- 1Department of Oceanography, School of Ocean Earth Science and Technology, University of Hawaii, Honolulu, HI, United States
- 2Ecosystem Sciences Division, Pacific Islands Fisheries Science Center, Honolulu, HI, United States
- 3Department of Biology, Aarhus University, Aarhus, Denmark
Enhancement of phytoplankton biomass near island and atoll reef ecosystems—termed the Island Mass Effect (IME)—is an ecologically important phenomenon driving marine ecosystem trophic structure and fisheries in the midst of oligotrophic tropical oceans. This study investigated the occurrence of IME at Rangiroa Atoll in the French Polynesian Tuamotu archipelago, and the physical mechanisms driving IME, through the analysis of satellite and in situ data. Comparison of chlorophyll-a concentration near Rangiroa Atoll with chlorophyll-a concentration in open ocean water 50 km offshore, over a 16-year period, showed phytoplankton enhancement as high as 130% nearshore, over 75.7% of the study period. Our statistical model examining physical drivers showed the magnitude of IME to be significantly enhanced by higher sea surface temperature (SST) and lower photosynthetically active radiation (PAR). Further, in situ measurements of water flowing through Tiputa Channel revealed outflowing lagoon water to be warmer, lower in salinity, and higher in particulate load compared to ocean water. We suggest that water inside Rangiroa’s lagoon is enriched in nutrients and organic material by biological processes and advected as a result of tidal and wave forcing to coastal ocean waters, where it fuels primary production. We suggest that a combination of oceanographic and biological mechanisms is at play driving frequency and magnitude of IME at Rangiroa Atoll. Understanding the underlying processes driving IME at Rangiroa is essential for understanding future changes caused by a warming climate and changing environmental conditions for the marine ecosystem.
Introduction
Phytoplankton production at the base of the food web provides a fundamental source of energy fueling entire marine ecosystems and production of the world’s fisheries (Duarte and Cebrian, 1996). In the midst of oligotrophic waters, biological biomass has been found to be significantly enhanced around coral reef islands and atolls across the Pacific basin supporting productive local ecosystems with high fish biomass and thriving reef communities (Doty and Oguri, 1956; Signorini et al., 1999; Palacios, 2002; Andrade et al., 2014; Gove et al., 2016; James et al., 2020). The increase in phytoplankton biomass near island and atoll reef ecosystems—termed the Island Mass Effect (IME)—has been attributed to an increase in available nutrients in the euphotic zone (Doty and Oguri, 1956).
Mechanisms contributing to enhancement of phytoplankton biomass in these regions have been found to be diverse and to vary locally. Important sources of nutrients and organic material are inputs from island terrestrial sources such as rivers or submarine groundwater discharge (Dandonneau and Charpy, 1985). In the water surrounding the islands of Tahiti, increased nutrients were found to occur after rain events caused runoff of nutrient-rich land sediment (Sauzède et al., 2018). Further, populated islands and atolls may cause anthropogenic inputs such as human waste or agricultural fertilizer enhancing nutrient and organic matter inputs to nearshore waters (Dandonneau and Charpy, 1985; Gove et al., 2016). Besides direct inputs from land, coral reef organisms can modify biogeochemical characteristics of water flowing over reefs through a multitude of biochemical mechanisms (e.g., Wyatt et al., 2010, 2012; Nelson et al., 2011), such as nitrogen fixation, nutrient regeneration, and recycling from other biota (Atkinson, 2011) and increase nutrient availability surrounding oceanic islands. Physical processes play an essential role in local mixing, transport, and retention of water masses near islands and atolls (Gilmartin and Revelante, 1974; Heywood et al., 1990; Palacios, 2002). Organic material and other sources of nutrients generated via coral reef ecosystem processes can be flushed out of the atoll via wave forcing (Callaghan et al., 2006) and tides (Chevalier et al., 2017) and provide nutrients to the surrounding oligotrophic waters. In tropical lagoons, water renewal time—regulated mainly by the flows through the passages and, when the reef is partially submerged, over the coral reef—strongly affects water quality, biological productivity, and ecological resilience (Delesalle and Sournia, 1991; Chevalier et al., 2017). Further, island and atoll bathymetry and slope can impact ocean currents and cause upwelling of cold nutrient enriched waters around islands and atolls (Hamner and Hauri, 1981; Gove et al., 2016). Internal waves interacting with bathymetry can lead to instabilities in the otherwise stably stratified water column and deliver nutrient rich water from below to the euphotic zone (Carter et al., 2006). Many studies have presented significant spatial and temporal variations in IME, as well as diversity in key forcing mechanisms and their importance in driving IME (Dandonneau and Charpy, 1985; Charpy, 1996; Charpy et al., 1997; Martinez and Maamaatuaiahutapu, 2004; Gove et al., 2016; Sauzède et al., 2018). Gove et al. (2016) analyzed chlorophyll-a measurements recorded over 10 years via satellite for 24 islands and 11 atolls in the tropical western Pacific and found the primary drivers of IME magnitude to be reef area, bathymetric slope, geomorphic type, and population status.
Here we present an investigation of the prevalence of IME at Rangiroa Atoll in French Polynesia. We use long-term satellite-derived observations of chlorophyll-a (a proxy for phytoplankton biomass) to show IME is a near-ubiquitous feature and identify key physical drivers of variations in the magnitude of IME at Rangiroa Atoll. Our findings of IME are contextualized with in situ data collected in Tiputa Channel, the main exchange point for water between Rangiroa Lagoon and the ocean. As our global climate changes, atoll ecosystems are increasingly affected by rising ocean temperatures, changes in wave, wind and light fields, as well as rising sea levels. Understanding the environmental factors that drive fluctuations in phytoplankton biomass can provide a basis for predicting food web shifts occurring as a result of climate change in this region. Understanding the relative influence of environmental mechanisms driving IME at Rangiroa Atoll is crucial in order to predict how atoll systems, such as those in the Tuamotu Archipelago, will respond to environmental changes in the future.
Materials and Methods
Study Site
Rangiroa Atoll is located in the northwest of the Tuamotu Archipelago at 15.1°S, 147.6°W (Figure 1A). The atoll is the largest in the French Polynesia Tuamotu archipelago with a lagoon surface area of 1446 km2, a maximum depth of 38 m, and an estimated volume of 37.71 km3 (Kumar et al., 2013). The atoll perimeter extends ∼ 200 km consisting of 415 reef islands and fringing reef. Rangiroa’s total land area covers 170 km2 (Kumar et al., 2013) with a population of 2709 inhabitants recorded in the 2017 census (Institute of Statistics of French Polynesia, 2017). In addition to ∼100 small passes in the fringing reef, two large passes connect the lagoon to the open ocean in the northwest of the atoll rim: Tiputa Channel is 310 m wide at the narrowest point and between 10 m (lagoon side) and 45 m (oceanside) deep with a steep drop off at the mouth to ocean depths below 60 m. Avatoru Pass is a wider, shallower pass located 9 km to the northwest of Tiputa Channel (Kumar et al., 2013).
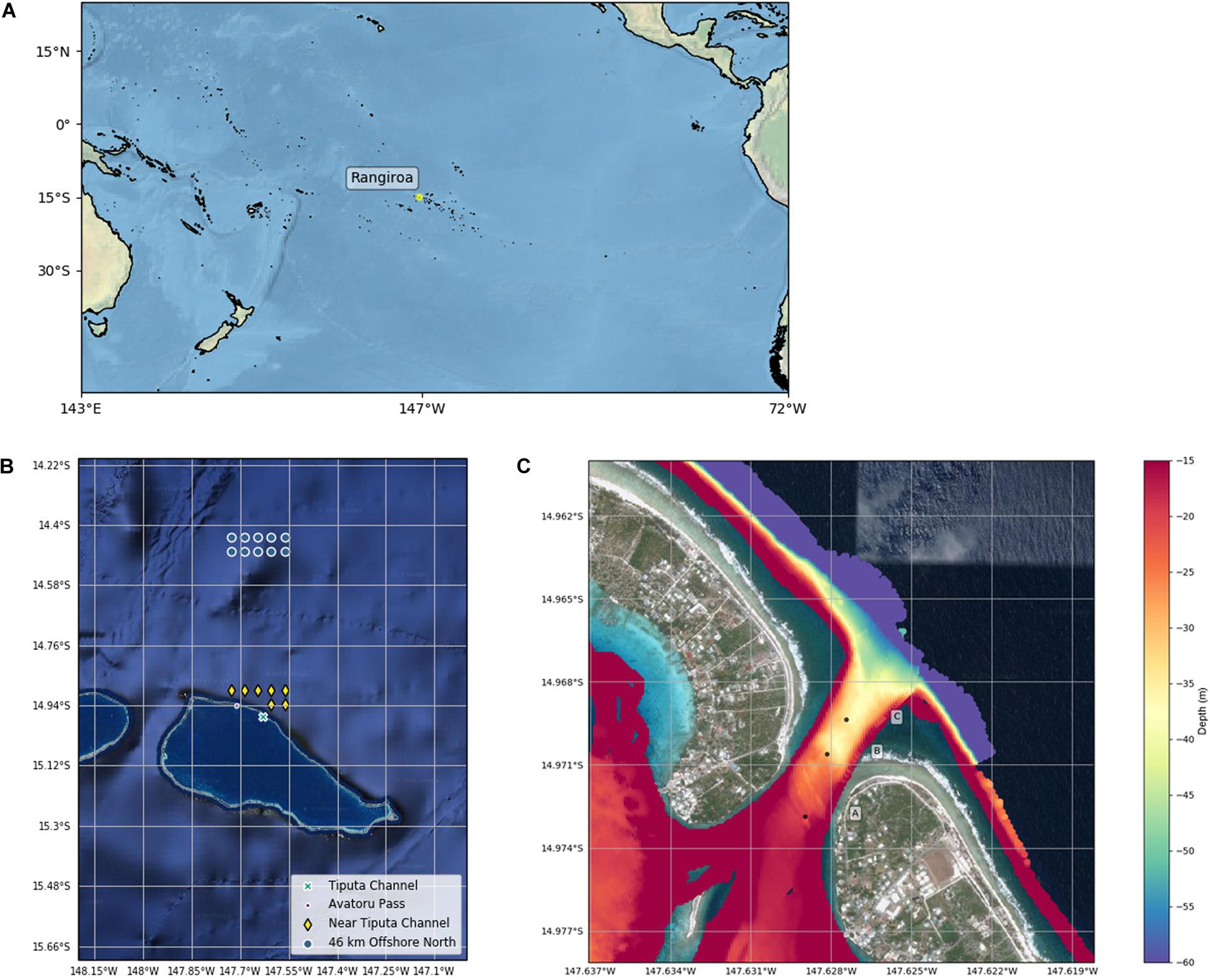
Figure 1. (A) Southern Pacific Ocean map with Rangiroa. (B) Satellite image of Rangiroa with Tiputa Channel, Avatoru Pass, and MODIS data near and approximately 50 km north of Tiputa Channel. (C) Bathymetric data from Tiputa Channel collected by the Secretariat of the Pacific Community Applied Geoscience Division (SOPAC) overlaid on a Google satellite image. Deployment locations of three moorings are marked A, B, and C. The color bar shows depth in meters.
