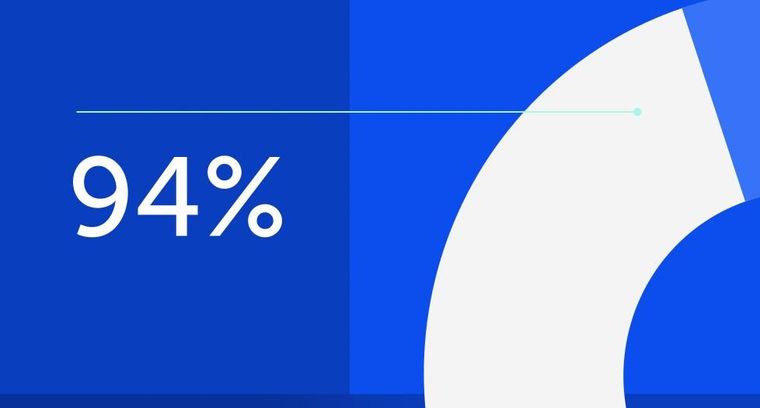
94% of researchers rate our articles as excellent or good
Learn more about the work of our research integrity team to safeguard the quality of each article we publish.
Find out more
REVIEW article
Front. Mar. Sci., 07 July 2021
Sec. Marine Fisheries, Aquaculture and Living Resources
Volume 8 - 2021 | https://doi.org/10.3389/fmars.2021.567266
This article is part of the Research TopicManaging for the Future: Understanding the Relative Roles of Climate and Fishing on Structure and Dynamics of Marine EcosystemsView all 22 articles
Global climate change is a key driver of change in coastal waters with clear effects on biological communities and marine ecosystems. Human activities in combination with climate change exert a tremendous pressure on marine ecosystems and threaten their integrity, structure, and functioning. The protection of these ecosystems is a major target of the 14th United Nations sustainable development goal “Conserve and sustainably use the oceans, seas and marine resources for sustainable development.” However, due to the complexity of processes and interactions of stressors, the status assessment of ecosystems remains a challenge. Holistic food web models, including biological and environmental data, could provide a suitable basis to assess ecosystem health. Here, we review climate change impacts on different trophic levels of coastal ecosystems ranging from plankton to ecologically and economically important fish and shellfish species. Furthermore, we show different food web model approaches, their advantages and limitations. To effectively manage coastal ecosystems, we need both a detailed knowledge base of each trophic level and a holistic modeling approach for assessment and prediction of future scenarios on food web-scales. A new model approach with a seamless coupling of physical ocean models and food web models could provide a future tool for guiding ecosystem-based management.
The complexity of the direct and indirect effects of global climate change on marine ecosystems and the immense diversity of scales call for novel multifaceted views and the combination of different types of analyses via models. Without this we will not be able to make management decisions and protect ecosystem services in a meaningful and sustainable manner.
Global climate change is a key driver of change in coastal marine ecosystems (Gissi et al., 2020). It is caused by increasing anthropogenic greenhouse gas emissions and is responsible for the rapid alteration of abiotic environmental factors in marine systems, such as water temperature, pH, hydrography, and salinity. These changes manifest with clear effects on biological communities and the structure and function of marine ecosystems (IPCC, 2014). Temperature has been suggested to be one of the most important physical drivers of aquatic environments and biological community structure (Richardson, 2008; IPCC, 2014). Rising temperatures are globally significant and result in reduced sea-ice in the Arctic and Antarctic. Glacier runoff and precipitation patterns have changed considerably with resultant redistribution of annual river discharge rates. Such shifts result in more evident changes in pelagic salinity and particulate matter loadings in coastal areas (IPCC, 2014). Climate change also alters ocean evaporation and current flows, which are likely to result in changes in the ocean circulation (Toggweiler and Russell, 2008; Greene et al., 2013; IPCC, 2014) with as yet unquantifiable and largely unknown effects for marine life and biodiversity.
Ocean waters take up CO2 from the atmosphere (Sabine et al., 2004). Since the beginning of the industrial era, ocean waters have absorbed about 30% of the atmospheric CO2 (IPCC, 2014). The resulting acidification alters the physical environment, the carbon cycle and it impacts marine communities, for instance by affecting larval development and calcification processes of ecologically and economically important shellfish species (Waldbusser and Salisbury, 2014; Wallace et al., 2014) or calcifying plankton (Riebesell, 2004). Also, the increasing trend of extreme weather events associated with climate change (IPCC, 2014) affects marine communities. Extreme hot summers, more frequently occurring hypoxia events and harmful algal blooms can lead to a higher mortality rate in fish stocks and shellfish populations (Rijnsdorp et al., 2009; Wendling and Wegner, 2013).
In addition to the effects of global climate change, marine ecosystems are also affected by local anthropogenic pressures, such as eutrophication (e.g., van Beusekom, 2005), fishing (e.g., Lindeboom and De Groot, 1998) or seabed degradation (e.g., Airoldi et al., 2008). The exploitation and the destruction of marine, inshore and catchment habitats strongly impact coastal ecosystems, in their complex buffer-like position between land and ocean (He and Silliman, 2019). The scales of impact are highly variable, ranging from the impact of stressors on a global to a local geographic scale. For instance, ocean warming and acidification are global phenomena with large-scale impacts on the entire marine environment, but these can also induce further local effects, such as hypoxia events or melting sea-ice (IPCC, 2014). In addition, stressors interact with each other resulting in potentially unpredictable changes and immensely complex impacts on the marine environment (He and Silliman, 2019; Gissi et al., 2020).
In short, direct human activities in combination with climate change exert a tremendous pressure on marine ecosystems and threaten their integrity, structure and functioning. The human population heavily relies on healthy coastal ecosystems, and the negative feedback on ecosystem services (ES) from anthropogenic pressures reduces the chance for sustainable resource use. Coasts provide a wide range of ES from which humans benefit directly or indirectly (Barbier et al., 2011; Liquete et al., 2013). ES are classified as supporting, provisioning, regulating or cultural services (Millennium Assessment Board, 2005) which include, for instance, the increase of water quality by filtration (regulating ES) or the provision of food (provisioning ES). Global and local stressors impact different trophic levels of coastal food webs, such as plankton, fish and shellfish, and thus, jeopardize the provision of ES (Poloczanska et al., 2013; He and Silliman, 2019).
Given the magnitude of the pressures exerted on coastal environments, their protection is a major target of the 14th United Nations sustainable development goal “Conserve and sustainably use the oceans, seas and marine resources for sustainable development”. Several guidelines, such as the European Marine Strategy Framework Directive (MSFD) (European Commission, 2008), have been established to achieve a “good environmental status” in coastal waters (Pogoda et al., 2020). However, due to the complexity of processes and interactions of stressors, the status assessment of ecosystems remains a challenge. Holistic food web models, including biological and environmental data, could provide a suitable basis to assess ecosystem health (de la Vega et al., 2018; Fath et al., 2019; Safi et al., 2019). These model approaches have significantly improved over the last decade (Coll et al., 2015; Geary et al., 2020), but their capacities to evaluate multiple-stressor impacts on different trophic levels are still limited (Coll et al., 2015).
Here, we focus on the following aims: (1) summarize current knowledge on climate change impact on different trophic levels of coastal food webs and their ES, (2) review current food web model approaches and their limitations, and (3) discuss a novel suggestion for a future high-resolution food web model approach, to assess climate change impacts in a holistic approach.
Climate change alters organisms’ abundance, distribution, physiology and phenology (IPCC, 2014). These changes are not restricted to one trophic level or one local region, but affect the entire ecosystem on a global scale. This highlights the challenge of assessing ecosystem status and climate change impacts to guide conservation and management.
Coastal waters are highly productive areas and supply approximately 80% of the global wild-captured seafood (Capuzzo et al., 2018). This production relies on planktonic organisms at the basis of coastal food webs. Plankton supports ecologically and economically important species, which structure and stabilize pelagic and benthic communities (e.g., in their role as key predators) and provide habitats (e.g., as ecosystem engineers). Based on their importance for food web functioning and the provision of ES (Atkins et al., 2015; Turner et al., 2015), we focus on climate induced changes of plankton communities, and of ecologically and economically important fish and shellfish species and their trophic interactions.
Phytoplankton provide half of the global net primary production despite their comparably low biomass (Field et al., 1998). Biogeochemical cycles, such as the carbon or nitrogen cycles (Falkowski, 1994), and energy transfer to higher trophic levels depend on phytoplankton diversity and community composition. Phytoplankton affect fluxes of elements in the ecosystem by the uptake and storage of nutrients (Falkowski et al., 1998). Phytoplankton can also serve as a major source of trace gases, such as dimethyl sulfide, which can in turn also affect atmospheric chemistry and thus influence climate (Charlson et al., 1987; Andreae and Crutzen, 1997). Phytoplankton can photosynthetically fix atmospheric CO2 into organic matter (Sunda, 2012), which is sequestered in the deep ocean through the biological pump (Tedesco and Thunell, 2003; Muller-Karger et al., 2005). Climate change induced alterations of the phytoplankton community are therefore likely to affect the regulating ES of marine phytoplankton.
Trends in phytoplankton responses to changing environmental conditions strongly vary across regional and temporal scales. Thus, very long and well understood database are required to link climate-related shifts to phytoplankton productivity. In addition, the fundamental controlling factors of plankton growth should be determined based on multi-decadal data (Wiltshire et al., 2015). For example, the continuous plankton recorder (CPR) operating in the North Atlantic and the North Sea provides one of the longest time series of plankton species composition and density in the world (Reid, 1975; Richardson et al., 2006). The CPR methodology was expanded to the North Pacific in 2000 with sister surveys in Narragansett, United States and Tasmania, Australia (Reid et al., 2003). Furthermore, several local but long-term plankton monitoring stations were established, for instance the German Helgoland Roads Time Series (HRTS) (Wiltshire et al., 2010), Hawaii Ocean Time series (HOT) (Bingham and Lukas, 1996), and Bermuda Atlantic Time Series (BATS) (Steinberg et al., 2001). The long-term data sets enable us to study multi-decadal patterns, and provide a unique basis to evaluate the influence of climate change on plankton.
Planktonic communities are affected at different scales by climate change (Figure 1). Analyses of CPR and HRTS data revealed warming induced changes in phytoplankton abundance (Richardson and Schoeman, 2004; Wiltshire et al., 2010). Richardson and Schoeman (2004) also showed a phytoplankton increase in cooler regions and a decrease in warmer regions. Declines in phytoplankton abundance and biomass are often linked to a nutrient depletion in surface waters since higher water temperatures generally lead to more stable and stratified water conditions resulting in less nutrient supply from deeper waters (Richardson, 2008; Gittings et al., 2018). For instance, this was shown for phytoplankton communities in the northern Red Sea (Gittings et al., 2018). Moreover, warming also alters the phenology of phytoplankton, such as growth and blooming behavior (Winder and Sommer, 2012; Wiltshire et al., 2015; Gittings et al., 2018; Scharfe and Wiltshire, 2019). Long-term studies of the phytoplankton community in the North Sea revealed that increasing temperatures change the timing of growth in more than half of the studied phytoplankton species (Scharfe and Wiltshire, 2019). Such phenological shifts can cause severe alterations in the food web if phytoplankton blooms are decoupled from zooplankton growth periods (Wiltshire et al., 2010; Winder and Sommer, 2012). A mismatch in times of peaking phytoplankton biomass and growth periods of their consumers can decrease the survival of those herbivores due to reduced food supply (Wiltshire and Manly, 2004; Richardson, 2008; Wiltshire et al., 2008). Because phytoplankton biomass appears to be one of the key drivers of zooplankton communities (Wiltshire et al., 2008; Capuzzo et al., 2018), changes at phytoplankton level are likely to propagate through the food web to higher trophic levels.
Figure 1. Graphical summary of climate change impacts on planktonic communities. Positive signs indicate an increase and negative signs indicate a decrease. Information are based on literature cited in the text.
