- 1Institute for Chemistry and Biology of the Marine Environment (ICBM) at the University of Oldenburg, Wilhelmshaven, Germany
- 2PSL Research University, USR EPHE-UPVD-CNRS 3278, CRIOBE, Université de Perpignan, Perpignan, France
- 3Labex Corail, Moorea, French Polynesia
- 4Helmholtz Institute for Functional Marine Biodiversity at the University of Oldenburg (HIFMB), Oldenburg, Germany
The high diversity of marine natural products represents promising opportunities for drug discovery, an important area in marine biotechnology. Within this context, high-throughput techniques such as metabolomics are extremely useful in unveiling unexplored chemical diversity at much faster rates than classical bioassay-guided approaches. Metabolomics approaches enable studying large sets of metabolites, even if they are produced at low concentrations. Although, metabolite identification remains the main metabolomics bottleneck, bioinformatic tools such as molecular networks can lead to the annotation of unknown metabolites and discovery of new compounds. A metabolomic approach in drug discovery has two major advantages: it enables analyses of multiple samples, allowing fast dereplication of already known compounds and provides a unique opportunity to relate metabolite profiles to organisms’ biology. Understanding the ecological and biological factors behind a certain metabolite production can be extremely useful in enhancing compound yields, optimizing compound extraction or in selecting bioactive compounds. Metazoan-associated microbiota are often responsible for metabolite synthesis, however, classical approaches only allow studying metabolites produced from cultivatable microbiota, which often differ from the compounds produced within the host. Therefore, coupling holobiome metabolomics with microbiome analysis can bring new insights to the role of microbiota in compound production. The ultimate potential of metabolomics is its coupling with other “omics” (i.e., transcriptomics and metagenomics). Although, such approaches are still challenging, especially in non-model species where genomes have not been annotated, this innovative approach is extremely valuable in elucidating gene clusters associated with biosynthetic pathways and will certainly become increasingly important in marine drug discovery.
Introduction
Natural products (i.e., compounds produced by living organisms) are key to drug development, and have proved especially useful in the development of anticancer and anti-infective agents (Rodrigues et al., 2016; Liu et al., 2019). The marine environment, which harbors a large and yet highly unexplored biodiversity, is an extremely rich source of novel and structurally unique compounds with antibacterial, antifungal, antiviral, antiparasitic, antitumor, anti-inflammatory, antioxidant and immunomodulatory activities (Abdelmohsen et al., 2017; Carroll et al., 2020; Mayer et al., 2020). Some marine organisms such as sponges are amongst the most prolific sources of natural products, suggesting that the marine environment is a reservoir of natural products with high relevance for marine biotechnology and drug discovery (including against resistant pathogens) (Thakur and Müller, 2004, Abdelmohsen et al., 2017; Liu et al., 2019). The development of high-throughput techniques such as genomics and metabolomics (i.e., the study of all small molecules, <2,000 Da, in an organism) promises to revolutionize natural product discovery, which according to some estimates, could outpace antibiotic discovery at its peak in the 1950s (Fortman and Mukhopadhyay, 2016). The use of these techniques, does not only offer an increase in the speed of natural product discovery and decrease in the re-discovery rate (Wolfender et al., 2019; van der Hooft et al., 2020), but provides a wide-array of unprecedented opportunities to discover unexplored chemical diversity and elucidate the biological and molecular mechanisms involved in metabolites production (e.g., Cantrell et al., 2019; Mohanty et al., 2020). Here, we provide a synthetic overview of the advantages of using metabolomic approaches in marine natural product discovery and marine biotechnology. Metabolomics is a scientific field at the interface of different disciplines (chemistry, bioinformatics, ecology, microbiology, and systems biology); therefore, this mini-review aims to illustrate how the multidisciplinarity of metabolomics is a key asset for the advancement of marine natural product discovery.
Speeding Up Molecule Identification
Bioassay-guided fractionation (i.e., extract fractionation and purification based on targeted activities) has been classically used for the discovery of new natural products (Kildgaard et al., 2017; El Menif et al., 2019). However, this approach is not only time-consuming, but often results in the isolation of previously known compounds, since molecule dereplication is performed at the end of the workflow (Herath et al., 2019; Pereira et al., 2019). In recent years, metabolomics has arisen as a new tool to circumvent some of the bioassay-guided fractionation limitations and has shown its effectiveness in the discovery of new active compounds from marine sources (Einarsdottir et al., 2017; Naman et al., 2017; Stuart et al., 2020). Metabolomics approaches are based on the simultaneous screening (using different spectrometry techniques such as LC-MS, GC-MS, and NMR spectroscopy) of a large number of samples and the use of numerical analyses and databases to detect patterns and relevant metabolites, according to a previously defined biological question (Gertsman and Barshop, 2018; Wolfender et al., 2019). For example, the study of shallow water hydrothermal vent sponges by mass spectrometry-based metabolomics combined with cytotoxicity bioassays led to the selection of three sponges harboring a unique chemical diversity and the putative identification of the minor original compound cyclostellettamine P (Einarsdottir et al., 2017). By combining direct analysis of complex extracts with machine learning (i.e., the use of computer algorithms that continuously improve from experience for example to find patterns), metabolomics allows studying complex chemical mixtures, including minor compounds, whilst accelerating the dereplication process and decreasing the re-discovery rate (Wolfender et al., 2019; Liebal et al., 2020; van der Hooft et al., 2020). Combining large-scale biological screening with untargeted metabolomics of multiple organisms can lead to the fast identification of specimens producing unique compounds and their prioritization in further investigations (Luzzatto-Knaan et al., 2017; Réveillon et al., 2019).
Metabolomic approaches require, however, method standardization (same extraction and analytical conditions) between the different samples analyzed, which can lead to metabolite losses (Gertsman and Barshop, 2018). Data processing and curation which can be done using several software (e.g., R, MetGem, OpenMS, MZmine 2) and online platforms (e.g., Workflow4Metabolomics1, XCMS Online – xcmsonline.scripps.edu, Metaboanalyst2), may be laborious and the identification of marine compounds remains challenging since marine databases are still scarce compared to terrestrial ones (Barbosa and Roque, 2019). The development of bioinformatics tools, such as mass spectrometry-based molecular networking, are emerging as approaches that could fill some of these gaps and facilitate dereplication (Ramos et al., 2019; Aron et al., 2020, Figure 1). In molecular networking (such as implemented by the GNPS: Global Natural Products Social Molecular Networking3), algorithms detect and group metabolites based on the similarity of their fragments (mass ion spectra from tandem mass spectrometry), allowing the identification of candidate molecule clusters (i.e., groups of molecules grouped together) (Wang et al., 2016). Then molecular networks can be annotated by identifying matching cluster members in existing databases or by using tools such as Unsupervised Substructure Discovery (MS2LDA4). For example, using this approach, two smenamide-related clusters containing unknown analogs with anti-proliferative activity against cancer cells were identified in Caribbean sponges (Caso et al., 2019). Then, a targeted isolation can be performed to confirm the activity and the structure of the bioactive compounds using NMR experiments (Li et al., 2018). Using this approach, new pyrrole derived alkaloids were isolated from a marine bacterium and were identified with the analysis of their HRMS, MS/MS, and NMR data (Zhang et al., 2019). Molecular networking is also a powerful tool in minor natural product dereplication (Cantrell et al., 2019). For example, sarasinosides and melophlins were identified using molecular networking tools, despite only small amounts of sponges being available (Mohanty et al., 2020). Finally, a recent tool called “bioactivity-based molecular networking” allows to accelerate the identification of bioactive candidate molecules by integrating data on the activity of extracts/fractions directly into the network via a bioactivity score (Nothias et al., 2018). The use of such a tool led to the isolation of new decalinoylspirotetramic acid derivatives (pyrenosetis A–C) from a seaweed-derived fungus (Fan et al., 2019).
