- 1Centre for Research into Ecological & Environmental Modelling, University of St Andrews, St Andrews, United Kingdom
- 2School of Mathematics and Statistics, University of St Andrews, St Andrews, United Kingdom
- 3Independent Scientist, Capel, WA, Australia
- 4Institute of Marine Sciences, School of Biological Sciences, University of Portsmouth, Portsmouth, United Kingdom
- 5Biodiversity and Conservation Science, Department of Biodiversity, Conservation and Attractions, Kensington, WA, Australia
- 6Cultural and Environmental Services Unit, Land and Sea Management Unit, Kimberley Land Council, Broome, WA, Australia
- 7Balanggarra Aboriginal Corporation, Wyndham, WA, Australia
- 8Bardi and Jawi Niimidiman Aboriginal Corporation, Broome, WA, Australia
- 9Dambimangari Aboriginal Corporation, Derby, WA, Australia
- 10Nyamba Buru Yawuru, Broome, WA, Australia
- 11Nyul Nyul PBC Aboriginal Corporation, Broome, Western Australia
- 12Wunambal Gaambera Aboriginal Corporation, Kalumburu, WA, Australia
Implementing conservation measures for data-limited species is a fundamental challenge for wildlife managers and policy-makers, and proves difficult for cryptic marine animals occurring in naturally low numbers across remote seascapes. There is currently scant information on the abundance and habitat preferences of Australian snubfin dolphins (Orcaella heinsohni) throughout much of their geographical range, and especially within the Kimberley region of northern Western Australia. Such knowledge gaps curtail rigorous threat assessments on both local and regional scales. To address this and assist future conservation listings, we built the first comprehensive catalog of snubfin dolphin sightings for the Kimberley. We used these data to estimate the species’ extent of occurrence (EOO) and area of occupancy (AOO) along the region’s 7,000 km coastline, following a simple Bootstrap bivariate kernel approach to combine datasets of varying quality and quantify uncertainty. Our catalog consists of 1,597 visual detections of snubfin dolphins made over a period of 17 years (2004–2020) and collated from multiple sources, including online biodiversity repositories, peer-reviewed scientific articles, citizen science programs, as well as dedicated marine wildlife surveys with local Indigenous communities and Ranger groups. Snubfin dolphins were consistently encountered in shallow waters (<21 m depth) close to (<15 km) freshwater inputs, with high detection rates in known hotspots (e.g., Roebuck Bay, Cygnet Bay) as well as in coastal habitats suspected to be suitable (e.g., Prince Regent River and surrounds, King Sound, Doubtful Bay, Napier Broome Bay and the upper Cambridge Gulf). Bootstrap estimates of EOO and AOO were 38,300 (95% CI: 25,451–42,437) km2 and 700 (656–736) km2 respectively, suggesting that snubfin dolphins in the Kimberley are likely Vulnerable under IUCN criteria B2 at a regional scale, in keeping with their global classification. Our study offers insights into the distribution of a vulnerable coastal cetacean species and demonstrates the value of integrating multiple data sources for informing conservation assessments in the face of uncertainty.
Introduction
Australian snubfin dolphins (Orcaella heinsohni, hereafter ‘snubfins’) are cryptic, small cetaceans endemic to the tropical waters of northern Australia and southern Papua New Guinea. Only differentiated from the closely related Irrawaddy dolphin (Orcaella brevirostris) less than two decades ago (Beasley et al., 2005), the species remains poorly known, and is listed as globally Vulnerable under criteria A and C [level VU A2cd + 3cd + 4cd; C2a(i)] of the International Union for the Conservation of Nature (IUCN)’s Red List of Threatened Species (Parra et al., 2017). This classification largely reflects suspected declines in population size stemming from incidental mortality in fishing nets (Parra and Jedensjö, 2014), as well as anticipated habitat loss in the face of growing industrial developments throughout much of nearshore tropical Australia (Allen et al., 2012; Cagnazzi et al., 2013b). A limited body of research indicates that snubfins occur in isolated populations (Brown et al., 2014b) occupying shallow, inshore waters in proximity to freshwater inputs such as estuaries and tidal rivers (Parra et al., 2006b; Palmer et al., 2014b). However, a comprehensive understanding of the spatial ecology, regional distribution, and environmental preferences of snubfins is still lacking.
In particular, while studies of snubfins have been conducted at selected sites in Queensland (Parra, 2006; Parra et al., 2006a,b, 2011; Cagnazzi et al., 2013a,b), the Northern Territory (Palmer et al., 2014a,b), and Western Australia (Thiele, 2010; Brown et al., 2014a, 2016, 2017), extensive portions of the species’ known Australian range have been subject to little or no survey effort (Kaschner et al., 2012). In Western Australia, prominent gaps in sampling coverage remain across the Kimberley, owing to the logistical difficulties and prohibitive costs associated with accessing many parts of the region’s remote, 7,000 km-long coastline (Kordi et al., 2016). Snubfins, named munumba in Wunambal and Gaambera languages, have been known to Traditional Owners for several millennia (Karadada et al., 2011), yet the earliest historical account of the species by Europeans within the Kimberley only dates back to 1821 at Prince Regent River (15.452°S; 125.065°E, Figure 1) (King, 1825). Notwithstanding, no targeted sampling was undertaken in the area until 2004, when a limited number of aerial and shipborne visual transect programs were kick-started (Jenner et al., 2008, 2014; Thiele, 2010). While these surveys confirmed the presence of snubfins in low densities within estuaries and coastal embayments, their initial implementation was largely sporadic. Furthermore, observations made in subsequent years by researchers, Indigenous Rangers, and government agencies have never been collated. Such patchy knowledge impedes assessments of the species’ conservation status under IUCN criterion B (“geographic range”) (Allen et al., 2012; Hunt et al., 2017; Smith et al., 2018; Waples and Raudino, 2018), and poses obstacles to its long-term management.
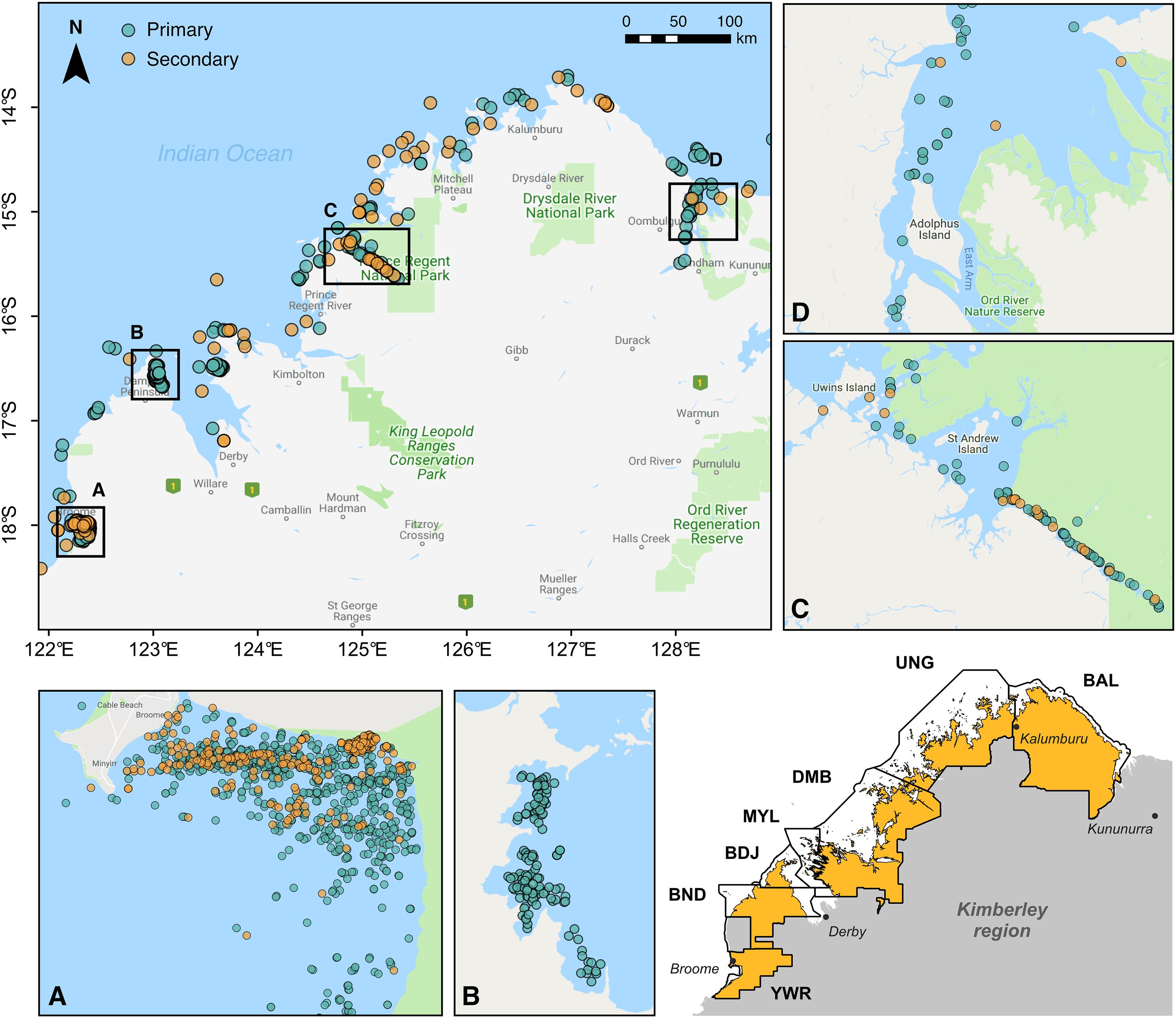
Figure 1. Visual detections of snubfin dolphins (Orcaella heinsohni) throughout the Kimberley region of Western Australia between 2004 and 2020. Labeled insets display the distribution of both primary and secondary sightings (see Table 1) at key sites, including (A) Roebuck Bay, (B) Cygnet Bay, (C) Prince Regent River, and (D) the Cambridge Gulf. The inset map in the lower right corner shows the boundaries of Indigenous Sea Countries covered by field surveys. These encompass the following Native Title determinations (from north to south): Balanggarra (BAL), Uunguu (UNG), Dambimangari (DMB), Mayala (MYL), Bardi Jawi (BDJ), Bindunbur (BND), and Yawuru (YWR) Sea Countries. Note that Rangers from the Bindunbur community are referred to as Nyul Nyul. Map baselayer: Google © 2019.
Despite early criticism (e.g., Possingham et al., 2002), there has been increasing appreciation of the utility of IUCN Red Listings (henceforth ‘RLs’) as quantitative instruments for supporting decision-making and conservation priority-setting in the face of such data deficiency (Rodrigues et al., 2006; Hoffmann et al., 2008; Maes et al., 2015; Le Breton et al., 2019). As anthropogenic impacts are not distributed evenly everywhere and management actions are commonly administered at local levels rather than globally (Brito et al., 2010; Pagel et al., 2014; Jenkins and Van Houtan, 2016), several countries around the world have begun to develop their own regional RL schemes aimed at quantifying threats within discrete parts of species’ ranges (Gärdenfors, 2001; Gärdenfors et al., 2008; Nourani et al., 2017). To be rigorous, such efforts ought to be supported by systematic monitoring programs designed around standardized scientific surveys, yet these remain difficult to execute and sustain over the long term in most remote areas (Grech et al., 2014). A promising solution to fill information gaps is to combine different data types from alternative sources, which are often opportunistic in nature (Cheney et al., 2013; Pagel et al., 2014). For instance, non-expert “citizen scientists” now make substantial and active contributions to species mapping by logging ad hoc observations of wildlife using smartphones or other mobile computing technologies (Devictor et al., 2010; Tiago et al., 2017). These can be particularly valuable in biogeographical studies of coastal cetaceans, and can complement or even replace dedicated surveys for tracking distributional changes over broad spatial extents (Embling et al., 2015; Lodi and Tardin, 2018; Alessi et al., 2019). Similarly, community-based approaches that harness the skills, interests, and capacity of Indigenous Ranger organizations are supporting conservation efforts locally, including the monitoring of trends in species and habitats (Grech et al., 2014; Jackson et al., 2015). The latter is particularly relevant where Indigenous people are recognized as land managers in their own right through Native Title, and where they have developed and implemented Healthy Country Management Plans that articulate their vision, objectives and targets for Indigenous Protected Areas (Rist et al., 2019).
In this study, we present the first regional conservation assessment of snubfin dolphins throughout northern Western Australia. Snubfins are currently earmarked as a key target species for fundamental research in Australia (Waples and Raudino, 2018). In 2013, with support from the Australian Federal Government, efforts were made to develop a National Research Strategy that could help deliver a better understanding of the ecology of tropical inshore dolphins in support of improved conservation decision-making (Department of the Environment, 2013, 2015). Part of this process entailed a technical workshop, during which marine mammal experts were asked to recommend suitable methods for determining the conservation status of snubfins nationally (Brooks et al., 2014). Estimating the species’ extent of occurrence (EOO) and area of occupancy (AOO) under IUCN criterion B was identified as a high priority, on the proviso that such efforts be underpinned by an exhaustive compilation of available datasets, with a strong emphasis on collaboration and Indigenous engagement (Department of the Environment, 2013, 2015). The EOO and AOO are standardized indices of wildlife distribution that are strong and consistent predictors of extinction risk in the marine environment, as larger range sizes provide a buffer against local population declines and mean that species are less likely to experience catastrophic or range-wide losses (Payne and Finnegan, 2007). Importantly, the EOO/AOO are suitable for assessing conservation status even when there is limited information on local threatening processes and the distribution of a taxon is only known from opportunistic observations, unlike other IUCN metrics (Dauby et al., 2017). In particular, criterion B tends to be more suitable than abundance metrics (i.e., IUCN criterion A) for coastal dolphin populations whose sizes are challenging to estimate but predicted to be declining (Brooks et al., 2017), and/or where such estimates are not available over most of the species’ range (Parra and Cagnazzi, 2016); both of which apply directly to snubfins. Nonetheless, crowdsourcing disparate datasets brings forth a number of challenges related to variability in both data quality (e.g., taxonomic misidentifications, measurement/recording errors, differences in field protocols) and quantity (e.g., spatio-temporal bias in sampling effort, different data storing/sharing/access policies) (Akçakaya et al., 2000; Guttmacher, 2016; Rueda-Cediel et al., 2018; Fletcher et al., 2019). While this uncertainty is inherently acknowledged in IUCN nomenclature (e.g., criterion A1 specifies population reductions as being “observed, estimated, projected, inferred, or suspected”) (IUCN Standards and Petitions Committee, 2019), it is seldom addressed in practice, with most assessments yielding single threat classifications that do not explicitly reflect the amount and reliability of their underlying inputs (Akçakaya et al., 2000). Some efforts to tackle this issue have been made (e.g., using fuzzy logic or Bayesian networks), but existing solutions are not readily accessible as they rely on proprietary software available only through expensive paywalls (e.g., the RAMAS Red List Pro package) (Akçakaya et al., 2000; Newton, 2010).
Here, we rely on a simple kernel-based weighted resampling approach that treats the veracity of sightings probabilistically, allowing a straightforward assessment of uncertainty. Our analyses build on what is, to our knowledge, the most comprehensive repository of geo-referenced snubfin sightings for the Kimberley. These were obtained from dedicated surveys carried out in partnership with the Indigenous Rangers associated with the Yawuru, Dambimangari, Wunambal Gaambera, Bardi Jawi, and Balanggarra Native Title groups, as well as from a range of additional sources such as citizen science initiatives, government archives, and the peer-reviewed literature, among others. Our study provides an example of how to successfully incorporate disparate datasets to conduct RLs of elusive cetaceans in isolated seascapes.
Materials and Methods
Study Area
The study area extends for approximately 7,000 km along the northern coast of Western Australia between Roebuck Bay (122.21°E, 18.17°S) and the Northern Territory border (129°E) (Figure 1). The inshore environment of the Kimberley is a complex matrix of convoluted narrow embayments, drowned valleys, rocky outcrops, and coral-fringed headlands or islands subject to monsoonal climate cycles (Wilson, 2014). Coastal waters are characterized by high levels of turbidity and some of the most extreme tidal ranges in the world, in excess of 10 m in some areas (Wood and Mills, 2008). An array of habitats can be found throughout the region, including mangroves, seagrass meadows, mudflats, and extensive reef systems. These provide foraging, resting, and breeding grounds for a large diversity of marine vertebrates, including cetaceans (Thums et al., 2018). Supplementary Figure S1 shows the locations of all marine areas, stream outflows, river systems, and reefs/islands referred to throughout the main text. In Western Australia, coastal waters out to three nautical miles fall under the jurisdiction of the State Government, with Indigenous people having management responsibility for their Sea Country where Native Title has been determined (Rist et al., 2019). A network of marine parks has also been implemented across much of the State’s coastal fringe (Supplementary Figure S2), with co-management agreements in place between the State Government and Indigenous people in some cases (e.g., the Uunguu Indigenous Protected Area also encompasses coastal waters). Both marine park and Healthy Country management plans identify marine values within their respective areas and include objectives and targets for their long-term management (Balanggarra Aboriginal Corporation, 2011; Bardi and Jawi Niimidiman Aboriginal Corporation, 2013). Snubfins are recognized as an ecologically important and culturally significant marine species by State and Healthy Country managers across their range.