Under climate change, numerical models predict a severe decline of phytoplankton production due to rising CO2 (Bopp et al., 2004, 2005). Furthermore, numerical simulations and observations suggested that increasing temperatures reduce plankton biomass, change the turnover time and break the stable coexistence of phytoplankton and zooplankton (Sarker et al., 2018, 2020). Sekerci and Petrovskii (2015) found that warming-induced oxygen depletion could cause plankton species extinction. Their model outputs also suggested that warming-induced stratification in tropical marine systems will reduce surface nutrient concentrations, and favor smaller phytoplankton cells at the expense of larger diatoms. An additional effect of warming is the melting of ice, which could impact planktonic communities. Reduced sea-ice cover results in an earlier attenuation of light limitation and induces a stabilization of stratification causing an earlier spring bloom in the Arctic and high-latitude Southern Ocean (Henson et al., 2018). While these models already predict climate change impacts on the phytoplankton level, they do not consider any propagations to higher trophic levels or feedback loops.
Zooplankton are a key component of coastal food webs, which link the energy generated by primary production to higher trophic levels. Zooplankton are poikilothermic organisms and may express changes in physiological responses to higher temperatures, including higher ingestion and respiration rates (Dam and Peterson, 1988; Vaquer-Sunyer et al., 2010; Chen et al., 2012) as well as faster reproductive development (Heinle, 1969; Weydmann et al., 2018). This affects primary production via increased grazing pressure and can lead to shifts in phytoplankton size and species structure (Bergquist et al., 1985; Granéli et al., 1993). Furthermore, climate change impacts the community structure of zooplankton when environmental conditions exceed the tolerance limits of certain species. Richardson and Schoeman (2004) found a shift of various herbivorous and carnivorous zooplankton species of the North Sea and Atlantic toward cooler regions based on CPR data. Changes in the environment can also allow the invasion of new organisms. For instance, species such as Penilia spp. and the introduced species Mnemiopsis leidyi have become more abundant in the southern North Sea due to warmer water temperatures (Johns et al., 2005; Boersma et al., 2007; Jaspers et al., 2018). Similarly, investigations of zooplankton in the Bering Sea revealed that warmer temperatures lead to changes in community structure with negative impacts on large, lipid-rich zooplankton and higher abundances of small zooplankton species in warm years (Eisner et al., 2014). In coral reefs, acidification results in changes in the zooplankton community structure and leads to an overall decrease of zooplankton abundance (Smith et al., 2016). Absorbed carbon from the atmosphere might also decrease the food quality for zooplankton by changing the carbon to nutrient ratio of the water (Van de Waal et al., 2010; Meunier et al., 2016) and thus the nutrient composition of planktonic primary producers (Sterner and Elser, 2002; Meunier et al., 2014). Consumers need to find a way to get rid of the surplus carbon, for instance by excreting carbon as dissolved organic carbon (DOC), which is potentially available for microbes and bacteria and can therefore influence the entire food web (Schoo et al., 2013).
To understand the impact of climate change on zooplankton communities, a significant number of model-based studies have been conducted. These studies include statistical models, small scale numerical simulations and large-scale numerical simulations. For example, Carter et al. (2017) used a statistical multivariate autoregressive model to reveal that warming was the dominant driver of change in the zooplankton community in an Alaskan lake from 1963 to 2009. Warming-induced oxygen depletion also caused a zooplankton biomass decrease in non-linear mathematical models (Sekerci and Ozarslan, 2020). Coupled population and hydrodynamic models were used to understand the impact of climate change on critical copepod life-history processes (Speirs et al., 2005). Wakelin et al. (2015) investigated the impact of climate change along with anthropogenic drivers on the zooplankton communities using a coupled hydrodynamic-ecosystem model. Their findings suggested that climate change has a negative impact on the zooplankton community. Model simulations in higher latitudes revealed that melting sea ice decreases the salinity of the surface layer causing an alteration in the timing of spring increase in copepod abundance (Ding et al., 2019). These models already successfully predict changes in zooplankton community structure and biomass related to climate change, but they do not provide effects on higher trophic levels or feedback loops to phytoplankton.
During their early life stages, larvae of fish and shellfish are part of the zooplankton community (meroplankton). Elevated temperatures influence the timing of gametogenesis, gonad and embryonic development, spawning and hence, the abundance of these larvae (Birchenough et al., 2015; Bayne, 2017). Shifts in spawning time in relation to climate change have already been described by Cushing (1969) who suggested the risk of a potential de-coupling between fish larvae and the production cycle of their prey. As pelagic fish larvae mainly feed on zooplankton (Daan et al., 1990), the climate change associated shifts in phytoplankton and zooplankton can result in a decrease of food quality and quantity for these higher trophic levels. Beaugrand et al. (2003) found a decline in cod recruitment from the mid-1980s onward due to a mismatch between the prey size and appearance of cod larvae. Zooplankton of the genus Calanus belong to the most important prey of larvae and juveniles of cod in the North Sea. The replacement of C. finmarchicus by C. helgolandicus due to higher water temperatures had a direct effect on cod recruitment survival because C. finmarchicus peaks in spring while C. helgolandicus peaks in late summer/autumn resulting in insufficient prey supply for cod recruitment (Beaugrand et al., 2003). Similarly, there has been an entire regime shift in the Central Baltic Sea in the late 1980s presumably due to a change in atmospheric forcing causing a sudden increase in temperature (Möllmann et al., 2009). However, effects of increased temperature are not negative per se. In Lake Aleknagkik, Alaska, increasing temperatures led to an earlier ice breakup in spring with positive effects on sockeye salmon (Oncorhynchus nerka) larvae due to increased zooplankton growth (Schindler et al., 2005).
Shellfish larvae pass through critical life-stages while depending on phytoplankton as an exclusive food source. Specific phytoplankton compositions within narrow time windows induce successful development of long-adapted populations and affect total recruitment by allowing or by impeding metamorphosis and settlement (Birchenough et al., 2015; Bayne, 2017). Furthermore, increased predation pressures, caused by reduced winter migration of predators in warming waters, raise mortality rates of early larval populations in spring (Beukema and Dekker, 2005). Kirby et al. (2008) showed a climate-induced decrease of bivalve larvae, but it is still unknown how reduced abundances of larvae affect adult populations (Birchenough et al., 2015). On community level, mismatch effects of primary consumers are suggested to induce trophic cascades and major changes in coastal ecosystems, confirming the role of benthic shellfish as central components regulating functioning of both pelagic and benthic systems (Jochum et al., 2012; Lindegren et al., 2012).
Shellfish are well known for their provisional ES. Global annual capture production of mollusk groups and crustaceans, as well as aquaculture production, sum up to over 45 million tons. Negative trends in bivalve fisheries are related to an increasing aquaculture production, but in some areas also to pollution and the degradation of marine environments (FAO, 2018; Wijsman et al., 2019). In addition, bivalves regulate the water quality of coastal waters by their filtration capacity (e.g., Cranford, 2019). Most shellfish species are less mobile or even sessile and are thus limited in their ability to actively move to other areas if habitat conditions decrease due to climate change.
Among crustaceans, pandalid shrimps occupy a central position in the food web because they prey upon zooplankton and are the main food of different fish species. In the Gulf of Alaska, different species of pandalid shrimps declined sharply in relation to increasing water temperatures with critical impacts on the fishing sector (Anderson, 2000). Pandalids also have been shown to react with phenological changes to temperature alterations. In the Gulf of Maine, the northern shrimp Pandalus borealis exhibited an earlier hatch initiation with a later hatching completion in relation to increasing temperature (Richards, 2012). Furthermore, temperature affects larval dispersal (Le Corre et al., 2020) and recruitment survival (Ouellet et al., 2017) of P. borealis.
Other shellfish taxa, such as bivalves, are commonly classified as ecosystem engineers, as their numerical abundance creates significant effects in benthic communities and they often provide a relevant structuring component for their habitat by creating biogenic reefs (Birchenough et al., 2015; Bayne, 2017). Bivalves are filter-feeding primary consumers and depend on phytoplankton availability in quantity, quality and time. Climate change induced shifts in phytoplankton availability affects growth and reproductive output of bivalves (Birchenough et al., 2015). Declines in bivalve abundance result in reduced filtration capacity, which allows for increased phytoplankton blooms, hypoxia events at the benthic-pelagic interface, sediment instability and reduced marine vegetation (Schulte et al., 2009; Cranford, 2019). The ecosystem is forced from benthic to pelagic production and recruitment and reproductive output of the benthic community will decrease (Birchenough et al., 2015; Bayne, 2017). Furthermore, shellfish are often exposed to climate related range and virulence extension of parasites and pathogens. Rowley et al. (2014) reviewed the influence of climate change on shellfish diseases in the Irish Sea and related an increase of the range and prevalence of different parasite and pathogen groups (e.g., Vibrio populations) to elevated summer and winter temperatures. However, due to a lack of knowledge on pathogen dispersal conclusions remain fragmentary. Also, ocean acidification affects species depending on calcification, such as bivalves (Gazeau et al., 2007) with unknown long-term effects for species survival and benthic communities.
A recent integrated model approach addressed the evaluation of climate change impact on fish and shellfish in Alaska, primarily considering the potential consequences for socio-ecological interactions under different fishing scenarios (Hollowed et al., 2020). Guyondet et al. (2015) used a coupled hydrodynamic-biogeochemical model to study the effects of warming and nutrient loads on mussel aquaculture. They found a production increase in mussels as long as the mussels can sustain summer temperatures. Nevertheless, model predictions for shellfish distribution, fitness and respective ecosystem service provision under climate change are scarce.
Fish play an important role in global nutrition (FAO, 2018) and sustaining healthy fish populations in a changing world is one of the key roles of global and local management strategies (ICSU, 2017). Fish are highly mobile and rising water temperatures cause a general poleward shift in distribution of tropical, temperate and subpolar fish species (Schindler et al., 2005). On a local scale, fishes also move to cooler water depths to avoid high water temperatures (Dulvy et al., 2008). The distribution of each species is constrained by its thermal tolerance (Rijnsdorp et al., 2009; Pörtner and Peck, 2010) and the shifting trend could affect especially those species which currently have a more poleward distribution since the suitable habitat decreases (Kleisner et al., 2017). Preferences for and dependencies on a specific habitat can complicate range adaptation to thermal changes for some fish species. In the North Sea, sandeel populations (e.g., Ammodytes marinus) are identified as highly threatened by climate change. Sandeels are ecological key species as they link primary and secondary (zooplankton) production to top predators (seabirds, fish, marine mammals) (Heath et al., 2012). But they are also of significant economic importance, supporting a large fishery in the North Sea (ICES, 2014). Sandeels are exclusively associated to coarse sediments and therefore indicate only limited potential for extending their distribution to the north (Heath et al., 2012). Similarly, the cape anchovy (Engraulis capensis) is unable to shift poleward lacking a refugium (Grant and Bowen, 2006). Most anchovy stocks are coastal and the cape anchovy habitat in the Benguela Current System is bounded by two warm currents: the Angola Current to the north and the Agulhas Current to the east. The cape anchovy off South Africa is unusually vulnerable to ocean warming (Checkley et al., 2017) and at the same time highly important for local fisheries. In addition to geographical range shifts, changing abiotic conditions can alter the annual migration of fishes to their feeding and spawning grounds (Rijnsdorp et al., 2009). A relationship between water temperatures and migration patterns have already been shown for fish species, such as flounders Platichthys flesus (Sims et al., 2004). However, alterations in fish migration with respect to climate change still require further investigations (Lennox et al., 2019).
Several model studies focus on the impact of climate change on fish stocks, especially due to their importance for fisheries and human nutrition. The model approach of Kleisner et al. (2017) supported the observed poleward shift of thermal fish habitats. This was also shown in the ensemble model study of Bryndum-Buchholz et al. (2020) with a fish biomass increase in Arctic regions and a decrease in tropical regions. The fish collapse in the Northwest Atlantic was explored with statistical models, which revealed that the reason was a mixture of fishing and environmental impacts (Dempsey et al., 2018). However, while these model approaches successfully represent potential responses to climate change, interactions with prey organisms are often not included.
The complex interactions within coastal food webs and the interactive effects of anthropogenic stressors such as fisheries and climate change make it difficult to assess changes on ecosystem scale (Gissi et al., 2020). However, this assessment and the projection of future changes are essential to provide the relevant knowledge to guide sustainable management of coastal ecosystems supporting the robust provision of ES, such as marine food supplies. Without such assessments, the regulation of ES becomes almost impossible, with particular regard to optimal spawning and recruitment conditions for stable populations of relevant key species. To effectively manage coastal ecosystems, both a detailed knowledge base of each trophic level and a holistic modeling approach for assessment and prediction of future scenarios on food web-scales are needed (Howell et al., 2021).
