- 1Conservation Biology Division, Northwest Fisheries Science Center, National Marine Fisheries Service, National Oceanic and Atmospheric Administration, Seattle, WA, United States
- 2Lynker Technologies, Leesburg, VA, United States
- 3Department of Wildlife, Fish, and Conservation Biology, University of California, Davis, Davis, CA, United States
- 4Cascadia Research Collective, Olympia, WA, United States
Accurate knowledge of behavior is necessary to effectively manage the effects of human activities on wildlife, including vessel-based whale-watching. Yet, the wholly aquatic nature of cetaceans makes understanding their basic behavioral ecology quite challenging. An endangered population of killer whales faces several identified threats including prey availability and disturbance from vessels and sound. We used bio-logging tags that were temporally attached to individuals of the endangered Southern Resident killer whale population to more fully understand their subsurface behavior and to investigate vessel effects on behavior, including foraging behavior involving prey capture. We collected tag data over three field seasons in the waters surrounding the San Juan Islands, WA, United States, corresponding to the core summer area of the critical habitat of the population. Here, we used hidden Markov models to identify latent behavioral states that include characterization of different foraging states from sound and movement variables recorded by the multi-sensor tags. We tested a number of vessel variables (e.g., vessel counts, distance, and speed) on state transition probabilities, state occurrence and time spent within each behavioral state. Whales made fewer dives involving prey capture and spent less time in these dives when vessels had an average distance less than 400 yd (366 m). Additionally, we found both a sex and vessel distance effect on the state transition probabilities, suggesting that females and males respond differently to nearby vessels. Specifically, females were more likely to transition to a non-foraging state when vessels had an average distance less than 400 yd (366 m). A female’s decision to forego foraging states due to the close proximity of vessels could have cascading effects on the ability to meet energetic requirements to support reproductive efforts. This is particularly concerning in an endangered population that is in decline. Our findings, suggesting that female killer whales are at greater risk to close approaches by vessels, highlight the importance of understanding sex-specific responses to disturbance. These findings can inform future management decisions seeking to preserve foraging opportunities and enhance recovery efforts relevant to many cetacean species, including vulnerable and endangered populations.
Introduction
Nature-based tourism, including the viewing of free-ranging and often charismatic wildlife, is a well-established industry in many parts of the world. Human activity, however, can have negative consequences on animals, including subsequent effects on the behavior of individuals, social groups and populations, which is especially concerning for vulnerable or endangered species. Knowledge of the basic aspects of animal behavior can lead to the success or failure of wildlife management programs (Knight, 2001; Coleman et al., 2013; Berger-Tal and Saltz, 2016). For example, cetaceans rely on sound for basic life functions and odontocetes (dolphins, porpoises, and toothed whales) use echolocation for navigation and foraging. Vessel-based whale-watching often introduces noise from motor-based propulsion in addition to obstacles at the surface to these air-breathing mammals (Senigaglia et al., 2016). Furthermore, vessel traffic from other activities (e.g., commercial shipping) is common in well-populated coastal corridors and many vessels emit sonar signals to aid in navigation or fishing (e.g., depth sounders and fish finders). Yet, we know little about how introduced signals might affect the use of sound and behavior in these aquatically obligate marine mammals, particular for species that rely on sound at similar frequencies for biosonar-based foraging. Given their cryptic nature, which imparts considerable challenges in quantifying anthropogenic effects, only a limited number of studies have been able to investigate behavioral effects of anthropogenic disturbance in odontocetes, particularly whether all individuals are equally affected, given contextually dependent responses to disturbance (Ellison et al., 2012). Recent technological advances have enabled the use of smaller bio-logging instruments that are temporally attached to cetaceans, allowing researchers to better understand their subsurface behavior and investigate relevant anthropogenic effects (Johnson and Tyack, 2003; Quick et al., 2016; DeRuiter et al., 2017).
Killer whales (Orcinus orca) are the largest delphinid species. North Pacific ecotypes are differentiated by genetics, foraging ecology, physical appearance, and acoustic behavior (Ford et al., 2000, 2011). The fish-eating “resident” ecotype consists of large matrilineal groups that heavily rely on echolocation for foraging (Barrett-Lennard et al., 1996; Au et al., 2004). Individuals produce slow repetition clicks while searching for prey, click faster during initial pursuit of individual prey, and produce buzzes (very rapid bout of clicks) immediately prior to prey capture attempts (Holt et al., 2019; Tennessen et al., 2019a). In the eastern North Pacific, Southern Resident killer whales are listed as Endangered in the United States and Canada (National Marine Fisheries Service [NMFS], 2016; Department of Fisheries Oceans Canada [DFO], 2017). They prefer salmonids, especially Chinook salmon (Oncorhynchus tshawytscha), but many stocks they rely on are also listed as endangered, threatened, or depleted (Ford and Ellis, 2006; Hanson et al., 2010; Ford et al., 2016). In addition, vessel traffic and associated noise from commercial shipping, whale-watching, fishing, and recreational activities, is pervasive in the core summer habitat that the whales use for feeding (Holt et al., 2009; Veirs et al., 2016; Cominelli et al., 2018). Both prey availability and disturbance from vessels and noise are identified risk factors to the Southern Resident population that has shown little recovery since ESA listing (National Marine Fisheries Service [NMFS], 2016; Center for Whale Research1, accessed 30 March 2020).
Given the recognized risk factors and documented effects, vessel regulations have restricted viewing distance of killer whales to varying degrees2. United States vessel regulations, effective May 2011, make it unlawful for vessels to approach within 200 yd (183 m) from most directions and 400 yd (366 m) of a killer whale’s path (National Oceanic Atmospheric Administration [NOAA], 2011). A state law (RCW 77.15.740), implemented in May 2019, prohibits approach within 300 yd (274 m) to the side and 400 yd in front/behind any Southern Resident killer whale, and vessel speed must be ≤ seven knots within one-half nautical mile (926 m3). An interim order, effective June 2019, prohibits all vessels from approaching any killer whale within a 400 m distance and vessel speed must be ≤ seven knots within 1 km in Canadian waters4. Empirical evaluation of the effectiveness of these unaligned regulations are needed to inform future decision making within an adaptive management framework.
Earlier studies documented effects of vessels and noise on resident killer whales, including changes in surface active and vocal behavior, diving and movement parameters, and behavioral activity states (Williams et al., 2006, 2009; Holt et al., 2009; Lusseau et al., 2009; Noren et al., 2009). A concerning finding of previous land-based observational studies was the reduction in time spent foraging in the presence of vessels (Williams et al., 2006; Lusseau et al., 2009). Indeed, a meta-analysis of several cetacean studies found disruptions of activity budget, in which individuals were less likely to rest and forage, to be one of the most consistent responses to whale-watching vessels (Senigaglia et al., 2016). Reduced foraging effort can have cascading effects on an individual’s ability to meet energetic requirements to support growth, survival and reproduction, and is especially concerning in vulnerable populations (Farmer et al., 2018; Pirotta et al., 2018). Yet, many earlier findings rely on observations of behavior at the surface that can introduce bias (Tuyttens et al., 2014). Additionally, accurately identifying different phases of biosonar-based foraging are challenging without assessment of both the acoustic and movement behavior of the whales (Holt et al., 2019; Tennessen et al., 2019a,b). In this study, we utilized multi-sensor tags to test different vessel effects on the subsurface behavior of killer whales, including acoustic behavior and foraging outcomes, to inform future management actions. Effects on behavior that we tested include vessel count, distance and speed related to current regulations, and echosounder signal presence, tested alone or in combination with one another and other effects. Specifically, we implemented hidden Markov models (HMM) to identify unobservable behavioral states from the variables obtained by the tags that were temporally attached to Southern Resident killer whales. We then examined a number of vessel effects on the state transition probabilities and on the occurrence and time spent in each behavioral state.
Materials and Methods
Study Location and Data Collection
Data collection took place in the transboundary waters surrounding the San Juan Islands, WA, United States (approximate range: 48.2° to 49.0° N, 122.7° to 123.6° W, Supplementary Figure 1) during daylight hours in September 2010, 2012, and 2014. The study location is part of the population’s critical habitat in both the United States and Canada, including their core summer habitat (National Oceanic Atmospheric Administration [NOAA], 2006). The area and season of data collection was chosen to reflect whale-watching activity by both commercial and private vessels. Twenty-three digital acoustic recording tags (Dtags) were temporarily attached to individually identified whales from photo-ID records (Center for Whale Research) using a pole from an inflatable research vessel. The Dtag is a suction cup-attached multi-sensor, bio-logging instrument containing two hydrophones, temperature, pressure and triaxial accelerometer and magnetometer sensors (Johnson and Tyack, 2003). Of the twenty-three tags deployed, we excluded data from 10 deployments in the analysis because of limited audio data quality, mainly due to high flow noise and/or suboptimal tag placement. All individuals were tagged only once, except for two individuals (K33 and L91) that were tagged twice but in separate years; thus, all deployment time series were considered independent of each other (Table 1).
