- Department of Biology and Environmental Science, Linnaeus University Centre of Ecology and Evolution and Microbial Model Systems, Linnaeus University, Kalmar, Sweden
Eutrophication coupled to climate change disturbs the balance between competition and coexistence in microbial communities including the partitioning of organic and inorganic nutrients between phytoplankton and bacteria. Competition for inorganic nutrients has been regarded as one of the drivers affecting the productivity of the eutrophied coastal Baltic Sea. Yet, it is unknown at the molecular expression level how resources are competed for, by phytoplankton and bacteria, and what impact this competition has on the community composition. Here we use metatranscriptomics and amplicon sequencing and compare known metabolic pathways of both phytoplankton and bacteria co-occurring during a summer bloom in the archipelago of Åland in the Baltic Sea to examine phytoplankton bacteria resource partitioning. The expression of selected pathways of carbon (C), nitrogen (N), and phosphorus (P) metabolism varied over time, independently, for both phytoplankton and bacteria, indicating partitioning of the available organic and inorganic resources. This occurs regardless of eukaryotic plankton growth phase (exponential or stationary), based on expression data, and microbial community composition. Further, the availability of different nutrient resources affected the functional response by the bacteria, observed as minor compositional changes, at class level, in an otherwise taxonomically stable bacterial community. Resource partitioning and functional flexibility seem necessary in order to maintain phytoplankton-bacteria interactions at stable environmental conditions. More detailed knowledge of which organisms utilize certain nutrient species are important for more accurate projections of the fate of coastal waters.
Introduction
Coastal marine ecosystems have a key role of filtering nutrients and pollutants coming from land, before entering the pelagic environment (Bonsdorff et al., 1997; Rabalais et al., 2009; Carstensen et al., 2019). In coastal oceans, as along the coast of the Baltic Sea, allochthonous organic matter will get incorporated into the microbial food web, which may have large consequences for the nutrient balance between phytoplankton and bacteria (Andersson et al., 2015; Diner et al., 2016). During summer, low levels of inorganic nutrients (Nausch et al., 2018; Savchuk, 2018) limits phytoplankton growth (Granéli et al., 1990; Kivi and Setäl, 1995), while high levels of organic nutrients (Nausch et al., 2018; Savchuk, 2018) are present.
Effects of global warming including increased freshwater flow and allochtonous input of dissolved nutrients are likely to aggravate the long-term consequences of eutrophication in coastal ecosystems, such as archipelagic systems common to the brackish Baltic Sea, making it a suitable study system for the impacts of these effects. The consequences may include higher phytoplankton growth, oxygen deficiency, and a transition into a less productive ecosystem (Rönnberg and Bonsdorff, 2004; Rabalais et al., 2009). A complex interplay between autotrophic and heterotrophic marine microbes will determine how the accumulation and turnover of organic matter will be affected in a future climate scenario (Andersson et al., 2015; Zhou Y. et al., 2018). An understanding of how organic and inorganic nutrients are partitioned between phytoplankton and bacteria, and the dependence of phytoplankton on bacterial remineralization, is therefore important for predictions of status and knowledge of how to manage coastal ecosystems (Heiskanen et al., 2019).
One of the ways to investigate phytoplankton and bacteria interactions is by looking for co-occurrence patterns in the natural environment (Elifantz et al., 2005; Teeling et al., 2012; Landa et al., 2016). Several studies have investigated interactions during open water phytoplankton bloom conditions, and have shown that the different growth phases of blooms cause compositional changes in the co-occurring bacterial community, with successions of different bacterial groups assigned to Alpha-, Beta-, and Gamma-proteobacteria, Flavobacteria, Cytophagia, Actinobacteria, and Firmicutes (Teeling et al., 2012; Landa et al., 2016; Sison-Mangus et al., 2016; Camarena-Gómez et al., 2018). These changes have been attributed to the succession of exudates released by phytoplankton, differing both in quality (Cottrell and Kirchman, 2000; Elifantz et al., 2005; Camarena-Gómez et al., 2018; Mühlenbruch et al., 2018) and quantity (Sarmento et al., 2016) during their lifespan (Grossart et al., 2005; Grossart and Simon, 2007; Mühlenbruch et al., 2018). This suggests that a diverse bacterial community is important for the degradation of amino acids, proteins, extracellular polysaccharides and carbohydrates released by phytoplankton. This is also seen in the taxonomical difference among bacterial groups found either free-living or particle attached (Grossart et al., 2005; Rink et al., 2007; Sapp et al., 2007; Bagatini et al., 2014). Compositional shifts are often assumed to involve functional changes (Hunt et al., 2008; Williams et al., 2012), especially for bacteria, as different taxa are attributed with different functions, e.g., being copiotroph or oligotroph (Lauro et al., 2009), or generalists or specialists (Sarmento et al., 2016). However, composition studies alone limit the conclusions about which underlying processes and functions are selected for.
Using metatranscriptomics, capturing the actively expressed mRNA pool, with the inclusion of polyA and total mRNA, both the eukaryotic and prokaryotic transcriptional repertoire of a microbial community can be investigated (Dupont et al., 2015). Metatranscriptomics provide a glimps into the possible functions within a community at the time of sampling, while amplicon sequencing, using the ribosomal gene pool (16S and 18S rRNA gene), can be used for taxonomic identification. By combining the two methods both functional and compositional characterization of a microbial community can be made (McCarren et al., 2010; Teeling et al., 2012; Gifford et al., 2013; Alexander et al., 2015), thereby allowing for analysis of the connection between taxonomy and function. Functional analyses using transcriptomic data have suggested that bacterial species may avoid competition by ecological niche formation by having preferences for different types of algal substrates (Teeling et al., 2012) and of marine dissolved organic matter (McCarren et al., 2010). Taken together, this emphasizes the importance of resource partitioning and microbial community dynamics, encompassing both phytoplankton and bacteria, in the global carbon cycle.
In this study planktonic eukaryotes (PE <90 μm) and bacterioplankton (BAC) of an archipelagic bay in the Baltic Sea were sampled at two timepoints during late summer, with the aim of investigating carbon and nutrient (nitrogen and phosporus) utilization and partitioning between the two groups. The PE, consisting of phytoplankton (auto- and heterotrophic flagellated forms) and ciliates, and the BAC, dominated by heterotrophic bacteria with some cyanobacteria, were characterized separately, taxonomically, through both microscopic observation and amplicon sequencing, and functionally, focusing on the transcription of genes associated with uptake and assimilation of carbon, nitrogen, and phosphorus. The combination of morphological and molecular methods for characterization of the PE allows a more accurate identification of microbes, in light of known weaknesses such as high copy numbers for some protists (Gong and Marchetti, 2019) and brittleness when fixed for others (Mironova et al., 2009). Few, if any, studies have previously characterized the functional repertoire or resource partitioning of both phytoplankton and bacteria together, in such a coastal environment dominated by photosynthetic and often mixotrophic dinoflagellates.
Materials and Methods
Field Sampling
Surface water (top 20 cm of water column, sieved through 90 μm) was collected 5–10 m off shore in a shallow bay off Lillängö, Åland archipelago, Northern Baltic Sea (60°5.86′N 20°30.3′E) at noon, on 27 July (T1) and 10 Aug (T2) 2016 (Supplementary Figure 1). Surface water temperature, salinity, and pH were recorded in situ at both occasions. Subsamples were taken for chlorophyll a measurement, filtered onto Whatman GF/C, stored at −20°C until extraction (ethanol) and fluorometric analysis (440 nm) according to Jespersen and Christoffersen (1987). Water samples for analyses of inorganic nutrients (200 mL, 2 replicates) such as nitrate, nitrite, phosphate and silica (Table 1) were kept frozen and dark until spectrophotometric analysis (UV-1600, VWR) following the method of Valderrama (1995).
For DNA and RNA collection at each sampling day, 3 replicates of 1 L of seawater were prefiltered through a 90 μm mesh, followed by filtration through a 3 μm membrane filter (Versapor, 47 mm), capturing both phytoplankton and the attached (AT) bacterial fraction. Subsequently, 300 mL of each filtrate were filtered through a 0.2 μm Supor filter (47 mm), capturing the free-living (FL) bacterial fraction. Filtrations were done using a hand operated vacuum pump. All filters were immediately placed in pre-prepared cryotubes with RNAlater (Sigma) and stored in a cooler with ice packs. Samples were then stored at −20°C until arrival in the laboratory (<30 h), where they were stored at −80°C until DNA/RNA extraction, within 7 months of sampling.
Morphological Identification and Enumeration
For phytoplankton, subsamples (50 mL) were fixed using pH neutral Lugol's solution and stored in darkness. Phytoplankton cells sedimented in Utermöhl chambers were counted at 100x magnification using an inverted microscope (Olympus CKX 41). Phytoplankton, identified according to Hällfors (2004), were grouped as planktonic eukaryotes (PE) and cyanobacteria (filamentous). Phytoplankton biomass was calculated from cell biovolume and carbon content according to Olenina et al. (2006). Bacterial abundance (BAC) was measured by flow cytometry in 1.8 mL water subsamples fixed using 200 μL formaldehyde (1.5% final concentration) stored at−20°C for transport to the laboratory, were stored at −80°C until further processing. Samples were stained with SYBR green (Life Technologies) and bacterial cells were counted using a Cube8 flow cytometer (Partec). The cytograms were analyzed using FCS Express 4 flow cytometry data analysis software.
DNA Extraction and Preparation for Sequencing
Filters were cut into 4 quarters, of which one quarter was used for DNA extraction and the remaining 3 for RNA extraction. DNA was extracted using a phenol:chloroform protocol according to Boström et al. (2004). The V3-V4 region of the 16S rRNA gene was amplified, from both the AT and the FL size fractions, using primers 341F (CCTACGGGNGGCWGCAG) and 805R (GACTACHVGGGTATCTAATCC), and the V4-V5-region of the 18S rRNA gene was amplified, from the AT size fraction, using primers 574*F (CGGTAAYTCCAGCTCYV) and 1132R (CCGTCAATTHCTTYAART). Primers were connected to Illumina adapters, in accordance with Herlemann et al. (2011) and Hugerth et al. (2014). The PCR protocol used for 16S rRNA gene amplification was: (1x[98°C, 30 s], 28x[98°C, 10 s, 54°C, 30 s, 72°C, 15 s], 1x[72°C, 2 min]), modified from Bunse et al. (2016) and the protocol used for 18S rRNA gene amplification was: (1x[98°C, 30 s], 28x [98°C, 20 s, 50.4°C, 20 s, 72°C, 15 s], 1x [72°C, 2 min]). In a second PCR, Illumina handles and indexes (i7 and i5) were attached to both the 16S and 18S products using: (1x[98°C, 30 s], 12x[98°C, 20 s, 62°C, 30 s, 72°C, 30 s], 1x[72°C, 2 min]), modified from Hugerth et al. (2014). Fusion Mastermix (ThermoScientific) was used for all PCRs. The PCR products were purified both after the first and the second amplification steps, using the EZNA Cycle Pure kit (Omega Bio-tek) according to the manufacturer's instructions. Products were quantified with Qubit fluorometer (Invitrogen) and quality checked with Nanodrop 2000 spectrophotometer (Thermo Fisher Scientific). Fragment sizes were validated using gel electrophoresis. This resulted in 18 samples [16S: 6xT1(3xFL, 3xAT), 6xT2(3xFL, 3xAT); 18S: 3xT1, 3xT2] in total, that were pooled at equimolar concentrations and sequenced using Illumina MiSeq v3, PE (Illumina Inc), 2 × 300 base pairs (bp), at SciLifeLab/NGI (Stockholm, Sweden).
RNA Extraction and Sequencing for Metatranscriptomes
Filters were placed in petri dishes, on ice, and cut into smaller wedges with scissors, then placed in MatrixE tubes (MPbio), along with RLT-buffer (Qiagen), TE-buffer, β-mercaptoethanol (0.1 %) and Lysozyme (0.04 mg mL−1). For the BAC samples, two vectors (pTZ19R, 546 bp and pFN18A, 970 bp) (Supplementary Material 1) at 0.1 ng μL−1 each, were added at this step to be used as internal standards, according to Satinsky et al. (2013). Cells were lysed using a FastPrep-24 instrument with a QuickPrep adaptor (MPbio), 3 rounds at 6 m s−1 for 40 s, with 1 min on ice in between, after which the Qiagen RNeasy mini kit was used for the extraction, according to protocol. The extracted RNA was treated with DNase to remove DNA (AMBIONTurbo DNA free, Invitrogen). The 6 samples intended for the BAC metatranscriptomes (0.2–3 μm size fraction) were rRNA depleted (RiboMinus Transcription isolation kit, Invitrogen) with a RiboMinus Concentration module, followed by cDNA to aRNA protocol (MessageAmp II-Bacteria RNA amplification kit, Invitrogen). To each of the 6 DNase treated samples intended for PE metatranscriptomes (3–90 μm size fraction) 0.02 pg spike-in ERCC Mix1 (Ambion) was added before they were sent for poly-A selection, which was followed by mRNA fragmentation and synthesis of cDNA, at SciLifeLab/NGI (Stockholm, Sweden). All 12 samples were then sequenced on one lane with Illumina HiSeq 2500 High Output mode v4, PE 2 × 125 bp, at SciLifeLab (Stockholm, Sweden).
Analysis of Sequencing Data
For the 16S and 18S gene amplicons, paired end sequences were analyzed in two separate rounds through the DADA2 pipeline, version 1.4.0 (Callahan et al., 2016) (available at https://github.com/benjjneb/dada2), implemented as a package in R, version 3.4.3. After the initial quality filtering 40% of the 16S reads and 25% of the 18S remained, resulting in 11 345 unique error corrected amplicon sequence variants (ASVs) for the 16S data and 29 642 unique error corrected ASVs for the 18S data (Supplementary Table 1). These were taxonomically assigned using Silva-128 db (Quast et al., 2013). To analyze the libraries, packages in R (version 3.4.1) were used, vegan (nMDS, beta-diversity) (Oksanen et al., 2008, 2019) and tidyR to sort ASVs by annotation, and ggplot2 for all plotting (Wickham, 2016).
