- 1Scottish Association for Marine Science, Scottish Marine Institute, Oban, United Kingdom
- 2Marine Institute, Galway, Ireland
- 3Fisheries and Aquatic Ecosystems Branch, Agri-Food and Biosciences Institute, Belfast, United Kingdom
- 4Institute of Marine Research, Bergen, Norway
- 5Bedford Institute of Oceanography, Fisheries and Oceans Canada, Dartmouth, NS, Canada
- 6European Marine Board, Oostende, Belgium
- 7Centre for Environment, Fisheries and Aquaculture Science, Lowestoft Laboratory, Lowestoft, United Kingdom
- 8National Institute of Oceanography and Applied Geophysics – OGS, Trieste, Italy
- 9Irish Seal Sanctuary, Dublin, Ireland
Although frequently suggested as a goal for ecosystem-based fisheries management, incorporating ecosystem information into fisheries stock assessments has proven challenging. The uncertainty of input data, coupled with the structural uncertainty of complex multi-species models, currently makes the use of absolute values from such models contentious for short-term single-species fisheries management advice. Here, we propose a different approach where the standard assessment methodologies can be enhanced using ecosystem model derived information. Using a case study of the Irish Sea, we illustrate how stock-specific ecosystem indicators can be used to set an ecosystem-based fishing mortality reference point (FECO) within the “Pretty Good Yield” ranges for fishing mortality which form the present precautionary approach adopted in Europe by the International Council for the Exploration of the Sea (ICES). We propose that this new target, FECO, can be used to scale fishing mortality down when the ecosystem conditions for the stock are poor and up when conditions are good. This approach provides a streamlined quantitative way of incorporating ecosystem information into catch advice and provides an opportunity to operationalize ecosystem models and empirical indicators, while retaining the integrity of current assessment models and the FMSY-based advice process.
Introduction
Fish stock productivity is affected by random environmental variability, environmental cycles and trends (Britten et al., 2017; Free et al., 2019). Fisheries management often relies on setting annual or multi-annual catch quotas which need to be constrained to avoid depleting the stock, but for economic reasons also need to avoid under-harvesting. This aim is enshrined in the maximum sustainable yield (MSY) concept which is incorporated into international ocean law (UNCLOS Article 61; UNCLOS, 1982), as well as many regional and national fisheries policies. Unexplained environmental fluctuations are generally dealt with by incorporating a degree of precaution in the resulting catch advice, or via the use of probabilistic state-based approaches (Harwood and Stokes, 2003). Fisheries scientists have also long appreciated the potential impact of environmental cycles on stock productivity (Ottersen et al., 2001; Chavez et al., 2003) and pointed out that these “productivity phases” pose a challenge to the concept of MSY (Punt and Smith, 2001), which assumes there is a long-term stationary state that stocks will reach under a particular fishing mortality regime (FMSY). Nevertheless, in theory FMSY values can be adjusted in response to environmentally driven productivity, the challenge being to distinguish a new productivity phase from the short-term environmental variability. In addition, both stochastic environmental variability and cycles can be confounded by longer-term trends. This is the case with anthropogenic increases in atmospheric CO2 levels, resulting in warming over large areas of the global oceans, reductions in pH, changes to ocean stratification patterns, and other secondary effects (Solomon et al., 2009; Henson et al., 2017).
Changing environmental conditions can impact individual species’ spatial ranges (Fredston-Hermann et al., 2020), phenology (Poloczanska et al., 2016), recruitment (Beggs et al., 2014), and growth (Baudron et al., 2014). Taken together, these species impacts may manifest as changes in the whole community (Burrows et al., 2019). There can also be a wide range of indirect impacts, e.g., on primary and secondary productivity (Capuzzo et al., 2018), on predator-prey match-mismatch (Régnier et al., 2017), and on overall prey availability (Alvarez-Fernandez et al., 2015). Such responses at individual, species and community levels can potentially affect both ecosystem structure and functioning (Walters and Christensen, 2019; Ye and Carocci, 2019). Accounting for ecosystem condition in marine management is therefore paramount for the maintenance of healthy ecosystems and the protection of stakeholder interests. Promisingly, recent studies found that one quarter of fish stock assessment models included at least one type of interaction between the assessed species and its ecosystem in the US (Marshall et al., 2019), with 27% of assessments in Canada making recommendations or providing advice based on climate, oceanographic or ecological considerations (Pepin et al., 2018). Thus, whilst some progress is being made in some jurisdictions, ecosystem information has not yet been widely incorporated into tactical fisheries management (Skern-Mauritzen et al., 2016).
Ecosystem-based management (EBM) encapsulates the movement toward a more cooperative and holistic approach to marine resource management (Leslie and McLeod, 2007). Whilst there is no single definition for EBM (Long et al., 2015), its general principles assert that resource managers must acknowledge the complexities and interspecific dynamics of ecological systems whilst accounting for human social and governance objectives (Long et al., 2015). Fisheries specific variations include ecosystem-based fisheries management (EBFM), the ecosystem approach to fisheries (EAF) (Garcia, 2003), and ecosystem approach to fisheries management (EAFM) (Link, 2002; Hall and Mainprize, 2004). EBFM recognizes the combined physical, biological, economic, and social trade-offs affecting the fisheries sector, and the need to consider not only the anthropogenic and environmental factors which impact stocks, but also how stock dynamics impact other components of the ecosystem (Link J., 2010; Link and Browman, 2014). In EAFM, ecosystem factors are included in the assessment of a stock, while the social and political dimensions are not necessarily explicitly addressed (Pitcher et al., 2009; Link and Browman, 2014). EAFM thus represents a small, yet important, step from traditional single-species stock assessment, toward fully holistic EBM.
Within the EU, EBM of all maritime activities is mandated through the Marine Strategy Framework Directive (MSFD) (EC, 2008). For fisheries, Article 13 of the Reformed Common Fisheries Policy (CFP) explicitly calls for implementation of EAFM (EC, 2013). However, movement toward EAFM has been hampered by the mismatch between CFP and MSFD policies in terms of competence, discourse, decision making, and their definitions of EAFM (Ramirez-Monsalve et al., 2016). Furthermore, the CFP fails to state at which level ecosystem concerns should be incorporated into fisheries management (Prellezo and Curtin, 2015). As a consequence, although the science to support ecosystem-level advice has grown, progress toward operational EAFM has been slow (Jennings and Rice, 2011; Patrick and Link, 2015; Ramirez-Monsalve et al., 2016).
The daunting challenge of moving toward a more holistic approach has led to skepticism among critics regarding the ability to operationalize ecosystem advice. Issues that have been raised include whether the existing governance structure can support an ecosystem approach, whether we have enough data to support an ecosystem approach, and whether the models required are too complex or uncertain to be useful (Collie et al., 2016; Patrick and Link, 2015). Underlying all of these issues is the worry that we simply do not know enough to take constructive action. Whilst we deliberate the merits of EBM, the threat of climate change and overexploitation grow (Daw et al., 2009; IPCC, 2019), so that the perceived benefits of moving toward an ecosystem-based approach may soon outweigh the perceived risks of a status quo approach (Pikitch et al., 2004). Despite the questions that impede action, Patrick and Link (2015) assert that perfect knowledge of every process is neither attainable nor needed to implement ecosystem-based actions, and that our existing knowledge of patterns and processes, along with existing modeling tools and approaches, should be more than enough to start implementing an ecosystem approach.
Whilst direct environmental effects have been incorporated into a number of single-species stock assessments, e.g., sea temperature affecting catchability in fisheries surveys, or recruitment time series (Marshall et al., 2019), models representing multiple species or the entire food web have had less uptake – although they have the capacity to produce both the strategic and tactical ecosystem advice to advance EAFM (Plagányi et al., 2014; Collie et al., 2016). Recent years have seen an increase in the capabilities of ecosystem models: those which simulate the interactions between food webs, fisheries, and the environment (Hyder et al., 2015; Heymans et al., 2018; Bryndum-Buchholz et al., 2019; Lotze et al., 2019), alongside a rapid expansion in the ability of oceanographic (Meehl et al., 2014) and climate models (Bauer et al., 2015; IPCC, 2019). These advances have led to the emergence of more capable ecological forecasts (Payne et al., 2017), ecosystem-level explorations into the cumulative impacts of fishing and climate change (Serpetti et al., 2017), and the emergence of multi-species MSY approaches which account for trophic interactions and environmental change (Spence et al., 2020a).
The jump from single-species modeling to ecosystem modeling requires an increase in model complexity and parameters across several classes of data to quantify trophic interactions, life-history properties, environmental signals, fishing activity, and many others, often across both temporal and spatial resolutions (Christensen and Walters, 2004). The development of these complex models has been facilitated by increased computing power and availability of environmental data. There is now a wealth of different ecosystem and multi-species models available (Hyder et al., 2015). Ecopath with Ecosim (EwE) has become one of the most widely used approaches for modeling marine food webs and was developed as a tool for modeling fisheries within an ecosystem context (e.g., Plagányi and Butterworth, 2004; Christensen and Walters, 2011; Coll and Libralato, 2012; Mackinson et al., 2018).
Numerous EwE studies have concluded with the recommendation that ecosystem information should be incorporated into management (e.g., Bentley et al., 2017; Serpetti et al., 2017) but most stop at this point and fail to provide any suggestion of how this should be done. A few EwE studies have provided revised fishing reference points based on multi-species considerations (Baudron et al., 2019), or compared trade-offs between different fishing strategies (Stäbler et al., 2016, 2019), but as far as we know, no EwE model has ever been used to set catch levels.
In Europe and North America there is typically an annual cycle of stock advice and implementation of management measures such as catch quotas. To provide advice on an annual basis across multiple stocks, assessment scientists require models of low or intermediate complexity which rely on minimal assumptions, minimal data, can be run quickly and can be easily updated (Methot, 2009; Plagányi et al., 2014). EwE models are good for highlighting plausible trends in the ecosystem and building an understanding of the underlying relationships which regulate productivity (e.g., Corrales et al., 2017; Bentley et al., 2020). However, input-data uncertainty and structural uncertainty makes their use in providing absolute values for single-species advice problematic (Collie et al., 2016).
In this paper we demonstrate that we do not have to replace the standard assessment methodologies, but rather we propose a way to supplement single-species assessments with strategic ecosystem information derived from ecosystem models.
We present an approach for the incorporation of ecosystem information into catch advice and demonstrate, by testing the approach on Irish Sea herring (Clupea harengus), cod (Gadus morhua), whiting (Merlangius merlangus), and Nephrops (N. norvegicus), how the approach has the potential to encourage more precautionary stock harvest during poor productivity phases and prevent overly cautious yields during high productivity phases. Our proposed approach was co-developed by scientists, stakeholders, and policy advisors aiming to enhance Irish Sea catch advice by accounting for the environmental impacts which had historically hindered stock production and fishing opportunities. We outline the framework applied in the current case study of the Irish Sea to allow further development and testing in other sea regions.
ICES Advice on Catch Options
For the North Atlantic waters under the EU CFP (excluding the Mediterranean), scientific advice on fishing opportunities is provided by ICES (International Council for the Exploration of the Sea) based on two principles under European legislation: MSY and the precautionary approach (Froese and Quaas, 2013). ICES applies the MSY concept to single-species stocks to maximize average long-term yield and maintain productive fish stocks within healthy ecosystems. Despite being on the international agenda for decades, MSY has always been a disputed concept, especially when put forward as the overarching objective of fishery management (Larkin, 1977). Criticisms include its top–down nature, inherent uncertainty, and concerns that it may not be conservative enough to ensure sustainability (Mesnil, 2012). Importantly, estimates of MSY generally assume ecosystem stability (and healthy ecosystems), such that populations will reach a theoretical equilibrium if subjected to a fixed fishing mortality rate (F) in the long-term. Given that marine ecosystems are increasingly affected by climate change, this assumption is less tenable and raises questions first posed in the 1950s about how often MSY values should be revised in response to ecosystem change (Finley and Oreskes, 2013). In addition, the common assumption that stocks fished at MSY will be able to support non-commercial species, including marine mammals and seabirds, is debatable since the required biomass to support predators is typically unknown (Hill et al., 2020). However, MSY conveys a beguilingly simple and politically attractive message (Cushing, 1977; Mesnil, 2012), which has likely led to its adoption in policies including the UN Convention of the Law of the Sea (UNCLOS), US fisheries policy (Finley and Oreskes, 2013) and the EU CFP (EC, 2013). In ICES advice, FMSY, is therefore the fishing mortality that should give long-term MSY from a single stock.
In addition, ICES conforms to the precautionary approach by providing advice for Total Allowable Catches (TAC) based on rules that aim to ‘safeguard’ stocks from overfishing (Figure 1). When spawning stock biomass (SSB) falls below a threshold (MSYBtrigger), the proposed F should be linearly reduced toward zero to enable the stock to rebuild to levels capable of producing MSY, assuming future recruitment follows historical levels. A further reference point, Blim sets the level of SSB where future recruitment is highly likely to be impaired and is based on the lowest SSB where large recruitment has been observed. If SSB falls below Blim, then an F of zero is advised. Because of the historical evolution of the reference points, precautionary reference points are also provided (Bpa and Fpa) to reduce the risk of reaching Blim or the F limit (Flim).