In order to achieve a realistic future simulation, such models should be able to simulate the different effects and drivers of climate change across trophic levels and on different scales. On the abiotic scale, models need to be able to predict future conditions under different climate change scenarios with a high temporal and spatial resolution. The predicted changes in the abiotic environment will then drive the identified biological changes in distribution, physiology and phenology of the different trophic levels and changes in food web interactions.
A plethora of different models are available to test different scenarios and hypotheses on different trophic levels and food web-scales (Plagányi, 2007; Jørgensen, 2008; Fulton, 2010; Tittensor et al., 2018). A detailed comparison of all available model approaches is beyond the scope of this manuscript. In contrast, we focus on those models representing trophic interactions from low trophic levels to high trophic levels in relation to climate change. These models are potential tools to guide sustainable actions because they provide a holistic representation of the ecosystem.
Many trophic models use the dispersion of nutrients as a basis for food web interactions (Fulton, 2010). On a basic scale, this includes the interaction between nutrients (N), phytoplankton (P), zooplankton (Z) and detritus (D), represented in NPZ(D) models (Wroblewski et al., 1988; Dadou et al., 1996). These models can be three-dimensional and have the ability to describe ecosystem structure and functions in detail. Thus, they are useful tools to address questions focusing on plankton dynamics and physical oceanography (Franks and Chen, 2001; Aumont et al., 2015; Leles et al., 2016). NPZD models allow the combination of species into functional groups (Fasham, 1993), and therefore, these models can be used to investigate the impact of climate change on these functional groups. Through advanced modeling approaches complexity of ecosystems can be added in NPZD models by splitting nutrients, phytoplankton and zooplankton into subgroups (Allen et al., 2001).
In the context of climate change, Aumont et al. (2003) considered four plankton functional groups under nutrient co-limitation of phytoplankton growth as a function of N, P, Si and Fe in NPZD models. Werner et al. (2007) and Aita et al. (2007) performed simulations using NPZD models to understand food web dynamics. Furthermore, there are approaches toward ecosystem models by coupling NPZD models with hydrodynamic modules, in order to assess the impact of environmental changes (Chen et al., 2006; Schrum et al., 2006a,b; Aumont et al., 2015). In proceeding attempts, components representing fish and macrofauna have been added to these NPZD-hydrodynamic models providing a model solution from nutrients to higher trophic levels, such as NEMURO.FISH (Megrey et al., 2007; Rose et al., 2008) or ECOSMO E2E (Daewel et al., 2008, 2019).
NPZD-based models provide a detailed representation of plankton dynamics in relation to changes in the nutrient supply. However, even advanced approaches focus on specific system components and do not integrate the entire food web (Table 1).
One of the most advanced marine trophic models with a biogeochemical fundament is the hybrid model Atlantis, which includes all ecosystem trophic levels and their interactions (Fulton et al., 2004). In Atlantis, the nutrient flow through the different biological groups is modeled, including non-living detritus components. In addition to the biogeochemical module, Atlantis includes a hydrographic submodel representing physical processes, a fisheries submodel and a management submodel, allowing for the test of different future scenarios (Fulton et al., 2004).
Atlantis has been applied to different coastal ecosystems worldwide (Fulton, 2010; Hansen et al., 2019), including studies on climate change effects. Ortega-Cisneros et al. (2016) used Atlantis in the Benguela upwelling system to assess impacts of climate change and fishing. They found that warming had the largest effects on species’ biomass, with mainly negative impacts. Furthermore, Atlantis was used to predict future changes in coral reef ecosystems (Weijerman et al., 2015). The study showed that climate change will have severe impacts on coral reefs in the near future, especially by interactions and cumulative effects with other stressors, such as fishing and pollution. Thus, first studies using Atlantis related to climate change appear promising.
The Atlantis framework includes all system components, changes in the abiotic environment and anthropogenic impacts, such as fisheries. These models could even provide suitable indicators for assessing the ecosystem health (Fulton et al., 2005). The management module allows for simulations of different strategies. Thus, the Atlantis framework provides advanced tools for exploring, e.g., climate change impacts on food web scales. However, there are also some limitations. Atlantis does not use the same grid coordinates as hydrodynamic models, but rather irregular polygons (Fulton et al., 2005, 2007) with relatively coarse resolution, which seems to represent a compromise between the complexity and computational costs. Physical parameters are coupled offline as time series data. They are taken from observations or from ocean model simulations. This means that important processes like vertical mixing or intertidal processes are largely parametrized rather than resolved. Parametrization leads to an averaging in space and time of the impact of different dynamic processes, which sometimes cannot be done in a consistent manner especially for relatively large areas. For example, vertical mixing depends on buoyancy and vertical shear stress, these terms are highly dynamic in coastal areas in time and space due to the presence of regions of the freshwater influence, shallowness of the area and the large role of tides in coastal dynamics. Therefore, biogeochemical and ecosystem processes become largely de-coupled to the physical processes. Additionally, an exchange between physical and biological modules assumes interpolation due to different physical and ecosystem grid geometries (ocean model grid/observation tracks versus polygons). Accordingly, the resolution of early life history stages including pelagic dispersal phases of organisms linked to coastal areas with complex dynamics is rather coarse in Atlantis-based models (Itoh et al., 2018).
In contrast to Atlantis and similar nutrient-based model approaches, trophodynamic models can also be based on species biomass data. Common biomass-based food web methodologies are network models analyzed with Ecological Network Analysis (ENA) (Kay et al., 1989; Ulanowicz, 2004) or the widely used framework Ecopath with Ecosim and Ecospace (EwE) (Christensen and Walters, 2004). Both approaches are based on a similarly structured snap-shot model, representing the studied trophodynamic system. ENA and Ecopath are based on the biomass of system components, their energetic requirements and the diet spectra. However, while ENA is a static model, representing an averaged ecosystem during a specific time, Ecopath also includes a temporal-dynamic component (Ecosim) and a spatial-temporal-dynamic component (Ecospace).
Both approaches, ENA and EwE, have been used in a variety of studies to describe the structure and functioning of coastal food webs (e.g., Baird et al., 2004; Scharler and Baird, 2005; Coll et al., 2011; Horn et al., 2019; Vilas et al., 2020), but few studies focus on climate change. ENA has been applied in shallow coastal areas of the south-eastern North Sea, the Wadden Sea, to investigate the impact of increasing temperatures on the food web (Baird et al., 2019). In this system, an increase in ambient water temperature resulted in a higher detritivory and recycling in the food web whereas the degree of organization, diversity of flows and specialization of the food web structure decreased. Furthermore, climate change caused alterations in the Wadden Sea food web due to long-term shifts in the species composition according to ENA results (Schückel et al., 2015). Range shifts of species are supposed to be a major driver in future food web changes. A dynamic EwE modeling approach of the food web in the Barents Sea focused on the impact of poleward migrating fish species due to warmer water temperatures (Kortsch et al., 2015). The study suggests a shift from the more modular, specialized Arctic food web toward a system with increased connectivity driven by boreal fish generalists. Further changes of the Barents Sea food web due to warming were studied in Bentley et al. (2017) who suggested a gradual shift toward a system dominated by pelagic species and a reduction in boreal species. On a multi-stressor level, the combined effects of eutrophication and overfishing (Vasas et al., 2007) as well as eutrophication and increased temperature (Binzer et al., 2016) have been studied using EwE implying unexpected interactions between the pressures. For the Baltic Sea, Niiranen et al. (2013) tested the influence of management strategies for fisheries and nutrient input under different IPCC climate change scenarios until the end of this century with EwE. Results ranged from a cod dominated food web with reduced nutrient levels and phytoplankton biomass close to today in the best case, to a sprat dominated, severely eutrophicated system in the worst case highlighting the importance of management strategies with regard to climate change.
Field data are included as model input (e.g., biomass data) in ENA and EwE models. Thus, trends of the system components are directly incorporated, if the models are based on regular monitoring data. In terms of EwE, additional long-term data can be used to parametrize the model over time. ENA and EwE are particularly interesting for management purposes because both methodologies provide a set of global system indices following the theory of Ulanowicz (1986). Some of these indices are potential candidate indicators for the MSFD to assess the health status of coastal food webs (Fath et al., 2019; Safi et al., 2019). However, changes of the candidate indicators provided by ENA and EwE models in relation to climate change impacts are largely unknown. The complexity of processes and a lack of data on trophodynamic relationships and feedback are reflected in model limitations. However, this fundamental gap can be attributed to all ecosystem models with advanced representation of the food web. Furthermore, dynamic shifts in the abiotic environment are difficult to represent in EwE and ENA. In ENA, the static model behavior permits a dynamic response over space and time. In EwE, abiotic changes are usually used to alter specific model parameters, such as primary production, consumption rates or fish egg production (Niiranen et al., 2013; de Mutsert et al., 2016; Serpetti et al., 2017), but not the entire food web (Table 1).
The evaluation of climate change impacts on coastal ecosystems requires a deep understanding of the abiotic parameters based on precise data (Wiltshire and Manly, 2004; Wiltshire et al., 2010). Shelf zones are characterized by a complex morphology and hydrography resulting from interactions of coastal currents and currents formed in the deep ocean, riverine input, tidal and atmospheric forcing (Gowen et al., 1995; Tett and Walne, 1995; Schrum, 1997; Orvik et al., 2001; Sundby and Drinkwater, 2007; Scharfe, 2013; Fofonova et al., 2019). Physical ocean models are powerful tools for prediction and assessment of climate change impacts on abiotic parameters. There is a rich diversity of models available to conduct climate change projections in coastal areas, such as GETM, FESOM-C, SCHISM/SELFE, NEMO, DELFT3D, ROMS, FVCOM, and others (Burchard and Bolding, 2002; Shchepetkin and McWilliams, 2005; Chen et al., 2006; Zhang and Baptista, 2008; Zhang et al., 2016; Madec et al., 2017; Androsov et al., 2019). The role of the physical models can be divided into four pieces: (1) predictions of the abiotic parameters’ distribution in space and time to supply the biogeochemical and food-web models (Hofmeister et al., 2017; Kerimoglu et al., 2017; Lemmen et al., 2018), (2) prediction of pathways of water parcels, passive and active tracers (van der Molen et al., 2018; Ricker and Stanev, 2020; Sprong et al., 2020), (3) predictions of the future “hot-spots” in a sense of largest changes in the abiotic parameters’ behavior (sensitivity studies) (Delworth et al., 2012; Yin, 2012; Schrum et al., 2016), and (4) evaluation of the representativeness of long-term observational stations for larger areas.
Making progress on identified roles requires an accurate representation of the physical environment in a coupled estuaries-shelf sea-deep ocean modeling system. The coupling of regional and large-scale models presents a challenging task since dynamics resolved by different models involve different temporal and spatial scales, and the simulations use grids of different types. Therefore, for example, upwelling events on the shelf, are not well reproduced based on one numerical solution. However, with increased computer power the larger range of process scales can be dealt within one modeling platform. Indeed, currently there are attempts to organize a seamless ocean modeling system based on available deep ocean and coastal solutions, for example, in frame of Earth System Modeling (ESM)1 or NEMO2 modeling frameworks.
Current physical ocean models extensively represent the abiotic changes and dynamics of the pelagic system and are useful for forecasting future abiotic scenarios. In contrast, food web models focus on the biological interactions in an ecosystem and possible effects of multiple stressors and management strategies. A dynamic coupling between both model types appears to be a challenging but essential step in order to achieve the realistic combination of abiotic dynamics with biotic interactions (Figure 2). The consideration of ecosystems dynamically related to the physical and biogeochemical processes requires seamless considerations of processes in a wide range of temporal and spatial scales and fundamental understanding of the dynamic response of ecosystems to the abiotic conditions. At the current stage, an ambitious goal such as the seamless dynamic coupling between food web models and physical and biogeochemical models becomes more feasible.