Metatranscriptome
The PE and the BAC metatranscriptome data were handled separately but using a similar pipeline for both datasets. After the initial quality check with FASTQC (0.11.5) (Andrews, 2009), adapters were cut with cutadapt (1.13) (Martin, 2011), and ERNE mapping program (Del Fabbro et al., 2013) was used for trimming and filtering with a custom rRNA database, removing <1% of the reads, in both datasets (Supplementary Table 2). Reads were digitally normalized using Khmer (2.1) (Crusoe et al., 2015). Internal standards were identified and removed using blast+. Both paired and single reads were included in the assembly, made with Megahit (1.1.2) (Li et al., 2016). The reads were assigned taxonomy with RefSeq using Diamond (0.8.22) (Buchfink et al., 2015). Megan (6.10.0) (Huson et al., 2016), with SEED db (version May 2015; Overbeek et al., 2014) was used for assigning functional annotations. The raw reads were mapped to the assembly using Bowtie2 (2.3.2) (Langmead and Salzberg, 2012). Samtools (1.5) (Li et al., 2009) were used to retrieve counts (Supplementary Table 3). The data was analyzed using R (3.4.4) (Core, 2018).
Statistics and Internal Standards
Differential expression analyses were made for both the PE and BAC metatranscriptome data using the DESeq2 (Love et al., 2014) package in R (version 3.4.4), with the internal standards (controlGenes) in the estimateSizeFactors function to normalize counts between samples (Supplementary Table 3) (Beier et al., 2018). Transcriptional changes of enzymes refer to relative log2 fold changes between contrasted timepoints (including triplicates), at an adjusted p < 0.01, unless otherwise stated (Supplementary Tables 5, 7). PE transcript raw counts were normalized using transcripts per kilobase million (TPM) for relative abundance analysis (Wagner et al., 2012). Bacterial absolute counts were calculated from raw counts using the formula from Satinsky et al. (2013) and used for abundance analyses. The vegan package (Oksanen et al., 2008) was used for permanova analysis.
Data Availability
Raw amplicon and metatranscriptome sequencing reads in our study were deposited at the European Nucleotide Archive under study accession numbers ERP107850 and EPR109469, with sample accession numbers ERS2571542-ERS2571562 and ERS2572272-ERS2572283, respectively.
Results
Environmental Parameters
Samples were taken during a 2-week peak in sea surface temperature (SST), general for the whole Åland archipelago, Northern Baltic Sea (Supplementary Figure 1), with similar, warm temperatures at both sampling occasions (Table 1). Salinity, Chl-a and nitrate/nitrite levels were similar, while levels of inorganic phosphate decreased from T1 to T2 along with an increase of Si, and pH (Table 1). The bacterial abundance was slightly lower at T2 compared to T1. In all, the environmental parameters indicated stable environmental conditions during the studied period.
Microbial Community Composition and Characterization of Subcommunities
Phytoplankton
The phytoplankton community consisted mostly of large dinoflagellates that contributed the most biomass (≈70%) to the photototrophic community at both sampling occasions. The planktonic eukaryotes (PE) community biomass increased 4-fold from T1 (50 μg C L−1) to T2 (228 μg C L−1) (Figure 1) while the proportion of dinoflagellates and green algae/Euglenoids (20–29%) remained stable. Among dinoflagellates, the potentially toxic Alexandrium ostenfeldii dominated the PE biomass (46 % of total biomass) at T2. The number of counted photosynthetic taxa increased between T1 and T2, among dinoflagellates (see list in legend of Figure 1) but also the filamentous nitrogen fixing cyanobacteria (Anabaena/Dolichospermum, 2 μg C L−1), small flagellates (1 μg C L−1) and the mixotrophic Mesodinium rubrum-like ciliate (9 μg C L−1) were observed.
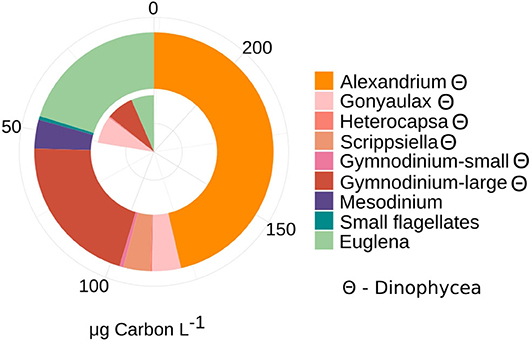
Figure 1. Patterns of phytoplankton and autotrophic ciliate Mesodinium-like community composition (>3 μm) and contribution to total biomass (μg Carbon L−1) at T1 and T2. Taxonomic composition was assessed by total microscopy counts and converted into biomass, using C conversion from cell to biomass as factors 0.17 for Cyanophycea (filamentous) and 0.15 for small phototrophic flagellates (Olenina et al., 2006). Carbon unit (pg C cell−1) were calculated based on cell volume for the genera Alexandrium (7,723 pg C cell−1), Gonyaulax (1,300 pg C cell−1), Heterocapsa (160 pg C cell−1), Scrippsiella (806 pg C cell−1), Gymnodinium (large: 1,730, small: 51 pg C cell−1), Euglena (120 pg C cell−1), Mesodinium (2,630 pg C cell−1) (Olenina et al., 2006). Θ denote taxa assigned to dinophycea (dinoflagellates). Twelve milliliter was counted in total at each date (T1 and T2).
Analyses of the PE (18S) amplicon sequence data with nMDS, showed a distinction between the communities at T1 and T2, though not significant (permAnova F = 5.76, R2 = 0.59, P < 0.1) (Supplementary Figure 2), while remaining at a similar diversity level [Shannon Index T1 (avg.) = 5.67, std = 0.26, T2 (avg.) = 5.87, std = 0.06] at respective timepoint (Supplementary Figure 2). contrary to direct observations, the amplicon data indicate that ciliates (Intramacronucleata) was the dominating group within the PE fraction, followed by dinoflagellates (Dinophyceae) (Figure 2). There were also lower levels of green algae (Chlorophyceae), cryptophytes (Cryptomonadales), diatoms (Diatomea), and parasitic dinoflagellates (Syndiniales). From sampling T1 to T2, there was a change in the ASVs assigned to ciliates from 46 to 36% in relative abundance, involving heterotrophic species of the class Prostomatea (higher at T2) and the orders Oligotrichida (lower at T2) while Choreotrichida remained at similar levels (Table 2). ASVs assigned to the class Dinophyceae (order Gonyaulacales e.g., Alexandrium ostenfeldii, Gonyaulax sp., subclass Gymnodiniphycidae e.g., Gymnodinium spp., order Peridiniales e.g., Heterocapsa triquetra, family Thoracosphaeraceae e.g., genus Scrippsiella, order Suessiaceae e.g., Symbiodinium) increased from 18 to 22% in relative abundance at T1 and T2, respectively (Table 2). This was paralleled by the occurrence of green algae at T1 (Tetracystis-like, 4%), and a higher occurrence of mixotrophic Cryptomonadales (2%) at T2 (Figure 2, Table 2).
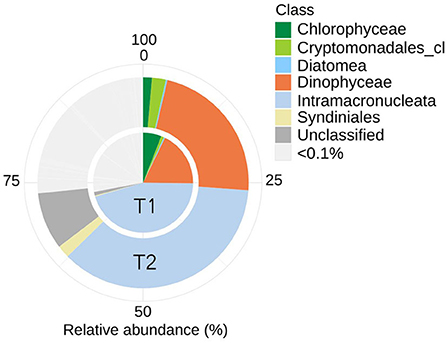
Figure 2. Eukaryotic community composition (18S rRNA) including ASVs with an average (of triplicates) relative abundance >0.1% per timepoint (T1 or T2). Assigned taxonomy at class level. Intramacronucleata is a subphylum of ciliates and consists of heterotrophic species.
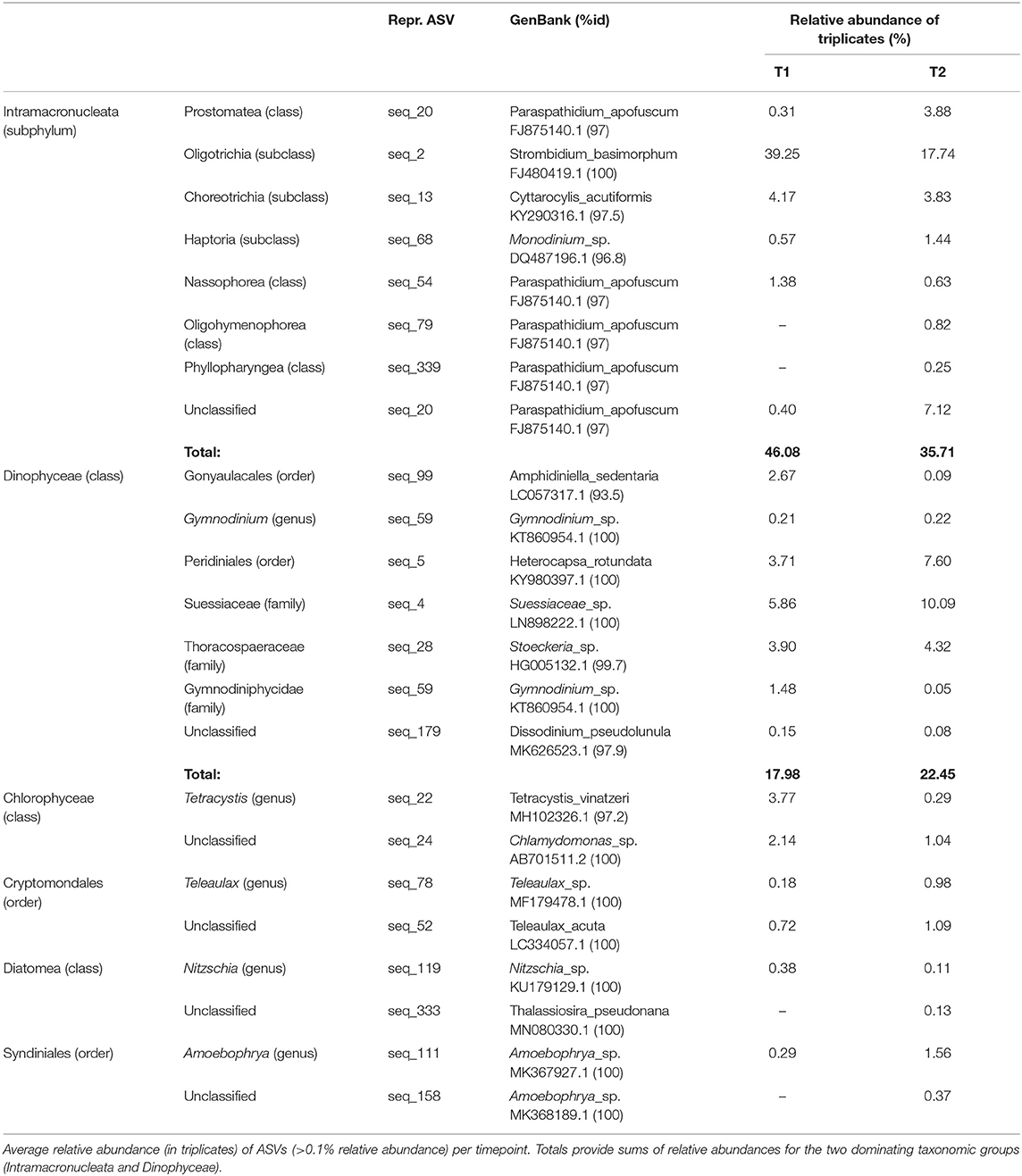
Table 2. Taxons, at two levels, of representative PE ASVs (18S), with accession numbers for matches in GenBank with % identity.
Bacteria
Bacterial abundance was similar (5–6 × 109 cells L−1, Table 1) at both sampling occasions. Taxonomic profiles of the domain Bacteria (BAC) in both the attached fraction (AT, > 3 μm) and the free-living fraction (FL, 0.2–3 μm) are visualized in Figure 3. Analysis with nMDS of BAC (16S) amplicon sequence data show a significant difference (permAnova F = 5.95, R2 = 0.33, P < 0.002**) between AT and FL (Supplementary Figure 3). However, within the same size fractions, no significant difference in BAC community composition was found between both sampling occasions: FL (T1 vs. T2): permAnova F = 4.72, R2 = 0.54, P < 0.1; AT (T1 vs. T2): permAnova F = 4.12, R2 = 0.51, P < 0.1. The FL fraction had a Shannon Index (avg.) = 6.41, std = 0.15, while AT (avg.) = 5.63, std = 0.57, indicating a higher diversity for the former (Supplementary Figure 3). The BAC community contained a large fraction of Actinobacteria (Acidimicrobiiaceae, Microbacteriaceae, Sporichtyaceae) mostly in the FL fraction, and Bacteroidetes (Cytophagaceae, Flavobacteriaceae, Sphingobacteriales), present in both the AT and the FL fractions (Figure 3, Table 3). The AT fraction was dominated by Gammaproteobacteria (Pseudomonadacea). Both Alpha- (Pelagibacterales, Rhodobacteraceae) and Betaproteobacteria (Alcaligenaceae, Comamonadaceae) were found in the AT and FL fractions, but they primarily occurred in the FL. There was a low occurrence of Verrucomicrobia (Opitutae) and Cyanobacteria in both size fractions (Figure 3, Table 3).
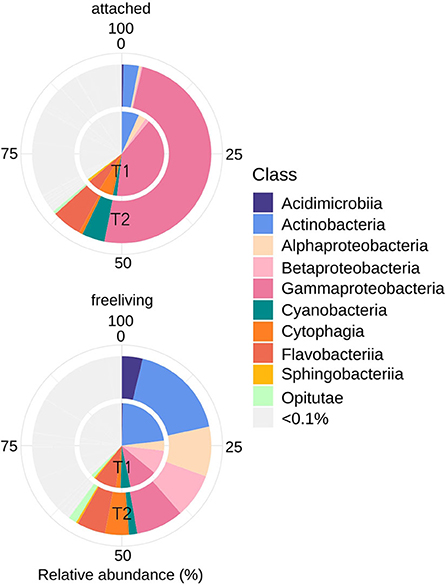
Figure 3. Bacterial community composition (16S rRNA) including ASVs with an average (of triplicates) relative abundance >0.1% per timepoint (T1 or T2). Assigned taxonomy at class level.