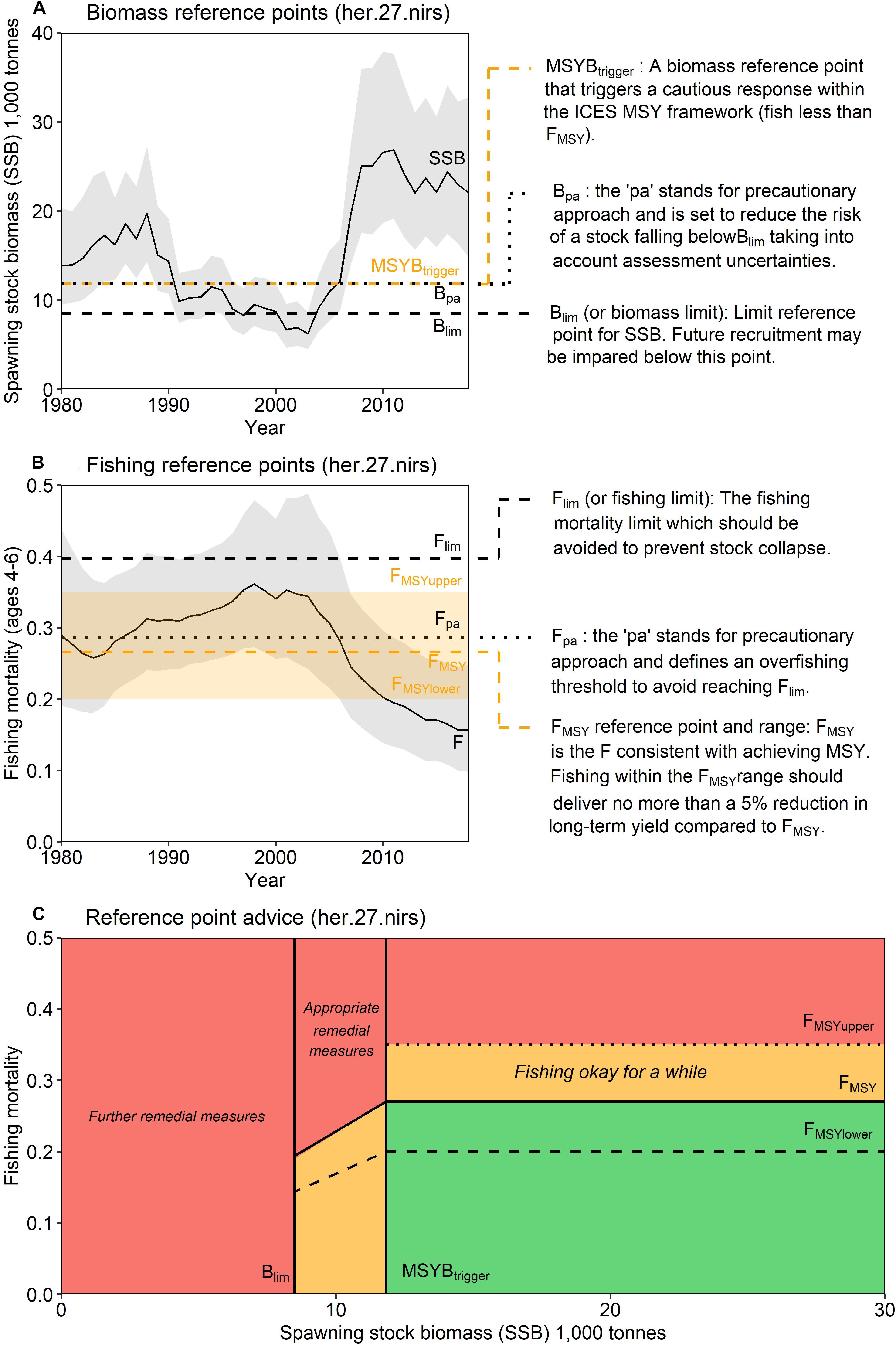
Figure 1. ICES fishing and reference points for Irish Sea herring (her.27.nirs). ICES provide (A) biomass reference points, (B) fishing reference points, (C) and an advice rule to ensure fisheries management advice is consistent with the precautionary principle.
Following concerns that FMSY gave managers insufficient flexibility to manage multispecies fisheries, ICES also now provides advice on plausible values around FMSY for several stocks in the Baltic Sea, North Sea and Western EU waters. These FMSY ranges (FMSYlower to FMSYupper), introduced in 2015, are intended to deliver no more than a 5% reduction in long-term yield compared with the MSY (ICES, 2015c). These ranges are derived through long-term simulation of observed stock dynamics and include appropriate assumptions of biological variability and model error. Precautionary and good yield are still achieved when fishing within the FMSY ranges (if stocks are above MSY Btrigger), however, the provision of an FMSY range introduces flexibility for quota setting. This flexibility is especially useful in mixed fisheries where the catch of one stock is coupled with the unavoidable catch of other stocks, which may or may not have a choke effect under the EU landings obligation (Rindorf et al., 2017; Garcia et al., 2020). However, there are no current guidelines to operationalize the use of FMSY ranges and they have not been widely used. We propose that relevant ecosystem indicators, derived from or identified by ecosystem models, could be used to provide an ecosystem informed target F within these established FMSY ranges. This concept arose from a series of workshops centered on building capacity for an ecosystem-based approach to fishery management for the Irish Sea (ICES, 2020). This approach adds to the utility of the FMSY ranges for mixed fisheries, by providing a precautionary reference point, contingent on the productivity and condition of the wider ecosystem.
The Irish Sea and ICES WKIrish
Irish Sea fisheries have undergone considerable change in recent years following the decline of commercially important finfish stocks and their slow response to management measures invoked through recovery plans. Addressing the challenges facing these fisheries requires a holistic approach, including modeling, to improve ecosystem understanding alongside the refinement of single-species assessment methods. This process was initiated in the first ICES Integrated Benchmark Assessment (WKIrish; ICES, 2015a) which established the WKIrish Framework. The WKIrish framework brought multiple stakeholder groups together, including fishermen, scientists, regulators, environmental non-governmental organizations and other interested parties to enhance fisheries advice and co-develop an operational route for EAFM. The work plan for the WKIrish Framework was a multi-year process focused on improving the single-species stock assessments and advice for the Irish Sea (ICES division VIIa) (Figure 2), incorporating a mixed fisheries model, and developing an approach for the integration of stakeholder knowledge and ecosystem science in order to work toward an integrated assessment1.
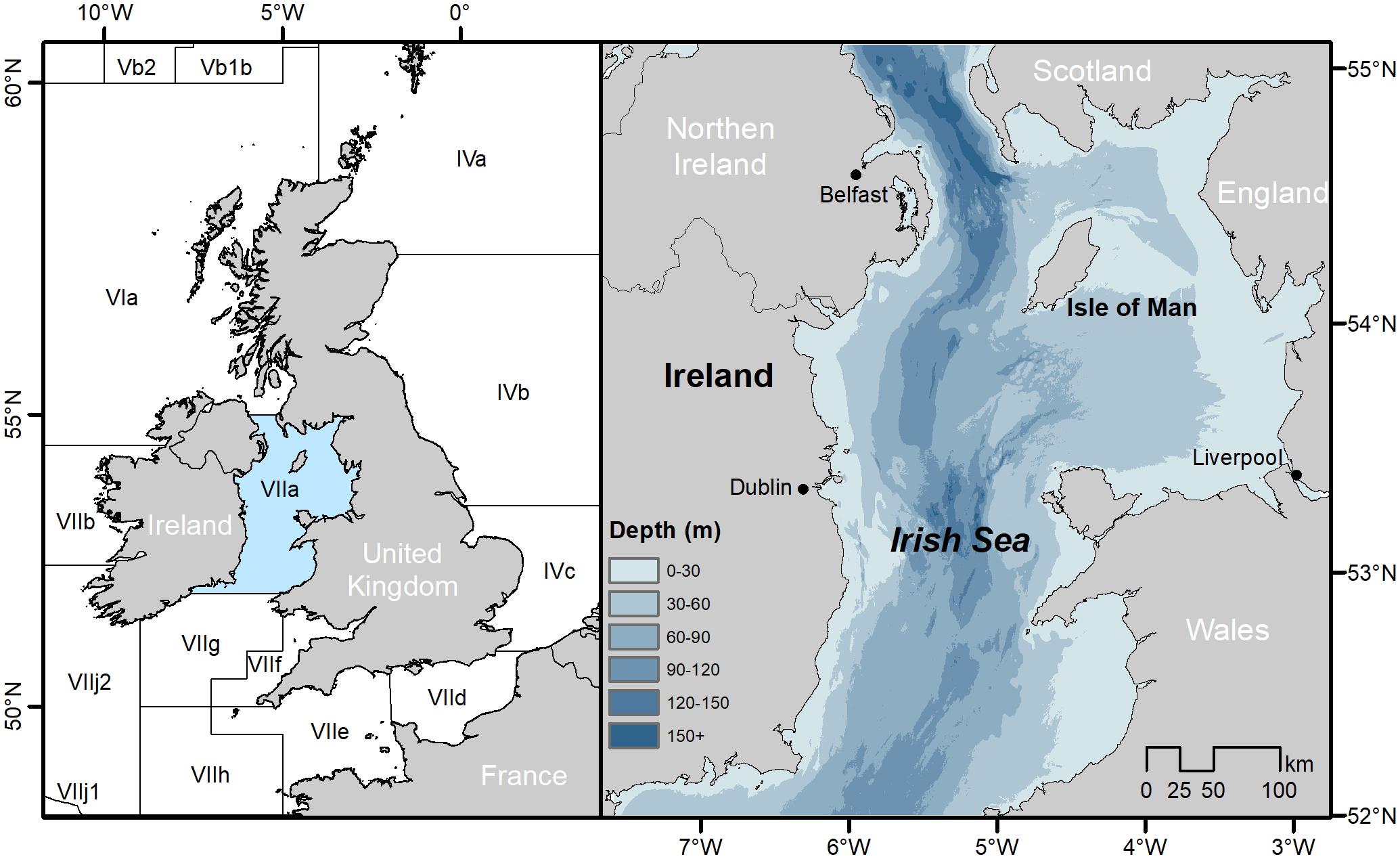
Figure 2. ICES fisheries divisions and the Irish Sea. Map of the United Kingdom and Ireland with the surrounding waters broken down into their ICES advisory areas (left), along with a map of the Irish Sea; ICES division VIIa (right).
The first WKIrish workshop (WKIrish1; ICES, 2015a) centered around the co-design and co-production of knowledge with information exchange between scientists and stakeholder groups concerning ecosystem processes, fisheries, management and policy issues, leading to the identification of data and tools that could be used to progress EBFM in the Irish Sea. Following this, further workshops were held to evaluate the scientific (fisheries) data available for the region (WKIrish2; ICES, 2016), update Irish Sea single-stock assessments (WKIrish3; ICES, 2017), integrate stakeholder knowledge into ecosystem models (WKIrish4; ICES, 2018b), and identify ways to operationalize EAFM for the Irish Sea (WKIrish5 and 6; ICES, 2018a, 2020).
WKIrish also motivated new ecosystem modeling. There are now four multi-species models for the region including an updated Ecopath with Ecosim model (EwE; Bentley et al., 2020), a ‘Length-based Multispecies analysis by numerical simulation’ model (LeMans; Thorpe et al., 2015; Spence et al., 2020b), the Model for the Simulation of Ecological Systems (MoSES; ICES, 2020), and a Fleet and Fisheries Forecast model (FCube; Ulrich et al., 2011; ICES, 2018b). The four models are closely aligned in their purpose yet differ structurally, opening the door for ensemble modeling opportunities such as those seen in the North and Baltic Seas (Spence et al., 2018; Bauer et al., 2019) and globally (Lotze et al., 2019). As a result of the WKIrish Framework, these models have been designed at the science-policy interface where they have benefited from stakeholder input and feedback and critique from the extended research community. Stakeholders were integral participants of all six WKIrish workshops, contributing toward research design, implementation, and dissemination. The EwE model was designed in principle to address questions from the fishing industry who were asking why fish stocks showed little sign of recovery despite their large reductions in fishing effort. Focusing on these questions through WKIrish streamlined the integration of outputs into ICES advice for Irish Sea fisheries, as it specifically addressed the needs of the stakeholder-led North Western Waters Advisory Council (NWWAC). Fishers’ knowledge was used in the co-production of studies on species diets (Bentley et al., 2019a), historical fishing effort (Bentley et al., 2019b), and integrated ecosystem assessments (Pedreschi et al., 2019).
Of the four models, the EwE is the most operationally complete (ICES, 2019c). It was evaluated by the ICES Working Group on Multispecies Assessment Methods (WGSAM) in 2019 and approved as a quality assured reference for scientific input to ICES advice products (ICES, 2019c). Hindcast simulations using the EwE model suggest that historic environmental change did suppress the overall production of several commercial finfish species, limiting opportunities for the fishing industry, whilst also dampening the rates of stock recovery despite marked reductions in fishing effort (Figure 3) (Bentley et al., 2020).
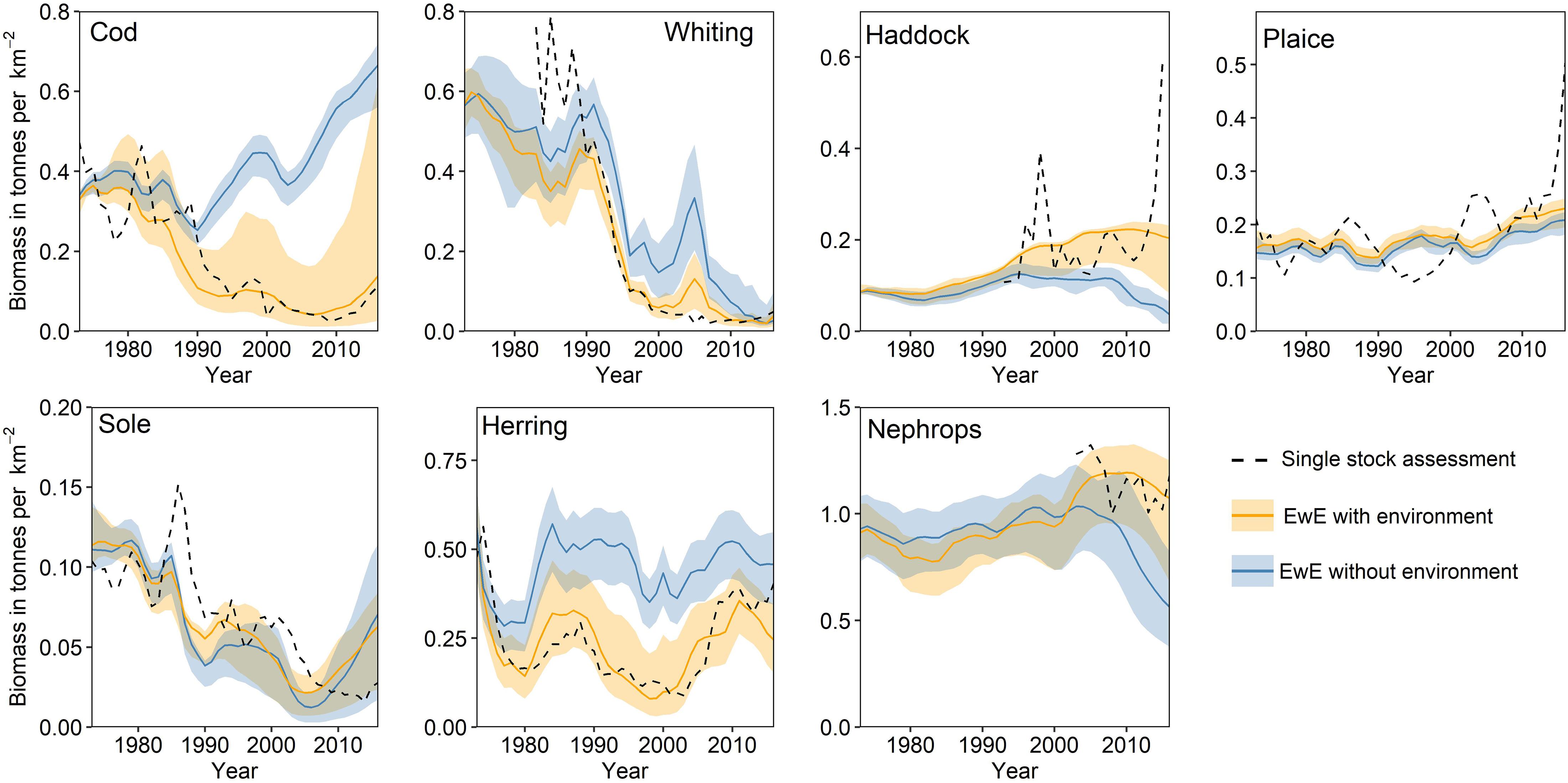
Figure 3. Biomass simulations for commercial stocks in the Irish Sea. Simulations were generated using the Irish Sea EwE key run (ICES, 2019c) with environmental drivers (orange) and without environmental drivers (blue) to discern the role of environmental change in stock trajectories. Solid lines indicate baseline model simulations and shaded areas indicate 95% confidence intervals based on input uncertainty (see Bentley et al., 2020). Dashed lines indicate biomass trends from single-stock assessment models.