Dolphin Data
Data Sources
Sightings of snubfins were collated from a variety of published and unpublished sources and split into two categories reflecting observers’ perceived level of expertise (Table 1). “Primary” sightings consisted of reports made by highly trained personnel (including Kimberley Indigenous Rangers and/or professional marine mammal scientists), and included data from: (a) two 14-day surveys (led by DT) undertaken between Darwin and Broome to describe the presence of snubfins in nearshore tidal river environments (datasets ID OH-001 and OH-002 in Table 1), (b) four 20-day offshore biodiversity surveys conducted under contract with industry (OH-003) in the waters of the Bonaparte Archipelago and Browse Basin (Jenner et al., 2014); (c) 46 daily surveys of Roebuck Bay run along pre-determined transect lines (OH-004) (Thiele, 2010); (d) 36 collaborative surveys undertaken by coastal Indigenous Ranger groups (Nyamba Buru Yawuru, Dambimangari, Balanggarra, and Uunguu) on their respective Sea Countries [OH-005005; see https://bit.ly/snubfin230dolphins-Yawuru for an example (last accessed November 2020)], in addition to a 3-day intensive census of snubfins in the Yawuru Nagulagan/Roebuck Bay Marine Park (Raudino et al., 2019) (Figure 2 and Supplementary Figure S2); (e) extensive dedicated genetic sampling and photo-identification surveys (OH-006) targeting important shallow-water sites along the Kimberley coast, including Beagle Bay, Roebuck Bay, Cygnet Bay, Cone Bay, and the Inner Cambridge Gulf (Brown et al., 2014b, 2016); (f) 14 surveys completed in the northern part of Roebuck Bay in July 2014 (OH-007) concurrently to the deployment of an acoustic logger used for soundscape monitoring (Brown et al., 2017); (h) an array of opportunistic detections reported by biologists and other experienced marine professionals from partner institutions (OH-008 to OH-012) during miscellaneous research and non-research activities, some of which have been published in the peer-reviewed literature (e.g., Allen et al., 2012). Complete or partial GPS tracks were available for most primary datasets and were used to characterize sampling effort (Supplementary Figure S3).
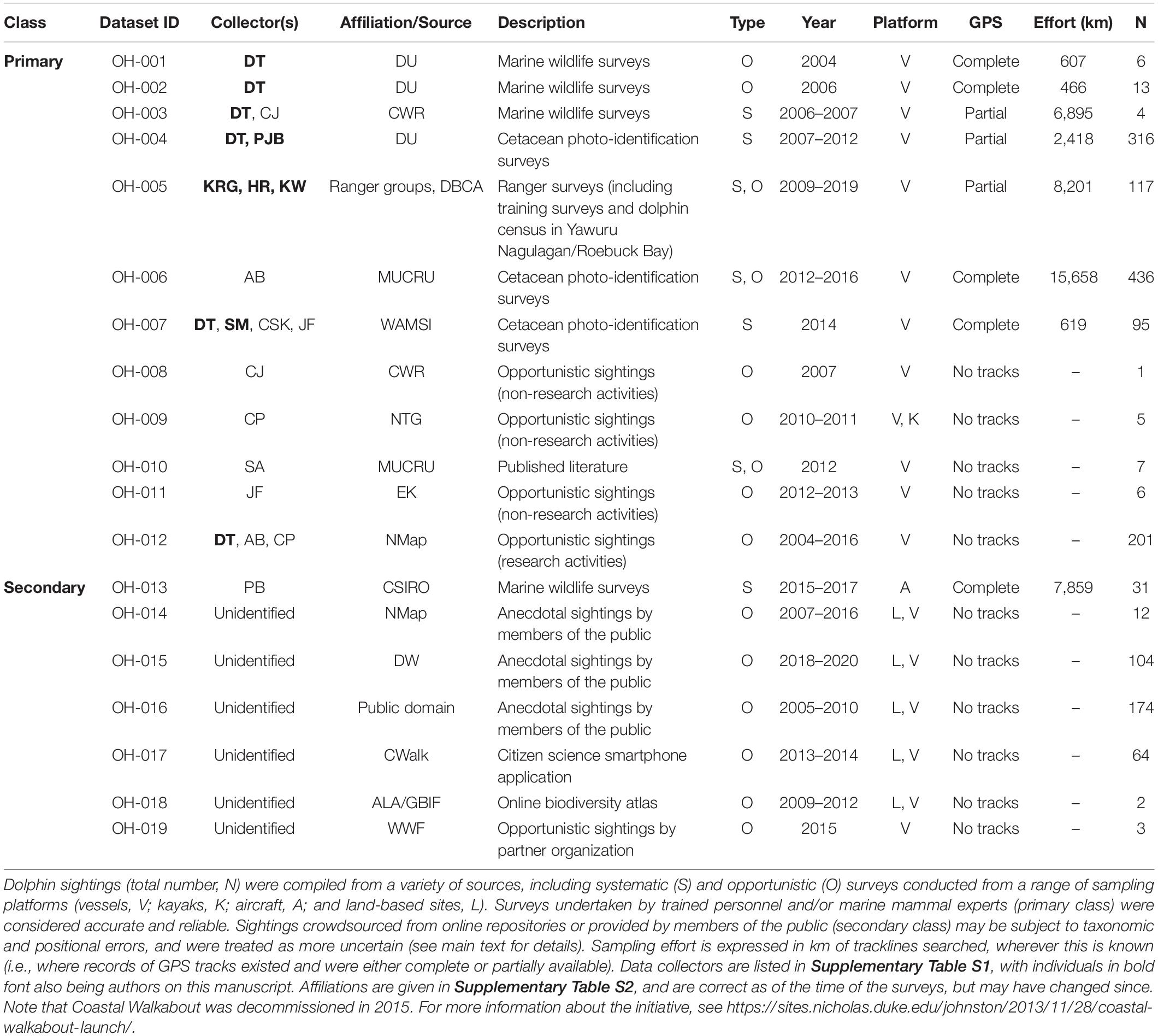
Table 1. Summary of datasets used for assessing the regional conservation status of snubfin dolphins (Orcaella heinsohni) in the Kimberley region.
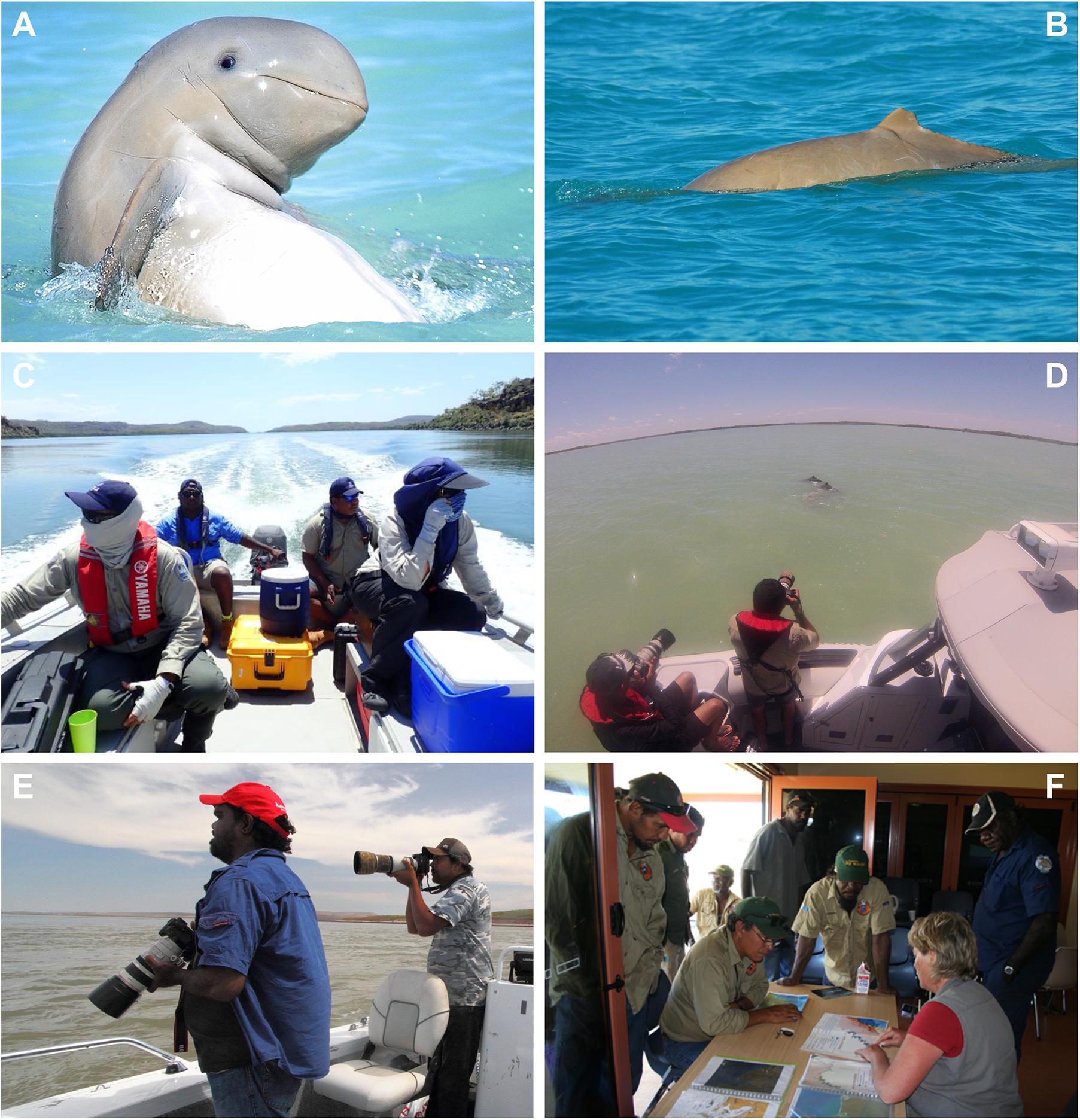
Figure 2. Collaborative snubfin dolphin research on Kimberley Sea Countries. (A) Australian snubfin dolphins (Orcaella heinsohni) are small-sized coastal cetaceans endemic to Northern Australia and Papua New Guinea. Adults can grow up to 2.7 m in length, and have a characteristically rounded head with no beak or rostrum. Most dolphins occur in small pods of 2–5 animals, though larger groups have occasionally been observed. Photo: Deborah Thiele. (B) Good quality photographs enable the reliable identification of snubfin dolphins during wildlife surveys and can facilitate the estimation of population abundance using mark-recapture methods. Photo: Phil Bouchet. (C–E) Ranger-led surveys such as those on Dambimangari, Yawuru, and Balanggarra Sea Countries are critical for supporting ongoing population monitoring throughout the remote Kimberley region. Photos: Ellen D’Cruz and Alex Brown. (F) Training workshops combine theoretical learning and practical activities to build capacity among Traditional Owners in the collection of scientific data on coastal marine wildlife. Photo: Tanya Vernes/WWF-Australia.
“Secondary” data included incidental sightings collected as part of large-scale aerial surveys targeting dugong (Dugong dugon) (OH-013), as well as records crowdsourced from open-access, online biodiversity portals (e.g., Atlas of Living Australia), citizen science mobile applications (DolphinWatch, and Coastal Walkabout), government archives (NatureMap), and anecdotal community reports from members of the public (e.g., recreational ocean users, wildlife tour operators, etc.; OH-014–OH-019, Table 1). With the exception of aerial surveys (Supplementary Figure S3), sampling effort for secondary datasets was unknown (Table 1).
Note that we only considered visual detections, although interest in developing long-term acoustic monitoring programs for the species is rising (Brown et al., 2017; Marley et al., 2017; de Freitas et al., 2018).
Survey Methods
A full description of field procedures for individual surveys is given elsewhere (e.g., Brown et al., 2016; Bayliss and Hutton, 2017) and these are only briefly summarized here. On-water surveys were conducted aboard a range of visual platforms, including catamarans, fishing vessels, small-sized rigid inflatables (i.e., zodiacs), research powerboats, and charter yachts (Table 1). All surveys adhered to well-established methodology (Smith and Reeves, 2000; Dawson et al., 2008) with 2–5 experienced observers maintaining constant watch 180° ahead of the platform from the bow, side, and upper bridges (on larger vessels). Visual searches took place during daylight hours, when the boats were motoring or sailing and both weather and visibility conditions were deemed suitable, i.e., Beaufort sea state ≤ 3 (Barlow, 2015). During systematic surveys, the locations of all dolphin sightings were marked using GPS. Animals were approached at close range whenever possible in order to confirm estimates of group sizes and photograph individual dolphins to support the development of a regional photo-identification catalog1.
Aerial surveys were flown aboard a fixed-wing GA8 Airvan in September–October 2015 and May 2017, traveling at 100 knots ground speed and 500 ft, an altitude at which small-bodied marine mammal species can be confidently detected (Bayliss, 1986), though not always differentiated with absolute certainty. Confidence in taxonomic identification typically varies with survey conditions (e.g., Beaufort sea state, glare, water clarity), as well as by observer (e.g., individual experience, eyesight, fatigue levels, and viewing angle from the airplane), and by species (e.g., group size, morphological traits, diving behavior) (Dunshea et al., 2020). Bayliss and Freeland (1989) claimed that after several flights, most observers had little difficulty in telling snubfins apart from other species under favorable viewing conditions. However, other studies reported up to 5–11% of uncertain sightings/disagreements between observers (Hodgson et al., 2013; Dunshea et al., 2020) (see section “Taxonomic and geographic errors” for the treatment of taxonomic uncertainty). Surveys followed the procedures outlined in Sobtzick et al. (2013) and Bayliss and Hutton (2017). A team of tandem observers recorded their sightings with at least one experienced observer on each side of the aircraft. Surveys encompassed coastal waters out to the 20 m bathymetric contour between Broome and the Northern Territory border, and were completed in collaboration with Indigenous Rangers following a 5-day intensive training course at Gambimerri Ranger station on Wunambal Gaambera Country (Bayliss and Wilcox, 2015).
Data Processing and Treatment
All analyses were undertaken in R v4.0.0. The underlying code is freely available at https://github.com/pjbouchet/orcaella_eoo.
Data integration is increasingly commonplace in ecological research (Isaac et al., 2020), yet efforts to synthesize vast amounts of heterogeneous information often lead to the assimilation of data burdened with different evidentiary standards (Domisch et al., 2016; Fletcher et al., 2019). Typically, structured datasets obtained during surveys that follow explicit sampling designs are deemed of high quality but remain in short supply, whereas unstructured data collected without strict protocols are growing plentiful but may be ambiguous and subject to important biases (Isaac and Pocock, 2015). Conservation decisions can be highly sensitive to these biases and the potential errors caused by falsely choosing to reject valid observations or accepting anomalous ones (McKelvey et al., 2008), yet many equivocal (but unverifiable) reports are made by experienced amateur naturalists or well-intentioned members of the public whose contributions are difficult to justify excluding (Lee et al., 2014; Brook et al., 2019). The careful treatment of occurrence records is therefore crucial in geographic range assessments of little-studied, rare, and elusive taxa from mixed-certainty datasets (Roberts et al., 2010). We adopted a two-pronged approach to data processing, focusing firstly on quality control and the correction of a range of common errors (Soberón and Peterson, 2004; Robertson et al., 2016; Zizka et al., 2019; Jin and Yang, 2020), and secondly on the calculation of plausibility (inclusion) scores, which can be interpreted as the probability that each sighting is a true record of the species. The latter step was essential given that snubfins are cryptic and can be easily mistaken for other visually similar and sympatric species (e.g., dugong), especially when detected momentarily from a distance (Bayliss, 1986; Dunshea et al., 2020), and permitted the probabilistic inclusion of all data points, including potentially controversial sightings (Jarić and Roberts, 2014).
Taxonomic and Geographic Errors
Primary sightings, most of which were supported by high-quality digital photographs of the animals (Figures 2A,B), were considered unambiguous and treated as geographically and taxonomically accurate. In contrast, secondary records were likely prone to greater inconsistencies owing to differences in the competence, commitment, and capacity of observers (e.g., eyesight, access to vessels), in addition to the wide range of surveying conditions associated with each data source (Dickinson et al., 2010). Two of the seven secondary datasets (OH-013 and OH-014, Table 1) included a measure of certainty in species identifications, which we relied on to filter out any “guesses,” retaining only those detections labeled as “certain,” “relatively certain,” and “probable.” Sightings from the Atlas of Living Australia (ALA) and the Global Biodiversity Information Facility (GBIF) (OH-018) are subjected to extensive quality tests, and only those that passed taxonomic checks were considered. In the absence of relevant taxonomic information, all other records were assumed to have been correctly assigned to Orcaella heinsohni. Any on-land observations were also randomly reassigned to the nearest ocean area within a 1 km-wide buffer surrounding each point. This allowed us to discard a small number of clearly erroneous sightings (i.e., located several tens to hundreds of km inland), whilst providing a reasonable approximation of the possible distribution of dolphins when the only sighting coordinates available were those of land-based vantage points (e.g., Broome Bird Observatory, Supplementary Figure S4). Both true and likely duplicate records were identified within and across datasets and excluded from the analysis. True duplicates stemmed mostly from the fact that online data infrastructures such as ALA or GBIF often harvest information from common source providers (including each other), and were defined as sightings with identical Julian dates, group sizes, and geographic coordinates (i.e., latitude and longitude) — these were manually discarded. Likely duplicates were taken as sightings made within 100 m of each other on the same date (e.g., repeat observations of the same dolphin group by different citizen scientists). These were spatially thinned using a randomized algorithm, as implemented in the R package spThin (Aiello-Lammens et al., 2015).
