- 1Center of Marine Sciences, University of Algarve, Faro, Portugal
- 2Phycology Laboratory, Department of Botany, Biological Sciences Center, Federal University of Santa Catarina, Florianopolis, Brazil
Climate driven range shifts are driving the redistribution of marine species and threatening the functioning and stability of marine ecosystems. For species that are the structural basis of marine ecosystems, such effects can be magnified into drastic loss of ecosystem functioning and resilience. Rhodoliths are unattached calcareous red algae that provide key complex three-dimensional habitats for highly diverse biological communities. These globally distributed biodiversity hotspots are increasingly threatened by ongoing environmental changes, mainly ocean acidification and warming, with wide negative impacts anticipated in the years to come. These are superimposed upon major local stressors caused by direct destructive impacts, such as bottom trawling, which act synergistically in the deterioration of the rhodolith ecosystem health and function. Anticipating the potential impacts of future environmental changes on the rhodolith biome may inform timely mitigation strategies integrating local effects of bottom trawling over vulnerable areas at global scales. This study aimed to identify future climate refugia, as regions where persistence is predicted under contrasting climate scenarios, and to analyze their trawling threat levels. This was approached by developing species distribution models with ecologically relevant environmental predictors, combined with the development of a global bottom trawling intensity index to identify heavily fished regions overlaying rhodoliths. Our results revealed the importance of light, thermal stress and pH driving the global distribution of rhodoliths. Future projections showed poleward expansions and contractions of suitable habitats at lower latitudes, structuring cryptic depth refugia, particularly evident under the more severe warming scenario RCP 8.5. Our results suggest that if management and conservation measures are not taken, bottom trawling may directly threaten the persistence of key rhodolith refugia. Since rhodoliths have slow growth rates, high sensitivity and ecological importance, understanding how their current and future distribution might be susceptible to bottom trawling pressure, may contribute to determine the fate of both the species and their associated communities.
Introduction
In a world where climate is changing rapidly affecting the distribution of marine species (Cheung et al., 2009; Leadley et al., 2010; Poloczanska et al., 2013; Pecl et al., 2017), climate refugia, i.e., regions with stable habitat conditions through variable time intervals, may play a fundamental role in enabling population persistence, preserving local ecosystem functioning and serving as sources for the replenishment of impacted regions (Hewitt, 2004; Provan and Bennett, 2008; Keppel et al., 2012). This is particularly relevant for low dispersive species whose limited ability to track climate change by migration to new suitable regions may result in regional extinctions (Assis et al., 2017b). Climate change impacts may be greater when affecting ecosystem structuring species that support rich and diverse communities (Hoegh-Guldberg and Bruno, 2010). A collapse of such species, already observed for coral reefs (Carpenter et al., 2008; Hudges et al., 2018), kelp forests (Wernberg et al., 2015; Assis et al., 2017a) and seagrass meadows (Marbà and Duarte, 2010; Arias-Ortiz et al., 2018), has produced cascading effects reducing local biodiversity levels and disrupting ecosystem services (García Molinos et al., 2015; Pecl et al., 2017). In this scope, climate refugia function as regions of climate stability providing effective conservation of global biodiversity (inter and intra-specific) under climate change (Keppel et al., 2012; Morelli et al., 2016, 2020).
Despite the potential role of climate refugia, additional localized stressors may still compromise the future fate of ecosystems (Halpern et al., 2008; O’Leary et al., 2017). Cumulative effects of localized disturbances can act in synergy, accelerating or exacerbating the outcomes of climate change alone, and decreasing overall ecosystem resilience (Carilli et al., 2010; Costello et al., 2010; Halpern et al., 2015). For instance, bottom trawling has particularly destructive effects on benthic communities, decreasing biomass and eroding biogenic structures on the sea bottom (de Groot, 1984; Thrush and Dayton, 2002; Tillin et al., 2006; Olsgard et al., 2008). Conversely, reducing disturbance can have direct short or middle term impacts on ecosystems, in contrast to global climate change mitigation that can only be achieved in the long-term (Gurney et al., 2013; Strain et al., 2015). Nonetheless, analyses show that there are almost no pristine or unaffected ecosystems left globally, with overfishing and pollution being the primary threats to biodiversity (Costello et al., 2010; Halpern et al., 2015).
Under such serious threats to the future of marine biodiversity, maintenance of ecosystem functions will be very dependent on the persistence of ecosystem structuring species, such as seagrass, kelp, mangroves, corals (Hoegh-Guldberg and Bruno, 2010; Sasaki et al., 2015; O’Leary et al., 2017). Among such structural species, free-living calcareous algae commonly designated rhodoliths (of the orders Corallines, Hapalidiales and Sporolithales), are globally distributed, from the intertidal down to 270 m depth (Abella et al., 1998; Amado-Filho et al., 2012a; Riosmena-Rodriguez et al., 2017). Their three-dimensional structure provides habitat complexity creating biodiversity hotspots of highly diverse assemblages, while acting as shelters for invertebrates and other organisms, and as seed banks for algae (Abella et al., 1998; Amado-Filho, 2010; Fredericq et al., 2019; Veras et al., 2020). Additionally, they are important carbonate factories, contributing to the global carbon cycling (Amado-Filho et al., 2012a; van der Heijden and Kamenos, 2015). Although their ecological value has been acknowledged since the 19th century (Gran, 1893; Pruvot, 1897), rhodolith habitats are globally decreasing, mostly as a result of bottom fishing disturbance, and are expected to continue to decrease under the upcoming environmental changes, especially ocean warming and acidification (Hall-Spencer and Moore, 2000; Noisette et al., 2013; Martin and Hall-Spencer, 2017; Bernard et al., 2019). Anticipating and reducing the cumulative impacts on these rich and important ecosystems could improve their resilience, and in turn, promote and guide well-informed conservation and management of marine biodiversity.
This study aims to investigate the global future distributional shifts of the rhodolith biome under different climate change scenarios, using species distribution models, to identify crucial refugial regions providing persistent suitable habitat in the long-term. The major goal is to compare such persistent refugial habitat locations with their primary local threat, bottom trawling. This is done by quantifying global intensity of bottom trawling and overlaying it on predicted rhodolith distributions and their future climate refugia, in order to identify key regions of high conservation priority.
Materials and Methods
Environmental and Occurrence Data
In order to examine the potential consequences of future climate change on the rhodolith biome, a set of environmental predictors were selected based on their biological relevance to the rhodolith physiology (Carvalho et al., 2020). Benthic predictors for the annual average values of temperature (minimum and maximum), pH, light, nitrate, phosphate, salinity and currents, were downloaded from Bio-ORACLE (Tyberghein et al., 2012; Assis et al., 2017b) for the present and for two contrasting best and worst future scenarios: the Representative Concentration Pathway (RCP) 2.6 characterized by a large reduction of greenhouse emissions over time and RCP 8.5, where emissions are predicted to continue to increase over time (Moss et al., 2010). Additionally, to account for sediment and pollutant load that may negatively influence the presence of rhodoliths (Wilson et al., 2004; Villas-Bôas et al., 2014), we developed environmental predictors accounting for the distance from major coastal cities (population >75th percentile of the distribution of population)1 and the distance from river deltas (Global Estuaries Database; UNEP + Sea Around Us, 2003) as a proxy. Distances were produced as raster layers matching the resolution of Bio-ORACLE.
Rhodolith data were compiled by selecting species that primarily form rhodoliths (Supplementary Material 1). This conservative approach was preferred to a more extensive selection of species, as information about the form of records (e.g., rhodoliths or crustose) is not available in the major online repositories and/or because of major taxonomic issues resulting to erratic records (e.g., the case of Lithophyllum stictiforme, where numerous species are passing under that name; Pezzolesi et al., 2019). Detailed georeferenced occurrence records of the selected species were compiled from OBIS (2020) and GBIF.org (2020). Additional records were extracted from a systematic search of the literature on the Web of Knowledge using each species name as a searching criterion (Supplementary Material 2). The compiled dataset was subjected to pruning by removing records on land, and outside known geographical and depth distributions (max 270 m; Riosmena-Rodriguez et al., 2017). Further, potential spatial autocorrelation was reduced by estimating the minimum correlated distance of the environmental predictors and leaving only one occurrence record within such distance.
As the biogeographical affinities and distributions of the selected species differed greatly (e.g., from polar to tropical species) with likely differences in physiological responses, the data were divided into two separate datasets for species distribution modeling: (1) polar-cold temperate and (2) tropical-warm temperate affiliated species (Supplementary Material 1).