During the fifth meeting of WKIrish it was proposed and agreed that ecosystem indicators derived from or identified by the EwE model could be used to inform fishing opportunities for each stock within the pre-defined range of FMSY values (ICES, 2018b). If ecosystem indicators for a stock are in a poor condition, managers should be advised to apply an FMSY at the lower end of the range to minimize the cumulative impact their actions may have on the stock. If indicators suggest that the stock is experiencing favorable ecosystem conditions and stock biomass is above MSY Btrigger, then an F in the upper range could be advised. This approach provides a streamlined, quantitative way to incorporate ecosystem information into an existing management framework following the objectives of the guiding fishery policy (CFP) (EC, 2013).
Ecosystem Advised F (FECO)
FECO Concept and Calculation
Ecosystem advised F, for a given stock, which we refer to as FECO, is a precautionary F within the predefined FMSY range based on the strategic understanding available from ecosystem models. This approach does not require additional complexity in stock assessment models, therefore maintaining their robustness for short-term forecasting of stock trajectories. FECO achieves EAFM and facilitates a move toward EBFM, providing an important step to bring ecosystem information into the advice process. Ecosystem indicators to inform FECO should be selected based on a biological understanding of the stock and the likely mechanism behind the indicator-stock relationship. We note though that mechanisms linking environmental trends with stock production can be unclear and are often inferred from correlation analyses (Zimmermann et al., 2019). However progress has been made toward quantifying the environmental mechanisms driving stock production (e.g., Brill and Lutcavage, 2001; Kristiansen et al., 2014; Régnier et al., 2017). It is important to recognize, however, that correlations can and may break down over time, and causation cannot be inferred.
The potential indicators identified using the Irish Sea EwE model ranged from single observable drivers such as sea surface temperature to more complex emergent food web indicators, such as indices of trophic level. The model was thus applied in two ways to provide ecosystem information: (1) model results were used to identify and recommend important indicators available from other sources (i.e., temperature or zooplankton biomass); and (2) emergent model products were identified as indicators (i.e., trophic indices or predation mortality).
The proposed method for calculating FECO for a given year uses the status of an indicator from that year (Is) relative to its long-term range (i.e., is the indicator high or low relative to values across the time series) which is calculated as:
where Iy is the ecosystem model indicator (I) value in year y, Iymin is the minimum value of I from the time series and Iymax is the maximum value of I from the time series. This equation provides a proportion, between zero (relatively poor status) and one (relatively good status), which ranks how the current status of the indicator compares with previous years, assuming low values of the indicators represent relatively poor status. This would not be the case for negative relationships, such as that seen between cod SSB and temperature (Brander, 1995; Planque and Fox, 1998), where higher spring sea temperatures appear to be linked to reduced subsequent recruitment. In such cases, IS should be calculated as shown in the second condition of Equation 1 so that high indicator values return low IS values. The status of the indicator determines the placement of the FECO reference point within the ICES FMSY range. If only a single indicator is considered and IS is equal to zero, FECO will equal FMSYlower, whilst if IS is equal to one, FECO will equal FMSYupper (Equation 2). Considering the ICES advice rule that FMSY should be linearly reduced toward zero when SSB is below MSY Btrigger, we have added a condition to Equation 2 to keep FECO for the coming year within the precautionary bounds of ICES advice when SSB is ≤MSY Btrigger. Below Blim FECO is equal to 0 following ICES advice:
Which indicator(s) is/are appropriate will vary by stock and environment. During the sixth meeting of WKIrish, candidate indicators were proposed and explored for each of the key Irish Sea stocks, including cod, whiting, haddock (Melanogrammus aeglefinus), sole (Solea solea), plaice (Pleuronectes platessa), herring, and Nephrops (ICES, 2020). The Irish Sea EwE model was used to explore the relationship between the candidate indicators and the EwE derived trends of stock size.
To date, only first order linear relationships have been tested, however, further exploration of more complex relationships may yield different candidate indicators. The production of some stocks may also be linked to multiple indicators. For example, indicators of temperature and predation pressure both correlated with Irish Sea sole SSB. Estimations of the contribution of these drivers to production rate of the stock could form the basis of indicator weightings between zero and one, making it possible to estimate the overall indicator status for the stock:
where IS is the current condition of the weighted set of indicators (Ii, calculated using Equation 1) multiplied by its proportional weighting (αi), where the sum of the weights equals one. Therefore, if temperature had a stronger effect on the production of sole than predation pressure, the overall indicator score (IS) could be weighted in favor of the current temperature.
The values of the indicator should be updated as soon as possible when derived from environmental time series or, in the case of model derived metrics should be updated through regular re-evaluation of the ecosystem models. For example, ICES review and update data, assessment methodologies, reference points, and models every 3–5 years during workshops known as benchmarks. During ICES benchmarks, the relationships between ecosystem indicators and stock production should be reviewed to determine if they are still valid.
FECO Example
The FECO approach is demonstrated here for four commercial Irish Sea stocks. During WKIrish6, experts used the EwE model and outputs and analysis from Bentley et al. (2020) to identify suitable FECO indicators for herring, cod, whiting and Nephrops (ICES, 2020). The process through which indicators were identified (demonstrated in Figure 4) involved identifying the drivers underpinning stock dynamics in the EwE model based on how their inclusion in the model impacted the statistical fit of model simulations to observed data. Herring production in the Irish Sea has been influenced by the top down impact of fishing but also the availability of large zooplankton (>2 mm), a functional group in the EwE model consisting mostly of Calanus spp. which are the main prey of herring (Rice, 1963). The large zooplankton biomass indicator, available from the Continuous Plankton Recorder (CPR) survey (Reid et al., 2003) (Figure 4D) was thus selected to be used in the calculation of herring FECO. Sea surface temperature was identified as an indicator of stock production for both cod and whiting (ICES, 2020). The identified relationship between temperature and SSB was improved by lagging the SST (3 years) to align the environmental condition with stock recruitment. Finally, the abundance of higher trophic levels (4+) was strongly and negatively related to the stock production of Nephrops, indicating higher predation when this indicator was high. Zooplankton and SST indicators were derived from primary sources (such as the CPR survey). The trophic level 4+ indicator used in calculation of FECO for Nephrops was taken as a direct EwE output.
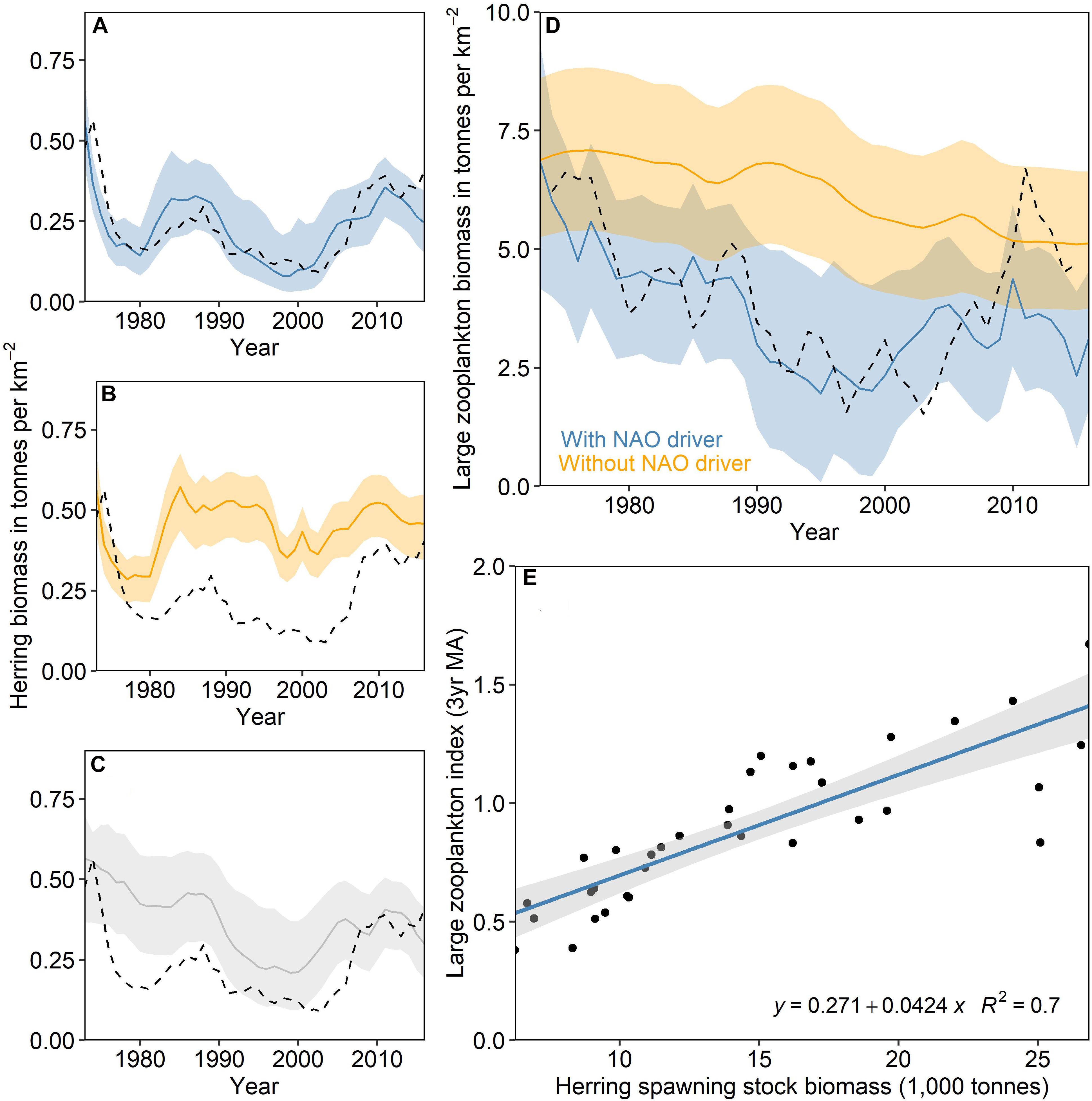
Figure 4. Relationship between large zooplankton biomass and Irish Sea herring production. The key drivers of Irish Sea herring biomass in the Irish Sea EwE model are: (i) fishing pressure, and (ii) the biomass trend of large zooplankton. (A) The solid line indicates the model simulation, the shaded area is the 95% uncertainty bounds based on input data uncertainty, and the dashed line is the single-stock assessment. (B) Agreement between EwE simulations and stock assessment results decreased when large zooplankton biomass was not well replicated (i.e. the NAOw is not used as a driver of zooplankton mortality) and (C) fishing mortality is not accounted for. (D) Large zooplankton biomass simulations are improved when production is driven by the North Atlantic Oscillation (dashed line is the index of large zooplankton (>2 mm) from the Continuous Plankton Recorder (CPR) with a three-year moving average). (E) Returning to the ‘raw’ data, a positive linear relationship is identified between herring SSB and CPR large zooplankton index with a 3-year moving average.
Equation 1 was used to calculate the status of each indicator (IS) for 2016, which was the final year of the EwE model, and Equation 2 was used to scale FMSY to produce FECO reference points for 2016 (Figure 5). The ecosystem model was driven under three F scenarios for each stock: (1) the actual annual F as calculated in the most recent stock assessment, (2) the FECO advice rule was followed, with F being adjusted on an annual basis in-line with the retrospective status of the stock-specific ecosystem indicators described above, and (3) FMSY held constant (with no advice rule applied) across the entire simulation. We also calculated the summed catch (across the entire time series) and interannual variation (IAV) of the catch for each scenario. The IAV for each fishing scenario was calculated as:
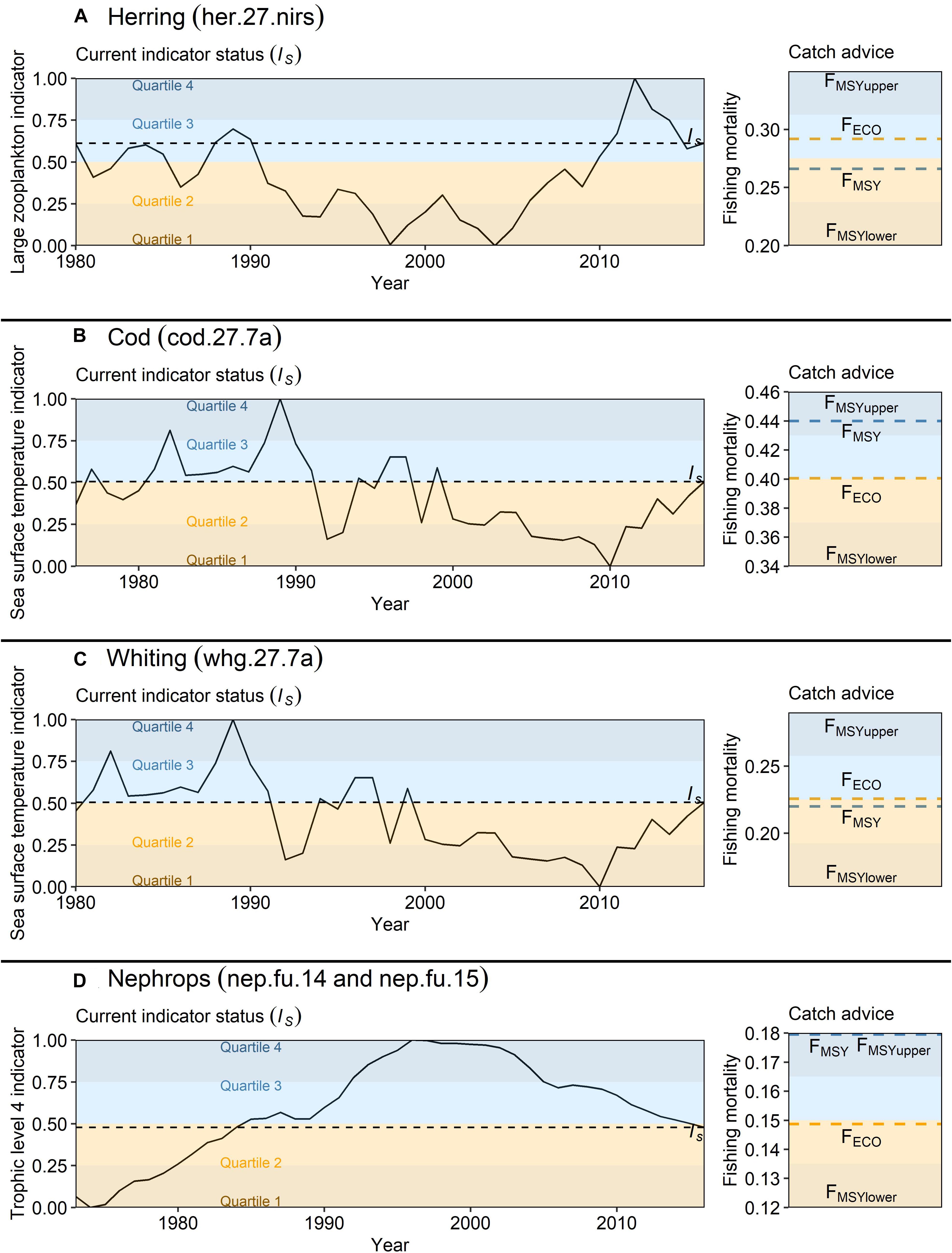
Figure 5. From indicator to FECO. Examples are presented for Irish Sea (A) herring with large zooplankton biomass as an indicator, (B) cod with inverted sea surface temperature (SST; 3-year lag) as an indicator, (C) whiting with SST (3-year lag) as an indicator, and (D) Nephrops with the inverted biomass of trophic level 4+ as an indicator. On the left, all indicators are rescaled between zero and one to provide a percentile value which ranks the status of the indicator (IS) in 2016 compared with previous years. On the right, the status of the indicator determines the placement of the FECO reference point within ICES FMSY ranges.
where n is the number of years (y) in the simulation and Cy is the catch during year y (A’mar et al., 2010).