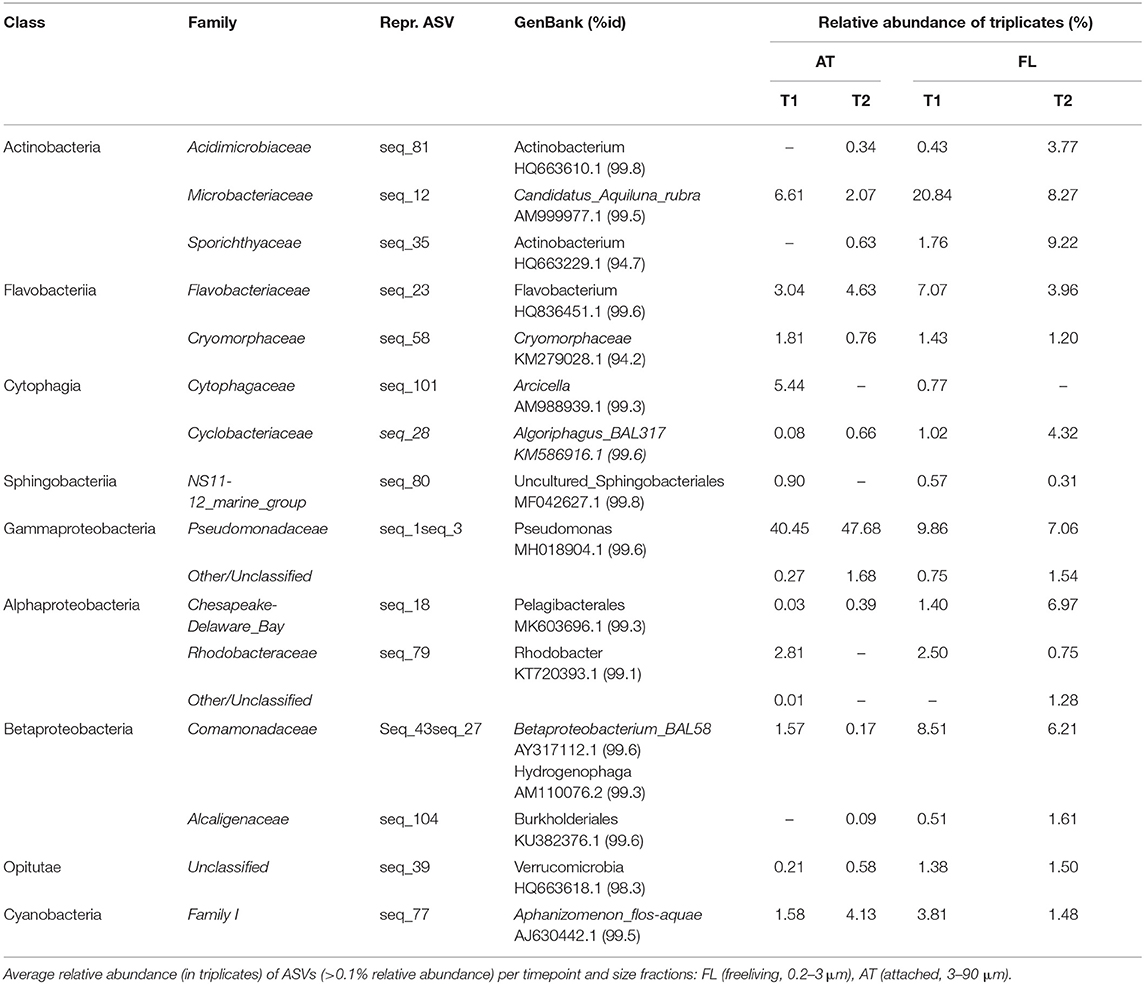
Table 3. Taxons, at class and family level, of representative BAC ASVs (16S), with accession numbers for matches in GenBank with % identity.
Microbial Community Functions
Metatranscriptome data from the PE and BAC parts of the community were analyzed to identify functional processes associated with uptake and assimilation of carbon, nitrogen and phosphorus, being the primary elements and nutrients utilized by both trophic levels. Differential expression (DE) analyses were made by contrasting the two timepoints (T1 and T2, in triplicates). For many of the ORFs assigned to the same functional annotation (SEED Rank1 and Rank3), the DE analysis resulted in a range of significant (padj < 0.01) log2 fold change values, spanning from positive to negative [Supplementary Tables 5 (PE), 7 (BAC)].
The taxonomic annotations of the PE metatranscriptome data did not correspond well with either the 18S rRNA gene amplicon annotations (Supplementary Figure 4) nor the microscopy counts at the family/genus level as only photosynthetic or potentially mixotrophic organisms were counted (Figure 1). Thus, no attempt was made to connect the identified PE functional processes with taxonomy. The taxonomy assigned to the BAC metatranscriptome data were more in accordance with the 16S rRNA gene amplicon results (Supplementary Figure 5), thus the assigned taxonomy from the BAC metatranscriptome was used together with that of the 16S rRNA gene amplicon-based annotation, to couple functional processes with taxonomy.
Carbon Utilization Pathways
Planktonic Eukaryotes (PE) expressed enzymes for uptake of both dissolved inorganic CO2 through carbon fixation, by the expression of ribulose bisphosphate carboxylase (EC4.1.1.39) (Rubisco), and organic carbon through the uptake, degradation and biosynthesis of amino acids, by the expression of branched-chain amino acid aminotransferase (EC2.6.1.42) and branched-chain acyl-CoA dehydrogenase (EC1.3.99.12), mainly at T1 (Figure 4A). At T1 there was likely uptake and utilization of the sugars inositol, through the expression of inositol transport system sugar-binding protein and myo-inositol2-dehydrogenase1 (EC1.1.1.18), and maltose, by the predicted maltose transporter MalT. The rate of C-fixation is determined by the activity of Rubisco (Beer et al., 1991; MacIntyre et al., 1997), which at SEED Rank3 level per timepoint, contained ORFs with both positive and negative log2 fold change values (padj < 0.01) (Supplementary Table 5), and the TPMs show very different levels of expression when comparing the replicates at each timepoints (Figure 4A). The expression analysis suggest that the rate of C-fixation was likely accelerated at T1 by the expression of carbonic anhydrase (EC4.2.1.1) (padj < 0.01) (Figure 4A). Expression levels of enzymes involved in glycolysis (NAD- and NADPH-dependent glyceraldehyde-3-phosphatedehydrogenase) (EC1.2.1.12, EC1.2.1.13) (padj < 0.01) and the pentose phosphate pathway [Fructose-bisphosphate aldolase (EC4.1.2.13), Fructose-1,6-bisphosphatase (EC3.1.3.11)] (padj < 0.01) (assimilation of carbon), indicate lower activity at T2 (Figure 4A). Also, the expression of manganese superoxide dismutase (EC1.15.1.1) (padj < 0.01) was lower at T2. Both inorganic and different organic carbon sources were utilized at a higher rate at T1 compared to T2.
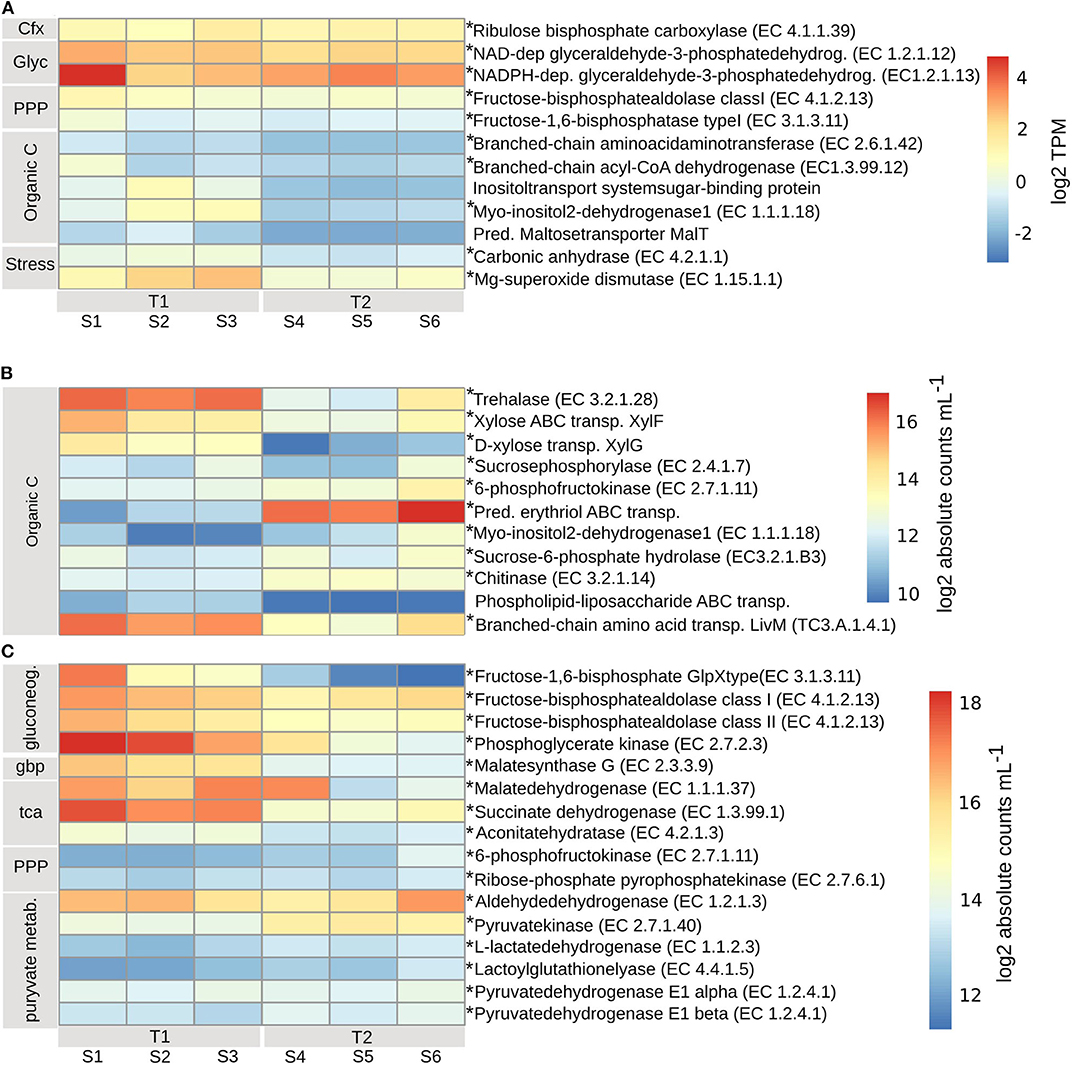
Figure 4. Transcripts involved in inorganic and organic carbon uptake and assimilation for (A) planktonic eukaryotes (PE), transcripts per kilobase million (TPM) (grouped by SEED Rank3) log2 transformed. TPM values with standard errors (SE) can be found in Supplementary Table 4. (B) bacterial (BAC) carbon uptake and utilization, (C) carbon cycling. Bacterial absolute transcript counts per mL (grouped by SEED Rank3), calculated according to formula in Satinsky et al. (2013) can be found in Supplementary Table 6. To account for transcript length, counts were normalized to one mRNA standard at a time: pTZ19R (546nt), pFN19R (970nt) from which the mean was calculated (Beier et al., 2018), and then log2 transformed. Bacterial absolute transcript counts per mL (grouped by SEED Rank3), with standard errors (SE), calculated according to formula in Satinsky et al. (2013) can be found in Supplementary Table 6. Asterisks indicate enzymes that significantly changed from T1 to T2 (adjusted p < 0.01). T1, first timepoint (samples S1–S3); T2, second timepoint (samples S4–S6); Cfx, carbon fixation; Glyc, glycolysis; PPP, pentose phosphate pathway; Organic C, organic carbon; Stress, response to nutrient stress; gluconeog, gluconeogenesis; gbp, glyoxylate bypass; tca, tca cycle; pyruvate metab, pyruvate metabolism.
At T1, the BAC community seemed to primarily utilize simpler carbon molecules (padj < 0.01), through the expression of trehalase (EC3.2.1.28) (Bacteroidetes), for the conversion of disaccharide trehalose into glucose; expression of high affinity ABC-transporter of monosaccharide xylose, XylF (Actinobacteria and Alphaproteobacteria), and expression of sucrosephosphorylase (EC2.4.1.7) (Actinobacteria, Betaproteobacteria, and Bacteroidetes), for uptake of disaccharide maltose (Figures 4B, 7A). At T2 the BAC mainly expressed (padj < 0.01) 6-phosphofructokinase (EC2.7.1.11) (Actinobacteria, Alpha- and Beta-proteobacteria, Bacteroidetes), for metabolism of linear monosaccharides tagatose and galactitol; predicted high-affinity ABC-transporter of the polyol erythritol (Alphaproteobacteria); myo-inositol-2-dehydrogenase (EC1.1.1.18) (Bacteroidetes and Gammaproteobacteria), for utilization of the polyol inositol; sucrose-6-phosphatehydrolase (EC3.2.1.B3) (Actinobacteria and Bacteroidetes), for metabolism of fructo-oligosaccharides and raffinose; and chitinase (EC3.2.1.14) (Bacteroidetes and other/unclassified), for utilization of high molecular weight chitin and its derivative acetyl glucosamine (Figures 4B, 7A). There was also higher expression of phospholipid-lipopolysaccharide ABC transporter (padj < 0.06) (Bacteroidetes and Cyanobacteria) and branched-chain amino acid transport permease protein LivM (TC3.A.1.4.1) (padj < 0.01) (Alpha-, Beta-, and Gamma-proteobacteria, Actinobacteria) at T1, compared to T2 (Figures 4B, 7A), for the possible uptake of alternative carbon sources.