Species Distribution Modeling
Species distribution models were developed using two machine learning algorithms: boosted regression trees (BRT; De’ath, 2007) and Adaptive Boosting (AdaBoost; Hothorn et al., 2010). Because these models use both presence and absence data (not available), a number of pseudo-absences equal to the number of presences were generated and chosen randomly from a kernel probability surface developed with the occurrence records (e.g., Assis et al., 2018). Cross-validation interactions (CV) were developed to optimize model parameters and assess for potential transferability by partitioning the occurrence records (presences and pseudo-absences) into independent random blocks (Valavi et al., 2019). Models were fitted with all combinations of model parameters for BRT (tree complexity: 1–6; learning rate: 0.01, 0.005, and 0.001; number of trees: 50–1,000, step 50) and AdaBoost (shrinkage: 0.25–1, step 0.25; number of interactions: 50–250, step 50; degrees of freedom: 1–12) and their predictive performance was evaluated by one withheld block at a time. For each model, a predictive map was developed and reclassified into a binary presence-absence surface, based on a threshold maximizing true skill statistics (Allouche et al., 2006). Model performance was assessed by AUC (Area Under Curve; Fielding and Bell, 1997) and sensitivity (true positive rate). Overfitting of final predictive models was reduced by using the parameters retrieving higher performance in CV and by forcing monotonic responses (e.g., negative for maximum temperatures and positive for all other environmental variables; Elith et al., 2008; Boavida et al., 2016; Assis et al., 2018).
In order to determine the relative contribution of each environmental predictor to the model, the mean increase in deviance (i.e., goodness of fit) was computed by adding a given predictor at a time to all alternative models (e.g., Assis et al., 2018). The significance of the models was estimated as the relative contribution of each environmental predictor to the model performance (Elith et al., 2008). Limiting thresholds were extracted from the individual fitting function of each predictor alone while averaging the effect of all alternative predictors, and were then compared with available literature for physiological limits (Assis et al., 2018). Reduced models with less predictors and higher potential for transferability were built by interactively removing one predictor at a time from the full model (i.e., all predictors), starting from the least to the highest contributing variable, until the difference in deviance between full and reduced model was higher than zero (Elith et al., 2008; Boavida et al., 2016; Assis et al., 2018). Final distribution maps for polar—cold temperate and tropical—warm temperate species were produced from the respective reduced models, averaging the BRT and AdaBoost with the highest predictive performance (Araújo and New, 2007). Forecasts for future refugia, contraction and expansion regions were inferred by comparing present and future predictions. In order to address conclusions at the biome level, these regions were aggregated into a unique map describing refugia, contraction and expansion areas for both polar—cold temperate and tropical—warm temperate species.
Bottom Trawling Overlay Estimation
Global data for bottom trawling reported for 2012–2016 (in days of activity per year) were downloaded from the Global Fishing Watch (Kroodsma et al., 2018). This information was used to generate a layer of “mean bottom trawling activity per year” matching the resolution and extent of Bio-ORACLE. This was used to infer the potential trawling impacts on rhodoliths globally, along the present and future distributions (local impacts on regions of refugia, contraction, and expansion). Areas were estimated per marine biogeographic realm (Spalding et al., 2007) after aggregating final distribution maps of polar—cold temperate and tropical—warm temperate species.
Species distribution modeling, trawling overlay area calculations and final maps were produced in R (R Development Core Team, 2018) using the packages blockCV, dismo, EMNeval, gbm, gdata, gstat, leaflet, mboost, parallel, raster, rgeos, rgdal, SDMTools, ggplot, and sp.
Results
The initial 19,477 georeferenced occurrence records compiled for the rhodolith biome resulted into a final pruned dataset of 802 occurrences (Supplementary Figure 1). The models developed to predict the occurrence of rhodoliths retrieved a good performance for both warm and cold affiliated species (AUC: 0.87 and 0.92; sensitivity: 0.73 and 0.87, respectively) and showed distributions mostly determined by light availability (relative contribution: 33.35 ± 9.29), maximum (18.81% ± 5.11) and minimum temperature (19.45% ± 9.67) and pH (6.32 ± 4.78; Figure 1). The partial dependency plots showed suitable conditions for the warm affiliated species where light was above ∼2 × 10–4 E ∗ m2 ∗ yr–1, temperature range 4–34°C and pH above 7.7 (Supplementary Figure 2); and for cold affiliated species where light was ∼ 2 × 10–4 E ∗ m2 ∗ yr–1, temperature range −1.7–31°C and pH 7.9, respectively (Supplementary Figure 3). Global suitable rhodolith area for present-day conditions was estimated to ∼4.1 million km2, distributed between 1 and 300 m depth (Figure 2 and Supplementary Figure 4). The largest suitable areas were predicted in the temperate northern Atlantic (∼46% of the total distribution), followed by the Temperate Australasia (∼15%) and Temperate South America realms (∼15%; Supplementary Table 1).
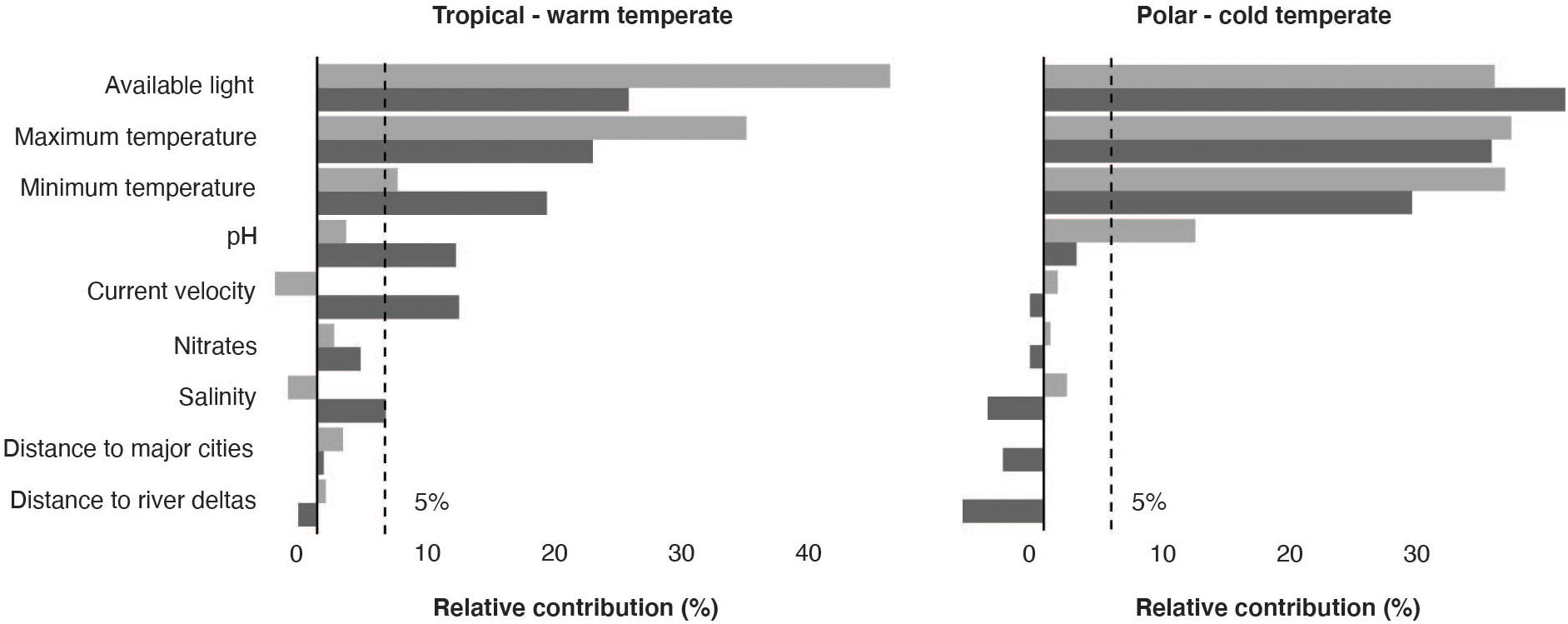
Figure 1. Benthic environmental predictors used in adaptive boosting (light gray) and boosted regression trees (dark gray) and their relative contribution to the models’ predictions for tropical—warm temperate and polar—cold temperate affiliated species.
