuscript. We would also like to thank the NOAA Fisheries Office of Science and Technology and the NOAA Fisheries Regional Science Centers for providing financial support for the workshop and the University of South Florida, College of Marine Science, especially Cameron Ainsworth, for hosting the workshop.
Supplementary Material
The Supplementary Material for this article can be found online at: https://www.frontiersin.org/articles/10.3389/fmars.2021.631400/full#supplementary-material
Footnotes
- ^ There is not a one- to-one correspondence between LME and Fishery Management Council jurisdiction. For example, the North Pacific Fishery Management Council straddles four LMEs; Aleutian Islands, Eastern Bering Sea, Gulf of Alaska, and the Arctic LME which includes both the Beaufort and Chukchi Seas.
- ^ One approach would be to provide small awards to research teams early in the project timeline to facilitate discussions of how best to integrate the research questions and study outputs to address management-specific questions prior to submitting a larger proposal to a(nother) funding agency.
References
Abbott, J. K., and Haynie, A. C. (2012). What are we protecting? fisher behavior and the unintended consequences of spatial closures as a fishery management tool. Ecol. Appl. 22, 762–777. doi: 10.1890/11-1319.1
Abbott, J. K., Haynie, A. C., and Reimer, M. N. (2015). Hidden flexibility: institutions. incentives, and the margins of selectivity in fishing. Land Econ. 91, 169–195. doi: 10.3368/le.91.1.169
Aminpour, P., Gray, S. A., Jetter, A. J., Introne, J. E., Singer, A., and Arlinghaus, R. (2020). Wisdom of stakeholder crowds in complex social–ecological systems. Nat. Sustainabil. 3, 1–9. doi: 10.1038/s41893-019-0467-z
Anderies, J. M., Janssen, M. A., and Ostrom, E. (2004). A framework to analyze the robustness of social-ecological systems from an institutional perspective. Ecol. Soc. 9:18. doi: 10.5751/ES-00610-090118
Audzijonyte, A., Pethybridge, H., Porobic, J., Gorton, R., Kaplan, I., and Fulton, E. A. (2019). Atlantis: a spatially explicit end-to-end marine ecosystem model with dynamically integrated physics, ecology and socio-economic modules. Methods Ecol. Evol. 10, 1814–1819. doi: 10.1111/2041-210X.13272
Berkes, F., and Folke, C. (1998). Linking Social and Ecological Systems: Management Practices and Social Mechanisms for Building Resilience. New York, NY: Cambridge University Press.
Bockstael, N. E., and Opaluch, J. J. (1983). Discrete modelling of supply response under uncertainty: the case of the fishery. J. Environ. Econ. Manag. 10, 125–137. doi: 10.1016/0095-0696(83)90021-9
Branch, T. A., Hilborn, R., Haynie, A. C., Fay, G., Flynn, L., Griffiths, J., et al. (2006). Fleet dynamics and fishermen behavior: lessons for fisheries managers. Can. J. Fish. Aquatic Sci. 63, 1647–1668. doi: 10.1139/f06-072
Brinson, A. A., and Thunberg, E. (2016). 2016. performance of federally managed catch share fisheries in the United States. Fish. Res. 179, 213–223. doi: 10.1016/j.fishres.2016.03.008
Brinson, A. A., and Thunberg, E. M. (2013). The Economic Performance of US Catch Share Programs. Washington, D.C: NOAA Technical Memorandum.
Chen, Y. A. (2018). Three Essays in Fisheries Economics. http://hdl.handle.net/1773/41765 (accessed December 10, 2019).