The assimilation of carbon within the BAC community was significantly different at the two timepoints (padj < 0.01). At T1 both gluconeogenesis [fructose-1,6-bisphosphatase GlpX-type (EC3.1.3.11), fructose-bisphosphate aldolase class I & II (EC4.1.2.13) and phosphoglycerate kinase (EC2.7.2.3)] and the glyoxylate bypass (Malatesynthase G, EC2.3.3.9) were higher expressed than at T2, together accommodating for the use of other carbon sources than glucose, such as lipids and amino acids (Figure 4C). Also, the TCA-cycle [Malatedehydrogenase (EC1.1.1.37)], succinate dehydrogenase flavoprotein (EC1.3.99.1) and aconitatehydratase (EC4.2.1.3) was expressed at a higher level at T1 than at T2 (padj < 0.01) (Figure 4C). These processed were all seen in Actinobacteria, Alpha-, Beta-, and Gamma-proteobacteria, and Bacteroidetes to varying extents, while Cyanobacteria were only assigned to phosphoglycerate kinase and Acidimicrobiia to malatedehydrogenase (Figure 7B). The more complex molecules taken up at T2 seem to be channeled partly through the pentose phosphate pathway [6-phosphofructokinase (EC2.7.1.11), Ribose-phosphatepyrophosphokinase (EC2.7.6.1)] (Acidimicrobiia, Actinobacteria, Alpha-, Beta-, and Gamma-proteobacteria, and Bacteroidetes) followed by pyruvate metabolism: Aldehydedehydrogenase (EC1.2.1.3) and Pyruvatekinase (EC2.7.1.40), assigned to Actinobacteria, Alpha-, Beta-, and Gamma-proteobacteria, Cyanobacteria, and Bacteroidetes; L-lactatedehydrogenase (EC1.1.2.3) and lactoylglutathionelyase (EC4.4.1.5), assigned to primarily Alpha- and Beta-proteobacteria; Pyruvate dehydrogenase (EC1.2.4.1), subunits alpha and beta, assigned to primarily Bacteroidetes, Alphaproteobacteria, Cyanobacteria, and Actinobacteria. Both the pentose phosphate pathway and pyruvate metabolism associated enzymes were expressed at similar or slightly higher levels at T2 compared to T1 (padj < 0.01) (Figures 4C, 7B).
Nitrogen Acquisition
The PE expressed high levels of inorganic nitrogen transporters (padj < 0.01), for high-affinity uptake of ammonium (Ammonium transporter, Amt), methylammonium permease and nitrate/nitrite (Nrt) (Figure 5A). The expression of Amt and Nrt was higher at T1 than at T2, while methylammonium permease was higher at T2. Nitrogen assimilation was lower at T2 (padj < 0.01), and occurred via the activity of nitrite reductase (EC1.7.1.4), allantoicase (EC3.5.3.4), the urea cycle [acetylornithine aminotransferase (EC2.6.1.11) and argininosuccinate synthase (EC6.3.4.5)], biosynthesis of arginine and glutamine synthetase type II (EC6.3.1.2) (located in chloroplast) and type III (EC6.3.1.2) [located in mitochondrion (Siaut et al., 2007)] with the ability to assimilate (Takabayashi et al., 2005) or (Glibert et al., 2016), respectively (Figure 5A). Expressed were also enzymes involved in the decomposition of cyanate [Cyanate hydratase (EC4.2.1.104)], as a possible source of nitrogen, at both timepoints, albeit lower at T2 (padj < 0.01) (Figure 5A).
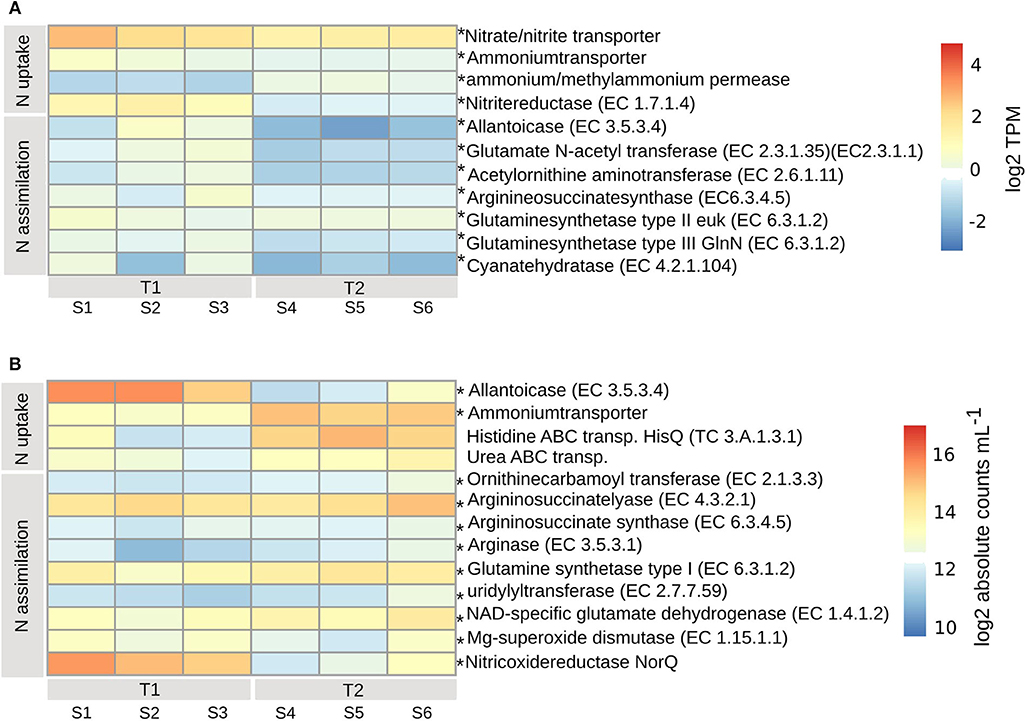
Figure 5. Transcripts involved in nitrogen uptake and assimilation for PE (A) and BAC (B), calculated according to legend in Figure 4. Asterisks indicate enzymes that were significantly changed from T1 to T2 (adjusted p < 0.01). T1, first timepoint (samples S1–S3); T2, second timepoint (samples S4–S6); N uptake, uptake of nitrogen; N assimilation, nitrogen assimilation.
The BAC expression pattern of enzymes associated with nitrogen uptake and assimilation suggest that different strategies dominated the two timepoints (Figure 5B). Initially, at T1, nitrogen was derived from degradation of nucleotides (purines), via the highly expressed allantoicase (EC3.5.3.4) (Pomati et al., 2001) (padj < 0.01) (Gammaproteobacteria) (Figure 7B). There was also expression of nitric oxide reductase (NorQ) (Other/Unclassified), and manganese superoxide dismutase (EC1.15.1.1) (Bacteroidetes, Cyanobacteria) (padj < 0.01) (Figures 5B, 7A), which both are involved in detoxification of NO, an intermediate from denitrification, resulting in nitrous oxide (N2O) or hydrogen peroxide (H2O2), respectively. In comparison, at T2 there was higher expression of high-affinity ammonium transporters (Amt) (padj < 0.01) (Actinobacteria, Alpha- and Beta-proteobacteria) together with lower expression of high-affinity ABC-transporters of urea (padj < 0.053) (Actinobacteria and Alphaproteobacteria) and the N-rich amino acid histidine (HisPQ) (TC3.A.1.3.1) (padj < 0.013) (Betaproteobacteria) (Figures 5B, 7A). Expression of both urea cycle and arginine biosynthesis related compounds [Ornithinecarbamoyltransferase (EC2.1.3.3), Argininosuccinatelyase (EC4.3.2.1), Argininosuccinatesynthase (EC6.3.4.5), Arginase (EC3.5.3.1)] were higher at T2 than at T1 (padj < 0.01) (Actinobacteria, Alpha- and Beta-proteobacteria, Bacteroidetes, and Cyanobacteria) (Figures 5B, 7A). The expression of glutamine synthetase (EC6.3.1.2) and NAD-specific glutamatedehydrogenase (EC1.4.1.2) was higher at T2 (padj < 0.01) (Actinobacteria, Alpha-, Beta-, and Gamma-proteobacteria, Cyanobacteria, and Bacteroidetes), along with its activator uridylyltransferase (EC2.7.7.59) (padj < 0.01) (Alpha-, Beta-, and Gamma-proteobacteria) (Figures 5B, 7A), together increasing the potential for ammonium assimilation.
Phosphorus Acquisition
The PE expressed both high and low affinity transporters for uptake of either inorganic or organic phosphorus. Expressed at both timepoints (padj < 0.01) was a probable low-affinity inorganic phosphate transporter (Figure 6A). The ABC transporters of less biologically available organic phosphate (TC3.A.1.7.1, PstS), phosphonate (TC3.A.1.9.1) (padj < 0.04) and oligopeptides (TC3.A.1.5.1, OppA) (padj < 0.01) were expressed at higher levels at T1 compared to T2, along with a sodium-dependent phosphate transporter (Figure 6A). A complementary strategy for the PE to obtain biologically available inorganic phosphorus, mainly at T1 but also at T2 (padj < 0.01), was through the expression of alkaline phosphatase (EC3.1.3.1), that transform extracellular dissolved organic phosphorus to inorganic phosphorus (Pi) for subsequent transporter mediated uptake. The likely sinks for intracellular inorganic phosphate, ubiquinone (EC7.1.1.2, EC1.6.99.3) and ATP-synthase (EC3.6.3.14), which are both part of the oxidative phosphorylation/electron transport chain, were higher in one replicate at T1, otherwise at similar or higher levels at T2, indicating a similar need for phosphorus at both timepoints (Figure 6A).
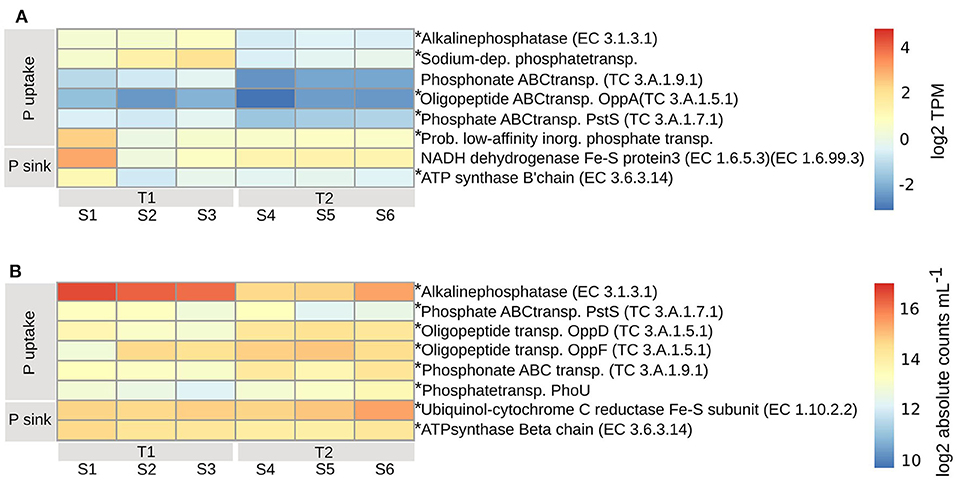
Figure 6. Transcripts involved in phosphorous uptake and assimilation for PE (A) and BAC (B), calculated according to legend in Figure 4. Asterisks indicate enzymes that were significantly changed from T1 to T2 (adjusted p < 0.01). T1, first timepoint (samples S1–S3); T2, second timepoint (samples S4–S6); P uptake, uptake of phosphorous; P sink, phosphorous utilization.
Among the BAC, alkaline phosphatase (EC3.1.3.1) (Actinobacteria, Alpha-, Beta-, and Gamma-proteobacteria, Cyanobacteria, and primarily Bacteroidetes) was expressed higher at T1 compared to T2 (padj < 0.01) while inorganic phosphate ABC transporter protein, PstS (TC3.A.1.7.1) (Acidimicrobiia, primarily Actinobacteria, Alpha-, Beta-, and Gamma-proteobacteria, Cyanobacteria, and Bacteroidetes), had a less distinct difference between the timepoints (padj < 0.01) (Figures 6B, 7A). At T2 oligopeptide ABC-transporter proteins, OppD and F (TC3.A.1.5.1) (Beta- and Gamma-proteobacteria, other/unclassified), were both expressed higher than at T1 (padj < 0.01), indicating uptake of organic phosphate. Also, phosphonate ABC-transporters (TC3.A.1.9.1) (Alphaproteobacteria, Cyanobacteria) were higher at T2 than T1 (padj < 0.01), along with phosphate transport system regulatory protein, PhoU (Actinobacteria, Alpha-, Beta-, and Gamma-proteobacteria) (Figures 6B, 7A), which is activated at low intracellular inorganic phosphate levels (Gardner et al., 2014). Both ATP-synthase (EC3.6.3.14) (Acidimicrobia, Actinobacteria, Alpha-, Beta-, and Gamma-proteobacteria, Cyanobacteria, and mainly Bacteroidetes) and ubiquinole (EC1.10.2.2) (Acidimicrobiia, Actinobacteria, Alpha-, Beta-, and Gamma-proteobacteria) were expressed at similar levels (padj < 0.01), indicating a similar need for energy production at both timepoints (Figures 6B, 7A).
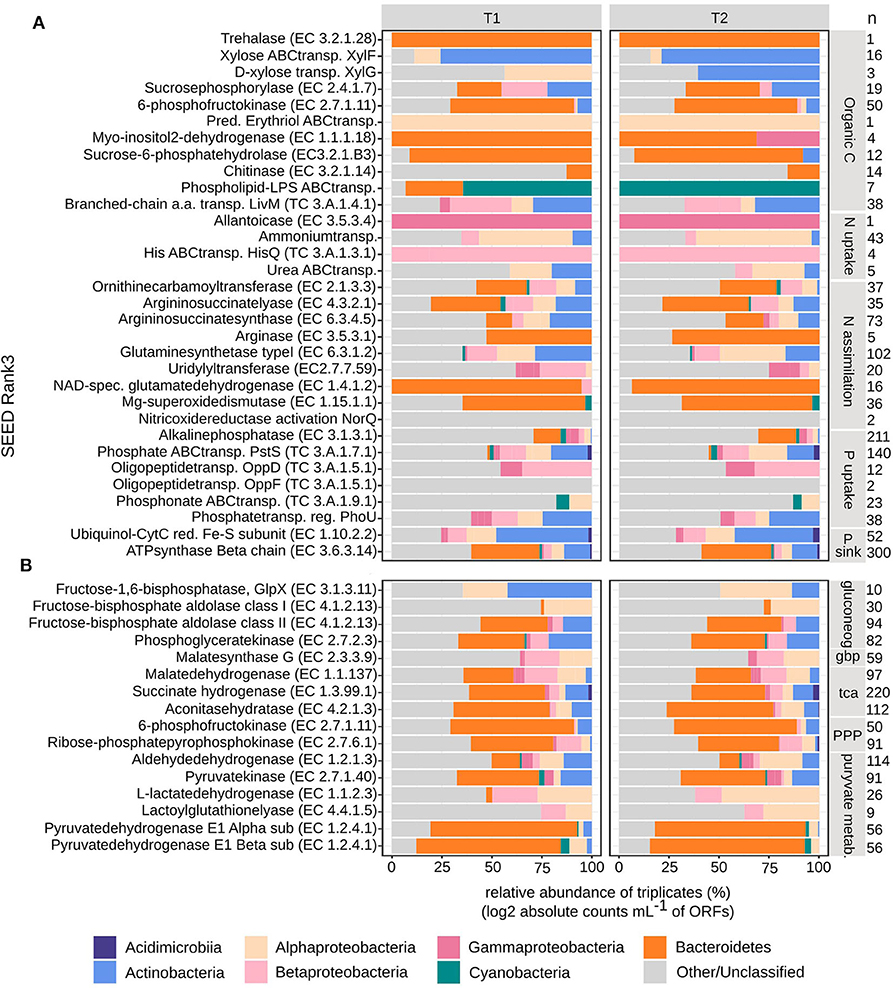
Figure 7. Taxonomical distribution of bacterial metatranscriptome ORFs grouped into SEED Rank3. Absolute counts mL−1 were log2 transformed and then relative abundances were calculated per timepoint (T1 and T2, in triplicates). Taxonomical groups are given at phylum or class level. (A) Carbon, nitrogen and phosphorous uptake and assimilation, (B) carbon cycling, n, signify the number of ORFs per Rank3 category.