Inclusion Probabilities
Recently, several studies have proposed ways of incorporating sighting veracity into ecological inference, either by applying scoring systems developed through expert elicitation (Jarić and Roberts, 2014; Lee et al., 2014, 2015), or via Bayesian frameworks (Solow et al., 2012; Solow and Beet, 2014). The idea of allocating inclusion probabilities to individual sightings is appealing as it relaxes the conventional ‘accept-or-reject’ paradigm of data processing and allows every data point to be fully considered and used, thereby maximizing its analytical value (Brook et al., 2019). However, Bayesian models can be mathematically and computationally intensive, may require prior information that is often missing for data-deficient species, and can be challenging to benchmark against other methods (Saltré et al., 2015). Likewise, expert judgments are necessarily subjective and unlikely to be thoroughly reproducible (i.e., they are prone to vary depending on personal beliefs and experiences, as well as the way in which information is elicited), ultimately limiting their applicability (Martin et al., 2012).
A simpler alternative is to assess ambiguous sightings in terms of their similarity to locations where the species has been documented to occur, for example by down-weighting (or filtering) records associated with environmental conditions that deviate from those encountered in verified datasets collected by professionals (Allouche et al., 2008; Lin et al., 2017; Fletcher et al., 2019; Robinson et al., 2020). We did this for snubfins using an adaptation of the 2D smoothing protocol described by Tarjan and Tinker (2016). Specifically, we fitted bivariate kernel density functions to primary sightings within the landscape space defined by both (i) seabed depth and (ii) proximity to the nearest freshwater outflow using the bkde2D function in the KernSmooth package (Wand, 2015). These variables were chosen as the most commonly reported putative drivers of snubfin occurrence throughout their range (Parra et al., 2002, 2006b, 2017; Parra, 2006; Cagnazzi et al., 2013b), and the resulting density surface can thus be thought of as mapping the relative intensity of species presence along two key dimensions of its niche. Depth values were extracted from a high-resolution (30 m) bathymetric grid of the Kimberley curated by Geoscience Australia2. Freshwater outflows were identified as all perennial streams (major and minor) registered in the Australian Bureau of Meteorology’s Hydrological Geospatial Fabric spatial database V2.1.13, which intersected a 1 km buffer around the shore (as marked from the Global, Self-consistent, Hierarchical, High-resolution Geography Database v2.3.74). To account for the complexity of the Kimberley coastline, geodesic distances to freshwater outflows (i.e., shortest ‘least-cost’ trajectories by water, taking into account the presence of intervening land such as islands or capes) (Parra et al., 2006b) were calculated using bespoke code modified from functions available in the movecost R package (Alberti, 2019). Kernel density estimators are known to be sensitive to the choice of smoothing parameter (i.e., the bandwidth, h), yet selecting an appropriate bandwidth is notoriously challenging, with currently no consensus on how to do so consistently (Gitzen et al., 2006). Here, we elected to use the hpi (‘plug-in’) estimation method implemented in package ks (Duong, 2007). The hpi estimator is gaining traction in aquatic research as it has been found to outperform other algorithms and to avoid issues related to convergence failures, skewness, as well as over- and under-smoothing (e.g., Schofield et al., 2013; Jones et al., 2015; Graham et al., 2016; Nifong and Silliman, 2017). Where necessary, h values were adjusted by visual inspection of the results to ensure that output kernels were in accordance with our prior knowledge about dolphins’ environmental preferences (Sprogis et al., 2016; Doherty et al., 2017). To account for sampling effort, we divided the dolphin kernel by the density surface obtained after applying the same procedure to a set of points placed at regular 10 km intervals along survey track lines (N = 3,389, Supplementary Figure S3; Pennay et al., 2011; Derville et al., 2016). Following the above approach, we set h to 1 m on the y-axis (depth) and 1.5 km on the x-axis (distance to freshwater outflows) for dolphin sightings, and 15 m and 25 km for effort points, respectively. Inclusion probabilities for secondary sightings were taken as the values of the final, standardized kernel (rescaled to 0–1, Brook et al., 2019) at the coordinates of each point in landscape space. All primary sightings were given an inclusion probability of 1 (Figure 3). We also calculated 25 and 90 percent volume contours (PVCs) from the fitted kernel surface. PVCs are slices through the three-dimensional kernel which contain a given percentage of the volume under the fitted surface (e.g., the 90 PVC encloses 90% of the volume beneath the kernel) (Tancell et al., 2012). Doing so allowed us to delineate regions encompassing the densest 25 and 90% of observations (after back-transformation to geographic space), which we interpreted as being indicative of the species’ core areas and full range, respectively (Sveegaard et al., 2011).
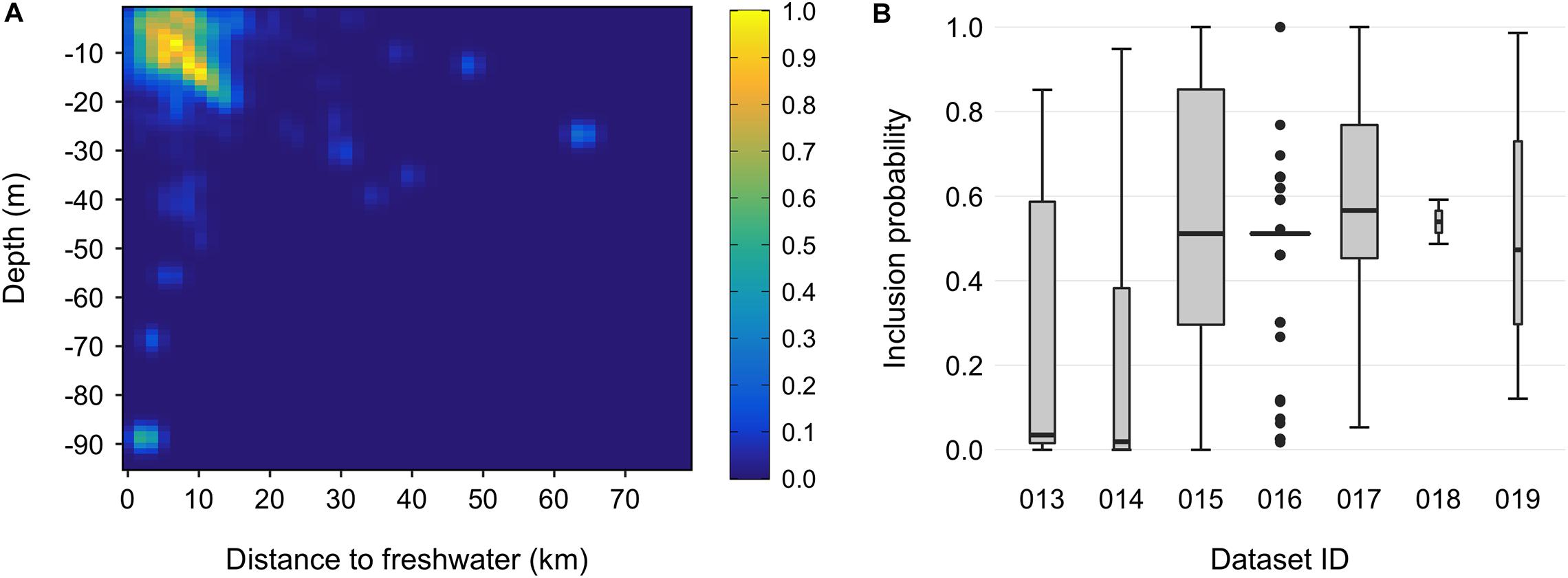
Figure 3. Inclusion probabilities for snubfin dolphin (Orcaella heinsohni) sightings derived from secondary sources. (A) Bivariate kernel probability density surface, defined in landscape space along the axes of water depth (in m) and least-cost distance to the nearest freshwater outflow (in km). The surface was calculated from primary sightings collected by trained marine mammal personnel. (B) Summary of the range of inclusion probability values for each secondary data source. Boxplot widths are proportional to sample sizes in each group. Dataset IDs and attributes are listed in Table 1.
Conservation Assessment
The IUCN relies on two key metrics to assess the conservation status of wildlife species under criterion B: the EOO and the AOO (IUCN Standards and Petitions Committee, 2019). The EOO (criterion B1) is defined as the area contained within the outermost boundaries of all known, projected, or inferred records of a species, excluding cases of vagrancy. The AOO (criterion B2) refers to the sub-area occupied by the species within the larger EOO. Both indices are highly relevant to the estimation of extinction risk, as population resilience to catastrophic events may be impaired if either is small or decreasing (Keith et al., 2017). The EOO can be calculated in a number of ways (see Gaston and Fuller, 2009), yet this is routinely done by drawing a minimum convex polygon (MCP) around occurrence points, i.e., the smallest polygon encompassing species records and in which no internal angle is greater than 180°. Despite known caveats (Burgman and Fox, 2003; Borger et al., 2006), IUCN guidelines strongly encourage the use of the MCP for RLs conducted against criterion B (IUCN Standards and Petitions Committee, 2019), largely for pragmatic reasons related to conceptual simplicity and the need to estimate EOO consistently across taxa (Joppa et al., 2016). Alternative methods include the α-hull, which is preferred when investigating temporal trends in the EOO, particularly in the context of suspected population declines (see Supplementary Appendix 1). Likewise, multiple techniques have been proposed for measuring the AOO, the most common of which entails superimposing a regular grid over the EOO and summing the area of those cells occupied by the species. The AOO is highly scale-dependent (Hartley and Kunin, 2003; Willis et al., 2003), yet a resolution of 4 km2 (i.e., 2 × 2 km cells) is deemed appropriate in most cases, as it ensures that AOO estimates are commensurate with the implicit scale of the thresholds used for delineating categories of threat (i.e., coarser scales preclude listings as critically endangered, whilst finer scales may list more taxa at higher threat categories than the definitions of these categories inherently imply) (Hartley and Kunin, 2003; Willis et al., 2003; Callmander et al., 2007). The AOO can also vary depending on the location of the grid origin (Breiner and Bergamini, 2018), however, this issue can be alleviated by iteratively testing different grid positions and choosing the one that minimizes the number of occupied cells (Moat et al., 2018). Several R packages exist for performing RLs, including Redlistr (Lee et al., 2019), red (Cardoso, 2017), and ConR (Dauby et al., 2017), all with largely similar functionalities. Here, we used ConR to quantify the EOO and the AOO for snubfins across the Kimberley. As per Cagnazzi et al. (2013b), both metrics were computed based on the positions of snubfin groups rather than individuals. We kept the package defaults, which align with IUCN guidelines and treat the EOO as an MCP, computing the AOO at 4 km2 resolution (IUCN Standards and Petitions Committee, 2019). For comparative purposes, we also ran a complementary analysis where the EOO was calculated as an α-hull (see Supplementary Appendix 1). We set the nbe.rep.rast.AOO argument to 50, so that 50 raster translations with random starting points would be generated during AOO calculations (Dauby et al., 2017). Importantly, failure to explicitly consider variance in IUCN metrics may lead to incorrect range assessments and inconsistent threat evaluations (Akçakaya et al., 2000; Currey et al., 2009). Following Brook et al. (2019), we quantified uncertainty in both the EOO and AOO using a resampling approach, whereby each data point is weighted in proportion to its reliability. In practice, we performed the analyses iteratively on n = 1,000 replicate datasets created by resampling (i) primary sightings with replacement, and (ii) secondary sightings without replacement, but with weights equal to their inclusion probability. We then calculated summary statistics, including means and 95% percentile confidence intervals.
In addition to the EOO/AOO, the focal taxon must fulfill at least two of the three criteria listed under criterion B, namely that: (a) populations are severely fragmented or known to exist in no more than a few locations, (b) a continuing decline has been observed, estimated, inferred, or projected in either the EOO, AOO, extent and/or quality of habitat, number of locations or subpopulations, or number of mature individuals, or (c) extreme fluctuations in the above are apparent (IUCN Standards and Petitions Committee, 2019). Recent photo-identification and molecular evidence indicates that snubfins form resident, genetically isolated populations with low migration rates, limited gene flow (Brown et al., 2014b), and potentially high site fidelity (Parra et al., 2006a). Although there is presently no range-wide abundance estimate for snubfins and only scant information on population trends (Parra et al., 2017), no subpopulation studied to date exceeds 250 mature individuals (Parra et al., 2006a; Cagnazzi et al., 2013b; Palmer et al., 2014a; Brown et al., 2016, 2017). Recent projections of the Kimberley marine system to the year 2050 suggest that snubfins are likely to undergo consistent declines in population size as a result of climate warming and other pressures (Boschetti et al., 2020), and it is expected that future changes in abundance will be sufficiently large and pervasive to cause a net reduction for the entire species of at least 20% over a period of two generations (Parra et al., 2017). For these reasons, we assumed that both criteria (a) and (b) were met.
Results
We obtained 3,099 occurrence records of snubfins throughout the Kimberley. After data cleaning and filtering, our final data comprised 1,207 primary and 390 secondary sightings (total N = 1,597) spanning the period 2004–2020 (Table 1). Primary sightings were collected along 34,865 km of vessel-based survey tracklines crossing seven Sea Countries and encompassing a diverse range of habitat types, from mangroves to mud/sand flats, reefs, and seagrass meadows. Sampling effort covered a total depth range of 902 m (median ± SD = 11.5 ± 80.2 m), both in proximity to, and away from, freshwater outflows (median ± SD = 9.7 ± 64.3 km) (Supplementary Figure S3). Consistent with previous findings (e.g., Parra et al., 2006b), the majority of visual detections were made in shallow, nearshore waters less than 21.3 m deep [i.e., N = 1,526 detections within the 95% highest density interval (HDI) of the associated univariate kernel distribution] and no more than 15.4 km from the nearest freshwater outflow (N = 1,533 within the 95% HDI) (Supplementary Figure S5). This pattern held true even after correcting for sampling effort (Figure 3). A small number of primary sightings (N = 11), however, also occurred in deeper waters (up to 39 m) far from any source of freshwater (up to 63.8 km). Most dolphins occurred in groups of five or less (mean ± SD = 4.4 ± 6.2). The mean inclusion probability (i.e., weights) for secondary sightings was 0.49 (± 0.25 SD), with some marginal variation apparent between datasets (Figure 3). Roebuck Bay (Yawuru Sea Country), Cygnet Bay (Bardi Jawi Sea Country), King Sound (Mayala, Bardi Jawi, Bindunbur Sea Countries), Doubtful Bay (Dambimangari Sea Country), Prince Regent River (and surrounding embayments, Dambimangari Sea Country), the seaward end of Napier Broome Bay (Wunambal Gaambera Sea Country) and the upper parts of the Cambridge Gulf (Balanggarra Sea Country) were identified as areas of high relative probability of snubfin dolphin occurrence (Supplementary Figure S6). The median EOO was 38,300 km2 (95% CI = 25,451–42,437 km2) and the median AOO was 700 km2 (95% CI = 656–736 km2) (Figure 4). Consequently, the species was identified as “Vulnerable” VU (B2 a,b) at a regional level. Note that range estimates were substantially smaller when the EOO was measured as an α-hull (11,110 km, 95% CI = 1890–19,354), leading to a potentially higher conservation listing of Endangered (EN) in some Bootstrap iterations (Supplementary Appendix 1).
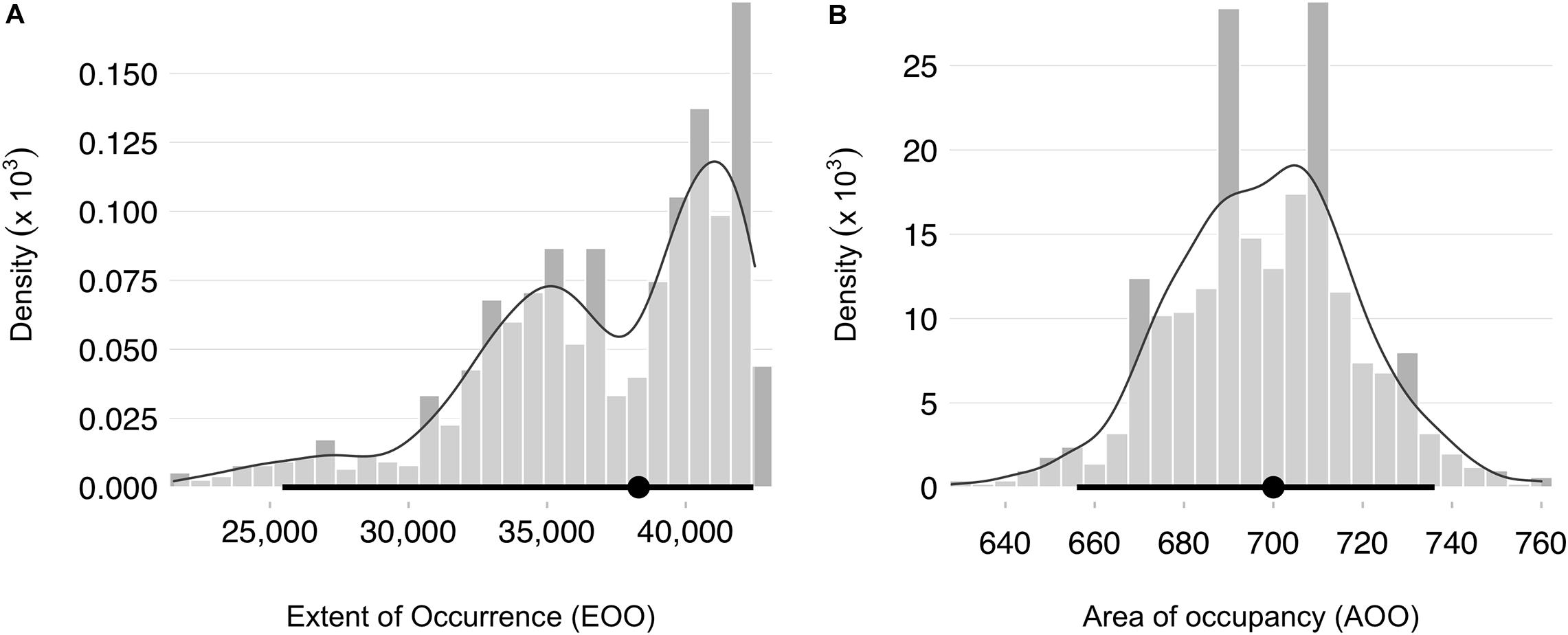
Figure 4. Distributions of regional geographic range estimates obtained by weighted resampling for snubfin dolphins (Orcaella heinsohni) in the Kimberley. (A) Extent of occurrence (EOO) calculated as the area of a minimum convex polygon encompassing all occurrence points. (B) Area of occupancy (AOO), measured as the number of 2 × 2 km grid cells occupied by dolphins. Both metrics are expressed in km. A smooth kernel density line is overlaid to facilitate interpretation. Medians and 95% percentile confidence intervals are shown in black as filled circles and segments, respectively.
