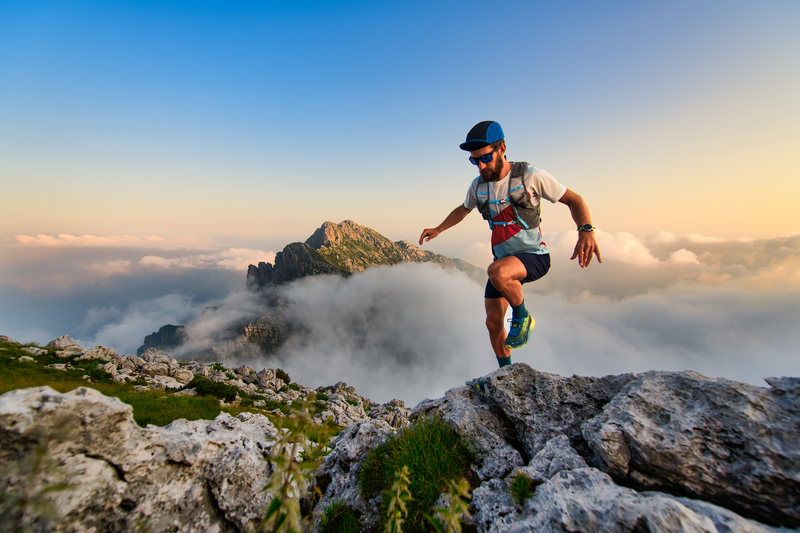
94% of researchers rate our articles as excellent or good
Learn more about the work of our research integrity team to safeguard the quality of each article we publish.
Find out more
OPINION article
Front. Mar. Sci. , 09 December 2020
Sec. Coral Reef Research
Volume 7 - 2020 | https://doi.org/10.3389/fmars.2020.603829
This article is part of the Research Topic Remote Sensing for Applied Coral Reef Science and Management View all 9 articles
Artificial intelligence is an exciting technological frontier for the coral reef remote sensing community, especially the emergence of machine learning algorithms for mapping and detecting features from aerial images of coral reef environments. Machine learning algorithms are finding uses in environmental remote sensing applications that are principally founded on three technological advances.
Firstly, the spatial resolution of remote sensing images has increased incrementally since Earth Observation images were first collected in the late 1960s. Greater detail and smaller features are now visible in coral reef environments. Notably, the widespread use of drone platforms for collecting images at low altitudes above coral reefs has made individual corals visible.
Secondly, more images than ever before are being collected. The “big data revolution” refers to the phenomenon of increased capture of earth observation images, which has delivered the information on which artificial intelligence relies to recognize environmental patterns and trends. Global repositories are now continuously updated to provide real-time satellite images, often freely downloadable, for observing coral reefs. A wealth of image-based information is now available for training and evaluating algorithms to interpret coral reefs from above.
Thirdly, computational advances have made low-cost machines with fast arithmetic units widely available, notably through virtual processing facilities. This has opened up the scope for numerical approaches to image analysis, including several families of machine learning approaches.
Collectively, these three advances are fundamentally shifting the way that the remote sensing community are interpreting images, with important implications for coral reef managers. Taking a fundamentally different approach to most commercial image interpretation softwares, machine learning algorithms work with data and desired results to generate a model that turns one into the other (Domingos, 2015). By continually adjusting the mathematical and logical models built through exposure to training datasets, machine learning algorithms recognize patterns and trends in a manner akin to learning. Here, we outline two distinct applications of machine learning algorithms to coral reef environments, before considering their future uses for coral reef managers:
1. Habitat classification for spatially continuous mapping, and
2. Detecting discrete features in a coral reef environment.
Habitat maps provide a foundation for marine spatial planning and the detection of changes to coral cover, reef health, and ecosystem dynamics (Hamylton, 2017; Purkis et al., 2019). Machine learning algorithms are now embedded into image classification routines for mapping coral reef habitat at large geographic scales. For the global Allen Coral Atlas, random forest learning algorithms classified groups of image pixels (objects) into habitat maps from a collection of covariate data layers, including satellite image reflectance (e.g., Landsat, Sentinel-2, Planet Dove, and Worldbiew-2), bathymetry, slope, seabed texture and wave data (Lyons et al., 2020). Multiple decision trees were trained from known occurrences of bottom type (“ground truthing data”) to classify unknown objects from the mode of the individual tree classes. Working with covariate data and desired results, a machine learning classifier reliably turned one into the other and applied at a global scale using Google Earth Engine to provide a repository of high resolution earth observation imagery and a remote supercomputer (Gorelick et al., 2017). Resulting habitat maps had clear potential management applications, for example, in evaluating reef connectivity for the dispersal of coral and crown of thorns larvae, modeling water quality, and evaluating reef restoration sites (Roelfsema et al., 2020).
While machine learning algorithms offer a reliable means of habitat classification, their use for mapping relies on input covariate biophysical data. Feature extraction provides an alternative application of machine learning algorithms to coral reef imagery.