Discussion
In this study we show that resources (organic and inorganic forms of C, N, and P) were partitioned between planktonic eukaryotes (PE) and heterotrophic bacteria (BAC). This indicates a broad functional ability for resource acquisition and utilization within a coastal microbial community at stable environmental conditions. Our findings also reveal that changes in resource partitioning between PE and BAC does not necessarily result in large-scale taxonomic shifts in the bacterial fraction. Previously, a decoupling between function and taxonomy among bacteria has been proposed (Louca et al., 2017, 2018), suggesting that the environmental conditions select for necessary metabolic functions leading to a specific, but unpredictable, taxonomic composition of the bacterial population. Partly contradicting this, our results indicate that both environmental (nutrient conditions) and biotic (plankton species present) conditions select which bacterial taxa, with suitable metabolic functions are found at the study site. This is exemplified by that members (Flavobacteriaceae and Comamonadaceae) of the previously suggested A. ostenfeldii core microbiome, isolated from the brackish Baltic Sea (Sörenson et al., 2019), being the dominating dinoflagellate species among the PE, were found to be frequent among the BAC, both in the free-living and the attached fractions. Further, our findings indicate that while only minor BAC taxonomic shifts occurred, in both size fractions, during the dinoflagellate bloom (T1: exponential growth, T2: stationary phase), the expression of functions both related to resource acquisition and to metabolic pathways varied in relation to the availability of carbon, nitrogen and phosphorus. This can be seen in the conceptual model in Figure 8, illustrating the relevant transcripts that has been more highly expressed at either T1 or T2, or remained at similar expression levels. When compared to systems with supposed weaker biotic interactions, such as bacteria found in bromeliad cups (Louca et al., 2017), our study suggests that the interactions between PE and BAC are of importance for which plankton and bacteria are found within the community and that resource partitioning and a functional flexibility are necessary in order to maintain these interactions at stable environmental conditions, such as those seen in this coastal bay. Resource partitioning, allowing different species to coexist both temporally and spatially by the division of available resources, has previously been shown to exist among organisms within planktonic microbial communities. Hunt et al. (2008) show that within a single bacterial family there are signs of broad resource partitioning, likely dependent on nutrient utilization and season. Also, among coexisting diatom species, different inorganic and organic nitrogen and phosphorus resources have been suggested to be partitioned (Alexander et al., 2015). Previous studies have focused either on the partitioning of carbon, between primary and secondary producers on a broad scale (encompassing viruses to fish) in the Baltic Sea (Sandberg et al., 2004), and among bacteria associated with different phases of a phytoplankton bloom (Landa et al., 2016; Berg et al., 2018), or of nitrogen and phosphorus (Alexander et al., 2015).
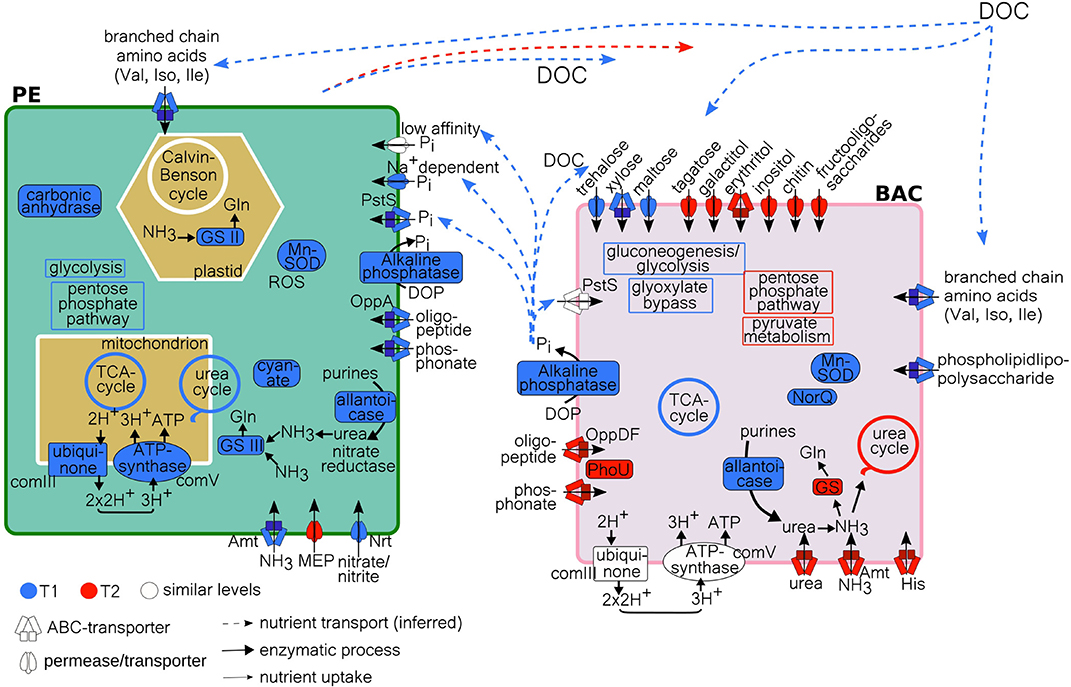
Figure 8. Model of PE and BAC C, N, and P processes at T1 and T2, with inferred nutrient transport of DOC and Pi, based on metatranscriptome data analysis. Uptake/transport by either high-affinity ABC-transporters, low-affinity transporters or permeases. Processes more highly expressed at T1 (blue), at T2 (red), or at similar levels (white), according to TPM (PE) and absolute counts (BAC) (Supplementary Tables 4, 6). GS, glutamine synthetase; MEP, methylammonium permease; DOC, dissolved organic carbon (allochtonous and autochtonous); Gln, glutamine; complex III and V, of oxidative phosphorylation/electron transport chain; Gln, glycine; Val, valine; Leu, leucine; Ile, isoleucine; His, histidine; ROS, reactive oxygen species; Mn-SOD, manganese sodium dismutase; NorQ, nitric oxide reductase Q; PE, planktonic eukaryotes; BAC, bacteria.
In this field study of a natural archipelagic environment, the microbial plankton community was dominated by mainly phototrophic dinoflagellates (Gonyaulacales, Peridiniales, Suessiaceae) including mixotrophic species, and heterotrophic ciliates (Oligotrichia, Choreotrichia) along with primarily heterotrophic bacteria [Actinobacteria (Microbacteriaceae, Sporichthyaceae), Bacteroidetes (Flavobacteriaceae, Cytophagaceae), Proteobacteria (Pseudomonadaceae, Pelagibacter, and Comamonadaceae)], in line with taxa frequently occurring in the Baltic Sea (Johansson et al., 2004; Riemann et al., 2008; Kremp et al., 2009; Herlemann et al., 2011; Wohlrab et al., 2018). Discrepancies in phytoplankton genus/taxa composition between light microscopy observations and 18S rRNA amplicon sequencing have been reported previously (Xiao et al., 2014) and illustrate the benefit of a combined approach. In our study, the discrepancies in PE community structure between observations using microscopy (dominance of Dinophycea) and those resulting from 18S rRNA (dominated by Intramacronucleata) could depend on that sequences in public database are still insufficient for identifying diverse eukaryotic microbes (Kataoka and Kondo, 2019) and that there are large differences in copy number of the 18S rRNA gene between ciliates (high copy number) and other protists such as dinoflagellates (Gong and Marchetti, 2019). Taking this into consideration, we did not pursue a function to taxonomy analysis of the PE community based on molecular results.
Carbon Partition
The functional analysis of the metatranscriptome indicated a partitioning in the acquisition and uptake of organic and inorganic carbon between the PE and BAC, which contribute to explaining the coexistence of both eukaryotes and prokaryotes (Figure 8). The microplankton PE part of the community was constituted of both phototrophic and heterotrophic taxa. Dinoflagellates were mainly phototrophic, including mixotrophic and primarily heterotrophic genera with kleptochloroplasts or algal endosymbionts (Stoecker, 1999; Gómez, 2012), while the ciliates mostly belonged to heterotrophic taxa (Johansson et al., 2004) and the mixotrophic Mesodinium rubrum (Lips and Lips, 2017). This explains the occurrence of both carbon fixation and assimilation of amino acids and uptake of sugars observed within this part of the community. During bloom development (T1), carbon uptake associated processes were highly expressed, indicating a larger need for both inorganic and organic carbon, suggesting a more rapidly growing community. This trend is supported by the higher expression of carbonic anhydrase at T1, known to accelerate the conversion to CO2 upon inorganic carbon limitation, caused by high levels of aerobic metabolism (Rost et al., 2006). The expression of carbonic anhydrase indicates an increased efficiency of carbon fixation leading to a reduction in oxygen production (Foyer and Harbinson, 1994). This would result in the formation of reactive oxygen species and their scavenger, manganese-superoxide dismutase. Both carbonic anhydrase and manganese-superoxide dismutase were expressed at higher levels at T1 compared to T2, an expression pattern previously seen during a dinoflagellate bloom peak (Zhuang et al., 2015). In addition, the number of 18S reads after quality filtration were lower at T1 (avg.) = 213,024, std = 15,201, compared to T2 (avg.) = 222,557, std = 3,330, pointing to a higher richness among the PE during T2, which indicate that the community had progressed through the more homogenous bloom phase (Sison-Mangus et al., 2016; Zhou J. et al., 2018). Taken together, this suggested that the PE community at T1 was sampled during an exponential growth phase while the community at T2 represents a more stationary growth phase.
In comparison, BAC had similar expression levels of uptake and assimilation of organic carbon at T1 and T2 (Figure 4C), indicating an actively growing community at both timepoints. Possibly, the difference between growth phases of the phytoplankton is reflected in the variety of organic carbon sources available to the heterotrophic bacteria. During active PE growth (T1), the BAC enzymes were associated with the utilization of the monosaccharides maltose and xylose and the disaccharide trehalose, while during PE slower growth (T2) the expression levels of organic carbon utilization mechanisms encompassed mono-, di- and tri-saccharides, two polyols, of which erythritol was especially highly expressed, and chitinase. The initial bacterial uptake of simple sugars appeared to go with the uptake of lipids and amino-acids, through the expression of phospholipid-lipopolysaccharide ABC transporter and branched-chain amino acid transport permease protein LivM. These carbon sources were likely channeled through gluconeogenesis and the glyoxylate bypass, more costly pathways that allow the conversion of proteins and lipids into glucose, which had higher expression levels at T1. Concomitantly, there was also a high occurrence of transcripts of components in the tricarboxylic acid cycle (TCA), likely providing glucose for growth, via pyruvate metabolism. This implies that during PE rapid growth, the need for carbon was higher also for the BAC, leading to a functional diversification to utilize several different variants of carbon.
While the PE, containing phototrophs, mixotrophs, and heterotrophs, utilizes both inorganic and simple organic carbon sources (amino-acids and sugars), the BAC has a wider functional repertoire to acquire varied forms of organic carbon, including amino-acids and a wide range of sugars, at both timepoints. During bloom development and active PE growth, when there are indications of a higher total energy demand (T1), there are however indications that the PE and BAC possibly compete for the same types of organic carbon (sugars and amino-acids), likely derived from both allochthonous sources and phytoplankton, though the BAC primarily utilizes other sugars than the PE. Taken together, this suggest that the PE and BAC partition the available organic carbon resources, primarily during PE active growth, in order to sustain growth and avoid competition for this valuable resource.
Nitrogen and Phosphorus Partition
Nutrient conditions in the Föglö archipelago indicated inorganic nitrogen (N) limitation and low phosphate concentrations, reflecting summer conditions in the shallow coastal Baltic Sea. In these conditions, phytoplankton and bacteria have to compete for limiting nutrients (Mayali and Doucette, 2002), and coexist despite competition for essential nutrient sources. Limiting levels of inorganic nutrients promote competition between trophic levels (Tilman, 1982), and the conditions in the bay, including the presence of mixotrophic eukaryotes, suggest that nutrient-based competition between eukaryotes and prokaryotes could be expected.
