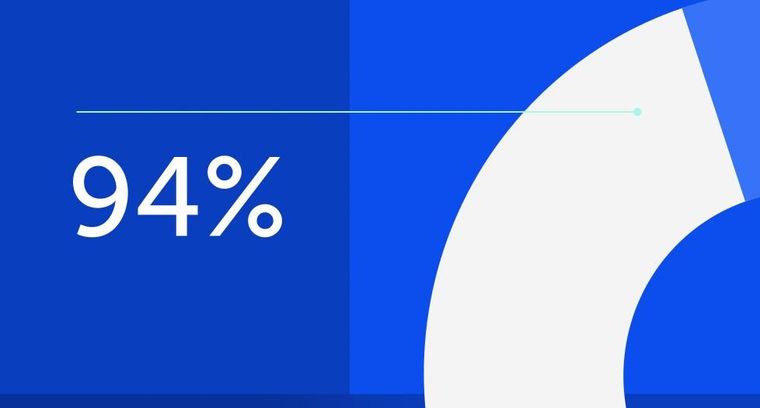
94% of researchers rate our articles as excellent or good
Learn more about the work of our research integrity team to safeguard the quality of each article we publish.
Find out more
ORIGINAL RESEARCH article
Front. Mar. Sci., 30 June 2021
Sec. Marine Ecosystem Ecology
Volume 8 - 2021 | https://doi.org/10.3389/fmars.2021.624161
This article is part of the Research TopicUsing Ecological Models to Support and Shape Environmental Policy DecisionsView all 28 articles
One of the significant challenges to using information and ideas generated through ecosystem models and analyses for ecosystem-based fisheries management is the disconnect between modeling and management needs. Here we present a case study from the U.S. West Coast, the stakeholder review of NOAA’s annual ecosystem status report for the California Current Ecosystem established by the Pacific Fisheries Management Council’s Fisheries Ecosystem Plan, showcasing a process to identify management priorities that require information from ecosystem models and analyses. We then assess potential ecosystem models and analyses that could help address the identified policy concerns. We screened stakeholder comments and found 17 comments highlighting the need for ecosystem-level synthesis. Policy needs for ecosystem science included: (1) assessment of how the environment affects productivity of target species to improve forecasts of biomass and reference points required for setting harvest limits, (2) assessment of shifts in the spatial distribution of target stocks and protected species to anticipate changes in availability and the potential for interactions between target and protected species, (3) identification of trophic interactions to better assess tradeoffs in the management of forage species between the diet needs of dependent predators, the resilience of fishing communities, and maintenance of the forage species themselves, and (4) synthesis of how the environment affects efficiency and profitability in fishing communities, either directly via extreme events (e.g., storms) or indirectly via climate-driven changes in target species availability. We conclude by exemplifying an existing management process established on the U.S. West Coast that could be used to enable the structured, iterative, and interactive communication between managers, stakeholders, and modelers that is key to refining existing ecosystem models and analyses for management use.
Fish stocks do not live isolated from, but exist as part of an ecosystem, and their dynamics are intrinsically related to those of their habitat, prey, and predators, from environmental conditions to humans. In recognition of the need to assess the cumulative of effects and trade-offs of fisheries management actions considering these ecological interactions there has been a longstanding worldwide push for ecosystem-based fisheries management (EBFM, May et al., 1979; Pikitch et al., 2004; Link, 2010; Fogarty, 2014; Holsman et al., 2017; Skern-Mauritzen et al., 2018; Fulton, 2021). In the United States, scientists have been exploring and coordinating the use of ecosystem models to address ocean ecosystem science and management questions for over a decade (Townsend et al., 2008, 2014, 2017; Link et al., 2010). The National Oceanic and Atmospheric Administration (NOAA), the U.S. federal agency responsible for marine ecosystem science and ecosystem-based fisheries management in Federal waters, has prioritized ecosystem modeling as necessary to better assess the trade-offs we make to maintain resilient and productive ecosystems, and to respond to climate, habitat, and ecological change (National Marine Fisheries Service, 2016a,b). Nevertheless, progress, in the United States and elsewhere, in using ecosystem models and analysis to guide fishery decision-making has been slow (Skern-Mauritzen et al., 2016; Townsend et al., 2019).
One of the significant challenges to using information and ideas generated through ecosystem modeling is a lack of connection between modeling and management priorities (Link et al., 2012). Ecosystem modelers are not necessarily asking the same questions of their models as those asked by legal mandates or by managers implementing those mandates. This disconnect between scientific interest and management needs may contribute to the perceived slow pace in the uptake and implementation of ecosystem-based management (Hilborn, 2011; Cowan et al., 2012; Marshall et al., 2018). Townsend et al. (2019) suggests that scientists can better understand and tune models to address management priorities by working more closely with managers, within existing processes to implement legal mandates.
Indeed, establishment of an effective scientists-decision makers knowledge exchange has been recognized as a major challenge to successful science-based management of complex socio-ecological systems (Cvitanovic et al., 2015). Frameworks for facilitating the uptake of scientific research in natural resources management, such as the System Approach Framework (SAF, Hopkins et al., 2011), structured decision making approaches (Gregory et al., 2012), and integrative assessments (see review by Mach and Field, 2017) stress that ongoing two-way exchange of information between scientists and decision makers and participatory communication methods are key to facilitate uptake of scientific analysis for management of complex systems (Lidström and Johnson, 2020). Use of scientific knowledge in support of decision-making is dependent on such knowledge being perceived as salient to the decision-makers (Cvitanovic et al., 2015; Mach and Field, 2017). Iterative dialogue between scientists, managers and stakeholders can ensure scientific analysis and models are relevant to the decision-making process (Hopkins et al., 2011; Cvitanovic et al., 2015; Mach and Field, 2017).
There are also technical issues that can limit use of ecosystem models in decision making. These have been widely discussed elsewhere (Skern-Mauritzen et al., 2016, 2018; Holsman et al., 2017; Schuwirth et al., 2019), but we synthesize them here. There needs to be sufficient data to develop a basic mechanistic understanding of the system (Skern-Mauritzen et al., 2016; Schuwirth et al., 2019) and few research programs exist to empirically quantify processes at this level of complexity (Wells et al., 2020). Such data requirements become more difficult to meet with increasing complexity of the approach being considered (Holsman et al., 2017; Skern-Mauritzen et al., 2018), which in turn comes at the cost of greater estimation uncertainty (Link et al., 2011). This uncertainty needs to be quantifiable and factored into management decisions (Holsman et al., 2017; Skern-Mauritzen et al., 2018; Schuwirth et al., 2019). For tactical management applications, predictive performance of ecosystem models also needs to be sufficient for the model to be useful (Skern-Mauritzen et al., 2018; Schuwirth et al., 2019). Thus, increases in estimation uncertainty need to be balanced by reductions in process uncertainty to maintain adequate predictive performance (Link et al., 2011). Model output also needs to be at an appropriate temporal and spatial resolution to inform management (Schuwirth et al., 2019). These issues, however, should not prevent the use of ecosystem-based approaches to improve the status quo and meet the needs of decision-makers for scientific information that considers feedback and interactions between multiple ecosystem components (Patrick and Link, 2015; Skern-Mauritzen et al., 2018). The most appropriate ecosystem model will necessarily vary in complexity depending on the policy issue and data availability, and guidelines exist to inform choice of analytical tool (e.g., Weijerman et al., 2015; Holsman et al., 2017).
In this paper, we demonstrate a practical process, based within the framework of national laws and on the practices identified by Townsend et al. (2019), to better connect ecosystem models and analyses with fisheries management (Figure 1). We define ecosystem models and analyses as a broad suite of analytical tools which incorporate interactions between physical, biological, and/or human components of the ecosystem, ranging from empirical approaches to end-to-end ecosystem models. Fisheries in the exclusive economic zone off the U.S. West Coast are managed under the advice of the Pacific Fishery Management Council (PFMC). The PFMC established a regular process through which new ecosystem initiatives are co-developed to address ideas and issues that affect multiple species and fisheries (Pacific Fishery Management Council (PFMC), 2013). In doing so, this process provided an avenue for managers, stakeholders, and scientists to work together to find solutions to policy issues, the type of forum identified as necessary by Townsend et al. (2019). Here, we use the second PFMC ecosystem initiative, the PFMC’s stakeholder review of ecosystem status indicators, to identify emerging fisheries policy issues in the U.S. West Coast that require ecosystem information. This process echoes the Issue Identification step in the SAF framework, in which a policy issue is identified in collaboration with stakeholders so that the analytical tool can be developed for the specific decision context defined with stakeholders (Dinesen et al., 2019). We then connect the management questions to existing ecosystem models by specifying how their output could address some of the concerns raised by stakeholders and decision-makers about future trade-offs expected for living marine resource management in the California Current Ecosystem (CCE).
Figure 1. Overview of the process to facilitate integration of ecosystem models and analyses into fisheries management proposed by Townsend et al. (2019) on left and adaptation of that process to address multiple issues as presented in this paper on right.
California Current ESRs are developed annually by the NOAA California Current Integrated Ecosystem Assessment (CCIEA) team. These reports focus on biophysical, economic and social indicators related to attributes such as abundance and population condition of key species, community composition and energy/material flows, extent and condition of habitat, and fisheries engagement and social vulnerability in coastal communities. In 2017, the PFMC formalized a process for technical review of individual indicators and analyses (Box 1) so that new topics for in-depth technical assessment are identified annually in March and then reviewed in detail in September (Pacific Fishery Management Council (PFMC), 2017b; Box 1).
BOX 1. Glossary of terms and acronyms related to the United States West Coast approach to Ecosystem Based Fisheries Management.
Pacific Fishery Management Council (PFMC, or Council) – Management entity established under the Magnuson-Stevens Fishery Conservation and Management Act (MSA) responsible for advising the federal government on managing fisheries within the exclusive economic zone (EEZ) off the United States West Coast. Develops fishery management plans (FMPs) and fishery regulations to implement the FMPs. Advised by stakeholders (U.S. states and tribes, commercial and recreational fisheries participants, environmental and other non-governmental organizations, and the public) through Advisory Subpanels (https://www.pcouncil.org/documents/2019/09/cop-2.pdf/), assisted with monitoring and analyses by Technical/Management Teams (https://www.pcouncil.org/documents/2019/09/cop-3.pdf/) and Workgroups (https://www.pcouncil.org/documents/2019/09/cop-8.pdf/) (including the Ecosystem Workgroup, EWG), and provided scientific advice by the Scientific and Statistical Committee (SSC) (https://www.pcouncil.org/documents/2019/09/cop-4.pdf/.
Fishery Ecosystem Plan (FEP) (https://www.pcouncil.org/documents/2013/07/fep_final.pdf/) – PFMC’s formalized approach to Ecosystem Based Fisheries Management (EBFM). Includes a process through which the PFMC takes up ecosystem initiatives to address ideas and issues that affect multiple species and fisheries.
California Current Ecosystem Status Report (ESR) (E.g., https://www.pcouncil.org/documents/2020/02/g-1-a-iea-team-report-1.pdf/) – Annual report to the PFMC providing an ecosystem overview outside of focal resource stocks and populations, considering how outside factors influence focal resources, identifying linkages between different ecosystem components. Prepared by the NOAA California Current Integrated Ecosystem Assessment (CCIEA) (https://www. integratedecosystemassessment.noaa.gov/regions/california-current-region/index.html).
Stakeholder review – Review of policy, regulatory, or scientific product by stakeholders and members of the public. Process followed under the second ecosystem initiative (https://www.pcouncil.org/actions/initiative-2-coordinated-ecosystem-indicator-review/) to review the reliability and utility of existing ESR indicators, and identify desirable additions. Involved Council, its advisory bodies, the SSC, and public comment. Technical review – Review of scientific or analytic product by the SSC or its subcommittees. For the ESR, technical review involves an annual process of topic selection by the Council in conjunction with its advisory bodies and the CCIEA, followed by reviews by the SSC’s Ecosystem Based Management Subcommittee.
Methodology review – In-depth technical reviews of methods that are held periodically and as needed. Reviewers include members of the SSC and often outside experts, and reviews follow specific Terms of Reference (TOR) (E.g., https://www.pcouncil.org/documents/2018/06/terms-of-reference-for-the-methodology-review-process-for-groundfish-and-coastal-pelagic-species-for-2019-2020-june-2018.pdf/) that may also reflect established Council Operating Procedures (COP) (https://www.pcouncil.org/documents/2019/09/cop-15.pdf/). Required for changes to assessment methods or forecasts, and used for other complex topics as warranted.
Management Strategy Evaluation (MSE) – A process and modeling framework used to assess performance of management strategies given uncertainty relative to a set of predefined management objectives.
While technical reviews of the statistical analyses and models are useful, they do not provide incentives for broad stakeholder and manager participation in ESR development and refinement. In 2015, the PFMC addressed this shortcoming by proposing a new ecosystem initiative, the “Coordinated Ecosystem Indicator Review” (Figure 2). This initiative outlined a stakeholder review process (Box 1) to address four questions (Pacific Fishery Management Council (PFMC), 2017a): (1) What can the PFMC reasonably expect to learn from, or monitor with, the existing indicators in the ESR? (2) How well do the existing indicators accomplish their intent, and are any redundant? (3) Are there alternate indicators, information, or analyses that may perform better in context? and (4) Are there additional ecosystem indicators that could help inform PFMC decision-making?
Figure 2. Overview of opportunities for stakeholder feedback (orange boxes) on ESR indicators and ecosystem models and analyses in the PFMC management process.
In early 2016, the PFMC hosted a series of webinars to present the ESR indicators and discuss the four questions detailed above. Webinars were open to the public and were widely advertised by the PFMC in advance during their meetings, on their website, through their ∼1500 address email list, and through notice in participating government publications. The PFMC compiled all comments and recommendations raised during the discussion portions of the webinars. From March to September 2016, the PFMC also directly solicited feedback on the initiative’s four focal questions from its scientific and technical advisors and stakeholders. Between the live webinars and the solicitation to review the recordings of the webinars, the PFMC received 88 comments and recommendations from stakeholders and the public.
In this paper, we consider how the ideas generated in the initiative’s public review process might be used in ecosystem modeling planning. From the 88 comments and recommendations (Pacific Fishery Management Council (PFMC), 2016a,b) we selected only those that emphasize the need for ecosystem-level understanding, which acknowledges that ESRs should include not only status and trends of different indicators, but also a synthesis of how indicators interact and affect one another. Comments were characterized as belonging to the ecosystem-level understanding theme if they related to interactions between ecosystem components. The interactions considered were (1) interactions between species, (2) interactions between fishers and species, (3) impacts of abiotic components on species, and (4) impacts of abiotic components on fisheries. The authors found 17 comments that matched these criteria and thus were salient to the ecosystem-level understanding theme and could be addressed through greater inclusion of ecosystem model outputs in the ESR or in other reports to or conversations with the PFMC. These are reported in Table 1. Per Townsend et al. (2019), for each comment, we identified the relevant policy issue, management objectives, and the existing management process that would be used to address the problem (Table 1). In the Results and Discussion section, we describe in more detail the ecosystem-information needs highlighted in the 17 comments and assess which EBFM modeling activities could contribute to resolving the management concerns. We connect the policy issues highlighted in the stakeholder comments to specific models and analyses in Table 2. In Table 2 we present existing modeling products, but also highlight the additional modeling needs required to improve management utility. Here, EBFM modeling activities are defined broadly as those models and analyses used to assess interactions between physical, biological, and/or human components of the ecosystem. These tools include a variety of empirical approaches, species distribution models, biophysical models, climate-informed population dynamic models, multispecies models, food web models, and end-to-end ecosystem models.