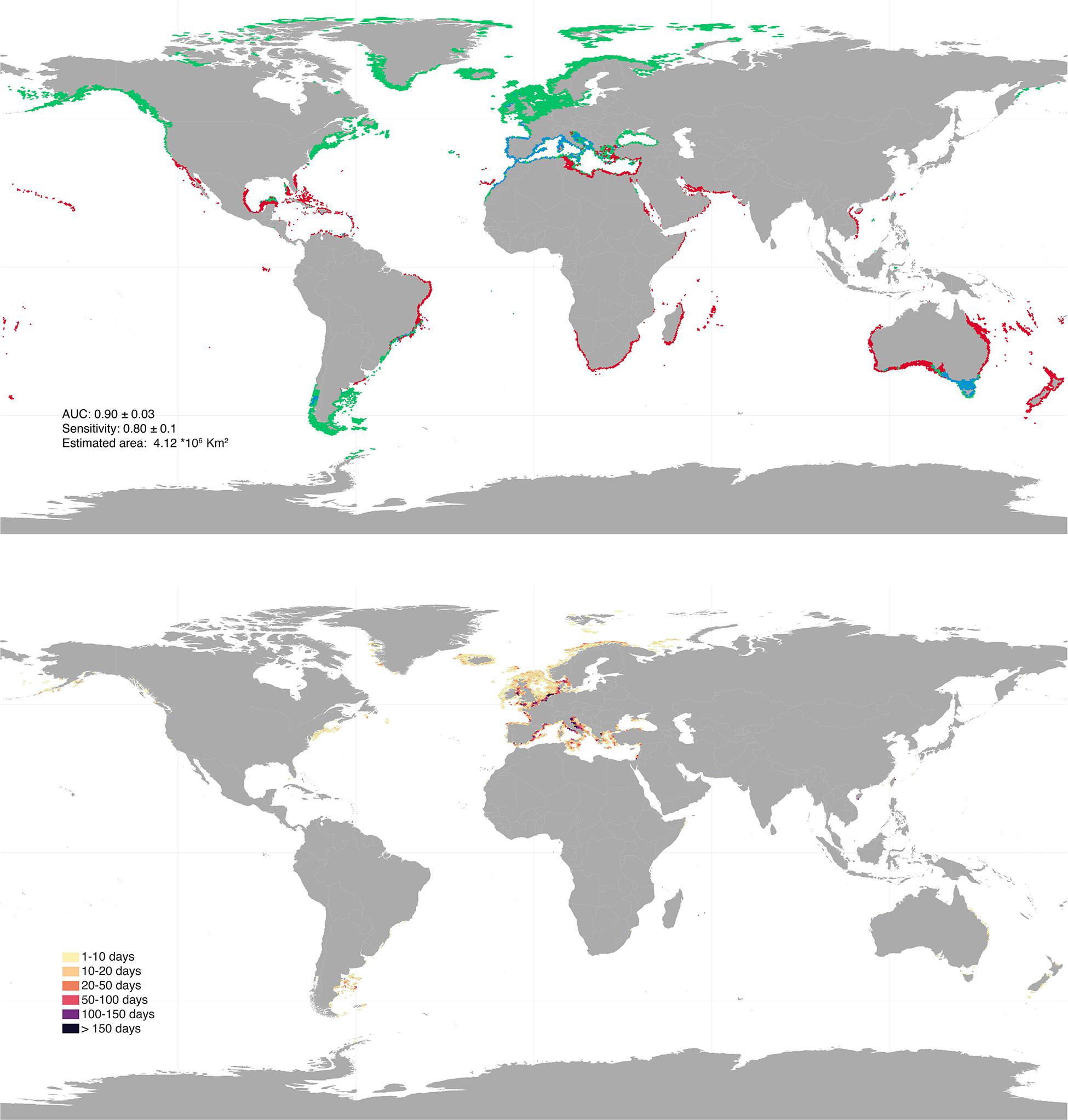
Figure 2. Upper panel: global present-day rhodolith distribution as inferred for polar—cold temperate (green) and tropical—warm temperate affiliated species (red). Regions of overlap are depicted in blue. Bottom panel: bottom trawling intensity (days per year) overlaying rhodolith suitable habitats for present day distribution. Results were aggregated for better visualization. High resolution maps can be found in Supplementary Material.
Future projections varied depending on the climatic scenarios used, with RCP 8.5 causing the most profound distributional changes (Figures 3, 4). Shifts to boreal and deeper areas were estimated to cause ∼19–50% range expansion, mostly in regions of the Temperate Northern Pacific (e.g., Japan, Okhotsk and Bering Seas) and the Artic (e.g., Labrador, Greenland and Norwegian Seas; Supplementary Table 1). At the same time, approximately 26–44% range contraction was estimated, particularly affecting shallower and tropical regions. Specifically, the tropical regions of the Eastern and Western Indo-Pacific were predicted to lose most of their rhodolith suitable habitats (67–80% and 62–81%, respectively; Supplementary Table 1). Taken together, these projections allowed to locate climatic refugia, i.e., regions providing suitable conditions for the present and the future (Figures 3, 4). These represented 56–74% of the present distribution and were mostly located in the temperate regions of Northern Atlantic (∼1.1–1.4 million km2), South America (∼346–353 thousands km2) and Australasia (∼219–496 thousands km2; Supplementary Table 1).
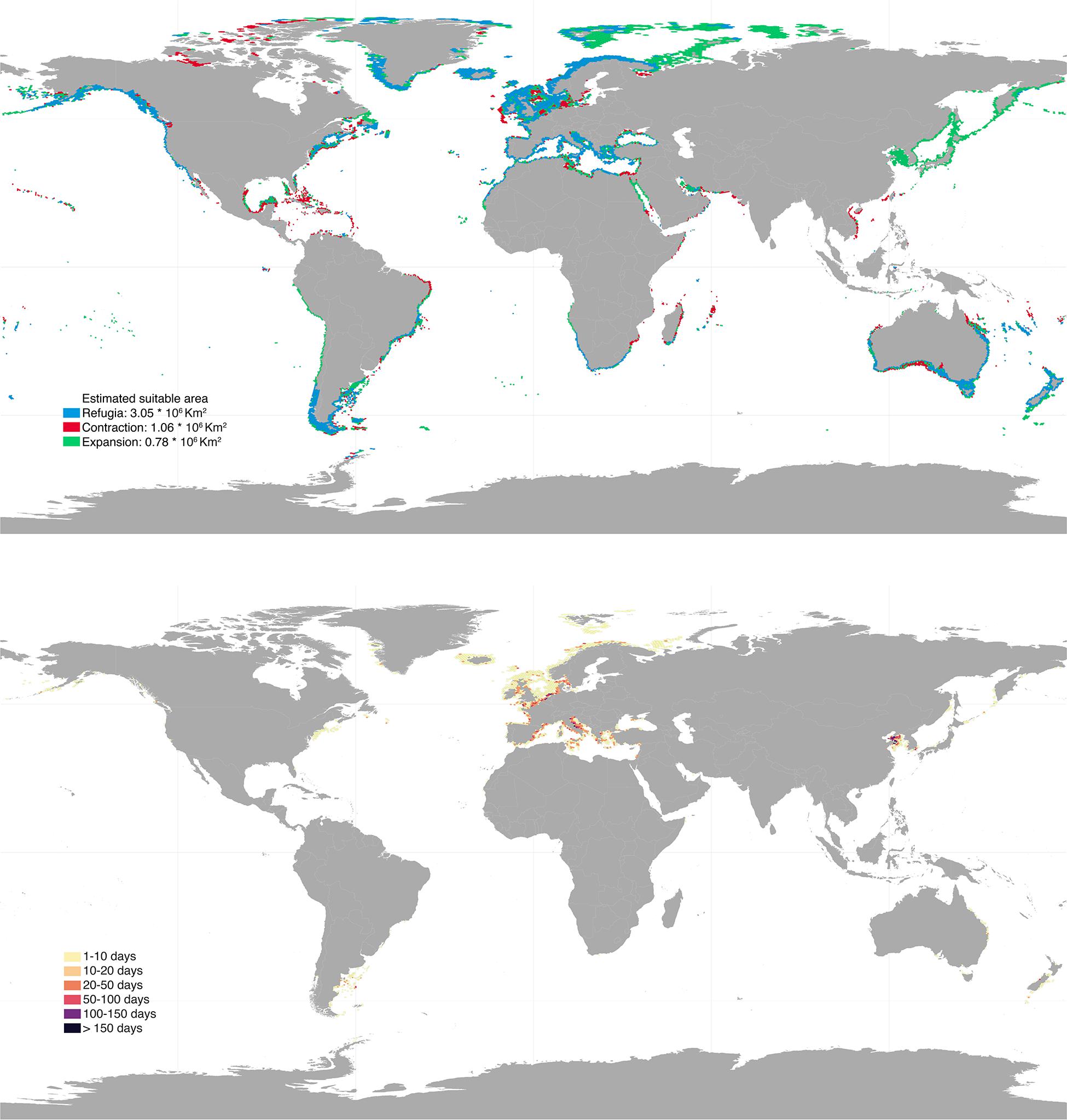
Figure 3. Upper panel: global projected future distribution for rhodoliths under RCP 2.6 scenario. Blue, red, and green colors depict regions of refugia, range contraction and range expansion, respectively. Bottom panel: bottom trawling intensity (days per year) overlaying rhodolith suitable habitats under RCP 2.6. Results were aggregated for better visualization. High resolution maps can be found in Supplementary Material.