Herring
Adjusting herring FMSY in line with the condition of the large zooplankton indicator reduced catches during poor ecosystem conditions and encouraged higher catches during periods of good ecosystem condition (Figure 6). The largest divergence in catches between scenarios was seen in 2011, where catches under the FECO scenario were 25% greater than those under the FMSY scenario and 57% greater than those from the actual F scenario. When compared with FECO, the stationary FMSY target led to lower biomasses during periods of low productivity and resulted in overly cautious harvest during periods of high productivity. Simulated herring biomass was higher using FECO, than when using FMSY or actual F during the periods of lower productivity (less zooplankton), and this was reversed in periods of higher zooplankton abundance. Summed catches were similar across scenarios, however, IAV was higher under FECO due to the relatively erratic zooplankton indicator trend.
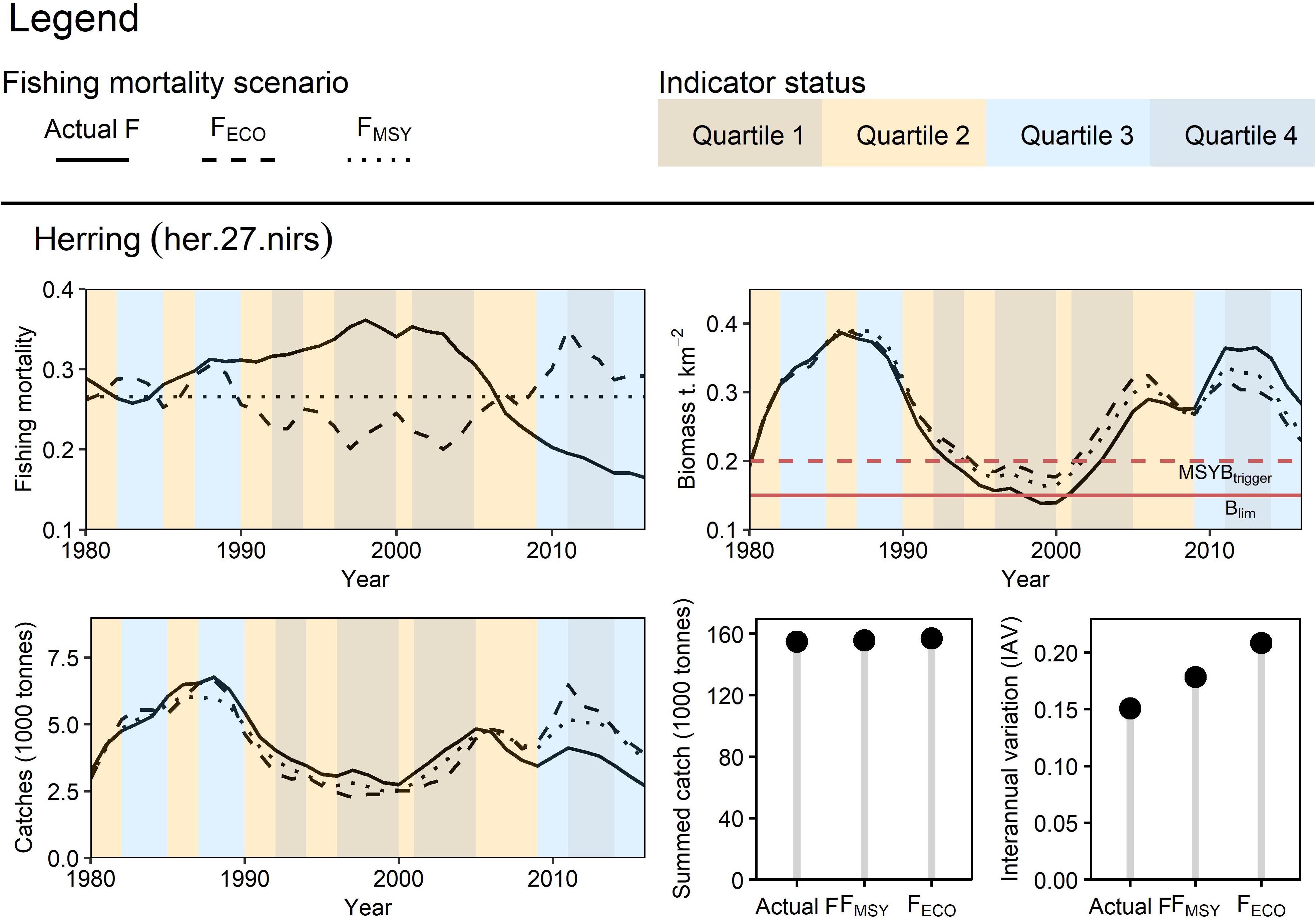
Figure 6. Retrospective application of FECO for Irish Sea herring: Three fishing mortality scenarios were prepared to simulate Irish Sea herring dynamics from 1980 to 2016. FECO was retrospectively calculated based on the condition of the large zooplankton indicator. The different fishing mortality trends were applied to herring in the EwE model to simulate their effect on the stock’s biomass and catch (trend, total yield, and interannual variation).
Cod
Fishing at FECO for cod, which was dynamic in response to SST changes (inverted trend with a 3-year lag), and stationary FMSY led to generally higher catches across the entire time series (Figure 7). This is because the lower initial F and catches allowed for a more productive cod stock in the long-term. As with herring, FECO proved to be the more precautionary scenario of the three as it resulted in higher biomasses following the reduction of fishing mortality during poor ecosystem conditions. We see this most prominently from the year 2000 onwards, where FECO was reduced due to high temperatures. The disparity between FECO and FMSY scenarios is greatest around the years 2010 to 2012, where biomass and catches are on average 58 and 35% higher respectively in the FECO scenario. The IAV at FECO was less than the actual F but still more than FMSY due to the addition of environmental variability.
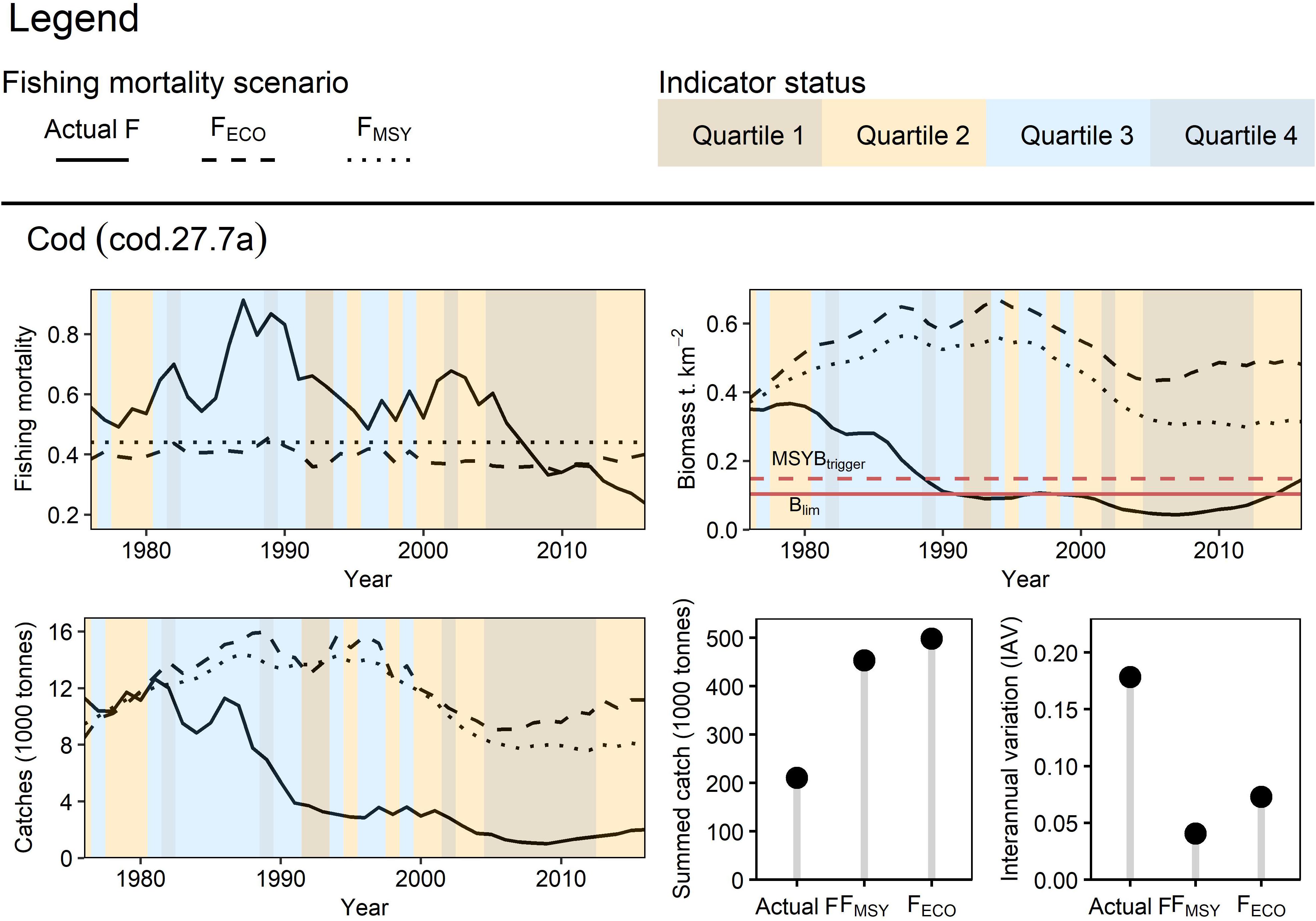
Figure 7. Retrospective application of FECO for Irish Sea cod: Three fishing mortality scenarios were prepared to simulate Irish Sea cod dynamics from 1976 to 2016. FECO was retrospectively calculated based on the condition of the sea surface temperature (3-year lag) indicator. The different fishing mortality trends were applied to cod in the EwE model to simulate their effect on the stock’s biomass and catch (trend, total yield, and interannual variation).
Whiting
Retrospective simulations suggest that fishing whiting at FECO or FMSY across the time series would have produced more stable long-term yield and kept the stock above MSYBtrigger (Figure 8). Fishing at the actual F led to higher summed catches however this scenario was unsustainable and led to the severe overexploitation (biomass < Blim) of the stock. There are few differences between the FECO and FMSY biomass and catch projections, however, like cod, fishing at FECO led to higher stock biomasses from 2000 onwards by reducing F during poor indicator conditions. Similarly to cod, the IAV at FECO was less than the actual F but greater than FMSY.
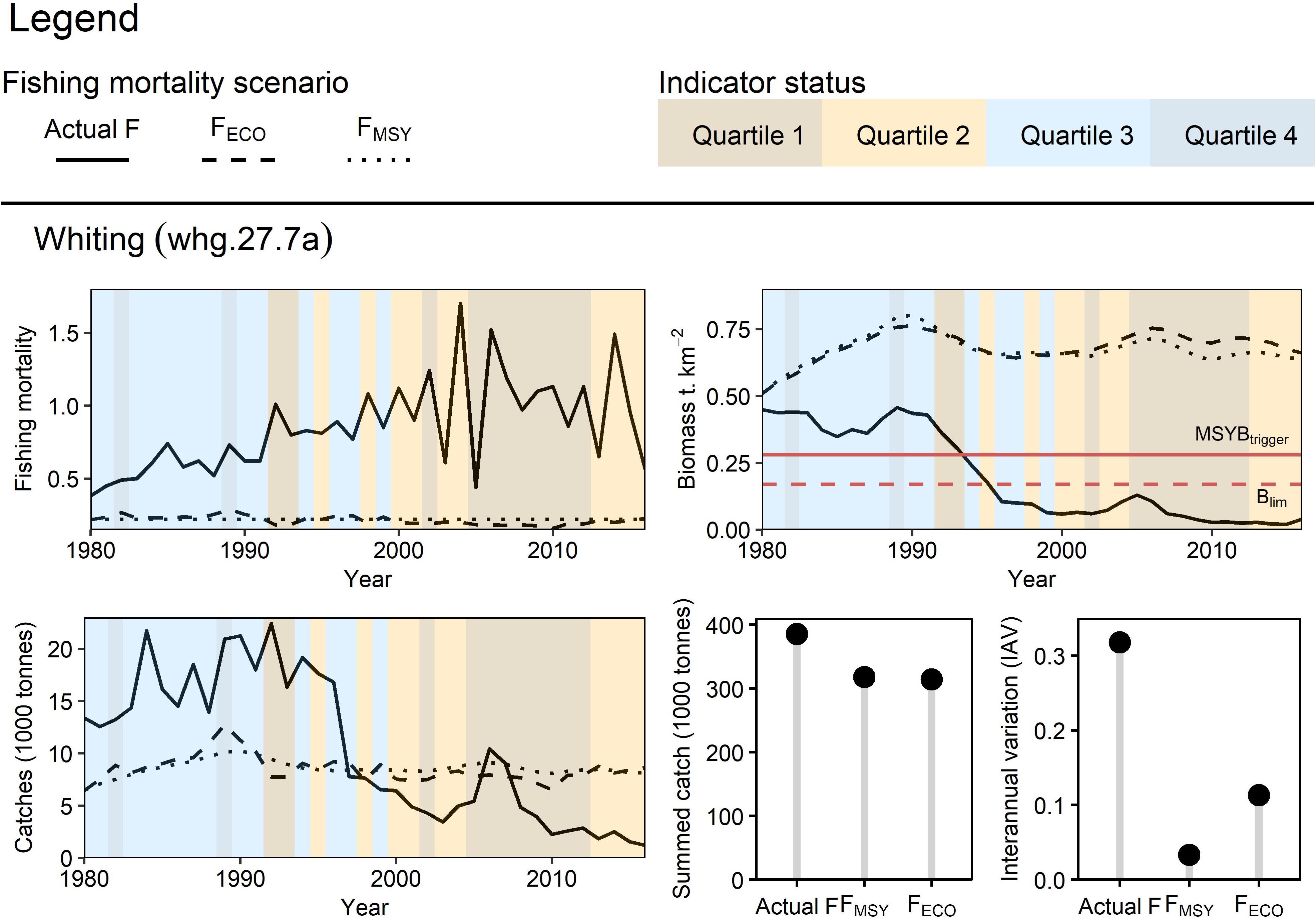
Figure 8. Retrospective application of FECO for Irish Sea whiting: Three fishing mortality scenarios were prepared to simulate Irish Sea whiting dynamics from 1976 to 2016. FECO was retrospectively calculated based on the condition of the sea surface temperature (3-year lag) indicator. The different fishing mortality trends were applied to whiting in the EwE model to simulate their effect on the stock’s biomass and catch (trend, total yield and interannual variation).
