- 1CRETUS-Department of Applied Economics, University of Santiago de Compostela, Santiago de Compostela, Spain
- 2Campus Do∗Mar-International Campus of Excellence, Vigo, Spain
- 3Marine Conservation Cambodia, Koh Ach Seh, Cambodia
- 4Plymouth Marine Laboratory, Plymouth, United Kingdom
Fishing activities continue to decimate populations of marine mammals, fish, and their habitats in the coastal waters of the Kep Archipelago, a cluster of tropical islands on the Cambodia-Vietnam border. In 2019, the area was recognized as an Important Marine Mammal Area, largely owing to the significant presence of Irrawaddy dolphins (Orcaella brevirostris). Understanding habitat preferences and distribution aids in the identification of areas to target for monitoring and conservation, which is particularly challenging in data-limited nations of Southeast Asia. Here, we test the hypothesis that accurate seasonal habitat models, relying on environmental data and species occurrences alone, can be used to describe the ecological processes governing abundance for the resident dolphin population of the Kep Archipelago, Cambodia. Leveraging two years of species and oceanographic data—depth, slope, distance to shore and rivers, sea surface temperature, and chlorophyll-a concentration—we built temporally stratified models to estimate distribution and infer seasonal habitat importance. Overall, Irrawaddy dolphins of Kep displayed habitat preferences similar to other populations, and were predominately encountered in three situations: (1) water depths ranging from 3.0 to 5.3 m, (2) surface water temperatures of 27–32°C, and (3) in close proximity to offshore islands (< 7.5 km). With respect to seasonality, statistical tests detected significant differences for all environment variables considered except seafloor slope. Four predictor sets, each with a unique combination of variables, were used to map seasonal variation in dolphin habitat suitability. Models with highest variable importance scores were water depth, pre- and during monsoon season (61–62%), and sea surface temperature, post-monsoon (71%), which suggests that greater freshwater flow during the wet season may alter primary productivity and dolphin prey abundance. Importantly, findings show the majority of areas with highest habitat suitability are not currently surveyed for dolphins and located outside Kep’s Marine Fisheries Management Area. This research confirms the need to expand monitoring to new areas where high-impact fisheries and other human activities operate. Baseline knowledge on dolphin distribution can guide regional conservation efforts by taking into account the seasonality of the species and support the design of tailored management strategies that address transboundary threats to an Important Marine Mammal Area.
Introduction
The globally endangered Irrawaddy dolphin (Orcaella brevirostris) is distributed in fragmented populations throughout the coastal, estuarine, and freshwater environments of Southeast Asia (Perrin et al., 1995, 2005; Hines et al., 2015). The species faces numerous anthropogenic threats throughout its range, including fisheries bycatch, habitat degradation and marine pollution (Reeves et al., 2003; Kannan et al., 2005; Jaaman et al., 2009). Irrawaddy dolphins are the only confirmed cetacean species to inhabit the coastal waters of the Cambodia-Vietnam border region (Beasley and Davidson, 2007; Minton et al., 2017; Tubbs et al., 2020), which includes the Kep Archipelago, Cambodia. Illegal, Unreported, and Unregulated (IUU) fishing is a daily threat to dolphins and the many species of coral, fish, and invertebrates that support local fisheries and tourism (Beasley and Davidson, 2007; Böhm, 2019; Tubbs et al., 2019). Despite regional conservation efforts, no Irrawaddy dolphin specific conservation plans are in place. A more detailed understanding of dolphin habitat distribution could support the establishment of tailored conservation measures for the Kep Archipelago as well as have broader implications for the management of Irrawaddy dolphins throughout their range.
Cetacean distribution patterns are governed by a combination of biotic and abiotic factors and the ability for species to access eco-geographical suitable locations (Baumgartner et al., 2001; O’Donoghue et al., 2010; Peterson, 2011). For Irrawaddy dolphins, previous studies indicate they inhabit shallow (2–15 m), warm (24–31°C), nearshore waters (1–20 km from the coast), of varying salinity (2–35 ppt), and in close proximity to river mouths (< 11 km; Dolar et al., 2002; Peter et al., 2016a; Kuit et al., 2019). These waters are likely to support the small fish, crustacean, and cephalopod species that Irrawaddy dolphins predate upon (Stacey and Leatherwood, 1997; Ponnampalam et al., 2013; Jackson-Ricketts et al., 2019). In the Eastern Gulf of Thailand, intense summer monsoonal rainfall precedes a period of high freshwater flows to coastal river mouths (Tsujimoto et al., 2018). The resulting changes in water depths and primary productivity have been shown to alter coastal dolphin prey abundance and the distribution of their habitats throughout the year (Hastie et al., 2005; Bearzi et al., 2008; McCluskey et al., 2016; Sprogis et al., 2017). The coastal-marine areas in the vicinity of the Kep Archipelago are also subject to seasonal high flow events, which present an opportunity to identify and distinguish different oceanographic factors that explain seasonal variation in Irrawaddy dolphin habitat.
Species Distribution Models (SDMs), also known as habitat models, link species observations to environmental variables (Briscoe et al., 2014; Gilles et al., 2016; Becker et al., 2017), and are becoming an increasingly common conservation planning tool (Cañadas et al., 2005; Bailey and Thompson, 2009; Hammond et al., 2013). For example, SDMs have been used to identify critical habitat (Redfern et al., 2006; Gregr et al., 2013), predict responses to environmental changes (Silber et al., 2017), and assess overlap in area usage between species and fisheries (Feist et al., 2015; Giralt Paradell et al., 2019). Nevertheless, there are practical considerations for applying correlative SDMs, including limits to their accuracy for differentiating presences from absences, the reliability of predictions (Liu et al., 2009), along with pitfalls when extrapolating outside the environment used to train a model (Elith et al., 2010). For applications that produce several habitat-selection models in one area, the environmental variables found to be significant predictors of distribution often differ between species (Garaffo et al., 2011), providing baseline knowledge on subtle habitat characteristics of sympatric cetacean species (Tobeña et al., 2016; Kuit et al., 2019). There are fewer examples, however, of multiple SDMs developed for one species that illuminate seasonal patterns of distribution, density, or behavior (Daura-Jorge et al., 2005; Campbell et al., 2015; Verutes et al., 2020).
The Cambodian Marine Mammal Conservation Project (CMMCP) was launched in 2017 by the Non-Governmental Organization Marine Conservation Cambodia (MCC) to support the conservation of Cambodian marine mammals through research and education (Tubbs et al., 2019). Boat surveys conducted by CMMCP in the Kep Archipelago between October 2017 and September 2019 revealed that Irrawaddy dolphins were present year-round, with seasonal variation in encounter rates and distribution (Tubbs et al., 2020). Tubbs et al. (2020) also identified the need to further investigate different factors affecting Irrawaddy dolphin seasonal habitat selection, including prey abundance and other explanatory variables of seasonal distribution related to freshwater input. Previous SDMs developed for Irrawaddy dolphins have shown depth to be the strongest predictor of their distribution (Smith et al., 2008; Jackson-Ricketts et al., 2020), followed by salinity (Smith et al., 2008). Smith et al. (2009) produced two SDMs for coastal Irrawaddy dolphins in Bangladesh, showing that during the season of “high [river] flow”, their distribution was dependent on low salinity and depths, and during the season of “low [river] flow” their distribution was dependent on high surface water temperatures and depths.
The current study uses CMMCP’s presence-only sightings, in combination with publicly accessible environmental data layers derived from earth observation techniques, to produce four distinct seasonal SDMs. We hypothesize that seasonal variation in habitat use by Irrawaddy dolphins of the Kep Archipelago differs from other marine populations in Southeast Asia due to local environmental conditions. Specifically, with increasing freshwater input following the summer monsoon, we expect the distribution of Kep’s Irrawaddy dolphins to expand further offshore and away from major river mouths. We test whether biophysical variables commonly associated with small cetaceans can be used to construct accurate models that describe the ecological processes governing Irrawaddy dolphin habitat selection and predict seasonal distribution of this freshwater-dependent species (Smith et al., 2009). This research aims to achieve three goals that relate to enhancing baseline knowledge of the coastal Irrawaddy dolphin population inhabiting waters of the Kep Archipelago, Cambodia: (1) to characterize their spatial distribution; (2) to map variation in seasonal occurrences and habitat preferences; and (3) to estimate the population’s ecological niche based on the influence of oceanographic variables (i.e., depth, slope, proximity to shore and river mouths, sea surface temperature, and chlorophyll-a concentration) on predicted probability of distribution. We intend to inform spatial plans for managing high conflict areas between small cetaceans and human activities, particularly IUU fishing. As the first Irrawaddy dolphin habitat modeling study at the Cambodian-Vietnamese border region, knowledge gained will support the design of transboundary conservation measures and augment the understanding of coastal dolphin habitat selection on a wider scale.
Materials and Methods
Study Area
The study area is comprised of the coastal waters of Cambodia’s Kep and Kampot provinces and Vietnam’s Kien Giang Biosphere Reserve, on the eastern coast of the Gulf of Thailand (2,112 km2; Figure 1). The region receives freshwater input from two sources, the Kampot River from the northwest, and Giang River to the east. Here, 13 Cambodian islands, known as the Kep Archipelago, are separated from the 16 Vietnamese Pirate islands, by the maritime border. The waters are shallow, ranging from 2 to 30 m, and support coral, mangrove and seagrass habitats (Reid et al., 2019). The archipelago is at the heart of the Kien Giang–Kep Archipelago Important Marine Mammal Area (IMMA; IUCN-MMPATF, 2019), containing critical habitat for the survival of Irrawaddy dolphins, which are frequently sighted in the area (Vu et al., 2017; Tubbs et al., 2019). Inside this IMMA, is the Kep Marine Fisheries Management Area (MFMA), Cambodia’s equivalent of a Marine Protected Area (Boon et al., 2014), which delineates conservation zones for the purposes of reducing maritime conflicts, controlling tourism activities, and protecting fish stocks, habitats and the breeding grounds of vulnerable species such as the Irrawaddy dolphin (Marine Conservation Cambodia [MCC], 2016).