Rather than classifying and mapping spatially continuous areas of the ground, discrete features visible within images can be directly detected as recurring patterns across multiple pixels. This is commonly achieved through a family of multi-layered deep learning algorithms known as convolutional neural networks (CNN) that were initially developed for facial and handwriting recognition. They define a series of mathematical convolutions to generate the output from the input, allowing all instances of a feature falling within an image to be located based on a predefined set of features (LeCun et al., 2015).
On sand cay islands and reef flats, features that are amenable to detection include fallen trees, particularly on narrow beaches, which signify erosion of sand cay shorelines (Lowe et al., 2019) and individual mangrove trees, from which forest expansion and contraction can be monitored over time (Hamylton et al., 2019). Excitingly, individual corals are now clearly visible in drone images acquired above reefs (Figure 1C) and machine learning algorithms hold good potential for detecting them, opening up a wealth of potential management applications for monitoring coral restoration and bleaching. For example, on degraded reefs, restoration activities include planting tens of thousands of juvenile corals (Lirman and Schopmeyer, 2016). For rehabilitating comparable terrestrial vegetation, CNN algorithms have detected and tracked the fate of individual plants to reveal spatial trends in planting success, and to identify areas for re-planting (Hamylton et al., 2020). Similar applications are feasible for coral reef restoration.
Figure 1. (A) A mosaic of 1400 UAV images over Nymph Island, GBR, including a map to indicate probability of microatoll detection based on intertidal areas and density of reef flat coral framework. (B) A porites microatoll coral on the reef flat. (C) The detail visible in an aerial drone image of a reef flat, showing individual corals. (D) Porites microatolls detected on the reef flat.
Here, we illustrate the application of a bespoke deep convolutional neural network feature extraction algorithm (Faster R-CNN) (Ren et al., 2016) to detect 507 Porites microatolls from 1400 UAV images of Nymph Island (GBR, Figure 1). Porites are distinctive corals, typically between 1 and 5 m in diameter. The algorithm was trained on a rule set that specific individual corals were circular, had a dark external margin and were found in moated intertidal reef flat areas, as specified by a “regions of interest” domain map. The detector had a tendency to overfit (i.e., it detected corals where they did not exist), which is a common feature detection problem in busy images. On the heterogeneous reef flat, this was addressed through: (i) adjustments to the non-maximum suppression parameter (NMS) that defines the threshold of confidence for detecting microatolls, (ii) use of Feature Pyramid Networks to specify the expected size of the microatolls, and (iii) use of Online Hard Example Mining to train the detection algorithm in areas of the reef flat where it was difficult to detect microatolls, thereby removing false positive detections. These three steps resulted in a faster R-CNN algorithm that could reliably detect microatolls on the neighboring reef flat at Three Isles (Recall, 94%, Average Precision 72%).
As illustrated by this example, the primary challenge for extracting features from coral reef images lies in reliably identifying individual corals against a heterogeneous background of shallow reef flat that is typically colonized by a mosaic of marine organisms. The detection of “false positives” can largely be overcome with the three aforementioned amendments in the initial algorithm development and training phase. Advantages of feature detection approaches are that they can incorporate spectral or color information, as well as spatial context and local or global texture features. Significantly, in contrast to the use of machine learning in habitat classification, they self-generate features from raw image data rather than relying on hand-crafted features from experts. Once adequately trained, this capacity to self-generate can yield features across many aerial photographs taken over comparable environments (for example, the algorithm can be trained on one reef, then applied to similar reef flats selected from the remaining 2,904 reefs of the Great Barrier Reef).
There is exciting potential for the coral reef management community to harness the powerful products provided by machine learning, although to do so may require changes to existing operational norms. The results of machine learning often take the form of a probabilistic output, which is subsequently degraded to a categorical map class (e.g., a sampling distribution of the individual tree classes, corresponding to a probability of class membership for each mapped object). Transitioning toward management applications that employ probabilistic spatial data can help to address the mismatch between the machine learning output and the discrete thematic maps that managers typically work with (e.g., a coral reef probability map, as opposed to “reef” vs. “non-reef” map; Li et al., 2020). Further, collecting spatially continuous blocks of field information rather than point records would better support the needs of deep learning applications in mapping habitat, rather than discrete features.
Learning algorithms are increasingly providing other useful services in coral reef environments, including in the analysis of benthic composition from in-situ field photos, with automated extraction of metrics like coral cover for reporting on reef condition (see https://reefcloud.ai/). The increased application of machine learning to aerial images is driving an important and exciting shift in how reef managers make use of spatial information. It is a shift that gives us the confidence, for example, to ask whether algorithms can help managers to control infestations of crown-of-thorns starfish by detecting the starfish themselves, rather than mapping their habitat as a surrogate for their presence.
Increasingly, machine learning algorithms are being run outside the automated toolbox of commercial image processing softwares and programmed for bespoke applications. Guided by users rather than computer programmers, logical rule sets can profitably incorporate biological, ecological and geomorphological principles into both habitat mapping and feature detection. Under such conditions, their application in coral reef environments can be creatively and productively expanded by turning around the title question to ask “what can the artificial intelligence community learn from coral reef managers?” The answers hold exciting prospects in the years ahead.