Figure 2. Illustration of the coupled ocean-food-web-model. The ocean model contains the physical drivers while the food web model represents biological interactions. The figure was inspired by https://www.gfdl.noaa.gov/ocean-mixing/ and https://intl.siyavula.com/read/science/grade-8/interactions-and-interdependence-within-the-environment/02-interactions-and-interdependence-within-the-environment?id=toc-id-9.
One of the main social motivations for such a coupling is deriving high quality predictions of coastal ecosystem stage and of indicators for sustainable ES management.
Holistic food web model studies focusing on the impacts of climate change on the system’s structure and functioning are still scarce. Our predictive capacities for a reliable future assessment using food web models are restricted because they consider the changes in the abiotic conditions only in a limited way. However, available model approaches trying to overcome this challenge have progressed significantly over the last decade. For example, Atlantis and EwE were used to explore possible management strategies under multi-stressor scenarios (e.g., Niiranen et al., 2013; Ortega-Cisneros et al., 2016). However, both frameworks have their limitations considered in section “Existing Food Web Model Approaches” (Table 1). These limitations underline the necessity of a seamless coupling between ocean physical and biological food web models in the future.
A major challenge is the great variability of temporal and spatial scales, considering the multi-scale nature of ecosystem dynamics as a simulated system, exacerbated by the additional involvement of multi-scale physical processes, which should be reproduced by a physical model. The simulated ecosystem and physical processes should be in agreement. This problem is relevant to both numeric and fundamental aspects and to the general understanding of the processes.
One example to highlight the challenge is the consideration of population connectivity in coastal areas with intertidal zones. Population connectivity is intricately linked to habitat heterogeneity and trophic interactions, which are biologically rich and complex in coastal areas compared to the open ocean. In the ocean numerical solutions, the vertical and spatial resolutions, the parametrization of vertical dynamics and reproduction of intertidal dynamics (wetting/drying option) are crucial to solve the connectivity task. The grid cell size is essential due to the large role of non-linear processes (e.g., bottom friction and advection of momentum) in coastal and shelf-sea dynamics. With a reduction in grid cell size, the model output can result in a completely different local circulation pattern. Concomitantly, different possible connections may be predicted between the considered positions (Sprong et al., 2020). Obviously, there is no universal answer for the optimal horizontal and vertical resolutions across all water bodies and coastal realms. The numerical consideration of relatively large areas requires an unstructured grid approach to maintain computational costs, gain geometrical flexibility and provide seamless modeling across the scales. In the case of structured grid approaches the necessity of higher resolution in some sub-areas requires nesting. However, the quality of the solution depends not only on the resolution itself but also on numerical filling of the model. For example, if the ocean model solution does not resolve the intertidal dynamics (wetting/drying option), the dynamics of higher tidal harmonics are presented incorrectly. This leads to significant errors in the simulation of transport and mixing (Stanev et al., 2016; Fofonova et al., 2019), which can play a major role in a realistic solving of connectivity tasks and in simulating ecosystem dynamics in general.
A further coupling between a chosen food web model and a physical ocean model with an irregular grid is challenging and reveals several gaps. We suggest using biomass-based food web models, such as ENA or EwE because of the empirical model input of all trophic levels. In a first step, the biomass-based food web model needs to be transferred into the unstructured grid-resolution of the ocean models. Although it is hardly possible to provide empirical data of biological components for each grid cell, approaches with the spatial-dynamic routine Ecospace have been promising and provide a suitable base for further coupling with ocean models. Additional tracer equations organize the passive exchange of materials (e.g., detritus, plankton) between the cells considering the hydrodynamics of the studied system. The inclusion of active tracers, such as fish or shrimps, remains challenging. The determination of these components in the studied area requires a formalization of their behavior (e.g., dispersal toward the best forage site) depending on different abiotic conditions. At this level, we identified a fundamental gap that requires further studies.
The next step is the representation of abiotic dynamics over a specified time and space influencing the biological components. As discussed, changes in the abiotic environment are not directly usable in most food web models but need a translation into biological responses (e.g., changes in physiology). Usually, physiological parameters are represented in the master equations (1) and (2) after Crisp (1971):
in autotrophs and
in heterotrophs. These master equations provide a crucial link to abiotic dynamics (Figure 3). Species’ physiologies are dependent on abiotic drivers such as temperature or salinity represented in physical ocean models. There are diverse studies of changes in species physiology in relation to abiotic parameters (Heinle, 1969; Pörtner and Knust, 2007; Marinov et al., 2010). One of the key challenges here is developing a theory that explicitly incorporates the separate components of climate change and their potential interactions. Some steps in this direction have been done, for example, Taherzadeh et al. (2019) proved an ability to explain when impacts become additive or non-additive based on a new size-based plankton model resolving major dependencies of plankton growth and interaction rates. Thus, each physiological parameter (i.e., production, respiration, consumption, egestion) can be represented as a function of abiotic conditions which can be implemented as a driver in coupled food web and physical ocean models. This requires combined work of ecologists, physiologists and mathematicians. Studies based on Atlantis and EwE have already achieved important steps in this direction, by altering specific parameters, such fish egg production, consumption or primary production in relation to abiotic changes. However, as discussed, climate change impacts all these parameters simultaneously. In the suggested coupled model, all physiological parameters can potentially be represented dependent on the abiotic environment.
Figure 3. Schematic coupling approach between ocean models driven by climate change and food web models via functions of physiological responses.
In the last step, additional information can be added to the coupled model. For instance, the resolution of lower trophic level dynamics can be improved by a coupling with biogeochemical models. This was already successfully done for EwE with the ECOTRAN model (Steele and Ruzicka, 2011; Ruzicka et al., 2016). With this, the information of nutrient dispersion can be included in the new approach. Furthermore, temporal-dynamic predator prey interactions need to be formalized and included to provide a realistic simulation of food web changes over space and time.
With snap-shot models, we can simulate scenarios by constructing additional setups with altered data reflecting the particular stress factor or driver. Like a film that represents a dynamic sequence of different fixed-images, the new type of coupled models should be a sequence of different holistic model scenarios to show trends and developments within total ecosystems. Multi-stressor scenarios should be the leading topic that determines the direction of the “film” and may lead to new possibilities of interpretations of ecosystem functions and services under stress. Thus, the coupled model would address the identified climate change effects on physiology and phenology of the different trophic levels. A high temporal resolution could even represent de-coupled shifts in prey availability and predator requirements, and changes of migration patterns. Furthermore, the spatial component allows for the inclusion of range shifts if environmental factors exceed tolerance limits of specific system components.
The large variety of approaches to study the ecosystem dynamics stresses the complexity of the task to investigate the impact of climate change on marine coastal ecosystems. At this stage, the synergy between approaches is intriguing, but the next step in this direction requires intensive work on cross-roads of science, large computer power and it brings numerical and fundamental challenges, as briefly discussed above. However, this step promises a more holistic and solid view on the ecosystem dynamics.
The protection of coastal ecosystems is a major target of politics and management to sustain ES. Against the background of biodiversity loss, habitat degradation, decreasing ES and climate change mitigation, there is a clear demand for precise and efficient management tools to reverse these negative trends in marine ecosystems. To achieve ecological and economical goals, ecosystem-based management (EBM) is the most relevant approach. EBM is supposed to consider all trophic levels and ES to sustain healthy and resilient ecosystems (O’Higgins et al., 2020). This becomes increasingly challenging given the multiple stressors impacting ecosystems and our limited knowledge of stressor interaction (Gissi et al., 2020). While climate change has already impacted coastal and shelf-sea ecosystems in diverse ways, local stressors add another dimension to the problem. For instance, commercial fisheries exert a constant pressure on the entire fish community and on benthic communities (Lindeboom and De Groot, 1998; Daan et al., 2005), including severe destructive effects on biogenic habitats, such as shellfish reefs (Pogoda, 2019). Effects of fishing, pollution and climate change are likely to interact with each other (Gissi et al., 2020). Accordingly, we need additional studies of stressor interactions and potential cumulative effects for a better representation in model studies.
To support EBM decisions, holistic, fine-scale and high-resolution ecosystem models, which are able to address multiple stressor effects on the entire food web are highly relevant. The suggested coupling between biomass-based food web models and physical ocean models with an unstructured grid will provide such a tool. However, while we have highlighted some technical challenges of the coupling, we also found several research gaps, which need to be addressed, such as the incorporation of active tracer behavior in models or defining the physiological responses of species to the environmental changes. In addition, a regular monitoring of the relevant ecosystem components is required to provide a solid model base. Indeed, every model we construct is just as good as the available data base. The more we know, the better we can include the information into models. Thus, detailed knowledge on climate change impacts on each trophic level, and advanced model approaches incorporating available information to provide a holistic representation are required.
SH, CM, and KW conceptualized and drafted the work. SS designed Figures 1, 2. All authors wrote and revised the manuscript and contributed expertise to their research area.
SH was partly supported by the project MERCES of the European Union’s Horizon 2020 research program (grant agreement no. 689518). CM was supported by the German Federal Ministry of Education and Research (BMBF grant no. 01LN1702A). BP was supported by the projects RESTORE and PROCEED of the German Federal Agency for Nature Conservation with funds from the German Federal Ministry for the Environment, Conservation and Nuclear Safety (FKZ 3520892013 and FKZ 3517685013).
The authors declare that the research was conducted in the absence of any commercial or financial relationships that could be construed as a potential conflict of interest.
We would like to thank the European Union’s Horizon 2020 research program (project MERCES, grant agreement number 689518), the German Federal Ministry of Education and Research (BMBF grant number 01LN1702A), and the German Federal Agency for Nature Conservation (grants 3520892013 and 3517685013) for supporting this work. Furthermore, we warmly thank our reviewers for their constructive comments, which helped to improve the manuscript substantially.
Airoldi, L., Balata, D., and Beck, M. W. (2008). The gray zone: relationships between habitat loss and marine diversity and their applications in conservation. J. Exp. Mar. Biol. Ecol. 366, 8–15. doi: 10.1016/j.jembe.2008.07.034
Aita, M. N., Yamanaka, Y., and Kishi, M. J. (2007). Interdecadal variation of the lower trophic ecosystem in the northern Pacific between 1948 and 2002, in a 3-D implementation of the NEMURO model. Ecol. Model. 202, 81–94. doi: 10.1016/j.ecolmodel.2006.07.045
Allen, J. I., Blackford, J., Holt, J., Proctor, R., Ashworth, M., and Siddorn, J. (2001). A highly spatially resolved ecosystem model for the North West European Continental Shelf. Sarsia 86, 423–440. doi: 10.1080/00364827.2001.10420484
Anderson, P. J. (2000). Pandalid shrimp as indicators of ecosystem regime shift. J. Northw. Atlant. Fish. Sci. 27, 1–10. doi: 10.2960/j.v27.a1
Andreae, M. O., and Crutzen, P. J. (1997). Atmospheric aerosols: biogeochemical sources and role in atmospheric chemistry. Science 276, 1052–1058. doi: 10.1126/science.276.5315.1052
Androsov, A., Fofonova, V., Kuznetsov, I., Danilov, S., Rakowsky, N., Harig, S., et al. (2019). FESOM-C v. 2: coastal dynamics on hybrid unstructured meshes. Geosci. Model Dev. 12, 1009–1028. doi: 10.5194/gmd-12-1009-2019
Atkins, J. P., Burdon, D., and Elliott, M. (2015). “Identification of a practicable set of ecosystem indicators for coastal and marine ecosystem services,” in Coastal Zones Ecosystem Services, eds R. K. Turner and M. Schaafsma (Berlin: Springer), 79–102. doi: 10.1007/978-3-319-17214-9_5
Aumont, O., Éthé, C., Tagliabue, A., Bopp, L., and Gehlen, M. (2015). PISCES-v2: an ocean biogeochemical model for carbon and ecosystem studies. Geosci. Model Dev. Discuss. 8, 2465–2513. doi: 10.5194/gmd-8-2465-2015
Aumont, O., Maier-Reimer, E., Blain, S., and Monfray, P. (2003). An ecosystem model of the global ocean including Fe, Si, P colimitations. Glob. Biogeochem. Cycles 17:GB001745.