Nephrops
Nephrops catches when fishing at FECO were often lower when compared to catches at FMSY or actual F, however, biomass was higher as a result (Figure 9). Catches under all scenarios followed similar trajectories at a similar magnitude, suggesting that fishers may have seen similar short-term and long-term yields at a reduced effort due to the higher stock biomass when fishing at FECO. It is important to note that the EwE model does not explicitly account for limiting factors such as space for burrows, therefore additional analyses would be needed to affirm whether fishing at FECO would have facilitated a higher stock biomass. Nephrops IAV was much lower when fished at FECO and FMSY compared to the actual F.
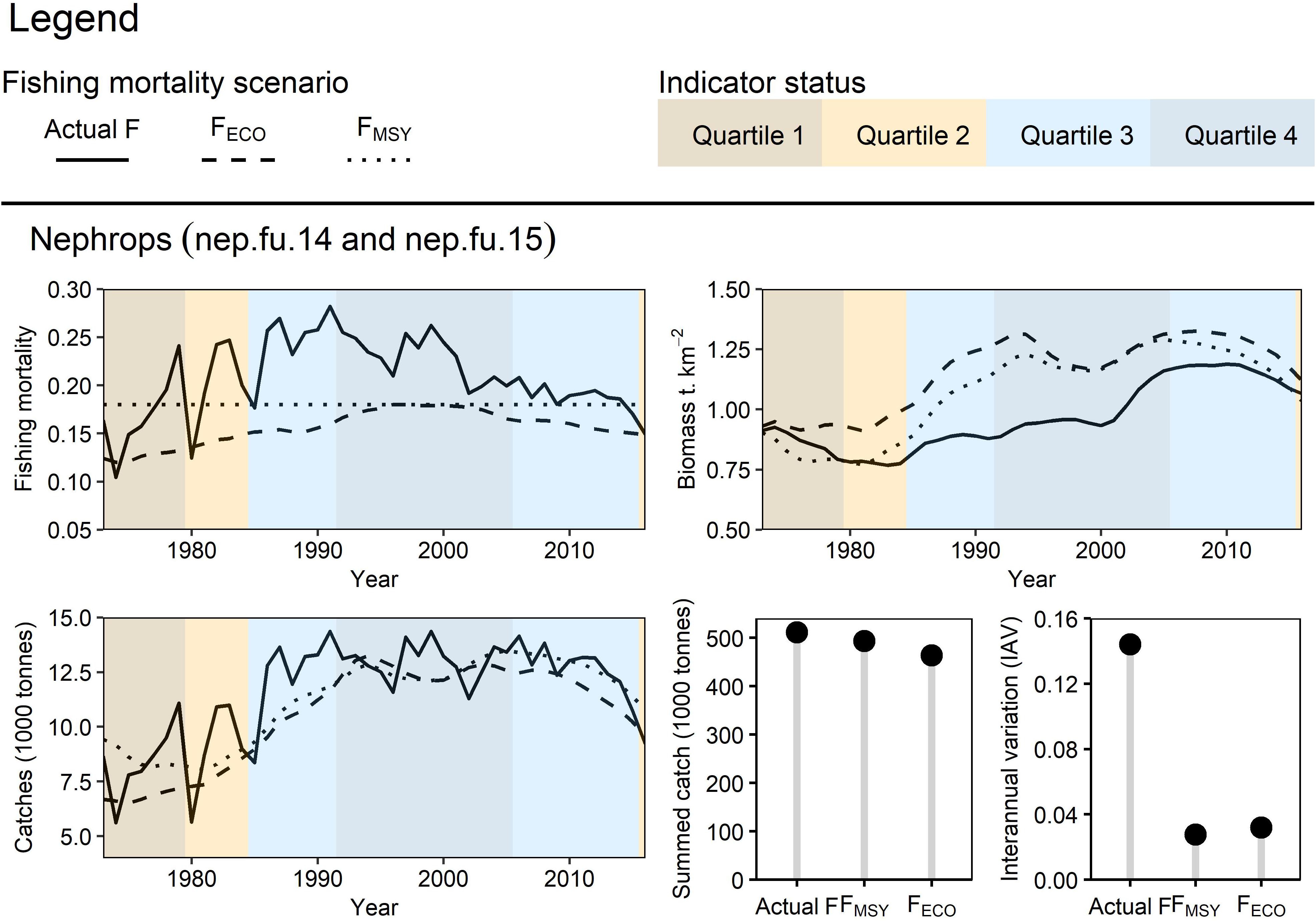
Figure 9. Retrospective application of FECO for Irish Sea Nephrops: Three fishing mortality scenarios were prepared to simulate Irish Sea Nephrops dynamics from 1976-2016. FECO was retrospectively calculated based on the condition of the trophic level 4+ biomass indicator. The different fishing mortality trends were applied to Nephrops in the EwE model to simulate their effect on the stock’s biomass and catch (trend, total yield, and interannual variation).
Discussion
This study introduces the FECO approach, which provides a quantitative mechanism for incorporating ecosystem information from empirical and model-derived indicators into the ICES single-species stock assessment process. The FECO concept helps deliver EAFM and is a stepping-stone toward EBFM, identifying a pragmatic, transparent route by which ecosystem information can be immediately incorporated into the current single-species stock assessment process without the need to revise any existing ICES protocols. The existing ICES protocols and their adoption by managers are part of a well-established process, some of which is set in the international legislation (e.g., MSY). Changing the system is not easy, and the use of more holistic models in practice has been hampered by their complexity and (to date) difficulty in adhering to the precautionary principle. Using the FECO approach, ecosystem processes can be accounted for without violating existing single-species precautionary reference points and while retaining ‘good yield.’ Furthermore, by using the ecosystem information to refine the target F in this way, quota setting can still be conducted within the single-species assessment models.
Fishing at FECO offered a more precautionary harvest approach for all stocks during periods of poor environmental conditions when compared to actual F or FMSY as, when fished at FECO, stock biomasses showed slower declines and earlier recoveries in the face of adverse conditions. Our results indicate that adapting the chosen F to changes in ecosystem indicators, even within F ranges predefined by single-stock assessment models, enhances the responsiveness of management to changes in stock production. The interannual variation (IAV) was much lower for the FECO scenario than the actual F scenario for all stocks except herring, although compared to the constant FMSY scenario it tended to be higher. This variability was linked to the variability in the stock specific environmental indicators, with the large zooplankton indicator simulating the greatest variability, followed by SST and then trophic level 4+ biomass. While increased variability may make it more difficult to adapt fishing strategies on an annual basis, the use of indicators such as SST with a 3-year lag allows us to project where FECO sits within the reference points range for the next few years, giving management more time to prepare.
Harvesting at FECO can be assumed not to increase the risk of stock collapse beyond that already included in the existing management system since it lies within the precautionary FMSY range. Simulations suggest that fishing at FECO could potentially reduce the risk of stock collapse by reducing exploitation during poor productivity phases. There is a risk that the indicators identified through model simulations do not represent reality, or relationships may break down over time. This should not detrimentally impact the stock, as F will remain within the (precautionary) FMSY ranges, however, the potential benefits of a more precautionary approach may not be realized. This risk can be best managed by frequently revisiting the FECO reference point and updating indicator time series as part of the annual assessment or the established stock benchmark processes.
The main limitations of using EwE to support the FECO approach are linked to: (1) the heavy data requirements, and (2) the question of whether the model is capturing real patterns. With more data, the predictive capacity of ecological models tends to improve as they can capture variability across interannual cycles and multidecadal shifts (Giron-Nava et al., 2017). However, if the model only covers a short time period due to data availability, the possibility that the environmental indicator will move out of the modeled range is increased, which may lead to unexpected changes. We did not include parameter or structural uncertainty in our simulations, which can lead to poor, or no, representation of some ecosystem processes and uncertainty in specific model outcomes. This limitation can be addressed through the use of Monte Carlo analysis to capture the impacts of parameter uncertainty (Kennedy and O’Hagan, 2001; Steenbeek et al., 2018) and ensemble modeling approaches (e.g., Spence et al., 2018; Lotze et al., 2019) which exploit the strengths of different modeling approaches to provide more robust outcomes and a deeper understanding of structural uncertainties. The WKIrish process has four potential models now at different stages of development, including EwE. We note a third limitation of this approach that relates to its application to data-limited stocks. While ICES provides advice on more than 260 stocks on an annual basis, it is estimated that more than sixty percent of these stocks have insufficient data to support short-term forecasts and MSY-based advice (ICES, 2019b). The approach for calculating FECO relies on the availability of MSY-based advice, meaning, in its current capacity, it may not be directly applicable to data-limited stocks without FMSY ranges. However, in the case of the current advice framework for stocks outside of the FMSY approach, arbitrary values are applied to ensure precautionary advice is provided. The concept of an ecosystem indicator within this process may provide a more justifiable advice framework, although this would require further testing.
Streamlining Ecosystem Model Outputs for Catch Advice
WKIrish facilitated the operational use of EwE through regional coordination of the ICES benchmark process. This process brought together industry stakeholders, policy advisors, eNGO’s, biologists, fishery scientists, multi-species/ecosystem modelers, social scientists, and stock assessment experts. Drawing on the experience of WKIrish, we outline four key lessons which have been essential in our aim to advance EAFM for the Irish Sea (Figure 10).
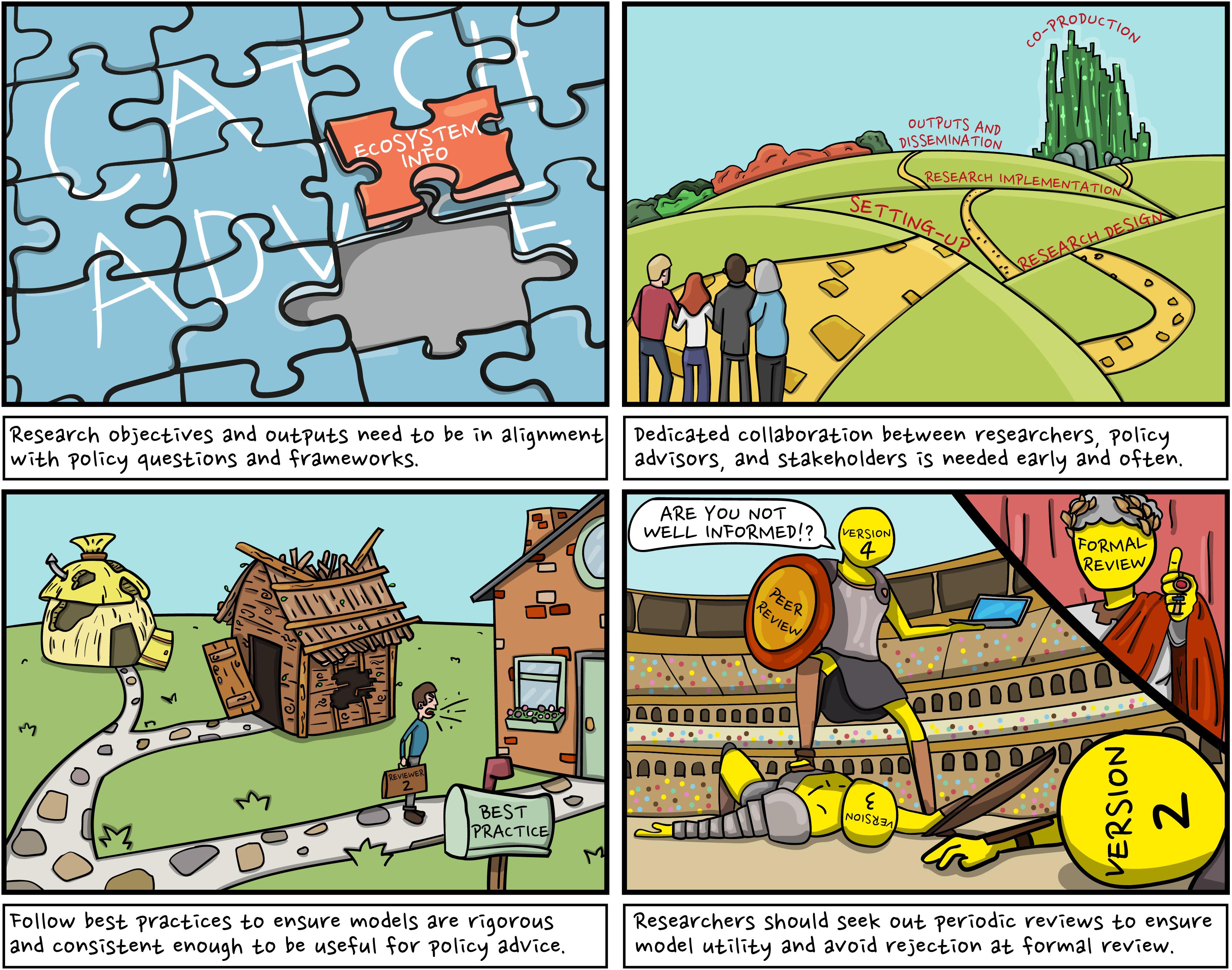
Figure 10. Preparing ecosystem models for policy advice. Drawing on the experience of WKIrish, these illustrations outline the four key lessons which have been fundamental in our pursuit to operationalize the Irish Sea EwE model for policy advice.