Table 1. Comments from the stakeholder review of ESR Indicators that could be informed by ecosystem models and analyses with relevant policy issues, management objectives, and existing management processes to address them.
Table 2. Overview of existing or potential modeling products that could be developed to address the specified comments from managers and stakeholders.
The first set of ecosystem-level understanding comments (Table 1, Comments 1–8) highlighted the need for improved scientific advice on how climate, physical oceanography and biogeochemistry indicators are related to biological productivity (i.e., recruitment, mortality, or growth of target and protected species). Comments 2 to 5 emphasized the requirement for improved quantification of how oceanographic processes, and in particular upwelling, affect species of management concern, such as salmon or groundfish. Comment 6 suggested, given the cumulative and potentially synergistic impacts of a variety of climate drivers on a species’ productivity, a need for a more in-depth synthesis of how environmental conditions interact to affect biological components. Comments 7 and 8 stress the need to also assess the utility of seabirds as indicators of forage or salmon productivity. Ultimately, as reflected in Comment 1, stakeholders are interested in anticipating the risk of an undesirable outcome and minimizing its impact, and thus need relevant indicators for forecasting and risk assessment.
This topic was associated with the highest number of comments (Table 1), perhaps because productivity indicators can inform the setting of species-specific harvest levels, one of the main management measures used by PMFC. Harvest levels are often dependent on a forecast of stock biomass and on reference points (fishing intensity or biomass thresholds that should not be crossed or targets to be achieved) derived from stock assessments. Use of climate-linked natural mortality in stock assessment can generate less variable reference points on which to base catch advice (O’Leary et al., 2019). If predictive skill is sufficient, the integration of environmental indicators of recruitment can also improve estimates of reference points (Basson, 1999). In addition, short-term recruitment forecasts can enable managers to alert fishing communities of potential changes in harvest levels, allowing for development of potential remediation strategies (Tommasi et al., 2017b). However, the added benefit of including environmental indicators into the estimation of stock-status depends on the species’ life-history type (Haltuch et al., 2019b). Environmentally informed short-term recruitment forecasts are particularly important for semelparous species like salmon, as there is no direct carryover of spawning biomass across years, or for forage species whose fishable biomass consists in large part of young age classes (Tommasi et al., 2017c). Catch advice for long-lived stocks may instead be more responsive to changes in natural mortality (Bax, 1998).
Usefulness of stock productivity indicators to management decisions is also dependent on their availability relative to the timing of council decision making. Some environmentally based forecasts of salmon returns are dependent on ocean conditions during first ocean entry 2 or 3 years prior (e.g., Rupp et al., 2012; Burke et al., 2013), and thus rely on past, observed environmental covariates. This has facilitated their inclusion in some management-relevant salmon forecasts (Burke et al., 2013; Litz and Hughes, 2020). However, for other species and salmon stocks (e.g., Satterthwaite et al., 2020) key indicators may need to be forecasted months to a year in advance to improve catch advice or even longer to inform stock status projections (e.g., for groundfish). Thanks to recent advancements in global climate prediction systems, forecasts of biologically relevant variables in coastal regions months to years in advance can be skillful in some regions (Stock et al., 2015; Tommasi et al., 2017a,b; Hervieux et al., 2019; Jacox et al., 2019a; Park et al., 2019; Jacox et al., 2020). Integration of such forecasts with environmentally informed single species population dynamics models can enable managers to set more effective catch limits, but their utility will be dependent on how well management needs align with the regions and times with adequate forecast skill. For example, sea surface temperature (SST) forecast skill in the CCE is variable in space (with highest skill in more northern latitudes) and time (with highest skill for late winter and early spring forecasts) (Jacox et al., 2019a). In an evaluation of management performance for sardine, ecological and economic metrics were improved by SST forecasts only up to 4 months in advance, as forecast skill degraded at longer lead times (Tommasi et al., 2017c). This 4-month lead time may not be sufficient for inclusion of such approaches into current CPS management timelines (Tommasi et al., 2017c), but other applications may be able to leverage greater predictability for different seasons, lead times, regions, or environmental variables.
Use of environmental indicators of stock productivity to inform tactical decisions (e.g., catch advice) require adequate process understanding of the environment-species response, long time series of both biological and environmental variables at appropriate spatial and temporal scales, a strong effect of the environmental covariate on stock dynamics, and the ability to monitor and skillfully forecast the indicator (Skern-Mauritzen et al., 2018; Haltuch et al., 2019b). Improvements of management performance with the use of climate-enhanced stock assessments and environmental covariate-based harvest control rules (HCRs, e.g., Howell et al., 2021) as compared to other methods needs to be carefully evaluated with management strategy evaluation (Haltuch et al., 2019b). In some cases, application of survey-derived recruitment indicators may be more appropriate (Walters and Collie, 1988). Nevertheless, since the stakeholder review of ESR indicators, some analyses and models that speak to the needs highlighted in Comments 1–8 have been developed and used to inform PFMC management decisions (Table 2). We highlight those examples below and then discuss future research avenues.
For salmon, in 2017, the Council’s advisory bodies expressed concern about increasing variability in salmon escapements and worsening performance of forecasts (Pacific Fishery Management Council (PFMC), 2017c). This, along with earlier calls to investigate potential threshold values in indicators reported in the ESR (Pacific Fishery Management Council (PFMC), 2015b), prompted Satterthwaite et al. (2020) to investigate non-linear relationships between environmental covariates and forecast performance for Chinook salmon stocks of particular management concern. While mechanistic drivers of salmon demographic rates need to be investigated further before direct inclusion into pre-season forecast models, the work demonstrates that environmental indicators could be used indirectly to alert managers that forecast performance may be poor and that a precautionary approach may be warranted (Satterthwaite et al., 2020).
Similar correlative approaches also inform the PFMC’s environmentally driven exploitation rates in the HCR for Pacific sardine (Sardinops sagax, Pacific Fishery Management Council (PFMC), 2020), based on the recognition that the spawner-recruit relationship barely extended above the replacement line during cool periods, while indicating substantial compensation (surplus production) during warm periods (Jacobson and MacCall, 1995). In this case, rather than the environmental indicator being included directly in the stock assessment to inform a short-term forecast of fishable biomass, an age-structured population dynamics model with an environment-recruitment link was first used to determine how the fishing mortality reference point depends on a temperature indicator, and then a management strategy evaluation (MSE) was employed to compare performance of different types of harvest control rules and potential environmental indicators (Hurtado-Ferro and Punt, 2014). Temperature-dependent fishing mortality target reference points are also utilized for tactical management of cod (Gadus morhua) and whiting (Merlangius merlangus) in the Celtic Sea (Howell et al., 2021).
A relationship between sea level and recruitment has also been identified and included in the 2019 assessment for sablefish (Anoplopoma fimbria, Haltuch et al., 2019c). However, inclusion of the environmental indicator in the stock assessment did not influence assessment output as it was consistent with survey length and compositions (Haltuch et al., 2019c). For long-lived species like groundfish that recruit into the fishery at older ages and for which recent recruits make up a smaller fraction of the biomass, a short-term forecast (sub-annual to annual) of fishable biomass is largely informed by the observed fishery and survey data. A short-term recruitment forecast may therefore not substantially improve short-term biomass forecast skill and derived management measures. For this life history type, environmentally informed recruitment forecasts may inform longer-term (2 years onward) projections of stock biomass or reference points. To date, projections have not considered environmental conditions; however, it may be beneficial to do so in cases like sablefish, for which an environment-recruitment relationship has been established. However, the environmental covariate would need to be forecasted with adequate skill.
At PFMC, efforts to improve our understanding of drivers of species productivity and the performance of biomass forecasts and projections will continue in the future and are of interest to managers and stakeholders (Table 1, comments 1–8). There are numerous avenues by which ecosystem science could contribute, which are highlighted below and in Table 2. Improvements to ecosystem indicator development, such as the use of multivariate statistical techniques that reduce the dimensionality of a large set of covariates with minimal information loss, could refine inputs to existing environmentally driven forecasts (e.g., Rupp et al., 2012; Burke et al., 2013; Muhling et al., 2018). Exploratory statistical analyses based on improved ecological understanding of species interactions (e.g., trophic relationships) and employing a variety of data sources can also inform development of new productivity indicators (e.g., Tolimieri et al., 2018). Wells et al. (2017), examining seabird diet and forage survey data, demonstrated that salmon survival decreases when common murre (Uria aalge) switch from foraging juvenile rockfish (Sebastes spp.) offshore to anchovy inshore following changes in upwelling. Where seabird data exists, such an analysis could be extended, as suggested by Comment 8, to inform development of indicators for salmon stocks in the Northern CCE. Similar statistical models could be used to identify ecosystem indicators, such as seabird abundance or reproductive success, that relate to forage fish abundance (Comment 7).
Ecosystem models capturing the mechanistic processes leading to changes in demographic rates are also a promising tool to develop indicators to inform forecasts. For instance, processes occurring during the critical early ocean entry period have long been thought to be a major driver of overall cohort abundance for salmon (Pearcy, 1992; Beamish and Mahnken, 2001). Fiechter et al. (2015) developed a spatially explicit bioenergetics model of salmon linked to a configuration of the Regional Ocean Modeling System (ROMS) with biogeochemistry, and an index of juvenile salmon growth potential derived from this model was capable of describing a large proportion of variation in cohort strength (Henderson et al., 2019). The ROMS-informed bioenergetic model enabled synthesis of how oceanographic indicators (including krill concentration) affect juvenile growth potential (Fiechter et al., 2015). Then, a multivariate statistical technique was used to summarize the spatial variation in growth across years to inform a regression model of Central Valley Chinook salmon survival (Henderson et al., 2019). This model could now provide projections of juvenile survival informing pre-season forecasts of Central Valley Chinook returns, and a similar approach could be expanded to other stocks and species.
It could also be fruitful for ecosystem modelers to turn their attention to factors operating later in the life cycle that could influence growth, maturation rates or mortality. For salmon, improved estimation of maturation rates and mortality can inform forecasts based on sibling regressions where the returns of younger age classes in the previous year are used to forecast returns of older ages from the same cohort in forecast years (Peterman, 1982), as well as projections of future fishable biomass. Indeed, integration of an environmentally informed mortality parameterization in a population dynamics model of summer flounder (Paralichthys dentatus) in the U.S. East Coast resulted in improved biomass estimates (O’Leary et al., 2018).
Development of the Henderson et al., 2019 ecosystem model and derived salmon productivity indicators were facilitated by advancements in ocean modeling of the CCE. Assimilation of observational oceanographic data with ROMS has now enabled the development of a fine-scale reconstruction of physical ocean conditions going back to 1980 (Neveu et al., 2016)1. These capabilities were also essential for the development of key indicators of rockfish recruitment (Schroeder et al., 2018), sablefish recruitment (Tolimieri et al., 2018), petrale sole (Eopsetta jordani) recruitment (Haltuch et al., 2020), and new indices of upwelling (Jacox et al., 2018) or upwelling habitat compression (Santora et al., 2020) that may be relevant to target and protected species.
However, as evidenced by Pacific sardine (McClatchie et al., 2010; Jacobson and McClatchie, 2013; Zwolinski and Demer, 2019), correlative relationships can break down over time (Myers, 1998). Thus, an adaptive process enabling regular re-evaluation of the relationships between environmental indicators and fish productivity needs to be in place if they are to inform management (Skern-Mauritzen et al., 2016). For salmon, the PFMC has established processes for annual technical review of proposed changes to forecast methodology (Pacific Fishery Management Council (PFMC), 2008). The existing ESR indicator technical review process could enable an annual re-evaluation of the correlative relationships between stocks and their environment and allow for periodic refinements to the oceanographic, ecosystem and statistical models used to estimate species responses to the environment. Predictions derived from ecosystem-based models (e.g., Henderson et al., 2019) might be considered as competing models of existing approaches, and the ESR process could also provide a platform where different approaches are discussed, compared, and potentially integrated in a forecast ensemble, as is regularly done in weather and climate forecasting (Kirtman et al., 2014; Bauer et al., 2015).
The second set of ecosystem-level understanding comments (9 and 10), reflects the management need for more spatial distribution information to minimize the risk of interactions between fisheries and protected species, thereby increasing opportunities to fish for the target species (Table 1). This information need has become particularly critical in recent years, as populations of protected predators (e.g., sea lions) in the CCE recover, increasing the potential for overlap with fisheries (McClatchie et al., 2018). In addition, Comment 10 highlights the need to assess the links between changes in prey availability over space and predator distribution (Table 1). Understanding spatiotemporal overlap between predators and potential prey species is particularly important for the development of a more ecosystem-focused approach to fisheries management (Carroll et al., 2019; Link et al., 2020). A number of negative ecological and economic events occurring within the CCE in recent years, including, but not limited to, unusual mortality events for sea lions and seabirds (Wells et al., 2013), and unprecedented whale entanglements (Santora et al., 2020), were the result of changes in predator distribution linked to changes in forage availability and unprecedented environmental conditions. These incidents served to highlight the need for spatial tools mapping changes in species overlap in response to changes in environmental conditions.
Species Distribution Models (SDMs) are a common tool used to describe the distribution of species, often in relation to their environment, or in relation to space and time covariates that act as proxies for unobserved processes. These geostatistical models allow for the inclusion of multiple predictors and are flexible enough to capture complex or non-linear relationships between a species and its environment (Guisan and Zimmermann, 2000; Elith and Leathwick, 2009; Norberg et al., 2019). SDMs developed using long observational time series can be used to describe the typical distributions of species. As such they have the potential to highlight anomalous changes in species distributions as a function of environmental change and to examine or anticipate how environmental conditions cause variability in species associations (Carroll et al., 2019; Table 2), making them useful tools to address Comments 9 and 10. Indeed, SDMs have been applied in various management contexts worldwide although predominantly in terrestrial systems. SDMs can be used to assess historical or climatological distributions (Valinia et al., 2014), dynamic distributions (Stanton et al., 2012), or predict how species distributions will change over multiple forecast horizons, from short-term forecasts (Payne et al., 2017) to climate change projections (Briscoe et al., 2016). A spatiotemporal mixed-effects model (vector autoregressive spatiotemporal model) has become an important SDM for fisheries scientists who seek to develop accurate historical indices of abundance for use in stock assessment (Thorson, 2019b). SDMs have also been used to produce climatological prediction maps of marine mammals to assess risk from sonar operations (Forney et al., 2012; Roberts et al., 2016; Robinson et al., 2017), to describe temperature-driven interannual variability in the distribution of Pacific hake (Merluccius productus) in the context of its joint management by the United States and Canada (Malick et al., 2020a), for spatial management planning (e.g., Leathwick et al., 2008; Valavanis et al., 2008; Esselman and Allan, 2011; Smith et al., 2020), and climate change impact assessments (e.g., Hazen et al., 2013; Kleisner et al., 2017). Of particular interest to managers is the use of SDMs to minimize interactions between fisheries and protected species or vulnerable life stages (e.g., Hobday et al., 2011; Howell et al., 2015; Lewison et al., 2015 and references therein, Druon et al., 2015; Little et al., 2015; Hazen et al., 2018).