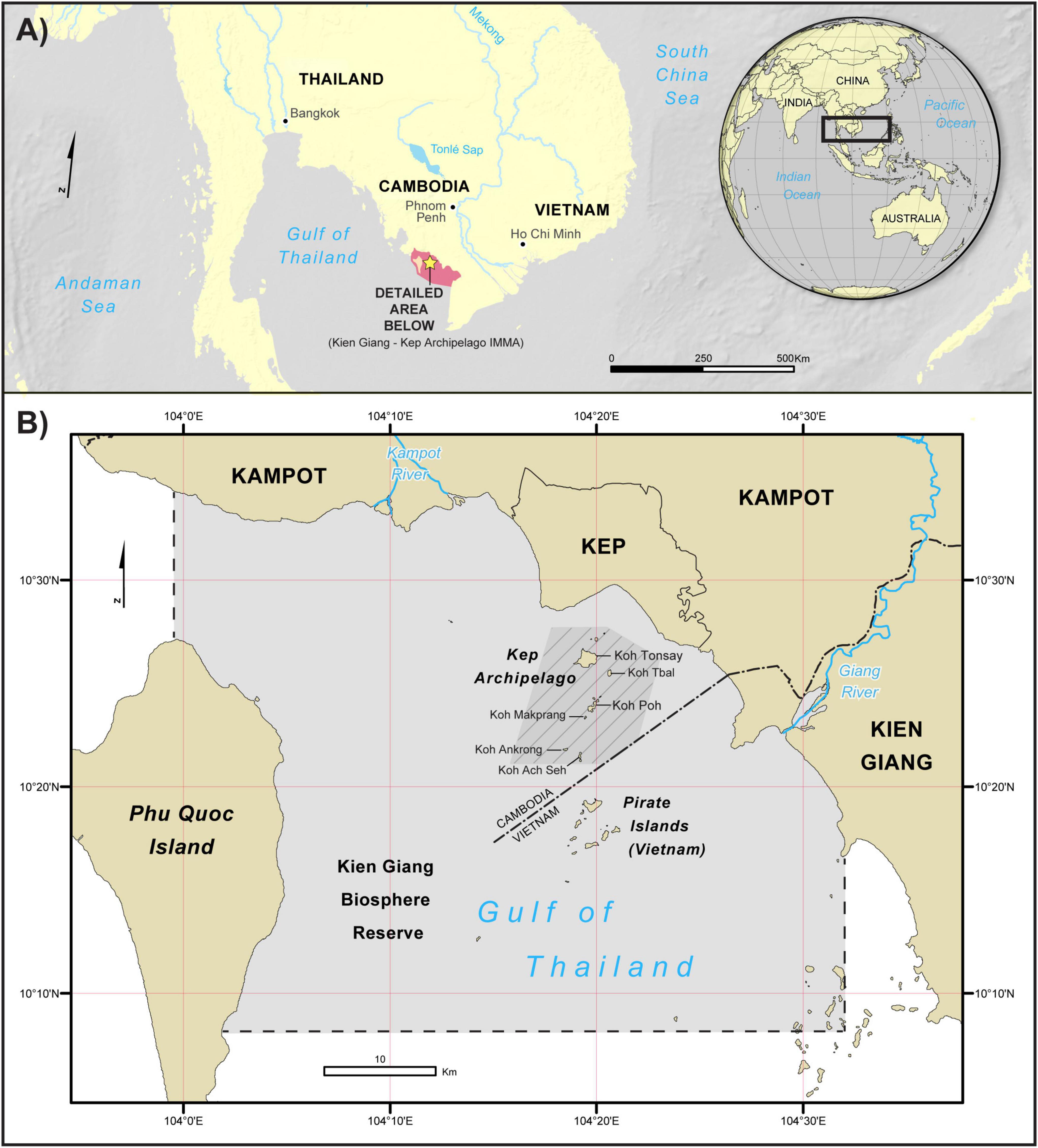
Figure 1. The study area: (A) situated in the Eastern Gulf of Thailand, including the Kien Giang-Kep Archipelago Important Marine Mammal Area (red color) and (B) The riverine inputs to the Kep Archipelago (Kampot and Giang Rivers). Light gray area indicates the extent of physiographic and remotely sensed environmental data. The darker area with gray hatching is the current extent of the 2018 Kep Marine Fisheries Management Area.
Boat Surveys
We utilized two years of Irrawaddy dolphin sightings data collected by CMMCP between October 2017 and September 2019 (average of 2.6 surveys/month ± SD 1.7). Surveys lasted between 3 and 5 h and followed one of three routes: route one followed a triangular-shaped track around the islands of the Kep Archipelago, bound by the maritime border and water depth; route two traveled between Koh Ach Seh and Kampot, on dive expeditions conducted by MCC; and route three traveled between Koh Ach Seh and Kep town on an MCC supply trip (Figure 2). Routes one and two took place on a converted Seine net vessel with a 200 HP inboard engine and a viewing platform 3.8 m above sea level, while route three took place on a converted trawling boat with a 120 HP inboard engine and a viewing platform 1.5 m above sea level. Vessels traveled at an average speed of 4 knots, with routes tracked using a Garmin 64s GPS.
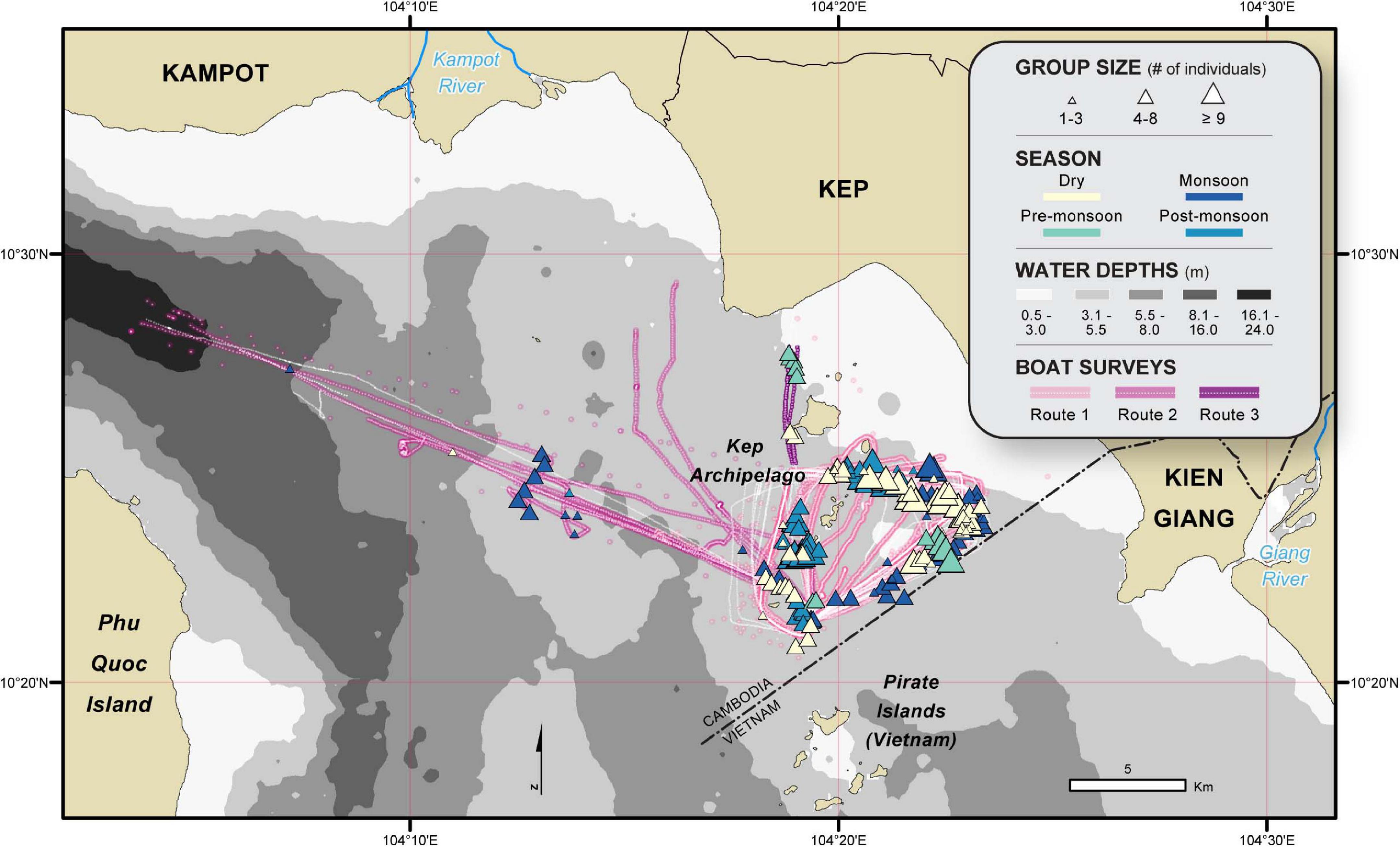
Figure 2. Irrawaddy dolphin occurrences recorded during the 2-year survey period (October 2017 through September 2019). The color of each triangle indicates the season of sighting and graduated symbols denote group size. White dotted lines with underlain colors show the different routes taken during boat surveys.
A team of five trained observers were on board each vessel. Three observers scanned the sea with binoculars in search of dolphin groups, while two rested. Observers rotated roles every 10 min to avoid fatigue effects. Environmental conditions were recorded every hour, or sooner, with surveys only taking place when the Beaufort sea state ≤ 3. For each sighting of one dolphin or group, defined as a collection of individuals with coordinated behavior (Connor et al., 2006), the time, group size, angle of the group from north and distance of the group from the vessel were recorded. Sighting data preparation included attaining dolphin group locations using the vessel’s GPS location, the distance of the group from the vessel, and bearing angle from north using trigonometric identities (Pythagoras, sine, and cosine rules).
Environmental Variables
A number of environmental factors influence cetacean distribution, and Irrawaddy dolphins are no different (Minton et al., 2011; Hines et al., 2020; Jackson-Ricketts et al., 2020). First, we acquired and prepared static oceanographic data about water depths, seafloor slope, and riverine input locations using QGIS v3.4 (QGIS Development Team, 2018). Shoreline distribution (mainland and offshore islands) for Cambodia and Vietnam were provided by Marine Conservation Cambodia and verified with data from the GADM v2 database1 and OpenStreetMap project2. The locations of riverine inputs from major distributaries of the Mekong Delta (Kampot and Giang Rivers) were digitized using satellite imagery. United States nautical chart, Dao Phu Quoc and Approaches to Kampot (HO3146), was georeferenced and digitized for a total of 1,727 depth soundings. Then, a continuous bathymetric surface was created using Inverse Distance Weighting (IDW) interpolation of the samples. Distance to shore and major river mouths were also calculated in GIS to compute the least cost path following a surface of marine grid cells. To minimize correlation among predictor variables, a variable inflation factor (VIF) measure was used to assess how much each independent variable is influenced by its interaction with other candidate variables. Both distance to shore measurements (all landmasses and offshore islands only) had VIF scores greater than 4, an indication of multicollinearity (Kutner et al., 2005). The distance to offshore islands variable was retained because its VIF dropped below 4 after distance to landmass was removed.
To investigate the role of varying environmental conditions during the year that influence Irrawaddy dolphin habitat selection, Plymouth Marine Laboratory processed grids of two environmental variables, chlorophyll-a concentration (Chl-a) and sea surface temperature (SST). Chl-a (mg/m3) was acquired from the Copernicus Sentinel-3 Ocean and Land Color Instrument (OLCI) at 300 m spatial resolution. SST (°C) was extracted from NOAA’s Advanced Very High Resolution Radiometer (AVHRR) at 1.1 km resolution. For both Chl-a and SST, we took all available satellite passes, applied the recommended quality masking3, and combined them as monthly median composites to reduce the effect of contaminated pixels (Miller et al., 1997). Sea surface salinity data, from the NASA Soil Moisture Active Passive (SMAP) observatory4, was not usable due to performance issues associated with land surface reflectance near the Kep offshore islands. Following the methods of Tobeña et al. (2016), we extracted values at each dolphin sighting location and, when applicable, the corresponding monthly composite for geospatial layers: depth (m); seafloor slope (degrees); distance to shore (km), time-lagged chlorophyll-a concentration for one and 2 months prior to sighting [Chl-a(-1 m), Chl-a(-2 m)], local variation of chlorophyll-a concentration (V-Chl-a; calculated as standard deviation within a 2 km focal radius); and local variation of sea surface temperature (V-SST; calculated as standard deviation within a 3 km focal radius).