Lesson 1: Make Sure Your Objectives Are Aligned With Policy Questions
The Irish Sea EwE model was created with the objective to address a specific problem which managers and stakeholders were already invested in: how can management use ecosystem information to work toward an integrated assessment and advice (ICES, 2015a)? This helped to keep the research aligned with issues relevant to policy advisors (in this case, the stock assessment experts up to and including the ICES Advisory Committee) and stakeholders, increasing its utility as a tool to inform catch advice. Researchers thus need to be aware of the policy making process and the relevant issues their research can address, whilst taking care to manage the expectations of industry stakeholders. Be aware that the ways in which EwE and other multi-species approaches can be applied may be limited; policy processes are frequently tied to legislative concepts (such as MSY) which affects the ability of ecosystem science to influence major policy progress (Townsend et al., 2019). It is important to recognize the constraints of existing policy processes and advice frameworks when attempting to integrate and operationalize ecosystem information.
Lesson 2: Engage Early and Often With Stakeholders and Policy Advisors
Dedicated collaboration between scientists, stakeholders, and policy advisors is needed from early in the project to streamline the potential for integration of science into policy advice. Frequent engagement gets people accustomed to seeing ecosystem models and their analysis, which helps build the credibility of the research. A co-design and co-production approach to knowledge generation offers an inclusive forum to share information and trigger positive social and ecological action (Armitage et al., 2011), whilst moving away from expert-built analytical frameworks that fail to capture local knowledge (Djenontin and Meadow, 2018). It also increases the degree to which researchers and stakeholders interact (Dilling and Lemos, 2011), improving the alignment of research to stakeholder needs (Shirk et al., 2012), stakeholders’ understanding of the ‘scientific’ approach, and relations between stakeholders and scientists. Taken together, the approach increases transparency, improving both trust relations and confidence in the science, and co-ownership of the resulting advice. Co-production methods are called for in European level policy documents such as the European Marine Board’s foresight document: Navigating the Future V (EMB, 2019), which highlights the need for transdisciplinarity, calls for which have been increasing globally.
Lesson 3: Use Best Practices Where Available
It is important to use best practices in both model development and stakeholder engagement processes. For stakeholder engagement, this requires inter alia an open-door inclusion policy, providing both the space and time to develop understanding and allow for (facilitated) stakeholder discussions, managing expectations, maintaining open communication, finding a common language (avoiding overuse of terminology/jargon), and maintaining a culture of mutual respect (e.g., Mackinson et al., 2008).
Best practices need to be followed during model development to ensure ecosystem models are rigorous and consistent enough to be useful for policy advice. Following established best practices improves model credibility within the research community and with advisory bodies and external reviewers who are familiar with the methodology. For EwE, best practices have been outlined by Link J.S. (2010) and Heymans et al. (2016) and center largely on ensuring models comply with ecological and thermodynamic rules (e.g., production rates generally decline with increasing trophic level). Best practice also includes tailoring the structure of models to the questions they intend to answer and acknowledging and visualizing the uncertainty in model inputs and outputs.
Lesson 4: Seek Out Informal and Formal Reviews
Formal reviews by advisory bodies act as quality assurance for models to be used in a management capacity. If possible, researchers should aim for periodic reviews with peers and stakeholders to help guide model development (Townsend et al., 2019). The Irish Sea model was reviewed through the process of scientific publications, informally by researchers and stakeholders at WKIrish workshops (ICES, 2018a, b), and formally by an ICES WGSAM review committee (ICES, 2019c). Early informal periodic reviews by WKIrish helped to identify issues with the model early on in its development, helping to avoid the rejection of the model at the late formal review by WGSAM.
Concept Development
While FECO is not currently designed to move outside of the precautionary bounds of the tactical advice, it could be used to highlight environmentally driven productivity changes and identify whether the conditions under which reference points such as FMSY were set, would no longer hold. Fishing reference points set during a high-productive ecosystem regime run a higher risk of leading to stock overexploitation if the ecosystem were to shift to a low-productivity regime (Vert-pre et al., 2013). A prolonged period of FECO being placed at the extremes of the FMSY range may signify that the FMSY advice no longer holds considering the ecosystem condition and may need to be revisited. The FECO approach could also work in concert with the current mixed-fisheries approach (Ulrich et al., 2017; Garcia et al., 2020) when FECO > FMSY, by using FECO as the upper limit of the FMSY range. The use of the revised range would facilitate the incorporation of ecosystem information into mixed fisheries advice and avoid fishing at FMSYupper when it may be harmful to do so. However, coupling mixed-fisheries models with multi-species models could provide a more long-term approach for the estimation of a safe operating space that meets mixed-fisheries goals while giving good harvest under multi-species considerations (ICES, 2014, 2015b).
Including appropriate ecosystem models alongside single-species models during future ICES benchmark assessments could foster a holistic self-perpetuating development of the work area, as was accomplished during WKIrish (ICES, 2020), encouraging further refinement of the FECO concept and a multidisciplinary approach for single-species assessments. Initiatives such as this promote the training of inter-disciplinary researchers capable of transferring ideas and methodologies across disciplines which is important for the progression of EBM (DePiper et al., 2017; Alexander et al., 2019).
The FECO approach can clearly be developed further, particularly when exploring the relationships between ecosystem indicators and stock production. First order linear relationships were used to identify ecosystem indicators in the example presented in this study, however, we recommend further exploration of more complex relationships which may yield different candidate indicators. Community and biomass-based ecological indicators generally respond to fishing mortality in a negative, linear way (Shin et al., 2010); however, these responses can vary depending on environmental change and the applied fishing strategy (Hunsicker et al., 2016; Fu et al., 2019b). Indicators may respond sharply to fishing pressures past certain thresholds which are often unique to specific ecosystems (Tam et al., 2017; Fu et al., 2019a). Future work should also explore the interaction of pressure indicators with responses to identify non-linear ecosystem responses, detect tipping points, and classify ‘early warning indicators’ to facilitate the development of adaptive management strategies and avoid detrimental ecosystem shifts (Foley et al., 2015).
Ecosystem models represent a multidimensional space. Reducing their complexity to one indicator may run the risk of missing other important drivers that may interact synergistically or antagonistically. Similarly, other impacts could be explored. For example, EwE could be used to explore the impacts of FECO on other consumers within the food web. In reality, the yield of one species depends not only on the applied F they experience, but the F applied to other species and the wider dynamics of the food web (Thorpe and De Oliveira, 2019). Maximizing the yield of each fish stock while accounting for the ecological links between them and the non-targeted components of the food web could lead to long-term ecological sustainability and economic growth (Thorpe, 2019; Spence et al., 2020a).
The usefulness of indicators may also be limited by the availability of data to measure/model indicators or our ability to predict short-term changes in indicators. Many of the trophic indicators derived from the Irish Sea EwE model (i.e., predation pressure, high trophic biomass, zooplankton biomass) were influenced by the inclusion of large-scale climatic drivers in the model. Planque et al. (2003) attempted to use statistical forecasts of sea temperature to improve advice for North Sea cod but concluded that the results were insufficiently accurate to be operationally useful (Planque et al., 2003). Since then, our ability to predict changes in large-scale climatic drivers has matured with the evolution of oceanographic and climate modeling. For example, models of the Atlantic Multidecadal Oscillation (AMO; an anomaly of sea-surface temperature) have been shown to have predictive skill in the range of 2–9 years depending on the model (Trenary and DelSole, 2016). Models that robustly predict the North Atlantic Oscillation (NAO; index based on surface sea-level pressure) tend to be restricted to intra-annual seasonal forecasts initialized a month before the onset of winter (Parker et al., 2019).
Beyond the current FECO proposal, there are other ways in which ecosystem models could both indirectly and quantitatively inform decision making within stock assessment benchmarking. These links may include exploration into the use of natural mortality time series in the same way that multi-species models (SMS) are used to estimate predation influenced natural mortalities for statistical catch at age models (SAM) for single-species assessments (ICES, 2019a). The outputs from ecosystem models could also inform the time series of stock dynamics that should be used when estimating reference points and their ranges. Beyond catch advice, ecosystem models could be operationally used within ICES fisheries and/or ecosystem overviews to provide qualitative or quantitative indicators of ecosystem state and the wider impact on non-target species either directly or indirectly through the food web, as is done by the National Oceanic and Atmospheric Administration (NOAA) Fisheries in their Ecosystem Assessments and Report Cards (Slater et al., 2017). Furthermore, ecosystem models can be used to evaluate the likely performance of a range of management measures through Management Strategy Evaluation (Mackinson et al., 2018; Thorpe and De Oliveira, 2019) and through the assessment cycle for Good Environmental Status (Lynam et al., 2016), as well as to anticipate the effects of climate change on fish stocks (Guenette et al., 2014; Bentley et al., 2017; Pennino et al., 2019).
Conclusion
Ecosystem modeling as a scientific discipline has matured considerably over the past decade, particularly in its capacity to address data uncertainty. Yet the parameter and structural uncertainty of ecosystem models means they may not yet be sufficiently mature to meet the standards required for providing useful tactical catch advice for fisheries management. However, we argue that this does not render ecosystem models useless in the realm of stock assessments. The FECO concept offers an approach to refine recommended fishing mortality by incorporating ecosystem information whilst ensuring that the methods for assessing stocks, setting reference points, and evaluating stock status do not need to be changed. We hope that interventions such as FECO, with its capacity to bridge disciplines, can contribute to continued progress toward EBFM.
Data Availability Statement
The raw data supporting the conclusions of this article will be made available by the authors, without undue reservation.
Author Contributions
JB, ML, DH, SB, AB, FC, JH, CL, DP, PS, JW, and DR attended the WKIrish workshops contributing to the development of concept presented in this work. JB led the analyses and wrote the manuscript with contributions and discussion from all authors.
Funding
This project (Grant-Aid Agreement No. CF/16/08) was carried out with the support of the Marine Institute and funded under the Marine Research Sub-programme by the Irish Government.
Conflict of Interest
The authors declare that the research was conducted in the absence of any commercial or financial relationships that could be construed as a potential conflict of interest.
Acknowledgments
We acknowledge the members of the ICES Benchmark Workshop WKIrish for their collaboration and Dr. Pierre Helaouët at the Marine Biological Association for the provision of continuous plankton recorder data. We would particularly like to thank the stakeholders who participated in and supported WKIrish, and without whom this work would not have happened; John Lynch, Hugo Boyle, Patrick Murphy, Francis O’Donnell, Sean O’Donoghue, Ashley Hayden, John Kirwan, Alan McCulla, Barry Deas, Dale Rodmell, Emiel Brouckaert, Liane Vietch, Jenni Grossmann, Debbie Crockard, and Leanne Llewellyn. We especially thank Hugo Boyle who stayed the pace and contributed wonderfully. We also thank the NWWAC and BIM for supporting and facilitating WKIrish meetings.
Footnotes
References
Alexander, K. A., Hobday, A. J., Cvitanovic, C., Ogier, E., Nash, K. L., Cottrell, R. S., et al. (2019). Progress in integrating natural and social science in marine ecosystem-based management research. Mar. Freshw. Res. 70, 71–83.
Alvarez-Fernandez, S., Licandro, P., Van Damme, C. J. G., and Hufnagl, M. (2015). Effect of zooplankton on fish larval abundance and distribution: a long-term study on North Sea herring (Clupea harengus). ICES J. Mar. Sci. 72, 2569–2577. doi: 10.1093/icesjms/fsv140
A’mar, Z. T., Punt, A. E., and Dorn, M. W. (2010). Incorporating ecosystem forcing through predation into a management strategy evaluation for the Gulf of Alaska walleye pollock (Theragra chalcogramma) fishery. Fisher. Res. 102, 98–114. doi: 10.1016/j.fishres.2009.10.014
Armitage, D., Berkes, F., Dale, A., Kocho-Schellenberg, E., and Patton, E. (2011). Co-management and the co-production of knowledge: learning to adapt in Canada’s Arctic. Glob. Environ. Chang. 21, 995–1004. doi: 10.1016/j.gloenvcha.2011.04.006
Baudron, A. R., Needle, C. L., Rijnsdorp, A. D., and Tara Marshall, C. (2014). Warming temperatures and smaller body sizes: synchronous changes in growth of North Sea fishes. Glob. Chang. Biol. 20, 1023–1031.
Baudron, A. R., Serpetti, N., Fallon, N. G., Heymans, J. J., and Fernandes, P. G. (2019). Can the common fisheries policy achieve good environmental status in exploited ecosystems: the west of Scotland demersal fisheries example. Fisher. Res. 211, 217–230. doi: 10.1016/j.fishres.2018.10.024
Bauer, B., Horbowy, J., Rahikainen, M., Kulatska, N., Müller-Karulis, B., Tomczak, M. T., et al. (2019). Model uncertainty and simulated multispecies fisheries management advice in the Baltic Sea. PLoS One 14:e0211320. doi: 10.1371/journal.pone.0211320
Bauer, P., Thorpe, A., and Brunet, G. (2015). The quiet revolution of numerical weather prediction. Nature 525:47. doi: 10.1038/nature14956
Beggs, S. E., Cardinale, M., Gowen, R. J., and Bartolino, V. (2014). Linking cod (Gadus morhua) and climate: investigating variability in Irish Sea cod recruitment. Fisher. Oceanogr. 23, 54–64. doi: 10.1111/fog.12043
Bentley, J. W., Hines, D. E., Borrett, S. R., Hernández-Milián, G., Serpetti, N., Fox, C. J., et al. (2019a). Combining scientific and fishers’ knowledge to co-create indicators of food web structure and function. ICES J. Mar. Sci. 76, 2218–2234. doi: 10.1093/icesjms/fsz121
Bentley, J. W., Serpetti, N., Fox, C., Heymans, J. J., and Reid, D. G. (2019b). Fishers’ knowledge improves the accuracy of food web model predictions. ICES J. Mar. Sci. 76, 897–912. doi: 10.1093/icesjms/fsz003
Bentley, J. W., Serpetti, N., Fox, C., Heymans, J. J., and Reid, D. G. (2020). Retrospective analysis of the influence of environmental drivers on commercial stocks and fishing opportunities in the Irish Sea. Fisher. Oceanogr. 29, 415–435. doi: 10.1111/fog.12486
Bentley, J. W., Serpetti, N., and Heymans, J. J. (2017). Investigating the potential impacts of ocean warming on the Norwegian and Barents Seas ecosystem using a time-dynamic food-web model. Ecol. Modell. 360, 94–107. doi: 10.1016/j.ecolmodel.2017.07.002
Brander, K. M. (1995). The effect of temperature on growth of Atlantic cod (Gadus morhua). ICES J. Mar. Sci. 52, 1–10. doi: 10.1016/1054-3139(95)80010-7
Brill, R. W., and Lutcavage, M. E. (2001). Understanding environmental influences on movements and depth distributions of tunas and billfishes can significantly improve population assessments. Am. Fisher. Soc. Symp. 25, 179–198.