Cheng, S. H., McKinnon, M. C., Masuda, Y. J., Garside, R., Jones, K. W., Miller, D. C., et al. (2020). Strengthen causal models for better conservation outcomes for human well-being. PLoS One 15:e0230495. doi: 10.1371/journal.pone.0230495
Colburn, L. L., Jepson, M., Weng, C., Seara, T., Weiss, J., and Hare, J. A. (2016). Indicators of climate change and social vulnerability in fishing dependent communities along the eastern and gulf coasts of the United States. Mar. Pol. 74, 323–333. doi: 10.1016/j.marpol.2016.04.030
Colding, J., and Barthel, S. (2019). Exploring the social-ecological systems discourse 20 years later. Ecol. Soc. 24:2. doi: 10.5751/ES-10598-240102
Cutter, S. L. (2003). The vulnerability of science and the science of vulnerability. Ann. Assoc. Am. Geograph. 93, 1–12. doi: 10.1111/1467-8306.93101
Cutter, S. L. (2009). “Social science perspectives on hazards and vulnerability science,” in Geophysical Hazards., 17–30, ed. T. Beer (Dordrecht: Springer). doi: 10.1007/978-90-481-3236-2_2
Cutter, S. L., and Finch, C. (2008). Temporal and spatial changes in social vulnerability to natural hazards. Proc. Natl. Acad. Sci. U S A. 105, 2301–2306. doi: 10.1073/pnas.0710375105
DePiper, G. S., Lipton, D. W., and Lipcius, R. N. (2017). Valuing ecosystem services: oysters, denitrification, and nutrient trading programs. Mar. Resource Econ. 32, 1–20. doi: 10.1086/688976
Düspohl, M., Frank, S., and Döll, P. (2012). A review of bayesian networks as a participatory modeling approach in support of sustainable environmental management. Int. J. Sustainable Dev. 5, 1–18. doi: 10.5539/jsd.v5n12p1
Eales, J., and Wilen, J. E. (1986). An examination of fishing location choice in the pink shrimp fishery. Mar. Resource Econ. 2, 331–351. doi: 10.1086/mre.2.4.42628909
Fay, G., DePiper, G., Steinback, S., Gamble, R. J., and Link, J. S. (2019). Economic and ecosystem effects of fishing on the northeast US Shelf. Front. Mar. Sci. 6:133. doi: 10.3389/fmars.2019.00133
Fleming, C. S., Dillard, M. K., Regan, S. D., Gorstein, M., Messick, E., and Blair, A. (2017). A Coastal Community Vulnerability Assessment for the Choptank Habitat Focus Area. Washington, D.C: NOAA Technical Memorandum.
Fulton, E. A., Link, J. S., Kaplan, I. C., Savina-Rolland, M., Johnson, P., Ainsworth, C., et al. (2011). Lessons in modelling and management of marine ecosystems: the atlantis experience. Fish Fish. 12, 171–188. doi: 10.1111/j.1467-2979.2011.00412.x
Fulton, E. A., Parslow, J. S., Smith, A. D. M., and Johnson, C. R. (2004). Biogeochemical marine ecosystem models II: the effect of physiological detail on model performance. Ecol. Modell. 173, 371–406. doi: 10.1016/j.ecolmodel.2003.09.024
Fulton, E. A., Punt, A. E., Dichmont, C. M., Harvey, C. J., and Gorton, R. (2019). Ecosystems say good management pays off. Fish Fish. 20, 66–96. doi: 10.1111/faf.12324
Girardin, R., Hamon, K. G., Pinnegar, J., Poos, J. J., Thébaud, O., Tidd, A., et al. (2017). Thirty years of fleet dynamics modelling using discrete-choice models: what have we learned? Fish Fish. 18, 638–655. doi: 10.1111/faf.12194
Goethel, D. R., Lucey, S. M., Berger, A. M., Gaichas, S. K., Karp, M. A., Lynch, P. D., et al. (2019). Closing the feedback loop: on stakeholder participation in management strategy evaluation. Can. J. Fish. Aquatic Sci. 76, 1895–1913. doi: 10.1139/cjfas-2018-0162
Gordon, H. S. (1954). “The economic theory of a common-property resource: the fishery,” in Classic Papers in Natural Resource Economics., 178–203, ed. C. Gopalakrishnan (London: Palgrave Macmillan). doi: 10.1057/9780230523210_10
Hare, J. A., Morrison, W. E., Nelson, M. W., Stachura, M. M., Teeters, E. J., Griffis, R. B., et al. (2016). A vulnerability assessment of fish and invertebrates to climate change on the northeast US continental shelf. PLoS One 11:e0146756. doi: 10.1371/journal.pone.0146756
Harvey, C. J., Reum, J. C. P., Poe, M. R., Williams, G. D., and Kim, S. J. (2016). Using conceptual models and qualitative network models to advance integrative assessments of marine ecosystems. Coastal Manag. 44, 486–503. doi: 10.1080/08920753.2016.1208881
Haynie, A. (2015). “Utilizing fishset to model the economic impacts of fisheries management actions and environmental change,” in Proceedings of the 145th Annual Meeting of the American Fisheries Society, (New York, NY: AFS).