Seasonal Habitat Models
To capture the spatial dimension of Irrawaddy dolphin habitat suitability in the Kep Archipelago, we built temporally stratified SDMs in Maxent (version 3.4.1; Phillips et al., 2020) following the monsoonal seasons defined by Tsujimoto et al. (2018): dry (December–February), pre-monsoon (March–April), summer monsoon (May–September), and post-monsoon (October–November). The Maxent algorithm infers species distribution as a function of relevant environmental covariates (Dudík et al., 2007). Presence-only data of Irrawaddy occurrence organized by these four seasons were used to quantify the statistical relationship between predictor environmental covariates at locations where a species had been observed vs. background locations in which no species had been observed (Phillips et al., 2006).
Sample selection bias is a key consideration when building presence-only models in terms of minimizing the risk of predictions that conflate species distribution with sampling effort (Elith et al., 2011; Merow et al., 2013). To account for environmentally biased sampling of the study area, we performed a spatial filtering routine. First, when multiple localities (species occurrence and background data) were present in a pixel of the 250 m-grid system defined by environmental data, we randomly selected one record. Consequently, a total of 125 on-effort species presence samples and 2,000+ background samples (“pseudo-absences”) remained, effectively minimizing sampling bias because it is common to both presences and absences. Next, we produced tables with the sample records corresponding to each season, the eight dynamic variables derived from SST and Chl-a measurements, and four static physiographic variables (Table 1). Finally, the Samples With Data (SWD) option of Maxent was used to select environmental samples from a distribution of locations with the same selection bias as the occurrence data.
Model Tuning, Variable Selection, and Suitability Thresholds
Parsimonious models, that balance complexity and fit, yield more accurate, interpretable, and transferable predictions (Liu et al., 2009; Warren and Seifert, 2011; Muscarella et al., 2014). This ensures that a model infers habitat suitability based on the most salient predictor variables, and not simply the noise inherent in a training dataset (Hastie et al., 2009). Beginning with five model fitting functions of Maxent—linear (L), quadratic (Q), product (P), threshold (T), and hinge (H)—we sequentially reduced the number of features by screening variable response curves that depict how candidate environmental covariates affect the model prediction. For responses with biologically implausible signals (e.g., multimodal shape, jagged lines, or abrupt jumps), we removed the culprit feature(s), and rescreened variable responses. Linear and quadratic features were chosen to fit all models except for the monsoon period, which used only hinged features to produce smoothed response curves similar to Generalized Additive Models (Merow et al., 2013). During the pre-monsoon, a period characterized by temperature extremes, a product feature was also included to account for the complex interplay between SST and Chl-a variables together with water depth. Table 2 documents the Maxent feature and parameters settings used to tune each seasonal SDM.

Table 2. Fitting functions and environmental variables used to build final SDMs, after model tuning, by season; L, linear; Q, quadratic; P, product; H, hinge.
Next, the Maxent algorithm computes permutation importance (PI) scores to compare the overall contribution of candidate variables, and provide guidance for eliminating redundant covariates (Phillips et al., 2020). This analysis of variable contribution randomly permutes the values for each predictor on occurrence and background localities. The resulting decrease in training AUC, expected to be larger for more important variables (Zhang et al., 2018), is normalized to percentages and reported by Maxent as the variable PI score. Searcy and Shaffer (2016) argue that variables with high PI scores capture ecologically relevant factors that define environmental niche and species distribution, assuming predictor variables are not highly correlated. To select from a suite of 13 environmental variables, we used a PI threshold of 5% (as in Tobeña et al., 2016) to iteratively prune each seasonal SDM down to the most important explanatory variables.
In the absence of population size or the location of true absences, MaxEnt predicts a relative occurrence rate (ROR) as a function of the environmental predictor values at each cell (Merow et al., 2013). To divide the study region into suitable and unsuitable areas and convert Maxent logistic outputs into discrete habitat suitability levels (lowest-intermediate-highest), we used a threshold of occurrence (minimum predicted value at a presence location) based on the data used to calibrate each model (Liu et al., 2005). A minimum ROR threshold of 5% was selected to allow for a certain amount of omission (false negatives), below which the species is not expected to occupy a given cell. While some occurrence localities were removed from the prediction, one key advantage of this classification method was to allow for standardization across multiple SDMs of the same species (Merow et al., 2013). As with three seasonal SDMs developed for Irrawaddy dolphins in Kuching Bay, Malaysia (Verutes et al., 2020), all locations with an ROR below the 10% ROR threshold were classified as limited or unsuitable habitat. The remaining cells where classified according to three levels of habitat suitability: (1) lowest, ranging from 5 to 10%; (2) intermediate, from 10 to 75%; and (3) highest suitability from 75 to 100% of the maximum ROR for each season (Supplementary Table 1).
Model Evaluation and Statistical Tests
The selection of SDM performance metrics should be guided by data availability, species ecology, and overall research goals (Pearson, 2007; Liu et al., 2009). First, we tested different Maxent regularization parameter settings (0.5, 1.0, 1.5, and 2.0) to constrain model complexity and minimize risk of over-fitting to the training data (Elith et al., 2011; Warren and Seifert, 2011). We then assessed model performance based on the area under the curve (AUC) of the receiver operating characteristic plot, a measure of Maxent’s ability to discriminate between randomly chosen presence and background records (Pearson, 2007). Cohen’s kappa, a common model evaluation statistic in ecology, is highly dependent on available prevalence data and may introduce biases in its assessment of accuracy (see Allouche et al., 2006 for empirical analysis). While similar to kappa, the True Skill Statistic (TSS) is not inherently dependent on prevalence data (Liu et al., 2009) and represents an alternative to AUC for model predictions that rely on smaller sample sizes, as was the case for SDMs of the pre- and post-monsoon seasons. In summary, AUC and TSS served as complementary performance statistics because the former is threshold independent and the latter is unaffected by the size of the validation set (Allouche et al., 2006; Merow et al., 2013).
To assess predictive performance of final models with held-out data, calculations were performed using Maxent and the biomod2 package for R (Thuiller et al., 2009; Elith et al., 2011). We used a k-fold cross-validation procedure, where 90% of data were kept for training and the remaining sample for evaluation. A total of 10 models (k = 10) were trained and evaluated against the excluded test data to estimate performance on the held-out folds and ensure the overall accuracy assessment was not an artifact of sub-sampling. We calculated (1) mean and standard deviation (SD) of AUC across all test models and (2) the difference between training AUC values of each species’ final SDM (using all presences) and the mean AUC of the test SDMs. Low test-AUC SD and/or small differences between the training AUC and mean test-AUC values are indicators of model robustness (Warren and Seifert, 2011; Herkt et al., 2016; Tobeña et al., 2016).
When projecting habitat models outside a surveyed area, the resulting predictions are more likely to be reliable if these new locations have environmental conditions similar to the training samples (Barbosa et al., 2009; Pearson, 2007). To reveal where novel conditions exist across predictors retained for each seasonal SDMs, we conducted Multivariate Environmental Similarity Surface analysis (MESS; Elith et al., 2010). In order to take into consideration differences in temporal variability and estimate Irrawaddy dolphin habitat suitability, SDMs were projected at seasonal midpoints for months with reasonable spatial coverage of environmental predictor variables between October 2018 and September 2019 (Figure 5). Guided by MESS analyses (Supplementary Figure 1), we excluded areas of the habitat suitability maps where at least one predictor variable was found to be outside the range of data used to train each seasonal SDM.
All explanatory variables were checked for normality using a Shapiro-Wilk test and the homogeneity of variances was tested using Fligner-Killeen test. Environmental data on Chl-a, seafloor slope, distance to islands and rivers were power transformed (log10 or square root) to normalize their respective distributions. Non-parametric tests were used for environmental variables that did not meet the assumptions of normality and homoscedasticity. We used the rank-based Kruskal-Wallis H-test to detect statistically significant differences across seasons for independent variables collected at sighting locations. For group size, a post hoc Dunn’s test pinpointed which environmental variable had significantly different mean values from season to season. An alpha value of 0.05 was used as the significance level. All statistical analyses were performed in R version 3.6.2 (R Core Team, 2020).
Results
A total of 187 dolphin groups were sighted over a 24-month period (2017–2019), of which 152 (81%) were on-effort occurrences (Table 3). During adequate sighting conditions, encounter rates were lowest pre- and post-monsoon as previously reported by Tubbs et al. (2020). The locations of on-effort sightings suggest these dolphins are evenly distributed around the Kep Archipelago islands, and in higher densities (1) eastward toward the mouth of the Kien Giang River, (2) in the southern direction of the Cambodian-Vietnamese maritime border, and (3) in the relatively shallow waters (3–5.5 m) approaching the channel between Phu Quoc Island, Vietnam and the Kampot River, Cambodia (Figure 2). With the exception of one dry season encounter, all sightings > 4 km east of the Kep Archipelago were during the summer monsoon. Furthermore, there was a dearth of dolphin encounters in areas exceeding depths of 5.5 m (< 1% of sightings), despite a higher proportion (6% of total boat survey effort across all seasons) occurring in deeper waters.

Table 3. On-effort Irrawaddy dolphin sightings and encounter rates by season for the survey period, from October 2017 to September 2019.
Overall, Kep’s Irrawaddy dolphins displayed habitat preferences for water depths between 3.0 and 5.3 m, SST from 27 to 32°C, and in close proximity to offshore islands (< 7.5 km) based on the central 90th percentile of variable distributions. A histogram of sightings vs. water depths (Figure 3A) showed a clear peak between 3.5 and 4.0 m (66% of sighting), with a roughly equal contribution from all seasons. Habitat areas corresponding to GIS measurements for distance to offshore islands and river mouths, utilized by dolphins during both the wet and dry seasons, were quite diverse (Figures 3B–C). A bimodal pattern was detected for variables of distance to offshore islands (peaks at 1.5 and 5.5 km) and river mouths (12 and 18 km), suggesting that fluctuating high and low river flows before and after the summer monsoon were a potential driver of change in seasonal habitat and, consequently, hotspots of Irrawaddy dolphin occurrence.