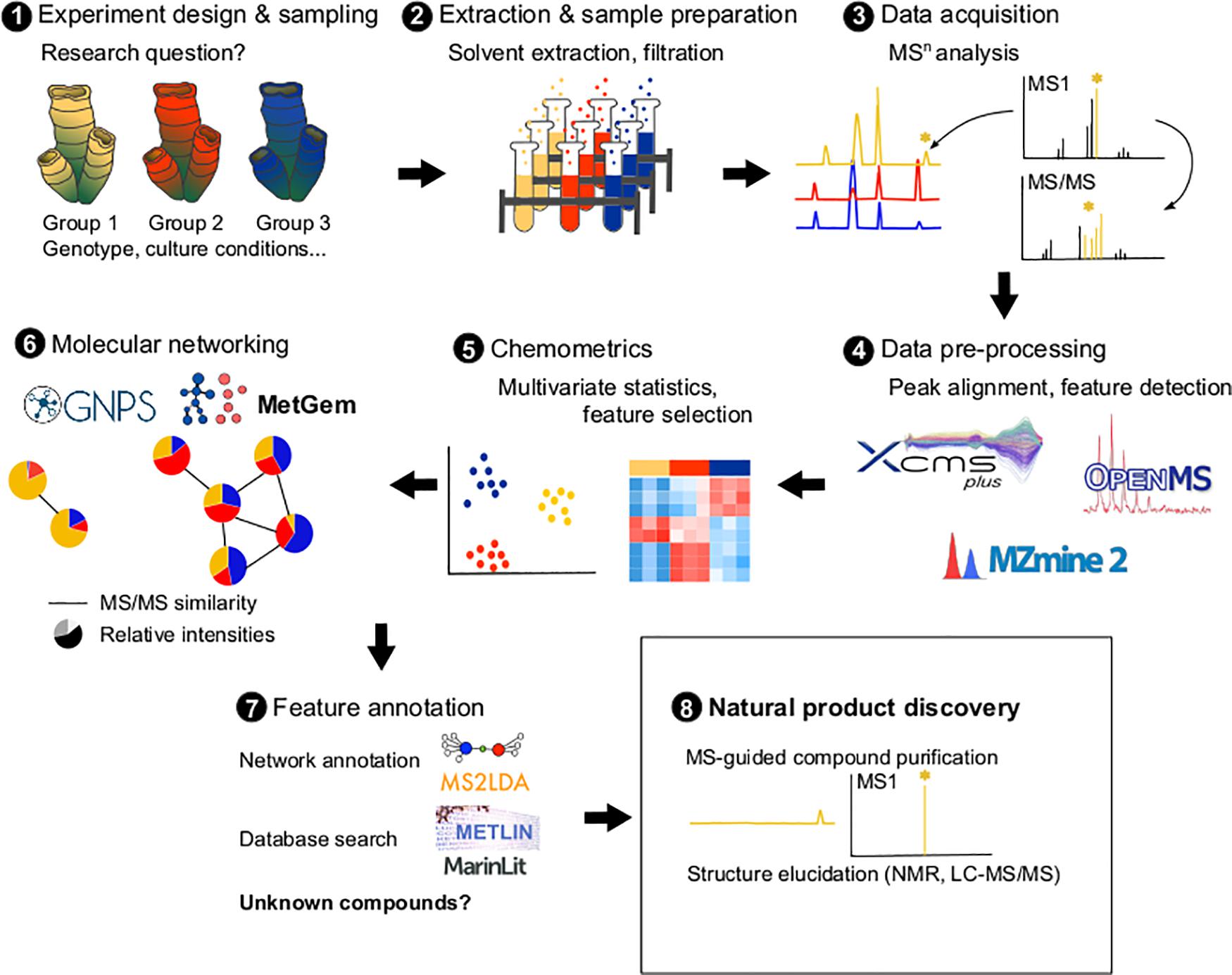
Figure 1. Metabolomics pipeline illustrating the different steps: experiment design according to the biological research question (1), sample extraction (2), MSn analyses (3), pre-processing the raw MSn data using softwares such as XCMS, mzMine2 or OpenMS (4), data numerical analyses to identify patterns and select interesting metabolites (5), molecular networking analysis using tools such as GNPS or MetGem (6), network and metabolite annotation using tools such as MS2LDA or databases (Metlin, MarinLit) (7), and purification and elucidation of new natural products (8).
Linking Chemical Diversity and Ecology
Secondary metabolites are responsible for a diverse array of ecological functions. While most ecological studies focusing on the function of known natural products investigate defense against predators, allelopathy, or interactions with microbes (Rohde et al., 2015; Puglisi and Becerro, 2018), metabolites are often involved in multiple additional life history traits such as reproduction, recruitment (Hay, 2009; Tebben et al., 2015), or resistance against abiotic stressors (Pavia and Brock, 2000). Because of the diverse array of ecological functions, the biosynthesis of metabolites is highly variable and strongly affected by environmental and biological conditions. Thus, biotechnological exploitation of natural products faces the challenge that metabolic variations occur at inter- and intraspecific scales, vary with time and space and as such make it difficult to interpret multiparametric responses. Before the emergence of metabolomics approaches, chemical ecologists relied on targeting few well-characterized compounds to identify metabolites’ ecological functions. For example, a study that investigated the spatial variation of six major metabolites of the sponge Stylissa massa showed high variation in metabolite concentrations, but most of the variability could not be assigned to specific factors (Rohde et al., 2012). The development of metabolomics provides significant opportunities to investigate patterns in metabolite variation, expanding the focus from few to hundreds of metabolites, which could assist in unraveling the multiple pathways affected by environmental factors (Figure 1). A study of the metabolome of the red algae Gracilaria vermiculophylla in response to herbivory revealed 19 upregulated metabolites with some compounds increasing more than 100-fold in concentration, illustrating the broad spectrum of metabolites that are relevant in single interactions (Nylund et al., 2011). The study of the metabolome of the brown algae Lobophora rosacea showed that out of 262 features identified by LC-MS (i.e., peaks with specific retention time and mass to charge ratio m/z), the production of 53 features changed under different pH conditions (Gaubert et al., 2020).