Haynie, A., and Layton, D. (2010). An expected profit model for monetizing fishing location choices. J. Environ. Econ. Manag. 59, 165–176. doi: 10.1016/j.jeem.2009.11.001
Hermann, A. J., Gibson, G. A., Cheng, W., Ortiz, I., Aydin, K., Wang, M., et al. (2019). Projected biophysical conditions of the bering sea to 2100 under multiple emission scenarios. ICES J. Mar. Sci. 76, 1280–1304. doi: 10.1093/icesjms/fsz111
Hodgson, E. E., Kaplan, I. C., Marshall, K. N., Leonard, J., Essington, T. E., Busch, D. S., et al. (2018). Consequences of spatially variable ocean acidification in the california current: lower PH drives strongest declines in benthic species in southern regions while greatest economic impacts occur in northern regions. Ecol. Modell. 383, 106–117. doi: 10.1016/j.ecolmodel.2018.05.018
Hollowed, A. B., Holsman, K. K., Haynie, A. C., Hermann, A. J., Punt, A. E., Aydin, K., et al. (2020). Integrated modeling to evaluate climate change impacts on coupled social-ecological systems in alaska. Front. Mar. Sci. 6:775. doi: 10.3389/fmars.2019.00775
Holsman, K. K., Haynie, A. C., Hollowed, A. B., Reum, J. C. P., Aydin, K., et al. (2020). Ecosystem-Based fisheries management forestalls climate-driven collapse. Nat. Commun. 11, 1–10. doi: 10.1038/s41467-020-18300-3
Hutniczak, B., Lipton, D., Wiedenmann, J., and Wilberg, M. (2019). Valuing changes in frequency of fish stock assessments. Can. J. Fish. Aquatic Sci. 76, 1640–1652. doi: 10.1139/cjfas-2018-0130
Ianelli, J. N., Hollowed, A. B., Haynie, A. C., Mueter, F. J., and Bond, N. A. (2011). Evaluating management strategies for eastern bering sea walleye pollock (Theragra Chalcogramma) in a changing environment. ICES J. Mar. Sci. 68, 1297–1304. doi: 10.1093/icesjms/fsr010
ICES (2021). Workshop of Fisheries Management Reference Points in a Changing Environment (WKRPChange, outputs from 2020 meeting). Available at http://doi.org/10.17895/ices.pub 7660 (accessed February 2, 2021).
Jepson, M., and Colburn, L. L. (2013). Development of Social Indicators of Fishing Community Vulnerability and Resilience in the US Southeast and Northeast Regions. Washington, D.C: NOAA Technical Memorandum.
Jin, D., Hoagland, P., Dalton, T. M., and Thunberg, E. M. (2012). Development of an integrated economic and ecological framework for ecosystem-based fisheries management in New England. Prog. Oceanography 102, 93–101. doi: 10.1016/j.pocean.2012.03.007
Kaplan, I. C., Horne, P. J., and Levin, P. S. (2012). Screening California current fishery management scenarios using the atlantis end-to-end ecosystem model. Prog. Oceanography 102, 5–18. doi: 10.1016/j.pocean.2012.03.009
Kaplan, I. C., and Leonard, J. (2012). From krill to convenience stores: forecasting the economic and ecological effects of fisheries management on the us west coast. Mar. Pol. 36, 947–954. doi: 10.1016/j.marpol.2012.02.005
Kaplan, I. C., and Marshall, K. N. (2016). A guinea Pig’s tale: learning to review end-to-end marine ecosystem models for management applications. ICES J. Mar. Sci. 73, 1715–1724. doi: 10.1093/icesjms/fsw047
Kasperski, S. (2015). Optimal multi-species harvesting in ecologically and economically interdependent fisheries. Environ. Resource Econom. 61, 517–557. doi: 10.1007/s10640-014-9805-9
Kirkley, J. E., Walden, J., and Färe, R. (2011) General equilibrium model for atlantic herring (Clupea harengus) with ecosystem considerations. ICES J. Mar. Sci. 68, 860–866. doi: 10.1093/icesjms/fsr023
Lee, M., Steinback, S., and Wallmo, K. (2017). Applying a bioeconomic model to recreational fisheries management: groundfish in the northeast United States. Mar. Resource Econom. 32, 191–216. doi: 10.1086/690676
Leonard, J. L., and Watson, P. S. (2011). Description of the Input-Output Model for Pacific Coast Fisheries. Washington, D.C: NOAA Technical Memorandum.