The southern perimeter of Rangiroa Atoll is primarily comprised of fringing reef and the northern perimeter has more islands. The atoll parameter has been estimated to have ∼22% of the perimeter open to exchange with the ocean (Andréfouët et al., 2001a). Thus, the porous rim allows both predominant southern and northern swells to exchange water to the lagoon, while atolls located to the east and west of Rangiroa Atoll block or reflect waves (Andréfouët et al., 2012). Rangiroa Atoll is classified as a partially enclosed atoll with tides and waves being primary mechanisms forcing circulation and exchange. Using water volume flux calculations and wave heights acquired by satellite altimetry data, residence time at Rangiroa Atoll was estimated to be between 130 and 155 days for wave heights in the range of 1.5–3.5 m (Andréfouët et al., 2001b; Pagès and Andréfouët, 2001). Additionally, water residence in Rangiroa’s lagoon was found to decrease linearly with wave forcing above 1.7 m (Andréfouët et al., 2001b).
Data
Chlorophyll-a (mg m–3): Long-term (January 2003 to February 2019) chlorophyll-a data were retrieved from NASA’s Moderate Resolution Imaging Spectroradiometer (MODIS) instrument using the 8-day, 4-km data product. Comparison of chlorophyll-a data sets 23 km north, 50 km north, and 23 km to the south of the atoll, using the Mann–Whitney U test (a non-parametric test used to indicate the probability that two independent samples are from the same distribution, Spiegel and Stephens, 2011), indicated that these locations are statistically of the same set, eliminating the sets 23 km north and south. Further, chlorophyll-a measurements near Tiputa Channel and near the southern fringing reef were found to be statistically of the same set, eliminating the set near the southern fringing reef. For that reason, we focused our analysis of IME at Rangiroa on two locations using 7 MODIS data points (center of each pixel) near the Tiputa Channel and 10 MODIS data points located approximately 50 km offshore and to the north of Rangiroa Atoll (Figure 1B and Table 1). To assess IME, the difference between average chlorophyll-a concentration at these two locations (near Tiputa Channel and offshore of Rangiroa Atoll) was calculated (Figure 1B). Data within ∼3.27 km of the 30 m isobath were removed to avoid optically shallow water and errors induced by terrigenous input, resuspended material, or bottom substrate properties (sensu Gove et al., 2013). Due to sensor limitations, any data points with chlorophyll differences less than 0.005 mg m–3 were excluded reducing the data set from 507 to 317 data points. Latitude and longitude of MODIS data locations near Tiputa Channel and offshore are given in Table 1.
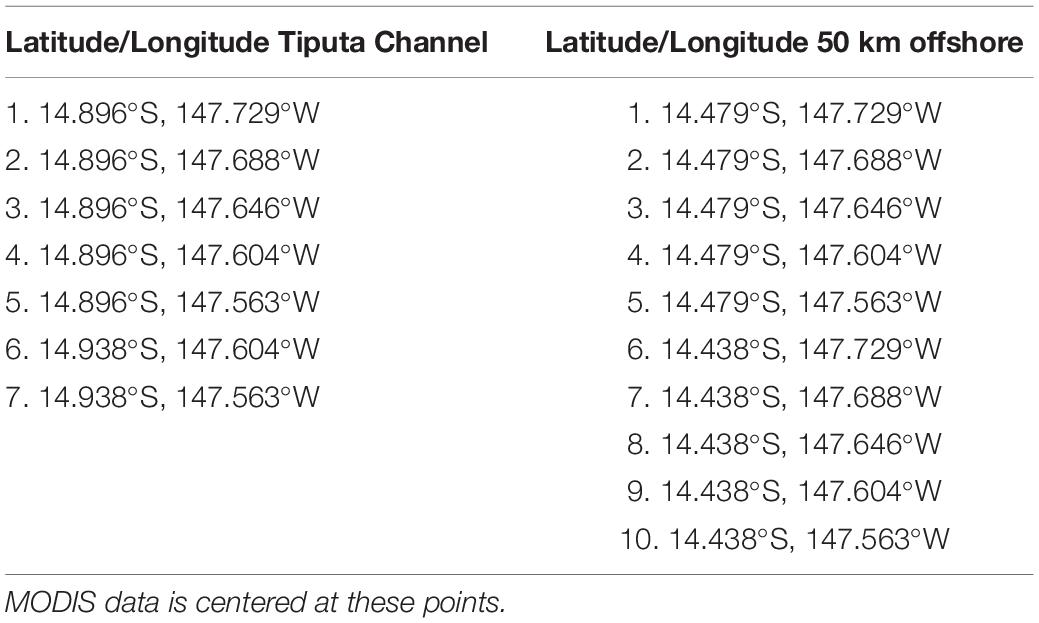
Table 1. Latitude and longitude of MODIS data near Tiputa Channel and approximately 50 km offshore of Tiputa Channel.
Sea surface temperature (°C): SST data were retrieved from the same instrument as chlorophyll-a using the 8-day, 4-km data product near Tiputa Channel.
Photosynthetically active radiation (Einstein m–2 d–1): PAR data were analyzed to identify the optimal amount required for peak chlorophyll-a. PAR data were retrieved from the same instrument as chlorophyll-a using the 8-day, 4-km data product near Tiputa Channel.
Wind forcing: An 8-day average of daily wind direction (°) and magnitude (m s–1) was calculated from NASA SeaWinds sensor on the QuikSCAT satellite1 (data are 0.125° spaced) from January 2003 to November 2009 and NOAA ASCAT sensor on the METOP satellite2 (data are 0.25° spaced) from November 2009 to February 2019. For each 8-day segment, the mean value of the box bounded by latitudes 14.5°S and 15°S and longitudes 147.25°W 147.5°W (to the east of the chlorophyll-a locations) was computed. Interference with winds caused by topography was not of significant concern as Rangiroa Atoll or neighboring atolls do not contain substantial vertical relief (highest point is ∼12 m). For alignment purposes, all wind data were time centered and averaged using the same time window that was used for chlorophyll-a.
Rainfall (in mm): Daily rainfall totals were downloaded from the multi-satellite Global Precipitation Measurement (GPM) mission, version 5. Data were available in a 0.1° × 0.1° grid. Area rainfall data were downloaded for the period 18–22 April 2014, to correspond with the in situ data3.
Wave forcing: Wave data were obtained from the WaveWatch III model from two sources: CAWCR Wave Hindcast 1979–20104 and the CAWCR wave Hindcast extension. Wave data past June 2013 is from WaveWatch III (WW3) Global Wave Model5. An 8-day average of daily maximum wave significant wave height, peak wave period, and peak wave direction was calculated from hourly wave data. Wave data were centered (south of the atoll, 15.5°S, 147.5°W) and aligned with chlorophyll-a dates. This location presented the closest model grid cell to the atoll that would capture the biggest swell during the winter months. Mean significant wave height (Hs) and peak period (tp) were used to calculate wave power (Ef) in kW m–1 using Eq. 1 below:
where ρ is the density of seawater (1024 kg m–3) and g is the acceleration of gravity (9.8 m s–2). The combination of mean significant wave height and peak wave period into wave power allows wave events to be identified in a single predictor variable.
Multivariate ENSO index: Multivariate ENSO Index Version 2 (MEI) data were retrieved from NOAA6. MEI has a range of -2.4 to 2.2 and is used to characterize the magnitude of ENSO events with positive MEI values indicating an El Niño event (warming) and negative values indicating a La Niña event (cooling). MEI combines oceanic and atmospheric variables [sea level pressure (SLP), sea surface temperature (SST), zonal and meridional components of the surface wind, and outgoing longwave radiation (OLR)] into a single index computed 12 times a year in overlapping two-month periods to account for seasonality.
In situ Measurements
To measure the current velocity in Tiputa Channel, three SonTek Acoustic Doppler Profilers (ADPs) were deployed in consecutively deeper locations from the lagoon to the ocean (Moorings A, B, and C at 22.5, 29.5, and 38 m, respectively) along the primary axis of the channel. A Sea-Bird Electronics 19plus CTD7 measuring conductivity, temperature, and pressure; a Wet Labs C-Star transmissometer8 measuring light transmittance (a proxy for water clarity); and a BioSpherical Instruments QSP-2200PD PAR Sensor9 measuring photosynthetically active radiation (PAR) were deployed on Mooring C to assess water conditions. Moving from mooring A to B the channel narrows and the seafloor depth drops from 22.5 to 25.9 m, continuing to mooring C the channel opens to the ocean and seafloor depth continues to drop to 38 m. Between moorings A and C spaced 0.4208 km apart, depth drops to a total of 15.5 m. Net water exchange through Tiputa Channel was calculated over a 24-h period following methods used by after Moehlenkamp et al. (2019). In brief, along channel water volume flux was calculated using Eq. 2:
where width is the channel width (175 m at mooring B), depth the channel depth (varies with tide, ∼29.5 m), and velocity the vector of measured along channel current velocity. Water velocity was recorded at mooring B due to its central location in the channel and its constant cross-channel depth. The flow was unidirectional, and the rate of flow for each time point was averaged over the recorded water column measurements (23 bins with 1 m vertical spacing, from a depth of 4.5–26.5 m were averaged to a single flow rate). This flow rate was then applied to the entire depth of 29.5 m.
Statistical Analysis
Our research explores factors affecting the difference in chlorophyll-a concentration (mg m–3) between Rangiroa Atoll (near Tiputa Channel; Figure 1B) and waters offshore (i.e., onshore–offshore). Here, IME can be seen as a positive difference in chlorophyll-a concentration (Δchl, mg m–3) between these onshore and offshore locations. We test the hypothesis that Δchl varies with sea surface temperature (SST; °C), photosynthetically available radiation (PAR; Einstein m–2 d–1), wind magnitude (m s–1), wind direction (°), wave power (kW m–1), wave direction (°), and the multivariate ENSO index (MEI). Note that MEI and wind magnitude are inherently correlated with one another. We choose to include both in our model to allow us to assess both wind effects (a combined effect of wind magnitude and direction), as well as more general ENSO forcing effects. To account for covariate collinearity, we model with a combination effect of wind direction and magnitude and use generalized additive modeling (more below) where the non-linear fitting is more robust (vs. linear modeling) to collinearity among predictor variables. Each predictor variable was averaged over the same 8-day period as the chlorophyll-a data with no lag between response and predictor variables. Entries missing a value were removed from the dataset, resulting in 310 time points for analysis. The response (Δchli) was modeled as observations from a normal distribution as they are continuous and can be both positive and negative. The expected value of the response was modeled as a function of the predictors using a generalized additive model (GAM). GAMs do not assume a priori any specific form of the relationship between the dependent variable and the covariates and can be used to reveal and estimate non-linear effects of the covariate on the dependent variable. To account for temporal autocorrelation, a variable (SampleDay) containing the number of days since the first sample was included in the model. Our GAM was:
and
Effects of wind magnitude and direction were assessed via a two-dimensional smoother with magnitude smoothed using a thin plate regression spline and direction smoothed with a cyclic cubic regression spline (the latter reflecting the cyclical nature of wind direction). The link function, g(…), providing the relationship between the expected value of the response and the linear predictor was set as the canonical identity link function, and model residuals were assessed regarding this assumption by inspecting residuals and determining the ability for the model to give rise to the data by comparing residuals to those simulated from the model. The importance of predictors in explaining response variability was assessed by comparing models representing all possible combinations of predictors via corrected Akaike Information Criteria (AICc). Models always included the SampleDay predictor to help adjust for temporal autocorrelation (as mentioned above). All statistical analysis was fit using R (R Core Team, 2018) via the mgcv, lubridate, ggplot2, DHARMa, MuMIn, visreg, reshape2, and metR packages (Wickham, 2007, 2016; Barton, 2009; Grolemund and Wickham, 2011; Wood, 2011; Breheny and Burchett, 2017).