We used the larger version 2 tag in 2010 and 2014 which sampled audio data at 192 kHz and non-audio data at 50 Hz, and the smaller version 3 tag in 2012 which sampled audio data at 240 kHz and non-audio data at 200 Hz. During Dtag deployments, we conducted focal follows on the (1) tagged whale, collecting geo-referenced data during surfacing and (2) all vessels, including the research vessel, within 1.5 km of the focal whale until the tag released from programming or fell off on its own (Holt et al., 2017). As conditions allowed, we recorded focal follow data using two integrated equipment packages, each consisting of a GPS/data collector, a laser range finder, and compass (Giles, 2014). One unit recorded whale data, the other recorded vessel data. Attribute data recorded for each vessel included a date and time stamp, its latitude and longitude position, vessel name and class (commercial whale-watching, private, research, enforcement, etc.) and estimated speed (sensu Holt et al., 2017). We recorded vessel data in concentric rings starting with those closest to the focal whale at least every 5 min. During focal follows, the research vessel operated at distances and speeds consistent with vessels engaged in whale-watching.
Data Processing and Calculation of Variables
We used the Dtag Toolbox5, along with custom-written routines in Matlab 2017a (Mathworks, Natick, MA, United States), to download, calibrate, and process tag data following established protocols (e.g., Holt et al., 2017; Tennessen et al., 2019a).
The unit of analysis was a dive derived from depth data down-sampled to 5 Hz. We calculated the start and end times of each dive using an automated detector that identified excursions from the surface (≤0.5 m) to depths ≥ 1 m that were checked for error and corrected accordingly. Individual reactions to tagging ranged from none to moderate, the latter which included flinching upon tag contact or diving and remaining submerged for a few minutes, but for all deployments individuals returned to pre-tagging surfacing behavior within 5 min of tagging. We therefore excluded dives during the first 5 min of each deployment time series to address any potential short-term behavioral responses to tagging (sensu Tennessen et al., 2019a,b). We used other non-audio tag data sampled at 50 Hz for analyses. For each dive, we populated six response variables described in Tennessen et al. (2019b), which were informed by previous studies (Holt et al., 2019; Tennessen et al., 2019a). These variables were (1) maximum depth, (2) jerk peak (3) median absolute roll (4) circular variance in heading (5) presence of echolocation clicks (slow/regular) (6) presence of buzzes. These variables were chosen to capture the different phases of foraging (including search and capture of prey) along with other behaviors. Only clicks and buzzes assigned to the tagged whale were included in the analysis (Holt et al., 2019). We calculated vessel variables from focal follow data as in Holt et al. (2017) using the midpoint in time of each dive to temporally align vessel (± 5 min) and dive data. If multiple observations of the same vessel occurred within the interval, we only used the observation closest in time to the dive midpoint. The horizontal distance from whale to each unique vessel was estimated from the latitude/longitude positions of the vessel relative to the whale’s latitude/longitude that was closest in time to the dive midpoint. We also scored the presence of echosounder signals, i.e., sonar signals emitted by vessels to aid in navigation and fishing, received by the tag (both transmitted and reflected signals) for each dive (Holt et al., 2017).
Statistical Analysis
We used HMMs as a multivariate framework to categorize the subsurface behavior of killer whales. This statistical approach has been widely applied in studies that investigate movement and behavior using animal-borne tags that yield time series data, including those on cetaceans (Quick et al., 2016; DeRuiter et al., 2017). HMMs identify the most likely unobservable (hidden) state from observable behavior in sequences that follow a first order Markov process. The number of latent or hidden states, N, is specified a priori and the HMM approach estimates a time series of estimated states (allowing animals to transition between states at each time step) conditioned on the observed data. HMMs are flexible and allow for the inclusion of covariates, on both the intercept parameters (“state dependent distributions”) and on the transition probability matrices. Our objective was to test vessel effects on killer whale behavior, using the six response variables described above. This approach consisted of (1) fitting several HMMs with different single covariates on the state transition probability matrix to determine the appropriate number of hidden states, (2) fitting additional models with up to two covariates on the state transition probabilities and comparing these with the original models to investigate vessel effects on killer whale likelihood of transitioning between behavioral states, and (3) investigating effects of vessel distance on state occurrence and time spent in each state.
We used the depmixS4 package (Visser and Speekenbrink, 2010) in R v.3.3.3 (R Core Team) to fit several candidate models. Here, the specified number of states and response variables were informed by and described in detail in Tennessen et al. (2019b). Briefly, we explored 3–5 state models using the six response variables with individual sex as a covariate on the state dependent distributions, and different single covariates on the transition probabilities among states (unconstrained). Depth, jerk, roll and heading variance were natural log transformed and modeled using a Gaussian distribution while clicking and buzz presence were modeled using a binomial distribution. For simplicity, we did not consider random effects potentially associated with the individual or environmental context (but see DeRuiter et al., 2017). Covariates populated for each dive included vessel counts, mean distance of all vessels within 1.5 km, distance of closest vessel, and median speed of all vessels within 1.5 km of the tagged whale (as in Holt et al., 2017), presence/absence of echosounder signals, year and individual sex. Sex was included based on previous results (Tennessen et al., 2019b) and year was included to address potential inter-annual variability (Holt et al., 2017). Vessel count, speed and distance were binary categorical variables (high/low or close/far) using different breakpoints to define levels among competing models according to sample size, distribution of covariates and implications for management (Table 2). For example, vessel distance was split into close/far categories by 200 (183 m) and 400 yd (366 m) to inform management actions related to vessel regulations. We attempted to populate received noise levels for each dive to investigate noise effects (Holt et al., 2017). However, tag-related flow noise contamination resulted in considerable missing noise data and thus, hindered the preservation of dive-by-dive time series required for the assumptions of HMMs.
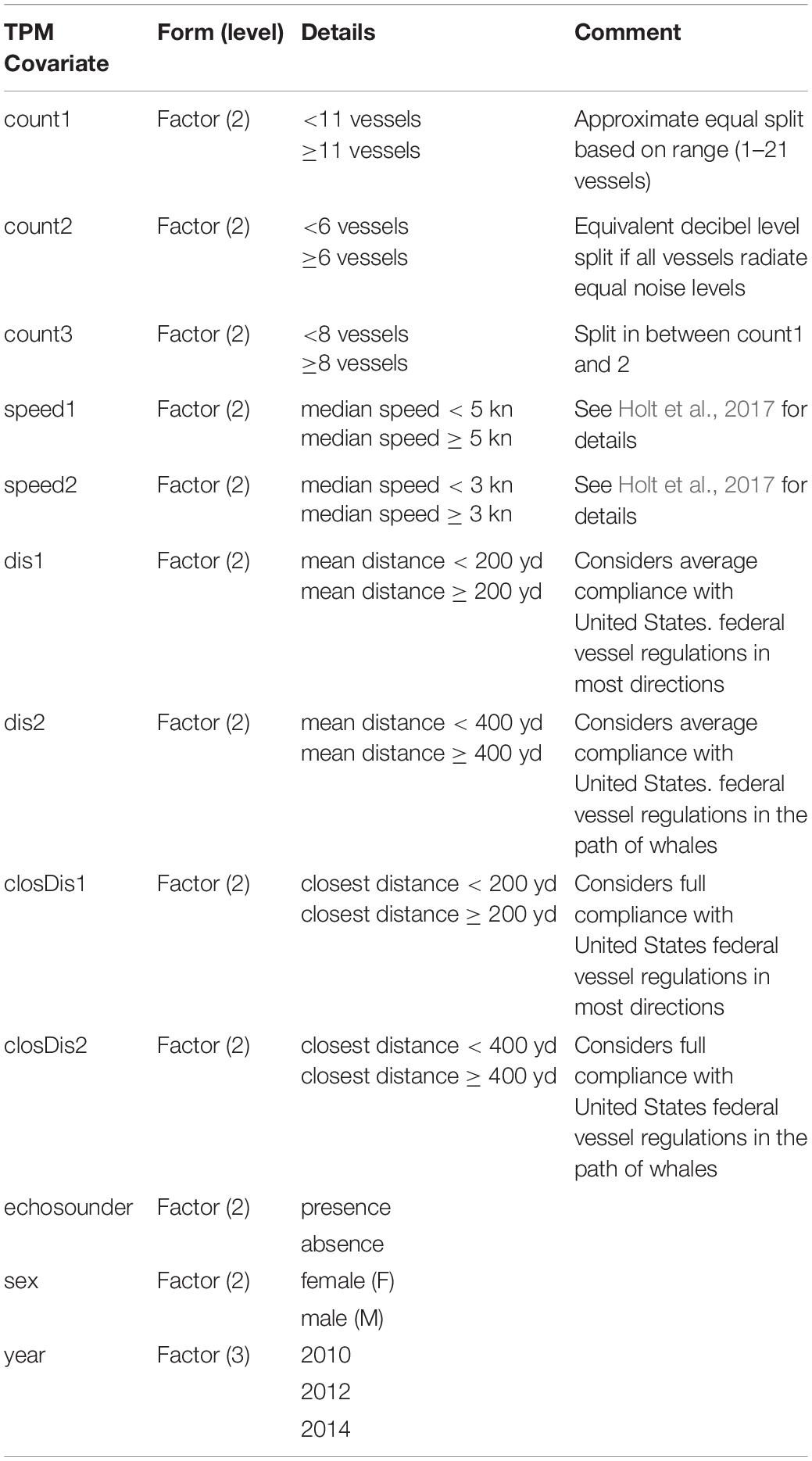
Table 2. Definitions of single covariates on the state transition probability matrix of candidate models.