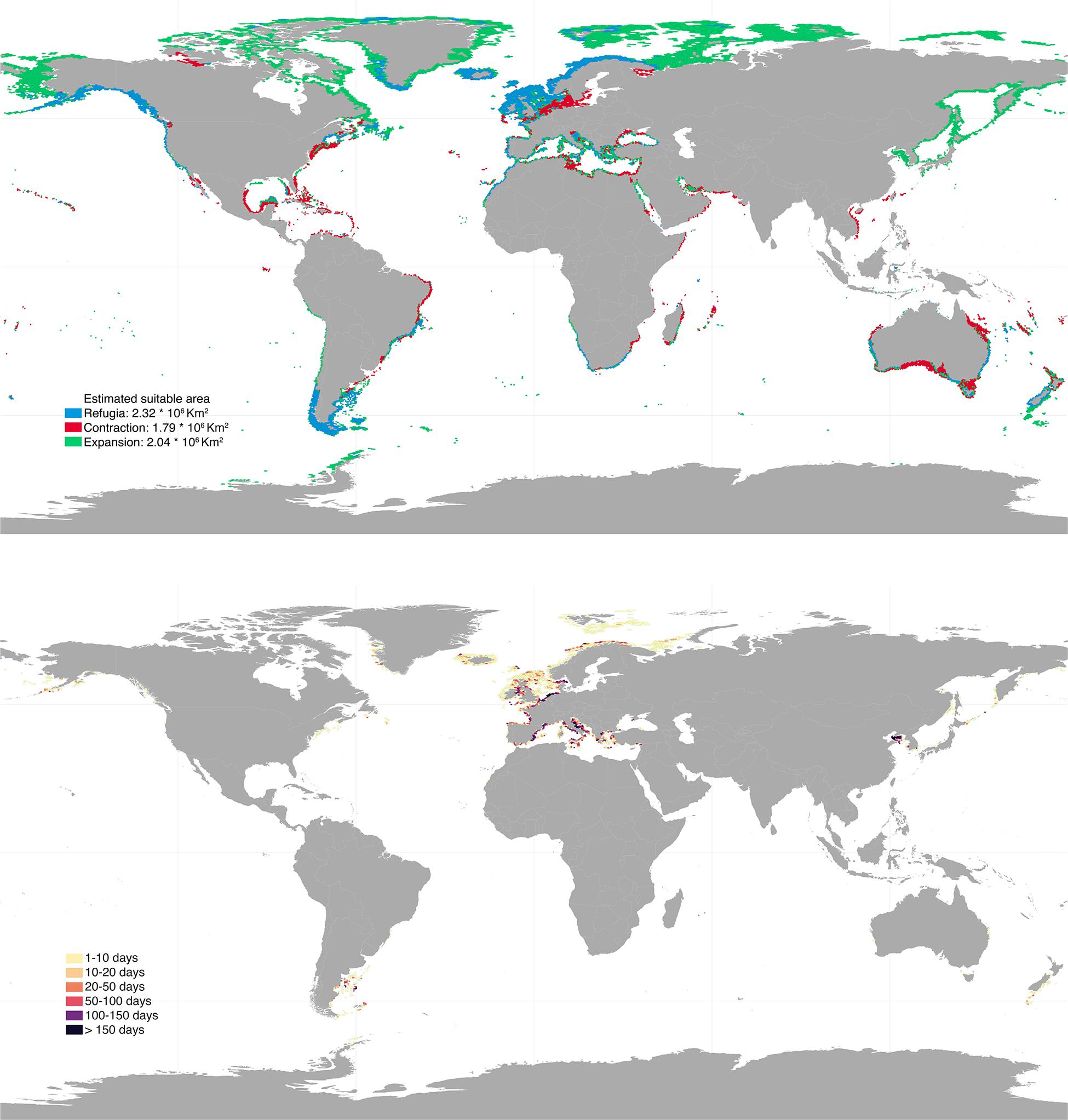
Figure 4. Upper panel: global projected future distribution for rhodoliths under RCP 8.5 scenario. Blue, red, and green colors depict regions of refugia, range contraction and range expansion, respectively. Bottom panel: bottom trawling intensity (days per year) overlaying rhodolith suitable habitats under RCP 8.5. Results were aggregated for better visualization. High resolution maps can be found in Supplementary Material.
Bottom Trawling and Rhodolith Distribution
Overlaying global bottom trawling data with the distribution predicted for the present, revealed that ∼684 thousand km2 of rhodolith suitable habitats are currently being trawled (Figure 2 and Supplementary Table 2). The majority of trawling takes place on refugia (60–78%) located mostly in the Temperate Northern Atlantic (∼629 thousand km2; Supplementary Table 2), with an overall estimated intensity of ∼140,000 days per year (Supplementary Table 3). Projected boreal expansions show an increase of suitable habitats in areas of current intensive bottom trawling activity, especially for the Temperate Northern Pacific, where suitable conditions in intensively trawled regions are estimated to increase by 900–1.750% depending on the RCP scenario (Supplementary Table 2).
Discussion
Our analyses showed that rhodolith potential suitable area is ∼4.1 million km2, widely distributed around the world’s coastal regions. Future distribution areas are projected to undergo poleward and depth range shifts, coupled with a 26–44% range contraction in the shallower and tropical regions, a pattern highly dependent on the climate scenario considered. Projected refugia are mostly situated in temperate regions, with broader areas in the Northern Atlantic. Despite their ecological, conservational and evolutionary importance, refugia are currently under great local stress by intense bottom trawling, reaching up to ∼140,000 days, annually. Such localized and intense disturbances, acting in synergy with ongoing and projected climate changes, are likely to compromise the fate of the whole biome and its associated communities, since long lasting or irreversible impacts have already been observed in previously dredged regions, on the scale of years post-dredging (Hall-Spencer and Moore, 2000; Bernard et al., 2019).
Models showed that the distribution of rhodoliths is mainly shaped by light availability (above a minimum threshold), maximum and minimum temperature and pH (above a minimum threshold); environmental predictors that are directly linked with the physiology of photosynthetic calcifying organisms (Carvalho et al., 2020). While at the species level, limiting thresholds differ between rhodolith species (Martin and Hall-Spencer, 2017), our aim was a model-based inference of suitable habitats for the warm and cold rhodolith biomes (e.g., Jayathilake and Costello, 2018). The use of the newly developed pH benthic layers (Assis et al., 2017b) allowed to account for the potential role of ocean acidification, a well-described threat for rhodoliths (Kroeker et al., 2010; Noisette et al., 2013; Qui-Minet et al., 2019). Models inferred limiting thresholds (pH 7.7–7.9) closely corroborated ecophysiological experiments performed on rhodolith species, such as Lithothamnion glaciale (Büdenbender et al., 2011) and Lithothamnion corallioides (Noisette et al., 2013). However, contrasting responses between and within rhodolith species have been reported due to many complex and occasionally site-specific conditions (Sañé et al., 2016; Qui-Minet et al., 2019). Such conditions cannot be fully incorporated into large-scale modeling due to lack of regional data, resulting in frequent interpolation of presence data into regions of suitable habitat, where species do not occur or are not known to occur. In this scope, models’ predicted suitable habitats can overestimate the potentially realized coverage. In pursuit of reducing potential overestimation, a selection of the most relevant, meaningful and available benthic predictors was used to achieve the best possible predictions at global scales (Carvalho et al., 2020). Our approach successfully identified well-known rhodolith banks, such as those in the northern Atlantic, Mediterranean, Caribbean, Australia and Brazil (Steller et al., 2003; Hall-Spencer et al., 2010; Amado-Filho et al., 2012a; Basso et al., 2016; Harvey et al., 2017), as well as recently discovered ones (e.g., South Africa; Adams et al., 2020). Additionally, by modeling the whole biome, we provide a first-order estimate of the potentially suitable rhodolith habitat globally, matching in scale that of macroalgae and exceeding by 10-fold the seagrass area (Short et al., 2007; Krause-Jensen and Duarte, 2016; Unsworth et al., 2019).
Our results further anticipate how future climate change might substantially affect the extent and geographical distribution of rhodoliths, particularly drastically under RCP 8.5. In line with global trends for marine biodiversity (e.g., Poloczanska et al., 2013; Beaugrand et al., 2015), the rhodolith biome is expected to undergo boreal and depth range shifts, coupled with habitat contractions at lower latitudes. Broader expansions are predicted along the Northern Pacific and Atlantic coasts (both east and west) and the Arctic, followed by smaller expansions in the southern hemisphere, constrained around the limited coastline of the few available islands. However, predicted expansions may be held back by the rhodolith limited dispersal and very slow growth rate (Blake and Maggs, 2003; Bosence and Wilson, 2003; Amado-Filho et al., 2012b; Pardo et al., 2019). Even if establishment is achieved, rhodoliths may affect the ecological balance and structure of the native communities (Ehrenfeld, 2010). Whereas, at the lower latitudes, depth range shifts might partially safeguard habitat suitability, extensive range contractions are predicted for many tropical and shallow regions.