Understanding the variability of metabolite production is not only relevant for ecological purposes, but is important for natural product research and potential drug development. One on side, understanding the biological and ecological factors that affect metabolite production is essential for optimal harvesting of the producing organisms. For example, production of peloruside A (a metabolite with potent anticancer activity, Altmann and Gertsch, 2007), from large-scale aquaculture of the sponge Mycale hentscheli has been challenging, since its production is altered by parameters such as light and fouling intensity in the farm setting (Page M. et al., 2005; Page M.J. et al., 2005). Similarly, the production of specialized metabolites such as the anticancer compound panicein A hydroquinone (Fiorini et al., 2015), decreased over 10-fold in the putative reproductive months (Reverter et al., 2018), highlighting the importance of timely sponge collection. On the other side, different biotic and abiotic conditions can result in the production of distinct metabolites (for example through the expression of silent genes), suggesting that a deep understanding on the factors underlying metabolite production can also contribute toward the discovery of novel compounds (Elias et al., 2006; Romano et al., 2018). This has triggered the development of new approaches mostly in the microbiology field such as the “OSMAC” (One Strain Many Compounds) and co-culture approaches that aim to produce differential compounds by modifying the abiotic or biotic culture conditions (Fan et al., 2019; Sproule et al., 2019).
Metabolomics have proven a powerful, although mostly descriptive, tool to identify metabolic patterns and responses (e.g., Reverter et al., 2018; Gaubert et al., 2020). However, bioassays are still needed to verify the functions of the identified chemomarkers. The development of ecological realistic bioassays to identify the function of metabolites could also lead to the identification of suitable conditions promoting metabolite production with direct implications for bioprospecting (Ledoux and Antunes, 2018). For example, several transplant and nutrient enrichment experiments showed that the production of the allelopathic compound sarcophytoxide by the soft coral Sarcophyton increased with higher nutrients and when the soft corals were in contact with other species (Fleury et al., 2004). While these in situ experiments provide an important tool to identify environmental factors that affect metabolite production, their reproducibility is often constrained by disregarded parameters that affect the metabolome, masking single factor effects.
Host-Microbe Interactions
Many animals and plants harbor exceptionally diverse communities of microorganisms (i.e., bacteria, fungi, viruses) that perform vital functions in the holobiont (Bewley et al., 1996; Thomas et al., 2016; Wilkins et al., 2019). In marine organisms, associated microorganisms are sometimes identified as the producers of the natural products found in their hosts (e.g., Wilson et al., 2014; Morita and Schmidt, 2018; Rust et al., 2020). For example, recent studies have demonstrated that many sponge-isolated compounds such as different polyketides, peptides and bromotyrosine-derived alkaloids, are in fact, produced by their associated microorganisms (Wilson et al., 2014; Nicacio et al., 2017). Another example includes γ-pyrones, which are often found in molluscs such as cone snails, and which were shown to be produced by the associated cultivable bacteria Nocardiopsis alba (Lin et al., 2013). Classical approaches used to investigate the contribution of host-associated microorganisms in natural product synthesis include isolation and host-independent culture of the microorganisms (Lin et al., 2013; Nicacio et al., 2017). Host-associated culture with miniature incubation chambers inside the host has also been attempted with varying success (Steinert et al., 2014). However, these approaches are unsuitable for uncultivable microorganisms, which have been proposed to be at least 70% of the known taxa for the prokaryotic phyla alone (Achtman and Wagner, 2008). Furthermore, the holobiont comprises a complex network of microorganisms that are in constant communication and signaling, which regulates gene expression and metabolite synthesis (Hughes and Sperandio, 2008; Pande and Kost, 2017). Therefore, isolation and repeated culture of host-associated microorganisms can result in a loss of compound production (Morita and Schmidt, 2018). Within this context, the combined study of the holobiont microbiome and metabolome provides new exciting opportunities to explore host-symbiont relationships. Identification of consistent co-associations between compounds and microorganisms using correlation tools can assist in metagenomics mining to search for natural product biosynthetic gene clusters (BGCs) in the holobionts (Paix et al., 2019; Reverter et al., 2020, Figure 2). For example, cyclic dipeptides found in the algae Taonia atomaria were found to be highly correlated with a BD1-7 clade bacterial taxon from the Alteromanadaceae family (Paix et al., 2019). Similarly, several hemoglobin-derived peptides found in the butterflyfish Chaetodon lunulatus were highly correlated to a Fusobacteriaceae strain (Reverter et al., 2020), suggesting a possible direct or indirect involvement of these bacteria in the production of the aforementioned compounds.
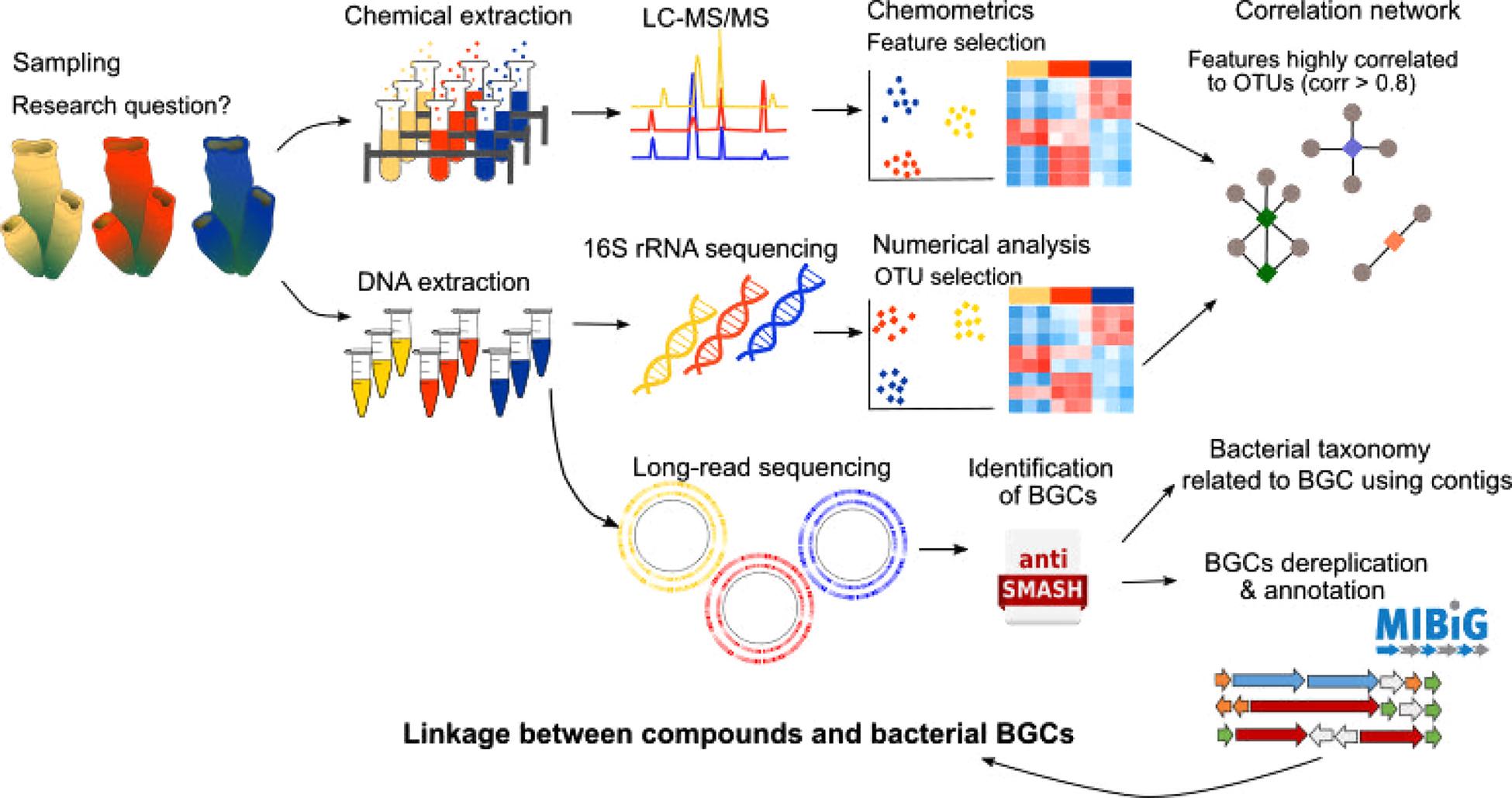