Because estimation of HMMs may be sensitive to starting values, we re-fit each candidate model 200 times using random initial parameters, and we retained the one with lowest AIC score as the best across the 200 iterations (see Tennessen et al., 2019b). We first ran candidate models with 3 to 5 states, each with only one covariate on the state transition probabilities and used the lowest AIC score along with the ability to biologically interpret results as criteria for selecting the top-ranked models following Tennessen et al. (2019b). A challenge in working with HMMs is that increasing the number of states generally improves model performance metrics, such as AIC with an incurred trade-off of reduced biological interpretability of the resulting state-dependent distributions (Quick et al., 2016, 2017; DeRuiter et al., 2017; Tennessen et al., 2019b). We found the same to be true here: all 5-state models had lower AIC scores than 4-state models, which had lower AIC scores than 3-state models. However, in contrast to Tennessen et al. (2019b), 5-state models were unusually complex with the available data and the state-dependent distributions were not easily interpretable in contrast to 4-state models. Thus, we took the top-ranked 4-state models (delta AIC ≤ 30, Table 3) and then combined up to two covariates on the transition probabilities and re-ran 4-state model fitting to see if model ranking improved with combined covariates (Table 4).
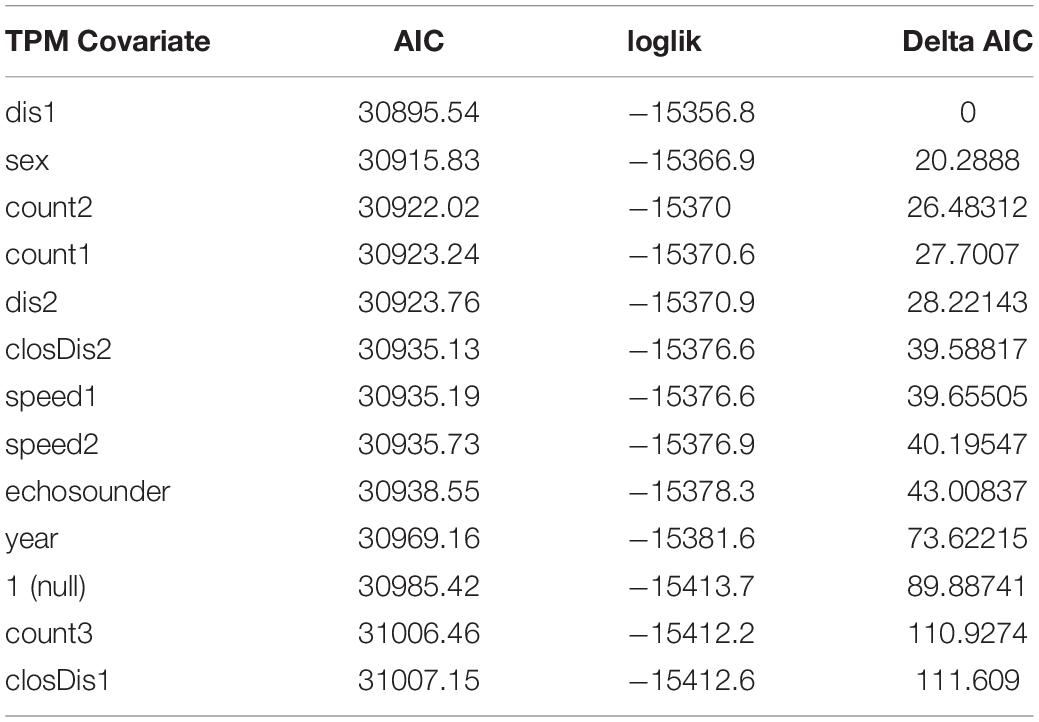
Table 3. AIC, log-likelihood and delta AIC values for 4-state models that include a single covariate on the state transition probability matrix.
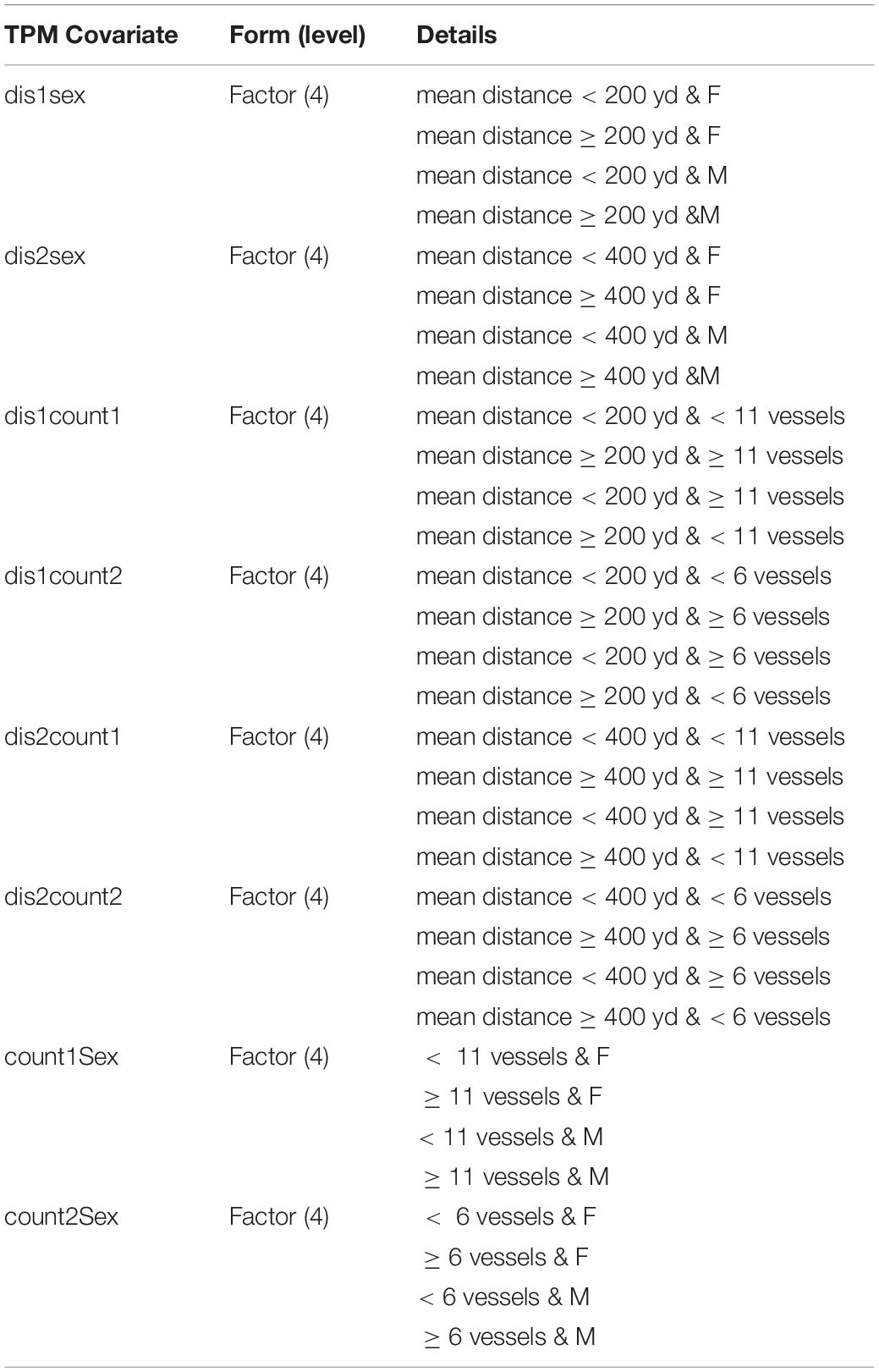
Table 4. Definitions of combined covariates on the state transition probability matrix for candidate models.
Using output from the HMMs, we used multinomial logistic regression models to ask whether vessel distance or sex affected state occurrence. We treated the estimated state assignment, based on the most likely (Viterbi) state sequence of the best model, as the response variable, and used vessel distance (2-level fixed effect) and deployment number (random effect) as predictors. All multinomial logistic regression models were fit using the brms package (Bürkner, 2017) in R. We ran these models using four MCMC (Markov chain Monte Carlo) chains, a burn-in period of 2000 samples, and retained another 1000 samples (Rhat values of all parameters were 1.0, supporting model convergence). We also explored the effect of sex on state occurrence (Tennessen et al., 2019b) by running models with and without a sex predictor and comparing posterior estimates of the coefficient using 10-fold cross-validation (k = 10) and information criterion scores (R loo package, Vehtari et al., 2019). Estimates of 10-fold expected log posterior density with and without sex were qualitatively similar (differing by < 7 units) with overlapping standard errors (33 and 31.1), suggesting no strong effect of including sex.
To investigate differences in time spent in each state, we treated dives as the sampling unit. We generated 1000 posterior samples, in which each vector of state assignments corresponded to the number of dives (n = 3609). For each posterior sample, we summarized the time spent in each state using the known duration (in minutes) of each dive and stratified this calculation by sex and vessel distance. To evaluate the effects of vessel distance, we calculated the difference between the amount of time spent in each state when vessels were close versus far for males and females separately and summarized the 95% credible intervals on the distribution of the difference.