To test correlations among the predictors, a Pearson’s pairwise correlation test was performed to test for a linear relationship between two sets of data. Equation 4 below calculates the correlation coefficient or r-value (r) using each sample point (i) in the two datasets (x and y)
where mx is the mean of the set x and my is the mean of the set y. Positive signs of R values indicate a positive correlation, negative signs indicate a negative correlation. The magnitude spans from 0 to 1 with larger numbers showing a higher correlation. P-values range from 0 to 1 and indicate the probability that the r-value relationship is not true (significant if p < 0.05, Abbott, 2017).
Results
Nearshore Phytoplankton Enhancement
We found that during 75.7% of the 16-year study period (from January 2003 to February 2019), Rangiroa Atoll displayed localized nearshore enhancement in chlorophyll-a, which is the IME, Δchl. Averaged chlorophyll-a concentration at Tiputa Channel was 16% greater than the concentration offshore to the north with a maximum enhancement nearshore as high as 130%. The chlorophyll-a difference between nearshore and offshore ranged from -0.043 mg m–3 (no IME) to 0.091 mg m–3 with a mean value of 0.008 mg m–3 (Figure 2). The mean positive chlorophyll-a difference (i.e., IME) was 0.015 mg m–3, and the mean negative chlorophyll-a difference was −0.013 mg m–3 (Figure 2A). Chlorophyll-a near Tiputa Channel and offshore followed similar, though not identical, patterns of both increases and decreases through the record (Figure 2B).
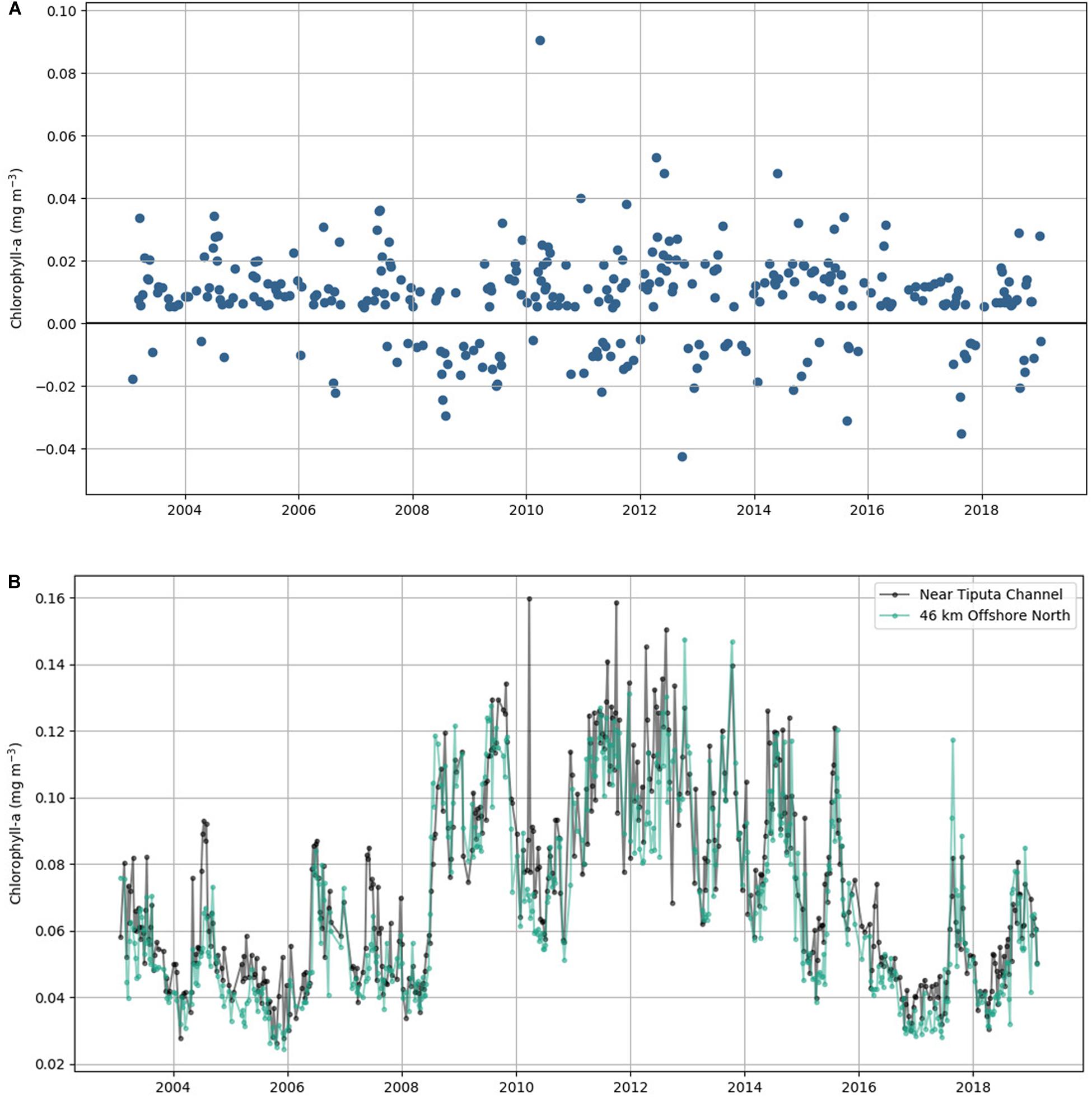
Figure 2. (A) Eight-day average of chlorophyll-a difference between near Tiputa Channel and offshore from January 2003 to February 2019. Positive values represent times when the IME was present; negative values represent times when the IME was not present. (B) Eight-day average of chlorophyll-a data near Tiputa Channel (black) and offshore (aqua).
We investigated the factors that influence the nearshore to offshore chlorophyll-a difference via a suite of physical predictor variables using a GAM with normal error distribution assumption. The resulting statistical model exhibited relatively uniform residuals with only a slightly skewed distribution from the expected (likely due to an outlier). The model was refit with a “log” link function but the change did not improve residual behavior.
A comparison of models representing all possible predictor combinations (but always including SampleDay; see Materials and Methods) revealed five models within ΔAICc < 2 of the lowest AICc model (Table 2). Best-specified models also exhibited well-behaved uniform residuals with no significant outliers. SST and PAR appeared in all best-specified models resulting in an importance estimate (via sum of model weights) of 1 for each (Table 2). Wind effects (magnitude and direction) appeared in three best-specified models with an importance estimate of 0.66. Wave effects (power and direction) appeared in two best-specified models with an importance estimate of 0.35. MEI effects appeared in one best-specified model with an importance estimate of 0.17. Effect plots were drawn from Model 1 in Table 2 which had the highest weight (0.337) relative to other best-specified models and explained 18% of the variation in Δchl. The highest proportion of variation in Δchl was explained by wind effects (magnitude and direction; 5.8%). Δchl declined with increasing wind magnitude and showed the highest values over a mid-range in wind directions (Figure 3A). SST explained the second highest proportion of variation in Δchl (4.7%). Δchl increased with increasing mean temperature (Figure 3B). Finally, PAR explained 3.3% of the variation in Δchl with declining Δchl with increasing PAR (Figure 3C). As all modeled effects have the prediction estimate above the 0 Δchl line on the y-axis, they are describing effects on the magnitude of IME for Rangiroa.
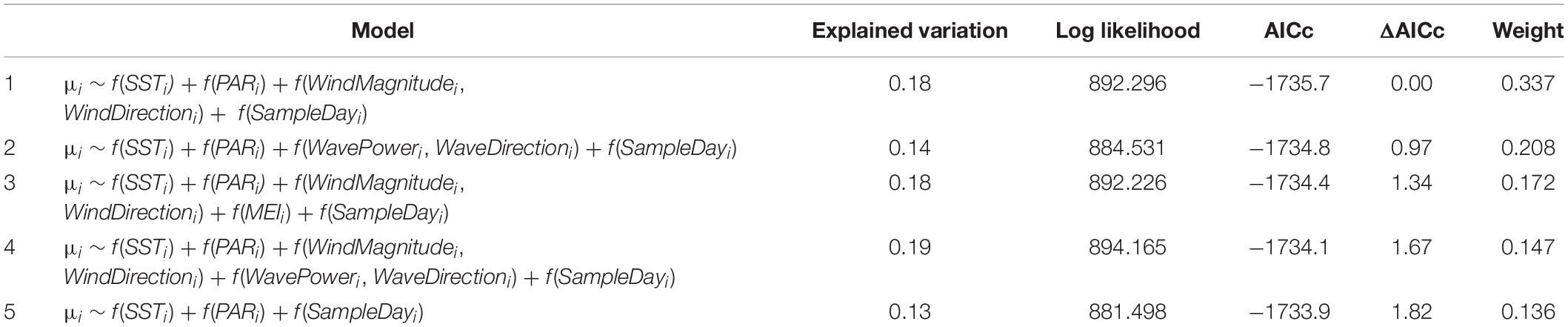
Table 2. Five models resulting from a comparison of models investigating Δchl representing all possible predictor combinations within ΔAICc < 2 of the lowest AICc model.
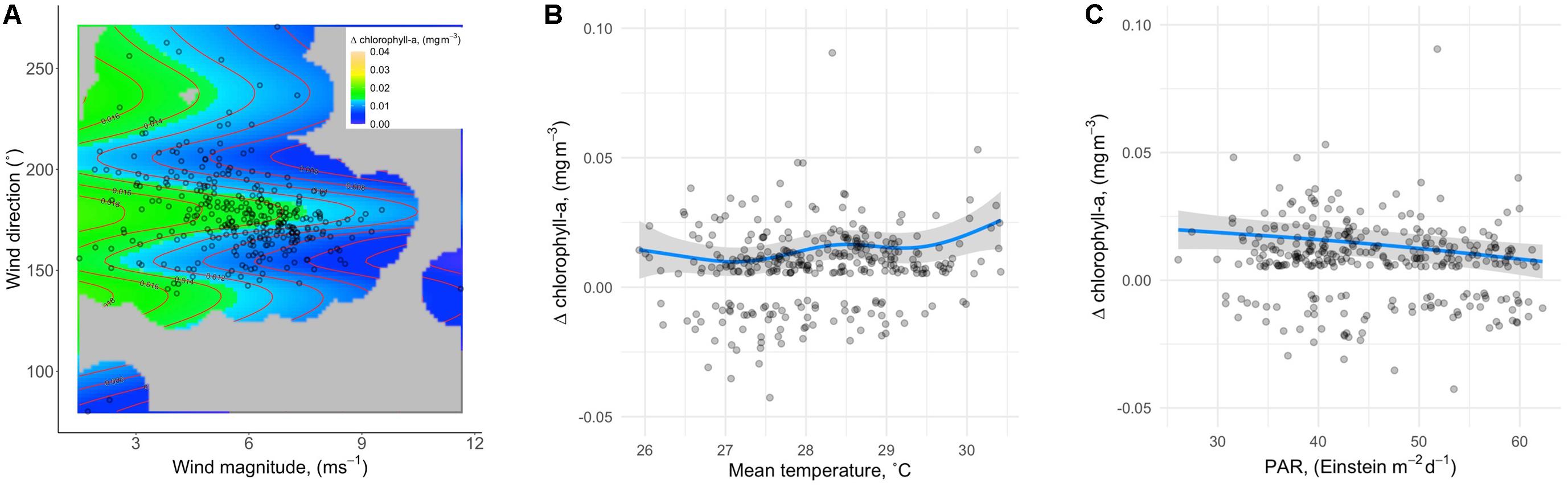
Figure 3. Effect plots from Model 1 in Table 2, which had the highest weight (0.337) relative to other best-specified models (A) IME magnitude as a function of wind. (B) IME magnitude as a function of SST. (C) IME magnitude as a function of PAR. As all modeled effects have the prediction estimate above the 0 Δchl line on the y-axis, they are describing effects on the magnitude of IME for Rangiroa.