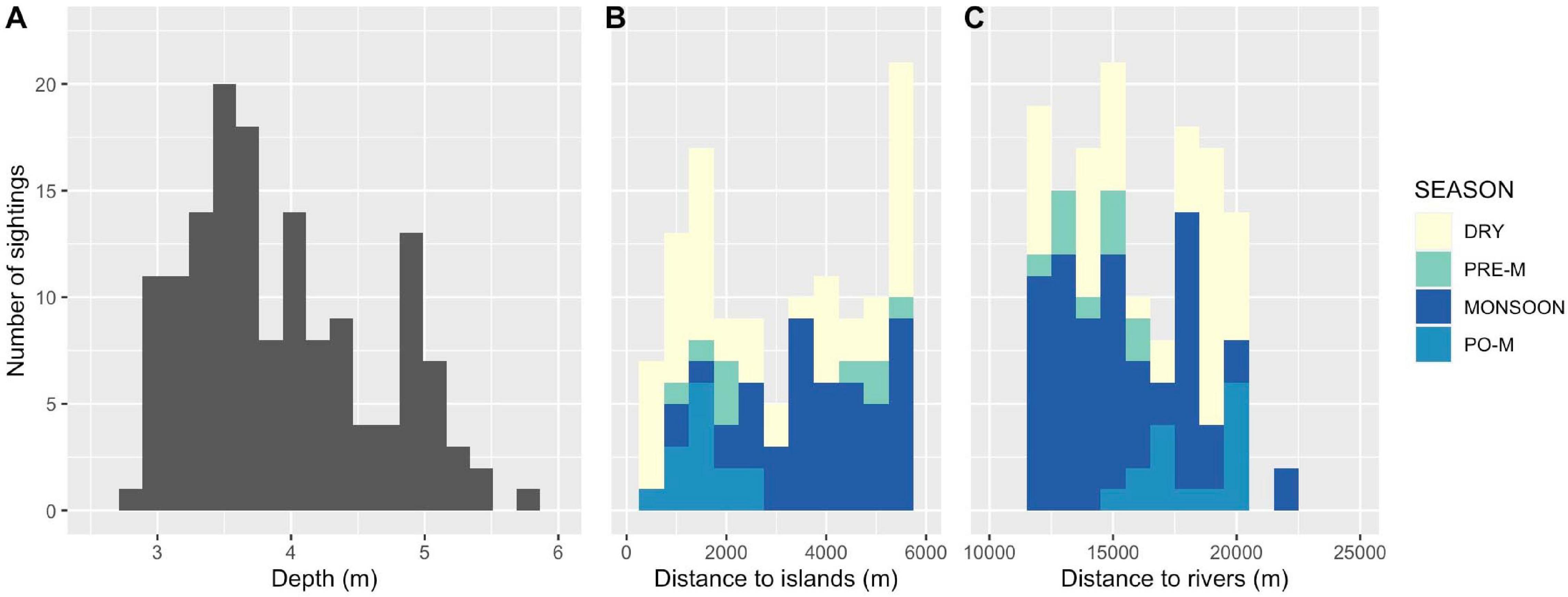
Figure 3. Histogram plots for of (A) water depth, (B) distance to offshore islands, and (C) distance to river mouths. Note that there is one outlier, 8.9 m, outside the range of depth values (A).
Group Size and Seasonal Habitat Patterns
Irrawaddy group sizes were significantly different across the four seasons (H = 27.94, P < 0.001). Post-monsoon season had the largest mean group size of 9.0 individuals (SE = 1.2; range = 1–32; n = 47), followed by the pre-monsoon with a mean group size of 7.1 individuals (SE = 0.7; range = 4–10; n = 12). The dry and the monsoon seasons had the smallest mean group sizes of 5.8 (SE = 0.8; range = 1–14; n = 58) and 4.0 individuals (SE = 0.4; range = 1–9; n = 70), respectively. Of the 187 Irrawaddy dolphin groups sighted between 2017 and 2019, the most frequently encountered group size was 3 individuals (n = 30), followed closely by groups of 4 individuals (n = 27). Following the group size definitions of Tubbs et al. (2020)—small, 1–3; medium, 4–8; and large, ≥ 9 individuals—pre-monsoon sightings were predominately medium to large-sized groups. Interestingly, this shifted to groups of small and medium sizes during the higher flow period of the monsoon season (Figure 4F). Because the Kruskal-Wallis H-test is global test statistic and cannot determine which specific seasons are significantly different from each other, a Dunn’s test (with Bonferroni correction for the accepted p-values) confirmed significant seasonal differences between wet and both the dry and pre-monsoon periods for mean group size (P < 0.015). Following the summer monsoon and through the dry season (October to February), sightings were more evenly distributed across the three group sizes.
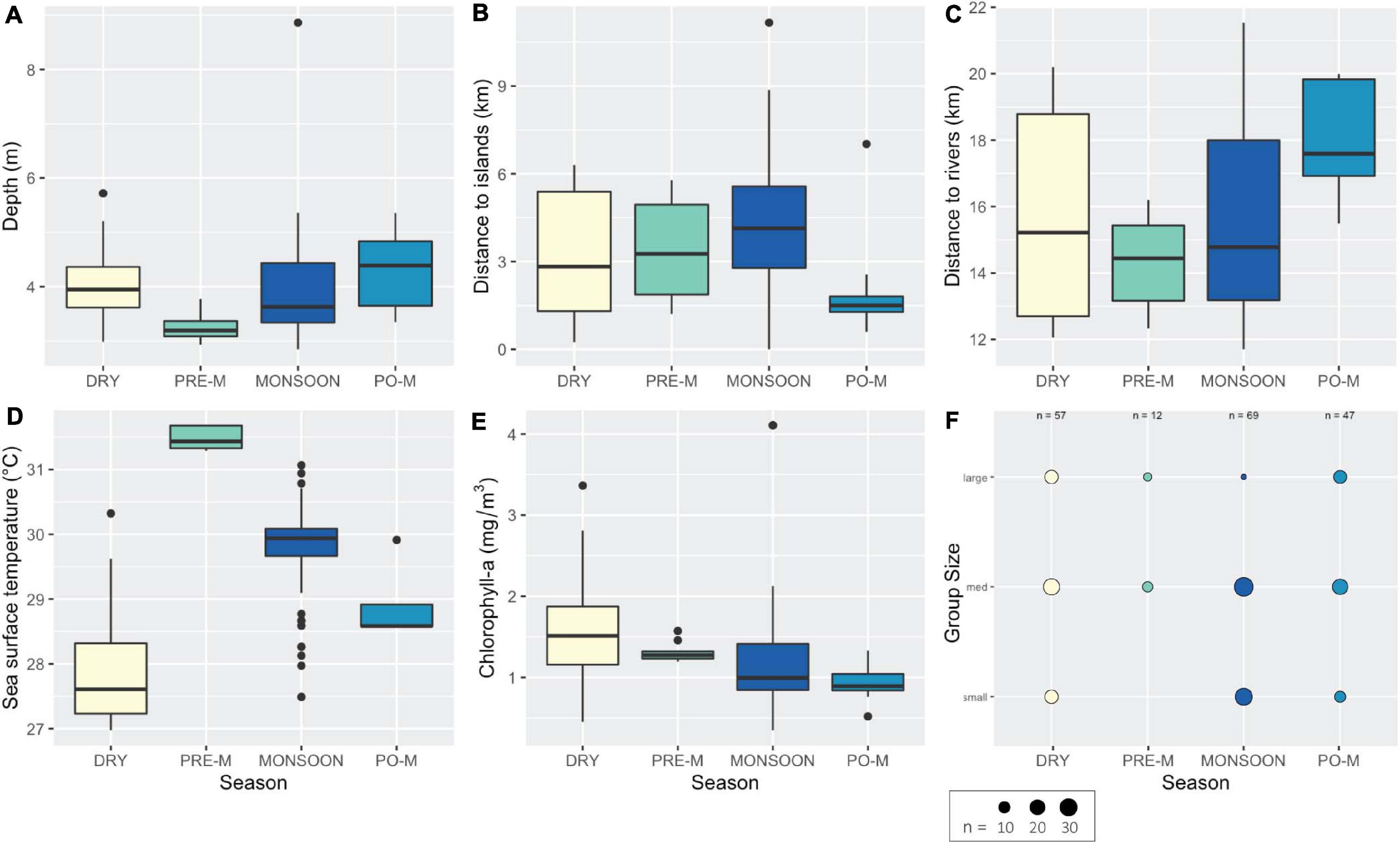
Figure 4. Box and dot-density plots of the habitat characteristics and group size of Irrawaddy dolphins as sighted in Kep waters during the four seasons in terms of: (A) water depth, (B) distance to offshore islands, (C) distance to river mouths, (D) sea surface temperature, (E) chlorophyll-a concentration, and (F) group size.
Boxplots revealed extreme environmental data values for the pre-monsoon (e.g., Figure 4D), which could be limiting suitable dolphin habitat prior to the wet season and was the period with the fewest dolphins encountered. All pre-monsoon Irrawaddy sightings (n = 12) were found to have the highest SST levels, as compared to other seasons. Median SST, based on monthly composites collected for the entire study area from March to April in 2018 and 2019, did not drop below 32°C during the pre-monsoon period. In comparison, only one sighting (during the summer monsoon) was found to exceed sea surface temperatures of 31°C. Low precipitation rates and higher air temperatures from December to March may initiate a shift to weaker river flows and shallower coastal waters, which can result in the highest sea surface temperature and salinity levels in the region from March to May (see sub section “Limitations and Simplifications” for further discussion of limiting factors to Irrawaddy habitat models during the pre-monsoon period).
Relative to other seasons, Irrawaddy dolphins were encountered closer to major river mouths and at greater distances from the offshore islands during the summer monsoon. The inverse was true post-monsoon, as dolphins gravitated back to the islands of the Kep Archipelago and away from two major river mouths (Figures 4A,B). This pattern of dolphin occurrence was less apparent during the dry and pre-monsoon seasons, as sightings were more evenly distributed across a range of environmental variables, especially proximity to rivers and offshore islands (Figures 4B,C). Kruskal-Wallis rank sum tests confirmed the possibility of significant seasonal differences in habitat use for depth (H = 17.06, P < 0.001), distance to islands (H = 12.88, P < 0.005), distance to river mouths (H = 16.21, P < 0.001), chlorophyll-a (H = 25.82, P < 0.001), and sea surface temperature (H = 80.86, P < 0.001), but not seafloor slope [one-way analysis of variance (ANOVA), F = 1.298, P = 0.278]. This finding supports the use of variable permutation importance (PI) scores to discern the relative importance of environmental variables to explain seasonal variation in dolphin habitat use.
Spatially Explicit Seasonal Habitat Models
Variable PI scores indicated conditional dependence of Irrawaddy dolphin habitat on the majority of predictors considered (9 of 13 had scores greater than the 5% threshold). Not surprisingly, no one variable combination was retained consistently across all four seasons and, ultimately, either 3 or 4 environmental predictors were used to build final SDMs (Table 2). PI scores pointed to water depth as a key explanatory variable prior to and during the wet season (61 and 62% overall contribution). Additionally, distance to rivers (30%), especially at intermediate distances, and either gradual or steep seafloor slopes (8%) were factors in Irrawaddy dolphin habitat selection during the monsoon period (Supplementary Figure 2C). Following the wet season (October–November), peaking during the dry season (January), and then waning pre-monsoon (March–April), sea surface temperature (22, 71, and 21%) was a strong contributor to predicted probability of dolphin presence along with time-lagged Chl-a (8–36%) and local variation in SST (13–42%) for the latter two seasons. Interestingly, the relative importance of SST and local variation in surface water temperature (V-SST) inverted from post-monsoon to the dry season.
Cross validation determined that all four SDMs had scores for test-AUC > 0.8 and TSS > 0.6, an indication of moderate to good discrimination (Table 4). Performance metrics for the monsoon SDM were lowest, followed by the dry season (TSS scores of 0.604 and 0.773, respectively). As evidence of model robustness, 3 of the 4 SDMs (all except summer monsoon) had small differences (< 5%) between mean test-AUC and the corresponding AUC score of the training set.