During bloom development (T1), along with a high carbon demand, the actively growing PE showed a high demand for the, likely limited resource, nitrogen. The PE expressed high-affinity N transporters for the inorganic N sources ammonium and nitrate/nitrite (Morey et al., 2011). This type of expression is indicative of low nutrient availability and these transporters have previously been seen to be upregulated in N starved diatoms (Alipanah et al., 2015). Another indication of the high N demand is the expression of cyanate hydratase (Figure 5A), shown to be related to limited nitrogen access (Alipanah et al., 2015). This expression coincides with expression of components for reduction of nitrite and N assimilation via the urea cycle. The expression of cyanate hydratase, primarily at T1, indicates that cyanate was also utilized as an organic N source. Cyanate has previously been observed to be taken up by dinoflagellates (Hu et al., 2012; Zhuang et al., 2015). This broad repertoire for accessing both inorganic and organic N has previously been reported for a coastal dinoflagellate bloom (Zhuang et al., 2015). In our study, the N uptake coincided with the expression of glutamine synthetase, both located in plastids and mitochondria, which is important for both nitrate and ammonium assimilation (Glibert et al., 2016). Expression of glutamine synthetase indicate limiting levels of internal nitrogen, and has previously been shown to be highly expressed in dinoflagellates during N stress (Parker and Armbrust, 2005; Morey et al., 2011; Zhuang et al., 2015). During PE slow growth (bloom stationary phase, T2), lower expression levels of these N assimilation enzymes with the addition of ammonium/methylammonium permease point to a lower N demand. As the PE community diversity increases at T2, a broadening of PE functions is likely, which could lead to a wider N uptake repertoire (Cardinale et al., 2006; Ptacnik et al., 2008). Nitrogen limited conditions seem to enforce the use of many strategies by the PE to access both organic and inorganic N, at both timepoints (Figure 8).
Nitrogen utilization mechanisms by aquatic bacteria in N-limited conditions have been much studied (Voss et al., 2013). Recent findings highlight the diverse N utilization strategies used by microbial communities to adapt to different N conditions and the ecological importance of the urea metabolism in N-limited regimes (Li et al., 2018). Our results confirm these findings as both the PE and BAC utilize a variation of strategies to acquire N. While the bacterial N demand was similar at both timepoints, the BAC expressed different N uptake mechanisms than the PE. Early in the bloom, the enzyme allantoicase was actively expressed, meeting the N demand by the intracellular formation of urea from purines (Solomon et al., 2010), as an organic N source. This process is known to occur in cyanobacteria and belongs to purine catabolism (Pomati et al., 2001; Solomon et al., 2010). Nitric oxide reductase activation protein NorQ, along with manganese superoxide dismutase, were also expressed in parallel, which suggests that both ammonium and nitrate transporters were inhibited by nitric oxide (Glibert et al., 2016). During late bloom (T2), direct acquisition of inorganic ammonium, organic urea, and amino acid histidine prevailed in the BAC N uptake strategy, of which all represent nitrogen species frequently associated with heterotrophic bacterial nutrient uptake (Kirchman, 1994; Fouilland et al., 2007).
Altogether, these results support a clear partition of the available N resources between PE and BAC at the different PE growth phases (Figure 8). Where the actively growing PE preferably utilize the scarce inorganic N sources and/or organic N-cyanate to sustain their growth, while the BAC is restricted to degrade organic purines in order to obtain nitrogen. In comparison, the PE, in a more stationary growth phase, utilize less inorganic N while the BAC switch to acquiring N from both inorganic and diverse organic extracellular resources. This illustrates how PE and BAC partition available N resources, in different ways depending on both the PE growth phase and likely the availability of different nitrogen species.
Phosphate is the preferred P substrate for photosynthetic and heterotrophic microbial growth in aquatic systems, and ambient phosphate concentrations were relatively high in the Föglö archipelago compared to the offshore Baltic Sea during summer (Savchuk, 2018). The rapid uptake of phosphate by phytoplankton, and to a minor extent by large macrophytes (e.g., Phragmites in this study) in the coastal zone, the subsequent production of dissolved organic phosphorus (DOP), and the P uptake (adsorption) by organic matter regulate the P availability for the microbial community (White and Dyhrman, 2013). At low phosphate levels, eukaryotic phytoplankton and heterotrophic bacteria can efficiently scavenge P from DOP through hydrolysis using alkaline phosphatase (AP) enzymes, which has been observed both in the coastal Baltic Sea and elsewhere (Chróst, 1991; Tanaka et al., 2006; Traving et al., 2017). Though, during bloom development (T1) in Föglö, high levels of AP were expressed among both BAC and in PE, suggesting limiting levels of internal Pi despite relatively high external Pi (PO4) levels (>0.2 μM). This could be reflecting high carbon and nitrogen turn-over (Baltar et al., 2016) in the coastal bay, rich in dissolved organic matter (DOM). Alternatively, as dissolved organic carbon (DOC) has a contributory effect on microbial AP response (Stewart et al., 1982), the release of simple sugars during PE active growth may trigger higher expression of AP among BAC (Anderson, 2018). Whether it is to alleviate bacterial P limitation or to satisfy BAC organic C demand is unclear. Dinoflagellates, that dominate the PE fraction, have a high diversity of AP genes that can confer diverse strategies to access and utilize DOP (Lin et al., 2012). Whether actively growing mixotrophic dinoflagellates would increase their AP response in relation to organic C sources and not P limitation is unknown. In addition, the PE expressed mechanisms for uptake of several inorganic and organic P sources during active growth, which was not seen in the BAC (Figure 8). This supports that some of the PO4 transformed by BAC-AP at T1 may be directly used by the PE, and that the BAC mainly used the resulting carbon products (Benitez-Nelson and Buesseler, 1999; Santos-Beneit, 2015). During late bloom (T2), the assumed lower eukaryotic C and N demand may link to the lower AP expression by PE and their expression of a low-affinity sodium dependent Pi transporter. The fact that BAC expressed uptake of two types of high-affinity organic P transporters, along with the expression of the Pi uptake repressor PhoU, suggests that there is a higher demand for organic P, but a lower demand for Pi at this timepoint (Santos-Beneit, 2015). Our findings demonstrate partitioning of the macronutrient P between PE and BAC, over bloom phase, by type (organic or inorganic) and nutrient species, beyond the direct coupling of composition and functional shifts.
Function and Community Composition
In the archipelago of Föglö, northern Baltic Proper, the taxonomic composition, based on amplicon sequencing analyses of both the PE and the BAC, did not vary significantly during the summer bloom while significant differences in resource acquisition were observed. The decoupling between bacterial community taxonomy and function previously suggested by Louca et al. (2016) has recently been evaluated experimentally (Louca et al., 2020) and showed that taxonomy shifts do not systematically affect metabolic rates. Our findings also point toward a decoupling between taxonomic composition and function in the field. For uptake of organic C, the same BAC taxa (Bacteroidetes, Actinobacteria, Alpha- and Beta-proteobacteria) initially utilize trehalose, xylose and maltose while during stationary bloom phase they instead utilize the more complex organic C compounds tagatose/galactitol, erythritol, inositol, raffinose, and chitin. The exception is gammaproteobacterial expression associated with uptake of inositol seen only during the later bloom stage. Traditionally, Bacteroidetes and Actinobacteria are correlated to algal blooms conditions (Riemann et al., 2008) and are often associated to late bloom phase due to their metabolic capacity to degrade HMW carbon compounds (Pinhassi et al., 1997) or polyaromatic compounds such as chitin (Beier and Bertilsson, 2013). This traditional view may perhaps not be so clear cut at this coastal location. Regarding BAC uptake of N, the results suggest that distinct organic and inorganic N resources were acquired and utilized during the two bloom phases. While these transcripts were assigned to several different BAC taxa, the same groups were represented throughout the bloom (Bacteroidetes, Actinobacteria, Cyanobacteria, Alpha-, Beta-, and Gamma-proteobacteria). Similarly, the transcripts associated with BAC acquisition and metabolism of P partly varied between the bloom phases, primarily with higher expression of alkaline phosphatase during the initial stage. These processes were annotated to a variety of BAC taxa, which were all represented during the whole bloom (Acidimicrobiia, Actinobacteria, Bacteroidetes, Cyanobacteria, Alpha-, Beta-, and Gamma-proteobacteria). Taken together, these results suggest that the community composition of BAC in association with a PE bloom is stable despite distinct metabolic and functional variations in C, N, and P resource usage. Thus, a decoupling between taxonomy and function is clear, it does however seem to be dependent on both the abiotic (availability of nutrients) and biotic (PE and BAC interactions) conditions in the bay.
Conclusions
In this eutrophied archipelagic environment, with high organic:inorganic nutrient ratio, interactions in the form of competition for highly desirable inorganic (nitrate, nitrite, ammonium, Pi) and organic (sugars, amino acids, oligo-peptides, phosphonates, urea, cyanate) nutrients, was characterized by an observed resource partitioning between PE (primarily phototrophs and mixotrophs) and BAC (primarily heterotrophs). This pattern was evident both when the PE were in exponential and stationary phase, implying that uptake and assimilation of resources may be partitioned regardless of growth stage. This resulted in a range of metabolic functions, dependent on the resources available, which did not result in taxonomic shifts, suggesting a decoupling of function from taxonomy among BAC. This study shows the importance of BAC remineralization of allochthonous organic nutrients and of how PE and BAC divide available C, N, and P species, both in type and over time. At unbalanced nutrient conditions, these mechanisms likely enable phytoplankton growth, and even the development of blooms. This study reveals the potential importance of mixotrophic nutrition of PE in brackish coastal systems, which is still not taken into account in biogeochemical models. The results provide insight into how phytoplankton and bacteria in natural communities interact, and opens up for new questions such as how are resources partitioned at low organic:inorganic nutrient ratio, and what effect would a reduction of land run-off have on the microbial community and the nutrient distribution?
Data Availability Statement
The datasets presented in this study can be found in online repositories. The names of the repository/repositories and accession number(s) can be found at: https://www.ebi.ac.uk/ena, ERS2571542-ERS2571562; https://www.ebi.ac.uk/ena, ERS2572272-ERS2572283; https://www.ebi.ac.uk/ena, PRJEB25883; https://www.ebi.ac.uk/ena, PRJEB27395.
Author Contributions
ES designed the study, performed the sampling together with EL, handled all bioinformatic work, and led the writing of the manuscript, to which all authors contributed. ES, EL, and HF performed the laboratory work. All authors contributed to the interpretation of the results and the methods used for analysis.
Funding
The study was supported by funding from the Strategic Research Environment-ECOCHANGE funded by the Swedish Research Council Formas (CL), the Marie Skłodowska-Curie grant, Grant/Award Number: 659453 (EL), the Linnaeus University Centre of Ecology and Evolution in Microbial Model Systems (EEMiS) (CL and HF).
Conflict of Interest
The authors declare that the research was conducted in the absence of any commercial or financial relationships that could be construed as a potential conflict of interest.
Acknowledgments
The authors are grateful to Moritz Buck, at the National Bioinformatics Infrastructure Sweden at SciLifeLab, for bioinformatic advice, and Adina Howe, at Iowa State University, and Sara Beier, at Leibniz Institute for Baltic Sea Research, for computational support (AH) and advice (SB) on the calculations for use of internal standards with the metatranscriptome data. The authors acknowledge support from the National Genomics Infrastructure in Stockholm funded by Science for Life Laboratory, the Knut and Alice Wallenberg Foundation and the Swedish Research Council, and SNIC/Uppsala Multidisciplinary Center for Advanced Computational Science for assistance with massively parallel sequencing and access to the UPPMAX computational infrastructure.
Supplementary Material
The Supplementary Material for this article can be found online at: https://www.frontiersin.org/articles/10.3389/fmars.2020.608244/full#supplementary-material
References
Alexander, H., Jenkins, B. D., Rynearson, T. A., and Dyhrman, S. T. (2015). Metatranscriptome analyses indicate resource partitioning between diatoms in the field. Proc. Natl. Acad. Sci. U.S.A. 112, E2182–E2190. doi: 10.1073/pnas.1421993112
Alipanah, L., Rohloff, J., Winge, P., Bones, A. M., and Brembu, T. (2015). Whole-cell response to nitrogen deprivation in the diatom Phaeodactylum tricornutum. J. Exp. Bot. 66, 6281–6296. doi: 10.1093/jxb/erv340
Anderson, O. R. (2018). Evidence for coupling of the carbon and phosphorus biogeochemical cycles in freshwater microbial communities. Front. Mar. Sci. 5, 1–6. doi: 10.3389/fmars.2018.00020
Andersson, A., Meier, H. E. M., Ripszam, M., Rowe, O., Wikner, J., Haglund, P., et al. (2015). Projected future climate change and Baltic sea ecosystem management. Ambio 44(Suppl. 3), 345–356. doi: 10.1007/s13280-015-0654-8
Andrews, S. (2009). FastQC. A Quality Control Tool for High Throughput Sequence Data. Available online at: http://www.bioinformatics.babraham.ac.uk/projects/fastqc/
Bagatini, I. L., Eiler, A., Bertilsson, S., Klaveness, D., Tessarolli, L. P., and Vieira, A. A. H. (2014). Host-specificity and dynamics in bacterial communities associated with bloom-forming freshwater phytoplankton. PLoS ONE 9:e85950. doi: 10.1371/journal.pone.0085950
Baltar, F., Lundin, D., Palovaara, J., Lekunberri, I., Reinthaler, T., Herndl, G. J., et al. (2016). Prokaryotic responses to ammonium and organic carbon reveal alternative CO2 fixation pathways and importance of alkaline phosphatase in the mesopelagic North Atlantic. Front. Microbiol. 7:1670. doi: 10.3389/fmicb.2016.01670
Beer, S., Sand-Jensen, K., Madsen, T. V., and Nielsen, S. L. (1991). The carboxylase activity of Rubisco and the photosynthetic performance in aquatic plants. Oecologia 87, 429–434. doi: 10.1007/BF00634602
Beier, S., and Bertilsson, S. (2013). Bacterial chitin degradation-mechanisms and ecophysiological strategies. Front. Microbiol. 4:149. doi: 10.3389/fmicb.2013.00149
Beier, S., Holtermann, P., Numberger, D., Schott, T., Umlauf, L., and Jürgens, K. (2018). A metatranscriptomics based assessment of small scale mixing of sulfidic and oxic waters on redoxcline prokaryotic communities. Environ. Microbiol. 21, 1462–2920. doi: 10.1111/1462-2920.14499
Benitez-Nelson, C. R., and Buesseler, K. O. (1999). Variability of inorganic and organic phosphorus turnover rates in the coastal ocean. Nature 398, 502–505. doi: 10.1038/19061
Berg, C., Dupont, C. L., Asplund-Samuelsson, J., Celepli, N. A., Eiler, A., Allen, A. E., et al. (2018). Dissection of microbial community functions during a cyanobacterial bloom in the Baltic sea via metatranscriptomics. Front. Mar. Sci. 5:55. doi: 10.3389/fmars.2018.00055
Bonsdorff, E., Blomqvist, E. M., Mattila, J., and Norkko, A. (1997). Coastal eutrophication: causes, consequences and perspectives in the Archipelago areas of the northern Baltic sea. Estuar. Coast. Shelf Sci. 44, 63–72. doi: 10.1016/S0272-7714(97)80008-X
Boström, K. H., Simu, K., Hagström, Å., and Riemann, L. (2004). Optimization of DNA extraction for quantitative marine bacterioplankton community analysis. Limnol. Oceanogr. Methods 2, 365–373. doi: 10.4319/lom.2004.2.365
Buchfink, B., Xie, C., and Huson, D. H. (2015). Fast and sensitive protein alignment using DIAMOND. Nat. Methods 12, 59–60. doi: 10.1038/nmeth.3176
Bunse, C., Bertos-Fortis, M., Sassenhagen, I., Sildever, S., Sjöqvist, C., Godhe, A., et al. (2016). Spatio-temporal interdependence of bacteria and phytoplankton during a Baltic sea spring bloom. Front. Microbiol. 7:517. doi: 10.3389/fmicb.2016.00517
Callahan, B. J., McMurdie, P. J., Rosen, M. J., Han, A. W., Johnson, A. J. A., and Holmes, S. P. (2016). DADA2: high-resolution sample inference from Illumina amplicon data. Nat. Methods 13, 581–583. doi: 10.1038/nmeth.3869
Camarena-Gómez, M. T., Lipsewers, T., Piiparinen, J., Eronen-Rasimus, E., Perez-Quemaliños, D., Hoikkala, L., et al. (2018). Shifts in phytoplankton community structure modify bacterial production, abundance and community composition. Aquat. Microb. Ecol. 81, 149–170. doi: 10.3354/ame01868
Cardinale, B. J., Srivastava, D. S., Emmett Duffy, J., Wright, J. P., Downing, A. L., Sankaran, M., et al. (2006). Effects of biodiversity on the functioning of trophic groups and ecosystems. Nature 443, 989–992. doi: 10.1038/nature05202
Carstensen, J., Conley, D. J., Almroth-Rosell, E., Asmala, E., Bonsdorff, E., Fleming-Lehtinen, V., et al. (2019). Factors regulating the coastal nutrient filter in the Baltic sea. Ambio 49, 1194–1210. doi: 10.1007/s13280-019-01282-y
Chróst, R. J. (Ed.). (1991). “Environmental control of the synthesis and activity of aquatic microbial ectoenzymes,” in Microbial Enzymes in Aquatic Environments (New York, NY: Springer Verlag), 29–59.