Most SDMs have been applied in a historical context, to describe and understand drivers of past changes in species distribution and their overlap. An increasing number of studies are also using SDMs for climate change applications (e.g., Shelton et al., 2020), but use of SDMs to anticipate short- to medium-term (days to years) changes in species availability has only recently begun to receive attention (e.g., Kaplan et al., 2016; Thorson, 2019a) despite the need for such products (Comment 9). Model-based distribution forecasts have been used to reduce unintended catch of southern bluefin tuna in the East Australia Current (Hobday et al., 2010) and to explore reducing seabird interactions in the North Pacific Transition Zone (Žydelis et al., 2011). Since the stakeholder review of ESR indicators, pioneering applications have also been developed for the CCE that address Comments 9 and 10, as outlined below and in Table 2.
SDMs and satellite data or ocean model output are providing near-real time likelihoods of ship strike risk for blue whales (Balaenoptera musculus) in the California Current (Hazen et al., 2017; Abrahms et al., 2019), and the ratio of catch to bycatch of protected species in the California swordfish fishery (Brodie et al., 2018; Hazen et al., 2018; Welch et al., 2019; Table 2). The latter example, termed EcoCast, integrates predictions of habitat suitability for a target species (swordfish, Xiphias gladius) and multiple bycatch species (blue sharks, Prionace glauca; leatherback turtles, Dermochelys coriacea; and California sea lions, Zalophus californianus) to provide an integrated map of opportunity and risk. This tool is now fully operational (Welch et al., 2019), providing daily predictions for use by fishery managers and fishers when deciding where to fish or adjust management regulations. (Operational, here, and throughout the paper is defined as in Welch et al. (2019), “self-contained workflows that run automatically at a prescribed temporal frequency”). In the northern CCE, the J-SCOPE project uses a ROMS model with biogeochemistry and provides twice-annual seasonal forecasts that have shown skill for physical and biochemical conditions, including hypoxia, at lead times up to ∼4 months (Siedlecki et al., 2016), and these are being used to forecast Pacific hake and sardine distributions and migration (Kaplan et al., 2016; Malick et al., 2020b) and inform the ESR (Harvey et al., 2019).
Several recent advancements may allow for further development of SDMs to anticipate changes in species distributions and their overlap in the CCE at longer lead times (1–12 months), and thus expand their relevance for management applications (Comments 9 and 10). Advancements include improvements in the availability of output from global climate prediction systems at lead times up to a year, the configuration of regional ocean models to downscale such predictions for the CCE, and the implementation of SDMs that use ocean model fields as input (e.g., Brodie et al., 2018). Indeed, decision support tools at these longer lead times have been used to model the distribution of target and bycatch species in Australian fisheries up to 4 months in advance using output from global climate prediction systems (Hobday et al., 2011; Eveson et al., 2015).
Continued development of such products would require further interactions between PFMC managers, stakeholders, and scientists to determine species, regions, and timeframes of interest, and to ensure that physical and ecological forecast skill aligns with management needs. The ESR technical review that has created opportunities to begin those discussions may continue to provide a forum moving forward. Predictions of extreme events may be of particular interest to managers, and several steps must be taken to evaluate whether such predictions can be useful. For example, temperature anomalies were predictable for some but not all periods of the persistent 2014–2016 CCE heatwave (Jacox et al., 2019b), and the ability of SDMs to capture species distribution shifts under these novel conditions, even with perfect environmental data, differs by SDM model type and species (Becker et al., 2020; Muhling et al., 2020). Thus, more work is required to assess whether SDM forecast skill is adequate for management applications, as skillful forecasting of species distribution changes requires that both environmental conditions and species responses to those environmental changes are accurately predicted. This research will include the determination of which SDM architectures are best suited to anticipate changes in species distributions over the timescales most relevant to managers.
Comments 11 and 12 highlight the need for ecosystem synthesis to examine the tradeoffs between protection of dependent predators, sustainability of fish populations, including both forage and the higher trophic level target species feeding on them, and the resilience of fishing communities (Table 1). It is becoming apparent that trophic cascades resulting from variability in forage can have substantial and surprising consequences on coastal communities on the U.S. West Coast (Wells et al., 2017; Santora et al., 2020). Therefore, in addressing Comments 11 and 12 (Table 1), modeling frameworks enabling a broad approach to evaluating tradeoffs should be considered. Below, we focus on tools well suited to address these tradeoffs: end-to-end models, management strategy evaluation, and spatial modeling, and highlight specific examples of their application in the CCE to inform management issues. These examples are also reported in Table 2 and avenues for further research are discussed.
In the CCE, fishery managers must weigh the provision of adequate forage for dependent species against the importance of the CPS (sardine, squid, anchovy and mackerel) fishery to West Coast communities, while also safeguarding the forage species themselves. This balancing act is not unique to this region, and tradeoffs between forage fish harvest and predators have long been the focus of global analyses, modeling, and task forces (e.g., Cury et al., 2011; Smith et al., 2011; Pikitch et al., 2012). The need for consideration of trophic interactions in the management of CCE CPS fisheries was recognized early on by the PFMC, with the earliest information on trophic interactions informing management advice being derived from simple correlative relationships. For example, the first FMP passed by the PFMC, the 1978 Northern Anchovy FMP, included a cutoff parameter below which large-scale harvest was not allowed to provide adequate forage for brown pelicans (Anderson et al., 1980). Information about similar trophic relationships were instrumental in the PFMC’s decision in the early 2000s to reduce the Allowable Biological Catch of shortbelly rockfish, a previously non-targeted species, based on the significance of pelagic juvenile shortbelly rockfish to seabirds, salmon and other higher trophic level predators.
End-to-end ecosystem models like Atlantis (Fulton et al., 2011) and Ecopath with Ecosim (Christensen and Walters, 2004), which model the entire food-web from plankton to top predators, can be used to assess the bottom-up effects of increased removals of forage fish on piscivorous fish species and protected species, such as marine mammals and seabirds, that depend on forage fish as prey, as well as the top-down impacts of increasing predator biomass on forage fish (Table 2). Given the long-standing objective of ensuring adequate forage for predators in the CPS FMP, and consistent with the 1998 Ecosystem Principles Advisory Panel (Ecosystem Principles Advisory Panel (EPAP), 1999), end-to-end ecosystem models became increasingly important to PFMC CPS management efforts during the early 2000s. For example, later amendments to the CPS FMP addressing krill management in the CCE were informed by both empirical data and insights from mass balance ecosystem models (Field et al., 2006). Confronting the management needs with the limitations of both the data and the models was helpful in this effort, as a key outcome was the recognition that the apparent high consumption of krill by key predators was often inconsistent with (considerably greater than) the estimates of krill abundance and productivity (Pacific Fishery Management Council (PFMC), 2009). Although the reasons for this inconsistency remain unknown, this limitation informed the decision to ultimately prohibit a directed CCE krill fishery in the absence of improved information for management.
Recognizing that models that include ecosystem processes and interactions are key to better informing the tradeoffs between forage needs and fisheries, among other things, the PFMC asked for a methodology review (Box 1) of the California Current Atlantis model in June 2014 (Pacific Fishery Management Council (PFMC), 2014; Kaplan and Marshall, 2016). The review process served as a platform to provide feedback on improvements required to increase utility of the model to management. For instance, the review noted that many of the management scenarios integrated in the CCE Atlantis model up to that point in time were not well aligned with specific PFMC management needs. Following the Atlantis methodology review and stakeholder review of ESR indicators, end-to-end ecosystem models for the CCE continue to be refined and have been used to evaluate long-term trophic impacts of U.S. West Coast groundfish fisheries (Pacific Fishery Management Council (PFMC), 2015a) and to assess the impacts of depleted forage species on predators (Koehn et al., 2016; Kaplan et al., 2017, 2019). The CCE Atlantis model can also be used to simulate the risks of climate-driven changes in the ocean environment, such as upwelling (Comments 3 and 4) and ocean acidification (Comment 13) on the CCE food-web, and it was linked to downscaled global climate projections to evaluate how the impacts of ocean acidification on benthic invertebrates may propagate through the CCE food-web and its fisheries (Marshall et al., 2017; Table 2). Clearly, end-to-end ecosystem models including species interactions and environmental drivers can potentially be further applied to assess tradeoffs between the ecosystem and economic impacts of management decisions (Table 2), but continued dialogue between managers and modelers is required to further tailor ecosystem models to answer management needs.
MSE has been widely used in fisheries management to highlight tradeoffs associated with alternative management actions, and to identify procedures that are robust to uncertainty (Punt et al., 2016a; ICES, 2021). MSE is therefore also an important tool to address Comments 11 and 12, related to how harvest rules for forage species may affect dependent predators, and vice versa. In simpler “one way” or bottom-up cases, an ecosystem model can be used to trace impacts of harvest rules on forage fish populations and fishery yields, and subsequently on predators. This was done in a recent herring MSE on the U.S. East Coast (Deroba et al., 2019; Feeney et al., 2019). Complex “two way” cases trace top-down impacts of predators on forage fish as well as bottom-up impacts on predators and are of interest when investigating multispecies harvest control rules or ecosystem reference points need to be tested. Kaplan et al. (2021) discuss additional applications of ecosystem models within MSE, including as operating models, and to simulate monitoring, assessment, and harvest control rules.
In one MSE example from the CCE, Punt et al. (2016b) applied a models of intermediate complexity for ecosystem assessments (MICE, Plagányi et al., 2014) rather than an end-to-end ecosystem model to assess the impact of CPS harvest rules on dependent predators. MICE typically simulate prey-predator interactions, but on a smaller set of ecosystem components. These simpler multispecies models are useful for answering targeted management questions relative to a specific policy concern. Their lower complexity allows, as in stock assessment, for parameter estimation based on fits to data, and uncertainty quantification, making them well suited for MSEs, and more readily understood by management bodies familiar with stock assessment models. The Punt et al. (2016a) MSE examined the links between the forage base and higher trophic level species (Comment 11), specifically the links between the population dynamics of sardine and anchovy and of two protected predator species, brown pelican (Pelecanus occidentalis) and California sea lion. The MSE was able to assess the tradeoffs between fishing on CPS and protection of predators (Comment 12, Table 2) by testing performance of current harvest policies for sardine with respect to both fishery and conservation goals. The MICE was developed in parallel with the Atlantis and Ecopath models mentioned above, facilitating model development, comparison and engagement with managers (Francis et al., 2018; Kaplan et al., 2019). Unlike the Ecopath or Atlantis applications, this MICE was able to quantify the performance of realistic management measures (including reproductive success and survival of protected species) while considering uncertainty in environmentally driven recruitment scenarios for sardine and anchovies as well as structural uncertainty regarding predator dependence on forage (Punt et al., 2016b).
Linking changes in the availability of forage species to higher trophic levels within particular geographic areas, the need highlighted by Comment 11, requires spatially explicit modeling for population dynamics of the species of interest. Both the CCE Atlantis and MICE model described above are spatial and can address Comment 11. However, the models have so far assumed a spatial distribution of forage species that remains constant over time. Considering the evidence for environmentally driven spatio-temporal variability in forage species (Muhling et al., 2020), with impacts on predator demographic rates, particularly for central place foragers such as sea lions (Fiechter et al., 2016), and on port-level availability to fishers (Smith et al., 2021), a valuable goal for future research is the refinement of existing ecosystem models in the CCE to include environmentally driven changes in forage distribution, as has been done elsewhere (e.g., Coll et al., 2019; Moullec et al., 2019). Given their fine-scale representation of spatial movement processes, individual based models (IBMs) are also suited to evaluate impacts of varying prey dynamics on central-place predator distribution and foraging behavior. For example, a multi-species IBM model of sardine, anchovy, and sea lions coupled to a regional ocean model with biogeochemistry was used to examine the impacts of environmental variability and prey availability on sea lion feeding success in the central CCE (Fiechter et al., 2016).
The final set of comments (14–17) underscores the need for understanding how changes in climate variability, mediated via ecosystem processes, affect fishing communities (Table 1). Climate change is expected to alter fish abundance and distribution (Cheung et al., 2010; Morley et al., 2018) and PFMC advisory bodies are interested in evaluating the potential risk of shifting species availability to coastal communities (Comments 14–15). Fine scale oceanographic data from remote sensing and ocean models, in combination with spatially explicit survey, tagging or logbook data, has enabled development of SDMs for a variety of PFMC-managed species (e.g., Thorson et al., 2016; Shelton et al., 2020). When data on fisher behavior (e.g., trip distance) is available from logbooks, port-specific fishing grounds can be identified and target species availability from SDMs over the fishing grounds can be computed (Rogers et al., 2019). Below, and in Table 2 we provide CCE-specific examples of how environmentally informed ecosystem models have been integrated with economic analyses to address comments 14–17, and what gaps remain.
With regards to the groundfish fishery in the CCE, indices of groundfish availability over distinct fishing grounds have been computed over the historical period (Selden et al., 2019; Table 2) and integrated into the ESRs (Harvey et al., 2019). To address comments 14–15, future work could develop such indices using environmentally informed SDMs to assess climate-induced shifts in economic opportunity (e.g., Smith et al., 2020), and project such changes into the future to assess the vulnerability of coastal communities to risk from climate change, as has been done for New England and Mid Atlantic fishing communities (Rogers et al., 2019). When spatially explicit other ecosystem models can also inform port-level socio-economic indices. In the CCE, the spatially explicit structure of the Atlantis model allowed translation of the results assessing climate impacts on the CCE food-web and its fisheries (Marshall et al., 2017) to port-based fishing communities and fleet-level economic effects (Hodgson et al., 2018). These model results have been presented to the PFMC’s to inform an ongoing strategic initiative on the effects of climate variability and change on fish stocks and fishing communities (Kaplan et al., 2018). By the explicit consideration of biological processes, end-to-end ecosystem models and MICE also have high potential to assess the cumulative effects of multiple environmental drivers (Comment 6, Table 2), e.g., under long-term climate change (Ainsworth et al., 2011; Koenigstein et al., 2016).