Britten, G. L., Dowd, M., Kanary, L., and Worm, B. (2017). Extended fisheries recovery timelines in a changing environment. Nat. Commun. 8:15325.
Bryndum-Buchholz, A., Tittensor, D. P., Blanchard, J. L., Cheung, W. W. L., Coll, M., Galbraith, E. D., et al. (2019). Twenty-first-century climate change impacts on marine animal biomass and ecosystem structure across ocean basins. Glob. Chang. Biol. 25, 459–472. doi: 10.1111/gcb.14512
Burrows, M. T., Bates, A. E., Costello, M. J., Edwards, M., Edgar, G. J., Fox, C. J., et al. (2019). Ocean community warming responses explained by thermal affinities and temperature gradients. Nat. Clim. Chang. 9, 959–963.
Capuzzo, E., Lynam, C. P., Barry, J., Stephens, D., Forster, R. M., Greenwood, N., et al. (2018). A decline in primary production in the North Sea over 25 years, associated with reductions in zooplankton abundance and fish stock recruitment. Glob. Chang. Biol. 24, e352–e364. doi: 10.1111/gcb.13916
Chavez, F. P., Ryan, J., Lluch-Cota, S. E., and Ñiquen, M. (2003). From anchovies to sardines and back: multidecadal change in the Pacific Ocean. Science 299, 217–221. doi: 10.1126/science.1075880
Christensen, V., and Walters, C. J. (2004). Ecopath with ecosim: methods, capabilities and limitations. Ecol. Modell. 172, 109–139. doi: 10.1016/j.ecolmodel.2003.09.003
Christensen, V., and Walters, C. J. (2011). Progress in the Use of Ecosystem Models for Fisheries Management. Ecosystem Approaches to Fisheries: A Global Perspective. Cambridge: Cambridge University Press, 189–205.
Coll, M., and Libralato, S. (2012). Contributions of food web modelling to the ecosystem approach to marine resource management in the Mediterranean Sea. Fish Fisher. 13, 60–88. doi: 10.1111/j.1467-2979.2011.00420.x
Collie, J. S., Botsford, L. W., Hastings, A., Kaplan, I. C., Largier, J. L., Livingston, P. A., et al. (2016). Ecosystem models for fisheries management: finding the sweet spot. Fish Fisher. 17, 101–125. doi: 10.1111/faf.12093
Corrales, X., Coll, M., Ofir, E., Piroddi, C., Goren, M., Edelist, D., et al. (2017). Hindcasting the dynamics of an Eastern Mediterranean marine ecosystem under the impacts of multiple stressors. Mar. Ecol. Prog. Ser. 580, 17–36.
Cushing, D. H. (1977). The Atlantic fisheries commissions. Mar. Policy 1, 230–238. doi: 10.1016/0308-597X(77)90029-X
Daw, T., Adger, W. N., Brown, K., and Badjeck, M.-C. (2009). “Climate change and capture fisheries: potential impacts, adaptation and mitigation,” in Climate Change Implications for Fisheries and Aquaculture: Overview of Current Scientific Knowledge, eds K. Cochrane, C. De Young, and T. Bahri (Rome: FAO), 107–150.
DePiper, G. S., Gaichas, S. K., Lucey, S. M., Pinto da Silva, P., Anderson, M. R., Breeze, H., et al. (2017). Operationalizing integrated ecosystem assessments within a multidisciplinary team: lessons learned from a worked example. ICES J. Mar. Sci. 74, 2076–2086.
Dilling, L., and Lemos, M. C. (2011). Creating usable science: opportunities and constraints for climate knowledge use and their implications for science policy. Glob. Environ. Chang. 21, 680–689. doi: 10.1016/j.gloenvcha.2010.11.006
Djenontin, I. N. S., and Meadow, A. M. (2018). The art of co-production of knowledge in environmental sciences and management: lessons from international practice. Environ. Manage. 61, 885–903. doi: 10.1007/s00267-018-1028-3
EC (2008). Directive 2008/56/EC of the European Parliament and of the Council of 17 June 2008 establishing a framework community action in the field of marine environmental policy (Marine Strategy Framework Directive). Off. J. Eur. Union 164, 19–40.
EC (2013). Regulation (EU) No 1380/2013 of the European Parliament and of the Council of 11 December 2013 on the Common Fisheries Policy, amending Council Regulations (EC) No 1954/2003 and (EC) No 1224/2009 and repealing Council Regulations (EC) No 2371/2002 and (EC. Off. J. Eur. Union 354, 22–61.
EMB (2019). Navigating the Future V: Marine Science for a Sustainable Future, Position Paper 24 of the European Marine Board. Ostend: European Marine Board. doi: 10.5281/zenodo.2809392
Finley, C., and Oreskes, N. (2013). Maximum sustained yield: a policy disguised as science. ICES J. Mar. Sci. 70, 245–250.
Foley, M. M., Martone, R. G., Fox, M. D., Kappel, C. V., Mease, L. A., Erickson, A. L., et al. (2015). Using ecological thresholds to inform resource management: current options and future possibilities. Front. Mar. Sci. 2:95. doi: 10.3389/fmars.2015.00095
Fredston-Hermann, A., Selden, R., Pinsky, M., Gaines, S. D., and Halpern, B. S. (2020). Cold range edges of marine fishes track climate change better than warm edges. Glob. Chang. Biol. 26, 2908–2922.
Free, C. M., Thorson, J. T., Pinsky, M. L., Oken, K. L., Wiedenmann, J., and Jensen, O. P. (2019). Impacts of historical warming on marine fisheries production. Science 363, 979–983. doi: 10.1126/science.aau1758
Froese, R., and Quaas, M. (2013). Rio+ 20 and the reform of the common fisheries policy in Europe. Mar. Policy 39, 53–55.
Fu, C., Xu, Y., Bundy, A., Grüss, A., Coll, M., Heymans, J. J., et al. (2019a). Making ecological indicators management ready: assessing the specificity, sensitivity, and threshold response of ecological indicators. Ecol. Indic. 105, 16–28. doi: 10.1016/j.ecolind.2019.05.055
Fu, C., Xu, Y., Grüss, A., Bundy, A., Shannon, L., Heymans, J. J., et al. (2019b). Responses of ecological indicators to fishing pressure under environmental change: exploring non-linearity and thresholds. ICES J. Mar. Sci. 77, 1516–1531. doi: 10.1093/icesjms/fsz182
Garcia, D., Dolder, P. J., Iriondo, A., Moore, C., Prellezo, R., and Urtizberea, A. (2020). A multi-stock harvest control rule based on “pretty good yield” ranges to support mixed-fisheries management. ICES J. Mar. Sci. 77, 119–135.
Garcia, S. M. (2003). The Ecosystem Approach to Fisheries: Issues, Terminology, Principles, Institutional Foundations, Implementation and Outlook. Rome: Food & Agriculture Org.
Giron-Nava, A., James, C. C., Johnson, A. F., Dannecker, D., Kolody, B., Lee, A., et al. (2017). Quantitative argument for long-term ecological monitoring. Mar. Ecol. Prog. Ser. 572, 269–274.
Guenette, S., Araujo, J. N., and Bundy, A. (2014). Exploring the potential effects of climate change on the Western Scotian Shelf ecosystem, Canada. J. Mar. Syst. 134, 89–100.
Hall, S. J., and Mainprize, B. (2004). Towards ecosystem-based fisheries management. Fish Fisher. 5, 1–20. doi: 10.1111/j.1467-2960.2004.00133.x
Harwood, J., and Stokes, K. (2003). Coping with uncertainty in ecological advice: lessons from fisheries. Trends Ecol. Evol. 18, 617–622. doi: 10.1016/j.tree.2003.08.001
Henson, S. A., Beaulieu, C., Ilyina, T., John, J. G., Long, M., Séférian, R., et al. (2017). Rapid emergence of climate change in environmental drivers of marine ecosystems. Nat. Commun. 8:14682.
Heymans, J. J., Coll, M., Link, J. S., Mackinson, S., Steenbeek, J., Walters, C., et al. (2016). Best practice in Ecopath with Ecosim food-web models for ecosystem-based management. Ecol. Modell. 331, 173–184. doi: 10.1016/j.ecolmodel.2015.12.007
Heymans, J. J., Skogen, M., Schrum, C., and Solidoro, C. (2018). Enhancing Europe’s Capability in Marine Ecosystem Modelling for Societal Benefit. Future Science Brief 4 of the European Marine Board. Ostend: European Marine Board.
Hill, S. L., Hinke, J., Bertrand, S., Fritz, L., Furness, R. W., Ianelli, J. N., et al. (2020). Reference points for predators will progress ecosystem-based management of fisheries. Fish Fisher. 21, 368–378.
Hunsicker, M. E., Kappel, C. V., Selkoe, K. A., Halpern, B. S., Scarborough, C., Mease, L., et al. (2016). Characterizing driver–response relationships in marine pelagic ecosystems for improved ocean management. Ecol. Appl. 26, 651–663.
Hyder, K., Rossberg, A. G., Allen, J. I., Austen, M. C., Barciela, R. M., Bannister, H. J., et al. (2015). Making modelling count - increasing the contribution of shelf-seas community and ecosystem models to policy development and management. Mar. Policy 61, 291–302. doi: 10.1016/j.marpol.2015.07.015
ICES (2014). Interim Report of the Working Group on Multispecies Assessment Methods (WGSAM). ICES CM 2014/SSGSUE:11. Burnaby, BC: ICES, 104.
ICES (2015a). Report of the Benchmark Workshop on Sharing Information on the Irish Sea Ecosystem, Stock Assessments and Fisheries Issues, and Scoping Needs for Assessment and Management Advice (WKIrish1). ICES CM 2015/BSG:01. Burnaby, BC: ICES, 37.
ICES (2015b). Report of the Working Group on Mixed Fisheries Advice Methodology (WGMIXFISH-METH). ICES CM 2015/ACOM:22. Burnaby, BC: ICES, 51.
ICES (2015c). Report of the Workshop to Consider FMSY Ranges for Stocks in ICES Categories 1 and 2 in Western Waters (WKMSYREF4). ICES CM 2015/ACOM:58. Burnaby, BC: ICES, 187.
ICES (2016). Report of the Second Workshop on the Impact of Ecosystem and Environmental Drivers on Irish Sea Fisheries Management (WKIrish2). ICES CM 2016/BSG:02. Burnaby, BC: ICES, 191.
ICES (2017). Report of the Benchmark Workshop on the Irish Sea Ecosystem (WKIrish3). ICES CM 2017/BSG:01. Burnaby, BC: ICES, 165.
ICES (2018a). Report of the Workshop on Stakeholder Input to, and Parameterization of, Ecosystem and Foodweb Models in the Irish Sea Aimed at a Holistic Approach to the Management of the Main Fish Stocks (WKIrish4), 23–27 October 2017, Dún Laoghaire, Ireland. ICES CM 2017/ACOM:54. Burnaby, BC: ICES, 35.
ICES (2018b). Report of the Workshop on an Ecosystembased Approach to Fishery Management for the Irish Sea (WKIrish5). 5– November 2018, Dublin, Ireland. ICES CM, 2018/ACOM:66. Burnaby, BC: ICES.
ICES (2019a). Baltic fisheries assessment working group (WGBFAS). ICES Sci. Rep. 1:653. doi: 10.17895/ices.pub.5256
ICES (2019b). Ninth workshop on the development of quantitative assessment methodologies based on LIFE-history traits, exploitation characteristics, and other relevant parameters for data-limited stocks (WKLIFE IX). ICES Sci. Rep. 1:131. doi: 10.17895/ices.pub.5550
ICES (2019c). Working group on multispecies assessment methods (WGSAM). ICES Sci. Rep. 1:320. doi: 10.17895/ices.pub.5758
ICES (2020). Workshop on an ecosystem based approach to fishery management for the Irish Sea (WKIrish6; outputs from 2019 meeting). ICES Sci. Rep. 2:32. doi: 10.17895/ices.pub.5551
IPCC (2019). “Summary for Policymakers,” in IPCC Special Report on the Ocean and Cryosphere in a Changing Climate, eds H.-O. Pörtner, D. C. Roberts, V. Masson-Delmotte, P. Zhai, M. Tignor, E. Poloczanska, et al. (Geneva: IPCC).
Jennings, S., and Rice, J. (2011). Towards an ecosystem approach to fisheries in Europe: a perspective on existing progress and future directions. Fish Fisher. 12, 125–137.
Kennedy, M. C., and O’Hagan, A. (2001). Bayesian calibration of computer models. J. R. Stat. Soc. Ser. B 63, 425–464. doi: 10.1111/1467-9868.00294
Kristiansen, T., Stock, C., Drinkwater, K. F., and Curchitser, E. N. (2014). Mechanistic insights into the effects of climate change on larval cod. Glob. Chang. Biol. 20, 1559–1584.
Larkin, P. A. (1977). An epitaph for the concept of maximum sustained yield. Trans. Am. Fisher. Soc. 106, 1–11.
Leslie, H. M., and McLeod, K. L. (2007). Confronting the challenges of implementing marine ecosystem-based management. Front. Ecol. Environ. 5:540–548. doi: 10.1890/060093
Link, J. (2010). Ecosystem-Based Fisheries Management: Confronting Tradeoffs. Cambridge: Cambridge University Press, doi: 10.1017/CBO9780511667091
Link, J. S. (2010). Adding rigor to ecological network models by evaluating a set of pre-balance diagnostics: a plea for PREBAL. Ecol. Modell. 221, 1580–1591. doi: 10.1016/j.ecolmodel.2010.03.012
Link, J. S., and Browman, H. I. (2014). Integrating what? Levels of marine ecosystem-based assessment and management. ICES J. Mar. Sci. 71, 1170–1173. doi: 10.1093/icesjms/fsu026
Long, R. D., Charles, A., and Stephenson, R. L. (2015). Key principles of marine ecosystem-based management. Mar. Policy 57, 53–60. doi: 10.1016/j.marpol.2015.01.013
Lotze, H. K., Tittensor, D. P., Bryndum-Buchholz, A., Eddy, T. D., Cheung, W. W. L., Galbraith, E. D., et al. (2019). Global ensemble projections reveal trophic amplification of ocean biomass declines with climate change. Proc. Natl. Acad. Sci. 116, 12907–12912. doi: 10.1073/pnas.1900194116
Lynam, C. P., Uusitalo, L., Patrício, J., Piroddi, C., Queirós, A. M., Teixeira, H., et al. (2016). Uses of innovative modeling tools within the implementation of the Marine strategy framework directive. Front. Mar. Sci. 3:182. doi: 10.3389/fmars.2016.00182
Mackinson, S., Neville, S., Raicevich, S., and Worsøe Clausen, L. (eds). (2008). Good Practice Guide to Participatory Research Between Fisheries Stakeholders and Scientists. GAP Project Deliverable, Vol. 1, 23.