Leontief, W. W. (1951). The Structure of American Economy, 1919–1939: An Empirical Application of Equilibrium Analysis. New York, NY: Oxford University Press, 264.
Link, J. S. (2010). Ecosystem-Based Fisheries Management: Confronting Tradeoffs. Cambridge: Cambridge University Press. doi: 10.1017/CBO9780511667091
Little, L. R., Sakari, K., Punt, A. E., Pantus, F., Davies, C. R., and Mapstone, B. D. (2004). Information flow among fishing vessels modelled using a bayesian network. Environ. Modell. Software 19, 27–34. doi: 10.1016/S1364-8152(03)00100-2
Loveridge, S. (2004). A typology and assessment of multi-sector regional economic impact models. Reg. Stud. 38, 305–317. doi: 10.1080/003434042000211051
Marshall, K. N., Levin, P. S., Essington, T. E., Koehn, L. E., Anderson, L. G., Bundy, A., et al. (2018). Ecosystem-based fisheries management for social–ecological systems: renewing the focus in the United States with next generation fishery ecosystem plans. Conserv. Lett. 11:e12367. doi: 10.1111/conl.12367
Martin, S. B., Blake, S., Freitag, A., Dorfman, D., Regan, S., and Jepson, M. (2019). An Ecosystem Status Report to Support Management Decisions in Barataria Basin. Paris: CERF.
Masi, M. D., Ainsworth, C. H., Kaplan, I. C., and Schirripa, M. J. (2018). Interspecific interactions may influence reef fish management strategies in the Gulf of Mexico. Mar. Coastal Fish. 10, 24–39. doi: 10.1002/mcf2.10001
Messick, E., Dillard, M. K., Blair, A., Buck, K., Effron, M., Fleming, C. S., et al. (2016). Identifying Priorities for Adaptation Planning: An Integrated Vulnerability Assessment for the Town of Oxford and Talbot County, Maryland. Washington, D.C: NOAA Technical Memorandum.
Nielsen, J. R., Thunberg, E., Holland, D. S., Schmidt, J. O., Fulton, E. A., Bastardie, F., et al. (2018). Integrated ecological–economic fisheries models—evaluation, review and challenges for implementation. Fish Fish. 19, 1–29. doi: 10.1111/faf.12232
Pikitch, E. K., Santora, C., Babcock, E. A., Bakun, A., Bonfil, R., Conover, D. O., et al. (2004). Ecosystem-based fishery management. Science 305:346. doi: 10.1126/science.1098222
Plagányi, ÉE. (2007). Models for an Ecosystem Approach to Fisheries. Rome: FAO. FAO Fisheries Technical Paper.