Seasonal Patterns in the Island Mass Effect
Chlorophyll-a difference data was plotted on 8-day increments across 16 years (January 2003 to February 2019) to investigate seasonality (Figure 4). IME across years was variable (Figure 4A): the mean value of IME was 0.008 mg m–3 with a large standard deviation of 0.015 mg m–3. The highest value 0.091 mg m–3 was recorded in March 2010, and the lowest value -0.043 mg m–3 was recorded in September 2012 (Figure 4B). The month with the highest chlorophyll-a difference across the 16-year time span was May with a mean chlorophyll-a difference of 0.014 mg m–3. The month with the lowest chlorophyll-a difference across the 16-year time span was September with a mean chlorophyll-a difference of 0.002 mg m–3. March had the highest variability with the standard deviation of 0.020 mg m–3, and February had the lowest variably with the standard deviation of 0.009 mg m–3 (Figure 4B). Data were spaced unevenly throughout the year due to clouds during the rainy summer season resulting in fewer chlorophyll-a records in the months of October through March. Chlorophyll-a near Tiputa Channel and offshore both showed a gradual rise in chlorophyll-a from March through August when all years are averaged; however, when comparing year to year, this pattern was not consistent and there was considerable variability across the years for each month (Figure 4). While seasonal trends in chlorophyll-a difference can be identified, these were not consistent across years. Mean chlorophyll-a concentration nearshore and offshore of Tiputa Channel visualized across the entire study period from January 2003 to February 2019 showed a clear IME (i.e., the enhancement of chlorophyll-a concentration nearshore) (Figure 5A). However, the degree to which IME was present varied strongly across years, with strong magnitudes in 2013 (Figure 5B) and weaker magnitudes in 2016 (Figure 5C).
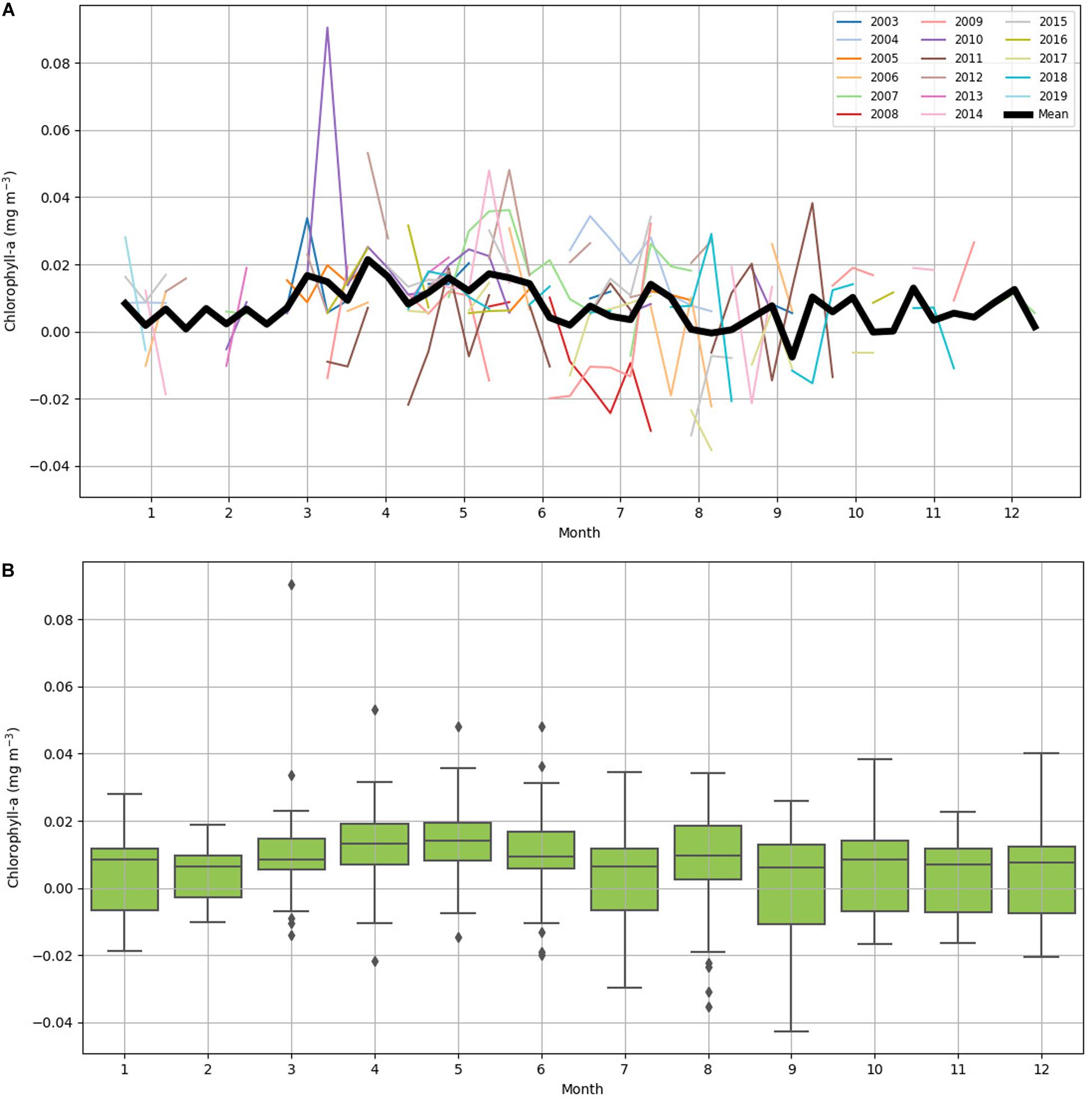
Figure 4. (A) Yearly 8-day mean of chlorophyll-a difference from January 2003 to February 2019. Each year has a color-coordinated line. The dark black line is the collective mean for all years. (B) Box plot of 8-day average chlorophyll-a difference between near Tiputa Channel and offshore by month, derived from data between January 2003 and February 2019. The central mark in each box is the median, the bottom and top edges of the box are the 25th and 75th percentiles. Diamonds indicate outliers.
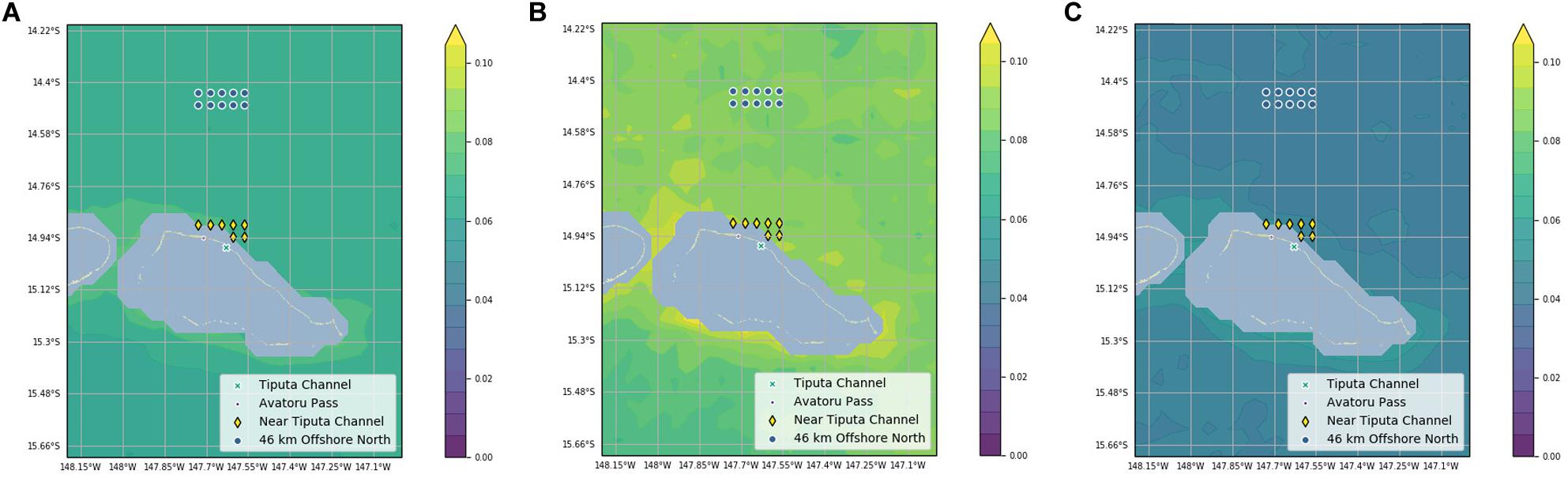
Figure 5. (A) Chlorophyll-a averaged across the entire study period (2003–2019). (B) Chlorophyll-a averaged across the year of 2013. (C) Chlorophyll-a averaged across the year of 2016. Chlorophyll-a concentration was projected onto a terrain map and plotted using filled contours. Contours are drawn in 0.005 increments from 0 to 0.12 mg m– 3. Study points are marked along with Tiputa Channel and Avatoru Pass. Pixels with land or within the 30-m isotherm are masked.
In situ Observations in Tiputa Channel
In situ observations of pressure (water depth), PAR, light transmittance, temperature, conductivity (salinity), and current velocity in Tiputa Channel (18–22 April 2014) revealed strong influence of tidal forcing (Figure 6). PAR followed a typical diurnal pattern with solar insolation increasing after sunrise and decreasing before sunset (Figure 6A). Water leaving the atoll on ebb tide was higher in turbidity indicating a higher concentration of particulate matter compared to ocean water flooding into the atoll (Figure 6B). In addition, water flowing into the atoll on the flood tide was colder, saltier, and denser compared to water flowing out of the atoll with the low tide (Figures 6C,D). Current flow at the three moorings was predictable, primarily following the tide (Figure 7A). Horizontal currents at moorings A, B, and C flowed in the along channel direction, oriented at 32.2° (true; northeast) for outgoing low tide, and for 212.2° (true; southwest) incoming high tide. Outgoing current speeds had a higher velocity than incoming velocities given the additional pressure gradient between the lagoon and the ocean (Table 3). During the sensor deployment period, the maximum outgoing current speed was 2.594 m s–1 recorded at mooring B; the maximum incoming current speed was 1.813 m s–1 recorded at mooring A. Water balance over the 24-h period summed 969,000 m3 entering the atoll and 2,810,000 m3 exiting the atoll highlighting a significant difference between inflow and outflow with more water exiting than entering Rangiroa Atoll through the Tiputa Channel at each tidal cycle. The net water exchange between Rangiroa lagoon and the ocean was 1,841,000 m3 (outward) for the 24-h period 20 April 2014 00:00 through 23:59. During the flood tide, as the water level increases so does the volume flux, until reaching the midpoint or highest water level at which point it decreases. On ebb tide the flow reverses following the same pattern (Figure 7B).