Mackinson, S., Platts, M., Garcia, C., and Lynam, C. (2018). Evaluating the fishery and ecological consequences of the proposed North Sea multi-annual plan. PLoS One 13:e0190015. doi: 10.1371/journal.pone
Marshall, K. N., Koehn, L. E., Levin, P. S., Essington, T. E., and Jensen, O. P. (2019). Inclusion of ecosystem information in US fish stock assessments suggests progress toward ecosystem-based fisheries management. ICES J. Mar. Sci. 76, 1–9.
Meehl, G. A., Goddard, L., Boer, G., Burgman, R., Branstator, G., Cassou, C., et al. (2014). Decadal climate prediction: an update from the trenches. Bull. Am. Meteorol. Soc. 95, 243–267. doi: 10.1175/BAMS-D-12-00241.1
Mesnil, B. (2012). The hesitant emergence of maximum sustainable yield (MSY) in fisheries policies in Europe. Mar. Policy 36, 473–480. doi: 10.1016/j.marpol.2011.08.006
Methot, R. D. (2009). “Stock assessment: operational models in support of fisheries management,” in The Future of Fisheries Science in North America, eds R. J. Beamish and B. J. Rothschild (Dordrecht: Springer), 137–165. doi: 10.1007/978-1-4020-9210-7_9
Ottersen, G., Planque, B., Belgrano, A., Post, E., Reid, P. C., and Stenseth, N. C. (2001). Ecological effects of the North Atlantic oscillation. Oecologia 128, 1–14.
Parker, T., Woollings, T., Weisheimer, A., O’Reilly, C., Baker, L., and Shaffrey, L. (2019). Seasonal predictability of the winter North Atlantic Oscillation from a jet stream perspective. Geophys. Res. Lett. 46, 10159–10167. doi: 10.1029/2019GL084402
Patrick, W. S., and Link, J. S. (2015). Myths that continue to impede progress in ecosystem-based fisheries management. Fisheries 40, 155–160. doi: 10.1080/03632415.2015.1024308
Payne, M. R., Hobday, A. J., MacKenzie, B. R., Tommasi, D., Dempsey, D. P., Fässler, S. M. M., et al. (2017). Lessons from the first generation of marine ecological forecast products. Front. Mar. Sci. 4:289. doi: 10.3389/fmars.2017.00289
Pedreschi, D., Bouch, P., Moriarty, M., Nixon, E., Knights, A. M., and Reid, D. G. (2019). Integrated ecosystem analysis in Irish waters; providing the context for ecosystem-based fisheries management. Fisher. Res. 209, 218–229. doi: 10.1016/j.fishres.2018.09.023
Pennino, M. G., Coll, M., Ramirez, J., Albo Puigserver, M., Steenbeek, J., and Bellido, J. M. (2019). “Coupling novel modeling techniques to predict future scenarios and alternative management options for small pelagic species in the Northwestern Mediterranean Sea,” in Proceedings of the Future Oceans2 Integrated Marine Biosphere Research (IMBeR) Open Science Conference, 17–21 June 2019, Brest.
Pepin, P., King, J., Holt, C., Gurney-Smith, H., Shackell, N., Hedges, K., et al. (2018). Incorporating Climate, Oceanographic and Ecological Change Considerations into Population Assessments: A Review of Fisheries and Oceans Canada’s Science Advisory Process. DFO Canadian Science Advisory Secretariat Research Document 1919–5044; 2019/043. Ottaw, ON: Canadian Science Advisory Secretariat (CSAS).
Pikitch, E., Santora, C., Babcock, E. A., Bakun, A., Bonfil, R., Conover, D. O., et al. (2004). Ecosystem-based fishery management. Science 305, 346–347. doi: 10.1126/science.1098222
Pitcher, T. J., Kalikoski, D., Short, K., Varkey, D., and Pramod, G. (2009). An evaluation of progress in implementing ecosystem-based management of fisheries in 33 countries. Mar. Policy 33, 223–232. doi: 10.1016/j.marpol.2008.06.002
Plagányi, ÉE., and Butterworth, D. S. (2004). A critical look at the potential of Ecopath with Ecosim to assist in practical fisheries management. Afr. J. Mar. Sci. 26, 261–287. doi: 10.2989/18142320409504061
Plagányi, ÉE., Punt, A. E., Hillary, R., Morello, E. B., Thébaud, O., Hutton, T., et al. (2014). Multispecies fisheries management and conservation: tactical applications using models of intermediate complexity. Fish Fisher. 15, 1–22.
Planque, B., and Fox, C. J. (1998). Interannual variability in temperature and the recruitment of Irish Sea cod. Mar. Ecol. Prog. Ser. 172, 101–105. doi: 10.3354/meps172101
Planque, B., Fox, C. J., Saunders, M. A., and Rockett, P. (2003). On the prediction of short term changes in the recruitment of North Sea cod (Gadus morhua) using statistical temperature forecasts. Sci. Mar. 67, 211–218. doi: 10.3989/scimar.2003.67s1211
Poloczanska, E. S., Burrows, M. T., Brown, C. J., García Molinos, J., Halpern, B. S., Hoegh-Guldberg, O., et al. (2016). Responses of marine organisms to climate change across oceans. Front. Mar. Sci. 3:62. doi: 10.3389/fmars.2016.00062
Prellezo, R., and Curtin, R. (2015). Confronting the implementation of marine ecosystem-based management within the Common fisheries policy reform. Ocean Coast. Manage. 117, 43–51. doi: 10.1016/j.ocecoaman.2015.03.005
Punt, A. E., and Smith, A. D. M. (2001). The gospel of maximum sustainable yield in fisheries management: birth, crucifixion and. Conserv. Exploit. Species 6:41.
Ramirez-Monsalve, P., Raakjær, J., Nielsen, K. N., Santiago, J. L., Ballesteros, M., Laksá, U., et al. (2016). Ecosystem approach to fisheries management (EAFM) in the EU–current science–policy–society interfaces and emerging requirements. Mar. Policy 66, 83–92.
Régnier, T., Gibb, F. M., and Wright, P. J. (2017). Importance of trophic mismatch in a winter-hatching species: evidence from lesser sandeel. Mar. Ecol. Prog. Ser. 567, 185–197.
Reid, P. C., Colebrook, J. M., Matthews, J. B. L., Aiken, J., and Team, C. P. R. (2003). The continuous Plankton recorder: concepts and history, from plankton indicator to undulating recorders. Prog. Oceanogr. 58, 117–173. doi: 10.1016/j.pocean.2003.08.002
Rice, A. L. (1963). The food of the Irish Sea herring in 1961 and 1962. ICES J. Mar. Sci. 28, 188–200.
Rindorf, A., Dichmont, C. M., Levin, P. S., Mace, P., Pascoe, S., Prellezo, R., et al. (2017). Food for thought: pretty good multispecies yield. ICES J. Mar. Sci. 74, 475–486. doi: 10.1093/icesjms/fsw071
Serpetti, N., Baudron, A. R., Burrows, M. T., Payne, B. L., Helaouët, P., Fernandes, P. G., et al. (2017). Impact of ocean warming on sustainable fisheries management informs the Ecosystem approach to fisheries. Sci. Rep. 7:13438. doi: 10.1038/s41598-017-13220-7
Shin, Y.-J., Shannon, L. J., Bundy, A., Coll, M., Aydin, K., Bez, N., et al. (2010). Using indicators for evaluating, comparing, and communicating the ecological status of exploited marine ecosystems. 2. Setting the scene. ICES J. Mar. Sci. 67, 692–716.
Shirk, J. L., Ballard, H. L., Wilderman, C. C., Phillips, T., Wiggins, A., Jordan, R., et al. (2012). Public participation in scientific research: a framework for deliberate design. Ecol. Soc. 17:29.
Skern-Mauritzen, M., Ottersen, G., Handegard, N. O., Huse, G., Dingsør, G. E., Stenseth, N. C., et al. (2016). Ecosystem processes are rarely included in tactical fisheries management. Fish Fisher. 17, 165–175. doi: 10.1111/faf.12111
Slater, W. L., DePiper, G. S., Gove, J. M., Harvey, C. J., Hazen, E. L., Lucey, S. M., et al. (2017). Challenges, Opportunities and Future Directions to Advance NOAA Fisheries Ecosystem Status Reports (ESRs): Report of the National ESR Workshop. NOAA Technical Memorandum F/SPO-174. Washington, DC: National Oceanic and Atmospheric Administration, 66.
Solomon, S., Plattner, G.-K., Knutti, R., and Friedlingstein, P. (2009). Irreversible climate change due to carbon dioxide emissions. Proc. Natl. Acad. Sci. U.S.A. 106, 1704–1709.
Spence, M. A., Alliji, K., Bannister, H. J., Walker, N. D., and Muench, A. (2020a). Fish should not be in isolation: calculating maximum sustainable yield using an ensemble model. arXiv [Preprint]. arXiv: 2005.02001,Google Scholar
Spence, M. A., Bannister, H. J., Ball, J. E., Dolder, P. J., Griffiths, C. A., and Thorpe, R. B. (2020b). LeMaRns: a length-based Multi-species analysis by numerical simulation in R. PLoS One 15:e0227767. doi: 10.1371/journal.pone.0227767
Spence, M. A., Blanchard, J. L., Rossberg, A. G., Heath, M. R., Heymans, J. J., Mackinson, S., et al. (2018). A general framework for combining ecosystem models. Fish Fisher. 19, 1031–1042. doi: 10.1111/faf.12310
Stäbler, M., Kempf, A., Mackinson, S., Poos, J. J., Garcia, C., and Temming, A. (2016). Combining efforts to make maximum sustainable yields and good environmental status match in a food-web model of the southern North Sea. Ecol. Modell. 331, 17–30. doi: 10.1016/j.ecolmodel.2016.01.020
Stäbler, M., Kempf, A., Smout, S., and Temming, A. (2019). Sensitivity of multispecies maximum sustainable yields to trends in the top (marine mammals) and bottom (primary production) compartments of the southern North Sea food-web. PLoS One 14:e0210882. doi: 10.1371/journal.pone.0210882
Steenbeek, J., Corrales, X., Platts, M., and Coll, M. (2018). Ecosampler: a new approach to assessing parameter uncertainty in Ecopath with Ecosim. SoftwareX 7, 198–204. doi: 10.1016/j.softx.2018.06.004
Tam, J. C., Link, J. S., Large, S. I., Andrews, K., Friedland, K. D., Gove, J., et al. (2017). Comparing apples to oranges: common trends and thresholds in anthropogenic and environmental pressures across multiple marine ecosystems. Front. Mar. Sci. 4:282. doi: 10.3389/fmars.2017.00282
Thorpe, R. B. (2019). What is multispecies MSY? A worked example from the North Sea. J. Fish Biol. 94, 1011–1018. doi: 10.1111/jfb.13967
Thorpe, R. B., and De Oliveira, J. A. A. (2019). Comparing conceptual frameworks for a fish community MSY (FCMSY) using management strategy evaluation—an example from the North Sea. ICES J. Mar. Sci. 76, 813–823.
Thorpe, R. B., Le Quesne, W. J., Luxford, F., Collie, J. S., and Jennings, S. (2015). Evaluation and management implications of uncertainty in a multispecies size-structured model of population and community responses to fishing. Methods Ecol. Evol. 6, 49–58. doi: 10.1111/2041-210X.12292
Townsend, H., Harvey, C. J., DeReynier, Y., Davis, D., Zador, S., Gaichas, S., et al. (2019). Progress on implementing ecosystem-based fisheries management in the US through the use of ecosystem models and analysis. Front. Mar. Sci. 6:641. doi: 10.3389/fmars.2019.00641
Trenary, L., and DelSole, T. (2016). Does the Atlantic Multidecadal Oscillation get its predictability from the Atlantic Meridional Overturning circulation? J. Clim. 29, 5267–5280. doi: 10.1175/JCLI-D-16-0030.1
Ulrich, C., Reeves, S. A., Vermard, Y., Holmes, S. J., and Vanhee, W. (2011). Reconciling single-species TACs in the North Sea demersal fisheries using the Fcube mixed-fisheries advice framework. ICES J. Mar. Sci. 68, 1535–1547. doi: 10.1093/icesjms/fsr060
Ulrich, C., Vermard, Y., Dolder, P. J., Brunel, T., Jardim, E., Holmes, S. J., et al. (2017). Achieving maximum sustainable yield in mixed fisheries: a management approach for the North Sea demersal fisheries. ICES J. Mar. Sci. 74, 566–575. doi: 10.1093/icesjms/fsw126
Vert-pre, K. A., Amoroso, R. O., Jensen, O. P., and Hilborn, R. (2013). Frequency and intensity of productivity regime shifts in marine fish stocks. Proc. Natl. Acad. Sci. U.S.A. 110, 1779–1784. doi: 10.1073/pnas.1214879110
Walters, C., and Christensen, V. (2019). Effect of non-additivity in mortality rates on predictions of potential yield of forage fishes. Ecol. Modell. 410:108776. doi: 10.1016/j.ecolmodel.2019.108776
Ye, Y., and Carocci, F. (2019). Control mechanisms and ecosystem-based management of fishery production. Fish Fisher. 20, 15–24. doi: 10.1111/faf.12321
Keywords: strategic advice, ecosystem approach to fisheries management, FECO, Ecopath with Ecosim, indicators
Citation: Bentley JW, Lundy MG, Howell D, Beggs SE, Bundy A, de Castro F, Fox CJ, Heymans JJ, Lynam CP, Pedreschi D, Schuchert P, Serpetti N, Woodlock J and Reid DG (2021) Refining Fisheries Advice With Stock-Specific Ecosystem Information. Front. Mar. Sci. 8:602072. doi: 10.3389/fmars.2021.602072
Received: 02 September 2020; Accepted: 17 March 2021;
Published: 09 April 2021.
Edited by:
Steven W. Purcell, Southern Cross University, AustraliaReviewed by:
Tommaso Russo, University of Rome Tor Vergata, ItalyNazli Demirel, Istanbul University, Turkey
Copyright © 2021 Bentley, Lundy, Howell, Beggs, Bundy, de Castro, Fox, Heymans, Lynam, Pedreschi, Schuchert, Serpetti, Woodlock and Reid. This is an open-access article distributed under the terms of the Creative Commons Attribution License (CC BY). The use, distribution or reproduction in other forums is permitted, provided the original author(s) and the copyright owner(s) are credited and that the original publication in this journal is cited, in accordance with accepted academic practice. No use, distribution or reproduction is permitted which does not comply with these terms.
*Correspondence: Jacob W. Bentley, ai13LWJlbnRsZXlAaG90bWFpbC5jby51aw==