Figure 2. Pipeline illustrating the integration of metabolomics, microbiomics and metagenomics tools. The parallel metabolome and microbiome analysis allows the identification of metabolites of interest that are highly correlated to specific bacterial taxons. Then by performing long-read sequencing and genome mining (using tools such as AntiSMASH or similar) biosynthetic gene clusters (BGCs) can be identified and annotated manually or by using databases such as MIBiG. 16S contigs (i.e., continuous fragments of DNA sequence from an incomplete genome) can then be used to assign the BGCs to specific bacterial taxon genomes. The predicted compounds encoded by these BGCs can then be compared with the metabolome data in order to link produced metabolites with specific bacterial BGCs.
Multi-Omics Integration
Although several natural product BGCs have been elucidated using metagenomics (e.g., Wakimoto et al., 2014; Storey et al., 2020), most of them have yet to be linked to the metabolites they encode (Amos et al., 2017). Metabolomics is considered the last link in the systems biology chain, therefore, its coupling with other high-throughput technologies, such as transcriptomics and genomics, provides a bridge to link molecular mechanisms with metabolite production (Amos et al., 2017; van der Hooft et al., 2020). Such integration techniques provide a deeper understanding of the molecular mechanisms involved in the production of biologically active compounds through the identification of BGCs, gene expression patterns and enzymes related to the produced metabolites (Paul et al., 2019; van der Hooft et al., 2020). For example, an integrated metabolomic-transcriptomic analysis of the sea urchin Strongylocentrotus intermedius allowed identification of critical genes related to eicosanoid acid biosynthesis (Wang et al., 2020). A study of Dysideidae sponges using mass spectrometry, molecular fragmentation and NMR spectroscopy identified a large-array of new polybrominated diphenyl ethers (PBDEs) in these sponges (Agarwal et al., 2015). Application of metagenomics then led to the identification of the genes responsible for PBDEs production within the sponge cyanobacterial endosymbionts (Agarwal et al., 2017; Schorn et al., 2019). Similarly, integration of molecular networking and genome-mining of several marine Salinispora bacteria led to several molecular family-BGC pairings, including the characterization of a new cytotoxic depsipeptide (retimycin) and its link to gene cluster NRPS40 (Duncan et al., 2015; Amos et al., 2017). Metabologenomics, a term that defines these integrated approaches, can therefore contribute to accelerate the linking of unknown BGCs to metabolites as well as assist in extract prioritization for structure elucidation (Goering et al., 2016; van der Hooft et al., 2020, Figure 2).
Natural products complexity is often a barrier in their synthesis and scale-up production, while harvest of the producing organisms (e.g., plants, marine macroorganisms or uncultivable microorganisms) might not be a reliable or sustainable option (Paddon and Keasling, 2014). For example, 13 tonnes of the marine bryozoan Bugula neritina were harvested for the isolation of 18 g of the potent anti-cancer compound bryostatin 1 for clinical phase 1 studies (Schaufelberger et al., 1991). In such cases, pairing interesting metabolites with their encoding genes provides new opportunities for the synthesis of natural products using synthetic biology approaches such as insertion of biosynthetic genes in heterologous hosts (Amos et al., 2017; Zhang et al., 2019; Ahmed et al., 2020). However, despite the huge potential of these methods and the global effort in increasing the number of annotated and well-curated genome data from marine bacteria and symbionts (e.g., Udwary et al., 2007; Machado et al., 2015), these tools are still in the infancy and need to be further developed for their widespread use in marine biotechnology.
Future Directions and Concluding Remarks
Amidst the increasing global concern over antimicrobial resistance, the SARS-CoV-2 virus pandemic, and the continuous need for anticancer and antiviral drugs, the natural product research field has attracted renewed attention as new tools such as metabolomics accelerate metabolite discovery and decrease re-discovery rates (Rodrigues et al., 2016; Liu et al., 2019). In particular, marine organisms arise as a prolific source of novel natural products, with an economic value estimated at US$ 563 billion – 5.7 trillion for only anticancer marine drugs (Erwinn et al., 2010; Abdelmohsen et al., 2017). Marine organisms have been much less studied than their terrestrial counterparts, however, they produce an incredibly diverse array of molecules with new chemical features and modes of action (Abdelmohsen et al., 2017, Carroll et al., 2020). For example, the production of highly bioactive organohalogens seems to be widespread amongst marine organisms, but is less common amongst terrestrial organisms (Gribble, 2010). Both the discovery of new species and improved taxonomic descriptions offer new natural product bioprospecting possibilities. However, not only taxonomy determines the production of metabolites but also biotic (e.g., life cycle, organisms interactions) and abiotic (e.g., environmental conditions) factors regulate metabolite production (e.g., Nylund et al., 2011; Gaubert et al., 2020). Metabolomics arises therefore not only as a fast alternative to elucidate new biologically active metabolites, but as a tool to study the variation of the organism’s chemical diversity in response to different factors. Linking the production of biologically active metabolites to environmental or biological conditions could have direct implications for bioprospecting and scaling-up of metabolite production. Where scaling-up of metabolite production from whole organism culture is not possible, the linking of metabolomics with metagenomics approaches, that may allow identifying responsible BGCs (either in the host or the associated microorganisms), also provides new opportunities for metabolite production through synthetic biology and bioengineering (Ahmed et al., 2020).