Discussion
We performed the first cross-cultural geographic range assessment for snubfins in northern Western Australia against IUCN criterion B, based on the largest database of visual sightings collated to date for Orcaella heinsohni in this area. In doing so, we directly addressed an important objective of the National Research Strategy on tropical inshore dolphins (Department of the Environment, 2013, 2015), and tackled several key knowledge gaps currently hindering cetacean conservation (questions 5, 12, and 13 in Parsons et al., 2015). Our results indicate that snubfins may be regionally listed as VU B2(a,b), consistent with the threat category currently assigned to the species globally (Parra et al., 2017). Given that much of the species’ range across the Kimberley lies within jointly managed marine parks or areas managed as Indigenous Protected Areas, this listing highlights the priority need for ongoing monitoring by both the State Government and Traditional Owners. Importantly, our study also confirms snubfins’ strong (though not exclusive) preferences for shallow (<21 m depth) inshore habitats in the vicinity of freshwater outflows (<15 km), in line with previous research (Parra, 2006; Parra et al., 2006b; Palmer et al., 2014b).
Conservation Assessment
Snubfins occur in dynamic nearshore habitats, and are exposed to heterogeneous threats throughout their range (Bejder et al., 2012). In both Queensland and the Northern Territory, strong concerns have been raised about snubfin bycatch in shark control (bather protection) meshing and gillnet fisheries (Soto et al., 2013; Meager and Sumpton, 2016; Tulloch et al., 2020), as well as chronic stress from vessel traffic, noise pollution, and the discharge of contaminants (Cagnazzi et al., 2013a; Palmer and Peterson, 2013) associated with port construction activities, land clearing, and the expansion of aquaculture operations (Cagnazzi et al., 2013b; Parra and Jedensjö, 2014; Munksgaard et al., 2019). By contrast, the Kimberley is renowned for its remoteness and low human population density, but it also has a long history of subsistence, recreational, and commercial fishing (e.g., Molony et al., 2011). Very little is known about or reported on fisheries interactions with snubfins from any of the fishing sectors throughout the Kimberley. Few commercial gillnet fishers operate in the area (only four licenses; DPIRD, 2020), but recreational fishing remains a popular activity, and although no incidental entanglements of snubfins have been documented to date (Gaughan and Santoro, 2020; Tulloch et al., 2020), the limited availability of observer programs, combined with ineffective mitigation technologies (Soto et al., 2013) and an absence of records of bycaught animals suggest that under-reporting is likely. Additionally to fishing, the Kimberley also boasts a rapidly growing nature-based tourism industry (Strickland-Munro et al., 2016), produces a large proportion of the country’s offshore/onshore hydrocarbons (Moore et al., 2016), and is affected by heatwaves and other climatic extremes that are growing in frequency and intensity (Le Nohaïc et al., 2017).
These diverse and spatio-temporally varying pressures are likely to have strong impacts on coastal dolphin populations (Wild et al., 2019), especially those following independent evolutionary trajectories (Crain et al., 2009; Wallace et al., 2010). As such, genetically isolated populations of snubfins may be unable to sustain even limited levels of anthropogenic mortality (Meager and Sumpton, 2016). Recent model simulations for Kimberley marine ecosystems indicate that snubfins will also be negatively impacted by future environmental and development pressures (Boschetti et al., 2020), making regional RLs paramount for establishing monitoring baselines for the species going forward (Brown et al., 2014b, 2016, 2017).
The applicability of IUCN criteria at sub-global level has received mounting scrutiny in recent years (e.g., Milner-Gulland et al., 2006; Brito et al., 2010; Maes et al., 2012) in light of disagreements between conservation assessments performed at different scales (Mounce et al., 2018). We are aware of only one other example of a regional RL being conducted for snubfins, which indicated a lower level of threat across the Northern Territory (Palmer et al., 2017). However, both the EOO and AOO can be calculated in multiple ways, including via cartographic (i.e., grid-based) and areographic (i.e., buffer-based) methods, as well as their individual variants (Breiner and Bergamini, 2018) and combinations (Hernández and Navarro, 2007). Different methods will often yield disparate estimates of threat (Gaston and Fuller, 2009), an issue which is exacerbated by the continuing emergence of alternative metrics (e.g., Area of Habitat, AOH; Ocampo-Peñuela et al., 2016; Brooks et al., 2019; Palacio et al., 2020), and by lingering confusion surrounding how the metrics recommended by the IUCN should be implemented in practice (Breiner and Bergamini, 2018). Without a clear consensus on which approach is most appropriate, comparisons of conservation status across areas are likely to be blurred by methodological decisions that remain largely subjective. For instance, ecologists have measured the AOO using grids constructed at a range of spatial resolutions (Gaston and Fuller, 2009; Maes et al., 2012; Groom et al., 2018), from fine (e.g., 1 × 1 km; Fordham et al., 2013) to very coarse (e.g., 100 × 100 km; Scott et al., 2012). Determining a suitable cell size is not trivial and has key implications for characterizing the occupancy-abundance relationship that underpins many conservation and monitoring programs (He and Gaston, 2000; Steenweg et al., 2018). All else being equal, coarse grains risk failing to detect local population declines, while fine grains demand levels of sampling coverage that are typically incompatible with most field budgets (Joseph and Possingham, 2008). Ideally, cell sizes would be selected according to biological relevance, e.g., to match the size of individual home ranges in mobile taxa (Gaston, 1991; Keith et al., 2000). Recent studies suggest that the accuracy of AOO as an indicator of extinction risk may be maximized when grid cells are between 0.1 and 1 times the largest area plausibly impacted by a pressure event (Keith et al., 2017). Under this definition, the optimal resolution for grid-based AOO may be more closely related to the spatial scale and intensity of threats faced by organisms than to their ecology (Keith et al., 2017). Regardless, both ideas pose challenges for data-deficient species like snubfins, for which limited quantitative estimates of habitat use, home ranges or threats currently exist. We note, however, that a number of efforts are underway to map human activities across northern Australian seascapes5. Such knowledge is crucial to performing spatial risk assessments and will be key to identifying the proximate factors underlying extinction risk for the species.
Criterion B is also the most commonly misused IUCN criterion (IUCN Standards and Petitions Committee, 2019). Given the aforementioned issues, it is paramount that the precision of EOO/AOO estimates should be considered and reported in RLs (Akçakaya et al., 2000; Knapp et al., 2003; Wilson et al., 2011; Fourcade et al., 2013; Connors et al., 2014; Rueda-Cediel et al., 2018). Uncertainty accumulates over the assessment process, meaning that the way in which it is handled can profoundly influence classifications of risk (Gillespie et al., 2011) and may ultimately affect management decisions (Regan et al., 2002; D’Eon-Eggertson et al., 2015). This is especially pertinent to RLs undertaken from mixed datasets with potentially ambiguous sightings, whereby multiple uncertainties are likely to be confounded and cannot necessarily be easily disentangled. When this is the case, one of the simplest ways to represent uncertainty is to specify a best estimate and a range of plausible values, as advocated in the Red List guidelines (IUCN Standards and Petitions Committee, 2019). Great strides have been made toward developing strategies for incorporating uncertainty into RLs (Akçakaya et al., 2000; Knapp et al., 2003; Currey et al., 2009; Newton, 2010), even though this is seldom apparent in the final IUCN listing itself. However, most of these methods are either data-intensive (e.g., Currey et al., 2009), technically advanced (e.g., Bayesian networks; Newton, 2010), or require proprietary software that is not freely available. In this study, we used an intuitive empirical approach to compute confidence bounds around EOO and AOO estimates, which is not predicated on any statistical assumptions and obviates the need for complex modeling machinery. The approach relies on the weighted resampling of the sighting record, and can be easily implemented in the free software package R (Brook et al., 2019) (see code on Github at https://github.com/pjbouchet/orcaella_eoo). Rather than being arbitrarily discarded, ambiguous observations are assigned lower weights (i.e., inclusion probabilities), thereby ensuring that their contribution to the estimation is proportional to their veracity. The assignment of inclusion probabilities can be tailored to specific scenarios and need not be subjective if benchmark datasets of high reliability are available, as was the case here. Specifically, we down-weighted snubfin records made in deeper areas away from freshwater stream networks, as these represent conditions in which animals were rarely detected by professional observers (Allouche et al., 2008; Lin et al., 2017; Fletcher et al., 2019) (Figure 3 and Supplementary Figure S5).
Snubfin Distribution and Habitat Use
Shallow bathymetry and proximity to freshwater are defining features of the preferred habitats of many dolphin species at cold-temperate latitudes, including Chilean (Cephalorhynchus eutropia) (Viddi et al., 2016; Heinrich et al., 2019), Commerson’s (Cephalorhynchus commersonii) (Garaffo et al., 2011), Hector’s (Cephalorhynchus hectori), Maui’s (Cephalorhynchus hectori maui) (Derville et al., 2016), and bottlenose (Tursiops truncatus) dolphins (Mendes et al., 2002). In the tropics, Guiana (Sotalia guianensis) (Rossi-Santos et al., 2010), snubfin, Irrawaddy (O. brevirostris), Indo-Pacific humpback (Sousa chinensis), and Australian humpback (S. sahulensis) dolphins are also commonly sighted in/around intertidal channels, coastal inlets, creeks, estuaries, and river mouths (Atkins et al., 2004; Parra, 2006; Parra et al., 2006b; Minton et al., 2013; Palmer et al., 2014b; Hanf et al., 2016), although anecdotal evidence of their presence in deeper areas, e.g. around offshore islands several tens of kms from river networks or estuaries also exists (Brown et al., 2017). The drivers of these distribution patterns are not fully understood but likely relate to the availability of prey, as riverine-estuarine systems are typically productive food-rich areas where fish tend to aggregate (Bräger et al., 2003; Travers et al., 2012; McCluskey et al., 2016). Some arguments have been made that higher levels of turbidity at the confluence of fluvial runoffs and tidal fronts/currents may additionally: (1) enhance foraging efficiency, by providing dolphins with visual/acoustic hunting cover (Bräger et al., 2003; Derville et al., 2016), although possibly at the cost of reduced echolocation range (Jensen et al., 2013), and (2) reduce predation risk and increase survival, by concealing dolphins from tiger (Galeocerdo cuvier) and bull (Carcharhinus leucas) sharks (Wells et al., 1987; Mann, 2000), both of which are regular predators of nearshore dolphins around Australia (Heithaus, 2001; Simpfendorfer et al., 2001; Heithaus and Dill, 2002). To our knowledge, there is presently limited empirical evidence to support either hypothesis. Turbidity fluctuates strongly on daily, monthly, and seasonal scales (Dawe and Collie, 2017), making relationships between dolphin occurrence and water clarity both dynamic and inconsistent across species and regions (Karczmarski et al., 2000; Gomez and Cassini, 2015; Derville et al., 2016). Likewise, patterns of predation risk are complex and geographically variable, with some odontocetes actively avoiding murky habitats (Gannier and Petiau, 2006), while others seem to prefer them (Derville et al., 2016). The prevalence of shark bites on snubfins in the Kimberley is among the highest ever recorded for any dolphin species (Smith et al., 2018), suggesting that snubfins continue to use shallow turbid areas despite high apparent levels of predation risk. In this context, it is thus possible that other forms of interspecific interactions such as antagonistic encounters or resource competition with sympatric species may dictate space use decisions by snubfins to a greater extent than predation. This has been suggested in Queensland, where snubfins may avoid the aggressive and sometimes dominant Australian humpback dolphin males (Parra, 2006).
Within Western Australia, snubfins have been recorded as far south as the Pilbara and the North West Cape/Exmouth Gulf (22.41°S) (Allen et al., 2012; Hanf, 2015). Such sightings remain uncommon, however, and it is unknown whether they reflect infrequent movements by vagrant individuals, the presence of a remnant population residing in the area (Raudino et al., 2018) and/or may signify the potential for a southward distribution shift of the species with warming seas (MacLeod, 2009). By contrast, our results confirm that the coastal fringe of the Kimberley is a stronghold for snubfins, consistent with previous knowledge of dolphin occurrence throughout the region (Supplementary Figure S6). Importantly, we found that the breadth of habitat space utilized by snubfins may be more limited than previously thought, as (1) our best AOO estimate is relatively low (i.e., <1,000 km2), and (2) the 90 PVC represents only 41% of the species’ State-wide range as documented by the IUCN (Parra et al., 2017). Previous studies have shown that numerous marine species tend to occupy relatively small areas within the marginal limits of their range (Strona et al., 2012). This makes strict adherence to IUCN guidelines problematic for species distributed within narrow bands (e.g., along coastlines), as the EOO-MCP is notorious for producing biased estimates of extinction risk (Joppa et al., 2016). Part of the hurdle is that the MCP is a purely spatial metric that does not consider the characteristics necessary to maintain viable populations and fails to exclude patches of unsuitable habitat (de Castro Pena et al., 2014). Because of this, the MCP is susceptible to range overestimation, which can lead to the down-listing of taxa to lower categories of threat (Joppa et al., 2016). By comparison, more flexible methods that allow for discontinuities within a species range may overcome some of these biases (Pelayo-Villamil et al., 2015) and provide more accurate RLs. We abided by IUCN rules to allow comparability with other assessments, yet we consider the results presented in Supplementary Appendix 1 to be more representative of the conservation status of snubfins currently. Again, we emphasize that species cannot be listed under criterion B on the basis of geographic range size alone and must exhibit other symptoms of risk to qualify for threatened status (Collen et al., 2016). Our assessment is therefore predicated on the validity of our assumptions regarding dolphin population size and structure (see section “Conservation assessment”). Further studies are urgently needed to validate these, and elucidate trends in snubfin abundance, connectivity, and home range size.
Combining Mixed-Certainty Datasets
We also showed that dolphins are present in areas where they were previously suspected to occur (e.g., Long Reef and Swift Bay, Uunguu Sea Country; King George River, Balanggarra Sea Country; Doubtful Bay and Talbot Bay, Dambimangari Sea Country) but had never been hitherto documented (Figure 1 and Supplementary Figure S6). Filling such geographic gaps is critical for supporting conservation listings in remote areas (Mounce et al., 2018), and was made possible by pooling information from both conventional and “non-traditional” data sources (sensu Wood et al., 2015) over a period of 17 years. In doing so, we made the implicit assumption that the animals’ distribution throughout the Kimberley has not changed significantly over the last two decades, and we believe this assumption to be reasonable given the current state of knowledge on snubfin ecology and human pressures throughout the Kimberley. Importantly, nearly a quarter of snubfin detections were crowdsourced from reports made by untrained personnel and members of the public (Table 1). Exploiting publicly contributed data from unconventional digital information streams like social media platforms or app-based citizen science programs has been flagged as a key step in improving the current RL framework (Collen et al., 2016). However, opportunistic or anecdotal data are often considered unreliable and have been criticized for compromising assessments of geographic ranges in rare and elusive taxa (McKelvey et al., 2008). Concerns relate primarily to the challenges associated with accounting for many potential — and often unquantifiable — sources of bias, such as imperfect detection, uneven sampling coverage, taxonomic misidentifications, or regional inequalities in resourcing, field capacity, and expertise (Dickinson et al., 2010; Isaac et al., 2014). These biases can undermine the credibility of both global and regional RLs (Mounce et al., 2018), and an ongoing challenge therefore lies in finding appropriate ways of dealing with the largely unstructured data collected outwith the remit of formal scientific studies (Tenan et al., 2016). Probabilistic data integration has accordingly become an active area of methodological innovation in recent years (Bird et al., 2014; Miller et al., 2019; Isaac et al., 2020), but efforts to date have largely focused on the development of integrated predictive models (sensu Isaac et al., 2020), which were outside the scope of the present work. Rather, our objective was to demonstrate a straightforward and practical approach to combining data from disparate sources in the context of RLs, as an alternative to simple data pooling (Fletcher et al., 2019). Key to our approach was the assignment of probabilistic weights to secondary data sources, in proportion to their similarity with the array of environmental conditions that characterize those sightings made by professional observers. In essence, this allowed us to borrow information from multiple vetted primary datasets to deal with the potential weaknesses of secondary datasets, an approach akin to the philosophy behind integrated modeling (Kindsvater et al., 2018). It also obviates the dangers of relying on subjective perceptions about ecological processes to define intrinsic reliability (McKelvey et al., 2008). We note that the strong imbalance in sample sizes between primary and secondary datasets precluded a comparison of their respective contributions to threat categorizations. However, continued data collection and public engagement will likely narrow this gap and allow more formal assessments of their merits and weaknesses, as has been done elsewhere (e.g., van der Velde et al., 2017; Torney et al., 2019).