Maxent outputs revealed site fidelity as well as temporally distinct patterns in Irrawaddy dolphin habitat use (Figure 5). For instance, both the pre-monsoon and monsoon seasons had overlapping areas of high habitat suitability inside the Kep MFMA (Figures 5B,C). Beginning in the wet season, however, suitability estimates suggested a preference for waters closer to major river mouths and with shallower depths, as indicated by the orange and red-colored areas surrounding the Kep Archipelago (Figure 5C). Post-monsoon, highest habitat suitability levels were more dispersed southwest of the offshore islands (Figure 5D), which corresponds to areas with less local variation in SST and medium levels of Chl-a two months prior to a sighting of the species. It is worth noting that the majority of these critical habitat areas were found to be outside the MFMA, in areas directly northwest and southeast of the Kep Archipelago. For coastal areas of Kampot, Cambodia and the Vietnamese Pirate Islands—well beyond the survey tracks of this study—Irrawaddy dolphin habitat suitability estimates tended to be in the low to intermediate range, with large gaps in predicted probability of occurrence due to MESS exclusions.
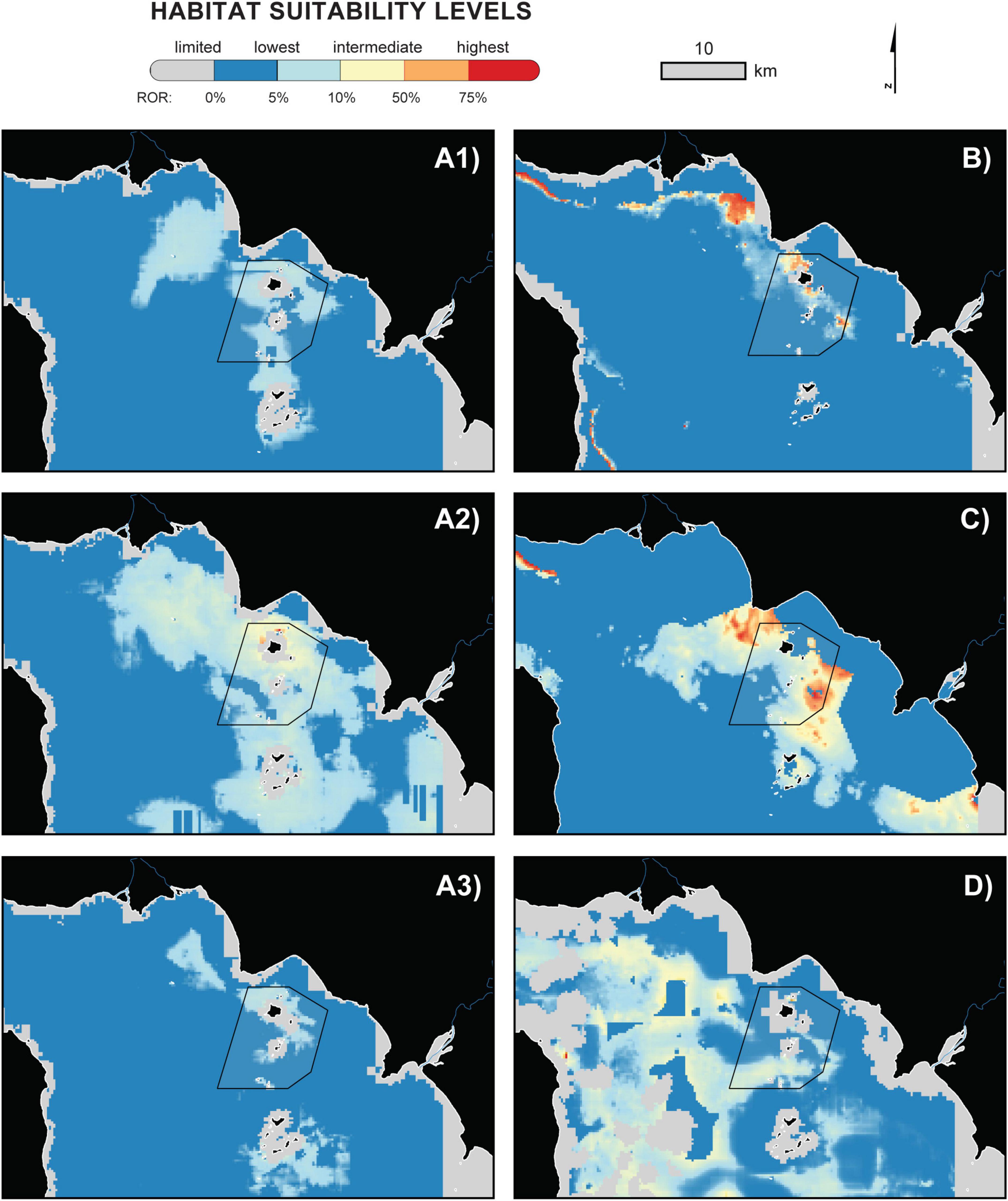
Figure 5. Maps of Irrawaddy dolphin habitat suitability based on the best performing models projected to seasonal midpoints for months between 2018 and 2019: (A1) December, (A2) January, and (A3) February 2019 of the dry season; (B) April 2019, pre-monsoon; (C) May to September 2019 of the summer monsoon; and (D) October 2018, post-monsoon. Some habitat suitability areas were masked out based on Multivariate Environmental Similarity Surface analyses (Elith et al., 2010), which coincide with the most restrictive results for each seasonal SDM. The black outline demarcates the Kep Marine Fisheries Management Area.
Discussion
In this article we present new evidence about spatiotemporal patterns in Irrawaddy dolphins’ occurrence and habitat suitability predictions for the Kien Giang–Kep Archipelago Important Marine Mammal Area. Habitat models were built, validated and projected across a transboundary region to map seasonal distributions of an endangered species of small cetacean. Overall, Irrawaddy dolphins from the Kep Archipelago displayed habitat preferences that were similar to the suite of environmental variables commonly associated with coastal-estuarine Irrawaddy populations (Minton et al., 2011; Mahmud et al., 2018; Jackson-Ricketts et al., 2020). Previous studies have linked Irrawaddy dolphins to shallow areas, close to river mouths, and with changing tidal states; conditions associated with low sea surface salinity and high turbidity (Dolar et al., 2002; Peter et al., 2016b). Specific to this study and based on the central 90th percentile of environmental data distributions, Irrawaddy dolphins were sighted in water depths 3.0–5.3 m, with SST between 27 and 32°C, and < 8 km from offshore islands. With the exception of two sightings, there were no dolphin encounters in the deepest waters (> 5.5 m) surveyed between Phu Quoc Island, Vietnam and Kampot Province, Cambodia (Figure 2).
Irrawaddy dolphins of the Kep Archipelago are likely year-round inhabitants, yet questions remain about population size and their exact whereabouts prior to summer monsoon. Kep’s dolphin population consists of at least 32 individuals (Tubbs, pers. observ.), and the only historical abundance estimates come from systematic line transect surveys in neighboring Trat province, Thailand (Hines et al., 2015). Spatiotemporal variation in Irrawaddy occurrence first reported by Tubbs et al. (2019, 2020) was the impetus for acquiring spatially explicit environmental data used by this study to detect emergent patterns in seasonal habitat use. Exploratory analysis and visualization (Figure 4) confirmed statistically significant differences in habitat preferences across seasons, warranting further investigation of which environmental variables could be used to explain changes in dolphin habitat suitability in the Kien Giang–Kep Archipelago IMMA.
Emergent Patterns of Habitat Suitability
Geographically, the Kep Archipelago is nestled inside the Eastern Gulf of Thailand, home to a wealth of natural assets, including coral and seagrass habitats (Reid et al., 2019), and phenological dynamics that influence river flows. Freshwater input to the Kep Archipelago from two major rivers (Giang and Kampot) is lowest pre-monsoon due to limited rainfall in the months prior to the wet season (Tsujimoto et al., 2018). Consequently, coastal water depths are low, while SST and salinity are at the highest levels from January through April. In the neighboring Kien Giang Biosphere Reserve during the month of April, 8-day running averages of salinity (see text footnote 4) and SST measurements approaching 36°C (Vu, unpubl. data) suggest that less precipitation and river discharge into the ocean (Learmonth et al., 2006) is correlated with increasing SST and salinity levels. Despite balanced survey effort throughout the year (Table 3), dolphin sightings were scant during the pre-monsoon (n = 12; Tubbs et al., 2020). While this study could not definitively determine Irrawaddy dolphin response to changes in salinity, there was relatively low habitat suitability predicted for when and where SST levels were highest. The higher proportion of medium to large group size encounters between December and April (Figure 4F) may also be a limiting factor to Irrawaddy prevalence prior to the wet season. Monitoring of areas closer to river mouths, pre-monsoon, is needed to determine if Kep’s dolphin population is adapted to tolerate extreme levels of salinity and SST, similar to the ecophysiological responses of other marine mammals (Fiedler, 2009).
Population-level studies are also needed to inform the design of new habitat models that detect foraging decisions based on the diversity of fish species known to comprise dolphin diets (Win and Bu, 2019), and the availability of cephalopods, crustaceans, and other prey (Santos et al., 2013). At least three fishing communities (Ankgrong, Phun Thmey, and Preak Tanin) use trawling and longtail boats to catch cephalopods, primarily squid, in the waters of the Kep Archipelago (Ferber et al., unpubl. data). Monthly self-reports from squid fishers indicate the highest levels of effort between November and January (post-monsoon), followed by January and February (dry season) and then June to September (summer monsoon). Lower fishing effort prior to the wet season may be related to a scarcity of dolphin prey, specifically during the months of March, April and May. Because Irrawaddy dolphins are generalist feeders (Parra, 2005) and maps of their prey are difficult to find, we included Chl-a and SST as dynamic variables in our predictor set. Variables retained in final SDMs (Table 2) suggest that time-lagged chlorophyll-a concentration [Chl-a (-1 m) and Chl-a (-2 m)] can play an important role in defining the distribution of Irrawaddy dolphins following the wet season, when a combination of low SST and higher Chl-a levels are present. Further study is needed to determine if a strong link exists between Irrawaddy prey abundance and seasonal habitat use, and to what extent this can be explained using proxy variables for primary productivity.
Limitations and Simplifications
When population size is known, Maxent predicts the species occurrence rate for each grid cell, which translates into the expected number of individuals in that location. Here, model predictions were interpreted as indices of habitat suitability, primarily for exploratory purposes. We deliberately avoided a reliance on default settings when making decisions about data preparation, parameter selection, and variables to retain. The regularization parameter in Maxent is conceptually similar to the Akaike Information Criterion (AIC), as it penalizes model complexity and can enhance the transferability of SDM predictions beyond locations used to train each model (Dudík et al., 2007). We could not use AICc to compare the quality of different model iterations, following a continuous distribution of regularization coefficients, due to the Samples with Data (SWD) approach used to train each seasonal SDM. Techniques that incorporate automated tuning of the regularization parameter into correlative SDMs are still under development for SWD, and this functionality is expected in the R package, ENMeval v2.0 (Muscarella et al., 2014). The true skill statistic (TSS) assesses model accuracy independent of species prevalence, and is preferable to the kappa statistic when relying on small sample sizes (Allouche et al., 2006), as was the case with two seasonal SDMs utilized by this study. When applied judiciously, Maxent performs well relative to other habitat models (Merow et al., 2013). Nevertheless, the acquisition of additional sightings records will be important for testing these SDMs against independent datasets, as only 9% of published studies report this type of performance assessment (Robinson et al., 2011).