Despite the many advantages and huge potential of metabolomics approaches for the discovery of new marine biologically active compounds, the major drawback continues to be the poor coverage of marine natural products in public databases (Pereira and Aires-de-Sousa, 2018; Barbosa and Roque, 2019). Such lack of public reference spectra (including fragmentation patterns) of marine-derived natural products prevents automated dereplication processes, and often results in the development of in-house libraries by the different research groups after labor-intensive efforts (e.g., purification and structural elucidation of each metabolite). Therefore, development of a free-access database with well-curated spectrometric data (e.g., MS1, MS2, UV, NMR) on marine natural products is urgently needed, along with the constant development and improvement of metabolomics tools (e.g., hardware and workflows) to increase sensitivity and repeatability to enable comparison and integration of a larger number of datasets (Guitton et al., 2017; Forsberg et al., 2018). This could be achieved, for example, with the integration of known and new marine natural products (from macro- and microorganisms elucidated by both classical approaches and metabolomics) into currently operational databases highly used in metabolomics such as GNPS.
Author Contributions
MR conceived the first idea of the article. MR, SR, and CP wrote the first draft of the article. All authors revised the first draft and worked on the final version of the article. All authors discussed throughout the conception of the article.
Funding
This research was funded by an Alexander von Humboldt post-doctoral fellowship to MR. The Ph.D. of CP is supported through a scholarship from the french region “Occitanie”.
Conflict of Interest
The authors declare that the research was conducted in the absence of any commercial or financial relationships that could be construed as a potential conflict of interest.
Footnotes
- ^ https://workflow4metabolomics.usegalaxy.fr
- ^ www.metaboanalyst.ca
- ^ https://gnps.ucsd.edu
- ^ https://ms2lda.org
References
Abdelmohsen, U. R., Balasubramanian, S., Oelschlaeger, T. A., Grkovic, T., Pham, N. B., Quinn, R. J., et al. (2017). Potential of marine natural products against drug-resistant fungal, viral, and parasitic infections. Lancet Infect. Dis. 17, e30–e41. doi: 10.1016/S1473-3099(16)30323-1
Achtman, M., and Wagner, M. (2008). Microbial diversity and the genetic nature of microbial species. Nat. Rev. Microbiol. 6, 431–440. doi: 10.1038/nrmicro1872
Agarwal, V., Blanton, J. M., Podell, S., Taton, A., Schorn, M. A., Busch, J., et al. (2017). Metagenomic discovery of polybrominated diphenyl ether biosynthesis by marine sponges. Nat. Chem. Biol. 13, 537–543. doi: 10.1038/nchembio.2330
Agarwal, V., Li, J., Rahman, I., Borgen, M., Aluwihare, L. I., Biggs, J. S., et al. (2015). Complexity of naturally produced polybrominated diphenyl ethers revealed via mass spectrometry. Env. Sci. Technol. 49, 1339–1346. doi: 10.1021/es505440j
Ahmed, Y., Rebets, Y., Estévez, M. R., Zapp, J., Myronovskyi, M., and Luzhetskyy, A. (2020). Engineering of Streptomyces lividans for heterologous expression of secondary metabolite gene clusters. Microb. Cell Fact. 19, 5. doi: 10.1186/s12934-020-1277-8
Altmann, K. H., and Gertsch, J. (2007). Anticancer drugs from nature – natural products as a unique source of new microtubule-stabilizing agents. Nat. Prod. Rep. 24, 327–357. doi: 10.1039/b515619j
Amos, G. C. A., Awakawa, T., Tuttle, R. N., Letzel, A.-C., Kim, M. C., Kudo, Y., et al. (2017). Comparative transcriptomics as a guide to natural product discovery and biosynthetic gene cluster functionality. Proc. Natl. Acad. Sci. U.S.A. 114, E11121–E11130. doi: 10.1073/pnas.1714381115
Aron, A. T., Gentry, E. C., McPhail, K. L., Nothias, L.-F., Nothias-Esposito, M., Bouslimani, A., et al. (2020). Reproducible molecular networking of untargeted mass spectrometry data using GNPS. Nat. Protoc. 15, 1954–1991. doi: 10.1038/s41596-020-0317-5
Barbosa, A. J. M., and Roque, A. C. A. (2019). Free marine natural product databases for biotechnology and bioengineering. Biotechnol. J. 14:1800607. doi: 10.1002/biot.201800607
Bewley, C. A., Holland, N. D., and Faulkner, D. J. (1996). Two classes of metabolites from Theonella swinhoei are localized in distinct bacterial symbionts. Experientia 52, 716–722. doi: 10.1007/BF01925581
Cantrell, T. P., Freeman, C. J., Paul, V. J., Agarwal, V., and Garg, N. (2019). Mass spectrometry-based integration and expansion of the chemical diversity harbored within a marine sponge. J. Am. Soc. Mass Spectrom. 30, 1373–1384. doi: 10.1007/s13361-019-02207-5
Carroll, A. R., Copp, B. R., Davis, R. A., Keyzers, R. A., and Prinsep, M. R. (2020). Marine natural products. Nat. Prod. Rep. 37, 175–223. doi: 10.1039/C9NP00069K
Caso, A., Esposito, G., Della Sala, G., Pawlik, J. R., Teta, R., Mangoni, A., et al. (2019). Fast detection of two smenamide family members using molecular networking. Mar. Drugs 17:618. doi: 10.3390/md17110618
Duncan, K. R., Crüsemann, M., Lechner, A., Sarkar, A., Li, J., Ziemert, N., et al. (2015). Molecular networking and pattern-based genome mining improves discovery of biosynthetic gene clusters and their products from Salinispora species. Chem. Biol. 22, 460–471. doi: 10.1016/j.chembiol.2015.03.010
Einarsdottir, E., Magnusdottir, M., Astarita, G., Köck, M., Ögmundsdottir, H. M., Thorsteinsdottir, M., et al. (2017). Metabolic profiling as a screening tool for cytotoxic compounds: identification of 3-alkyl pyridine alkaloids from sponges collected at shallow water hydrothermal vent site north of Iceland. Mar. Drugs 15, 52. doi: 10.3390/md15020052
El Menif, E., Offret, C., Labrie, S., and Beaulieu, L. (2019). Identification of peptides implicated in antibacterial activity of snow crab hepatopancreas hydrolysates by a bioassay-guided fractionation approach combined with mass spectrometry. Probiotics Antimicrob. Proteins 11, 1023–1033. doi: 10.1007/s12602-018-9484-x
Elias, B. C., Said, S., Albuquerque, S., and Tallarico-Pupo, M. (2006). The influence of culture conditions on the biosynthesis of secondary metabolites by Penicillum verucossum. Microbiol. Res. 161, 273–280. doi: 10.1016/j.micres.2005.10.003
Erwinn, P. M., López-Legentil, S., and Schuhmann, P. W. (2010). The pharmaceutical value of marine biodiversity for anti-cancer drug discovery. Ecol. Econ. 70, 445–451. doi: 10.1016/j.ecolecon.2010.09.030
Fan, B., Parrot, D., Blümel, M., Labes, A., and Tasdemir, D. (2019). Influence of OSMAC-based cultivation in metabolome and anticancer activity of fungi associated with the brown alga Fucus vesiculosus. Mar. drugs 17:67. doi: 10.3390/md17010067
Fiorini, L., Aude-Tribalat, M. A., Sauvard, L., Cazareth, J., Lalli, E., Broutin, I., et al. (2015). Natural paniceins from Mediterranean sponge inhibit the multidrug resistance activity of patched and increase chemotherapy efficiency on melanoma cells. Oncotarget 6, 22282–22297. doi: 10.18632/oncotarget.4162
Fleury, B. G., Coll, J. C., Sammarco, P. W., Tentori, E., and Duquesne, S. (2004). Complementary (secondary) metabolites in an octocoral competing with a scleractinian coral: effects of varying nutrient regimes. J. Exp. Mar. Biol. Ecol. 303, 115–131.