Core, T. R. (2018). R: A Language and Environment for Statistical Computing. Available online at: https://www.r-project.org/
Cottrell, M. T., and Kirchman, D. L. (2000). Natural assemblages of marine proteobacteria and members of the cytophaga-flavobacter cluster consuming low- and high-molecular-weight dissolved organic matter. Appl. Environ. Microbiol. 66, 1692–1697. doi: 10.1128/AEM.66.4.1692-1697.2000
Crusoe, M. R., Alameldin, H. F., Awad, S., Boucher, E., Caldwell, A., Cartwright, R., et al. (2015). The khmer software package: enabling efficient nucleotide sequence analysis. F1000Research 4:900. doi: 10.12688/f1000research.6924.1
Del Fabbro, C., Scalabrin, S., Morgante, M., and Giorgi, F. M. (2013). An extensive evaluation of read trimming effects on illumina NGS data analysis. PLoS ONE 8:e85024. doi: 10.1371/journal.pone.0085024
Diner, R. E., Schwenck, S. M., McCrow, J. P., Zheng, H., and Allen, A. E. (2016). Genetic manipulation of competition for nitrate between heterotrophic bacteria and diatoms. Front. Microbiol. 7:880. doi: 10.3389/fmicb.2016.00880
Dupont, C. L., McCrow, J. P., Valas, R., Moustafa, A., Walworth, N., Goodenough, U., et al. (2015). Genomes and gene expression across light and productivity gradients in eastern subtropical Pacific microbial communities. ISME J. 9, 1076–1092. doi: 10.1038/ismej.2014.198
Elifantz, H., Malmstrom, R. R., Cottrell, M. T., and Kirchman, D. L. (2005). Assimilation of polysaccharides and glucose by major bacterial groups in the Delaware Estuary. Appl. Environ. Microbiol. 71, 7799–7805. doi: 10.1128/AEM.71.12.7799-7805.2005
Fouilland, E., Gosselin, M., Rivkin, R. B., Vasseur, C., and Mostajir, B. (2007). Nitrogen uptake by heterotrophic bacteria and phytoplankton in Arctic surface waters. J. Plankton Res. 29, 369–376. doi: 10.1093/plankt/fbm022
Foyer, C., and Harbinson, J. (1994). “Oxygen metabolism and the regulation of photosynthetic electron transport.,” in Causes of Photooxidative Stress and Amelioration of Defense Systems in Plant, eds C. Foyer and P. Mullineaux (Boca Raton, FL: CRC Press), 1–42.
Gardner, S. G., Johns, K. D., Tanner, R., and McCleary, W. R. (2014). The PhoU protein from Escherichia coli interacts with PhoR, PstB, and metals to form a phosphate-signaling complex at the membrane. J. Bacteriol. 196, 1741–1752. doi: 10.1128/JB.00029-14
Gifford, S. M., Sharma, S., Booth, M., and Moran, M. A. (2013). Expression patterns reveal niche diversification in a marine microbial assemblage. ISME J. 7, 281–298. doi: 10.1038/ismej.2012.96
Glibert, P. M., Wilkerson, F. P., Dugdale, R. C., Raven, J. A., Dupont, C. L., Leavitt, P. R., et al. (2016). Pluses and minuses of ammonium and nitrate uptake and assimilation by phytoplankton and implications for productivity and community composition, with emphasis on nitrogen-enriched conditions. Limnol. Oceanogr. 61, 165–197. doi: 10.1002/lno.10203
Gómez, F. (2012). A quantitative review of the lifestyle, habitat and trophic diversity of dinoflagellates (Dinoflagellata, Alveolata). Syst. Biodivers. 10, 267–275. doi: 10.1080/14772000.2012.721021
Gong, W., and Marchetti, A. (2019). Estimation of 18S gene copy number in marine eukaryotic plankton using a next-generation sequencing approach. Front. Mar. Sci. 6:219. doi: 10.3389/fmars.2019.00219
Granéli, E., Wallström, K., Larsson, U., Granéli, W., and Elmgren, R. (1990). Nutrient limitation of primary production in the Baltic sea. Ambio 13, 142–151.
Grossart, H.-P., Levold, F., Allgaier, M., Simon, M., and Brinkhoff, T. (2005). Marine diatom species harbour distinct bacterial communities. Environ. Microbiol. 7, 860–873. doi: 10.1111/j.1462-2920.2005.00759.x
Grossart, H. P., and Simon, M. (2007). Interactions of planktonic algae and bacteria: effects on algal growth and organic matter dynamics. Aquat. Microb. Ecol. 47, 163–176. doi: 10.3354/ame047163
Hällfors, G. (2004). Checklist of Baltic Sea Phytoplankton Species. Helsinki Commission; Baltic Marine Environment protection Commission. Available online at: http://helcom.fi/Lists/Publications/BSEP95.pdf
Heiskanen, A. S., Bonsdorff, E., and Joas, M. (2019). “Baltic Sea: a recovering future from decades of eutrophication,” in Coasts and Estuaries, eds E. Wolanski, J. Day, M. Elliott, and R. Ramesh (Amsterdam: Elsevier), 343–362. doi: 10.1016/b978-0-12-814003-1.00020-4
Herlemann, D. P., Labrenz, M., Jürgens, K., Bertilsson, S., Waniek, J. J., and Andersson, A. F. (2011). Transitions in bacterial communities along the 2000 km salinity gradient of the Baltic sea. ISME J. 5, 1571–1579. doi: 10.1038/ismej.2011.41
Hu, Z., Mulholland, M. R., Duan, S., and Xu, N. (2012). Effects of nitrogen supply and its composition on the growth of Prorocentrum donghaiense. Harmful Algae 13, 72–82. doi: 10.1016/j.hal.2011.10.004
Hugerth, L. W., Muller, E. E. L., Hu, Y. O. O., Lebrun, L. A. M., Roume, H., Lundin, D., et al. (2014). Systematic Design of 18S rRNA gene primers for determining eukaryotic diversity in microbial consortia. PLoS ONE 9:e95567. doi: 10.1371/journal.pone.0095567
Hunt, D. E., David, L. A., Gevers, D., Preheim, S. P., Alm, E. J., and Polz, M. F. (2008). Resource partitioning and sympatric differentiation among closely related bacterioplankton. Science 320, 1081–1085. doi: 10.1126/science.1157890
Huson, D. H., Beier, S., Flade, I., Górska, A., El-Hadidi, M., Mitra, S., et al. (2016). MEGAN community edition - interactive exploration and analysis of large-scale microbiome sequencing data. PLOS Comput. Biol. 12:e1004957. doi: 10.1371/journal.pcbi.1004957
Jespersen, A., and Christoffersen, K. (1987). Measurements of chlorophyll-a from phytoplankton using ethanol as extraction solvent. Arch. Hydrobiol. 109, 445–454.
Johansson, M., Gorokhova, E., and Larsson, U. (2004). Annual variability in ciliate community structure, potential prey and predators in the open northern Baltic sea proper. J. Plankton Res. 26, 67–80. doi: 10.1093/plankt/fbg115
Kataoka, T., and Kondo, R. (2019). Data on taxonomic annotation and diversity of 18S rRNA gene amplicon libraries derived from high throughput sequencing. Data Br. 25:104213. doi: 10.1016/j.dib.2019.104213
Kirchman, D. L. (1994). The uptake of inorganic nutrients by heterotrophic bacteria. Microb. Ecol. 28, 255–271. doi: 10.1007/BF00166816
Kivi, K., and Setäl, O. (1995). Simultaneous measurement of food particle selection and clearance rates of planktonic oligotrich ciliates (Ciliophora:Oligotrichina). Mar. Ecol. Prog. Ser. 119, 125–137. doi: 10.3354/meps119125
Kremp, A., Lindholm, T., Dreßler, N., Erler, K., Gerdts, G., Eirtovaara, S., et al. (2009). Bloom forming Alexandrium ostenfeldii (Dinophyceae) in shallow waters of the Åland Archipelago, Northern Baltic sea. Harmful Algae 8, 318–328. doi: 10.1016/j.hal.2008.07.004
Landa, M., Blain, S., Christaki, U., Monchy, S., and Obernosterer, I. (2016). Shifts in bacterial community composition associated with increased carbon cycling in a mosaic of phytoplankton blooms. ISME J. 10, 39–50. doi: 10.1038/ismej.2015.105
Langmead, B., and Salzberg, S. L. (2012). Fast gapped-read alignment with Bowtie 2. Nat. Methods 9, 357–359. doi: 10.1038/nmeth.1923
Lauro, F. M., McDougald, D., Thomas, T., Williams, T. J., Egan, S., Rice, S., et al. (2009). The genomic basis of trophic strategy in marine bacteria. Proc. Natl. Acad. Sci. U.S.A. 106, 15527–15533. doi: 10.1073/pnas.0903507106
Li, D., Luo, R., Liu, C.-M., Leung, C.-M., Ting, H.-F., Sadakane, K., et al. (2016). MEGAHIT v1.0: a fast and scalable metagenome assembler driven by advanced methodologies and community practices. Methods 102, 3–11. doi: 10.1016/j.ymeth.2016.02.020
Li, H., Handsaker, B., Wysoker, A., Fennell, T., Ruan, J., Homer, N., et al. (2009). The sequence alignment/map format and SAMtools. Bioinformatics 25, 2078–2079. doi: 10.1093/bioinformatics/btp352
Li, Y.-Y., Chen, X.-H., Xie, Z.-X., Li, D.-X., Wu, P.-F., Kong, L.-F., et al. (2018). Bacterial diversity and nitrogen utilization strategies in the upper layer of the Northwestern Pacific Ocean. Front. Microbiol. 9:797. doi: 10.3389/fmicb.2018.00797
Lin, X., Zhang, H., Cui, Y., and Lin, S. (2012). High sequence variability, diverse subcellular localizations, and ecological implications of alkaline phosphatase in dinoflagellates and other eukaryotic phytoplankton. Front. Microbiol. 3:235. doi: 10.3389/fmicb.2012.00235
Lips, I., and Lips, U. (2017). The importance of Mesodinium rubrum at post-spring bloom nutrient and phytoplankton dynamics in the vertically stratified Baltic sea. Front. Mar. Sci. 4:407. doi: 10.3389/fmars.2017.00407
Louca, S., Jacques, S. M. S., Pires, A. P. F., Leal, J. S., Srivastava, D. S., Parfrey, L. W., et al. (2017). High taxonomic variability despite stable functional structure across microbial communities. Nat. Ecol. Evol. 1:15. doi: 10.1038/s41559-016-0015
Louca, S., Parfrey, L. W., and Doebeli, M. (2016). Decoupling function and taxonomy in the global ocean microbiome. Science 353, 1272–1277. doi: 10.1126/science.aaf4507
Louca, S., Polz, M. F., Mazel, F., Albright, M. B. N., Huber, J. A., O'Connor, M. I., et al. (2018). Function and functional redundancy in microbial systems. Nat. Ecol. Evol. 2, 936–943. doi: 10.1038/s41559-018-0519-1
Louca, S., Rubin, I. N., Madilao, L. L., Bohlmann, J., Doebeli, M., and Wegener Parfrey, L. (2020). Effects of forced taxonomic transitions on metabolic composition and function in microbial microcosms. Environ. Microbiol. Rep. 12, 514–524. doi: 10.1111/1758-2229.12866
Love, M. I., Huber, W., and Anders, S. (2014). Moderated estimation of fold change and dispersion for RNA-seq data with DESeq2. Genome Biol. 15:550. doi: 10.1186/s13059-014-0550-8
MacIntyre, H. L., Sharkey, T. D., and Geider, R. J. (1997). Activation and deactivation of ribulose- 1,5-bisphosphate carboxylase/oxygenase (Rubisco) in three marine microalgae. Photosynth. Res. 51, 93–106. doi: 10.1023/A:1005755621305
Martin, M. (2011). Cutadapt removes adapter sequences from high-throughput sequencing reads. EMBnet J. 17, 10–12. doi: 10.14806/ej.17.1.200
Mayali, X., and Doucette, G. J. (2002). Microbial community interactions and population dynamics of an algicidal bacterium active against Karenia brevis (Dinophyceae). Harmful Algae 1, 277–293. doi: 10.1016/S1568-9883(02)00032-X
McCarren, J., Becker, J. W., Repeta, D. J., Shi, Y., Young, C. R., Malmstrom, R. R., et al. (2010). Microbial community transcriptomes reveal microbes and metabolic pathways associated with dissolved organic matter turnover in the sea. Proc. Natl. Acad. Sci. U.S.A. 107, 16420–16427. doi: 10.1073/pnas.1010732107
Mironova, E. I., Telesh, I. V., and Skarlato, S. O. (2009). Planktonic ciliates of the Baltic sea (a review). Inl. Water Biol. 2, 13–24. doi: 10.1134/S1995082909010039
Morey, J. S., Monroe, E. A., Kinney, A. L., Beal, M., Johnson, J. G., Hitchcock, G. L., et al. (2011). Transcriptomic response of the red tide dinoflagellate, Karenia brevis, to nitrogen and phosphorus depletion and addition. BMC Genomics 12:346. doi: 10.1186/1471-2164-12-346