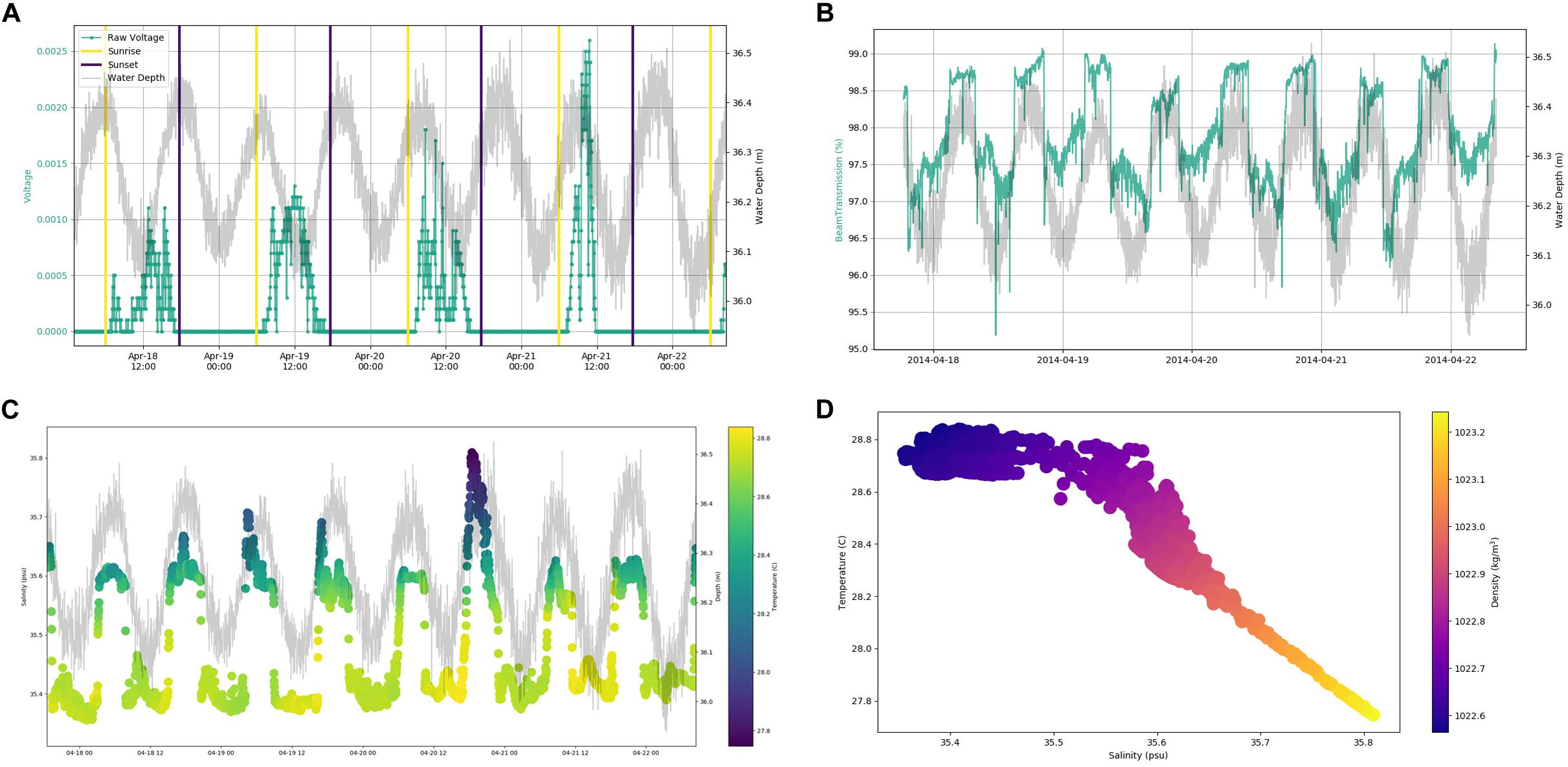
Figure 6. (A) Raw voltage from BioSpherical Instruments QSP-2200PD PAR sensor. Voltage indicated by aqua line. Yellow lines indicate sunrise. Dark blue lines indicate sunset. Sunrise and sunset are from the NOAA Solar Calculator (http://www.esrl.noaa.gov/gmd/grad/solcalc/). Gray line indicates water depth in meters derived from Sea-Bird Electronics 19plus CTD. (B) Light transmittance (%) over time with water depth in Tiputa Channel at mooring C. Light transmittance data from Wet Labs C-Star transmissometer. Transmittance (aqua) values. Gray line indicates water depth in meters derived from Sea-Bird Electronics 19plus CTD. (C) Temperature and salinity plotted with water depth over time. Color indicates temperature in °C with yellow being warmer and blue colder. Salinity is in psu. Water depth is (in m) is indicated by gray line. Temperature, salinity, and pressure data from Sea-Bird Electronics 19 plus CTD. (D) Temperature, salinity, and density. Temperature is in °C. Salinity is in psu. Colors indicate density in kg m– 3 with yellow being denser and blue being less dense. Data for A–C were retrieved from mooring C (depth 37 m) from 18 April 2014 through 22 April 2014.
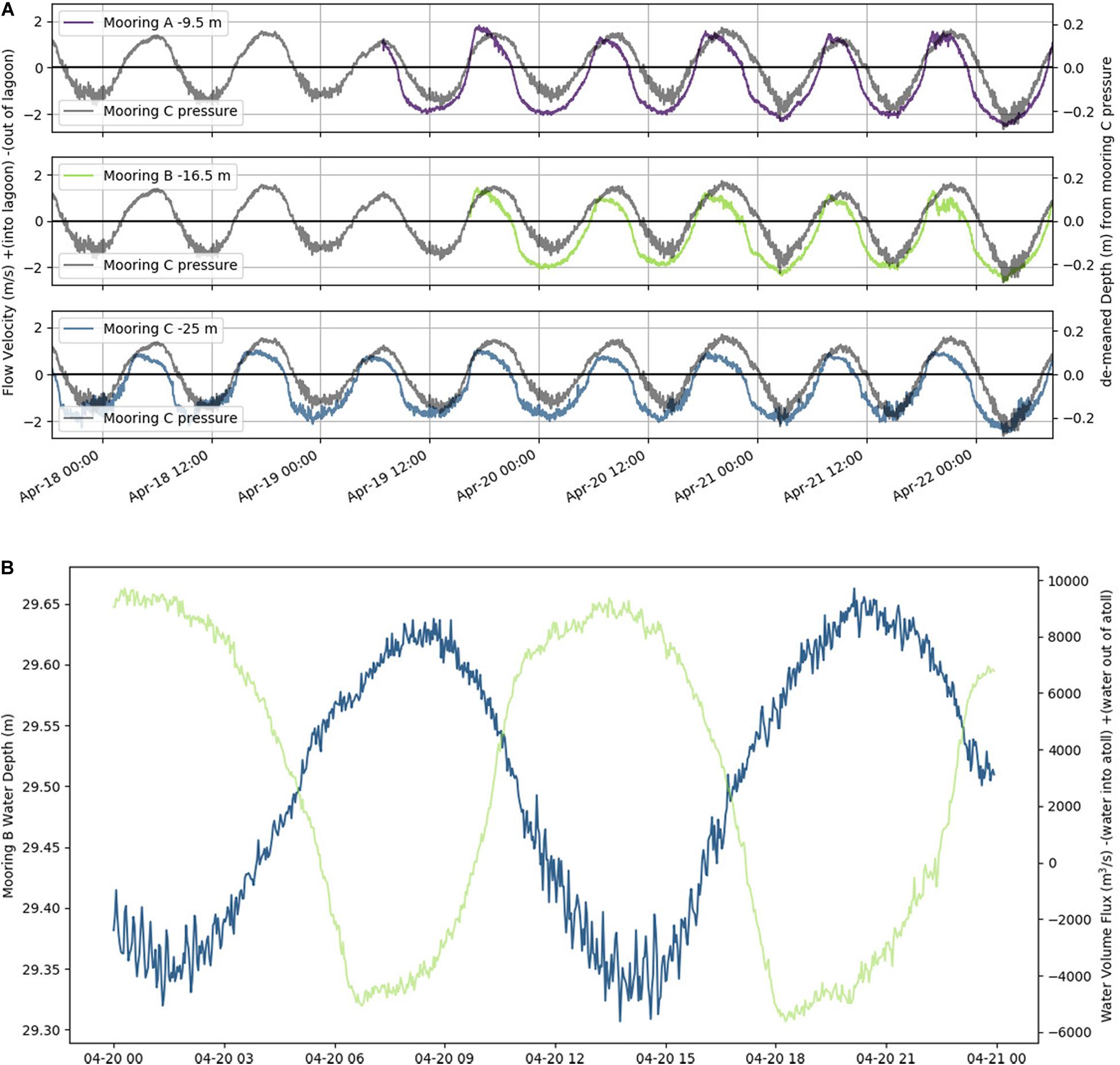
Figure 7. (A) Horizontal channel flow at a single water depth plotted with a demeaned tide taken from pressure at mooring C (gray line in each panel in April 2014). Top panel is mooring A; flow is at 9.5 m depth (purple). Middle panel is mooring B; flow is at 16.5 m depth (green). Bottom panel is mooring C; flow is at 25 m depth (blue). Flow velocity is in m s– 1. Relative water depth in m. (B) Water level (m, blue) and water flux (m3 s– 1, green) at mooring B over 24-h period on 20 April 2014. Negative water flux indicates water flowing into the lagoon, positive water flux indicates water flowing out of the lagoon.
Discussion
IME Present at Rangiroa Atoll
Our investigation of IME demonstrated that nearshore phytoplankton enhancement is a long-term, near-persistent feature at Rangiroa Atoll. IME is present in waters outside Tiputa Channel, Rangiroa Atoll 75.7% of a 16-year period with phytoplankton enhancement as high as 130% and an average enhancement of 16% near Rangiroa Atoll compared to offshore. The magnitude and frequency of enhanced chlorophyll-a concentration clearly indicate presence of the Island Mass Effect (IME) in coastal waters offshore of Tiputa Channel. These results agree with past studies that found increased phytoplankton biomass around islands and atolls in the Tuamotu Archipelago despite the surrounding oligotrophic waters of the South Pacific Subtropical Gyre (Ferrier-Pagès and Furla, 2001). Studies found enhanced biomass inside atoll lagoons with particulate organic matter to be 2–5 times higher inside the atolls compared to the open ocean (Charpy et al., 1997; Ferrier-Pagès and Furla, 2001). The Island Mass Effect was first described over 70 years ago (Doty and Oguri, 1956), since then, IME has been documented for numerous island-reef ecosystems (e.g., Signorini et al., 1999; Palacios, 2002; Andrade et al., 2014; Gove et al., 2016). In a 10-year study investigating IME across the tropical Pacific, Gove et al. (2016) found an increase of phytoplankton biomass in 91% of the 32 islands and atolls surveyed.