Forsberg, E. M., Huan, T., Rinehart, D., Benton, P. H., Warth, B., Hilmers, B., et al. (2018). Data processing, multi-omic pathway mapping, and metabolite activity analysis using XCMS Online. Nat. Prot. 13, 633–651. doi: 10.1038/nprot.2017.151
Fortman, J. L., and Mukhopadhyay, A. (2016). The Future of antibiotics: emerging technologies and stewardship. Trends Microbiol. 24, 515–517. doi: 10.1016/j.tim.2016.04.003
Gaubert, J., Rodolfo-Metalpa, R., Greff, S., Thomas, O. P., and Payri, C. E. (2020). Impact of ocean acidification on the metabolome of the brown macroalgae Lobophora rosacea from New Caledonia. Algal Res. 46:101783. doi: 10.1016/j.algal.2019.101783
Gertsman, I., and Barshop, B. A. (2018). Promises and pitfalls of untargeted metabolomics. J. Inherit. Metab. Dis. 41, 355–366. doi: 10.1007/s10545-017-0130-7
Goering, A. W., McClure, R. A., Doroghazi, J. R., Albright, J. C., Haverland, N. A., Zhang, Y., et al. (2016). Metabologenomics: correlation of microbial gene clusters with metabolites drives discovery of a nonribosomal peptide with an unusual amino acid monomer. ACS Cent. Sci. 2, 99–108. doi: 10.1021/acscentsci.5b00331
Gribble, G. W. (2010). Naturally Occurring Organohalogen Compounds – A Comprehensive Update. Vienna: Springer.
Guitton, Y., Tremblay-Franco, M., Le Corguillé, G., Martin, J.-F., Pétéra, M., Mele, P. R., et al. (2017). Create, run, share, publish, and reference your LC-MS, FIA-MS, GC-MS and NMR data analysis workflows with the Workfloy4Metabolomics 3.0 Galaxy online infrastructure for metabolomics. Int. J. Biochem. Cell Biol. 93, 89–101. doi: 10.1016/j.biocel.2017.07.002
Hay, M. E. (2009). Marine chemical ecology: chemical signals and cues structure marine populations, communities, and ecosystems. Ann. Rev. Mar. Sci. 1, 193–212. doi: 10.1146/annurev.marine.010908.163708
Herath, H. M. P., Preston, S., Jabbar, A., Garcia-Bustos, J., Taki, A. C., Addison, R. S., et al. (2019). Identification of fromiamycalin and halaminol a from Australian marine sponge extracts with anthelmintic activity against Haemonchus contortus. Mar. Drugs 17:598. doi: 10.3390/md17110598
Hughes, D. T., and Sperandio, V. (2008). Inter-kingdom signalling: communication between bacteria and their hosts. Nat. Rev. Microbiol. 6, 111–120. doi: 10.1038/nrmicro1836
Kildgaard, S., Subko, K., Phillips, E., Goidts, V., De la Cruz, M., Díaz, C., et al. (2017). A dereplication and bioguided discovery approach to reveal new compounds from a marine-derived fungus Stilbella fimetaria. Mar. Drugs 15:253. doi: 10.3390/md15080253
Ledoux, J. B., and Antunes, A. (2018). Beyond the beaten path: improving natural products bioprospecting using an eco-evolutionary framework – the case of the octocorals. Crit. Rev. Biotechnol. 38, 184–198. doi: 10.1080/07388551.2017.1331335
Li, F., Janussen, D., Peifer, C., Pérez-Victoria, I., and Tasdemir, D. (2018). Targeted isolation of tsitsikammamines from the Antarctic deep-sea sponge Latrunculia biformis by molecular networking and anticancer activity. Mar. Drugs 16:268. doi: 10.3390/md16080268
Liebal, U. W., Phan, A. N. T., Sudhakar, M., Raman, K., and Blank, L. M. (2020). Machine learning applications for mass-spectrometry based metabolomics. Metabolites 10:243. doi: 10.3390/metabo10060243
Lin, Z., Torres, J. P., Ammon, M. A., Marett, L., Teichert, R. W., Reilly, C. A., et al. (2013). A bacterial source for mollusk pyrone polyketides. Chem. Biol. 20, 73–81. doi: 10.1016/j.chembiol.2012.10.019
Liu, M., El-Hossary, E. M., Oelschlaeger, T. A., Donia, M. S., Quinn, R. J., and Abdelmohsen, U. R. (2019). Potential of marine natural products against drug-resistant bacterial infections. Lancet Infect. Dis. 19, e237–e245. doi: 10.1016/S1473-3099(18)30711-4
Luzzatto-Knaan, T., Garg, N., Wang, M., Glukhov, E., Peng, Y., Ackermann, G., et al. (2017). Digitizing mass spectrometry data to explore the chemical diversity and distribution of marine cyanobacteria and algae. Elife 6:e24214. doi: 10.7554/eLife.24214.001
Machado, H., Sonnenschein, E. C., Melchiorsen, J., and Gram, L. (2015). Genome mining reveals unlocked bioactive potential of marine Gram-negative bacteria. BMC Genomics 16:158. doi: 10.1186/s12864-015-1365-z
Mayer, M. S., Guerrero, A. J., Rodriguez, A. D., Taglialatela-Scafati, O., Nakamura, F., and Fusetani, N. (2020). Marine pharmacology in 2014-2015: marine compounds with antibacterial, antidiabetic, antifungal, anti-inflammatory, antiprotozoal, antituberculosis, antiviral and anthelmintic activities; affecting the immune and nervous systems, and other miscellaneous mechanisms of action. Mar. Drugs 18:5. doi: 10.3390/md18010005
Mohanty, I., Podell, S., Biggs, J. S., Garg, N., Allen, E. E., and Agarwal, V. (2020). Multi-omic rofiling of Melophlus Ssponges reveals diverse metabolomic and icrobiome architectures that are non-overlapping with ecological neighbors. Mar. Drugs 18:124. doi: 10.3390/md18020124
Morita, M., and Schmidt, E. W. (2018). Parallel lives of symbionts and hosts: chemical mutualism in marine animals. Nat. Prod. Rep. 35, 357–378. doi: 10.1039/C7NP00053G
Naman, C. B., Rattan, R., Nikoulina, S. E., Lee, J., Miller, B. W., Moss, N. A., et al. (2017). Integrating molecular networking and biological assays to target the isolation of a cytotoxic cyclic octapeptide, Samoamide A, from an American Samoan marine cyanobacterium. J. Nat. Prod. 80, 625–633. doi: 10.1021/acs.jnatprod.6b00907