Baird, D., Asmus, H., and Asmus, R. (2004). Energy flow of a boreal intertidal ecosystem, the sylt-rømø bight. Mar. Ecol. Prog. Ser. 279, 45–61. doi: 10.3354/meps279045
Baird, D., Asmus, H., Asmus, R., Horn, S., and de la Vega, C. (2019). Ecosystem response to increasing ambient water temperatures due to climate warming in the Sylt-Rømø Bight, northern Wadden Sea, Germany. Estuar. Coast. Shelf Sci. 228:106322. doi: 10.1016/j.ecss.2019.106322
Barbier, E. B., Hacker, S. D., Kennedy, C., Koch, E. W., Stier, A. C., and Silliman, B. R. (2011). The value of estuarine and coastal ecosystem services. Ecol. Monogr. 81, 169–193.
Bayne, B. (2017). Oysters and the ecosystem. Dev. Aquac. Fish. Sci. 41, 703–834. doi: 10.1016/b978-0-12-803472-9.00010-8
Beaugrand, G., Brander, K. M., Lindley, J. A., Souissi, S., and Reid, P. C. (2003). Plankton effect on cod recruitment in the North Sea. Nature 426, 661–664. doi: 10.1038/nature02164
Bentley, J. W., Serpetti, N., and Heymans, J. J. (2017). Investigating the potential impacts of ocean warming on the Norwegian and Barents Seas ecosystem using a time-dynamic food-web model. Ecol. Model. 360, 94–107. doi: 10.1016/j.ecolmodel.2017.07.002
Bergquist, A. M., Carpenter, S. R., and Latino, J. C. (1985). Shifts in phytoplankton size structure and community composition during grazing by contrasting zooplankton assemblages 1. Limnol. Oceanogr. 30, 1037–1045. doi: 10.4319/lo.1985.30.5.1037
Beukema, J., and Dekker, R. (2005). Decline of recruitment success in cockles and other bivalves in the Wadden Sea: possible role of climate change, predation on postlarvae and fisheries. Mar. Ecol. Prog. Ser. 287, 149–167. doi: 10.3354/meps287149
Bingham, F. M., and Lukas, R. (1996). Seasonal cycles of temperature, salinity and dissolved oxygen observed in the Hawaii Ocean Time-series. Deep Sea Res. Part II Top. Stud. Oceanogr. 43, 199–213. doi: 10.1016/0967-0645(95)00090-9
Binzer, A., Guill, C., Rall, B. C., and Brose, U. (2016). Interactive effects of warming, eutrophication and size structure: impacts on biodiversity and food-web structure. Glob. Change Biol. 22, 220–227. doi: 10.1111/gcb.13086
Birchenough, S. N., Reiss, H., Degraer, S., Mieszkowska, N., Borja, Á, Buhl-Mortensen, L., et al. (2015). Climate change and marine benthos: a review of existing research and future directions in the North Atlantic. Wiley Interdiscip. Rev. Clim. Change 6, 203–223. doi: 10.1002/wcc.330
Boersma, M., Malzahn, A. M., Greve, W., and Javidpour, J. (2007). The first occurrence of the ctenophore Mnemiopsis leidyi in the North Sea. Helgoland Mar. Res. 61, 153–155. doi: 10.1007/s10152-006-0055-2
Bopp, L., Aumont, O., Cadule, P., Alvain, S., and Gehlen, M. (2005). Response of diatoms distribution to global warming and potential implications: a global model study. Geophys. Res. Lett. 32:GL023653.
Bopp, L., Boucher, O., Aumont, O., Belviso, S., Dufresne, J.-L., Pham, M., et al. (2004). Will marine dimethylsulfide emissions amplify or alleviate global warming? A model study. Can. J. Fish. Aquat. Sci. 61, 826–835. doi: 10.1139/f04-045
Bryndum-Buchholz, A., Boyce, D. G., Tittensor, D. P., Christensen, V., Bianchi, D., and Lotze, H. K. (2020). Climate-change impacts and fisheries management challenges in the North Atlantic Ocean. Mar. Ecol. Prog. Ser. 648, 1–17. doi: 10.3354/meps13438
Burchard, H., and Bolding, K. (2002). GETM–a General Estuarine Transport Model. Scientific documentation, no EUR 20253. Brussels: European Commission.
Capuzzo, E., Lynam, C. P., Barry, J., Stephens, D., Forster, R. M., Greenwood, N., et al. (2018). A decline in primary production in the North Sea over 25 years, associated with reductions in zooplankton abundance and fish stock recruitment. Glob. Change Biol. 24, e352–e364.
Carter, J. L., Schindler, D. E., and Francis, T. B. (2017). Effects of climate change on zooplankton community interactions in an Alaskan lake. Clim. Change Responses 4, 1–12.
Charlson, R. J., Lovelock, J. E., Andreae, M. O., and Warren, S. G. (1987). Oceanic phytoplankton, atmospheric sulphur, cloud albedo and climate. Nature 326, 655–661. doi: 10.1038/326655a0
Checkley, D. M. Jr., Asch, R. G., and Rykaczewski, R. R. (2017). Climate, anchovy, and sardine. Annu. Rev. Mar. Sci. 9, 469–493. doi: 10.1146/annurev-marine-122414-033819
Chen, B., Landry, M. R., Huang, B., and Liu, H. (2012). Does warming enhance the effect of microzooplankton grazing on marine phytoplankton in the ocean? Limnol. Oceanogr. 57, 519–526. doi: 10.4319/lo.2012.57.2.0519
Chen, C., Beardsley, R., and Cowles, G. (2006). An unstructured grid, finite-volume coastal ocean model (FVCOM) system. Oceanography 19, 78–89. doi: 10.5670/oceanog.2006.92
Christensen, V., and Walters, C. J. (2004). Ecopath with Ecosim: methods, capabilities and limitations. Ecol. Model. 172, 109–139. doi: 10.1016/j.ecolmodel.2003.09.003
Coll, M., Akoglu, E., Arreguin-Sanchez, F., Fulton, E., Gascuel, D., Heymans, J., et al. (2015). Modelling dynamic ecosystems: venturing beyond boundaries with the Ecopath approach. Rev. Fish Biol. Fish. 25, 413–424. doi: 10.1007/s11160-015-9386-x
Coll, M., Schmidt, A., Romanuk, T., and Lotze, H. K. (2011). Food-web structure of seagrass communities across different spatial scales and human impacts. PLoS One 6:e22591. doi: 10.1371/journal.pone.0022591
Cranford, P. J. (2019). “Magnitude and extent of water clarification services provided by bivalve suspension feeding,” in Goods and Services of Marine Bivalves, eds Ø Strand, J. G. Ferreira, and J. K. Petersen (Cham: Springer), 119–141. doi: 10.1007/978-3-319-96776-9_8
Crisp, D. (1971). “Energy flow measurements,” in Methods for the study of marine benthos, ed. A. Eleftheriou (Hoboken, NJ: Wiley).
Cushing, D. (1969). The regularity of the spawning season of some fishes. ICES J. Mar. Sci. 33, 81–92. doi: 10.1093/icesjms/33.1.81
Daan, N., Bromley, P., Hislop, J., and Nielsen, N. (1990). Ecology of North sea fish. Netherlands J. Sea Res. 26, 343–386. doi: 10.1016/0077-7579(90)90096-y
Daan, N., Gislason, H., Pope, J. G., and Rice, J. C. (2005). Changes in the North Sea fish community: evidence of indirect effects of fishing? ICES J. Mar. Sci. 62, 177–188. doi: 10.1016/j.icesjms.2004.08.020
Dadou, I., Garçon, V., Andersen, V., Flierl, G., and Davis, C. (1996). Impact of the North Equatorial Current meandering on a pelagic ecosystem: a modeling approach. J. Mar. Res. 54, 311–342. doi: 10.1357/0022240963213411
Daewel, U., Peck, M. A., Kuehn, W., John, M. A. S. T., Alekseeva, I., and Schrum, C. (2008). Coupling ecosystem and individual-based models to simulate the influence of environmental variability on potential growth and survival of larval sprat (Sprattus sprattus L.) in the North Sea. Fish. Oceanogr. 17, 333–351. doi: 10.1111/j.1365-2419.2008.00482.x
Daewel, U., Schrum, C., and Macdonald, J. I. (2019). Towards end-to-end (E2E) modelling in a consistent NPZD-F modelling framework (ECOSMO E2E_v1. 0): application to the North Sea and Baltic Sea. Geosci. Model Dev. 12, 1765–1789. doi: 10.5194/gmd-12-1765-2019
Dam, H. G., and Peterson, W. T. (1988). The effect of temperature on the gut clearance rate constant of planktonic copepods. J. Exp. Mar. Biol. Ecol. 123, 1–14. doi: 10.1016/0022-0981(88)90105-0
de la Vega, C., Schückel, U., Horn, S., Kröncke, I., Asmus, R., and Asmus, H. (2018). How to include ecological network analysis results in management? A case study of three tidal basins of the Wadden Sea, south-eastern North Sea. Ocean Coast. Manag. 163, 401–416. doi: 10.1016/j.ocecoaman.2018.07.019
de Mutsert, K., Steenbeek, J., Lewis, K., Buszowski, J., Cowan, J. H. Jr., and Christensen, V. (2016). Exploring effects of hypoxia on fish and fisheries in the northern Gulf of Mexico using a dynamic spatially explicit ecosystem model. Ecol. Model. 331, 142–150. doi: 10.1016/j.ecolmodel.2015.10.013
Delworth, T. L., Rosati, A., Anderson, W., Adcroft, A. J., Balaji, V., Benson, R., et al. (2012). Simulated climate and climate change in the GFDL CM2. 5 high-resolution coupled climate model. J. Clim. 25, 2755–2781. doi: 10.1175/jcli-d-11-00316.1
Dempsey, D. P., Gentleman, W. C., Pepin, P., and Koen-Alonso, M. (2018). Explanatory power of human and environmental pressures on the fish community of the grand bank before and after the biomass collapse. Front. Mar. Sci. 5:37. doi: 10.3389/fmars.2018.00037
Ding, Q., Schweiger, A., L’Heureux, M., Steig, E. J., Battisti, D. S., Johnson, N. C., et al. (2019). Fingerprints of internal drivers of Arctic sea ice loss in observations and model simulations. Nat. Geosci. 12, 28–33. doi: 10.1038/s41561-018-0256-8
Dulvy, N. K., Rogers, S. I., Jennings, S., Stelzenmüller, V., Dye, S. R., and Skjoldal, H. R. (2008). Climate change and deepening of the North Sea fish assemblage: a biotic indicator of warming seas. J. Appl. Ecol. 45, 1029–1039. doi: 10.1111/j.1365-2664.2008.01488.x
Eisner, L. B., Napp, J. M., Mier, K. L., Pinchuk, A. I., and Andrews, A. G. III (2014). Climate-mediated changes in zooplankton community structure for the eastern Bering Sea. Deep Sea Res. II Top. Stud. Oceanogr. 109, 157–171. doi: 10.1016/j.dsr2.2014.03.004
European Commission (2008). Directive 2008/56/EC of the European Parliament and of the Council of 17 June 2008 establishing a framework for community action in the field of marine environmental policy (Marine Strategy Framework Directive). Off. J. Eur. Union 164, 19–40.
Falkowski, P. G. (1994). The role of phytoplankton photosynthesis in global biogeochemical cycles. Photosyn. Res. 39, 235–258. doi: 10.1007/bf00014586
Falkowski, P. G., Barber, R. T., and Smetacek, V. (1998). Biogeochemical controls and feedbacks on ocean primary production. Science 281, 200–206. doi: 10.1126/science.281.5374.200
FAO (2018). The State of World Fisheries and Aquaculture 2018–Meeting the Sustainable Development Goals. Rome: FAO.