Conclusion
Data deficiency remains a pervasive obstacle to wildlife management and conservation globally, and the IUCN is currently unable to assess thousands of organisms because adequate information for those species is lacking (Bland et al., 2015). This issue is exacerbated in tropical environments, where reports of inconspicuous or uncommon taxa are typically sparse and of lesser quality due to cryptic (e.g., boat-shy) animal behavior, uneven sampling effort, as well as the technical difficulties associated with surveying remote and vast seascapes (Braulik et al., 2018). Species that naturally occur in low numbers also make it difficult to assess population trends over time (Brooks et al., 2017). Snubfins are a compelling example of this, as few studies of the animals’ abundance or geographic range have been undertaken since the species was formally described in 2005, except at a few key sites (Parra et al., 2006a,b; Cagnazzi et al., 2013b; Palmer et al., 2014a; Brown et al., 2016). Knowledge gaps such as these limit our capacity to quantify threats and monitor the condition of populations subject to varying pressures (Braulik et al., 2018). Our work illustrates a collaborative, multi-stakeholder approach (Figures 2C–F) to bringing disparate datasets together to build a better picture of the distribution and range of snubfin dolphins within a region regarded as a hotspot of future extinction risk (Davidson et al., 2012). Indigenous Healthy Country management practices and cross-cultural engagement have been critical in informing other IUCN conservation assessments for threatened mammals in Australia and beyond (Balanggarra Aboriginal Corporation, 2011; Bardi and Jawi Niimidiman Aboriginal Corporation, 2013; Ziembicki et al., 2013), and have played a key role in supporting many facets of wildlife management across the Kimberley (Austin et al., 2017, 2019). Such an inclusive and complementary approach built on equal partnerships and mutually beneficial, two-way knowledge exchange should be the norm in areas belonging to and managed by Indigenous people (Woodward et al., 2020). Logical next steps for snubfin research will be to estimate the home range size of individual animals to better approximate their AOO, and to build predictive statistical models that can map dolphin density at fine scales (Syfert et al., 2014). Recent studies are lending growing support to the usefulness of the latter for delimiting the EOO/AOO while simultaneously accounting for the ecological requirements of focal species (e.g., de Castro Pena et al., 2014; Fivaz and Gonseth, 2014; Sousa-Silva et al., 2014; but see Attorre et al., 2013; Breiner et al., 2017 for counter-points); yet while preliminary models have been developed for Australian humpback dolphins in adjacent areas (Hanf, 2015), none are yet available for snubfins. The recent gazettal of the Greater Kimberley Marine Park6, in combination with the formation of Saltwater Indigenous Protected Areas (Rist et al., 2019) and the recognition of dolphins as a value within them, may provide the impetus necessary to sustain new Indigenous-driven, collaborative monitoring programs that can generate the data necessary to underpin these efforts.
Data Availability Statement
A video showcasing the collaborative snubfin dolphin surveys undertaken by the Nyamba Buru Yawuru Land and Sea Unit, the Western Australian Department of Biodiversity, Conservation and Attractions, and the World Wildlife Fund Australia on Yawuru Sea Country can be viewed at https://bit.ly/snubfin-dolphins-Yawuru. R code for reproducing the analyses can be found on Github (https://github.com/pjbouchet/orcaella_eoo). All sightings compiled in this study have either been previously published or are in the public domain (see below), with the exception of observations collected by Ranger groups (dataset OH-005, Table 1). Those data are currently supporting ongoing projects and cannot be made readily available at the time of publication. Any requests for information should be directed to the relevant data custodians, namely: Balanggarra Rangers (YmFsYW5nZ2FycmFyYW5nZXJzQGtsYy5vcmcuYXU=), Bardi Jawi Rangers (YmFyZGlqYXdpcmFuZ2Vyc0BrbGMub3JnLmF1), Dambimangari Rangers (bC52YXVnaGFuQGRhbWJpLm9yZy5hdQ==), Nyamba Buru Yawuru Rangers (ZGVhbi5tYXRoZXdzQHlhd3VydS5vcmcuYXU=), Nyul Nyul Rangers (bnl1bG55dWxyYW5nZXJzQGtsYy5vcmcuYXU=), and Uunguu Rangers (aW5mb0B3dW5hbWJhbGdhYW1iZXJhLm9yZy5hdQ==). The datasets OH-001, OH-002, OH-004, OH-008, OH-009, OH-011, OH-015, OH-016, OH-017, and OH-019 are available on the Open Science Framework (https://osf.io/kgdu9/). The datasets OH-003, OH-006, OH-007, OH-010 are published in Jenner et al. (2014), Brown et al. (2016), Brown et al. (2017), and Allen et al. (2012), respectively. OH-012 and OH-014 are hosted on NatureMap (https://naturemap.dbca.wa.gov.au/, free registration required). The datasets OH-013 and OH-018 are available from https://data.csiro.au/collections/ and https://ala.org.au/ respectively.
Ethics Statement
Ethical review and approval was not required for the animal study because no animals were used. All surveys from which sightings were sourced received relevant ethics approvals. Written informed consent was obtained from the individual(s) for the publication of any potentially identifiable images or data included in this article.
Author Contributions
DT, PB, SM, KW, HR, FW, Balanggarra Rangers, Bardi Jawi Rangers, Dambimangari Rangers, Nyamba Buru Yawuru Rangers, and Uunguu Rangers contributed to the acquisition of field data and survey designs in various capacities. PB, DT, HR, KW, and SM organized the database and compiled the literature. FW coordinated the participation of Indigenous Ranger groups in dolphin surveys led by DT. PB analyzed the data, in consultation with HR, KW, DT, and SM. PB led the writing of the manuscript, with all authors making active contributions to drafting, critical review, and editorial development. All the authors have approved the final version of the article.
Funding
This research was financially supported by the following organizations: The Australian Marine Mammal Centre, Mia J. Tegner Award, The Whale and Dolphin Conservation Society UK (WDCS), W. V. Scott Estate, Commonwealth Department of Environment (Caring for Our Country), WWF Australia, the Western Australian Department of Biodiversity, Conservation and Attractions, and Ghostnets Australia.
Conflict of Interest
The authors declare that the research was conducted in the absence of any commercial or financial relationships that could be construed as a potential conflict of interest.
Acknowledgments
In addition to co-authors, we are thankful to Mayala and Bindunbur Traditional Owners, who have generously shared their knowledge on dolphins in their Sea Countries. We acknowledge the significant contribution to this research provided by the Yawuru community, who have allowed us to conduct snubfin dolphin and marine wildlife research on Yawuru Sea Country since 2004 (>10 years). In particular, we would like to thank the following individuals: Julie Melbourne, Dean Mathews, Nathan Kay, Cornell Tolentino, Philip Pedro, Johani Mamid, Eduardo Maher, Pius Gregory, Ronald Wade, and Wayne Edgar, as well as the Dambimangari community including James Mansfield, Janet Oobagooma, Crystal Oobagooma, Kieran Bangmorra, Warren Barunga, Gary Umbagai, Frank Martin, Pete O’Connor, and Vicki Numaduma. The following Rangers took part in field research activities: (Balanggarra) Michael Morlumbun; (Bardi Jawi) Chris Sampi; (Nyul Nyul) Kenneth Callaghan; (Uunguu) Jason Adams, Dorothy Djanghara, Raphael Karadada, Sylvester Mangolamara, Neil Waina, Robert Warren, and Desmond Williams. We thank the skippers and crews of the vessels used during field surveys, namely: Garry McKechnie, Damien Cook (SV Pelican), Pam Canny and Ian Lew (RV Rachel), Tony Richards (The Orcaella), Trippy and Geoff (MV Kimberley Escape). The Northern Australia Quarantine Strategy (NAQS), the Australian Customs Service (ACS), and the Kimberley Land Council (KLC) coordinated and participated in surveys. DT would like to acknowledge assistance from the Broome Bird Observatory, Environs Kimberley, the Roebuck Bay Working Group, and the following dedicated individuals: Tanya Vernes and Jessica Chapman (formerly WWF-Australia), Debra Glasgow, Marguerite Tarzia, Marty Gent, Carol Palmer, Alexander Brown, Pete Gill, Lisa Ballance, Bob Pitman, Kate Stafford, Alexander Watson, Jason Fowler, Martin Pritchard, Brendan G. Mackey, Leslie Thiele, Chandra Salgado-Kent, Matthew Grey, Kandy Curran, and Kate Golson. DBCA staff Danny Barrow, Adrian Lane, Darren Stevens, Michael Hourn, Brett Francis, Cameron Smith, Luke Puertollano, Jason Fong, Anthony Richardson, Corrine Douglas, Ellen D’Cruz, Sarah Mullineux, Jesse Murdoch, and Chris Nutt have all been integral to snubfin records in Yawuru Nagulagun/Roebuck Bay and Lalang-garram/Camden Sound Marine Parks. We thank Peter Bayliss and Marlee Hutton for providing the aerial survey data. Lastly, we thank two reviewers for providing thoughtful and constructive comments on an earlier draft.
Supplementary Material
The Supplementary Material for this article can be found online at: https://www.frontiersin.org/articles/10.3389/fmars.2020.614852/full#supplementary-material
Footnotes
- ^ https://dolfin.dbca.wa.gov.au/
- ^ http://www.ausseabed.gov.au/surveys-data/data
- ^ http://www.bom.gov.au/water/geofabric/
- ^ https://www.soest.hawaii.edu/pwessel/gshhg/
- ^ https://bit.ly/nesp-a12-pressure-mapping
- ^ https://parks.dpaw.wa.gov.au/connect/read/great-kimberley-marine-park
References
Aiello-Lammens, M. E., Boria, R. A., Radosavljevic, A., Vilela, B., and Anderson, R. P. (2015). spThin: an R package for spatial thinning of species occurrence records for use in ecological niche models. Ecography 38, 541–545. doi: 10.1111/ecog.01132
Akçakaya, H. R., Ferson, S., Burgman, M. A., Keith, D. A., Mace, G. M., and Todd, C. R. (2000). Making consistent IUCN classifications under uncertainty. Conserv. Biol. 14, 1001–1013. doi: 10.1046/j.1523-1739.2000.99125.x
Alberti, G. (2019). movecost: an R package for calculating accumulated slope-dependent anisotropic cost-surfaces and least-cost paths. SoftwareX 10:100331. doi: 10.1016/j.softx.2019.100331
Alessi, J., Bruccoleri, F., and Cafaro, V. (2019). How citizens can encourage scientific research: the case study of bottlenose dolphins monitoring. Ocean Coast. Manag. 167, 9–19. doi: 10.1016/j.ocecoaman.2018.09.018
Allen, S. J., Cagnazzi, D. D., Hodgson, A. J., Loneragan, N. R., and Bejder, L. (2012). Tropical inshore dolphins of north-western Australia: unknown populations in a rapidly changing region. Pacific Conserv. Biol. 18, 56–63. doi: 10.1071/PC120056
Allouche, O., Steinitz, O., Rotem, D., Rosenfeld, A., and Kadmon, R. (2008). Incorporating distance constraints into species distribution models. J. Appl. Ecol. 45, 599–609. doi: 10.1111/j.1365-2664.2007.01445.x
Atkins, S., Pillay, N., and Peddemors, V. (2004). Spatial distribution of Indo-Pacific humpback dolphins (Sousa chinensis) at Richards Bay, South Africa: environmental influences and behavioural patterns. Aquat. Mamm. 30, 84–93. doi: 10.1578/am.30.1.2004.84
Attorre, F., De Sanctis, M., Farcomeni, A., Guillet, A., Scepi, E., Vitale, M., et al. (2013). The use of spatial ecological modelling as a tool for improving the assessment of geographic range size of threatened species. J. Nat. Conserv. 21, 48–55. doi: 10.1016/j.jnc.2012.10.001
Austin, B. J., Robinson, C. J., Mathews, D., Oades, D., Wiggin, A., Dobbs, R. J., et al. (2019). An Indigenous-led approach for regional knowledge partnerships in the Kimberley region of Australia. Hum. Ecol. 47, 577–588. doi: 10.1007/s10745-019-00085-9
Austin, B. J., Vigilante, T., Cowell, S., Dutton, I. M., Djanghara, D., Mangolomara, S., et al. (2017). The Uunguu monitoring and evaluation committee: intercultural governance of a land and sea management programme in the Kimberley. Australia. Ecol. Manag. Restor. 18, 124–133. doi: 10.1111/emr.12257
Balanggarra Aboriginal Corporation (2011). Balanggarra Healthy Country Plan 2012-2022. Available online at: https://www.klc.org.au/s/balanggarra-healthy-country-plan-2012-2022.pdf (accessed September 2020).
Bardi and Jawi Niimidiman Aboriginal Corporation (2013). Bardi Jawi Indigenous Protected Area Management Plan 2013-2023. Broome WA: Bardi and Jawi Niimidiman Aboriginal Corporation.
Barlow, J. (2015). Inferring trackline detection probabilities, g(0), for cetaceans from apparent densities in different survey conditions. Mar. Mammal Sci. 31, 923–943. doi: 10.1111/mms.12205
Bayliss, P. (1986). Factors affecting aerial surveys of marine fauna, and their relationship to a census of dugongs in the coastal waters of the northern territory. Wildl. Res. 13, 27–37. doi: 10.1071/WR9860027
Bayliss, P., and Freeland, W. J. (1989). Seasonal distribution and abundance of dugongs in the western Gulf of Carpentaria. Wildl. Res. 16, 141–149. doi: 10.1071/WR9890141
Bayliss, P., and Hutton, M. (2017). Integrating Indigenous Knowledge and Survey Techniques to Develop a Baseline for Dugong (Dugong dugon) Management in the Kimberley. Perth, WA.: Node of the Western Australian Marine Science Institution. Final Report of project 1.2.5 of the Kimberley Marine Research Program.
Bayliss, P., and Wilcox, C. (2015). Integrating Indigenous Knowledge and Survey Techniques to Develop a Baseline for Dugong (Dugong dugon) Management in the Kimberley. Perth, WA: Node of the Western Australian Marine Science Institution. Final Report for Phase 1 of Project 1.2.5 of the Kimberley Marine Research Program.
Beasley, I., Robertson, K., Arnold, P., Robertson Kelly, M., and Arnold, P. (2005). Description of a new dolphin, the Australian snubfin dolphin Orcaella heinsohni sp. (Cetacea, Delphinidae). Mar. Mammal Sci. 21, 365–400. doi: 10.1111/j.1748-7692.2005.tb01239.x
Bejder, L., Hodgson, A., Loneragan, N., and Allen, S. (2012). Coastal dolphins in north-western Australia: the need for re-evaluation of species listings and short-comings in the environmental impact assessment process. Pacific Conserv. Biol. 18, 22–25. doi: 10.1071/PC120022
Bird, T. J., Bates, A. E., Lefcheck, J. S., Hill, N. A., Thomson, R. J., Edgar, G. J., et al. (2014). Statistical solutions for error and bias in global citizen science datasets. Biol. Conserv. 173, 144–154. doi: 10.1016/j.biocon.2013.07.037
Bland, L. M., Collen, B., Orme, C. D. L., and Bielby, J. (2015). Predicting the conservation status of data-deficient species. Conserv. Biol. 29, 250–259. doi: 10.1111/cobi.12372
Borger, L., Franconi, N., De Michele, G., Gantz, A., Meschi, F., Manica, A., et al. (2006). Effects of sampling regime on the mean and variance of home range size estimates. J. Anim. Ecol. 75, 1393–1405. doi: 10.1111/j.1365-2656.2006.01164.x
Boschetti, F., Lozano-Montes, H., and Stelfox, B. (2020). Modelling regional futures at decadal scale: application to the Kimberley region. Sci. Rep. 10:849. doi: 10.1038/s41598-019-56646-x
Bräger, S., Harraway, J. A., and Manly, B. F. J. (2003). Habitat selection in a coastal dolphin species (Cephalorhynchus hectori). Mar. Biol. 143, 233–244. doi: 10.1007/s00227-003-1068-x
Braulik, G. T., Kasuga, M., Wittich, A., Kiszka, J. J., MacCaulay, J., Gillespie, D., et al. (2018). Cetacean rapid assessment: an approach to fill knowledge gaps and target conservation across large data deficient areas. Aquat. Conserv. Mar. Freshw. Ecosyst. 28, 216–230. doi: 10.1002/aqc.2833
Breiner, F. T., and Bergamini, A. (2018). Improving the estimation of area of occupancy for IUCN Red List assessments by using a circular buffer approach. Biodivers. Conserv. 27, 2443–2448. doi: 10.1007/s10531-018-1555-5
Breiner, F. T., Guisan, A., Nobis, M. P., and Bergamini, A. (2017). Including environmental niche information to improve IUCN Red List assessments. Divers. Distrib. 23, 484–495. doi: 10.1111/ddi.12545
Brito, D., Ambal, R. G., Brooks, T., De Silva, N., Foster, M., Hao, W., et al. (2010). How similar are national Red Lists and the IUCN Red List? Biol. Conserv. 143, 1154–1158. doi: 10.1016/j.biocon.2010.02.015
Brook, B. W., Buettel, J. C., and Jarić, I. (2019). A fast re-sampling method for using reliability ratings of sightings with extinction-date estimators. Ecology 100:e02787. doi: 10.1002/ecy.2787
Brooks, L., Carroll, E., and Pollock, K. H. (2014). ). Methods for Assessment of the Conservation Status of Australian Inshore Dolphins. Canberra: Final report to the Department of the Environment.