Results
Summary of Deployments and HMM Runs
We analyzed 13 tag deployments (7 female, 6 male) that totaled 40.5 h of on-animal time (Table 1). The mean duration per deployment was 3.1 h (range = 0.5–6.2 h). From these deployments, we analyzed a total of 3609 dives (1299 dives of females, 2310 dives of males). The mean number of analyzed dives per deployment was 278 (range = 32–572). The mean number of vessels populated per dive was 4 (range 1–21) and most were commercial whale-watching or private vessels. Private vessels included those that appeared to be viewing whales, engaged in recreational fishing, or transiting the area.
Compared to the null model, model fit was significantly improved by including sex, year, vessel covariates or a combination of covariates on the transition probabilities (Tables 3, 5). The 4-state model with the lowest AIC values included the combined transition probability covariate of sex and mean vessel distance split into close and far categories by a 400 yd (366 m) threshold. The next best model included a single transition probability covariate of mean vessel distance split by a 200 yd (183 m) threshold with a delta AIC score of 6, followed by the third ranked model which included the combined transition probability covariate of sex and mean vessel distance split by a 200 yd (183 m) threshold (delta AIC = 8.5). The other models had delta AIC scores > 25 relative to the top-ranked model (Table 5).
State Classification
The state-dependent distributions of the top-ranked model (Figure 1) were as follows: (1) State 1 dives were the shallowest with smallest values of heading variance, roll, and jerk, with little clicking and no buzzing, (2) State 2 dives were deepest with the largest values of heading variance, roll, and jerk, and with clicking in almost all dives and common buzzing, (3) State 3 dives were shallow with slightly less variance in depth than State 1 and small values of heading variance, roll, and jerk, and abundant clicking in males and some clicking in females but no buzzing for either sex, and (4) State 4 dives were shallow to intermediate with small-to-moderate values of heading variance, roll, and jerk, with some clicking and virtually no buzzing.
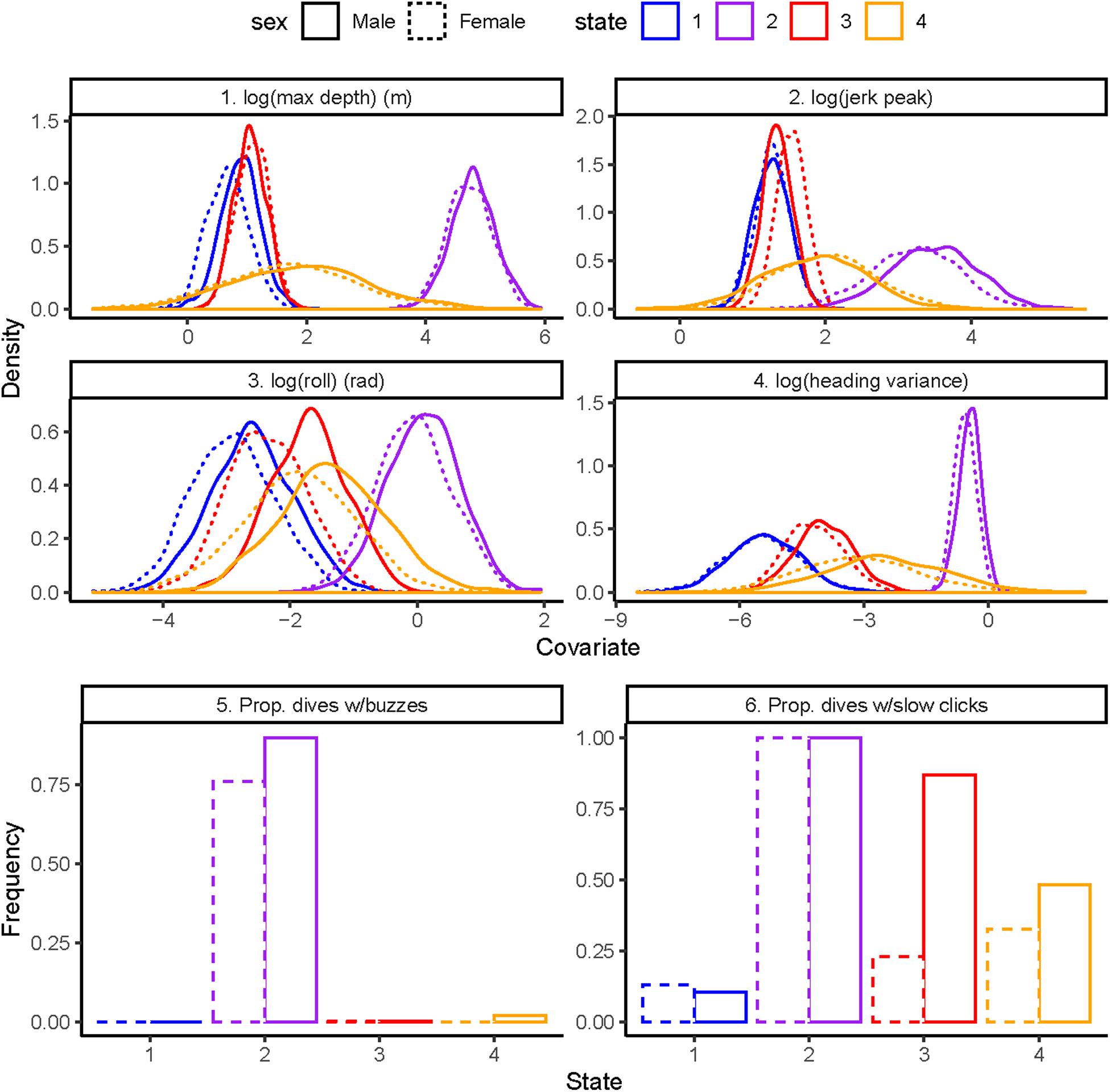
Figure 1. State-dependent distributions of the best model by sex: 1. Log maximum depth in meters (top left), 2. Log standardized jerk peak (top right), 3. Log absolute roll in radians (middle left), 4. Log heading variance (middle right), 5. Proportion of dives with buzzes (bottom left), 6. Proportion of dives with slow clicks (bottom right).
Transition Probabilities Among States
Whether the mean distance of vessels was < 400 yd (herein “close”) or ≥ 400 yd (herein “far”), persistence was especially high in state 1 and 3 and to a lesser extent in State 4 for both sexes, illustrating the behavior assigned to three of the four states was clustered in time. In contrast, it was unlikely that whales persisted in state 2 (made back-to-back state 2 dives), especially for females (Figure 2 and Supplementary Table 1). The effect of vessel distance on state transition probabilities differed between the sexes. Males were more likely to switch from state 2 to state 1 and to a lesser extent state 3 with far vessels whereas they were more likely to switch from state 2 to state 4 with close vessels. Moreover, it was extremely unlikely that males would switch from state 2 to state 3 with close vessels (Figure 2 and Supplementary Table 1). Females were more likely to switch from state 2 to state 3 or 4 as well as persist in state 4 with far vessels whereas they were more likely to switch from state 2 to state 1, and switch from state 4 to state 1 with close vessels (Figure 2 and Supplementary Table 1).
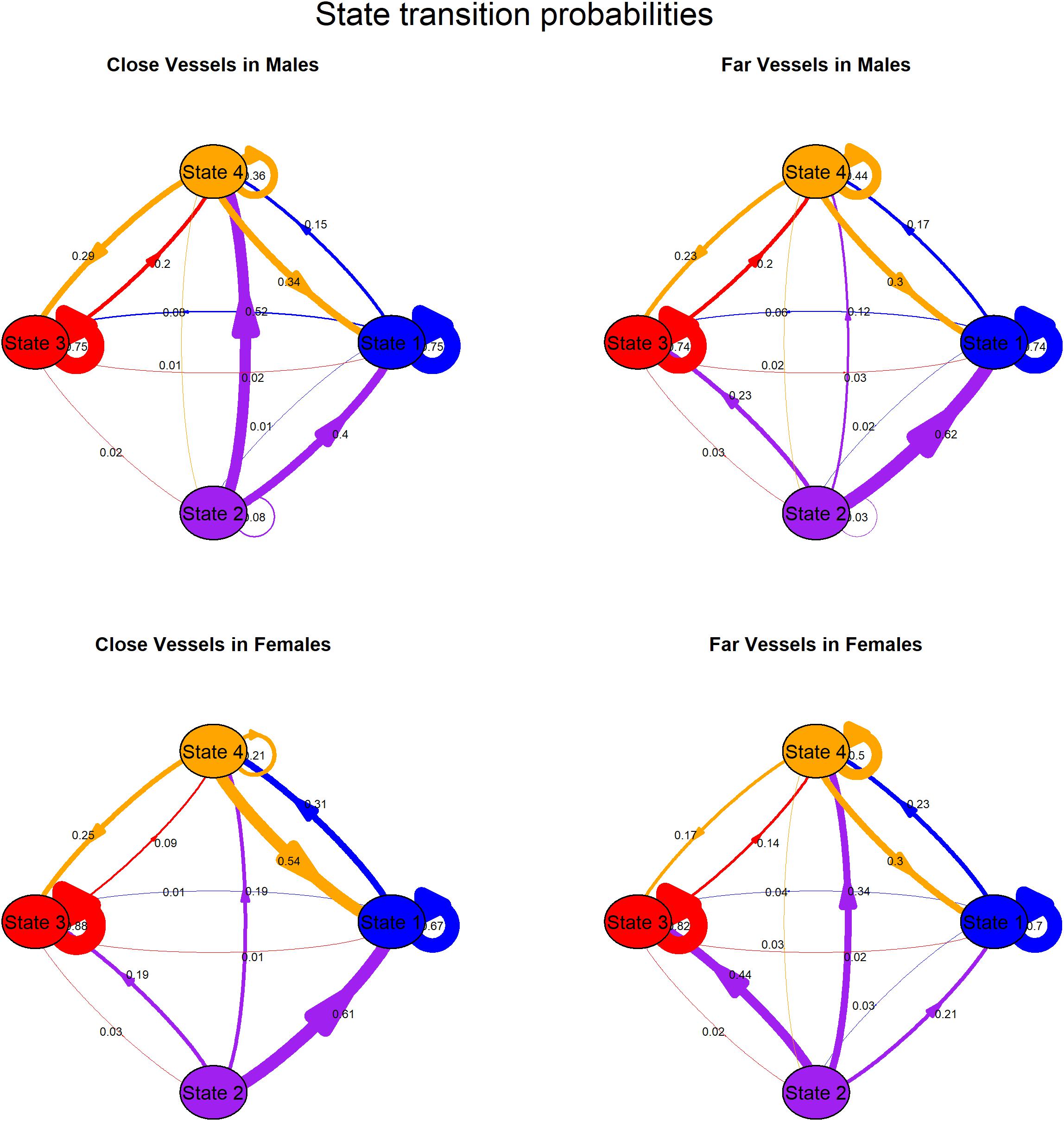
Figure 2. Transition probabilities among states for males (top plots) and females (bottom plots) by close (left plots, mean vessel distance < 400 yd) and far (right plots, mean vessel distance ≥ 400 yd) vessels. Arrows indicate direction of transitions from state of origin, arrow thickness scales with probability. See Supplementary Table 1 for details.