Fasham, M. J. (1993). “Modelling the marine biota,” in The Global Carbon Cycle, ed. M. Heimann (Berlin: Springer), 457–504. doi: 10.1007/978-3-642-84608-3_19
Fath, B. D., Asmus, H., Asmus, R., Baird, D., Borrett, S. R., de Jonge, V. N., et al. (2019). Ecological network analysis metrics: the need for an entire ecosystem approach in management and policy. Ocean Coast. Manag. 174, 1–14. doi: 10.1016/j.ocecoaman.2019.03.007
Field, C. B., Behrenfeld, M. J., Randerson, J. T., and Falkowski, P. (1998). Primary production of the biosphere: integrating terrestrial and oceanic components. Science 281, 237–240. doi: 10.1126/science.281.5374.237
Fofonova, V., Androsov, A., Sander, L., Kuznetsov, I., Amorim, F., Hass, H. C., et al. (2019). Non-linear aspects of the tidal dynamics in the Sylt-Rømø Bight, south-eastern North Sea. Ocean Sci. 15, 1761–1782. doi: 10.5194/os-15-1761-2019
Franks, P., and Chen, C. (2001). A 3-D prognostic model study of the ecosystem over Georges Bank and adjacent coastal regions. Part II: coupled biological and physical model. Deep Sea Res. 48, 457–482. doi: 10.1016/s0967-0645(00)00125-9
Fulton, E., Smith, A. D., and Smith, D. C. (2007). Alternative Management Strategies For Southeast Australian Commonwealth Fisheries: stage 2: Quantitative Management Strategy Evaluation. Hobart: Commonwealth Scientific and Industrial Research Organisation (CSIRO).
Fulton, E. A. (2010). Approaches to end-to-end ecosystem models. J. Mar. Syst. 81, 171–183. doi: 10.1016/j.jmarsys.2009.12.012
Fulton, E. A., Fuller, M., Smith, A., and Punt, A. (2004). Ecological Indicators of the Ecosystem Effects of Fishing. Hobart: Commonwealth Scientific and Industrial Research Organisation (CSIRO).
Fulton, E. A., Smith, A. D., and Punt, A. E. (2005). Which ecological indicators can robustly detect effects of fishing? ICES J. Mar. Sci. 62, 540–551. doi: 10.1016/j.icesjms.2004.12.012
Gazeau, F., Quiblier, C., Jansen, J. M., Gattuso, J. P., Middelburg, J. J., and Heip, C. H. (2007). Impact of elevated CO2 on shellfish calcification. Geophys. Res. Lett. 34:GL028554.
Geary, W. L., Bode, M., Doherty, T. S., Fulton, E. A., Nimmo, D. G., Tulloch, A. I., et al. (2020). A guide to ecosystem models and their environmental applications. Nat. Ecol. Evol. 4, 1459–1471.
Gissi, E., Manea, E., Mazaris, A. D., Fraschetti, S., Almpanidou, V., Bevilacqua, S., et al. (2020). A review of the combined effects of climate change and other local human stressors on the marine environment. Sci. Total Environ. 2020:142564. doi: 10.1016/j.scitotenv.2020.142564
Gittings, J. A., Raitsos, D. E., Krokos, G., and Hoteit, I. (2018). Impacts of warming on phytoplankton abundance and phenology in a typical tropical marine ecosystem. Sci. Rep. 8, 1–12.
Gowen, R., Stewart, B., Mills, D., and Elliott, P. (1995). Regional differences in stratification and its effect on phytoplankton production and biomass in the northwestern Irish Sea. J. Plankton Res. 17, 753–769. doi: 10.1093/plankt/17.4.753
Granéli, E., Granéli, W., Rabbani, M. M., Daugbjerg, N., Fransz, G., Roudy, J. C., et al. (1993). The influence of copepod and krill grazing on the species composition of phytoplankton communities from the Scotia Weddell sea. Polar Biol. 13, 201–213. doi: 10.1007/bf00238930
Grant, W. S., and Bowen, B. W. (2006). Living in a tilted world: climate change and geography limit speciation in Old World anchovies (Engraulis; Engraulidae). Biol. J. Linn. Soc. 88, 673–689. doi: 10.1111/j.1095-8312.2006.00651.x
Greene, C. H., Meyer-Gutbrod, E., Monger, B. C., McGarry, L. P., Pershing, A. J. I, Belkin, M., et al. (2013). Remote climate forcing of decadal-scale regime shifts in Northwest Atlantic shelf ecosystems. Limnol. Oceanogr. 58, 803–816. doi: 10.4319/lo.2013.58.3.0803
Guyondet, T., Comeau, L., Bacher, C., Grant, J., Rosland, R., Sonier, R., et al. (2015). Climate change influences carrying capacity in a coastal embayment dedicated to shellfish aquaculture. Estua. Coasts 38, 1593–1618. doi: 10.1007/s12237-014-9899-x
Hansen, C., Drinkwater, K. F., Jähkel, A., Fulton, E. A., Gorton, R., and Skern-Mauritzen, M. (2019). Sensitivity of the Norwegian and Barents Sea Atlantis end-to-end ecosystem model to parameter perturbations of key species. PLoS One 14:e0210419. doi: 10.1371/journal.pone.0210419
He, Q., and Silliman, B. R. (2019). Climate change, human impacts, and coastal ecosystems in the Anthropocene. Curr. Biol. 29, R1021–R1035.
Heath, M. R., Neat, F. C., Pinnegar, J. K., Reid, D. G., Sims, D. W., and Wright, P. J. (2012). Review of climate change impacts on marine fish and shellfish around the UK and Ireland. Aquat. Conserv. Mar. Freshw. Ecosyst. 22, 337–367. doi: 10.1002/aqc.2244
Heinle, D. R. (1969). Temperature and zooplankton. Chesapeake Sci. 10, 186–209. doi: 10.2307/1350456
Henson, S. A., Cole, H. S., Hopkins, J., Martin, A. P., and Yool, A. (2018). Detection of climate change-driven trends in phytoplankton phenology. Glob. Change Biol. 24, e101–e111.
Hofmeister, R., Flöser, G., and Schartau, M. (2017). Estuary-type circulation as a factor sustaining horizontal nutrient gradients in freshwater-influenced coastal systems. Geo Mar. Lett. 37, 179–192. doi: 10.1007/s00367-016-0469-z
Hollowed, A. B., Holsman, K. K., Haynie, A. C., Hermann, A. J., Punt, A. E., Aydin, K., et al. (2020). Integrated modeling to evaluate climate change impacts on coupled social-ecological systems in Alaska. Front. Mar. Sci. 6:775. doi: 10.3389/fmars.2019.00775
Horn, S., de la Vega, C., Asmus, R., Schwemmer, P., Enners, L., Garthe, S., et al. (2019). Impact of birds on intertidal food webs assessed with ecological network analysis. Estua. Coast. Shelf Sci. 219, 107–119. doi: 10.1016/j.ecss.2019.01.023
Howell, D., Schueller, A. M., Bentley, J. W., Buchheister, A., Chagaris, D., Cieri, M., et al. (2021). Combining ecosystem and single-species modeling to provide ecosystem-based fisheries management advice within current management systems. Front. Mar. Sci. 7:1163. doi: 10.3389/fmars.2020.607831
ICES (2014). Annex 12 Stock Sandeel in IV. Quality Handbook Annex SAN-NSEA Stock-Specific Documentation of Standard Assessment Procedures. Copenhagen: ICES.
ICSU (2017). Guide To SDG Interactions: From Science to implementation. Paris: International Council for Science.
Itoh, S., Takeshige, A., Kasai, A., and Kimura, S. (2018). Modeling the coastal ecosystem complex: present situation and challenges. Fish. Sci. 84, 293–307. doi: 10.1007/s12562-018-1181-x
Jaspers, C., Huwer, B., Antajan, E., Hosia, A., Hinrichsen, H. H., Biastoch, A., et al. (2018). Ocean current connectivity propelling the secondary spread of a marine invasive comb jelly across western Eurasia. Glob. Ecol. Biogeogr. 27, 814–827. doi: 10.1111/geb.12742
Jochum, M., Schneider, F. D., Crowe, T. P., Brose, U., and O’Gorman, E. J. (2012). Climate-induced changes in bottom-up and top-down processes independently alter a marine ecosystem. Philos. Trans. R. Soc. B Biol. Sci. 367, 2962–2970. doi: 10.1098/rstb.2012.0237
Johns, D., Edwards, M., Greve, W., and Sjohn, A. (2005). Increasing prevalence of the marine cladoceran Penilia avirostris (Dana, 1852) in the North Sea. Helgoland Mar. Res. 59, 214–218. doi: 10.1007/s10152-005-0221-y
Jørgensen, S. E. (2008). Overview of the model types available for development of ecological models. Ecol. Model. 215, 3–9. doi: 10.1016/j.ecolmodel.2008.02.041
Kay, J. J., Graham, L. A., and Ulanowicz, R. E. (1989). “A detailed guide to network analysis,” in Network Analysis in Marine Ecology, eds F. Wulff and J. G. Field (Berlin: Springer), 15–61. doi: 10.1007/978-3-642-75017-5_2
Kerimoglu, O., Hofmeister, R., Maerz, J., Riethmüller, R., and Wirtz, K. W. (2017). The acclimative biogeochemical model of the southern North Sea. Biogeosciences 14, 4499–4531. doi: 10.5194/bg-14-4499-2017
Kirby, R. R., Beaugrand, G., and Lindley, J. A. (2008). Climate-induced effects on the meroplankton and the benthic-pelagic ecology of the North Sea. Limnol. Oceanogr. 53, 1805–1815. doi: 10.4319/lo.2008.53.5.1805
Kleisner, K. M., Fogarty, M. J., McGee, S., Hare, J. A., Moret, S., Perretti, C. T., et al. (2017). Marine species distribution shifts on the US Northeast Continental Shelf under continued ocean warming. Prog. Oceanogr. 153, 24–36. doi: 10.1016/j.pocean.2017.04.001
Kortsch, S., Primicerio, R., Fossheim, M., Dolgov, A. V., and Aschan, M. (2015). Climate change alters the structure of arctic marine food webs due to poleward shifts of boreal generalists. Proc. R. Soc. B Biol. Sci. 282:20151546. doi: 10.1098/rspb.2015.1546
Le Corre, N., Pepin, P., Burmeister, A., Walkusz, W., Skanes, K., Wang, Z., et al. (2020). Larval connectivity of Northern Shrimp (Pandalus borealis) in the northwest Atlantic. Can. J. Fish. Aquat. Sci. [Epub ahead of print].
Leles, S. G., Valentin, J. L., and Figueiredo, G. M. (2016). Evaluation of the complexity and performance of marine planktonic trophic models. Anais da Acad. Brasil. Ciências 88, 1971–1991. doi: 10.1590/0001-3765201620150588
Lemmen, C., Hofmeister, R., Klingbeil, K., Nasermoaddeli, M. H., Kerimoglu, O., Burchard, H., et al. (2018). Modular System for Shelves and Coasts (MOSSCO v1. 0)–a flexible and multi-component framework for coupled coastal ocean ecosystem modelling. Geosci. Model Dev. 11, 915–935. doi: 10.5194/gmd-11-915-2018
Lennox, R. J., Paukert, C. P., Aarestrup, K., Auger-Méthé, M., Baumgartner, L., Birnie-Gauvin, K., et al. (2019). One hundred pressing questions on the future of global fish migration science, conservation, and policy. Front. Ecol. Evol. 7:286. doi: 10.3389/fevo.2019.00286
Lindeboom, H., and De Groot, S. (1998). IMPACT-II: The Effects of Different Types of Fisheries on the North Sea and Irish Sea Benthic Ecosystems. The Hague: Netherlands Institute for Fisheries Research.
Lindegren, M., Blenckner, T., and Stenseth, N. C. (2012). Nutrient reduction and climate change cause a potential shift from pelagic to benthic pathways in a eutrophic marine ecosystem. Glob. Change Biol. 18, 3491–3503. doi: 10.1111/j.1365-2486.2012.02799.x
Liquete, C., Piroddi, C., Drakou, E. G., Gurney, L., Katsanevakis, S., Charef, A., et al. (2013). Current status and future prospects for the assessment of marine and coastal ecosystem services: a systematic review. PLoS One 8:e67737. doi: 10.1371/journal.pone.0067737
Madec, G., Bourdallé-Badie, R., Bouttier, P., Bricaud, C., Bruciaferri, D., Calvert, D., et al. (2017). NEMO Ocean Engine (Version v3. 6). Zenodo: Du Pôle De Modélisation De L’institut Pierre-simon Laplace (IPSL).