Prellezo, R., Accadia, P., Andersen, J. L., Andersen, B. S., Buisman, E., Little, A., et al. (2012). A review of EU bio-economic models for fisheries: the value of a diversity of models. Mar. Pol. 36, 423–431. doi: 10.1016/j.marpol.2011.08.003
Punt, A. E., A’mar, T., Bond, N. A., Butterworth, D. S., de Moor, C. L., De Oliveira, J. A. A., et al. (2014a). Fisheries management under climate and environmental uncertainty: control rules and performance simulation. ICES J. Mar. Sci. 71, 2208–2220. doi: 10.1093/icesjms/fst057
Punt, A. E., Poljak, D., Dalton, M. G., and Foy, R. J. (2014b). Evaluating the impact of ocean acidification on fishery yields and profits: the example of red king crab in Bristol Bay. Ecol. Modell. 285, 39–53. doi: 10.1016/j.ecolmodel.2014.04.017
Punt, A. E., Foy, R. J., Dalton, M. G., Long, W. C., and Swiney, K. M. (2016). Effects of long-term exposure to ocean acidification conditions on future southern tanner crab (Chionoecetes bairdi) fisheries management. ICES J. Mar. Sci. 73, 849–864. doi: 10.1093/icesjms/fsv205
Reimer, M., Abbott, J., and Haynie, A. (2017). Empirical models of fisheries production: conflating technology with incentives? Mar. Resource Econ. 32, 169–190. doi: 10.1086/690677
Reimer, M. N., and Haynie, A. C. (2018). Mechanisms matter for evaluating the economic impacts of marine reserves. J. Environ. Econ. Manag. 88, 427–446. doi: 10.1016/j.jeem.2018.01.009
Reum, C. P. J., Blanchard, J. L., Holsman, K. K., Aydin, K., Hollowed, A. B., Hermann, A. J., et al. (2020). Ensemble projections of future climate change impacts on the eastern bering sea food web using a multispecies size spectrum model. Front. Mar. Sci. 7:124. doi: 10.3389/fmars.2020.00124
Rose, K. A., Allen, J. I., Artioli, Y., Barange, M., Blackford, J., Carlotti, F., et al. (2010). End-to-End models for the analysis of marine ecosystems: challenges. issues, and next steps. Mar. Coastal Fish. 2, 115–130. doi: 10.1577/C09-059.1
Rosellon-Druker, J., Szymkowiak, M., Cunningham, C. J., Kasperski, S., Kruse, G. H., Moss, J. H., et al. (2019). Development of social-ecological conceptual models as the basis for an integrated ecosystem assessment framework in Southeast Alaska. Ecol. Soc. 24:30. doi: 10.5751/ES-11074-240330
Ruzicka, J. J., Kasperski, S., Zador, S., and Himes-Cornell, A. (2019). Comparing the roles of pacific halibut and arrowtooth flounder within the gulf of alaska ecosystem and fishing economy. Fish. Oceanography 28, 576–596. doi: 10.1111/fog.12431
Sanchirico, J. N., Lew, D. K., Haynie, A. C., Kling, D. M., and Layton, D. F. (2013). Conservation Values in marine ecosystem-based management. Mar. Pol. 38, 523–530. doi: 10.1016/j.marpol.2012.08.008
Schaeffer, M. B. (1957). A study of the dynamics of the fishery for yellowfin tuna in the eastern tropical Pacific Ocean. Inter-Am. Trop. Tuna Comm. Bull. 2, 247–268.
Schluter, M., Mcallister, R. R. J., Arlinghaus, R., Bunnefeld, N., Eisenack, K., et al. (2012). New horizons for managing the environment: a review of coupled social-ecological systems modeling. Nat. Resource Model. 25, 219–272. doi: 10.1111/j.1939-7445.2011.00108.x
Seung, C., and Ianelli, J. (2016). Regional economic impacts of climate change: a computable general equilibrium analysis for an alaska fishery. Nat. Resource Model. 29, 289–333. doi: 10.1111/nrm.12092
Seung, C. K. (2015). Untangling economic impacts for alaska fisheries: a structural path analysis. Mar. Resource Econ. 30, 331–347. doi: 10.1086/680444
Seung, C. K., Dalton, M. G., Punt, A. E., Poljak, D., and Foy, R. (2015). Economic impacts of changes in an alaska crab fishery from ocean acidification. Climate Change Econom. 6:1550017. doi: 10.1142/S2010007815500177
Seung, C. K., and Ianelli, J. N. (2019). Evaluating alternative policies for managing an alaska pollock fishery with climate change. Ocean Coastal Manag. 178:104837. doi: 10.1016/j.ocecoaman.2019.104837
Seung, C. K., and Waters, E. C. (2006). A review of regional economic models for fisheries management in the US. Mar. Resource Econ. 21, 101–124. doi: 10.1086/mre.21.1.42629497
Smith, M. D., and Wilen, J. E. (2003). economic impacts of marine reserves: the importance of spatial behavior. J. Environ. Econ. Manag. 46, 183–206. doi: 10.1016/S0095-0696(03)00024-X
Smith, V. L. (1969). On models of commercial fishing. J. Political Econ. 77, 181–198. doi: 10.1086/259507
Steinback, S. R., and Thunberg, E. M. (2006). Northeast Region Commercial Fishing Input-Output Model. Washington, D.C: NOAA Technical Memorandum.