State Allocation by Dives
Figure 3 illustrates state allocation on a dive-by-dive basis for each tag deployment (summary across deployments provided in Supplementary Table 2). Results of the multinomial logistic regression supported differences in state occurrence (Supplementary Table 3). The negative log odds ratio (with state 1 as the reference baseline) indicated that whales were less likely to be in states 2, 3 or 4 than in state 1. Additionally, the positive log odds ratio for far vessels supported an effect of vessel distance on state allocation and was most different for state 2 (estimate = 0.74, s.e. = 0.35), followed by state 4. That is, state 2 occurrence was higher when vessels were farther away.
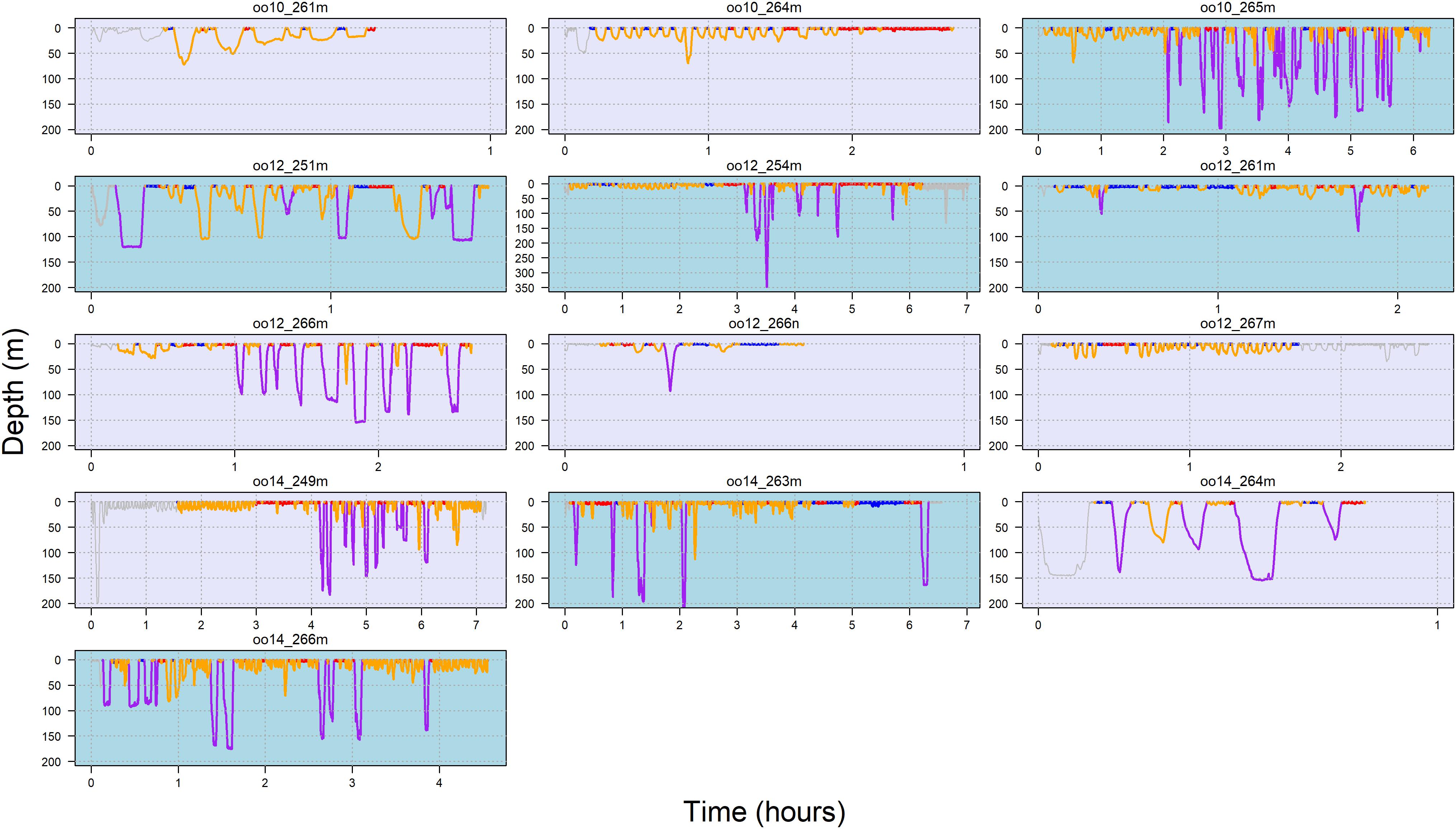
Figure 3. Dive profile by behavioral state for each deployments over time. Note the difference in both x- and y-axis scale among plots. Female = lavender box, male = light blue box, state 1 = dark blue, state 2 = purple, state 3 = red, state 4 = orange. Gray dives indicate omitted 5-min interval at start of deployment and periods which vessel data were not available.
Both females and males spent substantially more time in state 2 when vessels were far compared to when vessels were close (Figure 4, note in the case of females with close vessels, error bars are not visible because dives assigned to state 2 had very little uncertainty in assignment resulting in very small error bars). In contrast, whales spent less time in state 3 and 4 when vessels were far (Figure 4). For both sexes, the 95% credible interval of the difference in time spent for each state was different from zero in all cases except state 1 (Figure 4).