Marinov, I., Doney, S., and Lima, I. (2010). Response of ocean phytoplankton community structure to climate change over the 21st century: partitioning the effects of nutrients, temperature and light. Biogeosciences 7:3941. doi: 10.5194/bg-7-3941-2010
Megrey, B. A., Rose, K. A., Klumb, R. A., Hay, D. E., Werner, F. E., Eslinger, D. L., et al. (2007). A bioenergetics-based population dynamics model of Pacific herring (Clupea harengus pallasi) coupled to a lower trophic level nutrient–phytoplankton–zooplankton model: description, calibration, and sensitivity analysis. Ecol. Model. 202, 144–164. doi: 10.1016/j.ecolmodel.2006.08.020
Meunier, C. L., Boersma, M., Wiltshire, K. H., and Malzahn, A. M. (2016). Zooplankton eat what they need: copepod selective feeding and potential consequences for marine systems. Oikos 125, 50–58. doi: 10.1111/oik.02072
Meunier, C. L., Malzahn, A. M., and Boersma, M. (2014). A new approach to homeostatic regulation: towards a unified view of physiological and ecological concepts. PLoS One 9:e107737. doi: 10.1371/journal.pone.0107737
Millennium Assessment Board (2005). Millennium Ecosystem Assessment. Washington, DC: Millennium Assessment Board.
Möllmann, C., Diekmann, R., Müller-Karulis, B., Kornilovs, G., Plikshs, M., and Axe, P. (2009). Reorganization of a large marine ecosystem due to atmospheric and anthropogenic pressure: a discontinuous regime shift in the Central Baltic Sea. Glob. Change Biol. 15, 1377–1393. doi: 10.1111/j.1365-2486.2008.01814.x
Muller-Karger, F. E., Varela, R., Thunell, R., Luerssen, R., Hu, C., and Walsh, J. J. (2005). The importance of continental margins in the global carbon cycle. Geophys. Res. Lett. 32:GL021346.
Niiranen, S., Yletyinen, J., Tomczak, M. T., Blenckner, T., Hjerne, O., MacKenzie, B. R., et al. (2013). Combined effects of global climate change and regional ecosystem drivers on an exploited marine food web. Glob. Change Biol. 19, 3327–3342.
O’Higgins, T. G., Lago, M., and DeWitt, T. H. (2020). Ecosystem-Based Management, Ecosystem Services and Aquatic Biodiversity: Theory, Tools and Applications. Cham: Springer.
Ortega-Cisneros, K., Scharler, U., and Whitfield, A. (2016). Carbon and nitrogen system dynamics in three small South African estuaries, with particular emphasis on the influence of seasons, river flow and mouth state. Mar. Ecol. Prog. Ser. 557, 17–30. doi: 10.3354/meps11870
Orvik, K. A., and Skagseth, Ø, and Mork, M. (2001). Atlantic inflow to the Nordic Seas: current structure and volume fluxes from moored current meters, VM-ADCP and SeaSoar-CTD observations, 1995–1999. Deep Sea Res. Part I Oceanogr. Res. Pap. 48, 937–957. doi: 10.1016/s0967-0637(00)00038-8
Ouellet, P., Chabot, D., Calosi, P., Orr, D., and Galbraith, P. S. (2017). Regional variations in early life stages response to a temperature gradient in the northern shrimp Pandalus borealis and vulnerability of the populations to ocean warming. J. Exp. Mar. Biol. Ecol. 497, 50–60. doi: 10.1016/j.jembe.2017.09.007
Pogoda, B. (2019). Current status of European oyster decline and restoration in Germany. Humanities 8:9. doi: 10.3390/h8010009
Pogoda, B., Merk, V., Colsoul, B., Hausen, T., Peter, C., Pesch, R., et al. (2020). Site selection for biogenic reef restoration in offshore environments: the Natura 2000 area Borkum reef ground as a case study for native oyster restoration. Aquat. Conserv. Mar. Freshw. Ecosyst. 30, 2163–2179. doi: 10.1002/aqc.3405
Poloczanska, E. S., Brown, C. J., Sydeman, W. J., Kiessling, W., Schoeman, D. S., Moore, P. J., et al. (2013). Global imprint of climate change on marine life. Nat. Clim. Change 3, 919–925.
Pörtner, H. O., and Knust, R. (2007). Climate change affects marine fishes through the oxygen limitation of thermal tolerance. Science 315, 95–97. doi: 10.1126/science.1135471
Pörtner, H. O., and Peck, M. (2010). Climate change effects on fishes and fisheries: towards a cause-and-effect understanding. J. Fish Biol. 77, 1745–1779. doi: 10.1111/j.1095-8649.2010.02783.x
Reid, P., Colebrook, J., Matthews, J., Aiken, J., and Team, C. P. R. (2003). The continuous plankton recorder: concepts and history, from Plankton Indicator to undulating recorders. Prog. Oceanogr. 58, 117–173. doi: 10.1016/j.pocean.2003.08.002
Reid, P. C. (1975). Large scale changes in North Sea phytoplankton. Nature 257, 217–219. doi: 10.1038/257217a0
Richards, R. A. (2012). Phenological shifts in hatch timing of northern shrimp Pandalus borealis. Mar. Ecol. Prog. Ser. 456, 149–158. doi: 10.3354/meps09717
Richardson, A., Walne, A., John, A., Jonas, T., Lindley, J., Sims, D., et al. (2006). Using continuous plankton recorder data. Prog. Oceanogr. 68, 27–74. doi: 10.1016/j.pocean.2005.09.011
Richardson, A. J. (2008). In hot water: zooplankton and climate change. ICES J. Mar. Sci. 65, 279–295. doi: 10.1093/icesjms/fsn028
Richardson, A. J., and Schoeman, D. S. (2004). Climate impact on plankton ecosystems in the Northeast Atlantic. Science 305, 1609–1612. doi: 10.1126/science.1100958
Ricker, M., and Stanev, E. V. (2020). Circulation of the European northwest shelf: a lagrangian perspective. Ocean Science 16, 637–655. doi: 10.5194/os-16-637-2020
Riebesell, U. (2004). Effects of CO 2 enrichment on marine phytoplankton. J. Oceanogr. 60, 719–729. doi: 10.1007/s10872-004-5764-z
Rijnsdorp, A. D., Peck, M. A., Engelhard, G. H., Möllmann, C., and Pinnegar, J. K. (2009). Resolving the effect of climate change on fish populations. ICES J. Mar. Sci. 66, 1570–1583. doi: 10.1093/icesjms/fsp056
Rose, K. A., Megrey, B. A., Hay, D., Werner, F., and Schweigert, J. (2008). Climate regime effects on Pacific herring growth using coupled nutrient-phytoplankton-zooplankton and bioenergetics models. Trans. Am. Fish. Soc. 137, 278–297. doi: 10.1577/t05-152.1
Rowley, A. F., Cross, M. E., Culloty, S. C., Lynch, S. A., Mackenzie, C. L., Morgan, E., et al. (2014). The potential impact of climate change on the infectious diseases of commercially important shellfish populations in the Irish Sea—a review. ICES J. Mar. Sci. 71, 741–759. doi: 10.1093/icesjms/fst234
Ruzicka, J. J., Brink, K. H., Gifford, D. J., and Bahr, F. (2016). A physically coupled end-to-end model platform for coastal ecosystems: simulating the effects of climate change and changing upwelling characteristics on the Northern California Current ecosystem. Ecol. Model. 331, 86–99. doi: 10.1016/j.ecolmodel.2016.01.018
Sabine, C. L., Feely, R. A., Gruber, N., Key, R. M., Lee, K., Bullister, J. L., et al. (2004). The oceanic sink for anthropogenic CO2. Science 305, 367–371. doi: 10.1126/science.1097403
Safi, G., Giebels, D., Arroyo, N. L., Heymans, J. J., Preciado, I., Raoux, A., et al. (2019). Vitamine ENA: a framework for the development of ecosystem-based indicators for decision makers. Ocean Coast. Manag. 174, 116–130. doi: 10.1016/j.ocecoaman.2019.03.005
Sarker, S., Feudel, U., Meunier, C. L., Lemke, P., Dutta, P. S., and Wiltshire, K. H. (2018). To share or not to share? Phytoplankton species coexistence puzzle in a competition model incorporating multiple resource-limitation and synthesizing unit concepts. Ecol. Model. 383, 150–159. doi: 10.1016/j.ecolmodel.2018.05.021
Sarker, S., Yadav, A. K., Akter, M., Hossain, M. S., Chowdhury, S. R., Kabir, M. A., et al. (2020). Rising temperature and marine plankton community dynamics: Is warming bad? Ecol. Complex. 43:100857. doi: 10.1016/j.ecocom.2020.100857
Scharfe, M. (2013). Analyse Biologischer Langzeitveränderungen auf Basis Hydroklimatischer Parameter in Der Südlichen Nordsee (Helgoland). Ph.D. thesis. Hamburg: Universität Hamburg.
Scharfe, M., and Wiltshire, K. H. (2019). Modeling of intra-annual abundance distributions: constancy and variation in the phenology of marine phytoplankton species over five decades at Helgoland Roads (North Sea). Ecol. Model. 404, 46–60. doi: 10.1016/j.ecolmodel.2019.01.001
Scharler, U. M., and Baird, D. (2005). A comparison of selected ecosystem attributes of three South African estuaries with different freshwater inflow regimes, using network analysis. J. Mar. Syst. 56, 283–308. doi: 10.1016/j.jmarsys.2004.12.003
Schindler, D. E., Rogers, D. E., Scheuerell, M. D., and Abrey, C. A. (2005). Effects of changing climate on zooplankton and juvenile sockeye salmon growth in southwestern Alaska. Ecology 86, 198–209. doi: 10.1890/03-0408
Schoo, K. L., Malzahn, A. M., Krause, E., and Boersma, M. (2013). Increased carbon dioxide availability alters phytoplankton stoichiometry and affects carbon cycling and growth of a marine planktonic herbivore. Mar. Biol. 160, 2145–2155. doi: 10.1007/s00227-012-2121-4
Schrum, C. (1997). Thermohaline stratification and instabilities at tidal mixing fronts: results of an eddy resolving model for the German Bight. Continent. Shelf Res. 17, 689–716. doi: 10.1016/s0278-4343(96)00051-9
Schrum, C., Alekseeva, I., and John, M. S. (2006a). Development of a coupled physical–biological ecosystem model ECOSMO: part I: model description and validation for the North Sea. J. Mar. Syst. 61, 79–99. doi: 10.1016/j.jmarsys.2006.01.005
Schrum, C., John, M. S., and Alekseeva, I. (2006b). ECOSMO, a coupled ecosystem model of the North Sea and Baltic Sea: part II. Spatial-seasonal characteristics in the North Sea as revealed by EOF analysis. J. Mar. Syst. 61, 100–113. doi: 10.1016/j.jmarsys.2006.01.004
Schrum, C., Lowe, J., Meier, H. M., Grabemann, I., Holt, J., Mathis, M., et al. (2016). “Projected change—North sea,” in North Sea Region Climate Change Assessment, eds M. Quante and F. Colijn (Cham: Springer), 175–217. doi: 10.1007/978-3-319-39745-0_6
Schückel, U., Kröncke, I., and Baird, D. (2015). Linking long-term changes in trophic structure and function of an intertidal macrobenthic system to eutrophication and climate change using ecological network analysis. Mar. Ecol. Prog. Ser. 536, 25–38. doi: 10.3354/meps11391
Schulte, D. M., Burke, R. P., and Lipcius, R. N. (2009). Unprecedented restoration of a native oyster metapopulation. Science 325, 1124–1128. doi: 10.1126/science.1176516
Sekerci, Y., and Ozarslan, R. (2020). Oxygen-plankton model under the effect of global warming with nonsingular fractional order. Chaos Solitons Fractals 132:109532. doi: 10.1016/j.chaos.2019.109532
Sekerci, Y., and Petrovskii, S. (2015). Mathematical modelling of plankton–oxygen dynamics under the climate change. Bull. Math. Biol. 77, 2325–2353. doi: 10.1007/s11538-015-0126-0
Serpetti, N., Baudron, A. R., Burrows, M., Payne, B. L., Helaouet, P., Fernandes, P. G., et al. (2017). Impact of ocean warming on sustainable fisheries management informs the ecosystem approach to fisheries. Sci. Rep. 7, 1–15. doi: 10.1163/9789004242005_002
Shchepetkin, A. F., and McWilliams, J. C. (2005). The regional oceanic modeling system (ROMS): a split-explicit, free-surface, topography-following-coordinate oceanic model. Ocean Model. 9, 347–404. doi: 10.1016/j.ocemod.2004.08.002
Sims, D. W., Wearmouth, V. J., Genner, M. J., Southward, A. J., and Hawkins, S. J. (2004). Low-temperature-driven early spawning migration of a temperate marine fish. J. Anim. Ecol. 73, 333–341. doi: 10.1111/j.0021-8790.2004.00810.x
Smith, J. N., De’ath, G., Richter, C., Cornils, A., Hall-Spencer, J. M., and Fabricius, K. E. (2016). Ocean acidification reduces demersal zooplankton that reside in tropical coral reefs. Nat. Clim. Change 6, 1124–1129. doi: 10.1038/nclimate3122
Speirs, D. C., Gurney, W. S., Heath, M. R., and Wood, S. N. (2005). Modelling the basin-scale demography of Calanus finmarchicus in the north-east Atlantic. Fish. Oceanogr. 14, 333–358. doi: 10.1111/j.1365-2419.2005.00339.x
Sprong, P., Fofonova, V., Wiltshire, K. H., Neuhaus, S., Ludwichowski, K.-U., Käse, L., et al. (2020). Spatial dynamics of eukaryotic microbial communities in the German Bight. J. Sea Res. 163:101914. doi: 10.1016/j.seares.2020.101914
Stanev, E. V., Schulz-Stellenfleth, J., Staneva, J., Grayek, S., Grashorn, S., Behrens, A., et al. (2016). Ocean forecasting for the German Bight: from regional to coastal scales. Ocean Sci. 12, 1105–1136. doi: 10.5194/os-12-1105-2016
Steele, J. H., and Ruzicka, J. J. (2011). Constructing end-to-end models using ECOPATH data. J. Mar. Syst. 87, 227–238. doi: 10.1016/j.jmarsys.2011.04.005
Steinberg, D. K., Carlson, C. A., Bates, N. R., Johnson, R. J., Michaels, A. F., and Knap, A. H. (2001). Overview of the US JGOFS Bermuda Atlantic Time-series Study (BATS): a decade-scale look at ocean biology and biogeochemistry. Deep Sea Res. II Top. Stud. Oceanogr. 48, 1405–1447. doi: 10.1016/s0967-0645(00)00148-x
Sterner, R. W., and Elser, J. J. (2002). Ecological Stoichiometry: The Biology of Elements from Molecules to the Biosphere. Princeton, NJ: Princeton university press.