Stojanovic, T., McNae, H., Tett, P., Potts, T., Reis, J., Smith, H., et al. (2016). The social aspect of social-ecological systems: a critique of analytical frameworks and findings from a multisite study of coastal sustainability. Ecol. Soc. 21:15. doi: 10.5751/ES-08633-210315
Szymkowiak, M., and Kasperski, S. (2021). Sustaining an alaska coastal community: integrating place based well-being indicators and fisheries participation. Coastal Manag. 49, 107–131. doi: 10.1080/08920753.2021.1846165
Tam, J. C., Fay, G., and Link, J. S. (2019). Better together: the uses of ecological and socio-economic indicators with end-to-end models in marine ecosystem based management. Front. Mar. Sci. 6:560. doi: 10.3389/fmars.2019.00560
Townsend, H., Aydin, K., Brodie, S., DePiper, G., deReynier, Y., Harvey, C., et al. (2020). “Report of the 5th National Ecosystem Modeling Workshop (NEMoW 5),” in Progress in Ecosystem Modeling For Living Marine Resource Management, eds P. Lynch, K. Osgood, and J. Link (Silver Spring: National Marine Fisheries Service).
Watson, J. T., and Haynie, A. C. (2018). Paths to resilience: the walleye pollock fleet uses multiple fishing strategies to buffer against environmental change in the Bering Sea. Can. J. Fish. Aquatic Sci. 75, 1977–1989. doi: 10.1139/cjfas-2017-0315
Weijerman, M., Grace-McCaskey, C., Grafeld, S. L., Kotowicz, D. M., Oleson, K. L. L., et al. (2016). Towards an ecosystem-based approach of guam’s coral reefs: the human dimension. Mar. Pol. 63, 8–17. doi: 10.1016/j.marpol.2015.09.028
Keywords: social-ecological systems, EBFM, ecosystem based fisheries management, coupled natural human systems, end-to-end, management strategy evaluation, conceptual models
Citation: Kasperski S, DePiper GS, Haynie AC, Blake S, Colburn LL, Freitag A, Jepson M, Karnauskas M, Leong KM, Lipton D, Masi M, Speir C, Townsend H and Weijerman M (2021) Assessing the State of Coupled Social-Ecological Modeling in Support of Ecosystem Based Fisheries Management in the United States. Front. Mar. Sci. 8:631400. doi: 10.3389/fmars.2021.631400
Received: 20 November 2020; Accepted: 11 February 2021;
Published: 24 March 2021.
Edited by:
Tomaso Fortibuoni, Higher Institute for Environmental Protection and Research (ISPRA), ItalyReviewed by:
Éva Plagányi, Commonwealth Scientific and Industrial Research Organisation (CSIRO), AustraliaJoshua P. Kilborn, University of South Florida, United States
Copyright © 2021 Kasperski, DePiper, Haynie, Blake, Colburn, Freitag, Jepson, Karnauskas, Leong, Lipton, Masi, Speir, Townsend and Weijerman. This is an open-access article distributed under the terms of the Creative Commons Attribution License (CC BY). The use, distribution or reproduction in other forums is permitted, provided the original author(s) and the copyright owner(s) are credited and that the original publication in this journal is cited, in accordance with accepted academic practice. No use, distribution or reproduction is permitted which does not comply with these terms.
*Correspondence: Stephen Kasperski, Stephen.Kasperski@noaa.gov