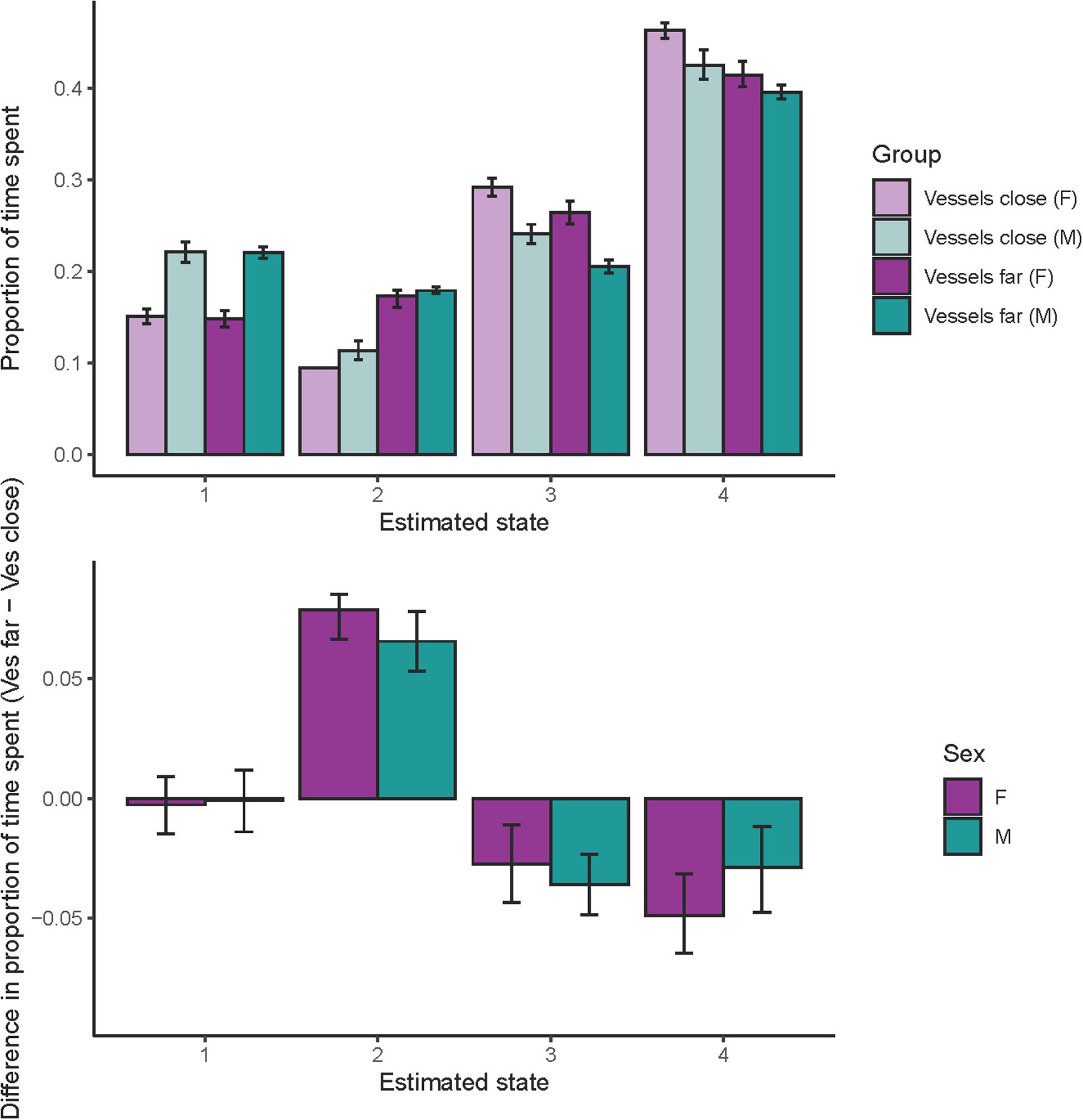
Figure 4. Proportion of time spent in each state. Top panel: proportion of time spent in each state by sex and vessel distance, light purple = females with close vessels, dark purple = females with far vessels, light teal = males with close vessels, and dark teal = males with far vessels. Bottom panel: difference in the proportion of time spent in each state with vessel distance (proportion of time with far vessels minus proportion of time with close vessels) for both sexes. Error bars in both plots indicate the 95% credible interval.
Discussion
In the current study, we used hidden Markov modeling of six observed sound and movement variables recorded from suction-cup tags attached to fish-eating killer whales, along with vessel data, to (1) characterize unobservable killer whale behavioral states and (2) identify vessel effects on foraging behavior. We found that females and males differed in their state transition probabilities depending on whether vessels were close (average vessel distance < 400 yd) or far (average vessel distance ≥ 400 yd). State frequency and cumulative time spent in these states also differed depending on vessel distance.
State 1 dives were characterized by the shallowest depth, with smallest values of heading variance, roll, and jerk, with very little clicking and no buzzing (Figure 1). State 1 involves traveling/respiratory dives given that persistence in state 1 was high for both males and females regardless of whether vessels were close or far. In contrast, state 2 was characterized by the deepest dives with the largest values of heading variance, roll, and jerk, with ubiquitous clicking and the greatest levels of buzzing. State 2 dives involve close pursuit, attempt to capture and successful capture of salmonid prey given the repeated direction changes in heading and rapid changes in acceleration (jerk) (Tennessen et al., 2019b). Indeed, the vast majority of state 2 dives mapped directly to predicted prey capture dives based on kinematic signatures, which were validated with direct observations of predation (Tennessen et al., 2019a).
Similar to state 1, state 3 was characterized by shallow depth, with small values of jerk and no buzzing. Average values of heading variance and roll were slightly greater in state 3 compared to state 1, especially in males (Figure 1), but there was considerable overlap in the two distributions. In contrast to state 1, state 3 dives had abundant clicking in males and higher values of clicking in females. High persistence in state 3 indicated that these dives reflect searching for prey whereby individuals produce echolocation click trains on repeated shallow dives to acoustically scan an area for prey targets (Holt et al., 2019; Tennessen et al., 2019b).
Lastly, state 4 was characterized by dives with intermediate values of depth, heading variance, roll, jerk, and clicking and almost no buzzing. Persistence in state 4 was moderate relative to state 1 and 3. These response variable distributions suggest that state 4 is associated with several behaviors including searching for prey at deeper depths than in state 3 and initiating pursuit of prey that, given the absence of buzzes, does not involve close pursuit/capture attempts or prey capture (Holt et al., 2019). State 4 dives might also involve socializing and/or prey-sharing among group members (Ford and Ellis, 2006; Wright et al., 2016).
States 1 (traveling/respiratory) and 3 (acoustic search) and, to a lesser extent, state 4 (intermediate dives) were characterized by state persistence, demonstrating that most behaviors occurred in bouts as in other cetacean studies (Figures 2, 3; DeRuiter et al., 2017; Quick et al., 2017; Tennessen et al., 2019b). In contrast, persistence in state 2 (deep forage) was rare in males and virtually absent in females, likely because lengthy prey chases at depth can incur significant energetic costs that require recovery periods. Furthermore, state 2 dives resulting in prey capture are often followed by prey-handling and sharing events (Holt et al., 2019; Tennessen et al., 2019b).
In the current study, 5-state models were unusually complex with the available data and the state-dependent distributions were not biologically interpretable. In contrast, 4-state models adequately characterized the behavior of fish-eating killer whales, including different phases of foraging that we expected (Holt et al., 2019). Three of the four states (Figure 1) were similar to three of the five states reported by Tennessen et al. (2019b). The three similar states from the present study were the deep forage, acoustic search, and intermediate states; the difference was that state 1 of the current study is likely a combination of travel and respiratory dives that are differentiated into two separate states in the previous study (Tennessen et al., 2019b). Given the different focus of the current study, namely to test effects of vessel covariates on foraging behavior, these 4-state models captured the most important structure in the data that was biologically informative to address our scientific objectives.
Vessel distance and sex significantly affected state transition probabilities (Figure 2 and Supplementary Table 1), with close vessels reducing the likelihood of foraging-related behaviors. In particular, females were more likely to switch from state 2 (deep forage) to state 3 (acoustic search) or state 4 (intermediate dives) as well as persist in state 4 with far vessels whereas they were more likely to switch from state 2 (deep forage) to state 1 (travel/respiratory), and switch from state 4 (intermediate dives) to state 1 (travel/respiratory) with close vessels. Furthermore, males were more likely to switch from state 2 (deep forage) to state 1 (travel/respiratory) and to a lesser extent, to state 3 (acoustic search) with far vessels whereas they were more likely to switch from state 2 (deep forage) to state 4 (intermediate dives, but recall that this state includes searching and prey pursuit, but not prey capture) with close vessels. Male response to close vessels could reflect vertical avoidance of vessels (and/or noise) while acoustically searching for prey and failure to track prey after initial pursuit.
Lusseau et al. (2009) used land-based surface observations of behavior to demonstrate that Southern Resident killer whales were more likely to switch from foraging to traveling in the presence of vessels. The aim of the current investigation was to use HMM to sufficiently characterize the subsurface behavior of fish-eating killer whales, including different phases of biosonar-based foraging, that are otherwise difficult to discriminate from surface observations, in order to test a variety of potential vessel effects on behavior. Our analysis, based on animal-borne tag data, characterized more foraging activity than had been reported in some previous studies based on surface-based observations (e.g., Noren and Hauser, 2016). Consistent with earlier studies, but unveiling a sex effect, we found that females switched to a state (state 1, traveling/respiratory) distinctly different from foraging when vessels were close, compared to when vessels were far. Our findings of a sex difference imply that females may experience risk to vessels differently than males, which might be related to group structure. Females are more likely to be associated with younger animals, including juveniles and dependent offspring. Furthermore, the energetic demand of deep diving to pursue prey is likely higher for females given their smaller body size compared to males (Schreer and Kovacs, 1997; Noren and Williams, 2000; Baird et al., 2005). Females of the Northern Resident killer whale population prefer to forage closer to shore compared to males that show no preference, perhaps because of their own physiological limitations or associations with younger individuals with limited dive capacity (Beerman et al., 2016). These findings suggest that females may simply have less three dimensional space to maneuver during prey chases. Thus, females may forego foraging altogether in the presence of close vessels as vessels might pose a higher risk to the group, which may hinder aspects of deep foraging, and/or cooperation, including prey sharing. Williams et al. (2002) found subtle sex-based differences in vessel avoidance in Northern Resident killer whales. Additionally, male and female bottlenose dolphins also respond differently in the presence of vessels (Lusseau, 2003; Symons et al., 2014).
We found that vessel distance affected state occurrence and time spent in each state (Figure 4). Overall, whales were less likely to be in states 2–4 (foraging states) than in state 1 (traveling/respiratory, a non-foraging state), and importantly, state 2–4 had a higher occurrence when vessels were far, with the biggest effect for state 2 (deep forage). Furthermore, when we considered time spent in each state, we found that both females and males spent less time in state 3 (acoustic search) and 4 (intermediate dives), and substantially more time in state 2 (deep forage, including prey capture) when vessels were far compared to when vessels were close (Figure 4). These findings suggest that deep foraging opportunities can be enhanced when vessels give whales, especially females, more space.