In summary, this research was limited by a paucity of dolphin sightings prior to and following the wet season, along with gaps in spatial data to characterize the nearshore marine environment. All pre-monsoon dolphin encounters occurred during 1 month (April 2019), and all but one post-monsoon sighting was in October 2017. It is difficult to determine why Irrawaddy dolphin encounter rates were lowest during these two periods (Table 3) without a detailed water parameter analysis. It is possible that certain areas of the Kep Archipelago may not offer suitable habitat at this time of year, leading the species to stray elsewhere. As previously mentioned, we expect salinity and SST levels to be highest in months leading up to the wet season, and before freshwater input increases. SST may be correlated with salinity and, as a consequence, dolphins avoid high surface water temperatures and/or extreme salinity areas, which are widespread during the pre-monsoon period. Additional monitoring could help detect these and other subtle environmental cues that influence seasonal dolphin habitat selection (Parra et al., 2006; Smith et al., 2009). More specifically, boat surveys would help overcome limitations of the L-band microwave radiometer instrument, which measures sea surface salinity at a relatively coarse spatial resolution (between 40 and 70 km2) and is subject to contamination by bright land signals (Miller et al., 1997). Artifacts in remotely sensed data utilized by this study were most commonly found in nearshore areas of the Kep islands (Figure 5).
Conservation and Management Opportunities
Evidence is mounting that illegal fishing activities, especially bottom-trawling, are ubiquitous to the Vietnam-Cambodia border region (Vu et al., 2017; Böhm, 2019; Reid et al., 2019). Addressing the vast monitoring deficiencies of the Southeast Asia region (Teh et al., 2015; Hines et al., 2020) is a necessary first step to understand where and when IUU fishing impacts, such as bycatch and habitat destruction, are most consequential to Irrawaddy dolphins and other marine mammals (Read, 2008; Jackson-Ricketts et al., 2020; Verutes et al., 2020). Moving forward, systematic surveys of the Kep Archipelago are needed to challenge our assumptions related to seasonal variability of the marine environment and fill gaps of earth observation data layers near shorelines and river mouths. We anticipate that emerging networks to monitor marine megafauna and fisheries of the Kien Giang–Kep Archipelago IMMA (e.g., Vu et al., 2017; Tubbs et al., 2020) will continue to expand boat survey coverage, public participation, and socio-ecological research that further defines threats to and the range of Kep’s resident Irrawaddy dolphin population. A recent genetic study by Caballero et al. (2018) suggests connectivity between the Irrawaddy populations of India, Thailand and Cambodia, which could have implications for SDM projection space (MESS) used to define species’ niche (Elith et al., 2010; Merow et al., 2013).
Against the backdrop of persistent, harmful, and unregulated threats to marine mammals and their habitats, the Kep Marine Fisheries Management Area (MFMA) is an opportunity to promote biodiversity conservation measures that spatially manage multiple human uses (Fisheries Administration Cambodia, 2018). For example, the highest density trawling areas mapped by Böhm (2019) appear to overlap dolphin hotspots identified by this research, specifically during the wet season (yellow and red-colored areas of Figure 5C). Cambodia’s 2016 international obligations via the Aichi Biodiversity Target 11 states that: “10 per cent of coastal and marine areas, especially areas of particular importance for biodiversity and ecosystem services, are conserved through effectively and equitably managed, ecologically representative and well-connected systems of protected areas…” (Secretariat of the Convention on Biological Diversity [SCBD], 2010, p. 9). This could be achieved by expanding the recently enacted Kep MFMA toward critical dolphin habitat areas where Marine Conservation Cambodia already promotes mixed human uses such as recreational activities, sustainable tourism, and the protection of biodiversity resources (Marine Conservation Cambodia [MCC], 2016).
Fisheries management, especially for data-limited small-scale fisheries, is more effective when considering the different objectives of communities participating in the process, and the existing local conditions (e.g., governance, socioeconomic, research and education) that enable or limit conservation success (Berkes, 2007; Teh et al., 2015). In one form or another, multi-sectoral zoning at the MFMA scale should continue to be implemented through a co-management program between local agencies and community organizations. Recent monitoring and evaluation along the maritime border suggests this area could be classified as in a critical state, due in large part to impacts from destructive fishing, unsustainable bycatch and marine pollution (Vu et al., 2017; Reid et al., 2019; Böhm, 2019). The data presented here serves as a baseline for comparison against future studies and to help formulate new hypotheses about dolphin spatiotemporal occurrence, abundance and habitat quality. Potential next steps include IUCN status assessment for the Kien Giang–Kep Archipelago IMMA (Minton et al., 2017) and mark-recapture studies to estimate population size of Irrawaddy dolphins and other marine mammals recorded in the area [e.g., Indo-Pacific finless porpoises (Neophocaena phocaenoides), Indo-Pacific humpback dolphins (Sousa chinensis), dugongs (Dugong dugon)].
Conclusion
The present study identified emergent patterns of habitat use by Irrawaddy dolphins inside the dynamic, transnational waters of the Kien Giang–Kep Archipelago Important Marine Mammal Area. Despite substantial data limitations, habitat suitability estimates identified where and when significant seasonal changes to the marine environment are likely to alter the distribution of a cetacean species of conservation significance. As the first fine-scale spatial characterization of Irrawaddy dolphin habitat in Cambodia’s Kep Archipelago, the results presented here suggest there are: (1) hotspots of dolphin use, which vary seasonally, (2) areas with highest habitat suitability situated along the active Cambodia-Vietnam border region, and (3) additional patterns, indicators, and habitat that remain to be uncovered, such as how changing freshwater flows affect water depth, salinity, primary productivity, and resource abundance. Most importantly, our findings have implications for the urgent need to spatially manage fisheries and other sectors of economic and traditional importance in the region. It is the intent of this research to set the stage for future assessments that consider cumulative impacts posed by anthropogenic activities to Irrawaddy dolphins and other species of conservation concern.
Data Availability Statement
The original contributions presented in the study are included in the article/Supplementary Materials, further inquiries can be directed to the corresponding author.
Ethics Statement
Ethical review and approval was not required for the animal study because it does not apply to boat survey research in Cambodia.
Author Contributions
GV and ST: conceived and designed the experiments. ST and GV: performed the experiments. GV, NS, and ST: analyzed the data. ST, NS, DC, PW, and OC: contributed with data. GV, ST, and NS: wrote the manuscript.
Conflict of Interest
The authors declare that the research was conducted in the absence of any commercial or financial relationships that could be construed as a potential conflict of interest.
Acknowledgments
We thank Marine Conservation Cambodia, The Heinrich Böll Foundation, The Rufford Foundation, Fondation Ensemble, The Mohammed Bin Zayed Species Conservation Fund, the International Conservation Fund of Canada, and Ouk Vibol, Phay Somany and Chheng Touch from the Cambodian Fisheries Administration for their support of The Cambodian Marine Mammal Conservation Project. We also thank the NERC Earth Observation Data Acquisition and Analysis Service (NEODAAS) for supplying remotely sensed data utilized by this study. Our article benefited substantially from field data collected by L. Vu and A. Böhm; M. Tobeña provided biomod2 scripting assistance; and finally, S. Villasante, M. B. Santos, A. F. Johnson, R. Lewison, and G. Pierce advised GV.
Supplementary Material
The Supplementary Material for this article can be found online at: https://www.frontiersin.org/articles/10.3389/fmars.2021.617921/full#supplementary-material
Footnotes
- ^ http://www.gadm.org
- ^ http://www.openstreetmap.org
- ^ https://www-cdn.eumetsat.int/files/2021-03/Sentinel-3%20OLCI%20Marine%20User%20Handbook.pdf
- ^ https://podaac.jpl.nasa.gov/dataset/SMAP_RSS_L3_SSS_SMI_8DAY-RUNNINGMEAN_V3_70KM
References
Allouche, O., Tsoar, A., and Kadmon, R. (2006). Assessing the accuracy of species distribution models: prevalence, kappa and the true skill statistic (TSS). J. Appl. Ecol. 43, 1223–1232. doi: 10.1111/j.1365-2664.2006.01214.x
Bailey, H., and Thompson, P. M. (2009). Using marine mammal habitat modelling to identify priority conservation zones within a marine protected area. Mar. Ecol. Prog. Ser. 378, 279–287. doi: 10.3354/meps07887
Barbosa, A. M., Real, R., and Vargas, J. M. (2009). Transferability of environmental favourability models in geographic space: the case of the Iberian desman (Galemys pyrenaicus) in Portugal and Spain. Ecol. Model. 220, 747–754. doi: 10.1016/j.ecolmodel.2008.12.004
Baumgartner, M. F., Mullin, K. D., May, L. N., and Leming, T. D. (2001). Cetacean habitats in the northern Gulf of Mexico. Fish. Bull. 99, 219–219.
Bearzi, G., Azzellino, A., Politi, E., Costa, M., and Bastianini, M. (2008). Influence of seasonal forcing on habitat use by bottlenose dolphins Tursiops truncatus in the Northern Adriatic Sea. Ocean Sci. J. 43, 175–182. doi: 10.1007/BF03029922
Beasley, I., and Davidson, P. (2007). Conservation status of marine mammals in Cambodian waters, including seven new cetacean records of occurrence. Aquat. Mamm. 33, 368–379. doi: 10.1578/AM.33.3.2007.368
Becker, E. A., Forney, K. A., Thayre, B. J., Debich, A. J., Campbell, G. S., Whitaker, K., et al. (2017). Habitat-based density models for three cetacean species off Southern California illustrate pronounced seasonal differences. Front. Mar. Sci. 4:121. doi: 10.3389/fmars.2017.00121
Berkes, F. (2007). Community-based conservation in a globalized world. Proc. Natl. Acad. Sci. U.S.A. 104, 15188–15193. doi: 10.1073/pnas.0702098104
Böhm, A. (2019). Marine Harvesting Network in Cambodia: Technical Report on Transnational Fishing Activities, Marine Conservation Cambodia. Available online at: https://www.marineconservationcambodia.org/ (accessed February 5, 2019).
Boon, P. Y., Mulligan, B., Benbow, S. L. P., Thorne, B. V., Leng, P., and Longhurst, K. (2014). Zoning Cambodia’s first Marine Fisheries Management Area. Cambodian J. Nat. Hist. 2014, 55–65.