SST and PAR Are Significant Drivers of IME Magnitude
We found that SST was a significant driver of IME. Multiple studies have documented an important relationship between SST and chlorophyll-a (Lo-Yat et al., 2011; Uz et al., 2017; Dunstan et al., 2018). Dunstan et al. (2018) found that chlorophyll-a change, as a response to SST, is highly heterogeneous with large regional differences: some regions showed increased chlorophyll-a with increased SST; other regions in contrast showed decreased chlorophyll-a with increased SST. Our study indicated a positive relationship between IME (the positive chlorophyll-a difference between coastal Rangiroa waters and offshore) and SST with increasing IME as a result of increasing SST. Chlorophyll-a concentration in coastal Rangiroa waters and offshore of Tiputa Channel are likely influenced by different mechanisms. Chlorophyll-a offshore can be influenced by broad scale climate forcing such as ENSO that lead to changes in SST (McPhaden, 2012). Uz et al. (2017) identified changes in the thermocline and upwelling intensity related to El Niño and La Niña in the equatorial Pacific between 10°N and 10°S. During an El Niño event, enhanced SST can lead to more stable stratification in the upper ocean and a reduction in surface mixing depth resulting in lower chlorophyll-a concentrations (Uz et al., 2017). The relationship of enhanced SST leading to decreased chlorophyll-a in oligotrophic waters offshore was also confirmed by Lo-Yat et al. (2011), who investigated satellite chlorophyll-a data between January 1996 and March 2000 in a study 124 km (14.1°S, 147.1°W) outside Rangiroa Atoll and found a strong relationship between the timing of an El Niño warming event and satellite-derived chlorophyll-a. While not a statistically significant driver of IME, the relationship between the Multivariate ENSO Index (MEI) and IME was found to be positive in this study (Figure 8). While open ocean chlorophyll-a can be depressed during strong warming events as a result of more stable stratification, chlorophyll-a in Rangiroa’s coastal waters may be affected less by this dynamic due to its proximity to the lagoon and atoll. Hence, increased SST could lead to a stronger IME (the positive chlorophyll-a difference between coastal Rangiroa waters and offshore). Pearson’s pairwise correlation on SST and chlorophyll-a (not IME) near Tiputa Channel (r-value -0.29) and offshore (r-value -0.37) found a negative linear relationship (increased SST, decreased chlorophyll-a) for both locations (Figure 8). However, this trend is more pronounced for the offshore location compared to near Tiputa Channel, supporting the hypothesis that warming water affects chlorophyll-a offshore and close to the coast differently.
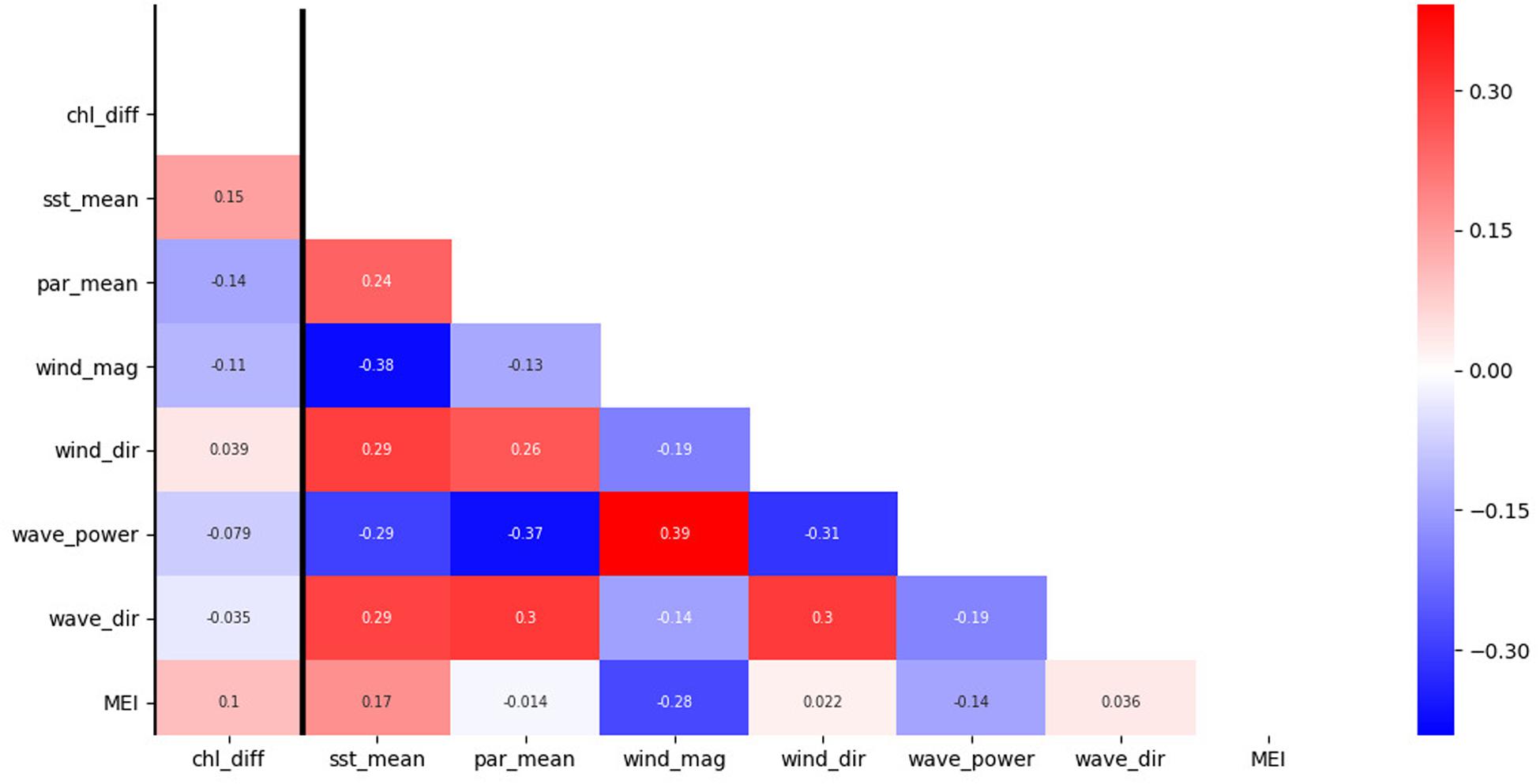
Figure 8. Correlation coefficient (r-value) heatmap from data between January 2003 to February 2019. The response variable (chl_diff) is the chlorophyll-a data differences between near Tiputa Channel and offshore. Data are 8-day averages with no lag between variables. The predictor variables are: sea surface temperature (SST) (sst_mean), photosynthetically available radiation (PAR) (par_mean), wind magnitude (wind_mag), wind direction (wind_dir), wave power (wave_power), wave direction (wave_dir), and the multivariate ENSO index (MEI). Colors indicate the correlation coefficient (r-value). Red indicates a positive relationship between variables. Blue indicates a negative relationship between variables. The strength of color indicates the strength of the relationship.
While IME increased with increasing SST, IME decreased with increasing PAR (Figure 3). High light levels driving reduced chlorophyll-a biomass has been documented in other studies (Flombaum et al., 2013; Jyothibabu et al., 2018). High levels of PAR can cause photoinhibition, which has been observed in Prochlorococcus with cell abundance reduced 31% off-peak in a global study (Flombaum et al., 2013). In contrast, cloud cover in the Bay of Bengal causing lower levels of PAR was found to increase chlorophyll-a (Jyothibabu et al., 2018). While sunlight is crucial for photosynthesis of phytoplankton, PAR may reduce surface chlorophyll-a concentration by causing photoinhibition both near and offshore of Rangiroa Atoll, hence reducing the magnitude of IME.
Other Mechanisms Contributing to IME
The total statistical model explains 18% of the variation in Δchl and SST and PAR explain 8% of the variation in Δchl. Therefore, 44% (i.e., 8%/18%) of the total variation explained by the model can be attributed to effects of SST and PAR. 5.8% of variation in Δchl was explained by wind effects (magnitude and direction). However, 82% of the variation in Δchl remains unexplained by the model, suggesting that a combination of biophysical drivers shape IME at Rangiroa. Autochthonous nutrient sources in coral reef ecosystems present one primary driver for increased phytoplankton biomass at island and atoll ecosystems. Processes including nitrogen fixation, regeneration through decomposition of primary producers or from sediment deposition, and recycling from other biota increase the availability of essential limiting nutrients in the water column (Suzuki and Casareto, 2011; Gove et al., 2016). Benthic nitrogen fixation was found to account for 24.4% of benthic primary production requirements at neighboring Tikehau Atoll Lagoon (Charpy-Roubaud et al., 1990, 2001). Further, animal waste products derived from sea birds, reef fish, and marine invertebrates have also been shown to enhance nutrient concentrations in coral reef ecosystems (Williams and Carpenter, 1988; McCauley et al., 2012; Burkepile et al., 2013). Human activities can increase nearshore nutrient concentrations through runoff from urban development and agricultural land use or wastewater (Smith et al., 1999), and population status has been shows to be a significant driver for IME in other studies (Gove et al., 2016). While Rangiroa Atoll is populated (2709 people), it has a flat terrain and lacks rivers, hence the amount of terrigenous material exported via run-off is expected to be limited.
Physical processes such as water residence time and incoming light energy affect biogeochemical processes within coral reefs and can cause variations in total reef-derived nutrients available to phytoplankton (Atkinson, 2011; Suzuki and Casareto, 2011). Wave- and tidal-driven flushing may export nutrient enriched water from lagoons to surrounding waters fueling enhanced nearshore phytoplankton biomass (Gove et al., 2016). Even though statistical analysis did not find wave forcing to be a significant driver of IME (p > 0.05) in the present study, wave forcing has been shown in other studies to force atoll flushing, advecting detritus, and other sources of nutrients generated via coral reef ecosystem processes (Callaghan et al., 2006). Further, bathymetric influences on ocean currents can force vertical transport of subsurface nutrient-rich waters that fuel nearshore productivity: divergence of flow around steep island/atoll systems has been documented to cause upwelling of deeper nutrient-rich water near coasts (Caldeira et al., 2002; Spall and Pedlosky, 2013; Gove et al., 2016). Internal waves breaking due to abrupt changes in topography can to play a role in the vertical transport of particles and thus fuel IME (Leichter et al., 2012; Gove et al., 2016; Zhao, 2018). Rangiroa Atoll has been documented to generate internal tides, as a result of tide-bottom interactions (Zhao, 2018). Recent satellite results clearly show that internal tides radiate from Rangiroa and other atolls (Figure 13 in Zhao, 2018). Internal tides may cause pulses of nutrient-rich deep water to be advected up coastal slopes and fuel primary production that enhances IME.