It is important to note that this is an observational study in which vessels that were engaged in whale-watching were subject to various vessel regulations over the course of the study and compliance varied (Eisenhardt and Koski, 2014; Shedd et al., 2018). Additionally, tag attachment sometimes failed before programmed release time. Thus, sample sizes were difficult to balance among covariates, including longer deployments in females. However, in a related study we found no statistical support for including deployment duration as a predictor or offset variable for modeling behavioral states (Tennessen et al., 2019b). Another limitation is that, relative to other dives, dives involving deep foraging and prey capture (state 2 dives reported here) are rare, and even rarer in females (Tennessen et al., 2019a,b), resulting in small sample sizes available for analysis and interpretation. It is also possible that vessel noise mediated the observed effect of vessel distance (via an avoidance response of the noise source). However, because water flow over the tag attached to a moving whale prevented us from calculating uncontaminated noise levels for all dives in a deployment time series, we could not specifically test for this. Lastly, data collection was limited to daylight hours to collect concurrent whale and vessel data, given the study’s focus. Thus, time spent in different activities is not fully characterized over a 24-h cycle. Future work to investigate full activity budgets of killer whales with both day and nighttime tag data collection would be valuable to understand if whales made up lost foraging time during different periods of the diel cycle.
The focus of the current investigation was to use data from animal-borne tags to test several vessel effects on foraging-related behavior in an endangered population. We found effects of vessel distance on the state transition probabilities, state occurrence, and time spent among states. Specifically, whales made fewer prey capture dives and spent less time in these dives when vessels had an average distance less than 400 yd. Reduction in foraging activity with vessels is consistent with findings of other cetacean studies (Senigaglia et al., 2016), including Southern Resident killer whales (Lusseau et al., 2009), and has substantial management implications, especially for a population with prey availability and vessel disturbance as risk factors. Furthermore, we found both a sex and vessel distance effect on the state transition probabilities, suggesting that females and males respond differently to nearby vessels. Specifically, females were more likely to transition to a non-foraging (travel/respiratory) state when vessels had an average distance less than 400 yd. A female’s decision to forego foraging in the presence of close vessels could hinder her ability to meet energetic requirements to support reproductive efforts, including fetal growth in pregnancy and lactation costs after calving. This is particularly concerning in an endangered mammalian population because recovery cannot occur without successful reproductive outcomes among breeding individuals, particularly in long-lived females with birthing intervals of 3–7 years (Olesiuk et al., 2005). Our findings, suggesting that female killer whales are at greater risk from close vessel approaches than males, can inform future management decisions seeking to preserve foraging opportunities and enhance recovery efforts in endangered populations.
Data Availability Statement
The datasets presented in this study can be found in online repositories. The names of the repository/repositories and accession number(s) can be found below: The NWFSC Public Access to Research Results (PARR)-Data Inventory, www.webapps.nwfsc.noaa.gov/apex/parrdata/inventory/datasets.
Ethics Statement
The animal study was reviewed and approved by the Northwest Fisheries Science Center’s Institutional Animal Care and Use Committee and conducted under research permits (in the United States, NMFS No. 781–1824/16163 and in Canada, DFO SARA/Marine Mammal License No. MML 2010-01/SARA-106B).
Author Contributions
MHo and MHa conceived the research idea, obtained permits, and secured funding. MHo, MHa, CE, DG, and JH collected the data. MHo, JT, and EW analyzed the data. MHo wrote the manuscript. All authors edited the manuscript.
Funding
This work was supported by the NOAA Ocean Acoustics Program and Northwest Fisheries Science Center provided funding for field data collection efforts. The National Fish and Wildlife Foundation (No. 50190) provided funding for analysis.
Conflict of Interest
JT was employed by the company Lynker Technologies.
The remaining authors declare that the research was conducted in the absence of any commercial or financial relationships that could be construed as a potential conflict of interest.
Acknowledgments
We thank Juliana Houghton, Jeff Foster, Robin Baird, Robert Hunt, and many others for field assistance. David Haas, Alessandro Bocconcelli, Tom Hurst, Stacy DeRuiter, and many others provided valuable support and feedback on Dtag logistics and analysis. We also thank Damon Holzer for the preparation of Supplementary Figure 1, Dawn Noren, Jameal Samhouri, Mike Ford, and three reviewers for providing constructive feedback on earlier manuscript versions.
Supplementary Material
The Supplementary Material for this article can be found online at: https://www.frontiersin.org/articles/10.3389/fmars.2020.582182/full#supplementary-material
Footnotes
- ^ https://www.whaleresearch.com/orca-population
- ^ www.bewhalewise.org
- ^ https://app.leg.wa.gov
- ^ www.pac.dfo-mpo.gc.ca/whales-baleines/srkw-measures-mesures-ers-eng.html
- ^ www.soundtags.org
References
Au, W. W. L., Ford, J. K. B., Horne, J. K., and Newman Allman, K. A. (2004). Echolocation signals of free-ranging killer whales (Orcinus orca) and modeling of foraging for Chinook salmon (Oncorhynchus tshawytscha). J. Acoust. Soc. Am. 115, 901–909. doi: 10.1121/1.1642628
Baird, R. W., Hanson, M. B., and Dill, L. M. (2005). Factors influencing the diving behavior of fish-eating killer whales: sex difference and diel and interannual variation in diving rates. Can. J. Zool. 83, 257–267. doi: 10.1139/z05-007
Barrett-Lennard, L. G., Ford, J. K. B., and Heise, K. A. (1996). The mixed blessings of echolocation: differences in sonar use by fish-eating and mammal-eating killer whales. Anim. Behav. 51, 553–565. doi: 10.1006/anbe.1996.0059
Beerman, A., Ashe, E., Preedy, K., and Williams, R. (2016). Sexual segregation when foraging in an extremely social killer whale population. Behav. Ecol. Sociobiol. 70, 189–198. doi: 10.1007/s00265-015-2038-2
Berger-Tal, O., and Saltz, D. (2016). Conservation Behavior: Applying Behavioral Ecology to Wildlife Conservation and Management. Cambridge: Cambridge University Press.
Bürkner, P. (2017). brms: an r package for bayesian multilevel models using stan. J. Stat. Software 80, 1–28. doi: 10.18637/jss.v080.i01
Coleman, T. H., Schwartz, C. C., Gunther, K. A., and Creel, S. (2013). Grizzly bear and human interaction in Yellowstone National Park. J. Wildl. Manage. 77, 1311–1320. doi: 10.1002/jwmg.602
Cominelli, S., Devillers, R., Yurk, H., MacGillivray, A., McWhinnie, L., and Canessa, R. (2018). Noise exposure from commercial shipping for the southern resident killer whale population. Mar. Poll. Bull. 136, 177–200. doi: 10.1016/j.marpolbul.2018.08.050
Department of Fisheries Oceans Canada [DFO] (2017). Action Plan for the Northern and Southern Resident Killer Whale (Orcinus orca) in Canada. Available online at: https://www.registrelep-sararegistry.gc.ca/virtual_sara/files/plans/Ap-ResidentKillerWhale-v00-2017Mar-Eng.pdf (accessed 26 May, 2020)
DeRuiter, S. L., Langrock, R., Skirbuta, T., Goldbogen, J. A., Calambokidis, J., Friedlaender, A. S., et al. (2017). A multivariate mixed hidden Markov model for blue whale behaviour and responses to sound exposure. Ann. Appl. Stat. 11, 362–392. doi: 10.1214/16-aoas1008
Eisenhardt, E. P., and Koski, K. (2014). Final 2014 Soundwatch Program Annual Contract Report. Friday Harbor, WA: The Whale Museum.
Ellison, W. T., Southall, B. L., Clark, C. W., and Frankel, A. S. (2012). A new context-based approach to assess marine mammal behavioral responses to anthropogenic sounds. Conserv. Biol. 26, 21–28. doi: 10.1111/j.1523-1739.2011.01803.x
Farmer, N. A., Baker, K., Zeddies, D. G., Denes, S. L., Noren, D. P., Garrison, L. P., et al. (2018). Population consequences of disturbance by offshore oil and gas activity for endangered sperm whales (Physeter macrocephalus). Biol. Conserv. 227, 189–204. doi: 10.1016/j.biocon.2018.09.006
Ford, J. K. B., and Ellis, G. M. (2006). Selective foraging by fish-eating killer whales Orcinus orca in British Columbia. Mar. Ecol. Prog. Ser. 316, 185–199. doi: 10.3354/meps316185
Ford, J. K. B., Ellis, G. M., and Balcomb, K. C. (2000). Killer Whales, 2nd Edn. Vancouver, BC: UBC Press.
Ford, J. K. B., Elllis, G. M., Matkin, C. O., Wetklo, M. H., Barrett-Lennard, L. G., and Withler, R. E. (2011). Shark predation and tooth wear in a population of northeastern Pacific killer whales. Aquat. Biol. 11, 213–224. doi: 10.3354/ab00307
Ford, M. J., Hempelmann, J., Hanson, M. B., Ayres, K. L., Baird, R. W., Emmons, C. K., et al. (2016). Estimation of a killer whale (Orcinus orca) population’s diet using sequencing analysis of DNA from feces. PLoS One 11:e0144956. doi: 10.1371/journal.pone.0144956
Giles, D. A. (2014). Southern Resident Killer Whales (Orcinus orca): a Novel non-invasive Method to Study Southern Resident Killer Whales And Vessel Compliance with Regulations. PhD dissertation thesis, Davis, CA: University of California.