Briscoe, D. K., Hiatt, S., Lewison, R., and Hines, E. (2014). Modeling habitat and bycatch risk for dugongs in Sabah, Malaysia. Endanger. Species Res. 24, 237–247. doi: 10.3354/esr00600
Caballero, S., Dove, V., Jackson-Ricketts, J., Junchompoo, C., Cohen, S., and Hines, E. (2018). Mitochondrial DNA diversity and population structure in the Irrawaddy dolphin (Orcaella brevirostris) from the Gulf of Thailand and the Mekong River. Mar. Mamm. Sci. 35, 300–310. doi: 10.1111/mms.12518
Campbell, G. S., Thomas, L., Whitaker, K., Douglas, A. B., Calambokidis, J., and Hildebrand, J. A. (2015). Inter-annual and seasonal trends in cetacean distribution, density and abundance off southern California. Deep Sea Res. II Top. Stud. Oceanogr. 112, 143–157. doi: 10.1016/j.dsr2.2014.10.008
Cañadas, A., Sagarminaga, R., De Stephanis, R., Urquiola, E., and Hammond, P. S. (2005). Habitat preference modelling as a conservation tool: proposals for marine protected areas for cetaceans in southern Spanish waters. Aquat. Conserv. Mar. Freshw. Ecosyst. 15, 495–521. doi: 10.1002/aqc.689
Connor, R. C., Smolker, R., and Bejder, L. (2006). Synchrony, social behaviour and alliance affiliation in Indian Ocean bottlenose dolphins, Tursiops aduncus. Anim. Behav. 72, 1371–1378. doi: 10.1016/j.anbehav.2006.03.014
Daura-Jorge, F. G., Wedekin, L. L., Piacentini, V. D. Q., and Simões-Lopes, P. C. (2005). Seasonal and daily patterns of group size, cohesion and activity of the estuarine dolphin, Sotalia guianensis, in southern Brazil. Rev. Bras. Zool. 22, 1014–1021. doi: 10.1590/S0101-81752005000400029
Dolar, M. L. L., Perrin, W. F., Gaudiano, J. P., Yaptinchay, A. A. S. P., and Tan, J. M. L. (2002). Preliminary report on a small estuarine population of Irrawaddy dolphins Orcaella brevirostris in the Philippines. Raffles Bull. Zool. 155–160.
Dudík, M., Phillips, S. J., and Schapire, R. E. (2007). Maximum entropy density estimation with generalized regularization and an application to species distribution modeling. J. Mach. Learn. Res. 8, 1217–1260.
Elith, J., Kearney, M., and Phillips, S. (2010). The art of modelling range-shifting species. Methods Ecol. Evol. 1, 330–342. doi: 10.1111/j.2041-210X.2010.00036.x
Elith, J., Phillips, S. J., Hastie, T., Dudík, M., Chee, Y. E., and Yates, C. J. (2011). A statistical explanation of MaxEnt for ecologists. Divers. Distrib. 17, 43–57. doi: 10.1111/j.1472-4642.2010.00725.x
Feist, B. E., Bellman, M. A., Becker, E. A., Forney, K. A., Ford, M. J., and Levin, P. S. (2015). Potential overlap between cetaceans and commercial groundfish fleets that operate in the California Current Large Marine Ecosystem. NOAA Prof. Pap. NMFS 17, 1–27. doi: 10.7755/PP.17
Fiedler, P. C. (2009). “The ocean environment,” in Encyclopedia of Marine Mammals, eds W. F. Perrin et al. (San Diego, CA: Academic Press), 792–797.
Fisheries Administration Cambodia (2018). Royal Decree of Community Fisheries. Available online at: http://www.fia.gov.kh/english/index.php?page=legislation
Garaffo, G. V., Dans, S. L., Pedraza, S. N., Degrati, M., Schiavini, A., González, R., et al. (2011). Modeling habitat use for dusky dolphin and Commerson’s dolphin in Patagonia. Mar. Ecol. Prog. Ser. 421, 217–227. doi: 10.3354/meps08912
Gilles, A., Viquerat, S., Becker, E. A., Forney, K. A., Geelhoed, S. C. V., Haelters, J., et al. (2016). Seasonal habitat-based density models for a marine top predator, the harbor porpoise, in a dynamic environment. Ecosphere 7:e01367. doi: 10.1002/ecs2.1367
Giralt Paradell, O., López, B. D., and Methion, S. (2019). Modelling common dolphin (Delphinus delphis) coastal distribution and habitat use: insights for conservation. Ocean Coast. Manage. 179:104836. doi: 10.1016/j.ocecoaman.2019.104836
Gregr, E. J., Baumgartner, M. F., Laidre, K. L., and Palacios, D. M. (2013). Marine mammal habitat models come of age: the emergence of ecological and management relevance. Endanger. Species Res. 22, 205–212. doi: 10.3354/esr00476
Hammond, P. S., Macleod, K., Berggren, P., Borchers, D. L., Burt, L., Cañadas, A., et al. (2013). Cetacean abundance and distribution in European Atlantic shelf waters to inform conservation and management. Biol. Conserv. 164, 107–122. doi: 10.1016/j.biocon.2013.04.010
Hastie, G. D., Swift, R. J., Slesser, G., Thompson, P. M., and Turrell, W. R. (2005). Environmental models for predicting oceanic dolphin habitat in the Northeast Atlantic. ICES J. Mar. Sci. 62, 760–770. doi: 10.1016/j.icesjms.2005.02.004
Hastie, T., Tibshirani, R., and Friedman, J. (2009). The Elements of Statistical Learning: Data Mining, Inference, and Prediction. Berlin: Springer.
Herkt, K. M. B., Barnikel, G., Skidmore, A. K., and Fahr, J. (2016). A high-resolution model of bat diversity and endemism for continental Africa. Ecol. Model. 320, 9–28. doi: 10.1016/j.ecolmodel.2015.09.009
Hines, E., Ponnampalam, L. S., Junchompoo, C., Peter, C., Vu, L., Huynh, T., et al. (2020). Getting to the bottom of bycatch: a GIS-based toolbox to assess the risk of marine mammal bycatch. Endanger. Species Res. 42, 37–57. doi: 10.3354/esr01037
Hines, E., Strindberg, S., Junchompoo, C., Ponnampalam, L. S., Ilangakoon, A. D., Jackson-Ricketts, J., et al. (2015). Line transect estimates of Irrawaddy dolphin abundance along the eastern Gulf Coast of Thailand. Front. Mar. Sci. 2:63. doi: 10.3389/fmars.2015.00063
IUCN-MMPATF (2019). Kien Giang and Kep Archipelago IMMA, Global Dataset of Important Marine Mammal Areas (IUCN-IMMA). Available online at: www.marinemammalhabitat.org/imma-eatlas (accessed September 10, 2020).
Jaaman, S. A., Lah-Anyi, Y. U., and Pierce, G. J. (2009). The magnitude and sustainability of marine mammal by-catch in fisheries in East Malaysia. J. Mar. Biol. Assoc. U.K. 89, 907–920. doi: 10.1017/S002531540800249X
Jackson-Ricketts, J., Junchompoo, C., Hines, E. M., Hazen, E. L., Ponnampalam, L. S., Ilangakoon, A., et al. (2020). Habitat modeling of Irrawaddy dolphins (Orcaella brevirostris) in the Eastern Gulf of Thailand. Ecol. Evol. 10, 2778–2792. doi: 10.1002/ece3.6023
Jackson-Ricketts, J., Ruiz-Cooley, R. I., Junchompoo, C., Thongsukdee, S., Intongkham, A., Ninwat, S., et al. (2019)). Ontogenetic variation in diet and habitat of Irrawaddy dolphins (Orcaella brevirostris) in the Gulf of Thailand and the Andaman Sea. Mar. Mamm. Sci. 35, 492–521. doi: 10.1111/mms.12547
Kannan, K., Ramu, K., Kajiwara, N., Sinha, R. K., and Tanabe, S. (2005). Organochlorine pesticides, polychlorinated biphenyls, and polybrominated diphenyl ethers in Irrawaddy dolphins from India. Arch. Environ. Contam. Toxicol. 49, 415–420. doi: 10.1007/s00244-005-7078-6
Kuit, S. H., Ponnampalam, L. S., Ng, J. E., Chong, V. C., and Then, A. Y. H. (2019). Distribution and habitat characteristics of three sympatric cetacean species in the coastal waters of Matang, Perak, Peninsular Malaysia. Aquat. Conserv. Mar. Freshw. Ecosyst. 29, 1681–1696. doi: 10.1002/AQC.3121
Kutner, M. H., Nachtsheim, C. J., Neter, J., and Li, W. (2005). Applied Linear Statistical Models, Vol. 5. New York: McGraw-Hill Irwin.
Learmonth, J. A., MacLeod, C. D., Santos, M. B., Pierce, G. J., Crick, H. Q. P., and Robinson, R. A. (2006). Potential effects of climate change on marine mammals. Oceanogr. Mar. Biol. 44, 431–464. doi: 10.1016/j.envint.2009.10.008
Liu, C., Berry, P. M., Dawson, T. P., and Pearson, R. G. (2005). Selecting thresholds of occurrence in the prediction of species distributions. Ecography 28, 385–393. doi: 10.1111/j.0906-7590.2005.03957.x
Liu, C., White, M., and Newell, G. (2009). “Measuring the accuracy of species distribution models: a review,” in Proceedings of the 18th World IMACs/MODSIM Congress, Cairns, 4241,4247.
Mahmud, A. I., Jaaman, S. A., Muda, A. M., Muhamad, H. M., Zhang, X., and Scapini, F. (2018). Population estimation, distribution, and habitat preference of Irrawaddy dolphins Orcaella brevirostris (Owen in Gray, 1866) in the Brunei Bay, Malaysian waters. Wildl. Biol. 2018:00383. doi: 10.2981/wlb.00383
Marine Conservation Cambodia [MCC] (2016). Marine fisheries management area. Fisheries Administration, Fisheries Conservation Department. Available online at: www.marineconservationcambodia.org/kep-reports/file/32-kep-mfma-revised-finaldraft.pdf (accessed August 7, 2020).
McCluskey, S., Bejder, L., and Loneragan, N. (2016). Dolphin prey availability and calorific value in an estuarine and coastal environment. Front. Mar. Sci. 3:30. doi: 10.3389/fmars.2016.00030
Merow, C., Smith, M. J., and Silander, J. A. Jr. (2013). A practical guide to MaxEnt for modeling species’ distributions: What it does, and why inputs and settings matter. Ecography 36, 1058–1069. doi: 10.1111/j.1600-0587.2013.07872.x
Miller, P., Groom, S., McManus, A., Selley, J., and Mironnet, N. (1997). “Panorama: a semi-automated AVHRR and CZCS system for observation of coastal and ocean processes,” in Proceedings of the Remote Sensing Society, Reading, 539–544.
Minton, G., Peter, C., and Tuen, A. A. (2011). Distribution of small cetaceans in the nearshore waters of Sarawak, East Malaysia. Raffles Bull. Zool. 59, 91–100.
Minton, G., Smith, B. D., Braulik, G. T., Kreb, D., Sutaria, D., and Reeves, R. R. (2017). Orcaella brevirostris (errata version published in 2018). IUCN Red List Threatened Species 2017:e.T15419A123790805. doi: 10.2305/IUCN.UK.2017-3
Muscarella, R., Galante, P. J., Soley-Guardia, M., Boria, R. A., Kass, J. M., Uriarte, M., et al. (2014). ENM eval: an R package for conducting spatially independent evaluations and estimating optimal model complexity for Maxent ecological niche models. Methods Ecol. Evol. 5, 1198–1205. doi: 10.1111/2041-210X.12261
O’Donoghue, S. H., Drapeau, L., and Peddemors, V. M. (2010). Broad-scale distribution patterns of sardine and their predators in relation to remotely sensed environmental conditions during the KwaZulu-Natal sardine run. Afr. J. Mar. Sci. 32, 279–291. doi: 10.2989/1814232X.2010.501584
Parra, G. J. (2005). Behavioural Ecology of Irrawaddy, Orcaella brevirostris (Owen in Gray, 1866), and Indo-Pacific Humpback Dolphins, Sousa Chinensis (Osbeck, 1765), in Northeast Queensland, Australia: A Comparative Study. Ph.D. dissertation. Douglas: James Cook University.