There is also a need to assess how extreme weather events directly affect safety of fishers (Comment 16, Table 1). Climate-change driven shifts in the frequency and strength of extreme weather events have the potential to directly affect the safety of commercial, recreational, and subsistence fishers. An active area of atmospheric research is concerned with how climate change may drive changes in storminess (Knutson et al., 2010; Dominguez et al., 2012; Kossin et al., 2016; Mölter et al., 2016; Ornes, 2018; Swain et al., 2018; Teich et al., 2018). Fishers and boaters are among the most sophisticated consumers of weather forecast information (Savelli and Joslyn, 2012; Finnis et al., 2019; Kuonen et al., 2019). Understanding how fishers respond to extreme weather events such as storms is essential to assessing the vulnerability of fishers and fishing communities to potential changes in storminess (Sainsbury et al., 2018), as well as consideration of how fishery-specific management and regulatory incentives affect fishers’ safety by influencing the level of risk fishers take to catch and land their fish (Pfeiffer and Gratz, 2016). Indeed, modeling work has shown that catch shares and other types of management that eliminate a race for fish and allow flexibility in the timing of trips decrease the propensity to take trips in hazardous weather (Petursdottir et al., 2001; Pfeiffer and Gratz, 2016; Petesch and Pfeiffer, 2019; Pfeiffer, 2020). Hidden Markov Models provide a tool to uncover underlying fisher behavior from vessel tracking data, such as from vessel monitoring systems or automatic identification systems. Such models and data sources are being increasingly used to determine simple behavioral states of fishers, e.g., ‘fishing’ or ‘searching’ (Joo et al., 2015), as well as to identify environmental factors that influence their behavior (Watson et al., 2018). Future work may employ behavioral models informed by environmental conditions to examine how fisher behavior changes in response to adverse weather conditions, produce estimates of fishers’ risk tolerance, and help promote safety at sea by evaluating the change in risk from fishery policies and climate change (Table 2).
Integration of environmental indicators with socio-economic models can also enable quantification of the impact of extreme events on fishing communities (Comment 17, Table 2). For instance, the 2014–2016 marine heatwave in the CCE triggered an unprecedented harmful algal bloom (HAB) (McCabe et al., 2016; Ryan et al., 2017), leading to considerable economic losses in fisheries for Dungeness crab (Metacarcinus magister) (Moore et al., 2019). To better alert communities of potential fisheries closures during HABs and mitigate their effects via adaptive actions, advisory bodies requested development of a HAB index at a localized scale and for a quantification of the economic impacts of HABs on fisheries participants (Comment 17). Moore et al. (2019) have developed a localized, community-specific index of lost fishing opportunity from HABs by computing the proportion of the Dungeness crab fishing season lost to HAB closures, which may be of interest to managers. In a follow-up study, Moore et al. (2020), using regression models built from fishers’ survey data, found that individuals who were exposed to longer fisheries closures, as measured by the HAB index, suffered greater income losses. Moore et al. (2020) also identified potential adaptive actions to reduce the impact of HABs on Dungeness crab fishery participants. These actions include income diversification and fishing for alternate species or in alternate areas. In addition, Anderson et al. (2016) developed a model to provide nowcasts and 1–3 day forecasts of HABs for the California coast2 by linking ROMS and satellite output to a statistical model of the likelihood of a toxic algal bloom. To better assess Comment 17 and assess the socioeconomic impacts of future shifts in HAB dynamics, future work could focus on developing more holistic models linking the socioeconomic analyses identifying the effects of HAB on fisheries described above to predictive HAB models.
For scientific information and analyses to directly support or affect public policies and regulations, the policymaking process should promote opportunities for scientists to engage with policymakers (Hopkins et al., 2011; Cvitanovic et al., 2015). Although ecosystem modeling is relatively new to fisheries management, it has entered a policymaking space where the ongoing examination of the best scientific information available to analyze management questions is both expected by fisheries managers and required by law [16 U.S.C. §1851(a)(2), see also 16 U.S.C. §1362(2), §1386(a), and §1536(a)(2)]. U.S. federal fisheries management has a 40 + year history of discussing, debating, and improving fisheries science by bringing that science into the public arena and testing it through application to ongoing fisheries. Engaging in the existing policy making space of the fishery management council process allows ecosystem modelers to make that needed connection between modeling and management priorities.
In assessing the responses of managers and stakeholders to the review of ESR indicators, we demonstrated that policy needs for ecosystem science go beyond the setting and use of environmental indicators to improve forecasts of biomass and reference points required for the setting of harvest limits. Other uses of ecosystem models and analysis identified included: (1) assessment of shifts in the spatial distribution of target stocks and protected species to anticipate changes in availability and the potential for interactions between fisheries and protected species, (2) identification of trophic interactions to better assess tradeoffs between protection of dependent predators and resilience of fishing communities in the management of forage species and to holistically assess the impact of climate change on PFMC-managed species, and (3) synthesis of how the environment affects fishing communities, either via extreme events such as HABs or storms or via climate-driven changes in target species availability, to promote efficiency and profitability of fisheries. The identified policy needs largely reflect the broad aims of EBFM (National Marine Fisheries Service, 2016a) but were brought forward directly by managers and stakeholders operating in the CCE and thus are relevant to their experience and specific requirements and are more regionally actionable. By including a stakeholder review of ESR indicators into an existing policy discussion process, other regions could replicate our work to ensure that their ecosystem modeling complements legally mandated avenues for using best available science in management and for setting research priorities (Box 1, Pacific Fishery Management Council (PFMC), 2018). Given limited resources, the process here outlined could then be followed by an ecosystem risk assessment (Holsman et al., 2017) to prioritize analyses and model development to focus on initially, as was done successfully by the U.S. Mid-Atlantic Fishery Management Council (Gaichas et al., 2018).
While existing ecosystem modeling capabilities in the region can address many of the policy needs identified by the ESR comments (Table 2), for some applications, improvements in ecosystem modeling capabilities are required to further the utility of ecosystem models and analyses to management needs (Table 2). Comments 1 and 10 stressed the need to anticipate future changes in productivity or species interactions. While ecosystem models and analyses have shown skill for some species in predicting changes in productivity and distribution over the historical period using observed data or data assimilative ocean model output (e.g., Brodie et al., 2018; Tolimieri et al., 2018) and have in some cases been used to assess impacts of climate change (Hazen et al., 2013; Haltuch et al., 2019a), the skill of near term (months to years in advance) ecological forecasts needs to be tested to assess their utility to the setting of catch limits, biomass projections, or spatial management measures at the spatiotemporal scales that are relevant to managers. Development of forecasting capabilities for fish productivity or distribution changes would also benefit from expansion of the use of ecosystem models and analyses linked to oceanographic models to improve mechanistic understanding and to develop indicators with high explanatory power in modeling changes in species responses to environmental variability (e.g., Brodie et al., 2018; Tolimieri et al., 2018; Henderson et al., 2019). Utility of such methods should be assessed relative to current approaches and as part of a forecasting ensemble.
The ESR comments also show a clear desire on the part of managers and stakeholders to better assess the broader ecosystem impacts of management actions, particularly with regards to the tradeoffs between the forage needs of predators, fisheries for prey and predator species, and protections for non-target predator stocks. In light of the stakeholders’ and managers’ comments, ecosystem models have the potential to be used more routinely to assess the impact of changes in forage to dependent predators when linked to stock assessments (e.g., Drew et al., 2021) or MSE model output (e.g., Deroba et al., 2019), and to develop multispecies harvest control rules (HCRs) or ecosystem-level reference points (Link, 2018; Fulton et al., 2019; Holsman et al., 2020). This is in addition to their demonstrated utility in addressing specific strategic questions, such as the role of krill in the ecosystem (e.g., Pacific Fishery Management Council (PFMC), 2009) or the impact of climate change on PFMC-managed species (e.g., Marshall et al., 2017). However, in some cases, model refinements to include more realistic fishing scenarios based on current harvest rules or more realistic responses to environmental variability, particularly with regards to changes in species distribution, may be required before implementation (Table 2).
Many comments also acknowledged the need to better integrate human dimension considerations when assessing impacts of management policies on port-level socioeconomic metrics, particularly within the context of climate variability and change. While case studies for specific regions and fisheries have shown promising approaches (e.g., Plagányi et al., 2013; Rogers et al., 2019; Selden et al., 2019), further development of methods linking spatially explicit biological models to socioeconomic outcomes, as well as improved consideration of the diversity of harvesting portfolios (Frawley et al., 2021), is required. In particular, links to on-shore community impacts, many of which are qualitative socio-cultural measures, have been neglected and may require direct consultation with communities rather than quantitative modeling (Okamoto et al., 2020). This will necessitate further communication not only between ecosystem modelers and managers, but also between ecosystem modelers, managers, and (non-economic) social scientists. While the findings presented here can, in collaboration with managers and stakeholders, help refine ecosystem modeling planning, ecosystem model development for improved management applicability also needs to be balanced with research and development innovations to identify emerging information needs.
As ecosystem modeling insights evolve to more explicitly inform both tactical and strategic management, the means to better quantify and present uncertainty in such model outputs or scenarios will become more critical (Link et al., 2012; Weijerman et al., 2015; Jacobsen et al., 2016; Haltuch et al., 2019b). Combining information across approaches via model averaging or ensembles (Marmion et al., 2009; Ianelli et al., 2016; Karp et al., 2019), or using Bayesian updating (Staton and Catalano, 2019) or state-space models (Fleischman et al., 2013) to formally integrate observations and modeled effects of drivers from multiple stages of a species life cycle may provide more reliable model output, and improved characterization of forecast uncertainty (i.e., model spread) on which to base decisions (Ianelli et al., 2016). Agreement in the predictions of an ensemble of structurally different ecosystem models can also increase stakeholder confidence in the model results (Jacobsen et al., 2016). MSE frameworks, which assess robustness of alternative management strategies to a range of uncertainties captured by a set of diverse operating models (Punt et al., 2016a), may be useful to both characterize uncertainty and communicate to stakeholders its impact on management performance.
Despite the growing need for ecosystem information and existing ecosystem modeling capabilities in the region potentially useful to the identified policy needs, only a few of these models or analyses have been implemented in management frameworks (Table 2). With regards to stock assessment science, there is a well-established routine review process that has enabled continued feedback between managers and modelers, and model refinement aimed at improving utility to management issues. Indeed, our work demonstrates that most implementations of ecosystem analysis in the PFMC have been via the development of indices for single-species climate informed population dynamics models (i.e., salmon forecasts, sardine HCR, sablefish stock assessment) aimed at deriving better estimates of biomass and reference points on which to base harvest decisions. These models are embedded in the PFMC process: council advisory bodies are familiar with them, they are regularly used to set catch limits, and their limitations and potential improvements are routinely discussed during their review process. This has facilitated faster uptake of ecosystem consideration in the PFMC via this type of vetted models. However, examples from other regions have demonstrated that regular dialogue between ecosystem modelers and advisory bodies via existing management council processes can foster, gradually, adoption of new management approaches (e.g., Holsman et al., 2016, 2019; Ianelli et al., 2019; Drew et al., 2021).
We suggest that in the PFMC and elsewhere, uses of ecosystem models and analyses could similarly be vetted and refined within the existing technical review, methodology review, stock assessment, and harvest setting process, or addressed in a more targeted review process such as for the Atlantis model in the CCE [Kaplan and Marshall, 2016, or as ‘key runs’ in ICES (2021)]. As for stock assessment, such interactions between managers and ecosystem modelers should be iterative. As highlighted in Figure 3, we propose that, for the PFMC, the annual technical review of ESR indicators, coupled with more in-depth methodology reviews when warranted, could serve as a forum for routine, iterative dialogue between managers and ecosystem modelers. This forum would enable discussion of ecosystem models and analyses showing potential utility but requiring further discussion on key details (e.g., species, timescales, and spatial scales of interest) with managers and stakeholders for implementation (Figure 3 and Table 2). For those analyses and models that have already been reviewed or implemented, this forum would provide a platform for periodic review of model refinements or new applications. The manager-modelers idea sharing process here presented (Figure 3) could enable the structured, iterative, and interactive communication between managers, stakeholders, and modelers that is key to refining existing ecosystem models and analyses for management use.
Figure 3. Overview of the PFMC forum for routine discussion of ecosystem models and analyses and the management process and policy issues informed by those models and analyses.
This paper explicitly looks at the comments on ESR indicators that pertained to an ecosystem-level understanding of fish stocks and fisheries. However, the comments that PFMC received on ESR indicators also ranged into questions about spatial management and links between climate variability and shifting stock distribution, extreme climate events, forecasting future risk, and about better understanding fishing community dependence on fishery resources and vulnerability to shifting stock availability. For natural resource managers, discussions of these wide-ranging questions and ideas are possible when working in an open, public process that involves stakeholders with diverse and sometimes competing goals. For ecosystem modelers, being open to the ideas that drive management processes and being willing to listen for how management processes communicate those ideas is key to successful connections between their models and management needs.
Several key aspects of our case study are present in other management systems for public trust resources, and this study may serve as a blueprint for matching models to management needs in a variety of policy making processes worldwide. To facilitate adoption of scientific knowledge in support of management decisions, existing natural resources management frameworks (e.g., Hopkins et al., 2011; Gregory et al., 2012; Mach and Field, 2017; Francis et al., 2018) highlight the need for continued, iterative engagement between scientists and decision makers. Here we find that both ecosystem scientists and managers have pre-existing tools in place, but nexus points between the science and management communities need to be present to foster information sharing and support the development of ecosystem models of interest and use to resource managers and the public. Development and use of ecosystem models should be guided by established best practices for model use (e.g., Collie et al., 2016; Punt et al., 2016a), forums like ecosystem modeling workshops that focus on model improvements and information sharing (e.g., Weijerman et al., 2016; Townsend et al., 2017), and science integration templates like integrated ecosystem assessment (e.g., Levin et al., 2009; Harvey et al., 2020). Resource management processes that require regular assessments of key resources (stocks, habitats, protected species) and activities (fishing, conservation actions) foster the scientific data collection that supports ecosystem modeling. Management processes, like fishery management councils, that maintain space in their processes for discussing ecosystem science and EBFM signal their openness to considering and using new ecosystem information as it arises and can serve as forums to facilitate matchmaking between models and management needs (see Figures 2, 3).
The original contributions presented in the study are included in the article, further inquiries can be directed to the corresponding author/s.
DT, YR, HT, and CH developed the initial idea for this manuscript. DT, CH, SB, and SK reviewed and categorized comments from the Coordinated Ecosystem Indicators Review. All authors have contributed writing and editing.
This work was supported by the NOAA Climate Program Office’s Coastal and Ocean Climate Applications Program (NA17OAR4310268), Modeling, Analysis, Predictions, and Projections Program (NA17OAR4310108), and the NOAA Fisheries Office of Science and Technology.