Connecting Satellite Data to in situ Measurements
The in situ sensors measured warmer, fresher, less transparent water exiting the atoll at each ebb tide (Figure 6). This suggests that Rangiroa lagoon provides a source of particulates, chlorophyll-a and likely nutrients into the surrounding oligotrophic ocean. As described previously, we suggest that benthic processes increasing available nutrients in the lagoon. Water residence time has been found to cause nutrients and organic matter to be recycled multiple times and increase biomass (Chevalier et al., 2017). Rangiroa’s long residence time (estimated between 130 and 155 days (Andréfouët et al., 2001b; Pagès and Andréfouët, 2001) could allow nutrients and organic matter to be recycled several times by biota before being exported toward the open ocean. Charpy et al. (1997) found that phytoplankton biomass inside the lagoon was inversely related to the water exchanged between the lagoon and ocean. In contrast, rapid flushing leading to short residence times can inhibit the recycling of nutrients that fuel primary production (Ferrier-Pagès and Furla, 2001). Hence warmer, particulate and likely nutrient enriched water are advected into the oligotrophic waters surrounding Rangiroa, where it fuels phytoplankton production (Kirk, 2011). Over the 5-day span, 18–22 April 2014, 48 mm of precipitation fell on the far west of the lagoon. Lower rainfall amounts were recorded over the remainder of the atoll ranging from 12 mm in the far east upward to 48 mm in the far west. Large fluctuations in daily rainfall (between 0 and 360 mm) observed during the study period did result in a strong salinity signal in our measurements (Figure 6C). Instead, fluctuations in salinity were strongly correlated with the tidal cycle (Figure 6C). Lower salinity of outgoing lagoon water compared to incoming ocean water can likely be attributed to submarine groundwater discharge (SGD) originating from an atoll fresh water lens. Atoll fresh water lenses have been described to fill during the rainy season (November–April) and discharge freshwater into lagoon and surrounding oceanic water via processes such as SGD (Rougerie et al., 2004). SGD can be enriched in nutrients and may play a significant role in nutrient cycling enriching primary productivity in the coastal ocean (Caroline and Van Cappellen, 2004). Water exchange calculations show that 2.9 times more water volume was exiting than entering Rangiroa Atoll through Tiputa Channel indicating that a large amount of water exchange can be attributed primarily to wave forcing of water over the fringing reef. In a study examining permeability of various atoll rims, Rangiroa was found to have ∼22% of the perimeter open to exchange with the ocean (Andréfouët et al., 2001a). The porous rim allows both predominant southern and northern swells to add water to the lagoon. Other atolls to the east and west block or alter waves from those directions (Andréfouët et al., 2012). A comparison of horizontal current at mooring B to wave power during the deployment period, associated higher wave power with a faster current flow out of Rangiroa Atoll (r2 values of 0.04). This relationship is supported by Pagès and Andréfouët (2001), who found that net outflow of Tiputa Channel increases with wave heights above 1.4 m.
Future Implications
Climate change has many effects on our oceans including warming sea surface temperatures, sea level rise, and acidification (Goeldner-Gianella et al., 2019), as well as increasing frequencies and intensity of El Niño events (Wang et al., 2019). These changes are likely impacting IME at Rangiroa Atoll, now and will in the future. Sea level rise threatens to change Rangiroa from a semi-enclosed atoll to a more open atoll, through both erosion and by submerging the southern fringing reef. Open atolls allow lagoon water to be flushed more quickly resulting in shorter residence times and water with properties more similar to the surrounding ocean (Ferrier-Pagès and Furla, 2001). Further, ocean warming and acidification are likely to limit coral growth, prohibiting vertical atoll growth via coral as sea level rises and suppressing an important nutrient source (Goeldner-Gianella et al., 2019). In a study by Andréfouët et al. (2001b), biomass and atoll residence time were found to be linearly related for large Tuamotu atolls, a more open atoll would have a reduced residence time leading to reduced biomass contribution to the coastal ocean and a reduction to IME. Moreover, atmospheric and ocean warming are affecting wind and wave patterns, which may alter atoll reef structure and could affect water residence time (Andréfouët et al., 2001a; Duvat et al., 2017; Goeldner-Gianella et al., 2019).
Conclusion
We investigated the occurrence of IME at Rangiroa Atoll and its relationship to physical forcing by analyzing satellite data and in situ data. Comparison of chlorophyll-a concentration near Tiputa Channel with offshore resulted in a phytoplankton enhancement nearshore as high as 130% and an average enhancement nearshore of 16% during the 16-year record. Our statistical model examining physical drivers showed the magnitude of IME to be significantly enhanced by higher sea surface temperature (SST) and lower photosynthetically active radiation (PAR). In situ measurements and historical studies indicate that the lagoon likely presents a source of increased nutrients for phytoplankton production compared to the oligotrophic ocean that surrounds it. A combination of biological processes and long water residence time in the lagoon causes particulate-rich and likely nutrient-rich water to be advected to surrounding coastal waters by tidal and wave forcing. A biological oasis in the vast oligotrophic Pacific could not exist without increased phytoplankton biomass in Rangiroa’s coastal waters. Tourism and pearl farming are the main sources of income in the Tuamotu Archipelago (Chevalier et al., 2017), making the biologically productive lagoon Rangiroa’s primary resource. Understanding long term trends of chlorophyll enhancement in Rangiroa as well as the dual impact of physical and biological processes driving enhanced IME has important implications for the marine ecosystem and economy of the entire Tuamotu Archipelago now and in the future.
Data Availability Statement
The raw data supporting the conclusions of this article will be made available by the authors, without undue reservation.
Author Contributions
CV contributed with satellite data analysis, observational data analysis, and writing the manuscript. PM conducted the statistical analysis and contributed to writing the manuscript. JG contributed in the formulation of ideas, planning of field study, participation in field study, and writing the manuscript. AN conducted the statistical analysis and contributed to writing the manuscript. MM contributed in the formulation of ideas, planning of field study, and writing the manuscript. All authors contributed to the article and approved the submitted version.
Funding
U.S. Veterans Affairs paid CV’s tuition through the Post-9/11 GI Bill. This is an earned benefit granted by law to people who served in the US active duty military for at least 90 days.
Conflict of Interest
The authors declare that the research was conducted in the absence of any commercial or financial relationships that could be construed as a potential conflict of interest.
Acknowledgments
This work was the basis of the Master thesis of CV. We thank Dr. Brian Powell and Dr. Jeff Drazen for serving on CV’s committee and reviewing her thesis. We thank Kamaki Worthington and Brian Zgliczynski for diving support in Rangiroa, Conor Jerolmon for early analysis of the in situ CTD data, and Gordon Walker for providing significant logistical support for the field program. Finally, we thank John Hillsman and Howard McPherson for their guidance.
Footnotes
- ^ https://coastwatch.pfeg.noaa.gov/erddap/griddap/erdQSwind1day.html
- ^ https://coastwatch.pfeg.noaa.gov/erddap/griddap/erdQAwind1day.html
- ^ https://pmm.nasa.gov/data-access/downloads/gpm
- ^ https://www.pacificclimatechange.net/document/cawcr-wave-hindcast-1979-2010
- ^ http://oos.soest.hawaii.edu/erddap/griddap/NWW3_Global_Best.html
- ^ https://www.esrl.noaa.gov/psd/enso/mei/
- ^ http://www.seabird.com
- ^ http://www.wetlabs.com
- ^ http://www.biospherical.com
References
Abbott, M. L. (2017). Using Statistics in the Social and Health Sciences with SPSS §and Excel §. Hoboken, NJ: John Wiley & Sons, Inc.
Andrade, I., Sangrà, P., Hormazabal, S., and Correa-Ramirez, M. (2014). Island mass effect in the Juan Fernández Archipelago (33°S), Southeastern Pacific. Deep Sea Res. Part I Oceanogr. Res. Pap. 84, 86–99. doi: 10.1016/j.dsr.2013.10.009
Andréfouët, S., Claereboudt, P., Matsakis, J., Pagès, J., and Dufour, P. (2001a). Typology of atoll rims in Tuamotu Archipelago (French Polynesia) at landscape scale using SPOT HRV images. Int. J. Remote Sens. 22, 987–1004. doi: 10.1080/014311601300074522
Andréfouët, S., Pagès, J., and Tartinville, B. (2001b). Water renewal time for classification of atoll lagoons in the Tuamotu Archipelago (French Polynesia). Coral Reefs. 20, 399–408. doi: 10.1007/s00338-001-0190-9
Andréfouët, S., Ardhuin, F., Queffeulou, P., and Le Gendre, R. (2012). Island shadow effects and the wave climate of the Western Tuamotu Archipelago (French Polynesia) inferred from altimetry and numerical model data. Mar. Poll. Bull. 65, 415–424. doi: 10.1016/j.marpolbul.2012.05.042
Atkinson, M. (2011). “An ecosystem in transition,” in Coral Reefs: An Ecosystem in Transition, eds Z. Dubinsky and N. Stambler (New York, NY: Springer), 199–206.
Barton, K. (2009). Mu-MIn: Multi-Model Inference. R Package Version 0.12.2/r18. Available online at: http://R-Forge.R-project.org/projects/mumin/ (accessed May 8, 2020).
Breheny, P., and Burchett, W. (2017). Visualization of regression models using visreg. R J. 9, 56–71. doi: 10.32614/rj-2017-046
Burkepile, D. E., Allgeier, J. E., Shantz, A. A., Pritchard, C. E., Lemoine, N. P., Bhatti, L. H., et al. (2013). Nutrient supply from fishes facilitates macroalgae and suppresses corals in a Caribbean coral reef ecosystem. Sci. Rep. 3:1493.
Caldeira, R. M. A., Groom, S., Miller, P., Pilgrim, D., and Nezlin, N. P. (2002). Sea-surface signatures of the island mass effect phenomena around Madeira Island, Northeast Atlantic. Remote Sens. Environ. 80, 336–360. doi: 10.1016/s0034-4257(01)00316-9
Callaghan, D. P., Nielsen, P., Cartwright, N., Gourlay, M. R., and Baldock, T. E. (2006). Atoll lagoon flushing forced by waves. Coast. Eng. 53, 691–704. doi: 10.1016/j.coastaleng.2006.02.006
Caroline, P., and Van Cappellen, S. P. (2004). Nutrient inputs to the coastal ocean through submarine groundwater discharge: controls and potential impact. J. Hydrol. 295, 64–86. doi: 10.1016/j.jhydrol.2004.02.018
Carter, G. S., Gregg, M. C., and Merrifield, M. A. (2006). Flow and mixing around a small seamount on kaena ridge. Hawaii. J. Phys. Oceanogr. 36, 1036–1052. doi: 10.1175/jpo2924.1
Charpy, L. (1996). Phytoplankton biomass and production in two tuamotu atoll lagoons (French polynesia). Mar. Ecol. Progr. Ser. 145, 133–142. doi: 10.3354/meps145133
Charpy, L., Dufour, P., and Garcia, N. (1997). Particulate organic matter in sixteen Tuamotu atoll lagoons (French Polynesia). Mar. Ecol. Prog. Ser. 151, 55–65. doi: 10.3354/meps151055
Charpy-Roubaud, C., Charpy, L., and Cremoux, J. L. (1990). Nutrient budget of the lagoonal waters in an open central South Pacific atoll (Tikehau, Tuamotu, French Polynesia). Mar. Biol. 107, 67–73. doi: 10.1007/bf01313243
Charpy-Roubaud, C., Charpy, L., and Larkum, A. W. D. (2001). Atmospheric dinitrogen fixation by benthic communities of Tikehau Lagoon (Tuamotu Archipelago, French Polynesia) and its contribution to benthic primary production. Mar. Biol. 139, 991–998. doi: 10.1007/s002270100636
Chevalier, C., Devenon, J. L., Pagano, M., Rougier, G., Blanchot, J., and Arfi, R. (2017). The atypical hydrodynamics of the Mayotte Lagoon (Indian Ocean): effects on water age and potential impact on plankton productivity. Estuar.Coast. Shelf Sci. 196, 182–197. doi: 10.1016/j.ecss.2017.06.027
Dandonneau, Y., and Charpy, L. (1985). An empirical approach to the island mass effect in the south tropical Pacific based on sea surface chlorophyll concentrations. Deep Sea Res. Part I Oceanogr. Res. Pap. 32, 707–721. doi: 10.1016/0198-0149(85)90074-3
Delesalle, B., and Sournia, A. (1991). Residence time of water and phytoplankton biomass in coral reef lagoons. Cont. Shelf Res. 12, 939–949. doi: 10.1016/0278-4343(92)90053-m
Duarte, C., and Cebrian, J. (1996). The fate of marine autotrophic production. Limnol. Oceanogr. 41, 1758–1766. doi: 10.4319/lo.1996.41.8.1758
Dunstan, P. K., Foster, S. D., King, E., Risbey, J., O’Kane, T. J., Monselesan, D., et al. (2018). Global patterns of change and variation in sea surface temperature and chlorophyll a. Sci. Rep. 8:14624.