Nicacio, K. J., Ióca, L. P., Fróes, A. M., Leomil, L., Appolinario, L. R., Thompson, C. C., et al. (2017). Cultures of the marine bacterium Pseudovibrio denitrificans Ab134 produce bromotyrosine-derived alkaloids previously only isolated from marine sponges. J. Nat. Prod. 80, 235–240. doi: 10.1021/acs.jnatprod.6b00838
Nothias, L.-F., Nothias-Esposito, M., da Silva, R., Wang, M., Protsyuk, I., Zhang, Z., et al. (2018). Bioactivity-based molecular networking for the discovery of drug leads in natural product bioassay-guided fractionation. J. Nat. Prod. 81, 758–767. doi: 10.1021/acs.jnatprod.7b00737
Nylund, G. M., Weinberger, F., Rempt, M., and Pohnert, G. (2011). Metabolomic assessment of induced and activated chemical defence in the invasive red alga Gracilaria vermiculophylla. PLoS One 6:e29359. doi: 10.1371/journal.pone.0029359
Paddon, C. J., and Keasling, J. D. (2014). Semi-synthetic artemisinin: a model for the use of synthetic biology in pharmaceutical development. Nat. Microbiol. 12, 355–367. doi: 10.1038/nrmicro3240
Page, M. J., Northcote, P. T., Webb, V. L., Mackey, S., and Handley, S. J. (2005). Aquaculture trials for the production of biologically active metabolites in the New Zealand sponge Mycale hentscheli (Demospongiae: Poecilosclerida). Aquaculture 250, 256–269. doi: 10.1016/j.aquaculture.2005.04.069
Page, M., West, L., Northcote, P., Battershill, C., and Kelly, M. (2005). Spatial and temporal variability of cytotoxic metabolites in populations of the New Zealand sponge Mycale hentscheli. J. Chem. Ecol. 31, 1161–1174. doi: 10.1007/s10886-005-4254-0
Paix, B., Othmani, A., Debroas, D., Culioli, G., and Briand, J. F. (2019). Temporal covariation of epibacterial community and surface metabolome in the Mediterranean seaweed holobiont Taonia atomaria. Environ. Microbiol. 21, 3346–3363. doi: 10.1111/1462-2920.14617
Pande, S., and Kost, C. (2017). Bacterial unculturability and the formation of intercellular metabolic networks. Trends Microbiol. 25, 349–361. doi: 10.1016/j.tim.2017.02.015
Paul, V. J., Freeman, C. J., and Agarwal, V. (2019). Chemical ecology of marine sponges: new opportunities through “-Omics”. Integr. Comp. Biol. 59, 765–776. doi: 10.1093/icb/icz014
Pavia, H., and Brock, E. (2000). Extrinsic factors influencing phlorotannin production in the brown alga Ascophyllum nodosum. Mar. Ecol. Prog. Ser. 193, 285–294. doi: 10.3354/meps193285
Pereira, F., and Aires-de-Sousa, J. (2018). Computational methodologies in the exploration of marine natural product leads. Mar. Drugs 16:236. doi: 10.3390/md16070236
Pereira, R. B., Pereira, D. M., Jiménez, C., Rodríguez, J., Nieto, R. M., Videira, R. A., et al. (2019). Anti-inflammatory effects of 5α, 8α-epidioxycholest-6-en-3β-ol, a steroidal endoperoxide isolated from Aplysia depilans, based on bioguided fractionation and NMR analysis. Mar. Drugs 17:330. doi: 10.3390/md17060330
Puglisi, M. P., and Becerro, M. A. (2018). Chemical Ecology: The Ecological Impacts of Marine Natural Products. Boca Raton, FL: CRC Press.
Ramos, A. E. F., Evanno, L., Poupon, E., Champy, P., and Beniddir, M. A. (2019). Natural products targeting strategies involving molecular networking: different manners, one goal. Nat. Prod. Rep. 36, 960–980. doi: 10.1039/c9np00006b
Réveillon, D., Tunin-Ley, A., Grondin, I., Othmani, A., Zubia, M., Bunet, R., et al. (2019). Exploring the chemodiversity of tropical microalgae for the discovery of natural antifouling compounds. J. Appl. Phycol. 31, 319–333. doi: 10.1007/s10811-018-1594-z
Reverter, M., Sasal, P., Suzuki, M. T., Raviglione, D., Inguimbert, N., Pare, A., et al. (2020). Insights into the natural defences of a coral reef fish against gill ectoparasites: integrated metabolome and microbiome approach. Metabolites 10:227. doi: 10.3390/metabo10060227
Reverter, M., Tribalat, M.-A., Pérez, T., and Thomas, O. P. (2018). Metabolome variability for two Mediterranean sponge species of the genus Haliclona: specificity, time, and space. Metabolomics 14:114. doi: 10.1007/s11306-018-1401-5
Rodrigues, T., Reker, D., Schneider, P., and Schneider, G. (2016). Counting on natural products for drug design. Nat. Chem. 8, 531–541. doi: 10.1038/nchem.2479
Rohde, S., Gochfeld, D. J., Ankisetty, S., Avula, B., Schupp, P. J., and Slattery, M. (2012). Spatial variability in secondary metabolites of the Indo-Pacific sponge Stylissa massa. J. Chem. Ecol. 38, 463–475. doi: 10.1007/s10886-012-0124-8
Rohde, S., Nietzer, S., and Schupp, P. J. (2015). Prevalence and mechanisms of dynamic chemical defenses in tropical sponges. PLoS One 10:e0132236. doi: 10.1371/journal.pone.0132236
Romano, S., Jackson, S. A., Patry, S., and Dobson, A. D. W. (2018). Extending the “One Strain Many Compounds” OSMAC principle to marine organisms. Mar. Drugs 16:244. doi: 10.3390/md16070244
Rust, M., Helfrich, E. J. N., Freeman, M. F., Nanudorn, P., Field, C. M., Rückert, C., et al. (2020). A multiproducer microbiome generates chemical diversity in the marine sponge Mycale hentscheli. Proc. Nat. Acad. Sci. U.S.A. 117, 9508–9518. doi: 10.1073/pnas.1919245117
Schaufelberger, D. E., Koleck, M. P., Beutler, J. A., Vatakis, A. M., Alvarado, A. B., Andrews, P., et al. (1991). The large-scale isolation of bryostatin 1 from Bugula neritina following current good manufacturing practices. J. Nat. Prod. 54, 1265–1270. doi: 10.1021/np50077a004