Our models suggest that range contractions will intensify under the combination of high temperatures and ocean acidification (e.g., in the Gulf of Mexico and the Caribbean Sea), corroborating previous experimental evidence with temperate (Noisette et al., 2013; Qui-Minet et al., 2019; Sordo et al., 2020) and tropical populations (Steller et al., 2007). Even in colder regions, such as the North Sea, the anticipated increase in acidification and temperature regimes is expected to result in range contraction (Supplementary Figure 5). The extensive projected losses may threaten regions of higher conservation value, with rich genetic and species diversity (Bittner et al., 2011; Basso et al., 2015; Gabrielson et al., 2018). Additionally, as rhodolith genetic and species diversity are still greatly underestimated (Twist et al., 2019; Caragnano et al., 2020), putative diversity losses may occur undetected, leading to potentially misperceived assessments of the rhodolith biome diversity and phylogeography, and gene pool conservation status (i.e., shifting genetic baselines; Assis et al., 2014). The exact ramifications of climate change on rhodolith ecosystems are hard to predict, as new insights on their role as bio-engineers and their complex relationships with associated species are only starting to be discovered (Steller et al., 2003; Horta et al., 2016; Gabara et al., 2018; Fredericq et al., 2019; Carvalho et al., 2020). For example, recent insights indicate that rhodoliths may be functioning as banks of microscopic stages (equivalent to seedbanks) for marine biodiversity, safeguarding species after local environmental stress incidents, as in the case of the 2010 Deepwater Horizon Oil Spill (Fredericq et al., 2019). As climate change is threatening the ecological balance of the complex and dynamic relationships shaped within the rhodolith ecosystems, potential cascading effects caused by species turnover (e.g., predominance of competitive fleshy algae) may lead to substantial biodiversity and biomass reduction of their entire ecosystem (Horta et al., 2016; Legrand et al., 2017; Gabara et al., 2018).
Combining distributional predictions through time yielded an estimate of climate refugia, i.e., key regions presenting suitable conditions for rhodoliths to thrive in both the present and future (Morelli et al., 2020). As rhodoliths support and promote high levels of biodiversity (Teichert, 2014; Gabara et al., 2018; Fredericq et al., 2019), their refugia may become important biodiversity hotspots and, therefore, high-priority conservation regions (Hoegh-Guldberg and Bruno, 2010; Groves et al., 2012). Such refugia were mainly predicted to persist in cold—temperate regions of the world, namely northern Mediterranean, northeast Atlantic and Pacific and south America (Figures 3, 4). In the tropical regions, limited rhodolith suitable habitats may still be safeguarded by depth, particularly under the RCP 8.5 scenario, but, the mitigation of climate change impacts may not ensure persistence, if other, more localized disturbances (such as trawling) are acting in synergy with climate change (Halpern et al., 2008; O’Leary et al., 2017).
Rhodolith refugia are under great local threat from intensive bottom trawling (∼79,000–114,000 days annually), requiring urgent conservation management measures and regulation of this activity. Bottom trawling causes massive mortality of rhodoliths and their associated organisms, as it crushes, buries and eliminates any natural bottom features, while the plume of sediments lifted by trawling reduces light availability, blocks photosynthesis and contributes to additional losses of nearby, non-trawled rhodoliths (Hall-Spencer and Moore, 2000; Thrush and Dayton, 2002; Steller et al., 2003; Wilson et al., 2004; Gabara et al., 2018; Bernard et al., 2019). Such profound impacts are mostly irreversible and damages can still be observed for years post-trawling (Hall-Spencer and Moore, 2000; but see Barberá et al., 2017; Ordines et al., 2017). The limited rhodolith growth rate confers them an almost non-renewable resource status over human-relevant timescales (Blake and Maggs, 2003; Bosence and Wilson, 2003; Wilson et al., 2004).
Although rhodoliths are listed as an endangered landscape and trawling is prohibited over two of the most common species in Europe (Lithothamnion coralliodes and Phymatolithon calcareum; Barbera et al., 2003), we show that globally, the rhodolith biome may be lacking efficient protection. All the more, our findings might be underestimating the extent of trawled rhodoliths, since not all countries have or report fishing data and because the available trawling data were just a snapshot of the global fishing activity between 2012 and 2015 (Anticamara et al., 2011; Kroodsma et al., 2018). In fact, bottom trawling has been going on for centuries and especially around Europe (de Groot, 1984; Thurstan et al., 2014), where most of the long-term suitable rhodolith habitats are predicted. Such evidence indicates that trawling could have already caused broad-scale habitat disturbance on the European rhodolith ecosystems.
To our knowledge, our findings provide the first global estimate of the (present and future) rhodolith biome distribution, while discussing the potential combined effects of local stressors and future climate change. The identification of key climate refugia areas can guide future conservation management strategies and help prioritize areas for conservation in which populations will persist under climate change (Groves et al., 2012; Morelli et al., 2016). Extensive range shifts are already being observed, but more aggressive changes are expected. Considering the uncertainty of climate scenarios and the difficulty of ensuring international cooperation to meet the goals of the Paris agreement to reduce the rate of emissions (UN Framework Convention on Climate Change), reducing stressors in the local scale is an effective way to successfully mitigate some of the major impacts these ecosystems face. This can be achieved in the short/mid-term by governmental regulations to change fishing grounds or replace highly destructive fishing gears. Such actions may contribute to a rapid reduction of the direct destruction of these ecosystems, determining the fate of important biomes to sustain marine biodiversity in general.
Data Availability Statement
The original contributions presented in the study are included in the article/Supplementary Material. Original rasters with model predictions and the modeling scripts can be found in figshare: https://doi.org/10.6084/m9.figshare.13295786.v2. Any further inquiries can be directed to the corresponding author/s.
Author Contributions
ES, JA, and EF conceived the study. JA produced and provided the scripts for the analyses. EF compiled the data and conducted the analyses and drafted the manuscript with the support of JA, ES, PH, and GK. All authors edited the manuscript before submission.
Funding
This study was supported by the Foundation for Science and Technology (FCT) of Portugal through the project UIDB/04326/2020, PTDC/BIA-CBI/6515/2020, fellowships SFRH/BD/144878/2019, SFRH/BSAB/150485/2019, the transitional norm—DL57/2016/CP1361/CT0035, and by a Pew Marine Fellowship.
Conflict of Interest
The authors declare that the research was conducted in the absence of any commercial or financial relationships that could be construed as a potential conflict of interest.
Acknowledgments
We thank the two reviewers for their very constructive suggestions that help substantially to the improvement of our manuscript.
Supplementary Material
The Supplementary Material for this article can be found online at: https://www.frontiersin.org/articles/10.3389/fmars.2020.594537/full#supplementary-material
Footnotes
References
Abella, E., Barberá, C., Borg, J. A., Glemarec, M., Grall, J., Hall-Spencer, J. M., et al. (1998). “Maërl grounds: habitats of high biodiversity in European seas,” in Proceedings of the Third European Marine Science and Technology Conference, Lisbon, 170–179.