Duvat, V. K. E., Salvat, B., and Salmon, C. (2017). Drivers of shoreline change in atoll reef islands of the Tuamotu Archipelago, French Polynesia. Glob. Planet. Change. 158, 134–154. doi: 10.1016/j.gloplacha.2017.09.016
Ferrier-Pagès, C., and Furla, P. (2001). Pico- and nanoplankton biomass and production in the two largest atoll lagoons of French Polynesia. Mar. Ecol. Prog. Ser. 211, 63–76. doi: 10.3354/meps211063
Flombaum, P., Gallegos, J. L., Gordillo, R. A., Rincón, J., Zabala, L. L., and Jiao, N. (2013). Present and future global distributions of the marine Cyanobacteria Prochlorococcus and Synechococcus. PNAS 110, 9824–9829.
Gilmartin, M., and Revelante, N. (1974). The ‘island mass’ effect on the phytoplankton and primary production of the Hawaiian Islands. J. Exp. Mar. Biol. Ecol. 16, 181–204. doi: 10.1016/0022-0981(74)90019-7
Goeldner-Gianella, L., Grancher, D., Magnan, A. K., de Belizal, E., and Duvat, V. K. E. (2019). The perception of climate-related coastal risks and environmental changes on the Rangiroa and Tikehau atolls, French Polynesia: the role of sensitive and intellectual drivers. Ocean. Coast. Manag. 172, 14–29. doi: 10.1016/j.ocecoaman.2019.01.018
Gove, J. M., McManus, M. A., Neuheimer, A. B., Polovina, J. J., Drazen, J. C., Smith, C. R., et al. (2016). Near-island biological hotspots in barren ocean basins. Nat. Commu. 7, 1–8.
Gove, J. M., Williams, G. J., McManus, M. A., Heron, S. F., Sandin, S. A., Vetter, O. J., et al. (2013). Quantifying climatological ranges and anomalies for pacific coral reef ecosystems. PloS One 8: e61974. doi: 10.1371/journal.pone.0061974
Grolemund, G., and Wickham, H. (2011). Dates and times made easy with lubridate. J. Stat. Softw. 40, 1–25.
Hamner, W. M., and Hauri, I. R. (1981). Effects of island mass: water flow and plankton pattern around a reef in the Great Barrier Reef lagoon, Australia1. Limnol. Oceanogr. 26, 1084–1102. doi: 10.4319/lo.1981.26.6.1084
Heywood, K. J., Barton, E. D., and Simpson, J. H. (1990). The effects of flow disturbance by an oceanic island. J. Mar. Res. 48, 55–73. doi: 10.1357/002224090784984623
Institute of Statistics of French Polynesia (2017). French Polynesia Population and Housing Census 2017. Available online at: http://ghdx.healthdata.org/record/french-polynesia-population-and-housing-census-2017 (accessed October 27, 2020).
James, A. K., Washburn, L., Gotschalk, C., Maritorena, S., Alldredge, A., Nelson, C. E., et al. (2020). An Island mass effect resolved near Mo’orea, French Polynesia. Front. Mar. Sci. 7:16. doi: 10.3389/fmars.2020.00016
Jyothibabu, R., Arunpandi, N., Jagadeesan, L., Karnan, C., Lallu, K. R., and Vinayachandran, P. N. (2018). Response of phytoplankton to heavy cloud cover and turbidity in the northern Bay of Bengal. Sci. Rep. 8:11282.
Kirk, J. T. O. (2011). Light and Photosynthesis in Aquatic Ecosystems. New York, NY: Cambridge University Press.
Kumar, S., Kruger, J., Begg, Z., Handerson, E., and Alvis, E. (2013). Multibeam Bathymetry Survey Rangiroa, French Polynesia. Suva: SPC Applied Geoscience and Technology Division (SOPAC).
Leichter, J. J., Stokes, M. D., Hench, J. L., Witting, W., and Washburn, L. (2012). The island-scale internal wave climate of Moorea, French Polynesia. J. Geophys. Res. Oceans 117, 1–16. doi: 10.5479/si.00775630.309.1
Lo-Yat, A., Simpson, S. D., Meekan, M., Lecchini, D., Martinez, E., and Galzin, R. (2011). Extreme climatic events reduce ocean productivity and larval supply in a tropical reef ecosystem. Glob. Change Biol. 17, 1695–1702. doi: 10.1111/j.1365-2486.2010.02355.x
Martinez, E., and Maamaatuaiahutapu, K. (2004). Island mass effect in the Marquesas Islands: time variation. Geophys. Res. Lett. 31:L18307.
McCauley, D. J., DeSalles, P. A., Young, H. S., Dunbar, R. B., Dirzo, R., Mills, M. M., et al. (2012). From wing to wing: the persistence of long ecological interaction chains in less-disturbed ecosystems. Sci. Rep. 2:409.
McPhaden, M. J. (2012). A 21st century shift in the relationship between ENSO SST and warm water volume anomalies. Geophys. Res. Let. 39:L09706. doi: 10.1029/2012GL051826
Moehlenkamp, P., Beebe, C. K., McManus, M. A., Kawelo, A. H., Kotubetey, K., Lopez-Guzman, M., et al. (2019). Ku Hou Kuapa: cultural restoration improves water budget and water quality dynamics in He’eia Fishpond. Sustainability 11:161. doi: 10.3390/su11010161
Nelson, C. E., Alldredge, A. L., McCliment, E. A., Amaral-Zettler, L. A., and Carlson, C. A. (2011). Depleted dissolved organic carbon and distinct bacterial communities in the water column of a rapid-flushing coral reef ecosystem. ISME J. 5, 1374–1387. doi: 10.1038/ismej.2011.12
Pagès, J., and Andréfouët, S. (2001). A reconnaissance approach for hydrology of atoll lagoons. Coral Reefs 20, 409–414. doi: 10.1007/s00338-001-0192-7
Palacios, D. M. (2002). Factors influencing the island-mass effect of the Galápagos Archipelago. Geophys. Res. Lett. 29, 1–4.
R Core Team (2018). R: A Language and Environment for Statistical Computing. Vienna: R Foundation for Statistical Computing.
Rougerie, F., Fichez, R., and Déjardin, R. (2004). “Chapter 15 geomorphology and hydrogeology of selected Islands of French Polynesia: tikehau (Atoll) and tahiti (Barrier Reef),” in Developments in Sedimentology, Vol. 54, eds M. Rebesco and A. Camerlenghi Vol (Amsterdam: Elsevier Science & Technology). doi: 10.5479/si.00775630.415-part_iii.1
Sauzède, R., Martinez, E., de Fommervault, O. P., Poteau, A., Mignot, A., Maes, C., et al. (2018). Seasonal dynamics and disturbance of phytoplankton biomass in the wake of Tahiti as observed by biogeochemical-argo floats. Biogeosci. Discuss. 1–35. doi: 10.5194/bg-2017-541
Signorini, S. R., McClain, C. R., and Dandonneau, Y. (1999). Mixing and phytoplankton bloom in the wake of the Marquesas Islands. Geophys. Res. Lett. 26, 3121–3124. doi: 10.1029/1999gl010470
Smith, V. H., Tilman, G. D., and Nekola, J. C. (1999). Eutrophication: impacts of excess nutrient inputs on freshwater, marine, and terrestrial ecosystems. Environ. Pol. 100, 179–196. doi: 10.1016/s0269-7491(99)00091-3
Spall, M. A., and Pedlosky, J. (2013). Interaction of ekman layers and Islands. J. Phys. Oceanogr. 43, 1028–1041. doi: 10.1175/jpo-d-12-0159.1
Spiegel, M. R., and Stephens, L. J. (2011). Schaums Outlines Statistics. New York, NY: McGraw-Hill, 348–350.
Suzuki, Y., and Casareto, B. E. (2011). “The role of dissolved organic nitrogen (DON) in coral biology and reef ecology,” in Coral Reefs: An Ecosystem in Transition, eds Z. Dubinsky and N. Stambler (Berlin: Springer).
Uz, S. S., Busalacchib, A. J., Smith, T. M., Evans, M. N., Brown, C. W., and Hackert, E. C. (2017). Interannual and decadal variability in tropical Pacific chlorophyll from a statistical reconstruction: 1958-2008. J. Clim. 30, 7293–7315. doi: 10.1175/jcli-d-16-0202.1
Wang, B., Luo, X., Yang, Y. M., Sun, W., Cane, M. A., Cai, W., et al. (2019). Historical change of El Niño properties sheds light on future changes of extreme El Niño. PNAS 116, 22512–22517.
Williams, S. L., and Carpenter, R. C. (1988). Nitrogen-limited primary productivity ofcoral reef algal turfs: potential contribution of ammonium excreted by Diadema antillarum. Mar. Ecol. Prog. Ser. 47, 145–152. doi: 10.3354/meps047145
Wood, S. N. (2011). Fast stable restricted maximum likelihood and marginal likelihood estimation of semiparametric generalized linear models. J. R. Stat. Soc. Series B Stat. Methodol. 73, 3–36. doi: 10.1111/j.1467-9868.2010.00749.x
Wyatt, A. S. J., Lowe, R. J., Humphries, S., and Waite, A. M. (2010). Particulate nutrient fluxes over a fringing coral reef: relevant scales of phytoplankton production and mechanisms of supply. Mar. Ecol. Prog. Ser. 405, 113–130. doi: 10.3354/meps08508
Wyatt, A. S. J., Falter, J., Lowe, R., Humphries, S., and Waite, A. (2012). Oceanographic forcing of nutrient uptake and release over a fringing coral reef. Limnol. Oceanogr. 57, 401–419. doi: 10.4319/lo.2012.57.2.0401
Keywords: Island Mass Effect, Rangiroa atoll, phytoplankton biomass, Chlorophyll-a enhancement, IME
Citation: Vollbrecht C, Moehlenkamp P, Gove JM, Neuheimer AB and McManus MA (2021) Long-Term Presence of the Island Mass Effect at Rangiroa Atoll, French Polynesia. Front. Mar. Sci. 7:595294. doi: 10.3389/fmars.2020.595294
Received: 15 August 2020; Accepted: 04 December 2020;
Published: 13 January 2021.
Edited by:
Charitha Bandula Pattiaratchi, University of Western Australia, AustraliaReviewed by:
Zhongxiang Zhao, University of Washington, United StatesAdam Thomas Devlin, The Chinese University of Hong Kong, China
Copyright © 2021 Vollbrecht, Moehlenkamp, Gove, Neuheimer and McManus. This is an open-access article distributed under the terms of the Creative Commons Attribution License (CC BY). The use, distribution or reproduction in other forums is permitted, provided the original author(s) and the copyright owner(s) are credited and that the original publication in this journal is cited, in accordance with accepted academic practice. No use, distribution or reproduction is permitted which does not comply with these terms.
*Correspondence: Paula Moehlenkamp, cG1vZWhsZW5AaGF3YWlpLmVkdQ==