The scientific results and conclusions, as well as any views or opinions expressed herein, are those of the author(s) and do not necessarily reflect the views of NOAA or the Department of Commerce.
The authors declare that the research was conducted in the absence of any commercial or financial relationships that could be construed as a potential conflict of interest.
We thank all the stakeholders, managers, and scientists who participated in the PFMC stakeholder review of ESR indicators as well as the participants of the NOAA NEMoW 5 workshop for useful suggestions on an early idea for the paper. We also thank J. Samhouri for helpful comments on an earlier draft of the manuscript and the two journal reviewers for insightful comments that helped improve the manuscript.
Abrahms, B., Welch, H., Brodie, S., Jacox, M. G., Becker, E. A., Bograd, S. J., et al. (2019). Dynamic ensemble models to predict distributions and anthropogenic risk exposure for highly mobile species. Divers. Distrib. 25, 1182–1193. doi: 10.1111/ddi.12940
Ainsworth, C., Samhouri, J., Busch, D., Cheung, W. W. L., Dunne, J., and Okey, T. A. (2011). Potential impacts of climate change on Northeast Pacific marine foodwebs and fisheries. ICES J. Mar. Sci. 68, 1217–1229.
Anderson, C. R., Kudela, R. M., Kahru, M., Chao, Y., Rosenfeld, L. K., Bahr, F. L., et al. (2016). Initial skill assessment of the California Harmful Algae Risk Mapping (C-HARM) system. Harmful Algae 59, 1–18. doi: 10.1016/j.hal.2016.08.006
Anderson, D. W., Gress, F., Mais, K. F., and Kelly, P. R. (1980). “Brown Pelicans as anchovy stock indicators and their relationship to commercial fishing,” in California Cooperative Oceanic Fisheries. Reports XXI. La Jolla, CA.
Bailey, R. M., Carrella, E., Axtell, R., Burgess, M. G., Cabral, R. B., Drexler, M., et al. (2019). A computational approach to managing coupled human–environmental systems: the POSEIDON model of ocean fisheries. Sustain. Sci. 14, 259–275. doi: 10.1007/s11625-018-0579-9
Basson, M. (1999). The importance of environmental factors in the design of management procedures. ICES J. Mar. Sci. 56, 933–942.
Bauer, P., Thorpe, A., and Brunet, G. (2015). The quiet revolution of numerical weather prediction. Nature 525, 47–55. doi: 10.1038/nature14956
Bax, N. J. (1998). The significance and prediction of predation in marine fisheries. ICES J. Mar. Sci. 55, 997–1030.
Beamish, R. J., and Mahnken, C. (2001). A critical size and period hypothesis to explain natural regulation of salmon abundance and the linkage to climate and climate change. Prog. Oceanogr. 49, 423–437. doi: 10.1016/S0079-6611(01)00034-9
Becker, E. A., Carretta, J. V., Forney, K. A., Barlow, J., Brodie, S., Hoopes, R., et al. (2020). Performance evaluation of cetacean species distribution models developed using generalized additive models and boosted regression trees. Ecol. Evol. 10, 5759–5784. doi: 10.1002/ece3.6316
Briscoe, N. J., Kearney, M. R., Taylor, C. A., and Wintle, B. A. (2016). Unpacking the mechanisms captured by a correlative species distribution model to improve predictions of climate refugia. Glob. Change Biol. 22, 2425–2439. doi: 10.1111/gcb.13280
Brodie, S., Jacox, M. G., Bograd, S. J., Welch, H., Dewar, H., Scales, K. L., et al. (2018). Integrating dynamic subsurface habitat metrics into species distribution models. Front. Mar. Sci. 5:219. doi: 10.3389/fmars.2018.00219
Burke, B. J., Peterson, W. T., Beckman, B. R., Morgan, C., Daly, E. A., and Litz, M. (2013). Multivariate models of adult pacific salmon returns. PLoS One 8:e0054134. doi: 10.1371/journal.pone.0054134
Carroll, G., Holsman, K. K., Brodie, S., Thorson, J. T., Hazen, E. L., Bograd, S. J., et al. (2019). A review of methods for quantifying spatial predator–prey overlap. Glob. Ecol. Biogeogr. 28, 1561–1577. doi: 10.1111/geb.12984
Cheung, W. W. L., Lam, V. W. Y., Sarmiento, J. L., Kearney, K., Watson, R., Zeller, D., et al. (2010). Large-scale redistribution of maximum fisheries catch potential in the global ocean under climate change. Glob. Chang. Biol. 16, 24–35. doi: 10.1111/j.1365-2486.2009.01995.x
Christensen, V., and Walters, C. J. (2004). Ecopath with Ecosim: methods, capabilities and limitations. Ecol. Modell. 172, 109–139. doi: 10.1016/j.ecolmodel.2003.09.003
Coll, M., Pennino, M. G., Steenbeek, J., Sole, J., and Bellido, J. M. (2019). Predicting marine species distributions: complementarity of food-web and Bayesian hierarchical modelling approaches. Ecol. Model. 405, 86–101.
Collie, J. S., Botsford, L. W., Hastings, A., Kaplan, I. C., Largier, J. L., Livingston, P. A., et al. (2016). Ecosystem models for fisheries management: finding the sweet spot. Fish Fish. 17, 101–125. doi: 10.1111/faf.12093
Cowan, J. H., Rice, J. C., Walters, C. J., Hilborn, R., Essington, T. E., Day, J. W., et al. (2012). Challenges for implementing an ecosystem approach to fisheries management. Mar. Coast. Fish. 4, 496–510. doi: 10.1080/19425120.2012.690825
Cury, P. M., Boyd, I. L., Bonhommeau, S., Anker-Nilssen, T., Crawford, R. J. M., Furness, R. W., et al. (2011). Global seabird response to forage fish depletion - one-third for the birds. Science 334, 1703–1706.
Cvitanovic, C., Hobday, A., van Kerkhoff, L., Wilson, S., Dobbs, K., and Marshall, N. A. (2015). Improving knowledge exchange among scientists and decision-makers to facilitate the adaptive governance of marine resources: a review of knowledge and research needs. Ocean Coast Manag. 112, 25–35.
Deroba, J. J., Gaichas, S. K., Lee, M.-Y., Feeney, R. G., Boelke, D., and Irwin, B. J. (2019). The dream and the reality: meeting decision-making time frames while incorporating ecosystem and economic models into management strategy evaluation. Can. J. Fish. Aquat. Sci. 76, 1112–1133. doi: 10.1139/cjfas-2018-0128
Dinesen, G. E., Neuenfeldt, S., Kokkalis, A., Andreas, L., Egekvist, J., Kristensen, K., et al. (2019). Cod and climate: a systems approach for sustainable fisheries management of Atlantic cod (Gadus morhua) in coastal Danish waters. J. Coast. Conserv. 23, 943–958. doi: 10.1007/s11852-019-00711-0
Dominguez, F., Rivera, E., Lettenmaier, D. P., and Castro, C. L. (2012). Changes in winter precipitation extremes for the western United States under a warmer climate as simulated by regional climate models. Geophys. Res. Lett. 39:L05803. doi: 10.1029/2011GL050762
Drew, K., Cieri, M., Schueller, A., Buchheister, A., Chagaris, D., Nesslage, G., et al. (2021). Balancing model complexity, data requirements, and management objectives in developing ecological reference points for atlantic menhaden. Front. Mar. Sci. 8:608059. doi: 10.3389/fmars.2021.608059
Druon, J. N., Fiorentino, F., Murenu, M., Knittweis, L., Colloca, F., Osio, C., et al. (2015). Modelling of European hake nurseries in the Mediterranean Sea: an ecological niche approach. Prog. Oceanogr. 130, 188–204.
Ecosystem Principles Advisory Panel (EPAP) (1999). Ecosystem-Based Fishery Management: A Report to Congress by the Ecosystem Principles Advisory Panel. Washington, D.C: U.S. Dept. of Commerce.
Elith, J., and Leathwick, J. R. (2009). Species distribution models: ecological explanation and prediction across space and time. Annu. Rev. Ecol. Evol. Syst. 40, 677–697. doi: 10.1146/annurev.ecolsys.110308.120159
Esselman, P. C., and Allan, J. D. (2011). Application of species distribution models and conservation planning software to the design of a reserve network for the riverine fishes of northeastern Mesoamerica. Freshw. Biol. 56, 71–88. doi: 10.1111/j.1365-2427.2010.02417.x
Eveson, J. P., Hobday, A. J., Hartog, J. R., Spillman, C. M., and Rough, K. M. (2015). Seasonal forecasting of tuna habitat in the Great Australian Bight. Fish. Res. 170, 39–49. doi: 10.1016/j.fishres.2015.05.008
Feeney, R. G., Boelke, D. V., Deroba, J. J., Gaichas, S., Irwin, B. J., and Lee, M. (2019). Integrating management strategy evaluation into fisheries management: advancing best practices for stakeholder inclusion based on an MSE for Northeast US Atlantic herring. Can. J. Fish. Aquat. Sci. 76, 1103–1111. doi: 10.1139/cjfas-2018-0125
Fiechter, J., Huckstadt, L. A., Rose, K. A., and Costa, D. P. (2016). A fully coupled ecosystem model to predict the foraging ecology of apex predators in the California Current. Mar. Ecol. Prog. Ser. 556, 273–285.
Fiechter, J., Huff, D. D., Martin, B. T., Jackson, D. W., Edwards, C. A., Rose, K. A., et al. (2015). Environmental conditions impacting juvenile Chinook salmon growth off central California: an ecosystem model analysis. Geophys. Res. Lett. 42, 2910–2917. doi: 10.1002/2015GL063046
Field, J. C., Francis, R. C., and Aydin, K. (2006). Top-down modeling and bottom-up dynamics: linking a fisheries-based ecosystem model with climate hypotheses in the Northern California Current. Prog. Oceanogr. 68, 238–270.
Finnis, J., Shewmake, J. W., Neis, B., and Telford, D. (2019). Marine forecasting and fishing safety: improving the fit between forecasts and harvester needs. J. Agromed. 24, 324–332. doi: 10.1080/1059924X.2019.1639576
Fleischman, S. J., Catalano, M. J., Clark, R. A., and Bernard, D. R. (2013). An age-structured state-space stock–recruit model for Pacific salmon (Oncorhynchus spp.). Can. J. Fish. Aquat. Sci. 70, 401–414. doi: 10.1139/cjfas-2012-0112
Fogarty, M. J. (2014). The art of ecosystem-based fishery management. Can. J. Fish. Aquat. Sci. 71, 479–490.
Forney, K. A., Ferguson, M. C., Becker, E. A., Fiedler, P. C., Redfern, J. V., Barlow, J., et al. (2012). Habitat-based spatial models of cetacean density in the eastern Pacific Ocean. Endanger. Species Res. 16, 113–133. doi: 10.3354/esr00393
Francis, T. B., Levin, P. S., Punt, A. E., Kaplan, I. C., Varney, A., and Norman, K. (2018). Linking knowledge to action in ocean ecosystem management: the Ocean Modeling Forum. Elementa Sci. Anthrop. 6:83. doi: 10.1525/elementa.338
Frawley, T. H., Muhling, B. A., Brodie, S., Fisher, M. C., Tommasi, D., Le Fol, G., et al. (2021). Changes to the structure and function of an albacore fishery reveal shifting social-ecological realities for Pacific Northwest fishermen. Fish Fish. 22, 280–297. doi: 10.1111/faf.12519
Fulton, E. A. (2021). Opportunities to improve ecosystem-based fisheries management by recognizing and overcoming path dependency and cognitive bias. Fish Fish. 22, 428–448. doi: 10.1111/faf.12537
Fulton, E. A., Link, J. S., Kaplan, I. C., Savina-Rolland, M., Johnson, P., Ainsworth, C., et al. (2011). Lessons in modelling and management of marine ecosystems: the Atlantis experience. Fish Fish. 12, 171–188. doi: 10.1111/j.1467-2979.2011.00412.x
Fulton, E. A., Punt, A. E., Dichmont, C. M., Harvey, C. J., and Gorton, R. (2019). Ecosystems say good management pays off. Fish Fish. 20, 66–96. doi: 10.1111/faf.12324
Gaichas, S. K., DePiper, G. S., Seagraves, R. J., Muffley, B. W., Sabo, M. G., Colburn, L. L., et al. (2018). Implementing ecosystem approaches to fishery management: risk assessment in the US Mid-Atlantic. Front. Mar. Sci. 5:442. doi: 10.3389/fmars.2018.00442
Gregory, R., Failing, I., Harstone, M., Long, G., McDaniels, T., and Ohlson, D. (2012). Structured Decision Making: A Practical Guide to Environmental Management Choices. Hoboken, NJ: Wiley-Blackwell.
Guisan, A., and Zimmermann, N. E. (2000). Predictive habitat distribution models in ecology. Ecol. Modell. 135, 147–186. doi: 10.1016/S0304-3800(00)00354-9
Haltuch, M. A., A’mar, Z. T., Bond, N. A., and Valero, J. L. (2019a). Assessing the effects of climate change on US West Coast sablefish productivity and on the performance of alternative management strategies. ICES J. Mar. Sci. 76, 1524–1542. doi: 10.1093/icesjms/fsz029
Haltuch, M. A., Brooks, E. N., Brodziak, J., Devine, J. A., Johnson, K. F., Klibansky, N., et al. (2019b). Unraveling the recruitment problem: a review of environmentally-informed forecasting and management strategy evaluation. Fish. Res. 217, 198–216.
Haltuch, M. A., Johnson, K. F., Tolimieri, N., Kapur, M. S., and Castillo-Jordán, C. A. (2019c). Status of the Sablefish Stock in U.S. Waters in 2019. Portland, OR. Available online at: https://www.pcouncil.org/documents/2019/09/agenda-item-h-5-attachment-7-2.pdf/.
Haltuch, M. A., Tolimieri, N., Lee, Q., and Jacox, M. G. (2020). Oceanographic drivers of petrale sole recruitment in the California Current Ecosystem. Fish Oceanogr. 29, 122–136. doi: 10.1111/fog.12459
Harvey, C. J., Garfield, N., Williams, G., and Tolimieri, N. (eds) (2020). California Current Integrated Ecosystem Assessment (CCIEA) California Current ecosystem status report, 2020. Report to the Pacific Fishery Management Council. Washington, DC: U.S. Department of Commerce.
Harvey, C. J., Garfield, N., Williams, G., Tolimieri, N., Schroeder, I., Andrews, K., et al. (2019). Ecosystem Status Report of the California Current for 2019: A Summary of Ecosystem Indicators Compiled by the California Current Integrated Ecosystem Assessment Team (CCEIA). Washington, DC: U.S. Department of Commerce, doi: 10.25923/p0ed-ke21 NOAA Technical Memorandum NMFS-NWFSC-149.