Adams, L. A., Maneveldt, G. W., Green, A., Karenyi, N., Parker, D., Samaai, T., et al. (2020). Rhodolith bed discovered off the South African coast. Diversity 12:125. doi: 10.3390/d12040125
Allouche, O., Tsoar, A., and Kadmon, R. (2006). Assessing the accuracy of species distribution models: prevalence, kappa and the true skill statistic (TSS). J. Appl. Ecol. 43, 1223–1232. doi: 10.1111/j.1365-2664.2006.01214.x
Amado-Filho, G. (2010). Seaweed diversity associated with a Brazilian tropical rhodolith bed. Ciencias Mar. 36, 371–391. doi: 10.7773/cm.v36i4.1782
Amado-Filho, G. M., Moura, R. L., Bastos, A. C., Salgado, L. T., Sumida, P. Y., Guth, A. Z., et al. (2012a). Rhodolith beds are major CaCO 3 BIO-factories in the tropical south West Atlantic. PLoS One 7:e0035171. doi: 10.1371/journal.pone.0035171
Amado-Filho, G. M., Pereira-Filho, G. H., Bahia, R. G., Abrantes, D. P., Veras, P. C., and Matheus, Z. (2012b). Occurrence and distribution of rhodolith beds on the Fernando de Noronha Archipelago of Brazil. Aquat. Bot. 101, 41–45. doi: 10.1016/j.aquabot.2012.03.016
Anticamara, J. A., Watson, R., Gelchu, A., and Pauly, D. (2011). Global fishing effort (1950-2010): trends, gaps, and implications. Fish. Res. 107, 131–136. doi: 10.1016/j.fishres.2010.10.016
Araújo, M. B., and New, M. (2007). Ensemble forecasting of species distributions. Trends Ecol. Evol. 22, 42–47. doi: 10.1016/j.tree.2006.09.010
Arias-Ortiz, A., Serrano, O., Masqué, P., Lavery, P. S., Mueller, U., Kendrick, G. A., et al. (2018). A marine heatwave drives massive losses from the world’s largest seagrass carbon stocks. Nat. Clim. Chang. 8, 1–7. doi: 10.1038/s41558-018-0096-y
Assis, J., Araújo, M. B., and Serrão, E. A. (2018). Projected climate changes threaten ancient refugia of kelp forests in the North Atlantic. Glob. Chang. Biol. 24, e55–e66. doi: 10.1111/gcb.13818
Assis, J., Berecibar, E., Claro, B., Alberto, F., Reed, D., Raimondi, P., et al. (2017a). Major shifts at the range edge of marine forests: the combined effects of climate changes and limited dispersal. Sci. Rep. 7, 1–10. doi: 10.1038/srep44348
Assis, J., Serrão, E. A., Claro, B., Perrin, C., and Pearson, G. A. (2014). Climate-driven range shifts explain the distribution of extant gene pools and predict future loss of unique lineages in a marine brown alga. Mol. Ecol. 23, 2797–2810. doi: 10.1111/mec.12772
Assis, J., Tyberghein, L., Bosch, S., Verbruggen, H., Serrão, E. A., and De Clerck, O. (2017b). Bio-ORACLE v2.0: extending marine data layers for bioclimatic modelling. Glob. Ecol. Biogeogr. 27, 277–284. doi: 10.1111/geb.12693
Barbera, C., Bordehore, C., Borg, J. A., Glémarec, M., Grall, J., Hall-Spencer, J. M., et al. (2003). Conservation and management of northeast Atlantic and Mediterranean maerl beds. Aquat. Conserv. Mar. Freshw. Ecosyst. 13, 65–76. doi: 10.1002/aqc.569
Barberá, C., Mallol, S., Vergés, A., Cabanellas-Reboredo, M., Díaz, D., and Goñi, R. (2017). Maerl beds inside and outside a 25-year-old no-take area. Mar. Ecol. Prog. Ser. 572, 77–90. doi: 10.3354/meps12110
Basso, D., Babbini, L., Kaleb, S., Bracchi, V. A., and Falace, A. (2016). Monitoring deep Mediterranean rhodolith beds. Aquat. Conserv. Mar. Freshw. Ecosyst. 26, 549–561. doi: 10.1002/aqc.2586
Basso, D., Caragnano, A., Le Gall, L., and Rodondi, G. (2015). The genus Lithophyllum in the north-western Indian Ocean, with description of L. yemenense sp. nov., L. socotraense sp. nov., L. subplicatum comb. et stat. nov., and the resumed L. affine, L. kaiseri, and L. subreduncum (Rhodophyta, Corallinales). Phytotaxa 208, 183–200. doi: 10.11646/phytotaxa.208.3.1
Beaugrand, G., Edwards, M., Raybaud, V., Goberville, E., and Kirby, R. R. (2015). Future vulnerability of marine biodiversity compared with contemporary and past changes. Nat. Clim. Chang. 5, 695–701. doi: 10.1038/nclimate2650
Bernard, G., Romero-Ramirez, A., Tauran, A., Pantalos, M., Deflandre, B., Grall, J., et al. (2019). Declining maerl vitality and habitat complexity across a dredging gradient: insights from in situ sediment profile imagery (SPI). Sci. Rep. 9, 1–12. doi: 10.1038/s41598-019-52586-8
Bittner, L., Payri, C. E., Maneveldt, G. W., Couloux, A., Cruaud, C., de Reviers, B., et al. (2011). Evolutionary history of the Corallinales (Corallinophycidae, Rhodophyta) inferred from nuclear, plastidial and mitochondrial genomes. Mol. Phylogenet. Evol. 61, 697–713. doi: 10.1016/j.ympev.2011.07.019
Blake, C., and Maggs, C. A. (2003). Comparative growth rates and internal banding periodicity of maerl species (Corallinales, Rhodophyta) from northern Europe. Phycologia 42, 606–612. doi: 10.2216/i0031-8884-42-6-606.1
Boavida, J., Assis, J., Silva, I., and Serrão, E. A. (2016). Overlooked habitat of a vulnerable gorgonian revealed in the Mediterranean and Eastern Atlantic by ecological niche modelling. Sci. Rep. 6:36460. doi: 10.1038/srep36460
Bosence, D., and Wilson, J. (2003). Maerl growth, carbonate production rates and accumulation rates in the northeast Atlantic. Aquat. Conserv. Mar. Freshw. Ecosyst. 13, S21–S31. doi: 10.1002/aqc.565
Büdenbender, J., Riebesell, U., and Form, A. (2011). Calcification of the Arctic coralline red algae Lithothamnion glaciale in response to elevated CO 2. Mar. Ecol. Prog. Series 441, 79–87. doi: 10.3354/meps09405
Caragnano, A., Rodondi, G., Basso, D., Peña, V., le Gall, L., and Rindi, F. (2020). Circumscription of Lithophyllum racemus (Corallinales, Rhodophyta) from the western Mediterranean Sea reveals the species Lithophyllum pseudoracemus sp. nov. Phycologia [Epub ahead of print]. doi: 10.1080/00318884.2020.1829348
Carilli, J. E., Norris, R. D., Black, B., Walsh, S. M., and Mcfield, M. (2010). Century-scale records of coral growth rates indicate that local stressors reduce coral thermal tolerance threshold. Glob. Chang. Biol. 16, 1247–1257. doi: 10.1111/j.1365-2486.2009.02043.x
Carpenter, K. E., Abrar, M., Aeby, G., Aronson, R. B., Banks, S., Bruckner, A., et al. (2008). One-Third of Reef-Building Corals Face Elevated Extinction Risk from Climate Change and Local Impacts. Science 321, 560–563. doi: 10.1126/science.1159196
Carvalho, V. F., Assis, J., Serrão, E. A., Nunes, J. M., Anderson, A. B., Batista, M. B., et al. (2020). Environmental drivers of rhodolith beds and epiphytes community along the South Western Atlantic coast. Mar. Environ. Res. 154:104827. doi: 10.1016/j.marenvres.2019.104827
Cheung, W. W. L., Lam, V. W. Y., Sarmiento, J. L., Kearney, K., Watson, R., and Pauly, D. (2009). Projecting global marine biodiversity impacts under climate change scenarios. Fish Fish. 10, 235–251. doi: 10.1111/j.1467-2979.2008.00315.x
Costello, M. J., Coll, M., Danovaro, R., Halpin, P., Ojaveer, H., and Miloslavich, P. (2010). A census of marine biodiversity knowledge, resources, and future challenges. PLoS One 5:e0012110. doi: 10.1371/journal.pone.0012110
de Groot, S. J. (1984). The impact of bottom trawling on benthic fauna of the North Sea. Ocean Manag. 9, 177–190. doi: 10.1016/0302-184X(84)90002-7
Ehrenfeld, J. G. (2010). Ecosystem consequences of biological invasions. Annu. Rev. Ecol. Evol. Syst. 41, 59–80. doi: 10.1146/annurev-ecolsys-102209-144650
Elith, J., Leathwick, J. R., and Hastie, T. (2008). A working guide to boosted regression trees. J. Anim. Ecol. 77, 802–813. doi: 10.1111/j.1365-2656.2008.01390.x
Fielding, A. H., and Bell, J. F. (1997). A review of methods for the assessment of prediction errors in conservation presence/absence models. Environ. Conserv. 24, 38–49. doi: 10.1017/S0376892997000088
Fredericq, S., Krayesky-Self, S., Sauvage, T., Richards, J., Kittle, R., Arakaki, N., et al. (2019). The critical importance of rhodoliths in the life cycle completion of both macro- and microalgae, and as holobionts for the establishment and maintenance of marine biodiversity. Front. Mar. Sci. 5:502. doi: 10.3389/fmars.2018.00502
Gabara, S. S., Hamilton, S. L., Edwards, M. S., and Steller, D. L. (2018). Rhodolith structural loss decreases abundance, diversity, and stability of benthic communities at santa catalina island. CA Mar. Ecol. Prog. Ser. 595, 71–88. doi: 10.3354/meps12528
Gabrielson, P. W., Hughey, J. R., and Diaz-Pulido, G. (2018). Genomics reveals abundant speciation in the coral reef building alga Porolithon onkodes (Corallinales, Rhodophyta). J. Phycol. 54, 429–434. doi: 10.1111/jpy.12761
García Molinos, J., Halpern, B. S. S., Schoeman, D. S. S., Brown, C. J. J., Kiessling, W., Moore, P. J. J., et al. (2015). Climate velocity and the future global redistribution of marine biodiversity. Nat. Clim. Chang. 6, 4–11. doi: 10.1038/nclimate2769
GBIF.org (2020). GBIF Home Page. Available online at: https://www.gbif.org (accessed October 20, 2020).