Hanson, M. B., Baird, R. W., Ford, J. K. B., Hempelmann-Halos, J., Van Doornik, D. M., Candy, J. R., et al. (2010). Species and stock identification of prey consumed by endangered Southern Resident killer whales in their summer range. Endang. Species Res. 11, 69–82. doi: 10.3354/esr00263
Holt, M. M., Hanson, M. B., Emmons, C. K., Haas, D. K., Giles, D. A., and Hogan, J. T. (2019). Sounds associated with foraging and prey capture in individual fish-eating killer whales. Orcinus orca. J. Acoust. Soc. Am. 146, 3475–3486. doi: 10.1121/1.5133388
Holt, M. M., Hanson, M. B., Giles, D. A., Emmons, C. K., and Hogan, J. T. (2017). Noise levels received by endangered killer whales (Orcinus orca) before and after the implementation of vessel regulations. Endang. Species Res. 34, 15–26. doi: 10.3354/esr00841
Holt, M. M., Noren, D. P., Veirs, V., Emmons, C. K., and Veirs, S. (2009). Speaking up: killer whales (Orcinus orca) increase their call amplitude in response to vessel noise. J. Acoust. Soc. Am. 125, EL27–EL32.
Johnson, M. P., and Tyack, P. L. (2003). A digital acoustic recording tag for measuring the response of wild marine mammals to sound. IEEE J. Oceanic Eng. 28, 3–12. doi: 10.1109/joe.2002.808212
Lusseau, D. (2003). Male and female bottlenose dolphins Tursiops spp. have different strategies to avoid interactions with tour boats in Doubtful Sound. New Zealand. Mar. Ecol. Prog. Ser. 257, 267–274. doi: 10.3354/meps257267
Lusseau, D., Bain, D. E., Williams, R., and Smith, J. C. (2009). Vessel traffic disrupts the foraging behavior of southern resident killer whales Orcinus orca. Endang. Species Res. 6, 211–221. doi: 10.3354/esr00154
National Marine Fisheries Service [NMFS] (2016). Southern Resident Killer Whales (Orcinus orca) 5-Year Review: Summary and Evaluation. Seattle, WA: National Marine Fisheries Service West Coast Region.
National Oceanic Atmospheric Administration [NOAA] (2006). Designation of critical habitat for southern resident killer whale. Federal Registrar. 71, 69054–69070.
National Oceanic Atmospheric Administration [NOAA] (2011). Protective regulations for killer whales in the northwest region under the endangered species act and marine mammal protection act. Federal Registrar. 76, 20870–20890.
Noren, D. P., and Hauser, D. D. W. (2016). Surface-based observations can be used to assess behavior and fine-scale habitat use by an endangered killer whale (Orcinus orca) population. Aquat. Mamm. 42, 168–183. doi: 10.1578/am.42.2.2016.168
Noren, D. P., Johnson, A. H., Rehder, D., and Larson, A. (2009). Close approaches by vessels elicit surface active behaviors by southern resident killer whales. Endang. Species Res. 8, 179–192. doi: 10.3354/esr00205
Noren, S. R., and Williams, T. M. (2000). Body size and skeletal muscle myoglobin of cetaceans: adaptations for maximizing dive duration. Comp. Biochem. Physiol. A 126, 181–191. doi: 10.1016/s1095-6433(00)00182-3
Olesiuk, P. F., Ellis, G. M., and Ford, J. K. B. (2005). Life History and Population Dynamics of Northern Resident Killer Whales (Orcinus orca) in British Columbia, Canadian Science Advisory Secretariat. Available online at: https://www.dfo-mpo.gc.ca/csas-sccs/publications/resdocs-docrech/2005/2005_045-eng.htm (accessed 26 May, 2020)
Pirotta, E., Booth, C. G., Costa, D. P., Fleishman, E., Kraus, S. D., Lusseau, D., et al. (2018). Understanding the population consequences of disturbance. Ecol. Evol. 8, 9934–9946.
Quick, N., Isojunno, S., Sadykova, D., Bowers, M., Nowacek, D. P., and Read, A. J. (2017). Hidden Markov models reveal complexity in the diving behavior of short-finned pilot whales. Sci. Rep. 7:45765. doi: 10.1038/srep45765
Quick, N., Scott-Hayward, L., Sadykova, D., Nowacek, D., and Read, A. (2016). Effects of a scientific echo sounder on the behavior of short-finned pilot whales (Globicephala macrorhynchus). Can. J. Fish. Aquat. Sci. 74, 716–726. doi: 10.1139/cjfas-2016-0293
Schreer, J. F., and Kovacs, K. M. (1997). Allometry of diving capacity in air-breathing vertebrates. Can. J. Zool. 75, 339–358. doi: 10.1139/z97-044
Senigaglia, V., Christiansen, F., Bejder, L., Gendron, D., Lundquist, D., and Noren, D. P. (2016). Meta-analyses of whale-watching impact studies: comparisons of cetacean responses to disturbance. Mar. Ecol. Prog. Ser. 542, 251–263. doi: 10.3354/meps11497
Shedd, T., Seely, E., and Osborne, R. (2018). 2018 Soundwatch Program Annual Contract Report. Friday Harbor, WA: The Whale Museum.
Symons, J., Pirotta, E., and Lusseau, D. (2014). Sex differences in risk perception in deep-diving bottlenose dolphins leads to decreased foraging efficiency when exposed to human disturbance. J. Applied Ecol. 51, 1584–1592. doi: 10.1111/1365-2664.12337
Tennessen, J. B., Holt, M. M., Hanson, M. B., Emmons, C. K., Giles, D. A., and Hogan, J. T. (2019a). Kinematic signatures of prey capture from archival tags reveal sex differences in killer whale foraging activity. J. Exp. Biol. 222:jeb191874. doi: 10.1242/jeb.191874
Tennessen, J. B., Holt, M. M., Ward, E. J., Hanson, M. B., Emmons, C. K., Giles, D. A., et al. (2019b). Hidden Markov models reveal temporal patterns and sex differences in killer whale diving behavior. Sci. Rep. 9:14951.
Tuyttens, F. A. M., de Graaf, S., Heerkens, J. L. T., Jacobs, L., Nalon, E., Ott, S., et al. (2014). Observer bias in animal behaviour research: can we believe what we score, if we score what we believe? Anim. Behav. 90, 273–280. doi: 10.1016/j.anbehav.2014.02.007
Vehtari, A., Gabry, J., Magnusson, M., Yao, Y., and Gelman, A. (2019). loo: Efficient Leave-one-out Cross-validation and WAIC for Bayesian models. R package version 2.2.0, URL: https://mc-stan.org/loo.
Veirs, S., Veirs, V., and Wood, J. D. (2016). Ship noise extends to frequencies used for echolocation by endangered killer whales. Peer J 4:e1657. doi: 10.7717/peerj.1657
Visser, I., and Speekenbrink, M. (2010). depmixS4: an R package for hidden Markov models. J. Stat. Softw. 36, 1–21.
Williams, R., Bain, D. E., Smith, J. C., and Lusseau, D. (2009). Effects of vessels on behavior patterns of individual southern resident killer whales Orcinus orca. Endang. Species Res. 6, 199–209. doi: 10.3354/esr00150
Williams, R., Lusseau, D., and Hammond, P. S. (2006). Estimating relative energetic costs of human disturbance to killer whales (Orcinus orca). Biol. Conserv. 133, 301–311. doi: 10.1016/j.biocon.2006.06.010
Williams, R., Trites, A. W., and Bain, D. E. (2002). Behavioural responses of killer whales (Orcinus orca) to whale-watching boats: opportunistic observations and experimental approaches. J. Zool. Lond. 256, 255–270. doi: 10.1017/s0952836902000298
Keywords: Killer whale (Orcinus orca), behavior, foraging, vessel effects, whale-watching, hidden Markov model
Citation: Holt MM, Tennessen JB, Ward EJ, Hanson MB, Emmons CK, Giles DA and Hogan JT (2021) Effects of Vessel Distance and Sex on the Behavior of Endangered Killer Whales. Front. Mar. Sci. 7:582182. doi: 10.3389/fmars.2020.582182
Received: 10 July 2020; Accepted: 16 December 2020;
Published: 12 January 2021.
Edited by:
Maritza Sepulveda, Universidad de Valparaiso, ChileReviewed by:
Daniel Paul Costa, University of California, Santa Cruz, United StatesPaola Tepsich, CIMA Research Foundation, Italy
Jason Donald Wood, SMRU Consulting, United States
Copyright © 2021 Holt, Tennessen, Ward, Hanson, Emmons, Giles and Hogan. This is an open-access article distributed under the terms of the Creative Commons Attribution License (CC BY). The use, distribution or reproduction in other forums is permitted, provided the original author(s) and the copyright owner(s) are credited and that the original publication in this journal is cited, in accordance with accepted academic practice. No use, distribution or reproduction is permitted which does not comply with these terms.
*Correspondence: Marla M. Holt, bWFybGEuaG9sdEBub2FhLmdvdg==
†Present address: Deborah A. Giles, Center for Conservation Biology, University of Washington, Seattle, WA, United States