Hazen, E. L., Jorgensen, S., Rykaczewski, R. R., Bograd, S. J., Foley, D. G., Jonsen, I. D., et al. (2013). Predicted habitat shifts of Pacific top predators in a changing climate. Nat. Clim. Chang. 3, 234–238. doi: 10.1038/nclimate1686
Hazen, E. L., Palacios, D. M., Forney, K. A., Howell, E. A., Becker, E., Hoover, A. L., et al. (2017). WhaleWatch: a dynamic management tool for predicting blue whale density in the California Current. J. Appl. Ecol. 54, 1415–1428. doi: 10.1111/1365-2664.12820
Hazen, E. L., Scales, K. L., Maxwell, S. M., Briscoe, D. K., Welch, H., Bograd, S. J., et al. (2018). EcoCast: a dynamic ocean management tool to reduce bycatch and support sustainable fisheries. Sci. Adv. 4:EAAR3001.
Henderson, M., Fiechter, J., Huff, D. D., and Wells, B. K. (2019). Spatial variability in ocean-mediated growth potential is linked to Chinook salmon survival. Fish. Oceanogr. 28, 334–344. doi: 10.1111/fog.12415
Hervieux, G., Alexander, M. A., Stock, C. A., Jacox, M. G., Pegion, K., Becker, E., et al. (2019). More reliable coastal SST forecasts from the North American multimodel ensemble. Clim. Dyn. 53, 7153–7168. doi: 10.1007/s00382-017-3652-7
Hilborn, R. (2011). Future directions in ecosystem based fisheries management: a personal perspective. Fish. Res. 108, 235–239. doi: 10.1016/j.fishres.2010.12.030
Hobday, A. J., Hartog, J. R., Spillman, C. M., and Alves, O. (2011). Seasonal forecasting of tuna habitat for dynamic spatial management. Can. J. Fish. Aquat. Sci. 68, 898–911. doi: 10.1139/f2011-031
Hobday, A. J., Hartog, J. R., Timiss, T., and Fielding, J. (2010). Dynamic spatial zoning to manage southern bluefin tuna (Thunnus maccoyii) capture in a multi-species longline fishery. Fish. Oceanogr. 19, 243–253. doi: 10.1111/j.1365-2419.2010.00540.x
Hodgson, E. E., Kaplan, I. C., Marshall, K. N., Leonard, J., Essington, T. E., Busch, D. S., et al. (2018). Consequences of spatially variable ocean acidification in the California Current: lower pH drives strongest declines in benthic species in southern regions while greatest economic impacts occur in northern regions. Ecol. Modell. 383, 106–117. doi: 10.1016/j.ecolmodel.2018.05.018
Holsman, K., Samhouri, J., Cook, G., Hazen, E., Olsen, E., Dillard, M., et al. (2017). An ecosystem-based approach to marine risk assessment. Ecosyst. Health Sustain. 3:e01256. doi: 10.1002/ehs2.1256
Holsman, K. K., Ianelli, J., Aydin, K., and Punt, A. E. (2016). A comparison of fisheries biological reference points estimated from temperature-specific multi-species and single-species climate-enhanced stock assessment models. Deep Sea Res. Part II Top. Stud. Oceanogr. 134, 360–378. doi: 10.1016/j.dsr2.2015.08.001
Holsman, K. K., Haynie, A. C., Hollowed, A. B., Reum, J. C. P., Aydin, K., Hermannn, A. J., et al. (2020). Ecosystem-based fisheries management forestalls climate-driven collapse. Nat. Commun. 11:4579. doi: 10.1038/s41467-020-18300-3
Holsman, K. K., Ianelli, J., Aydin, K., and Spies, I. (2019). “2019 Climate-enhanced multi-species Stock Assessment for walleye pollock, Pacific cod, and arrowtooth flounder in the Eastern Bering Sea,” in Stock Assessment and Fishery Evaluation Report for the Groundfish Resources for the Bering Sea/Aleutian Islands Regions, Anchorage, AK: North Pacific Fishery Management Council.
Hopkins, T. S., Bailly, D., and Støttrup, J. G. (2011). A systems approach framework for coastal zones. Ecol. Soc. 16:25. doi: 10.5751/ES-04553-160425
Howell, D., Schueller, A. M., Bentley, J. W., Buchheister, A., Chagaris, D., Cieri, M., et al. (2021). Combining ecosystem and single-species modeling to provide ecosystem-based fisheries management advice within current management systems. Front. Mar. Sci. 7:607831. doi: 10.3389/fmars.2020.607831
Howell, E. A., Hoover, A., Benson, S. R., Bailey, H., Polovina, J. J., Seminoff, J. A., et al. (2015). Enhancing the TurtleWatch product for leatherback sea turtles, a dynamic habitat model for ecosystem-based management. Fish. Oceanogr. 24, 57–68. doi: 10.1111/fog.12092
Hurtado-Ferro, F., and Punt, A. E. (2014). Agenda Item I.1.b Revised Analyses Related to Pacific Sardine Harvest Parameters. Portland, OR.
Ianelli, J., Holsman, K. K., Punt, A. E., and Aydin, K. (2016). Multi-model inference for incorporating trophic and climate uncertainty into stock assessments. Deep Sea Res. Part II Top. Stud. Oceanogr. 134, 379–389.
Ianelli, J. N., Fissel, B., Holsman, K., Honkalehto, T., Kotwicki, S., Monnahan, C., et al. (2019). “Chapter 1: assessment of the walleye pollock stock in the Eastern Bering Sea,” in Stock Assessment and Fishery Evaluation Report for the Groundfish Resources of the Bering Sea/Aleutian Islands Regions (Anchorage, AK: North Pacific Fishery Management Council).
ICES (2021). “Working group on multispecies assessment methods,” in WGSAM; outputs from 2020 Meet-Ing, Copenhagen, 231. doi: 10.17895/ices.pub.7695 ICES Scientific Reports. 3:10.
Jacobsen, N. S., Essington, T. E., and Andersen, K. H. (2016). Comparing model predictions for ecosystem-based management. Can. J. Fish. Aquat. Sci. 73, 666–676.
Jacobson, L. D., and MacCall, A. D. (1995). Stock-recruitment models for Pacific sardine (Sardinops sagax). Can. J. Fish. Aquat. Sci. 52, 566–577. doi: 10.1139/f95-057
Jacobson, L. D., and McClatchie, S. (2013). Comment on temperature-dependent stock–recruit modeling for Pacific sardine (Sardinops sagax) in Jacobson and MacCall (1995), McClatchie et al. (2010), and Lindegren and Checkley (2013). Can. J. Fish. Aquat. Sci. 70, 1566–1569. doi: 10.1139/cjfas-2013-0128
Jacox, M. G., Alexander, M. A., Siedlecki, S., Chen, K., Kwon, Y.-O., Brodie, S., et al. (2020). Seasonal-to-interannual prediction of North American coastal marine ecosystems: forecast methods, mechanisms of predictability, and priority developments. Prog. Oceanogr. 183:102307. doi: 10.1016/j.pocean.2020.102307
Jacox, M. G., Alexander, M. A., Stock, C. A., and Hervieux, G. (2019a). On the skill of seasonal sea surface temperature forecasts in the California Current System and its connection to ENSO variability. Clim. Dyn. 53, 7519–7533. doi: 10.1007/s00382-017-3608-y
Jacox, M. G., Tommasi, D., Alexander, M., Hervieux, G., and Stock, C. (2019b). Predicting the evolution of the 2014-16 California current system marine heatwave from an ensemble of coupled global climate forecasts. Front. Mar. Sci. 6:497. doi: 10.3389/fmars.2019.00497
Jacox, M. G., Edwards, C. A., Hazen, E. L., and Bograd, S. J. (2018). Coastal upwelling revisited: ekman, bakun, and improved upwelling indices for the U.S. West Coast. J. Geophys. Res. Ocean 123, 7332–7350. doi: 10.1029/2018JC014187
Joo, R., Salcedo, O., Gutierrez, M., Fablet, R., and Bertrand, S. (2015). Defining fishing spatial strategies from VMS data: insights from the world’s largest monospecific fishery. Fish. Res. 164, 223–230. doi: 10.1016/j.fishres.2014.12.004
Kaplan, I., Trainer, V., Jacox, M., and Siedlecki, S. (2018). ‘The State of the Art for Ecological Forecasting at Short-, Medium- and Longterm Time Frames’ [Slide presentation]. Pacific Fishery Management Council Climate and Communities Initiative. Available online at: https://www.pcouncil.org/actions/climate-and-communities-initiative/ (accessed July 13, 2020).
Kaplan, I. C., Williams, G. D., Bond, N. A., Hermann, A. J., and Siedlecki, S. A. (2016). Cloudy with a chance of sardines: forecasting sardine distributions using regional climate models. Fish. Oceanogr. 25, 15–27. doi: 10.1111/fog.12131
Kaplan, I. C., Francis, T. B., Punt, A. E., Koehn, L. E., Curchitser, E., Hurtado-Ferro, F., et al. (2019). A multi-model approach to understanding the role of Pacific sardine in the California Current food web. Mar. Ecol. Prog. Ser. 617–618, 307–321. doi: 10.3354/meps12504
Kaplan, I. C., Gaichas, S. K., Stawitz, C. C., Lynch, P. D., Marshall, K. N., Deroba, J. J., et al. (2021). Management strategy evaluation: allowing the light on the hill to illuminate more than one species. Front. Mar. Sci. 8:688.
Kaplan, I. C., Koehn, L. E., Hodgson, E. E., Marshall, K. N., and Essington, T. E. (2017). Modeling food web effects of low sardine and anchovy abundance in the California Current. Ecol. Modell. 359, 1–24. doi: 10.1016/j.ecolmodel.2017.05.007
Kaplan, I. C., and Marshall, K. N. (2016). A guinea pig’s tale: learning to review end-to-end marine ecosystem models for management applications. ICES J. Mar. Sci. 73, 1715–1724.
Karp, M. A., Blackhart, K., Lynch, P. D., Deroba, J., Hanselman, D., Gertseva, V., et al. (2019). Proceedings of the 13th National Stock Assessment Workshop: Model Complexity, Model Stability, and Ensemble Modeling. Washington, DC: U.S. Department of Commerce. NOAA Tech. Memo. NMFS-F/SPO-189.
Kirtman, B. P., Min, D., Infanti, J. M., Kinter, J. L., Paolino, D. A., Zhang, Q., et al. (2014). The North American multimodel ensemble: phase-1 seasonal-to-interannual prediction; phase-2 toward developing intraseasonal prediction. Bull. Am. Meteorol. Soc. 95, 585–601. doi: 10.1175/BAMS-D-12-00050.1
Kleisner, K. M., Fogarty, M. J., McGee, S., Hare, J. A., Moret, S., Perretti, C. T., et al. (2017). Marine species distribution shifts on the U.S. Northeast Continental Shelf under continued ocean warming. Prog. Oceanogr. 153, 24–36. doi: 10.1016/j.pocean.2017.04.001
Knutson, T. R., McBride, J. L., Chan, J., Emanuel, K., Holland, G., Landsea, C., et al. (2010). Tropical cyclones and climate change. Nat. Geosci. 3, 157–163. doi: 10.1038/ngeo779
Koehn, L. E., Essington, T. E., Marshall, K. N., Kaplan, I. C., Sydeman, W. J., Szoboszlai, A. I., et al. (2016). Developing a high taxonomic resolution food web model to assess the functional role of forage fish in the California Current ecosystem. Ecol. Modell. 335, 87–100. doi: 10.1016/j.ecolmodel.2016.05.010
Koenigstein, S., Mark, F. C., Gößling-Reisemann, S., Reuter, H., and Poertner, H.-O. (2016). Modelling climate change impacts on marine fish populations: process-based integration of ocean warming, acidification and other environmental drivers. Fish Fish. 17, 972–1004. doi: 10.1111/faf.12155
Kossin, J. P., Emanuel, K. A., and Camargo, S. J. (2016). Past and projected changes in Western North Pacific tropical cyclone exposure. J. Clim. 29, 5725–5739. doi: 10.1175/JCLI-D-16-0076.1
Kuonen, J., Conway, F., and Strub, T. (2019). Relating Ocean condition forecasts to the process of end-user decision making: a case study of the oregon commercial fishing community. Mar. Technol. Soc. J. 53, 53–66. doi: 10.4031/MTSJ.53.1.1
Leathwick, J., Moilanen, A., Francis, M., Elith, J., Taylor, P., Julian, K., et al. (2008). Novel methods for the design and evaluation of marine protected areas in offshore waters. Conserv. Lett. 1, 91–102. doi: 10.1111/j.1755-263x.2008.00012.x
Levin, P. S., Fogarty, M. J., Murawski, S. A., and Fluharty, D. (2009). Integrated ecosystem assessments: developing the scientific basis for ecosystem-based management of the ocean. PLoS Biol. 7:e1000014. doi: 10.1371/journal.pbio.1000014
Lewison, R., Hobday, A. J., Maxwell, S., Hazen, E., Hartog, J. R., Dunn, D. C., et al. (2015). Dynamic ocean management: identifying the critical ingredients of dynamic approaches to ocean resource management. BioScience 65, 486–498. doi: 10.1093/biosci/biv018
Lidström, S., and Johnson, A. F. (2020). Ecosystem-based fisheries management: a perspective on the critique and development of the concept. Fish Fish. 21, 216–222.
Link, J., Ihde, T., Townsend, H., Osgood, K., Schirripa, M., Kobayashi, D., et al. (2010). Report of the 2nd National Ecosystem Modeling Workshop (NEMoW II), Bridging the Credibility Gap - Dealing with Uncertainty in Ecosystem Models. Washington, DC: U.S. Department of Commerce. NOAA Technical Memorandum NMFS-F/SPO-102.
Link, J. S. (2010). Ecosystem-Based Fishery Management: Confronting Tradeoffs. Cambridge, MA: Cambridge University Press.
Link, J. S. (2018). System-level optimal yield: increased value, less risk, improved stability, and better fisheries. Can. J. Fish. Aquat. Sci. 75, 1–16. doi: 10.1139/cjfas-2017-0250
Link, J. S., Bundy, A., Overholtz, W. J., Shackell, N., Manderson, J., Duplisea, D., et al. (2011). Ecosystem-based fisheries management in the Northwest Atlantic. Fish Fish. 12, 152–170.
Link, J. S., Huse, G., Gaichas, S., and Marshak, A. R. (2020). Changing how we approach fisheries: a first attempt at an operational framework for ecosystem approaches to fisheries management. Fish Fish. 21, 393–434. doi: 10.1111/faf.12438
Link, J. S., Ihde, T. F., Harvey, C. J., Gaichas, S. K., Field, J. C., Brodziak, J. K. T., et al. (2012). Dealing with uncertainty in ecosystem models: the paradox of use for living marine resource management. Prog. Oceanogr. 102, 102–114. doi: 10.1016/j.pocean.2012.03.008
Little, A. S., Needle, C. L., Hilborn, R., Holland, D. S., and Marshall, C. T. (2015). Real-time spatial management approaches to reduce bycatch and discards: experiences from Europe and the United States. Fish Fish. 16, 576–602.