Gran, H. H. (1893). Algevegetationen i Tønsbergfjorden. Skrifter udgivne af Videnskabsselskabet i Christiania. I. Math. Naturvidenskabelig Klasse 1893, 1–38.
Groves, C. R., Game, E. T., Anderson, M. G., Cross, M., Enquist, C., Ferdaña, Z., et al. (2012). Incorporating climate change into systematic conservation planning. Biodivers. Conserv. 21, 1651–1671. doi: 10.1007/s10531-012-0269-3
Gurney, G. G., Melbourne-Thomas, J., Geronimo, R. C., Aliño, P. M., and Johnson, C. R. (2013). Modelling coral reef futures to inform management: can reducing local-scale stressors conserve reefs under climate change? PLoS One 8:e0080137. doi: 10.1371/journal.pone.0080137
Hall-Spencer, J. M., Kelly, J., and Maggs, C. A. (2010). Background Document for Maërl beds Biodiversity Series. London: OSPAR.
Hall-Spencer, J. M., and Moore, P. G. (2000). Scallop dredging has profound, long-term impacts on maerl habitats. ICES J. Mar. Sci. 57, 1407–1415. doi: 10.1006/jmsc.2000.0918
Halpern, B. S., Frazier, M., Potapenko, J., Casey, K. S., Koenig, K., Longo, C., et al. (2015). Spatial and temporal changes in cumulative human impacts on the world’s ocean. Nat. Commun. 6:7615. doi: 10.1038/ncomms8615
Halpern, B. S., Walbridge, S., Selkoe, K. A., Kappel, C. V., Micheli, F., D’Agrosa, C., et al. (2008). A global map of human impact on marine ecosystems. Science 319, 948–952. doi: 10.1126/science.1149345
Harvey, A. S., Harvey, R. M., and Merton, E. (2017). The distribution, significance and vulnerability of Australian rhodolith beds: a review. Mar. Freshw. Res. 68, 411–428. doi: 10.1071/MF15434
Hewitt, G. M. (2004). Genetic consequences of climatic oscillations in the Quaternary. Philos. Trans. R. Soc. B Biol. Sci. 359, 183–195. doi: 10.1098/rstb.2003.1388
Hoegh-Guldberg, O., and Bruno, J. F. (2010). The impact of climate change on the World’s marine ecosystems. Science 328, 1523–1528. doi: 10.1126/science.1189930
Horta, P. A., Riul, P., Amado Filho, G. M., Gurgel, C. F. D., Berchez, F., Nunes, J. M., et al. (2016). Rhodoliths in Brazil: current knowledge and potential impacts of climate change. Braz. J. Oceanogr. 64, 117–136. doi: 10.1590/S1679-875920160870064sp2
Hothorn, T., Bühlmann, P., Kneib, T., Schmid, M., and Hofner, B. (2010). Model-based boosting 2.0. J. Mach. Learn. Res. 11, 2109–2113.
Hudges, T. P., Kerry, J. T., Baird, A. H., Connolly, S. R., Dietzel, A., Eakin, C. M., et al. (2018). Global warming transforms coral reef assemblages. Nature 556, 492–496.
Jayathilake, D. R. M., and Costello, M. J. (2018). A modelled global distribution of the seagrass biome. Biol. Conserv. 226, 120–126. doi: 10.1016/j.biocon.2018.07.009
Keppel, G., Van Niel, K. P., Wardell-Johnson, G. W., Yates, C. J., Byrne, M., Mucina, L., et al. (2012). Refugia: identifying and understanding safe havens for biodiversity under climate change. Glob. Ecol. Biogeogr. 21, 393–404. doi: 10.1111/j.1466-8238.2011.00686.x
Krause-Jensen, D., and Duarte, C. M. (2016). Substantial role of macroalgae in marine carbon sequestration. Nat. Geosci. 9, 737–742. doi: 10.1038/ngeo2790
Kroeker, K. J., Kordas, R. L., Crim, R. N., and Singh, G. G. (2010). Meta-analysis reveals negative yet variable effects of ocean acidification on marine organisms. Ecol. Lett. 13, 1419–1434. doi: 10.1111/j.1461-0248.2010.01518.x
Kroodsma, D. A., Mayorga, J., Hochberg, T., Miller, N. A., Boerder, K., Ferretti, F., et al. (2018). Tracking the global footprint of fisheries. Science 359, 904–908. doi: 10.1126/science.aao5646
Leadley, P., Pereira, H., Alkemade, R., Fernandez, J. F., Scharlemann, J. P. W., Proenca, V., et al. (2010). Biodiversity Scenarios: Projections of 21st century change in biodiversity and Associated Ecosystem Services. Technical Series no. 50. Cambridge, MA: WCMC.
Legrand, E., Riera, P., Lutier, M., Grall, J., and Martin, S. (2017). Species interactions can shift the response of a maerl bed community to ocean acidification and warming. Biogeosciences 14, 5359–5376. doi: 10.5194/bg-14-5359-2017
Marbà, N., and Duarte, C. M. (2010). Mediterranean warming triggers seagrass (Posidonia oceanica) shoot mortality. Glob. Chang. Biol. 16, 2366–2375. doi: 10.1111/j.1365-2486.2009.02130.x
Martin, S., and Hall-Spencer, J. M. (2017). “Effects of ocean warming and acidification on rhodolith/maërl beds,” in Rhodolith/Maërl Beds: A Global Perspective, eds W. Nelson, J. Aguirre, and R. Riosmena-Rodríguez (Cham: Springer), 55–85.
Morelli, T. L., Barrows, C. W., Ramirez, A. R., Cartwright, J. M., Ackerly, D. D., Eaves, T. D., et al. (2020). Climate change refugia: biodiversity change in the slow lane. Front. Ecol. Environ. 18, 228–234. doi: 10.1002/fee.2189
Morelli, T. L., Daly, C., Dobrowski, S. Z., Dulen, D. M., Ebersole, J. L., Jackson, S. T., et al. (2016). Managing climate change refugia for climate adaptation. PLoS One 11:e0159909. doi: 10.1371/journal.pone.0159909
Moss, R. H., Edmonds, J. A., Hibbard, K. A., Manning, M. R., Rose, S. K., Van Vuuren, D. P., et al. (2010). The next generation of scenarios for climate change research and assessment. Nature 463, 747–756. doi: 10.1038/nature08823
Noisette, F., Duong, G., Six, C., Davoult, D., and Martin, S. (2013). Effects of elevated pCO2 on the metabolism of a temperate rhodolith Lithothamnion corallioides grown under different temperatures. J. Phycol. 49, 746–757. doi: 10.1111/jpy.12085
O’Leary, J. K., Micheli, F., Airoldi, L., Boch, C., De Leo, G., Elahi, R., et al. (2017). The resilience of marine ecosystems to climatic disturbances. Bioscience 67, 208–220. doi: 10.1093/biosci/biw161
Olsgard, F., Schaanning, M. T., Widdicombe, S., Kendall, M. A., and Austen, M. C. (2008). Effects of bottom trawling on ecosystem functioning. J. Exp. Mar. Bio. Ecol. 366, 123–133. doi: 10.1016/j.jembe.2008.07.036
Ordines, F., Ramón, M., Rivera, J., Rodríguez-Prieto, C., Farriols, M. T., Guijarro, B., et al. (2017). Why long term trawled red algae beds off Balearic Islands (western Mediterranean) still persist? Reg. Stud. Mar. Sci. 15, 39–49. doi: 10.1016/j.rsma.2017.07.005
Pardo, C., Guillemin, M. L., Peña, V., Bárbara, I., Valero, M., and Barreiro, R. (2019). Local coastal configuration rather than latitudinal gradient shape clonal diversity and genetic structure of Phymatolithon calcareum maerl beds in North European Atlantic. Front. Mar. Sci. 6:149. doi: 10.3389/fmars.2019.00149
Pecl, G. T., Araújo, M. B., Bell, J. D., Blanchard, J., Bonebrake, T. C., Chen, I. C., et al. (2017). Biodiversity redistribution under climate change: impacts on ecosystems and human well-being. Science 355:eaai9214. doi: 10.1126/science.aai9214
Pezzolesi, L., Peña, V., Le Gall, L., Gabrielson, P. W., Kaleb, S., Hughey, J. R., et al. (2019). Mediterranean Lithophyllum stictiforme (Corallinales, Rhodophyta) is a genetically diverse species complex: implications for species circumscription, biogeography and conservation of coralligenous habitats. J. Phycol. 55, 473–492. doi: 10.1111/jpy.12837
Poloczanska, E. S., Brown, C. J., Sydeman, W. J., Kiessling, W., Schoeman, D. S., Moore, P. J., et al. (2013). Global imprint of climate change on marine life. Nat. Clim. Chang. 3, 919–925. doi: 10.1038/Nclimate1958
Provan, J., and Bennett, K. D. (2008). Phylogeographic insights into cryptic glacial refugia. Trends Ecol. Evol. 23, 564–571. doi: 10.1016/j.tree.2008.06.010
Pruvot, G. (1897). Essai sur les fonds et la faune de la Manche occidentale comparés à ceux du Golfe du Lion. Irch. Zooz. Exp. Gén. 5, 511–617.