Schorn, M. A., Jordan, P. A., Podell, S., Blanton, J. M., Agarwal, V., Biggs, J. S., et al. (2019). Comparative genomics of cyanobacterial symbionts reveals distinct, specialized metabolism in tropical Dysideidae sponges. mBio 10:e00821-19. doi: 10.1128/mBio.00821-19
Sproule, A., Correa, H., Decken, A., Haltli, B., Berrué, F., Overy, D. P., et al. (2019). Terrosamycins A and B, bioactive polyether ionophores from Streptomyces sp. RKND004 from Prince Edward Island sediment. Mar. Drugs 17:347. doi: 10.3390/md17060347
Steinert, G., Whitfield, S., Taylor, M. W., Thoms, C., and Schupp, P. J. (2014). Application of diffusion growth chambers for he cultivation of marine sponge-associated bacteria. Mar. Biotechnol. 16, 594–603. doi: 10.1007/s10126-014-9575-y
Storey, M. A., Andreassend, S. K., Bracegirdle, J., Brown, A., Keyzers, R. A., Ackerley, D. F., et al. (2020). Metagenomic exploration of the marine sponge Mycale hentscheli uncovers multiple polyketide-producing bacterial symbionts. mBio 11:e02997-19. doi: 10.1128/mBio.02997-19
Stuart, K. A., Welsh, K., Walker, M. C., and Edrada-Ebel, R. (2020). Metabolomic tools used in marine natural product drug discovery. Expert Opin. Drug Discov. 15, 499–522. doi: 10.1080/17460441.2020.1722636
Tebben, J., Motti, C. A., Siboni, N., Tapiolas, D. M., Negri, A. P., Schupp, P. J., et al. (2015). Chemical mediation of coral larval settlement by crustose coralline algae. Sci. Rep. 5:10803.
Thakur, N. L., and Müller, W. E. G. (2004). Biotechnological potential of marine sponges. Curr. Sci. 86, 1506–1512.
Thomas, T., Moitinho-Silva, L., Lurgi, M., Björk, J. R., Easson, C., Astudillo-Garcia, C., et al. (2016). Diversity, structure and convergent evolution of the global sponge microbiome. Nat. Comm. 7:11870. doi: 10.1038/ncomms11870
Udwary, D. W., Zeigler, L., Asolkar, R. N., Singan, V., Lapidus, A., Fenical, W., et al. (2007). Genome sequencing reveals complex secondary metabolome in the marine actinomycete Salinispora tropica. Proc. Natl. Acad. Sci. U.S.A. 104, 10376–10381. doi: 10.1073/pnas.0700962104
van der Hooft, J. J. J., Mohimani, H., Bauermeister, A., Dorrestein, P. C., Duncan, K. R., and Medema, M. H. (2020). Linking genomics and metabolomics to chart specialized metabolic diversity. Chem. Soc. Rev. 11, 3297–3314. doi: 10.1039/D0CS00162G
Wakimoto, T., Egami, Y., Nakashima, Y., Wakimoto, Y., Mori, T., Awakawa, T., et al. (2014). Calyculin biogenesis from a pyrophosphate protoxin produced by a sponge symbiont. Nat. Chem. Biol. 10, 648–655. doi: 10.1038/nchembio.1573
Wang, H., Ding, J., Ding, S., and Chang, Y. (2020). Integrated metabolomic and transcriptomic analyses identify critical genes in eicosapentaenoic acid biosynthesis and metabolism in the sea urchin Strongylocentrotus intermedius. Sci. Rep. 10:1697. doi: 10.1038/s41598-020-58643-x
Wang, M., Carver, J. J., Phelan, V. V., Sanchez, L. M., Garg, N., Peng, Y., et al. (2016). Sharing and community curation of mass spectrometry data with Global Natural Products Social Molecular Networking. Nat. Biotechnol. 34, 828–837. doi: 10.1038/nbt.3597
Wilkins, L. G. E., Leray, M., O’Dea, A., Yuen, B., Peixoto, R. S., Pereira, T. J., et al. (2019). Host-associated microbiomes drive structure and function of marine ecosystems. PLoS Biol. 17:e3000533. doi: 10.1371/journal.pbio.3000533
Wilson, M. C., Mori, T., Rückert, C., Uria, A. R., Helf, M. J., Takada, K., et al. (2014). An environmental bacterial taxon with a large and distinct metabolic repertoire. Nature 506, 58–62. doi: 10.1038/nature12959
Wolfender, J.-L., Nuzillard, J.-M., van der Hooft, J. J. J., Renault, J.-H., and Bertrand, S. (2019). Accelerating metabolite identification in natural product research: toward an ideal combination of liquid chromatography–high-resolution tandem mass spectrometry and NMR Profiling, in silico databases, and chemometrics. Anal. Chem. 91, 704–742. doi: 10.1021/acs.analchem.8b05112
Zhang, F., Braun, D. R., Chanana, S., Rajski, S. R., and Bugni, T. S. (2019). Phallusialides A–E, pyrrole-derived alkaloids discovered from a marine-derived Micromonospora sp. bacterium using MS-based metabolomics approaches. J. Nat. Prod. 82, 3432–3439. doi: 10.1021/acs.jnatprod.9b00808
Keywords: metabolomics, marine biotechnology, marine natural products, chemical ecology, omics integration
Citation: Reverter M, Rohde S, Parchemin C, Tapissier-Bontemps N and Schupp PJ (2020) Metabolomics and Marine Biotechnology: Coupling Metabolite Profiling and Organism Biology for the Discovery of New Compounds. Front. Mar. Sci. 7:613471. doi: 10.3389/fmars.2020.613471
Received: 02 October 2020; Accepted: 13 November 2020;
Published: 10 December 2020.
Edited by:
Susana P. Gaudêncio, New University of Lisbon, PortugalReviewed by:
Usama Ramadan Abdelmohsen, Minia University, EgyptS. López-Legentil, University of North Carolina at Wilmington, United States
Copyright © 2020 Reverter, Rohde, Parchemin, Tapissier-Bontemps and Schupp. This is an open-access article distributed under the terms of the Creative Commons Attribution License (CC BY). The use, distribution or reproduction in other forums is permitted, provided the original author(s) and the copyright owner(s) are credited and that the original publication in this journal is cited, in accordance with accepted academic practice. No use, distribution or reproduction is permitted which does not comply with these terms.
*Correspondence: Miriam Reverter, bWlyaXJldmVydGVyQGdtYWlsLmNvbQ==