Litz, M., and Hughes, K. M. (2020). Willapa Bay Coho Forecast Methodology. Pacific Fishery Management Council Briefing Book, March 2020, Agenda Item E.2, Attachment 1. Available online at: https://www.pcouncil.org/documents/2020/02/e-2-supplemental-attachment-1-willapa-bay-coho-forecast-methodology-electronic-only.pdf/.
Mach, K. J., and Field, C. B. (2017). Toward the next generation of assessment. Annu. Rev. Environ. Resour. 42, 569–597.
Malick, M. J., Hunsicker, M. E., Haltuch, M. A., Parker-Stetter, S. L., Berger, A. M., and Marshall, K. N. (2020a). Relationships between temperature and Pacific hake distribution vary across latitude and life-history stage. Mar. Ecol. Prog. Ser. 639, 185–197.
Malick, M. J., Siedlecki, S. A., Norton, E. L., Kaplan, I. C., Haltuch, M. A., Hunsicker, M. E., et al. (2020b). Environmentally driven seasonal forecasts of Pacific hake distribution. Front. Mar. Sci. 7:578490. doi: 10.3389/fmars.2020.578490
Marmion, M., Parviainen, M., Luoto, M., Heikkinen, R. K., and Thuiller, W. (2009). Evaluation of consensus methods in predictive species distribution modelling. Divers. Distrib. 15, 59–69. doi: 10.1111/j.1472-4642.2008.00491.x
Marshall, K. N., Kaplan, I. C., Hodgson, E. E., Hermann, A., Busch, D. S., McElhany, P., et al. (2017). Risks of ocean acidification in the California Current food web and fisheries: ecosystem model projections. Glob. Chang. Biol. 23, 1525–1539. doi: 10.1111/gcb.13594
Marshall, K. N., Levin, P. S., Essington, T. E., Koehn, L. E., Anderson, L. G., Bundy, A., et al. (2018). Ecosystem-based fisheries management for social–ecological systems: renewing the focus in the United States with next generation fishery ecosystem plans. Conserv. Lett 11:e12367. doi: 10.1111/conl.12367
May, R. M., Beddington, J. R., Clark, C. W., Holt, S. J., and Laws, R. M. (1979). Management of multispecies fisheries. Science 205, 267–277. doi: 10.1126/science.205 4403.267
McCabe, R. M., Hickey, B. M., Kudela, R. M., Lefebvre, K. A., Adams, N. G., Bill, B. D., et al. (2016). An unprecedented coastwide toxic algal bloom linked to anomalous ocean conditions. Geophys. Res. Lett. 43, 10366–10376. doi: 10.1002/2016GL070023
McClatchie, S., Goericke, R., Auad, G., and Hill, K. (2010). Re-assessment of the stock–recruit and temperature–recruit relationships for Pacific sardine (Sardinops sagax). Can. J. Fish. Aquat. Sci. 67, 1782–1790. doi: 10.1139/F10-101
McClatchie, S., Vetter, R. D., and Hendy, I. L. (2018). Forage fish, small pelagic fisheries and recovering predators: managing expectations. Anim. Conserv. 21, 445–447. doi: 10.1111/acv.12421
Mölter, T., Schindler, D., Albrecht, A., and Kohnle, U. (2016). Review on the projections of future storminess over the North Atlantic European region. Atmosphere 7:60. doi: 10.3390/atmos7040060
Moore, K. M., Allison, E. H., Dreyer, S. J., Ekstrom, J. A., Jardine, S. L., Klinger, T., et al. (2020). Harmful algal blooms: identifying effective adaptive actions used in fishery-dependent communities in response to a protracted event. Front. Mar. Sci. 6:803. doi: 10.3389/fmars.2019.00803
Moore, S. K., Cline, M. R., Blair, K., Klinger, T., Varney, A., and Norman, K. (2019). An index of fisheries closures due to harmful algal blooms and a framework for identifying vulnerable fishing communities on the U.S. West Coast. Mar. Policy 110:103543. doi: 10.1016/j.marpol.2019.103543
Morley, J. W., Selden, R. L., Latour, R. J., Frölicher, T. L., Seagraves, R. J., Pinsky, M. L., et al. (2018). Projecting shifts in thermal habitat for 686 species on the North American continental shelf. PLoS One 13:e0196127. doi: 10.1371/journal.pone.0196127
Moullec, F., Barrier, N., Drira, S., Guilhaumon, F., Marsaleix, P., Somot, S., et al. (2019). An end-to-end model reveals losers and winners in a warming Mediterranean Sea. Front. Mar. Sci. 6:345. doi: 10.3389/fmars.2019.00345
Muhling, B. A., Brodie, S., Smith, J. A., Tommasi, D., Gaitan, C. F., Hazen, E. L., et al. (2020). Predictability of species distributions deteriorates under novel environmental conditions in the California Current System. Front. Mar. Sci. 7:589. doi: 10.3389/fmars.2020.00589
Muhling, B. A., Tommasi, D., Ohshimo, S., Alexander, M. A., and Dinardo, G. (2018). Regional-scale surface temperature variability allows prediction of Pacific bluefin tuna recruitment. ICES J. Mar. Sci. 75, 1341–1352. doi: 10.1093/icesjms/fsy017
Myers, R. A. (1998). When do environment–recruitment correlations work? Rev. Fish Biol. Fish. 8, 285–305. doi: 10.1023/A:1008828730759
National Marine Fisheries Service (2016a). Ecosystem-Based Fisheries Management Policy. National Marine Fisheries Service Policy 01-120, Vol. 9. Available online at: https://www.fisheries.noaa.gov/resource/document/ecosystem-based-fisheries-management-policy (accessed May 23, 2016).
National Marine Fisheries Service (2016b). Ecosystem-Based Fisheries Management Road Map. National Marine Fisheries Service Procedure 01-120-01, 50. Available online at: https://www.fisheries.noaa.gov/resource/document/ecosystem-based-fisheries-management-road-map (accessed November 17, 2016).
Neveu, E., Moore, A. M., Edwards, C. A., Fiechter, J., Drake, P., Crawford, W. J., et al. (2016). An historical analysis of the California Current circulation using ROMS 4D-Var: system configuration and diagnostics. Ocean Model. 99, 133–151. doi: 10.1016/j.ocemod.2015.11.012
Norberg, A., Abrego, N., Blanchet, F. G., Adler, F. R., Anderson, B. J., Anttila, J., et al. (2019). A comprehensive evaluation of predictive performance of 33 species distribution models at species and community levels. Ecol. Monogr. 89:e01370. doi: 10.1002/ecm.1370
Okamoto, D. K., Poe, M. R., Francis, T. B., Punt, A. E., Levin, P. S., Shelton, A. O., et al. (2020). Attending to spatial social–ecological sensitivities to improve trade-off analysis in natural resource management. Fish Fish. 21, 1–12. doi: 10.1111/faf.12409
O’Leary, C. A., Miller, T. J., Thorson, J. T., and Nye, J. A. (2018). Understanding historical summer flounder (Paralichthys dentatus) abundance patterns through the incorporation of oceanography-dependent vital rates in Bayesian hierarchical models. Can. J. Fish. Aquat. Sci. 76, 1275–1294. doi: 10.1139/cjfas-2018-0092
O’Leary, C. A., Thorson, J. T., Miller, T. J., and Nye, J. A. (2019). Comparison of multiple approaches to calculate time-varying biological reference points in climate-linked population-dynamics models. ICES J. Mar. Sci. 77, 930–941. doi: 10.1093/icesjms/fsz215
Ornes, S. (2018). How does climate change influence extreme weather? Impact attribution research seeks answers. Proc. Natl. Acad. Sci. U.S.A. 115, 8232–8235. doi: 10.1073/pnas.1811393115
Pacific Fishery Management Council (PFMC) (2008). “Council operating procedure 15: salmon estimation methodology updates and review,” in Pacific Fishery Management Council, Portland, OR, 2.
Pacific Fishery Management Council (PFMC) (2009). “Management of krill as an essential component of the california current ecosystem: amendment 12 to the coastal pelagic species fishery management plan,” in Pacific Fishery Management Council, Portland, OR, 112.
Pacific Fishery Management Council (PFMC) (2013). “Pacific coast fishery ecosystem plan for the U.S. portion of the California current large marine ecosystem,” in Pacific Fishery Management Council, Portland, OR, 190.
Pacific Fishery Management Council (PFMC) (2014). Report on the Atlantis Model Review. Agenda Item H.1. Available online at: https://www.pcouncil.org/documents/2014/11/h-ecosystem-based-management-november-2014.pdf/.
Pacific Fishery Management Council (PFMC) (2015a). Harvest Specifications and Management Measures for 2015-2016 and Biennial Periods Thereafter-Final Environmental Impact Statement. Available online at: https://www.pcouncil.org/documents/2015/01/2015-16-harvest-specifications-amendment-24-feis.pdf/.
Pacific Fishery Management Council (PFMC) (2015b). Scientific and Statistical Committee Statement on Fisheries Ecosystem Plan Initiative Scoping. Agenda Item D.1.a, Supplemental SSC Report. Available online at: https://www.pcouncil.org/documents/2015/09/agenda-item-d-1-a-supplemental-ssc-report.pdf/.
Pacific Fishery Management Council (PFMC) (2016a). “Agenda Item D.1: fishery ecosystem plan coordinated ecosystem indicator review initiative,” in 236th Session of the Pacific Fishery Management Council. Held: September 12-20, 2016, Boise, ID.
Pacific Fishery Management Council (PFMC) (2016b). “Agenda Item D.2: update on coordinated ecosystem indicator review initiative,” in 233rd Session of the Pacific Fishery Management Council. Held: March 8-14, 2016, Sacramento, CA.
Pacific Fishery Management Council (PFMC) (2017a). “Ecosystem initiatives appendix to the pacific coast fishery ecosystem Plan for the U.S,” in Portion of the California Current Large Marine Ecosystem. Pacific Fishery Management Council, Portland, OR, 20.
Pacific Fishery Management Council (PFMC) (2017b). Scientific and Statistical Committee Report on Future Council Meeting Agenda and Workload Planning. Agenda Item G.5.a, Supplemental SSC Report1. Available online at: https://www.pcouncil.org/documents/2017/11/agenda-item-g-5-a-supplemental-ssc-report-1-2.pdf.
Pacific Fishery Management Council (PFMC) (2017c). Scientific and Statistical Committee Report on the Review of 2016 Fisheries and Summary of 2017 Stock Abundance Forecasts. Agenda Item E.2.a, Supplemental SSC Report. Available online at: https://www.pcouncil.org/documents/2017/03/e2a_sup_ssc_rpt_mar2017bb.pdf/.
Pacific Fishery Management Council (PFMC) (2018). Research and Data Needs. Portland, OR: Pacific Fishery Management Council, 226.
Pacific Fishery Management Council (PFMC) (2020). Status of the Pacific Coast Coastal Pelagic Species Fishery and Recommended Acceptable Biological Catches. Stock Assessment and Fishery Evaluation for 2019. Available online at: https://www.pcouncil.org/documents/2020/10/2019-cps-safe-june-2020.pdf/.
Park, J.-Y., Stock, C. A., Dunne, J. P., Yang, X., and Rosati, A. (2019). Seasonal to Multiannual Marine Ecosystem Prediction With a Global Earth System Model. Science 365, 284–288. doi: 10.1126/science.aav6634
Patrick, W. S., and Link, J. S. (2015). Myths that continue to impede progress in ecosystem-based fisheries management. Fish 40, 155–160.
Payne, M. R., Hobday, A. J., MacKenzie, B. R., Tommasi, D., Dempsey, D. P., Fässler, S. M., et al. (2017). Lessons from the first generation of marine ecological forecast products. Front. Mar. Sci. 4:289.
Pearcy, W. G. (1992). Ocean Ecology of North Pacific Salmonids. Seattle: Washington Sea Grant Program.
Peterman, R. M. (1982). Model of salmon age structure and its use in preseason forecasting and studies of marine survival. Can. J. Fish. Aquat. Sci. 39, 1444–1452. doi: 10.1139/f82-195
Petesch, T., and Pfeiffer, L. (2019). Impacts of rationalization on exposure to high winds in Alaska’s Crab Fisheries. J. Agromed. 24, 364–373. doi: 10.1080/1059924X.2019.1646683
Petursdottir, G., Hannibalsson, O., and Turner, J. M. M. (2001). Safety at Sea as an Integral Part of Fisheries Management. FAO Fisheries Circular 966. Rome: Food and Agriculture Organization of the United Nations.
Pfeiffer, L. (2020). How storms affect fishers’ decisions about going to sea. ICES J. Mar. Sci. 77, 2753–2762.
Pfeiffer, L., and Gratz, T. (2016). The effect of rights-based fisheries management on risk taking and fishing safety. Proc. Natl. Acad. Sci. U.S.A. 113, 2615–2620. doi: 10.1073/pnas.1509456113
Pikitch, E. K., Boersma, P. D., Boyd, I. L., Conover, D. O., et al. (2012). “Little fish, big impact: managing a crucial link in ocean food webs,” in Lenfest Ocean Program, Washington, DC.
Pikitch, E. K., Santora, C., Babcock, E. A., Bakun, A., Bonfil, R., Conover, D. O., et al. (2004). Ecosystem-based fishery management. Science 305, 346–347.
Plagányi, ÉE., Punt, A. E., Hillary, R., Morello, E. B., Thébaud, O., Hutton, T., et al. (2014). Multispecies fisheries management and conservation: tactical applications using models of intermediate complexity. Fish Fish. 15, 1–22. doi: 10.1111/j.1467-2979.2012.00488.x
Plagányi, ÉE., van Putten, I., Hutton, T., Deng, R. A., Dennis, D., Pascoe, S., et al. (2013). Integrating indigenous livelihood and lifestyle objectives in managing a natural resource. Proc. Natl. Acad. Sci. U.S.A. 110, 3639–3644.