Qui-Minet, Z. N., Coudret, J., Davoult, D., Grall, J., Mendez-Sandin, M., Cariou, T., et al. (2019). Combined effects of global climate change and nutrient enrichment on the physiology of three temperate maerl species. Ecol. Evol. 9, 13787–13807. doi: 10.1002/ece3.5802
R Development Core Team (2018). A Language and Environment for Statistical Computing. Vienna: R Found. Stat. Comput.
Riosmena-Rodriguez, R., Wendy, N., and Aguirre, J. (2017). Rhodolith/Maërl Beds: A Global Perspective. Cham: Springer International Publishing.
Sañé, E., Chiocci, F. L., Basso, D., and Martorelli, E. (2016). Environmental factors controlling the distribution of rhodoliths: an integrated study based on seafloor sampling, ROV and side scan sonar data, offshore the W-Pontine Archipelago. Cont. Shelf Res. 129, 10–22. doi: 10.1016/j.csr.2016.09.003
Sasaki, T., Furukawa, T., Iwasaki, Y., Seto, M., and Mori, A. S. (2015). Perspectives for ecosystem management based on ecosystem resilience and ecological thresholds against multiple and stochastic disturbances. Ecol. Indic. 57, 395–408. doi: 10.1016/j.ecolind.2015.05.019
Short, F., Carruthers, T., Dennison, W., and Waycott, M. (2007). Global seagrass distribution and diversity: a bioregional model. J. Exp. Mar. Bio. Ecol. 350, 3–20. doi: 10.1016/j.jembe.2007.06.012
Sordo, L., Santos, R., Barrote, I., Freitas, C., and Silva, J. (2020). Seasonal photosynthesis, respiration, and calcification of a temperate maërl bed in Southern Portugal. Front. Mar. Sci. 7:136. doi: 10.3389/fmars.2020.00136
Spalding, M. D., Fox, H. E., Allen, G. R., Davidson, N., Ferdaña, Z. A., Finlayson, M., et al. (2007). Marine ecoregions of the world: a bioregionalization of coastal and shelf areas. Bioscience 57:573. doi: 10.1641/B570707
Steller, D. L., Riosmena-Rodríguez, R., Foster, M. S., and Roberts, C. A. (2003). Rhodolith bed diversity in the Gulf of California: the importance of rhodolith structure and consequences of disturbance. Aquat. Conserv. Mar. Freshw. Ecosyst. 13, 5–20. doi: 10.1002/aqc.564
Steller, D. L., Hernández-Ayon, J., Riosmena-Rodríguez, R., and Cabello-Pasini, A. (2007). Effect of temperature on photosynthesis, growth and calcification rates of the free-living coralline alga Lithophyllum margaritae. Cienc. Mar. 33, 441–456. doi: 10.7773/cm.v33i4.1255
Strain, E. M. A., Van Belzen, J., Van Dalen, J., Bouma, T. J., and Airoldi, L. (2015). Management of local stressors can improve the resilience of marine canopy algae to global stressors. PLoS One 10:e0120837. doi: 10.1371/journal.pone.0120837
Teichert, S. (2014). Hollow rhodoliths increase Svalbard’s shelf biodiversity. Sci. Rep. 4:6972. doi: 10.1038/srep06972
Thrush, S. F., and Dayton, P. K. (2002). Disturbance to marine benthic habitats by trawling and dredging: implications for marine biodiversity. Annu. Rev. Ecol. Syst. 33, 449–473. doi: 10.1146/annurev.ecolsys.33.010802.150515
Thurstan, R. H., Hawkins, J. P., and Roberts, C. M. (2014). Origins of the bottom trawling controversy in the British Isles: 19th century witness testimonies reveal evidence of early fishery declines. Fish Fish. 15, 506–522. doi: 10.1111/faf.12034
Tillin, H. M., Hiddink, J. G., Jennings, S., and Kaiser, M. J. (2006). Chronic bottom trawling alters the functional composition of benthic invertebrate communities on a sea-basin scale. Mar. Ecol. Prog. Ser. 318, 31–45. doi: 10.3354/meps318031
Twist, B. A., Neill, K. F., Bilewitch, J., Jeong, S. Y., Sutherland, J. E., and Nelson, W. A. (2019). High diversity of coralline algae in New Zealand revealed: knowledge gaps and implications for future research. PLoS One 14:e0225645. doi: 10.1371/journal.pone.0225645
Tyberghein, L., Verbruggen, H., Pauly, K., Troupin, C., Mineur, F., and De Clerck, O. (2012). Bio-ORACLE: a global environmental dataset for marine species distribution modelling. Glob. Ecol. Biogeogr. 21, 272–281. doi: 10.1111/j.1466-8238.2011.00656.x
Unsworth, R. K. F., McKenzie, L. J., Collier, C. J., Cullen-Unsworth, L. C., Duarte, C. M., Eklöf, J. S., et al. (2019). Global challenges for seagrass conservation. Ambio 48, 801–815. doi: 10.1007/s13280-018-1115-y
Valavi, R., Elith, J., Lahoz-Monfort, J. J., and Guillera-Arroita, G. (2019). Block CV: an R package for generating spatially or environmentally separated folds for k-fold cross-validation of species distribution models. Methods Ecol. Evol. 10, 225–232. doi: 10.1111/2041-210X.13107
van der Heijden, L. H., and Kamenos, N. A. (2015). Calculating the global contribution of coralline algae to carbon burial. Biogeosci. Discuss. 12, 7845–7877. doi: 10.5194/bgd-12-7845-2015
Veras, P., de, C., Pierozzi, I. Jr., Lino, J. B., Amado-Filho, G. M., Senna, A. R., et al. (2020). Drivers of biodiversity associated with rhodolith beds from euphotic and mesophotic zones: insights for management and conservation. Perspect. Ecol. Conserv. 18, 37–43. doi: 10.1016/j.pecon.2019.12.003
Villas-Bôas, A. B., de Sousa Tâmega, F. T., Andrade, M., Coutinho, R., and de Oliveira Figueiredo, M. A. (2014). Experimental effects of sediment burrial and light attenuation on two coralline algae of a deep water rhodolith bed in Rio de Janeiro, Brazil. Cryptogamie Algologie 35, 67–76. doi: 10.7872/crya.v35.iss1.2014.67
Wernberg, T., Bennett, S., Babcock, R. C., Bettignies, T., De Cure, K., Depczynski, M., et al. (2015). Climate-driven regime shift of a temperate marine ecosystem. Science 353, 169–172. doi: 10.1126/science.aad8745
Keywords: climate change, distribution shifts, coralligenous reefs, ecosystem structuring species, coralline algae, species distribution modeling, maerl
Citation: Fragkopoulou E, Serrão EA, Horta PA, Koerich G and Assis J (2021) Bottom Trawling Threatens Future Climate Refugia of Rhodoliths Globally. Front. Mar. Sci. 7:594537. doi: 10.3389/fmars.2020.594537
Received: 13 August 2020; Accepted: 28 December 2020;
Published: 20 January 2021.
Edited by:
Massimo Ponti, University of Bologna, ItalyReviewed by:
Fabio Rindi, Polytechnic University of Marche, ItalySebastian Teichert, Friedrich-Alexander Universität Erlangen-Nürnberg, Germany
Copyright © 2021 Fragkopoulou, Serrão, Horta, Koerich and Assis. This is an open-access article distributed under the terms of the Creative Commons Attribution License (CC BY). The use, distribution or reproduction in other forums is permitted, provided the original author(s) and the copyright owner(s) are credited and that the original publication in this journal is cited, in accordance with accepted academic practice. No use, distribution or reproduction is permitted which does not comply with these terms.
*Correspondence: Eliza Fragkopoulou, eli_frag@hotmail.com