Mühlenbruch, M., Grossart, H.-P., Eigemann, F., and Voss, M. (2018). Mini-review: Phytoplankton-derived polysaccharides in the marine environment and their interactions with heterotrophic bacteria. Environ. Microbiol. 20, 2671–2685. doi: 10.1111/1462-2920.14302
Nausch, M., Achterberg, E. P., Bach, L. T., Brussaard, C. P. D., Crawfurd, K. J., Fabian, J., et al. (2018). Concentrations and uptake of dissolved organic phosphorus compounds in the Baltic sea. Front. Mar. Sci. 5:386. doi: 10.3389/fmars.2018.00386
Oksanen, J., Blanchet, F. G., Friendly, M., Kindt, R., Legendre, P., McGlinn, D., et al. (2019). vegan: Community Ecology Package. Available online at: https://cran.r-project.org/package=vegan
Oksanen, J., Kindt, R., Legendre, P., O'Hara, B., Simpson, G. L., Solymos, P. M., et al. (2008). The vegan Package. Community Ecology Package, 190. Available online at: https://bcrc.bio.umass.edu/biometry/images/8/85/Vegan.pdf
Olenina, I., Edler, L., Andersson, A., Wasmund, N., Busch, S., Göbel, J., et al. (2006). Biovolumes and Size-Classes of Phytoplankton in the Baltic Sea. Helsinki Commission; Baltic Marine Environment Protection Commission. Available online at: http://helcom.fi/Lists/Publications/BSEP106.pdf
Overbeek, R., Olson, R., Pusch, G. D., Olsen, G. J., Davis, J. J., Disz, T., et al. (2014). The SEED and the Rapid Annotation of microbial genomes using Subsystems Technology (RAST). Nucl. Acids Res. 42, D206–D214. doi: 10.1093/nar/gkt1226
Parker, M. S., and Armbrust, E. V. (2005). Synergistic effects of light, temperature, and nitrogen source on transcription of genes for carbon and nitrogen metabolism in the centric diatom Thalassiosire pseudonana (Bacillariophyceae). J. Phycol. 41, 1142–1153. doi: 10.1111/j.1529-8817.2005.00139.x
Pinhassi, J., Zweifel, U. L., and Hagström, A. (1997). Dominant marine bacterioplankton species found among colony-forming bacteria. Appl. Environ. Microbiol. 63, 3359–3366. doi: 10.1128/AEM.63.9.3359-3366.1997
Pomati, F., Manarolla, G., Rossi, O., Vigetti, D., and Rossetti, C. (2001). The purine degradation pathway. Environ. Int. 27, 463–470. doi: 10.1016/S0160-4120(01)00101-5
Ptacnik, R., Solimini, A. G., Andersen, T., Tamminen, T., Brettum, P., Lepistö, L., et al. (2008). Diversity predicts stability and resource use efficiency in natural phytoplankton communities. Proc. Natl. Acad. Sci. U.S.A. 105, 5134–5138. doi: 10.1073/pnas.0708328105
Quast, C., Pruesse, E., Yilmaz, P., Gerken, J., Schweer, T., Yarza, P., et al. (2013). The SILVA ribosomal RNA gene database project: improved data processing and web-based tools. Nucl. Acids Res. 41, 590–596. doi: 10.1093/nar/gks1219
Rabalais, N. N., Turner, R. E., Díaz, R. J., and Justić, D. (2009). Global change and eutrophication of coastal waters. ICES J. Mar. Sci. 66, 1528–1537. doi: 10.1093/icesjms/fsp047
Riemann, L., Leitet, C., Pommier, T., Simu, K., Holmfeldt, K., Larsson, U., et al. (2008). The native bacterioplankton community in the central Baltic sea is influenced by freshwater bacterial species. Appl. Environ. Microbiol. 74, 503–515. doi: 10.1128/AEM.01983-07
Rink, B., Seeberger, S., Martens, T., Duerselen, C., Simon, M., and Brinkhoff, T. (2007). Effects of phytoplankton bloom in a coastal ecosystem on the composition of bacterial communities. Aquat. Microb. Ecol. 48, 47–60. doi: 10.3354/ame048047
Rönnberg, C., and Bonsdorff, E. (2004). “Baltic sea eutrophication: area-specific ecological consequences,” in Biology of the Baltic Sea, eds H. Kautsky and P. Snoeijs (Dordrecht: Springer Netherlands), 227–241.
Rost, B., Richter, K.-U., Riebesell, U., and Hansen, P. J. (2006). Inorganic carbon acquisition in red tide dinoflagellates. Plant Cell Environ. 29, 810–822. doi: 10.1111/j.1365-3040.2005.01450.x
Sandberg, J., Andersson, A., Johansson, S., and Wikner, J. (2004). Pelagic food web structure and carbon budget in the northern Baltic sea: potential importance of terrigenous carbon. Mar. Ecol. Prog. Ser. 268, 13–29. doi: 10.3354/meps268013
Santos-Beneit, F. (2015). The Pho regulon: a huge regulatory network in bacteria. Front. Microbiol. 6, 1–13. doi: 10.3389/fmicb.2015.00402
Sapp, M., Schwaderer, A. S., Wiltshire, K. H., Hoppe, H. G., Gerdts, G., and Wichels, A. (2007). Species-specific bacterial communities in the phycosphere of microalgae? Microb. Ecol. 53, 683–699. doi: 10.1007/s00248-006-9162-5
Sarmento, H., Morana, C., and Gasol, J. M. (2016). Bacterioplankton niche partitioning in the use of phytoplankton-derived dissolved organic carbon: quantity is more important than quality. ISME J. 10, 2582–2592. doi: 10.1038/ismej.2016.66
Satinsky, B. M., Gifford, S. M., Crump, B. C., and Moran, M. A. (2013). “Use of internal standards for quantitative metatranscriptome and metagenome analysis,” in Methods in Enzymology 531, 237–250. doi: 10.1016/B978-0-12-407863-5.00012-5
Savchuk, O. P. (2018). Large-scale nutrient dynamics in the Baltic sea, 1970–2016. Front. Mar. Sci. 5:95. doi: 10.3389/fmars.2018.00095
Siaut, M., Heijde, M., Mangogna, M., Montsant, A., Coesel, S., Allen, A., et al. (2007). Molecular toolbox for studying diatom biology in Phaeodactylum tricornutum. Gene 406, 23–35. doi: 10.1016/j.gene.2007.05.022
Sison-Mangus, M. P., Jiang, S., Kudela, R. M., and Mehic, S. (2016). Phytoplankton-associated bacterial community composition and succession during toxic diatom bloom and non-bloom events. Front. Microbiol. 7:1433. doi: 10.3389/fmicb.2016.01433
Solomon, C., Collier, J., Berg, G., and Glibert, P. (2010). Role of urea in microbial metabolism in aquatic systems: a biochemical and molecular review. Aquat. Microb. Ecol. 59, 67–88. doi: 10.3354/ame01390
Sörenson, E., Bertos-Fortis, M., Farnelid, H., Kremp, A., Krüger, K., Lindehoff, E., et al. (2019). Consistency in microbiomes in cultures of Alexandrium species isolated from brackish and marine waters. Environ. Microbiol. Rep. 11, 425–433. doi: 10.1111/1758-2229.12736
Stewart, A. J., Robert, G., and Wetzel, W. K. (1982). Influence of dissolved humic materials on carbon assimilation and alkaline phosphatase activity in natural algal-bacterial assemblages. Freshw. Biol. 12, 369–380. doi: 10.1111/j.1365-2427.1982.tb00630.x
Stoecker, D. K. (1999). Mixotrophy among Dinoflagellates. J. Eukaryot. Microbiol. 46, 397–401. doi: 10.1111/j.1550-7408.1999.tb04619.x
Takabayashi, M., Wilkerson, F. P., and Robertson, D. (2005). Response of glutamine synthetase gene transcription and enzyme activity to external nitrogen sources in the diatom Skeletonema costatum (Bacillariophyceae). J. Phycol. 41, 84–94. doi: 10.1111/j.1529-8817.2005.04115.x
Tanaka, T., Henriksen, P., Lignell, R., Olli, K., Seppälä, J., Tamminen, T., et al. (2006). Specific affinity for phosphate uptake and specific alkaline phosphatase activity as diagnostic tools for detecting phosphorus-limited phytoplankton and bacteria. Estuaries Coasts 29, 1226–1241. doi: 10.1007/BF02781823
Teeling, H., Fuchs, B. M., Becher, D., Klockow, C., Gardebrecht, A., Bennke, C. M., et al. (2012). Substrate-controlled succession of marine bacterioplankton populations induced by a phytoplankton bloom. Science 336, 608–611. doi: 10.1126/science.1218344
Tilman, D. (1982). Resource Competition and Community Structure. Princeton, NJ: Princeton University Press.
Traving, S. J., Rowe, O., Jakobsen, N. M., Sørensen, H., Dinasquet, J., Stedmon, C. A., et al. (2017). The effect of increased loads of dissolved organic matter on estuarine microbial community composition and function. Front. Microbiol. 8:351. doi: 10.3389/fmicb.2017.00351
Valderrama, J. C. (1995). “Methods of nutrient analysis,” in Manual on Harmful Marine Microalgae, IOC Manuals and Guides, eds G. M. Hallagraeff, D. M. Anderson, and A. D. Cembella (Paris: UNESCO), 251–268.
Voss, M., Bange, H. W., Dippner, J. W., Middelburg, J. J., Montoya, J. P., and Ward, B. (2013). The marine nitrogen cycle: recent discoveries, uncertainties and the potential relevance of climate change. Philos. Trans. R. Soc. B Biol. Sci. 368:0121. doi: 10.1098/rstb.2013.0121
Wagner, G. P., Kin, K., and Lynch, V. J. (2012). Measurement of mRNA abundance using RNA- seq data: RPKM measure is inconsistent among samples. Theory Biosci. 131, 281–285. doi: 10.1007/s12064-012-0162-3
White, A., and Dyhrman, S. (2013). The marine phosphorus cycle. Front. Microbiol. 4:105. doi: 10.3389/fmicb.2013.00105
Wickham, H. (2016). ggplot2: Elegant Graphics for Data Analysis. New York, NY: Springer International Publishing.
Williams, T. J., Long, E., Evans, F., DeMaere, M. Z., Lauro, F. M., Raftery, M. J., et al. (2012). A metaproteomic assessment of winter and summer bacterioplankton from Antarctic Peninsula coastal surface waters. ISME J. 6, 1883–1900. doi: 10.1038/ismej.2012.28
Wohlrab, S., Falcke, J. M., Lin, S., Zhang, H., Neuhaus, S., Elferink, S., et al. (2018). Metatranscriptome profiling indicates size-dependent differentiation in plastic and conserved community traits and functional diversification in dinoflagellate communities. Front. Mar. Sci. 5:358. doi: 10.3389/fmars.2018.00358
Xiao, X., Sogge, H., Lagesen, K., Tooming-Klunderud, A., Jakobsen, K. S., and Rohrlack, T. (2014). Use of high throughput sequencing and light microscopy show contrasting results in a study of phytoplankton occurrence in a freshwater environment. PLoS ONE 9:e106510. doi: 10.1371/journal.pone.0106510
Zhou, J., Richlen, M. L., Sehein, T. R., Kulis, D. M., Anderson, D. M., and Cai, Z. (2018). Microbial community structure and associations during a marine dinoflagellate bloom. Front. Microbiol. 9:1201. doi: 10.3389/fmicb.2018.01201
Zhou, Y., Davidson, T. A., Yao, X., Zhang, Y., Jeppesen, E., de Souza, J. G., et al. (2018). How autochthonous dissolved organic matter responds to eutrophication and climate warming: evidence from a cross-continental data analysis and experiments. Earth Sci. Rev. 185, 928–937. doi: 10.1016/j.earscirev.2018.08.013
Keywords: phytoplankton, bacteria, coastal, community, interactions, resource partitioning, eutrophication
Citation: Sörenson E, Farnelid H, Lindehoff E and Legrand C (2020) Resource Partitioning Between Phytoplankton and Bacteria in the Coastal Baltic Sea. Front. Mar. Sci. 7:608244. doi: 10.3389/fmars.2020.608244
Received: 19 September 2020; Accepted: 29 October 2020;
Published: 25 November 2020.
Edited by:
Konstantinos Ar. Kormas, University of Thessaly, GreeceReviewed by:
Patricia M. Glibert, University of Maryland Center for Environmental Science (UMCES), United StatesAndreas Oikonomou, Institute of Oceanography, Greece
Copyright © 2020 Sörenson, Farnelid, Lindehoff and Legrand. This is an open-access article distributed under the terms of the Creative Commons Attribution License (CC BY). The use, distribution or reproduction in other forums is permitted, provided the original author(s) and the copyright owner(s) are credited and that the original publication in this journal is cited, in accordance with accepted academic practice. No use, distribution or reproduction is permitted which does not comply with these terms.
*Correspondence: Catherine Legrand, Y2F0aGVyaW5lLmxlZ3JhbmQmI3gwMDA0MDtsbnUuc2U=