Punt, A. E., Butterworth, D. S., de Moor, C. L., De Oliveira, J. A. A., and Haddon, M. (2016a). Management strategy evaluation: best practices. Fish Fish. 17, 303–334. doi: 10.1111/faf.12104
Punt, A. E., MacCall, A. D., Essington, T. E., Francis, T. B., Hurtado-Ferro, F., Johnson, K. F., et al. (2016b). Exploring the implications of the harvest control rule for Pacific sardine, accounting for predator dynamics: a MICE model. Ecol. Modell. 337, 79–95. doi: 10.1016/j.ecolmodel.2016.06.004
Roberts, J. J., Best, B. D., Mannocci, L., Fujioka, E., Halpin, P. N., Palka, D. L., et al. (2016). Habitat-based cetacean density models for the U.S. Atlantic and Gulf of Mexico. Sci. Rep. 6:22615. doi: 10.1038/srep22615
Robinson, N. M., Nelson, W. A., Costello, M. J., Sutherland, J. E., and Lundquist, C. J. (2017). A systematic review of marine-based species distribution models (SDMs) with recommendations for best practice. Front. Mar. Sci. 4:421. doi: 10.3389/fmars.2017.00421
Rogers, L. A., Griffin, R., Young, T., Fuller, E., St. Martin, K., and Pinsky, M. L. (2019). Shifting habitats expose fishing communities to risk under climate change. Nat. Clim. Chang 9, 512–516. doi: 10.1038/s41558-019-0503-z
Rupp, D. E., Wainwright, T. C., Lawson, P. W., and Peterson, W. T. (2012). Marine environment-based forecasting of coho salmon (Oncorhynchus kisutch) adult recruitment. Fish. Oceanogr. 21, 1–19. doi: 10.1111/j.1365-2419.2011.00605.x
Ryan, J. P., Kudela, R. M., Birch, J. M., Blum, M., Bowers, H. A., Chavez, F. P., et al. (2017). Causality of an extreme harmful algal bloom in Monterey Bay, California, during the 2014–2016 northeast Pacific warm anomaly. Geophys. Res. Lett. 44, 5571–5579. doi: 10.1002/2017GL072637
Sainsbury, N. C., Genner, M. J., Saville, G. R., Pinnegar, J. K., O’Neill, C. K., Simpson, S. D., et al. (2018). Changing storminess and global capture fisheries. Nat. Clim. Chang. 8, 655–659. doi: 10.1038/s41558-018-0206-x
Santora, J. A., Mantua, N. J., Schroeder, I. D., Field, J. C., Hazen, E. L., Bograd, S. J., et al. (2020). Habitat compression and ecosystem shifts as potential links between marine heatwave and record whale entanglements. Nat. Commun. 11:536. doi: 10.1038/s41467-019-14215-w
Satterthwaite, W. H., Andrews, K. S., Burke, B. J., Gosselin, J. L., Greene, C. M., Harvey, C. J., et al. (2020). Ecological thresholds in forecast performance for key United States West Coast Chinook salmon stocks. ICES J. Mar. Sci. 77, 1503–1515. doi: 10.1093/icesjms/fsz189
Savelli, S., and Joslyn, S. (2012). Boater safety: communicating weather forecast information to high-stakes end users. Weather. Clim. Soc. 4, 7–19. doi: 10.1175/WCAS-D-11-00025.1
Schroeder, I. D., Santora, J. A., Bograd, S. J., Hazen, E. L., Sakuma, K. M., Moore, A. M., et al. (2018). Source water variability as a driver of rockfish recruitment in the California Current Ecosystem: implications for climate change and fisheries management. Can. J. Fish. Aquat. Sci. 76, 950–960. doi: 10.1139/cjfas-2017-0480
Schuwirth, N., Borgwardt, F., Domisch, S., Friedrichs, M., Kattwinkel, M., Kneis, D., et al. (2019). How to make ecological models useful for environmental management. Ecol. Modell. 411:108784.
Selden, R. L., Thorson, J. T., Samhouri, J. F., Bograd, S. J., Brodie, S., Carroll, G., et al. (2019). Coupled changes in biomass and distribution drive trends in availability of fish stocks to US West Coast ports. ICES J. Mar. Sci. 77, 188–199. doi: 10.1093/icesjms/fsz211
Shelton, A. O., Sullaway, G. H., Ward, E. J., Feist, B. E., Somers, K. A., et al. (2020). Redistribution of salmon populations in the northeast Pacific ocean in response to climate. Fish Fish. 22, 503–517. doi: 10.1111/faf.12530
Siedlecki, S. A., Kaplan, I. C., Hermann, A. J., Nguyen, T. T., Bond, N. A., Newton, J. A., et al. (2016). Experiments with Seasonal Forecasts of ocean conditions for the Northern region of the California Current upwelling system. Sci. Rep. 6:27203. doi: 10.1038/srep27203
Skern-Mauritzen, M., Olsen, E., and Huse, G. (2018). Opportunities for advancing ecosystem-based management in a rapidly changing, high latitude ecosystem. ICES J. Mar. Sci. 75, 2425–2433. doi: 10.1093/icesjms/fsy150
Skern-Mauritzen, M., Ottersen, G., Handegard, N. O., Huse, G., Dingsør, G. E., Stenseth, N. C., et al. (2016). Ecosystem processes are rarely included in tactical fisheries management. Fish Fish. 17, 165–175. doi: 10.1111/faf.12111
Smith, A. D. M., Brown, C. J., Bulman, C. M., Fulton, E. A., Johnson, P., Kaplan, I., et al. (2011). Impacts of fishing low-trophic level species on marine ecosystems. Science 333, 1147–1150.
Smith, J. A., Tommasi, D., Sweeney, J., Brodie, S., Welch, H., Hazen, E. L., et al. (2020). Lost opportunity: quantifying the dynamic economic impact of time-area fishery closures. J. Appl. Ecol. 57, 502–513. doi: 10.1111/1365-2664.13565
Smith, J. A., Muhling, B., Sweeney, J., Tommasi, D., Pozo Buil, M., Fiechter, J., et al. (2021). The potential impact of a shifting Pacific sardine distribution on U.S. West Coast landings. Fish. Oceanogr. doi: 10.1111/fog.12529
Stanton, J. C., Pearson, R. G., Horning, N., Ersts, P., and Reşit Akçakaya, H. (2012). Combining static and dynamic variables in species distribution models under climate change. Methods Ecol. Evol. 3, 349–357. doi: 10.1111/j.2041-210X.2011.00157.x
Staton, B. A., and Catalano, M. J. (2019). Bayesian information updating procedures for Pacific salmon run size indicators: evaluation in the presence and absence of auxiliary migration timing information. Can. J. Fish. Aquat. Sci. 76, 1719–1727. doi: 10.1139/cjfas-2018-0176
Stock, C. A., Pegion, K., Vecchi, G. A., Alexander, M. A., Tommasi, D., Bond, N. A., et al. (2015). Seasonal sea surface temperature anomaly prediction for coastal ecosystems. Prog. Oceanogr. 137, 219–236. doi: 10.1016/j.pocean.2015.06.007
Swain, D. L., Langenbrunner, B., Neelin, J. D., and Hall, A. (2018). Increasing precipitation volatility in twenty-first-century California. Nat. Clim. Chang. 8, 427–433. doi: 10.1038/s41558-018-0140-y
Teich, T., Groll, N., and Weisse, R. (2018). Long-term statistics of potentially hazardous sea states in the North Sea 1958–2014. Ocean Dyn. 68, 1559–1570. doi: 10.1007/s10236-018-1210-4
Thorson, J. T. (2019a). Forecast skill for predicting distribution shifts: a retrospective experiment for marine fishes in the Eastern Bering Sea. Fish Fish. 20, 159–173. doi: 10.1111/faf.12330
Thorson, J. T. (2019b). Guidance for decisions using the Vector Autoregressive Spatio-Temporal (VAST) package in stock, ecosystem, habitat and climate assessments. Fish. Res. 210, 143–161. doi: 10.1016/j.fishres.2018.10.013
Thorson, J. T., Pinsky, M. L., and Ward, E. J. (2016). Model-based inference for estimating shifts in species distribution, area occupied and centre of gravity. Methods Ecol. Evol. 7, 990–1002. doi: 10.1111/2041-210X.12567
Tolimieri, N., Haltuch, M. A., Lee, Q., Jacox, M. G., and Bograd, S. J. (2018). Oceanographic drivers of sablefish recruitment in the California Current. Fish. Oceanogr. 27, 458–474. doi: 10.1111/fog.12266
Tommasi, D., Stock, C. A., Alexander, M. A., Yang, X., Rosati, A., and Vecchi, G. A. (2017a). Multi-annual Climate predictions for fisheries: an assessment of skill of sea surface temperature forecasts for large marine ecosystems. Front. Mar. Sci. 4:201. doi: 10.3389/fmars.2017.00201
Tommasi, D., Stock, C. A., Hobday, A. J., Methot, R., Kaplan, I. C., Eveson, J. P., et al. (2017b). Managing living marine resources in a dynamic environment: the role of seasonal to decadal climate forecasts. Prog. Oceanogr. 152, 15–49. doi: 10.1016/j.pocean.2016.12.011
Tommasi, D., Stock, C. A., Pegion, K., Vecchi, G. A., Methot, R. D., Alexander, M. A., et al. (2017c). Improved management of small pelagic fisheries through seasonal climate prediction. Ecol. Appl. 27, 378–388. doi: 10.1002/eap.1458
Townsend, H., Aydin, K., Holsman, K., Harvey, C., Kaplan, I., Hazen, E., et al. (2017). Report of the 4th National Ecosystem Modeling Workshop (NEMoW 4): Using Ecosystem Models to Evaluate Inevitable Trade-offs. Washington, D.C: U.S. Department of Commerce. NOAA Tech. Memo. NMFS-F/SPO-173.
Townsend, H., Harvey, C. J., deReynier, Y., Davis, D., Zador, S. G., Gaichas, S., et al. (2019). Progress on implementing ecosystem-based fisheries management in the United States Through the use of ecosystem models and analysis. Front. Mar. Sci. 6:641. doi: 10.3389/fmars.2019.00641
Townsend, H. M., Harvey, C., Aydin, K. Y., Gamble, R., Grüss, A., Levin, P. S., et al. (2014). Report of the 3rd National Ecosystem Modeling Workshop (NEMoW 3): Mingling Models for Marine Resource Management – Multiple Model Inference. Washington, D.C: U.S. Department of Commerce. NOAA Technical Memorandum NMFS-F/SPO-149.
Townsend, H. M., Link, J. S., Osgood, K. E., Gedamke, T., Watters, G. M., Polovina, J. J., et al. (2008). National Marine Fisheries Service Report of the National Ecosystem Modeling Workshop (NEMoW). Washington, D.C: U.S. Department of Commerce. NOAA Technical Memorandum NMFS-F/SPO-87, 93.
Valavanis, V. D., Pierce, G. J., Zuur, A. F., Palialexis, A., Saveliev, A., Katara, I., et al. (2008). Modelling of essential fish habitat based on remote sensing, spatial analysis and GIS. Hydrobiologia 612, 5–20. doi: 10.1007/s10750-008-9493-y
Valinia, S., Englund, G., Moldan, F., Futter, M. N., Köhler, S. J., Bishop, K., et al. (2014). Assessing anthropogenic impact on boreal lakes with historical fish species distribution data and hydrogeochemical modeling. Glob. Change Biol. 20, 2752–2764. doi: 10.1111/gcb.12527
Walters, C. J., and Collie, J. S. (1988). Is research on environmental factors useful to fisheries management? J. Fish. Aquat. Sci. 45, 1848–1854.
Watson, J. R., Fuller, E. C., Castruccio, F. S., and Samhouri, J. F. (2018). Fishermen follow fine-scale physical ocean features for finance. Front. Mar. Sci. 5:46. doi: 10.3389/fmars.2018.00046
Weijerman, M., Fulton, E. A., Janssen, A. B. G., Kuiper, J. J., Leemans, R., Robson, B. J., et al. (2015). How models can support ecosystem-based management of coral reefs. Prog. Oceanogr. 138, 559–570.
Weijerman, M., Link, J. S., Fulton, E. A., Olsen, E., Townsend, H., Gaichas, S., et al. (2016). Atlantis ecosystem model summit: report from a workshop. Ecol. Modell. 335, 35–38. doi: 10.1016/j.ecolmodel.2016.05.007
Welch, H., Hazen, E. L., Bograd, S. J., Jacox, M. G., Brodie, S., Robinson, D., et al. (2019). Practical considerations for operationalizing dynamic management tools. J. Appl. Ecol. 56, 459–469. doi: 10.1111/1365-2664.13281
Wells, B. K., Huff, D. D., Burke, B. J., Brodeur, R. D., Santora, J. A., Field, J. C., et al. (2020). Implementing ecosystem-based management principles in the design of a salmon ocean ecology program. Front. Mar. Sci. 7:342. doi: 10.3389/fmars.2020.00342
Wells, B. K., Santora, J. A., Henderson, M. J., Warzybok, P., Jahncke, J., Bradley, R. W., et al. (2017). Environmental conditions and prey-switching by a seabird predator impact juvenile salmon survival. J. Mar. Syst. 174, 54–63. doi: 10.1016/j.jmarsys.2017.05.008
Wells, B. K., Schroeder, I. D., Santora, J. A., Hazen, E. L., Bograd, S. J., Bjorkstedt, E. P., et al. (2013). State of the California Current 2012-2013: no such thing as an ‘average’ year. Calif. Coop 54, 37–71.
Zwolinski, J. P., and Demer, D. A. (2019). Re-evaluation of the environmental dependence of Pacific sardine recruitment. Fish. Res. 216, 120–125. doi: 10.1016/j.fishres.2019.03.022
Keywords: ecosystem-based fisheries management, ecosystem modeling, fisheries science, fisheries management, natural resource management
Citation: Tommasi D, deReynier Y, Townsend H, Harvey CJ, Satterthwaite WH, Marshall KN, Kaplan IC, Brodie S, Field JC, Hazen EL, Koenigstein S, Lindsay J, Moore K, Muhling B, Pfeiffer L, Smith JA, Sweeney J, Wells B and Jacox MG (2021) A Case Study in Connecting Fisheries Management Challenges With Models and Analysis to Support Ecosystem-Based Management in the California Current Ecosystem. Front. Mar. Sci. 8:624161. doi: 10.3389/fmars.2021.624161
Received: 30 October 2020; Accepted: 31 May 2021;
Published: 30 June 2021.
Edited by:
Michael Arthur St. John, Technical University of Denmark, DenmarkReviewed by:
Geir Huse, Norwegian Institute of Marine Research (IMR), NorwayCopyright © 2021 Tommasi, deReynier, Townsend, Harvey, Satterthwaite, Marshall, Kaplan, Brodie, Field, Hazen, Koenigstein, Lindsay, Moore, Muhling, Pfeiffer, Smith, Sweeney, Wells and Jacox. This is an open-access article distributed under the terms of the Creative Commons Attribution License (CC BY). The use, distribution or reproduction in other forums is permitted, provided the original author(s) and the copyright owner(s) are credited and that the original publication in this journal is cited, in accordance with accepted academic practice. No use, distribution or reproduction is permitted which does not comply with these terms.
*Correspondence: Desiree Tommasi, ZGVzaXJlZS50b21tYXNpQG5vYWEuZ292
Disclaimer: All claims expressed in this article are solely those of the authors and do not necessarily represent those of their affiliated organizations, or those of the publisher, the editors and the reviewers. Any product that may be evaluated in this article or claim that may be made by its manufacturer is not guaranteed or endorsed by the publisher.
Research integrity at Frontiers
Learn more about the work of our research integrity team to safeguard the quality of each article we publish.