Parra, G. J., Schick, R., and Corkeron, P. (2006). Spatial distribution and environmental correlates of Australian snubfin and Indo-Pacifichumpback dolphins. Ecography 29, 396–406.
Pearson, R. G. (2007). Species’ distribution modeling for conservation educators and practitioners. Synth. Am. Mus. Natl. Hist. 50, 54–89.
Perrin, W., Reeves, R., Dolar, M., Jefferson, T., Marsh, H., Wang, J., et al. (2005). Report of the Second Workshop on The Biology and Conservation of Small Cetaceans and Dugongs of South East Asia. CMS Technical Series Publication No. 9. Paper Presented at the Meeting of the Scientific Committee of the International Whaling Commission SC/66b/SM09, Bonn.
Perrin, W. F., Leatherwood, S., and Dolar, M. L. L. (1995). The status of marine mammal research in Southeast Asia. IBI Reports 5, 1–16. doi: 10.14203/mri.v39i1.80
Peter, C., Ngeian, J., Minton, G., Zulkifli Poh, A., Grinang, J., and Tuen, A. (2016a). Artisanal Fisheries and Cetaceans in Kuching Bay, Sarawak, East Malaysia: Threats and potential mitigation (SC/66b/SM09). Report Presented to the Meeting of the Scientific Committee of the International Whaling Commission. Cambridge: International Whaling Commission.
Peter, C., Poh, A. N. Z., Ngeian, J., Tuen, A. A., and Minton, G. (2016b). “Identifying habitat characteristics and critical areas for Irrawaddy dolphin, Orcaella brevirostris: implications for conservation,” in Naturalists, Explorers and Field Scientists in South-East Asia and Australasia, eds I. Das and A. A. Tuen (Cham: Springer), 225–238. doi: 10.1007/978-3-319-26161-4_15
Peterson, A. T. (2011). Ecological niche conservatism: a time-structured review of evidence. J. Biogeogr. 38, 817–827. doi: 10.1111/j.1365-2699.2010.02456.x
Phillips, S. J., Anderson, R. P., and Schapire, R. E. (2006). Maximum entropy modeling of species geographic distributions. Ecol. Model. 190, 231–259. doi: 10.1016/j.ecolmodel.2005.03.026
Phillips, S. J., Dudík, M., and Schapire, R. E. (2020). Maxent Software for Modeling Species Niches and Distributions (Version 3.4.1). Available online at: http://biodiversityinformatics.amnh.org/open_source/maxent (accessed May 22, 2021)
Ponnampalam, L. S., Hines, E. M., Monanunsap, S., Ilangakoon, A. D., Junchompoo, C., Adulyanukosol, K., et al. (2013). Behavioral observations of coastal Irrawaddy dolphins (Orcaella brevirostris) in Trat province, eastern Gulf of Thailand. Aquat. Mamm. 39, 401–408. doi: 10.1578/AM.39.4.2013.401
QGIS Development Team (2018). QGIS Geographic Information System. Open Source Geospatial Foundation Project. Available online at: http://qgis.osgeo.org (accessed January 10, 2020).
R Core Team (2020). R: A Language and Environment for Statistical Computing. Available online at: https://www.r-project.org/ (accessed March 20, 2020).
Read, A. J. (2008). The looming crisis: interactions between marine mammals and fisheries. J. Mammal. 89, 541–548. doi: 10.1644/07-MAMM-S-315R1.1
Redfern, J. V., Ferguson, M. C., Becker, E. A., Hyrenbach, K. D., Good, C., Barlow, J., et al. (2006). Techniques for cetacean–habitat modeling. Mar. Ecol. Prog. Ser. 310, 271–295. doi: 10.3354/meps310271
Reeves, R. R., Smith, B. D., Crespo, E. A., and di Sciara, G. N. (2003). Dolphins, Whales, and Porpoises: 2002-2010 Conservation Action Plan for the World’s Cetaceans, Vol. 58. Gland: IUCN. doi: 10.2305/IUCN.CH.2003.SSC-AP.2.en
Reid, A. E. A., Haissoune, A., and Ferber, P. (2019). The status of coral reefs and seagrass meadows in the Kep Archipelago. Cambodian J. Nat. Hist. 2019, 24–39.
Robinson, L. M., Elith, J., Hobday, A. J., Pearson, R. G., Kendall, B. E., Possingham, H. P., et al. (2011). Pushing the limits in marine species distribution modelling: lessons from the land present challenges and opportunities. Glob. Ecol. Biogeogr. 20, 789–802. doi: 10.1111/j.1466-8238.2010.00636.x
Santos, M. B., German, I., Correia, D., Read, F. L., Cedeira, J. M., Caldas, M., et al. (2013). Long-term variation in common dolphin diet in relation to prey abundance. Mar. Ecol. Prog. Ser. 481, 249–268. doi: 10.3354/meps10233
Searcy, C. A., and Shaffer, H. B. (2016). Do ecological niche models accurately identify climatic determinants of species ranges? Am. Nat. 187, 423–435. doi: 10.1086/685387
Secretariat of the Convention on Biological Diversity [SCBD] (2010). COP-10 Decision X/2. Secretariat of the Convention on Biological Diversity, Nagoya. Available online at: https://www.cbd.int/doc/decisions/cop-10/cop-10-dec-02-en.pdf (accessed August 7, 2020).
Silber, G. K., Lettrich, M. D., Thomas, P. O., Baker, J. D., Baumgartner, M., Becker, E. A., et al. (2017). Projecting marine mammal distribution in a changing climate. Front. Mar. Sci. 4:413. doi: 10.3389/fmars.2017.00413
Smith, B. D., Ahmed, B., Mowgli, R. M., and Strindberg, S. (2008). Species occurrence and distribution ecology of nearshore cetaceans in the Bay of Bengal, Bangladesh, with abundance estimates for Irrawaddy dolphins Orcaella brevirostris and finless porpoise Neophocaena phocaenoides. J. Cetacean Res. Manage. 10, 45–58.
Smith, B. D., Braulik, G., Strindberg, S., Mansur, R., Diyan, M. A. A., and Ahmed, B. (2009). Habitat selection of freshwater-dependent cetaceans and the potential effects of declining freshwater flows and sea-level rise in waterways of the Sundarbans mangrove forest, Bangladesh. Aquat. Conserv. Mar. Freshw. Ecosyst. 19, 209–225. doi: 10.1002/aqc.987
Sprogis, K., Christiansen, F., Wandres, M., and Bejder, L. (2017). El Niño Southern Oscillation influences the abundance and movements of a marine top predator in coastal waters. Glob. Change Biol. 24, 1085–1096. doi: 10.1111/gcb.13892
Stacey, P. J., and Leatherwood, S. (1997). The Irrawaddy dolphin, Orcaella brevirostris: a summary of current knowledge and recommendations for conservation action. Asian Mar. Biol. 14, 195–214.
Teh, L. S., Teh, L. C., Hines, E., Junchompoo, C., and Lewison, R. L. (2015). Contextualising the coupled socio-ecological conditions of marine megafauna bycatch. Ocean Coast. Manage. 116, 449–465. doi: 10.1016/j.ocecoaman.2015.08.019
Thuiller, W., Lafourcade, B., Engler, R., and Araújo, M. B. (2009). BIOMOD–a platform for ensemble forecasting of species distributions. Ecography 32, 369–373. doi: 10.1111/j.1600-0587.2008.05742.x
Tobeña, M., Prieto, R., Machete, M., and Silva, M. A. (2016). Modeling the potential distribution and richness of cetaceans in the Azores from fisheries observer program data. Front. Mar. Sci. 3:202. doi: 10.3389/fmars.2016.00202
Tsujimoto, K., Ohta, T., Aida, K., Tamakawa, K., and Im, M. S. (2018). Diurnal pattern of rainfall in Cambodia: its regional characteristics and local circulation. Prog. Earth Planet. Sci. 5:39. doi: 10.1186/s40645-018-0192-7
Tubbs, S. E., Baş, A. A., Côté, G., Jones, A. L., and Notman, G. (2019). Sighting and stranding reports of Irrawaddy dolphins (Orcaella brevirostris) and Dugongs (Dugong dugon) in Kep and Kampot, Cambodia. Aquat. Mamm. 45, 563–568. doi: 10.1578/AM.45.5.2019.563
Tubbs, S. E., Keen, E., Jones, A. L., and Thap, R. (2020). On the Distribution, Behaviour and Seasonal Variation of Irrawaddy Dolphins (Orcaella brevirostris) in the Kep Archipelago, Cambodia. Raffles Bull. Zool. 68, 137–149. doi: 10.26107/RBZ-2020-0015
Verutes, G. M., Johnson, A. F., Caillat, M., Ponnampalam, L. S., Peter, C., Vu, L., et al. (2020). Using GIS and stakeholder involvement to innovate marine mammal bycatch risk assessment in data-limited fisheries. PLoS One 15:e0237835. doi: 10.1371/journal.pone.0237835
Vu, L., Tho, T. A., Hung, N. N., and Duy, L. (2017). Conservation of cetaceans in Kien Giang Biosphere Reserve, Vietnam. Conservation Leadership Program, 3234115. Available online at: https://www.conservationleadershipprogramme.org/media/2017/12/03234115-Conservation-of-Cetacean-in-Kien-Giang-Biosphere-Reserve-Vietnam.pdf (accessed February 5, 2019).
Warren, D. L., and Seifert, S. N. (2011). Ecological niche modeling in Maxent: the importance of model complexity and the performance of model selection criteria. Ecol. Appl. 21, 335–342. doi: 10.1890/10-1171.1
Win, N., and Bu, S. S. H. (2019). Food and feeding aspect of Irrawaddy dolphin Orcaella brevirostris (Owen in gray, 1866) related to fish abundance. Int. J. Fish. Aquat. Stud. 7, 321–326.
Keywords: species distribution, Maxent, biodiversity conservation, seasonality, cetacean habitat model
Citation: Verutes GM, Tubbs SE, Selmes N, Clark DR, Walker P and Clements O (2021) Modeling Seasonal Distribution of Irrawaddy Dolphins (Orcaella brevirostris) in a Transnational Important Marine Mammal Area. Front. Mar. Sci. 8:617921. doi: 10.3389/fmars.2021.617921
Received: 15 October 2020; Accepted: 18 May 2021;
Published: 11 June 2021.
Edited by:
Jeffrey C. Mangel, Prodelphinus, PeruReviewed by:
Gail Schofield, Queen Mary University of London, United KingdomSeverine Methion, Bottlenose Dolphin Research Institute (BDRI), Spain
Copyright © 2021 Verutes, Tubbs, Selmes, Clark, Walker and Clements. This is an open-access article distributed under the terms of the Creative Commons Attribution License (CC BY). The use, distribution or reproduction in other forums is permitted, provided the original author(s) and the copyright owner(s) are credited and that the original publication in this journal is cited, in accordance with accepted academic practice. No use, distribution or reproduction is permitted which does not comply with these terms.
*Correspondence: Gregory M. Verutes, Z3JlZ2dAbWFyZmlzaGVjby5jb20=