SH carried out the fieldwork, including image collection, processing and training machine learning algorithms, and co-authored the paper. ZZ annotated the dataset, implemented the CNN algorithms, and conducted the experiments. LW carried out the image analysis, algorithm framework design and performance evaluation, and co-authored the paper. All authors contributed to the article and approved the submitted version.
This work was funded by the GeoQuest Research Institute and the Global Challenges Program, University of Wollongong and the Regional Sciences Program, Australian Academy of Sciences.
The authors declare that the research was conducted in the absence of any commercial or financial relationships that could be construed as a potential conflict of interest.
Domingos, P. (2015). The Master Algorithm: How the Quest for the Ultimate Learning Machine Will Remake Our World. Penguin: Basic Books.
Gorelick, N., Hancher, M., Dixon, M., Ilyushchenko, S., Thau, D., and Moore, R. (2017). Google earth engine: planetary-scale geospatial analysis for everyone. Remote Sens Environ. 202, 18–27. doi: 10.1016/j.rse.2017.06.031
Hamylton, S. M. (2017). Mapping coral reef environments: a review of historical methods, recent advances and future opportunities. Progr. Phys. Geogr. 41, 803–833. doi: 10.1177/0309133317744998
Hamylton, S. M., McLean, R., Lowe, M., and Adnan, F. A. F. (2019). Ninety years of change on a low wooded island, great barrier reef. R Soc. Open Sci. 6:181314. doi: 10.1098/rsos.181314
Hamylton, S. M., Morris, R. H., Carvalho, R. C., Roder, N., Barlow, P., Mills, K., et al. (2020). Evaluating techniques for mapping island vegetation from unmanned aerial vehicle (UAV) images: pixel classification, visual interpretation and machine learning approaches. Int. J. Appl. Earth Observ. Geoinform. 89:102085. doi: 10.1016/j.jag.2020.102085
LeCun, Y., Bengio, Y., and Hinton, G. (2015). Deep learning. Nature 521:436. doi: 10.1038/nature14539
Li, J., Knapp, D. E., Fabina, N. S., Kennedy, E. V., Larsen, K., Lyons, M. B., et al. (2020). A global coral reef probability map generated using convolutional neural networks. Coral Reefs 39, 1805–1815. doi: 10.1007/s00338-020-02005-6
Lirman, D., and Schopmeyer, S. (2016). Ecological solutions to reef degradation: optimizing coral reef restoration in the caribbean and western Atlantic. PeerJ 4:e2597. doi: 10.7717/peerj.2597
Lowe, M. K., Adnan, F. A. F., Hamylton, S. M., Carvalho, R. C., and Woodroffe, C. D. (2019). Assessing reef-island shoreline change using UAV-derived orthomosaics and digital surface models. Drones 3:44. doi: 10.3390/drones3020044
Lyons, M. B., Roelfsema, C. M., Kennedy, E. V., Kovacs, E. M., Borrego-Acevedo, R., Markey, K., et al. (2020). Mapping the world's coral reefs using a global multiscale earth observation framework. Remote Sens. Ecol. Conserv. doi: 10.1002/rse2.157. [Epub ahead of print].
Purkis, S. J., Gleason, A. C., Purkis, C. R., Dempsey, A. C., Renaud, P. G., Faisal, M., et al. (2019). High-resolution habitat and bathymetry maps for 65,000 sq. km of Earth's remotest coral reefs. Coral Reefs 38, 467–488. doi: 10.1007/s00338-019-01802-y
Ren, S., He, K., Girshick, R., and Sun, J. (2016). Faster r-cnn: towards real-time object detection with region proposal networks. IEEE Trans. Pattern Anal. Mach. Intell. 39, 1137–1149. doi: 10.1109/TPAMI.2016.2577031
Keywords: machine learning, convolutional neural network, remote sensing, big data revolution, drone, UAV (unmanned aerial vehicle)
Citation: Hamylton SM, Zhou Z and Wang L (2020) What Can Artificial Intelligence Offer Coral Reef Managers? Front. Mar. Sci. 7:603829. doi: 10.3389/fmars.2020.603829
Received: 08 September 2020; Accepted: 11 November 2020;
Published: 09 December 2020.
Edited by:
Sam Purkis, University of Miami, United StatesReviewed by:
Deepak R. Mishra, University of Georgia, United StatesCopyright © 2020 Hamylton, Zhou and Wang. This is an open-access article distributed under the terms of the Creative Commons Attribution License (CC BY). The use, distribution or reproduction in other forums is permitted, provided the original author(s) and the copyright owner(s) are credited and that the original publication in this journal is cited, in accordance with accepted academic practice. No use, distribution or reproduction is permitted which does not comply with these terms.
*Correspondence: Sarah M. Hamylton, c2hhbXlsdG9AdW93LmVkdS5hdQ==
Disclaimer: All claims expressed in this article are solely those of the authors and do not necessarily represent those of their affiliated organizations, or those of the publisher, the editors and the reviewers. Any product that may be evaluated in this article or claim that may be made by its manufacturer is not guaranteed or endorsed by the publisher.
Research integrity at Frontiers
Learn more about the work of our research integrity team to safeguard the quality of each article we publish.