Brooks, L., Palmer, C., Griffiths, A. D., and Pollock, K. H. (2017). Monitoring variation in small coastal dolphin populations: an example from Darwin, Northern Territory, Australia. Front. Mar. Sci. 4:94. doi: 10.3389/fmars.2017.00094
Brooks, T. M., Pimm, S. L., Akçakaya, H. R., Buchanan, G. M., Butchart, S. H. M., Foden, W., et al. (2019). Measuring terrestrial area of habitat (AOH) and its utility for the IUCN Red List. Trends Ecol. Evol. 34, 977–986. doi: 10.1016/j.tree.2019.06.009
Brown, A. M., Bejder, L., Pollock, K. H., and Allen, S. J. (2014a). Abundance of Coastal Dolphins in Roebuck Bay, Western Australia: Updated results from 2013 and 2014 Sampling Periods. Perth, WA: WWF-Australia. Report to WWF-Australia.
Brown, A. M., Kopps, A. M., Allen, S. J., Bejder, L., Littleford-Colquhoun, B., Parra, G. J., et al. (2014b). Population differentiation and hybridisation of Australian snubfin (Orcaella heinsohni) and Indo-Pacific humpback (Sousa chinensis) dolphins in North-Western Australia. PLoS One 9:e101427. doi: 10.1371/journal.pone.0101427
Brown, A. M., Bejder, L., Pollock, K. H., and Allen, S. J. (2016). Site-specific assessments of the abundance of three inshore dolphin species to inform conservation and management. Front. Mar. Sci. 3:4. doi: 10.3389/fmars.2016.00004
Brown, A. M., Smith, J., Salgado-Kent, C., Marley, S. A., Allen, S. J., Thiele, D., et al. (2017). Relative Abundance, Population Genetic Structure and Acoustic Monitoring of Australian Snubfin and Humpback Dolphins in Regions Within the Kimberley. Perth WA: Western Australian Marine Science Institution. Final report of Project 1.2.4 prepared for the Kimberley Marine Research Program.
Burgman, M. A., and Fox, J. C. (2003). Bias in species range estimates from minimum convex polygons: implications for conservation and options for improved planning. Anim. Conserv. 6, 19–28. doi: 10.1017/s1367943003003044
Cagnazzi, D., Fossi, M. C., Parra, G. J., Harrison, P. L., Maltese, S., Coppola, D., et al. (2013a). Anthropogenic contaminants in Indo-Pacific humpback and Australian snubfin dolphins from the central and southern Great Barrier Reef. Environ. Pollut. 182, 490–494. doi: 10.1016/j.envpol.2013.08.008
Cagnazzi, D., Parra, G. J., Westley, S., and Harrison, P. L. (2013b). At the heart of the industrial boom: Australian snubfin dolphins in the Capricorn coast, Queensland, need urgent conservation action. PLoS One 8:e56729. doi: 10.1371/journal.pone.0056729
Callmander, M. W., Schatz, G. E., Lowry, P. P. II, Laivao, M. O., Raharimampionona, J., Andriambololonera, S., et al. (2007). Identification of priority areas for plant conservation in Madagascar using Red List criteria: rare and threatened Pandanaceae indicate sites in need of protection. Oryx 41, 168–176. doi: 10.1017/S0030605307001731
Cardoso, P. (2017). red - An R package to facilitate species red list assessments according to the IUCN criteria. Biodivers. Data J. 5:e20530. doi: 10.3897/BDJ.5.e20530
Cheney, B., Thompson, P. M., Ingram, S. N., Hammond, P. S., Stevick, P. T., Durban, J. W., et al. (2013). Integrating multiple data sources to assess the distribution and abundance of bottlenose dolphins Tursiops truncatus in Scottish waters. Mamm. Rev. 43, 71–88. doi: 10.1111/j.1365-2907.2011.00208.x
Collen, B., Dulvy, N. K., Gaston, K. J., Gärdenfors, U., Keith, D. A., Punt, A. E., et al. (2016). Clarifying misconceptions of extinction risk assessment with the IUCN Red List. Biol. Lett. 12:20150843. doi: 10.1098/rsbl.2015.0843
Connors, B. M., Cooper, A. B., Peterman, R. M., and Dulvy, N. K. (2014). The false classification of extinction risk in noisy environments. Proc. R. Soc. B Biol. Sci. 281:20132935. doi: 10.1098/rspb.2013.2935
Crain, C. M., Halpern, B. S., Beck, M. W., and Kappel, C. V. (2009). Understanding and managing human threats to the coastal marine environment. Ann. N. Y. Acad. Sci. 1162, 39–62. doi: 10.1111/j.1749-6632.2009.04496.x
Currey, R. J. C., Dawson, S. M., and Slooten, E. (2009). An approach for regional threat assessment under IUCN Red List criteria that is robust to uncertainty: the Fiordland bottlenose dolphins are critically endangered. Biol. Conserv. 142, 1570–1579. doi: 10.1016/j.biocon.2009.02.036
Dauby, G., Stévart, T., Droissart, V., Cosiaux, A., Deblauwe, V., Simo-Droissart, M., et al. (2017). ConR: an R package to assist large-scale multispecies preliminary conservation assessments using distribution data. Ecol. Evol. 7, 11292–11303. doi: 10.1002/ece3.3704
Davidson, A. D., Boyer, A. G., Kim, H., Pompa-Mansilla, S., Hamilton, M. J., Costa, D. P., et al. (2012). Drivers and hotspots of extinction risk in marine mammals. Proc. Natl. Acad. Sci. U.S.A. 109, 3395–3400. doi: 10.1073/pnas.1121469109
Dawe, S., and Collie, T. (2017). “Turbidity dynamics in a macro-tidal tropical channel,” in Australasian Coasts & Ports 2017: Working with Nature (Barton, ACT: Engineers Australia), 335–342.
Dawson, S. D., Wade, P., Slooten, E., and Barlow, J. A. Y. (2008). Design and field methods for sighting surveys of cetaceans in coastal and riverine habitats. Mamm. Rev. 38, 19–49. doi: 10.1111/j.1365-2907.2008.00119.x
de Castro Pena, J. C., Kamino, L. H. Y., Rodrigues, M., Mariano-Neto, E., and de Siqueira, M. F. (2014). Assessing the conservation status of species with limited available data and disjunct distribution. Biol. Conserv. 170, 130–136. doi: 10.1016/j.biocon.2013.12.015
de Freitas, M., Smith, J. N., Jensen, F. H., Beedholm, K., and Madsen, P. T. (2018). Echolocation click source parameters of Australian snubfin dolphins (Orcaella heinsohni). J. Acoust. Soc. Am. 143, 2564–2569. doi: 10.1121/1.5034174
D’Eon-Eggertson, F., Dulvy, N. K., and Peterman, R. M. (2015). Reliable identification of declining populations in an uncertain world. Conserv. Lett. 8, 86–96. doi: 10.1111/conl.12123
Department of the Environment (2013). A Coordinated Research Framework to Assess the National Conservation Status of Australian Snubfin Dolphins (Orcaella heinsohni) and Other Tropical Inshore Dolphins. Canberra: Australian Government Department of the Environment.
Department of the Environment (2015). A Coordinated National Research Framework to Inform the Conservation and Management of Australia’s Tropical Inshore Dolphins. Canberra: Australian Government Department of the Environment.
Derville, S., Constantine, R., Baker, C. S., Oremus, M., and Torres, L. G. (2016). Environmental correlates of nearshore habitat distribution by the Critically Endangered Māui dolphin. Mar. Ecol. Prog. Ser. 551, 261–275. doi: 10.3354/meps11736
Devictor, V., Whittaker, R. J., and Beltrame, C. (2010). Beyond scarcity: citizen science programmes as useful tools for conservation biogeography. Divers. Distrib. 16, 354–362. doi: 10.1111/j.1472-4642.2009.00615.x
Dickinson, J. L., Zuckerberg, B., and Bonter, D. N. (2010). Citizen science as an ecological research tool: challenges and benefits. Annu. Rev. Ecol. Evol. Syst. 41, 149–172. doi: 10.1146/annurev-ecolsys-102209-144636
Doherty, P. D., Baxter, J. M., Godley, B. J., Graham, R. T., Hall, G., Hall, J., et al. (2017). Testing the boundaries: seasonal residency and inter-annual site fidelity of basking sharks in a proposed marine protected area. Biol. Conserv. 209, 68–75. doi: 10.1016/j.biocon.2017.01.018
Domisch, S., Wilson, A. M., and Jetz, W. (2016). Model-based integration of observed and expert-based information for assessing the geographic and environmental distribution of freshwater species. Ecography 39, 1078–1088. doi: 10.1111/ecog.01925
DPIRD (2020). 2020 Annual Report From the Department of Primary Industries and Regional Development. Waroona WA: DPIRD.
Dunshea, G., Groom, R., and Griffiths, A. D. (2020). Observer performance and the effect of ambiguous taxon identification for fixed strip-width dugong aerial surveys. J. Exp. Mar. Bio. Ecol. 526:151338. doi: 10.1016/j.jembe.2020.151338
Duong, T. (2007). Ks: kernel density estimation and kernel discriminant analysis for multivariate data in R. J. Stat. Softw. 21, 1–16. doi: 10.18637/jss.v021.i07
Embling, C. B., Walters, A. E. M., and Dolman, S. J. (2015). How much effort is enough? the power of citizen science to monitor trends in coastal cetacean species. Glob. Ecol. Conserv. 3, 867–877. doi: 10.1016/j.gecco.2015.04.003
Fivaz, F. P., and Gonseth, Y. (2014). Using species distribution models for IUCN Red lists of threatened species. J. Insect Conserv. 18, 427–436. doi: 10.1007/s10841-014-9652-6
Fletcher, R. J., Hefley, T. J., Robertson, E. P., Zuckerberg, B., McCleery, R. A., and Dorazio, R. M. (2019). A practical guide for combining data to model species distributions. Ecology 100:e02710. doi: 10.1002/ecy.2710
Fordham, D. A., Mellin, C., Russell, B. D., Akçakaya, R. H., Bradshaw, C. J. A., Aiello-Lammens, M. E., et al. (2013). Population dynamics can be more important than physiological limits for determining range shifts under climate change. Glob. Chang. Biol. 19, 3224–3237. doi: 10.1111/gcb.12289
Fourcade, Y., Engler, J. O., Besnard, A. G., Rödder, D., and Secondi, J. (2013). Confronting expert-based and modelled distributions for species with uncertain conservation status: a case study from the corncrake (Crex crex). Biol. Conserv. 167, 161–171. doi: 10.1016/j.biocon.2013.08.009
Gannier, A., and Petiau, E. (2006). Environmental variables affecting the residence of spinner dolphins (Stenella longirostris) in a bay of Tahiti (French Polynesia). Aquat. Mamm. 32, 202–211. doi: 10.1578/AM.32.2.2006.202
Garaffo, G. V., Dans, S. L., Pedraza, S. N., Degrati, M., Schiavini, A., and González, R. Ú, et al. (2011). Modeling habitat use for dusky dolphin and Commerson’s dolphin in Patagonia. Mar. Ecol. Prog. Ser. 421, 217–227. doi: 10.3354/meps08912
Gärdenfors, U. (2001). Classifying threatened species at national versus global levels. Trends Ecol. Evol. 16, 511–516. doi: 10.1016/S0169-5347(01)02214-5
Gärdenfors, U., Hilton-Taylor, C., Mace, G. M., and Rodríguez, J. P. (2008). The application of IUCN Red List criteria at regional levels. Conserv. Biol. 15, 1206–1212. doi: 10.1111/j.1523-1739.2001.00112.x
Gaston, K. J. (1991). How large Is a species’ geographic range? Oikos 61, 434–438. doi: 10.2307/3545251
Gaston, K. J., and Fuller, R. A. (2009). The sizes of species’ geographic ranges. J. Appl. Ecol. 46, 1–9. doi: 10.2307/27695914
Gaughan, D. J., and Santoro, K. (2020). Status Reports of the Fisheries and Aquatic Resources of Western Australia 2018/19: The State of the Fisheries. Perth WA: Department of Primary Industries and Regional Deve.
Gillespie, G. R., Scroggie, M. P., Roberts, J. D., Cogger, H. G., Mahony, M. J., and McDonald, K. R. (2011). The influence of uncertainty on conservation assessments: Australian frogs as a case study. Biol. Conserv. 144, 1516–1525. doi: 10.1016/j.biocon.2010.10.031
Gitzen, R. A., Millspaugh, J. J., and Kernohan, B. J. (2006). Bandwidth selection for fixed-kernel analysis of animal utilization distributions. J. Wildl. Manag. 70, 1334–1344. doi: 10.2193/0022-541x(2006)70[1334:bsffao]2.0.co;2
Gomez, J. J., and Cassini, M. H. (2015). Environmental predictors of habitat suitability and biogeographical range of Franciscana dolphins (Pontoporia blainvillei). Glob. Ecol. Conserv. 3, 90–99. doi: 10.1016/j.gecco.2014.11.007
Graham, F., Rynne, P., Estevanez, M., Luo, J., Ault, J. S., and Hammerschlag, N. (2016). Use of marine protected areas and exclusive economic zones in the subtropical western North Atlantic Ocean by large highly mobile sharks. Divers. Distrib. 22, 534–546. doi: 10.1111/ddi.12425
Grech, A., Parra, G. J., Beasley, I., Bradley, J., Johnson, S., Whiting, S., et al. (2014). Local assessments of marine mammals in cross-cultural environments. Biodivers. Conserv. 23, 3339–3340. doi: 10.1007/s10531-014-0800-9
Groom, Q. J., Marsh, C. J., Gavish, Y., and Kunin, W. E. (2018). How to predict fine resolution occupancy from coarse occupancy data. Methods Ecol. Evol. 9, 2273–2284. doi: 10.1111/2041-210X.13078
Guttmacher, R. (2016). Automating Quality Control in a Crowd-Sourced Marine mAmmal. PhD thesis, University of Redlands, Redlands, CA.
Hanf, D. M. (2015). Species Distribution Modelling of Western Pilbara Inshore Dolphins. Masters thesis, Murdoch University, Murdoch WA.
Hanf, D. M., Hunt, T., and Parra, G. J. (2016). Humpback dolphins of Western Australia: a review of current knowledge and recommendations for future management. Adv. Mar. Biol. 73, 193–218. doi: 10.1016/bs.amb.2015.07.004
Hartley, S., and Kunin, W. E. (2003). Scale dependency of rarity, extinction risk, and conservation priority. Conserv. Biol. 17, 1559–1570. doi: 10.1111/j.1523-1739.2003.00015.x
He, F., and Gaston, K. J. (2000). Occupancy-abundance relationships and sampling scales. Ecography 23, 503–511. doi: 10.1111/j.1600-0587.2000.tb00306.x
Heinrich, S., Genov, T., Fuentes Riquelme, M., and Hammond, P. S. (2019). Fine-scale habitat partitioning of Chilean and Peale’s dolphins and their overlap with aquaculture. Aquat. Conserv. Mar. Freshw. Ecosyst. 29, 212–226. doi: 10.1002/aqc.3153
Heithaus, M. R. (2001). Predator–prey and competitive interactions between sharks (order Selachii) and dolphins (suborder Odontoceti): a review. J. Zool. 253:S0952836901000061. doi: 10.1017/S0952836901000061
Heithaus, M. R., and Dill, L. M. (2002). Food availability and tiger shark predation risk influence bottlenose dolphin habitat use. Ecology 83, 480–491. doi: 10.1890/0012-9658(2002)083[0480:faatsp]2.0.co;2
Hernández, H. M., and Navarro, M. (2007). A new method to estimate areas of occupancy using herbarium data. Biodivers. Conserv. 16, 2457–2470. doi: 10.1007/s10531-006-9134-6
Hodgson, A., Kelly, N., and Peel, D. (2013). Unmanned aerial vehicles (UAVs) for surveying marine fauna: a dugong case study. PLoS One 8:e79556. doi: 10.1371/journal.pone.0079556
Hoffmann, M., Brooks, T. M., Da Fonseca, G. A. B., Gascon, C., Hawkins, A. F. A., James, R. E., et al. (2008). Conservation planning and the IUCN Red List. Endanger. Species Res. 6, 113–125. doi: 10.3354/esr00087
Hunt, T., Bejder, L., Allen, S., Rankin, R., Hanf, D., and Parra, G. (2017). Demographic characteristics of Australian humpback dolphins reveal important habitat toward the southwestern limit of their range. Endanger. Species Res. 32, 71–88. doi: 10.3354/esr00784
Isaac, N. J. B., Jarzyna, M. A., Keil, P., Dambly, L. I., Boersch-Supan, P. H., Browning, E., et al. (2020). Data integration for large-scale models of species distributions. Trends Ecol. Evol. 35, 56–67. doi: 10.1016/j.tree.2019.08.006
Isaac, N. J. B., and Pocock, M. J. O. (2015). Bias and information in biological records. Biol. J. Linn. Soc. 115, 522–531. doi: 10.1111/bij.12532
Isaac, N. J. B., van Strien, A. J., August, T. A., de Zeeuw, M. P., and Roy, D. B. (2014). Statistics for citizen science: extracting signals of change from noisy ecological data. Methods Ecol. Evol. 5, 1052–1060. doi: 10.1111/2041-210X.12254
IUCN Standards and Petitions Committee (2019). Guidelines for Using the IUCN Red List Categories and Criteria, Version 14. Available online at: https://www.iucnredlist.org/resources/redlistguidelines (accessed March 15, 2020).