Sunda, W. (2012). Feedback interactions between trace metal nutrients and phytoplankton in the ocean. Front. Microbiol. 3:204. doi: 10.3389/fmicb.2012.00204
Sundby, S., and Drinkwater, K. (2007). On the mechanisms behind salinity anomaly signals of the northern North Atlantic. Prog. Oceanogr. 73, 190–202. doi: 10.1016/j.pocean.2007.02.002
Taherzadeh, N., Bengfort, M., and Wirtz, K. W. (2019). A trait-based framework for explaining non-additive effects of multiple stressors on plankton communities. Front. Mar. Sci. 6:351. doi: 10.3389/fmars.2019.00351
Tedesco, K., and Thunell, R. (2003). High resolution tropical climate record for the last 6,000 years. Geophys. Res. Lett. 30:GL017959.
Tett, P., and Walne, A. (1995). Observations and simulations of hydrography, nutrients and plankton in the southern North Sea. Ophelia 42, 371–416. doi: 10.1080/00785326.1995.10431514
Tittensor, D. P., Eddy, T. D., Lotze, H. K., Galbraith, E. D., Cheung, W., Barange, M., et al. (2018). A protocol for the intercomparison of marine fishery and ecosystem models: fish-MIP v1. 0. Geosci. Model Dev. 11, 1421–1442.
Toggweiler, J. R., and Russell, J. (2008). Ocean circulation in a warming climate. Nature 451, 286–288. doi: 10.1038/nature06590
Turner, R., Schaafsma, M., Mee, L., Elliott, M., Burdon, D., Atkins, J. P., et al. (2015). “Conceptual framework,” in Coastal Zones Ecosystem Services, eds R. Kerry Turner and M. Schaafsma (Berlin: Springer), 11–40.
Ulanowicz, R. E. (2004). Quantitative methods for ecological network analysis. Comput. Biol. Chem. 28, 321–339. doi: 10.1016/j.compbiolchem.2004.09.001
van Beusekom, J. (2005). A historic perspective on Wadden Sea eutrophication. Helgoland Mar. Res. 59, 45–54. doi: 10.1007/s10152-004-0206-2
Van de Waal, D. B., Verschoor, A. M., Verspagen, J. M., van Donk, E., and Huisman, J. (2010). Climate-driven changes in the ecological stoichiometry of aquatic ecosystems. Front. Ecol. Environ. 8, 145–152. doi: 10.1890/080178
van der Molen, J., García-García, L. M., Whomersley, P., Callaway, A., Posen, P. E., and Hyder, K. (2018). Connectivity of larval stages of sedentary marine communities between hard substrates and offshore structures in the North Sea. Sci. Rep. 8, 1–14.
Vaquer-Sunyer, R., Duarte, C. M., Santiago, R., Wassmann, P., and Reigstad, M. (2010). Experimental evaluation of planktonic respiration response to warming in the European Arctic Sector. Polar Biol. 33, 1661–1671. doi: 10.1007/s00300-010-0788-x
Vasas, V., Lancelot, C., Rousseau, V., and Jordán, F. (2007). Eutrophication and overfishing in temperate nearshore pelagic food webs: a network perspective. Mar. Ecol. Prog. Ser. 336, 1–14. doi: 10.3354/meps336001
Vilas, D., Coll, M., Pedersen, T., Corrales, X., Filbee-Dexter, K., Pedersen, M. F., et al. (2020). Kelp-carbon uptake by Arctic deep-sea food webs plays a noticeable role in maintaining ecosystem structural and functional traits. J. Mar. Syst. 203:103268. doi: 10.1016/j.jmarsys.2019.103268
Wakelin, S. L., Artioli, Y., Butenschön, M., Allen, J. I., and Holt, J. T. (2015). Modelling the combined impacts of climate change and direct anthropogenic drivers on the ecosystem of the northwest European continental shelf. J. Mar. Syst. 152, 51–63. doi: 10.1016/j.jmarsys.2015.07.006
Waldbusser, G. G., and Salisbury, J. E. (2014). Ocean acidification in the coastal zone from an organism’s perspective: multiple system parameters, frequency domains, and habitats. Annu. Rev. Mar. Sci. 6, 221–247. doi: 10.1146/annurev-marine-121211-172238
Wallace, R. B., Baumann, H., Grear, J. S., Aller, R. C., and Gobler, C. J. (2014). Coastal ocean acidification: the other eutrophication problem. Estuarine Coast. Shelf Sci. 148, 1–13. doi: 10.1016/j.ecss.2014.05.027
Weijerman, M., Fulton, E. A., Kaplan, I. C., Gorton, R., Leemans, R., Mooij, W. M., et al. (2015). An integrated coral reef ecosystem model to support resource management under a changing climate. PLoS One 10:e0144165. doi: 10.1371/journal.pone.0144165
Wendling, C. C., and Wegner, K. M. (2013). Relative contribution of reproductive investment, thermal stress and Vibrio infection to summer mortality phenomena in Pacific oysters. Aquaculture 412, 88–96. doi: 10.1016/j.aquaculture.2013.07.009
Werner, F. E., Ito, S.-I., Megrey, B. A., and Kishi, M. J. (2007). Synthesis of the NEMURO model studies and future directions of marine ecosystem modeling. Ecol. Model. 202, 211–223. doi: 10.1016/j.ecolmodel.2006.08.019
Weydmann, A., Walczowski, W., Carstensen, J., and Kwaśniewski, S. (2018). Warming of Subarctic waters accelerates development of a key marine zooplankton Calanus finmarchicus. Glob. Change Biol. 24, 172–183. doi: 10.1111/gcb.13864
Wijsman, J., Troost, K., Fang, J., and Roncarati, A. (2019). “Global production of marine bivalves. Trends and challenges,” in Goods and Services of Marine Bivalves, ed. R. E. Ulanowicz (Cham: Springer), 7–26. doi: 10.1007/978-3-319-96776-9_2
Wiltshire, K. H., Boersma, M., Carstens, K., Kraberg, A. C., Peters, S., and Scharfe, M. (2015). Control of phytoplankton in a shelf sea: determination of the main drivers based on the helgoland roads time series. J. Sea Res. 105, 42–52. doi: 10.1016/j.seares.2015.06.022
Wiltshire, K. H., Kraberg, A., Bartsch, I., Boersma, M., Franke, H.-D., Freund, J., et al. (2010). Helgoland roads, North Sea: 45 years of change. Estua. Coasts 33, 295–310. doi: 10.1007/s12237-009-9228-y
Wiltshire, K. H., Malzahn, A. M., Wirtz, K., Greve, W., Janisch, S., Mangelsdorf, P., et al. (2008). Resilience of North Sea phytoplankton spring bloom dynamics: an analysis of long-term data at Helgoland Roads. Limnol. Oceanogr. 53, 1294–1302. doi: 10.4319/lo.2008.53.4.1294
Wiltshire, K. H., and Manly, B. F. (2004). The warming trend at Helgoland Roads, North Sea: phytoplankton response. Helgoland Mar. Res. 58, 269–273. doi: 10.1007/s10152-004-0196-0
Winder, M., and Sommer, U. (2012). Phytoplankton response to a changing climate. Hydrobiologia 698, 5–16. doi: 10.1007/978-94-007-5790-5_2
Wroblewski, J. S., Sarmiento, J. L., and Flierl, G. R. (1988). An Ocean Basin Scale Model of plankton dynamics in the North Atlantic: 1. Solutions For the climatological oceanographic conditions in May. Glob. Biogeochem. Cycles 2, 199–218. doi: 10.1029/gb002i003p00199
Yin, J. (2012). Century to multi-century sea level rise projections from CMIP5 models. Geophys. Res. Lett. 39:17709.
Zhang, Y., and Baptista, A. M. (2008). SELFE: a semi-implicit Eulerian–Lagrangian finite-element model for cross-scale ocean circulation. Ocean Model. 21, 71–96. doi: 10.1016/j.ocemod.2007.11.005
Keywords: global warming, ecosystem effects, species interactions, marine, food webs, ecosystem models
Citation: Horn S, Meunier CL, Fofonova V, Wiltshire KH, Sarker S, Pogoda B and Asmus H (2021) Toward Improved Model Capacities for Assessment of Climate Impacts on Coastal Bentho-Pelagic Food Webs and Ecosystem Services. Front. Mar. Sci. 8:567266. doi: 10.3389/fmars.2021.567266
Received: 29 May 2020; Accepted: 07 June 2021;
Published: 07 July 2021.
Edited by:
Jamie C. Tam, Bedford Institute of Oceanography (BIO), CanadaReviewed by:
Danielle P. Dempsey, Dalhousie University, CanadaCopyright © 2021 Horn, Meunier, Fofonova, Wiltshire, Sarker, Pogoda and Asmus. This is an open-access article distributed under the terms of the Creative Commons Attribution License (CC BY). The use, distribution or reproduction in other forums is permitted, provided the original author(s) and the copyright owner(s) are credited and that the original publication in this journal is cited, in accordance with accepted academic practice. No use, distribution or reproduction is permitted which does not comply with these terms.
*Correspondence: Sabine Horn, c2FiaW5lLmhvcm5AYXdpLmRl
Disclaimer: All claims expressed in this article are solely those of the authors and do not necessarily represent those of their affiliated organizations, or those of the publisher, the editors and the reviewers. Any product that may be evaluated in this article or claim that may be made by its manufacturer is not guaranteed or endorsed by the publisher.
Research integrity at Frontiers
Learn more about the work of our research integrity team to safeguard the quality of each article we publish.