Jackson, M. V., Kennett, R., Bayliss, P., Warren, R., Waina, N., Adams, J., et al. (2015). Developing collaborative marine turtle monitoring in the Kimberley region of northern Australia. Ecol. Manag. Restor. 16, 163–176. doi: 10.1111/emr.12184
Jarić, I., and Roberts, D. L. (2014). Accounting for observation reliability when inferring extinction based on sighting records. Biodivers. Conserv. 23, 2801–2815. doi: 10.1007/s10531-014-0749-8
Jenkins, C. N., and Van Houtan, K. S. (2016). Global and regional priorities for marine biodiversity protection. Biol. Conserv. 204, 333–339. doi: 10.1016/j.biocon.2016.10.005
Jenner, C., Jenner, M., and Pirzl, R. (2008). A Study of Cetacean Distribution and Oceanography in the Scott Reef/Browse Basin Development Areas durIng the Austral Winter of 2008. Harbor, WA: Centre for Whale Research WA Inc.
Jenner, K. C. S., Jenner, M.-N. M., and Pirzl, R. (2014). “Cetaceans,” in Ecological studies of the Bonaparte Archipelago and Browse Basin, eds J. Comrie-Greig and L. Abdo (Perth, WA: INPEX Operations Australian Pty Ltd.), 401–454.
Jensen, F. H., Rocco, A., Mansur, R. M., Smith, B. D., Janik, V. M., and Madsen, P. T. (2013). Clicking in shallow rivers: short-range echolocation of Irrawaddy and Ganges river dolphins in a shallow, acoustically complex habitat. PLoS One 8:e59284. doi: 10.1371/journal.pone.0059284
Jin, J., and Yang, J. (2020). BDcleaner: a workflow for cleaning taxonomic and geographic errors in occurrence data archived in biodiversity databases. Glob. Ecol. Conserv. 21:e00852. doi: 10.1016/j.gecco.2019.e00852
Jones, E. L., McConnell, B. J., Smout, S., Hammond, P. S., Duck, C. D., Morris, C. D., et al. (2015). Patterns of space use in sympatric marine colonial predators reveal scales of spatial partitioning. Mar. Ecol. Prog. Ser. 534, 235–249. doi: 10.3354/meps11370
Joppa, L. N., Butchart, S. H. M., Hoffmann, M., Bachman, S. P., Akçakaya, H. R., Moat, J. F., et al. (2016). Impact of alternative metrics on estimates of extent of occurrence for extinction risk assessment. Conserv. Biol. 30, 362–370. doi: 10.1111/cobi.12591
Joseph, L. N., and Possingham, H. P. (2008). Grid-based monitoring methods for detecting population declines: sensitivity to spatial scale and consequences of scale correction. Biol. Conserv. 141, 1868–1875. doi: 10.1016/j.biocon.2008.05.003
Karadada, J., Karadada, L., Goonack, W., Mangolamara, G., Bunjuck, W., Karadada, L., et al. (2011). Uunguu Plants and Animals: Aboriginal Biological Knowledge From Wunambal Gaambera Country in the North-West Kimberley. Darwin, NT: Environment, the Arts and Sport.
Karczmarski, L., Cockcroft, V. G., and McLachlan, A. (2000). Habitat use and preferences of Indo-Pacific humpback dolphins Sousa chinensis in Algoa Bay. South Africa. Mar. Mammal Sci. 16, 65–79. doi: 10.1111/j.1748-7692.2000.tb00904.x
Kaschner, K., Quick, N. J., Jewell, R., Williams, R., and Harris, C. M. (2012). Global coverage of cetacean line-transect surveys: status quo, data gaps and future challenges. PLoS One 7:e44075. doi: 10.1371/journal.pone.0044075
Keith, D. A., Akçakaya, H. R., and Murray, N. J. (2017). Scaling range sizes to threats for robust predictions of risks to biodiversity. Conserv. Biol. 32, 322–332. doi: 10.1111/cobi.12988
Keith, D. A., Auld, T. D., Ooi, M. K. J., and MacKenzie, B. D. E. (2000). Sensitivity analyses of decision rules in World Conservation Union (IUCN) Red List criteria using Australian plants. Biol. Conserv. 94, 311–319. doi: 10.1016/S0006-3207(99)00194-9
Kindsvater, H. K., Dulvy, N. K., Horswill, C., Juan-Jordá, M. J., Mangel, M., and Matthiopoulos, J. (2018). Overcoming the data crisis in biodiversity conservation. Trends Ecol. Evol. 33, 676–688. doi: 10.1016/j.tree.2018.06.004
King, P. P. (1825). Narrative of a Survey of the Intertropical and Western Coasts of Australia - Performed Between the Years 1818 and 1822. Cambridge: Cambridge University Press.
Knapp, S. M., Russell, R. E., and Swihart, R. K. (2003). Setting priorities for conservation: the influence of uncertainty on species rankings of Indiana mammals. Biol. Conserv. 111, 223–234. doi: 10.1016/S0006-3207(02)00278-1
Kordi, M. N., Collins, L. B., O’Leary, M., and Stevens, A. (2016). ReefKIM: an integrated geodatabase for sustainable management of the Kimberley Reefs, North West Australia. Ocean Coast. Manag. 119, 234–243. doi: 10.1016/J.OCECOAMAN.2015.11.004
Le Breton, T. D., Zimmer, H. C., Gallagher, R. V., Cox, M., Allen, S., and Auld, T. D. (2019). Using IUCN criteria to perform rapid assessments of at-risk taxa. Biodivers. Conserv. 28, 863–883. doi: 10.1007/s10531-019-01697-9
Le Nohaïc, M., Ross, C. L., Cornwall, C. E., Comeau, S., Lowe, R., McCulloch, M. T., et al. (2017). Marine heatwave causes unprecedented regional mass bleaching of thermally resistant corals in northwestern Australia. Sci. Rep. 7:14999. doi: 10.1038/s41598-017-14794-y
Lee, C. K. F., Keith, D. A., Nicholson, E., and Murray, N. J. (2019). Redlistr: tools for the IUCN Red Lists of ecosystems and threatened species in R. Ecography 42, 1050–1055. doi: 10.1111/ecog.04143
Lee, T. E., Black, S. A., Fellous, A., Yamaguchi, N., Angelici, F. M., Al Hikmani, H., et al. (2015). Assessing uncertainty in sighting records: an example of the Barbary lion. PeerJ 3:e1224. doi: 10.7717/peerj.1224
Lee, T. E., McCarthy, M. A., Wintle, B. A., Bode, M., Roberts, D. L., and Burgman, M. A. (2014). Inferring extinctions from sighting records of variable reliability. J. Appl. Ecol. 51, 251–258. doi: 10.1111/1365-2664.12144
Lin, Y.-P., Lin, W.-C., Lien, W.-Y., Anthony, J., and Petway, J. (2017). Identifying reliable opportunistic data for species distribution modeling: a benchmark data optimization approach. Environments 4:81. doi: 10.3390/environments4040081
Lodi, L., and Tardin, R. (2018). Citizen science contributes to the understanding of the occurrence and distribution of cetaceans in southeastern Brazil – a case study. Ocean Coast. Manag. 158, 45–55. doi: 10.1016/j.ocecoaman.2018.03.029
MacLeod, C. D. (2009). Global climate change, range changes and potential implications for the conservation of marine cetaceans: a review and synthesis. Endanger. Species Res. 7, 125–136. doi: 10.3354/esr00197
Maes, D., Isaac, N. J. B., Harrower, C. A., Collen, B., van Strien, A. J., and Roy, D. B. (2015). The use of opportunistic data for IUCN Red List assessments. Biol. J. Linn. Soc. 115, 690–706. doi: 10.1111/bij.12530
Maes, D., Vanreusel, W., Jacobs, I., Berwaerts, K., and Van Dyck, H. (2012). Applying IUCN Red List criteria at a small regional level: a test case with butterflies in Flanders (north Belgium). Biol. Conserv. 145, 258–266. doi: 10.1016/j.biocon.2011.11.021
Mann, J. (2000). Female reproductive success in bottlenose dolphins (Tursiops sp.): life history, habitat, provisioning, and group-size effects. Behav. Ecol. 11, 210–219. doi: 10.1093/beheco/11.2.210
Marley, S. A., Kent, C. P. S., Erbe, C., and Thiele, D. (2017). A tale of two soundscapes: comparing the acoustic characteristics of urban versus pristine coastal dolphin habitats in Western Australia. Acoust. Aust. 45, 159–178. doi: 10.1007/s40857-017-0106-7
Martin, T. G., Burgman, M. A., Fidler, F., Kuhnert, P. M., Low-Choy, S., Mcbride, M., et al. (2012). Eliciting expert knowledge in conservation science. Conserv. Biol. 26, 29–38. doi: 10.1111/j.1523-1739.2011.01806.x
McCluskey, S. M., Bejder, L., and Loneragan, N. R. (2016). Dolphin prey availability and calorific value in an estuarine and coastal environment. Front. Mar. Sci. 3:30. doi: 10.3389/fmars.2016.00030
McKelvey, K. S., Aubry, K. B., and Schwartz, M. K. (2008). Using anecdotal occurrence data for rare or elusive Species: the illusion of reality and a call for evidentiary standards. Bioscience 58, 549–555. doi: 10.1641/b580611
Meager, J. J., and Sumpton, W. D. (2016). Bycatch and strandings programs as ecological indicators for data-limited cetaceans. Ecol. Indic. 60, 987–995. doi: 10.1016/j.ecolind.2015.08.052
Mendes, S., Turrell, W., Lütkebohle, T., and Thompson, P. (2002). Influence of the tidal cycle and a tidal intrusion front on the spatio-temporal distribution of coastal bottlenose dolphins. Mar. Ecol. Prog. Ser. 239, 221–229. doi: 10.3354/meps239221
Miller, D. A. W., Pacifici, K., Sanderlin, J. S., and Reich, B. J. (2019). The recent past and promising future for data integration methods to estimate species’ distributions. Methods Ecol. Evol. 10, 22–37. doi: 10.1111/2041-210X.13110
Milner-Gulland, E. J., Kreuzberg-Mukhina, E., Grebot, B., Ling, S., Bykova, E., Abdusalamov, I., et al. (2006). Application of IUCN Red Listing criteria at the regional and national levels: a case study from Central Asia. Biodivers. Conserv. 15, 1873–1886. doi: 10.1007/s10531-005-4304-5
Minton, G., Peter, C. I., Poh, A., Ngeian, J., Braulik, G., Hammond, P. S., et al. (2013). Population estimates and distribution patterns of Irrawaddy dolphins (Orcaella brevirostris) and Indo-Pacific finless porpoises (Neophocaena phocaenoides) in the Kuching Bay, Sarawak. Raffles Bull. Zool. 61, 877–888.
Moat, J., Bachman, S. P., Field, R., and Boyd, D. S. (2018). Refining area of occupancy to address the modifiable areal unit problem in ecology and conservation. Conserv. Biol. 32, 1278–1289. doi: 10.1111/cobi.13139
Molony, B. W., Newman, S. J., Joll, L., Lenanton, R. C. J., and Wise, B. (2011). Are Western Australian waters the least productive waters for finfish across two oceans? a review with a focus on finfish resources in the Kimberley region and North Coast Bioregion. J. R. Soc. West. Aust. 94, 323–332.
Moore, C. H., Radford, B. T., Possingham, H. P., Heyward, A. J., Stewart, R. R., Watts, M. E., et al. (2016). Improving spatial prioritisation for remote marine regions: optimising biodiversity conservation and sustainable development trade-offs. Sci. Rep. 6:32029. doi: 10.1038/srep32029
Mounce, R., Rivers, M., Sharrock, S., Smith, P., and Brockington, S. (2018). Comparing and contrasting threat assessments of plant species at the global and sub-global level. Biodivers. Conserv. 27, 907–930. doi: 10.1007/s10531-017-1472-z
Munksgaard, N. C., Hutley, L. B., Metcalfe, K. N., Padovan, A. C., Palmer, C., and Gibb, K. S. (2019). Environmental challenges in a near-pristine mangrove estuary facing rapid urban and industrial development: darwin Harbour, Northern Australia. Reg. Stud. Mar. Sci. 25:100438. doi: 10.1016/j.rsma.2018.11.001
Newton, A. C. (2010). Use of a bayesian network for red listing under uncertainty. Environ. Model. Softw. 25, 15–23. doi: 10.1016/j.envsoft.2009.07.016
Nifong, J. C., and Silliman, B. (2017). Abiotic factors influence the dynamics of marine habitat use by a highly mobile “freshwater” top predator. Hydrobiologia 802, 155–174. doi: 10.1007/s10750-017-3255-7
Nourani, E., Kaboli, M., Farhoodinia, M., and Collen, B. (2017). National assessment of threatened species using sparse data: IUCN Red List classification of Anatidae in Iran. Anim. Conserv. 20, 42–50. doi: 10.1111/acv.12282
Ocampo-Peñuela, N., Jenkins, C. N., Vijay, V., Li, B. V., and Pimm, S. L. (2016). Incorporating explicit geospatial data shows more species at risk of extinction than the current Red List. Sci. Adv. 2:e1601367. doi: 10.1126/sciadv.1601367
Pagel, J., Anderson, B. J., O’Hara, R. B., Cramer, W., Fox, R., Jeltsch, F., et al. (2014). Quantifying range-wide variation in population trends from local abundance surveys and widespread opportunistic occurrence records. Methods Ecol. Evol. 5, 751–760. doi: 10.1111/2041-210X.12221
Palacio, R. D., Negret, P. J., Velásquez-Tibatá, J., and Jacobson, A. P. (2020). A data-driven geospatial workflow to improve mapping species distributions and assessing extinction risk under the IUCN Red List. bioRxiv [Preprint] doi: 10.1101/2020.04.27.064477
Palmer, C., Brooks, L., Fegan, M., and Griffiths, T. (2017). Conservation Status of Coastal Dolphins in the Northern Territory - Final Report. Darwin, NY: Department of Environment and Natural Resources.
Palmer, C., Brooks, L., Parra, G. J., Rogers, T., Glasgow, D., and Woinarski, J. C. Z. (2014a). Estimates of abundance and apparent survival of coastal dolphins in Port Essington harbour. Northern Territory, Australia. Wildl. Res. 41, 35–45. doi: 10.1071/WR14031
Palmer, C., Parra, G. J., Rogers, T. T., and Woinarski, J. (2014b). Collation and review of sightings and distribution of three coastal dolphn species in waters of the Northern Territory. Australia. Pacific Conserv. Biol. 20, 116–125. doi: 10.1071/pc140116
Palmer, C., and Peterson, A. (2013). First report of a lacaziosis-like disease (LLD) observed in the Australian Snubfin Dolphin (Orcaella heinsohni) in Darwin Harbour, Northern Territory, Australia. North. Territ. Nat. 25, 3–6.
Parra, G., Azuma, C., Preen, A., Corkeron, P., and Marsh, H. (2002). Distribution of Irrawaddy dolphins, Orcaella brevirostris, in Australian waters. Raffles Bull. Zool. 10, 141–154.
Parra, G., Cagnazzi, D., and Beasley, I. (2017). Orcaella heinsohni (errata version published in 2018). IUCN Red List Threat. Species 2017 e.T136315A123793740. Available online at: https://www.iucnredlist.org/species/136315/123793740 (accessed March 20, 2020).
Parra, G. J. (2006). Resource partitioning in sympatric delphinids: space use and habitat preferences of Australian snubfin and Indo-Pacific humpback dolphins. J. Anim. Ecol. 75, 862–874. doi: 10.1111/j.1365-2656.2006.01104.x
Parra, G. J., and Cagnazzi, D. (2016). Conservation status of the Australian humpback dolphin (Sousa sahulensis) using the IUCN Red List criteria. Adv. Mar. Biol. 73, 157–192. doi: 10.1016/bs.amb.2015.07.006
Parra, G. J., Corkeron, P. J., and Arnold, P. (2011). Grouping and fission–fusion dynamics in Australian snubfin and Indo-Pacific humpback dolphins. Anim. Behav. 82, 1423–1433. doi: 10.1016/j.anbehav.2011.09.027
Parra, G. J., Corkeron, P. J., and Marsh, H. (2006a). Population sizes, site fidelity and residence patterns of Australian snubfin and Indo-Pacific humpback dolphins: implications for conservation. Biol. Conserv. 129, 167–180. doi: 10.1016/j.biocon.2005.10.031
Parra, G. J., Schick, R., and Corkeron, J. (2006b). Spatial distribution and environmental correlates of Australian snubfin and Indo-Pacific humpback dolphins. Ecography 29, 396–406. doi: 10.1111/j.2006.0906-7590.04411.x
Parra, G. J., and Jedensjö, M. (2014). Stomach contents of Australian snubfin (Orcaella heinsohni) and Indo-Pacific humpback dolphins (Sousa chinensis). Mar. Mammal Sci. 30, 1184–1198. doi: 10.1111/mms.12088
Parsons, E. C. M., Baulch, S., Bechshoft, T., Bellazzi, G., Bouchet, P. J., Cosentino, A. M., et al. (2015). Key research questions of global importance for cetacean conservation. Endanger. Species Res. 27, 113–118. doi: 10.3354/esr00655
Payne, J. L., and Finnegan, S. (2007). The effect of geographic range on extinction risk during background and mass extinction. Proc. Natl. Acad. Sci. U.S.A. 104, 10506–10511. doi: 10.1073/pnas.0701257104
Pelayo-Villamil, P., Guisande, C., Vari, R. P., Manjarrés-Hernández, A., García-Roselló, E., González-Dacosta, J., et al. (2015). Global diversity patterns of freshwater fishes - potential victims of their own success. Divers. Distrib. 21, 345–356. doi: 10.1111/ddi.12271
Pennay, M., Law, B., and Lunney, D. (2011). ““Review of the distribution and status of the bat fauna of New South Wales and the Australian Capital Territory,” in The Biology and Conservation of Australasian Bats, eds B. Law, P. Eby, D. Lunney, and L. Lumsden (Mosman: Royal Zoological Society of New South Wales), 226–257. doi: 10.7882/fs.2011.025
Possingham, H. P., Andelman, S. J., Burgman, M. A., Medellín, R. A., Master, L. L., and Keith, D. A. (2002). Limits to the use of threatened species lists. Trends Ecol. Evol. 17, 503–507. doi: 10.1016/S0169-5347(02)02614-9
Raudino, H., Douglas, C., D’Cruz, E., and Waples, K. (2019). Snubfin Dolphin Census in Yawuru Nagulagan/ Roebuck Bay. PerthWA: Western Australian Department of Biodiversity.
Raudino, H. C., Douglas, C. R., and Waples, K. A. (2018). How many dolphins live near a coastal development? Reg. Stud. Mar. Sci. 19, 25–32. doi: 10.1016/j.rsma.2018.03.004
Regan, H. M., Colyvan, M., and Burgman, M. A. (2002). A taxonomy and treatment of uncertainty for ecology and conservation biology. Ecol. Appl. 12, 618–628. doi: 10.2307/3060967
Rist, P., Rassip, W., Yunupingu, D., Wearne, J., Gould, J., Dulfer-Hyams, M., et al. (2019). Indigenous protected areas in Sea Country: Indigenous-driven collaborative marine protected areas in Australia. Aquat. Conserv. Mar. Freshw. Ecosyst. 29, 138–151. doi: 10.1002/aqc.3052
Roberts, D. L., Elphick, C. S., and Reed, J. M. (2010). Identifying anomalous reports of putatively extinct species and why it matters. Conserv. Biol. 24, 189–196. doi: 10.1111/j.1523-1739.2009.01292.x
Robertson, M. P., Visser, V., and Hui, C. (2016). Biogeo: an R package for assessing and improving data quality of occurrence record datasets. Ecography 39, 394–401. doi: 10.1111/ecog.02118
Robinson, O. J., Ruiz-Gutierrez, V., Reynolds, M. D., Golet, G. H., Strimas-Mackey, M., and Fink, D. (2020). Integrating citizen science data with expert surveys increases accuracy and spatial extent of species distribution models. Divers. Distrib. 26, 976–986. doi: 10.1111/ddi.13068
Rodrigues, A. S. L., Pilgrim, J. D., Lamoreux, J. F., Hoffmann, M., and Brooks, T. M. (2006). The value of the IUCN Red List for conservation. Trends Ecol. Evol. 21, 71–76. doi: 10.1016/j.tree.2005.10.010
Rossi-Santos, M. R., Wedekin, L. L., and Monteiro-Filho, E. L. A. (2010). Habitat use of the Guiana dolphin, Sotalia guianensis (Cetacea, Delphinidae), in the Caravelas River Estuary, eastern Brazil. Lat. Am. J. Aquat. Mamm. 8, 111–116. doi: 10.5597/lajam00159
Rueda-Cediel, P., Anderson, K. E., Regan, T. J., and Regan, H. M. (2018). Effects of uncertainty and variability on population declines and IUCN Red List classifications. Conserv. Biol. 32, 916–925. doi: 10.1111/cobi.13081
Saltré, F., Brook, B. W., Rodríguez-Rey, M., Cooper, A., Johnson, C. N., Turney, C. S. M., et al. (2015). Uncertainties in dating constrain model choice for inferring extinction time from fossil records. Quat. Sci. Rev. 112, 128–137. doi: 10.1016/j.quascirev.2015.01.022
Schofield, G., Scott, R., Dimadi, A., Fossette, S., Katselidis, K. A., Koutsoubas, D., et al. (2013). Evidence-based marine protected area planning for a highly mobile endangered marine vertebrate. Biol. Conserv. 161, 101–109. doi: 10.1016/j.biocon.2013.03.004
Scott, R., Griffiths, C., and Robinson, T. (2012). Patterns of endemicity and range restriction among southern African coastal marine invertebrates. African J. Mar. Sci. 34, 341–347. doi: 10.2989/1814232X.2012.725284
Simpfendorfer, C. A., Goodreid, A. B., and Mcauley, R. B. (2001). Size, sex and geographic variation in the diet of the tiger shark, Galeocerdo cuvier, from Western Australian waters. Environ. Biol. Fishes 61, 37–46. doi: 10.1023/A:1011021710183
Smith, B. D., and Reeves, R. R. (2000). “Survey methods for population assessment of Asian river dolphins,” in Biology and Conservation of Freshwater Cetaceans in Asia, eds R. R. Reeves, B. D. Smith, B. D. Smith, and T. Kasuya (Gland: IUCN), 97–115.
Smith, F., Allen, S. J., Bejder, L., and Brown, A. M. (2018). Shark bite injuries on three inshore dolphin species in tropical northwestern Australia. Mar. Mammal Sci. 34, 87–99. doi: 10.1111/mms.12435
Soberón, J., and Peterson, A. T. (2004). Biodiversity informatics: managing and applying primary biodiversity data. Philos. Trans. R. Soc. B Biol. Sci. 359, 689–698. doi: 10.1098/rstb.2003.1439
Sobtzick, S., Hodgson, A., and Marsh, H. (2013). Dugong Aerial Survey observer Training Course Handbook. Townsville, QLD: James Cook University.
Solow, A., Smith, W., Burgman, M., Rout, T., Wintle, B., and Roberts, D. (2012). Uncertain sightings and the extinction of the ivory-billed woodpecker. Conserv. Biol. 26, 180–184. doi: 10.1111/j.1523-1739.2011.01743.x
Solow, A. R., and Beet, A. R. (2014). On uncertain sightings and inference about extinction. Conserv. Biol. 28, 1119–1123. doi: 10.1111/cobi.12309
Soto, A. B., Cagnazzi, D., Everingham, Y., Parra, G. J., Noad, M., and Marsh, H. (2013). Acoustic alarms elicit only subtle responses in the behaviour of tropical coastal dolphins in Queensland. Australia. Endanger. Species Res. 20, 271–282. doi: 10.3354/esr00495
Sousa-Silva, R., Alves, P., Honrado, J., and Lomba, A. (2014). Improving the assessment and reporting on rare and endangered species through species distribution models. Glob. Ecol. Conserv. 2, 226–237. doi: 10.1016/j.gecco.2014.09.011
Sprogis, K. R., Raudino, H. C., Rankin, R., Macleod, C. D., and Bejder, L. (2016). Home range size of adult Indo-Pacific bottlenose dolphins (Tursiops aduncus) in a coastal and estuarine system is habitat and sex-specific. Mar. Mammal Sci. 32, 287–308. doi: 10.1111/mms.12260
Steenweg, R., Hebblewhite, M., Whittington, J., Lukacs, P., and McKelvey, K. (2018). Sampling scales define occupancy and underlying occupancy–abundance relationships in animals. Ecology 99, 172–183. doi: 10.1002/ecy.2054
Strickland-Munro, J., Kobryn, H., Moore, S. A., and Brown, G. (2016). Valuing the wild, remote and beautiful: using public participation gis to inform tourism planning in the Kimberley, Western Australia. Int. J. Sustain. Dev. Plan. 11, 355–364. doi: 10.2495/SDP-V11-N3-355-364
Strona, G., Galli, P., Montano, S., Seveso, D., and Fattorini, S. (2012). Global-scale relationships between colonization ability and range size in marine and freshwater fish. PLoS One 7:e49465. doi: 10.1371/journal.pone.0049465
Sveegaard, S., Teilmann, J., Tougaard, J., Dietz, R., Mouritsen, K. N., Desportes, G., et al. (2011). High-density areas for harbor porpoises (Phocoena phocoena) identified by satellite tracking. Mar. Mammal Sci. 27, 230–246. doi: 10.1111/j.1748-7692.2010.00379.x
Syfert, M. M., Joppa, L., Smith, M. J., Coomes, D. A., Bachman, S. P., and Brummitt, N. A. (2014). Using species distribution models to inform IUCN Red List assessments. Biol. Conserv. 177, 174–184. doi: 10.1016/j.biocon.2014.06.012
Tancell, C., Phillips, R. A., Xavier, J. C., Tarling, G. A., and Sutherland, W. J. (2012). Comparison of methods for determining key marine areas from tracking data. Mar. Biol. 160, 15–26. doi: 10.1007/s00227-012-2050-2
Tarjan, L. M., and Tinker, M. T. (2016). Permissible home range estimation (PHRE) in restricted habitats: a new algorithm and an evaluation for sea otters. PLoS One 11:e0150547. doi: 10.1371/journal.pone.0150547
Tenan, S., Pedrini, P., Bragalanti, N., Groff, C., and Sutherland, C. S. (2016). Investigating the potential of opportunistic sighting data to inform wildlife conservation strategies. bioRxiv [Preprint]. doi: 10.1101/075945
Thiele, D. (2010). Collision Course: Snubfin Dolphin Injuries in Roebuck Bay. Broome: WWF-Australia. Report prepared for WWF-Australia.
Thums, M., Jenner, C., Waples, K., Salgado-Kent, C., and Meekan, M. (2018). Humpback Whale use of the Kimberley: Understanding and Monitoring Spatial Distribution. Perth, WA: Western Australian Marine Science Institution. Report of Project 1.2.1 prepared for the Kimberley Marine Research Program.
Tiago, P., Pereira, H. M., and Capinha, C. (2017). Using citizen science data to estimate climatic niches and species distributions. Basic Appl. Ecol. 20, 75–85. doi: 10.1016/j.baae.2017.04.001
Torney, C. J., Lloyd-Jones, D. J., Chevallier, M., Moyer, D. C., Maliti, H. T., Mwita, M., et al. (2019). A comparison of deep learning and citizen science techniques for counting wildlife in aerial survey images. Methods Ecol. Evol. 10, 779–787. doi: 10.1111/2041-210X.13165
Travers, M. J., Potter, I. C., Clarke, K. R., and Newman, S. J. (2012). Relationships between latitude and environmental conditions and the species richness, abundance and composition of tropical fish assemblages over soft substrata. Mar. Ecol. Prog. Ser. 446, 221–241. doi: 10.3354/meps09501
Tulloch, V., Pirotta, V., Grech, A., Crocetti, S., Double, M., How, J., et al. (2020). Long-term trends and a risk analysis of cetacean entanglements and bycatch in fisheries gear in Australian waters. Biodivers. Conserv. 29, 251–282. doi: 10.1007/s10531-019-01881-x
van der Velde, T., Milton, D. A., Lawson, T. J., Wilcox, C., Lansdell, M., Davis, G., et al. (2017). Comparison of marine debris data collected by researchers and citizen scientists: is citizen science data worth the effort? Biol. Conserv. 208, 127–138. doi: 10.1016/j.biocon.2016.05.025
Viddi, F. A., Harcourt, R. G., and Hucke-Gaete, R. (2016). Identifying key habitats for the conservation of Chilean dolphins in the fjords of southern Chile. Aquat. Conserv. Mar. Freshw. Ecosyst. 26, 506–516. doi: 10.1002/aqc.2553
Wallace, B. P., DiMatteo, A. D., Hurley, B. J., Finkbeiner, E. M., Bolten, A. B., Chaloupka, M. Y., et al. (2010). Regional management units for marine turtles: a novel framework for prioritizing conservation and research across multiple scales. PLoS One 5:e0015465. doi: 10.1371/journal.pone.0015465
Wand, M. (2015). KernSmooth: Functions for Kernel Smoothing Supporting Wand & Jones (1995). R Package Version 2.23-17. Available online at: https://cran.r-project.org/package=KernSmooth (accessed October 29, 2020).
Waples, K., and Raudino, H. (2018). Setting a course for marine mammal research in Western Australia. Pacific Conserv. Biol. 24, 289–303. doi: 10.1071/PC18014
Wells, R. S., Scott, M. D., and Irvine, A. B. (1987). “The social structure of free-ranging bottlenose dolphins,” in Current Mammalogy, ed. H. Genoways (Berlin: Springer), 247–305. doi: 10.1007/978-1-4757-9909-5_7
Wild, S., Krützen, M., Rankin, R. W., Hoppitt, W. J. E., Gerber, L., and Allen, S. J. (2019). Long-term decline in survival and reproduction of dolphins following a marine heatwave. Curr. Biol. 29, R239–R240. doi: 10.1016/j.cub.2019.02.047
Willis, F., Moat, J., and Paton, A. (2003). Defining a role for herbarium data in Red List assessments: a case study of Plectranthus from eastern and southern tropical Africa. Biodivers. Conserv. 12, 1537–1552. doi: 10.1023/A:1023679329093
Wilson, B. (2014). “The kimberley region,” in Ecological Studies of the Bonaparte Archipelago and Browse Basin, ed. A. L. Comrie-Greig (Perth, WA: INPEX Operations Australian Pty Ltd), 7–8.
Wilson, H. B., Kendall, B. E., and Possingham, H. P. (2011). Variability in population abundance and the classification of extinction risk. Conserv. Biol. 25, 747–757. doi: 10.1111/j.1523-1739.2011.01671.x
Wood, J. S., Moretzsohn, F., and Gibeaut, J. (2015). Extending marine species distribution maps using non-traditional sources. Biodivers. Data J. 3:4900. doi: 10.3897/BDJ.3.e4900
Wood, M., and Mills, D. (2008). A Turning of the Tide: Science for Decisions in the Kimberley-Browse Marine Region. Perth, WA: Western Australian Marine Science Institution.
Woodward, E., Hill, R., Harkness, P., and Archer, R. (2020). Our Knowledge Our Way in Caring for Country: Indigenous-led Approaches to Strengthening and Sharing our Knowledge for Land and Sea Management. Brinkin NT: NAILSMA and CSIRO. Best Practice Guidelines from Australian experiences.
Ziembicki, M. R., Woinarski, J. C. Z., and Mackey, B. (2013). Evaluating the status of species using Indigenous knowledge: novel evidence for major native mammal declines in northern Australia. Biol. Conserv. 157, 78–92. doi: 10.1016/j.biocon.2012.07.004
Keywords: area of occupancy (AOO), extent of occurrence (EOO), citizen science (CS), bootstrap, data integration, geographic range, cetacean marine mammal
Citation: Bouchet PJ, Thiele D, Marley SA, Waples K, Weisenberger F, Balanggarra Rangers, Bardi Jawi Rangers, Dambimangari Rangers, Nyamba Buru Yawuru Rangers, Nyul Nyul Rangers, Uunguu Rangers and Raudino H (2021) Regional Assessment of the Conservation Status of Snubfin Dolphins (Orcaella heinsohni) in the Kimberley Region, Western Australia. Front. Mar. Sci. 7:614852. doi: 10.3389/fmars.2020.614852
Received: 07 October 2020; Accepted: 18 December 2020;
Published: 21 January 2021.
Edited by:
Jeremy Kiszka, Florida International University, United StatesReviewed by:
Simon J. Allen, University of Bristol, United KingdomRebecca Ruth McIntosh, Phillip Island Nature Parks, Australia
Copyright © 2021 Bouchet, Thiele, Marley, Waples, Weisenberger, Balanggarra Rangers, Bardi Jawi Rangers, Dambimangari Rangers, Nyamba Buru Yawuru Rangers, Nyul Nyul Rangers, Uunguu Rangers and Raudino. This is an open-access article distributed under the terms of the Creative Commons Attribution License (CC BY). The use, distribution or reproduction in other forums is permitted, provided the original author(s) and the copyright owner(s) are credited and that the original publication in this journal is cited, in accordance with accepted academic practice. No use, distribution or reproduction is permitted which does not comply with these terms.
*Correspondence: Phil J. Bouchet, cGIyODJAc3QtYW5kcmV3cy5hYy51aw==; cGpib3VjaGV0QGdtYWlsLmNvbQ==
†Present address: Frank Weisenberger, Frank Weisenberger Consulting, Melbourne, VIC, Australia
‡Balanggarra Rangers, Bardi Jawi Rangers, Dambimangari Rangers, Nyamba Buru Yawuru Rangers, Nyul Nyul Rangers, Uunguu Rangers Collaborative authors are included in the acknowledgments