- 1Department of Ecology, Leibniz Centre for Tropical Marine Research (ZMT), Bremen, Germany
- 2Department of Marine Ecology, Faculty of Biology and Chemistry (FB2), University of Bremen, Bremen, Germany
- 3Stockholm Resilience Centre, Stockholm University, Stockholm, Sweden
- 4School of Marine Studies, Faculty of Science, Technology and Environment, The University of the South Pacific, Suva, Fiji
- 5Global Economic Dynamics and the Biosphere Academy Programme, Royal Swedish Academy of Sciences, Stockholm, Sweden
- 6Pacific Community (SPC), Noumea, New Caledonia
- 7Institute for Marine and Antarctic Studies, University of Tasmania, Hobart, TAS, Australia
- 8National Institute of Water and Atmospheric Research, Nelson, New Zealand
- 9EPHE, PSL Research University, UPVD-CNRS, USR3278 CRIOBE, Paris, France
- 10Labex Corail, CRIOBE, Moorea, French Polynesia
- 11School of Ocean Sciences, Bangor University, Anglesey, United Kingdom
- 12Reef Systems Research Group, Leibniz Centre for Tropical Marine Research (ZMT), Bremen, Germany
Human activities are changing ecosystems at an unprecedented rate, yet large-scale studies into how local human impacts alter natural systems and interact with other aspects of global change are still lacking. Here we provide empirical evidence that local human impacts fundamentally alter relationships between ecological communities and environmental drivers. Using tropical coral reefs as a study system, we investigated the influence of contrasting levels of local human impact using a spatially extensive dataset spanning 62 outer reefs around inhabited Pacific islands. We tested how local human impacts (low versus high determined using a threshold of 25 people km−2 reef) affected benthic community (i) structure, and (ii) relationships with environmental predictors using pre-defined models and model selection tools. Data on reef depth, benthic assemblages, and herbivorous fish communities were collected from field surveys. Additional data on thermal stress, storm exposure, and market gravity (a function of human population size and reef accessibility) were extracted from public repositories. Findings revealed that reefs subject to high local human impact were characterised by relatively more turf algae (>10% higher mean absolute coverage) and lower live coral cover (9% less mean absolute coverage) than reefs subject to low local human impact, but had similar macroalgal cover and coral morphological composition. Models based on spatio-physical predictors were significantly more accurate in explaining the variation of benthic assemblages at sites with low (mean adjusted-R2 = 0.35) rather than high local human impact, where relationships became much weaker (mean adjusted-R2 = 0.10). Model selection procedures also identified a distinct shift in the relative importance of different herbivorous fish functional groups in explaining benthic communities depending on the local human impact level. These results demonstrate that local human impacts alter natural systems and indicate that projecting climate change impacts may be particularly challenging at reefs close to higher human populations, where dependency and pressure on ecosystem services are highest.
Introduction
Humans have become a dominant force of planetary change (Steffen et al., 2007). Ecosystems worldwide are being fundamentally altered by climate change impacts against a diverse backdrop of local anthropogenic stressors. Our ability to reliably predict the future configuration of affected ecosystems requires a thorough understanding of interactions between these different stressor types (Williams et al., 2019). Increasing evidence indicates that ecosystems are being reorganised or homogenised into stress-tolerant or opportunistic communities, leading to novel systems that differ from their previous state in terms of their dominant constituents (Graham et al., 2014; Morse et al., 2014). Quantifying ecological reorganisation over broad scales remains challenging due to coarse taxonomic resolution inherent to large datasets that are necessary to address this topic. Nonetheless, this phenomenon may significantly alter a system’s relationship with various environmental and climate change-related drivers (Côté and Darling, 2010; Williams G.J. et al., 2015). Understanding what role local human impacts play in driving ecological reorganisation and changing a system’s relationship with its biophysical environment is thus pivotal to improving predictive models and informing local management (Robinson et al., 2018; Jouffray et al., 2019; Henderson et al., 2020).
Inherent high biodiversity and the presence of multiple stressors acting at local and global scales make tropical coral reefs a unique system to examine how local human impacts drive ecological states (Hoegh-Guldberg et al., 2007; Ban et al., 2014; Norström et al., 2016; Darling et al., 2019). Locally, rapidly expanding coastal development, sewage input, and agricultural practices are reducing water quality, whilst the modernisation of fishing gear and expedited market access are facilitating overexploitation of coastal fisheries resources (Fabricius et al., 2005; Brewer et al., 2012; Hamilton et al., 2012). Globally, the intensity and frequency of thermal anomalies, unusual weather patterns, and destructive storm events are increasing (Gattuso et al., 2015; van Hooidonk et al., 2016; IPCC, 2019), and recovery windows between stress events are narrowing (Riegl et al., 2013; Hughes et al., 2018). The productivity and provision of ecosystem services (e.g., Woodhead et al., 2019) of coral reef benthic communities differ depending on their composition (Ferrario et al., 2014; Rogers et al., 2018), underlining the importance of quantifying how communities are affected by global change. The widespread negative effects of climate change-related stressors are relatively well-understood. However, despite more than half the world’s coral reefs being located within 30 min travel time from human populations (Maire et al., 2016), the role of local stressors remains somewhat contested. These local stressors can range greatly in their intensity depending on inherent factors such as human population density and the level of exploitation of, or reliance on, marine resources.
Higher human population densities have been linked to reductions in reef fish biomass and coral cover, and to increases in fleshy (turf and macro-) algal cover (Sandin et al., 2008; Williams I.D. et al., 2015; Heenan et al., 2016; Smith et al., 2016). However, coarse taxonomic resolution (inherent to large-scale datasets) can lead to the conclusion that there is no link between local stressors and reef condition (e.g., Bruno and Valdivia, 2016). This may be a particular issue in regions such as the tropical Pacific where species diversity is exceptionally high and fleshy macroalgae, frequently stated as principal alternative organisms on degraded reefs, do not play such a dominant role in benthic dynamics compared, for instance, to the Caribbean region (Roff and Mumby, 2012). Furthermore, reefs are increasingly existing in a partially degraded condition between distinct regimes (Mumby, 2017), likely having undergone ecological homogenisation resulting from the non-random removal of species with particular traits in response to environmental factors (McWilliam et al., 2020). In this case, coral cover can remain moderate but comprises a less diverse community of stress-tolerant and opportunistic types (Côté and Darling, 2010; van Woesik et al., 2011; Riegl et al., 2013). Ecological homogenisation is visible across reef habitat types, with inshore reefs that are naturally exposed to a more challenging environment (e.g., in terms of light, temperature, and sediment input) favouring a smaller species pool of stress-resistant corals compared to nearby outer reef habitats (Rogers, 1990; Browne et al., 2013; Williams et al., 2013; Schoepf et al., 2015; Morgan et al., 2016).
A recent study demonstrated that whilst sea surface temperature (SST), chlorophyll concentration, and wave energy have strong power in predicting benthic assemblages at remote reefs, this predictive power is lost or the relationships fundamentally altered at reefs closer to human populations (Williams G.J. et al., 2015). Considering the dominant role of humans in shaping ecosystems, factors associated with local anthropogenic impacts may have overtaken biophysical drivers in structuring these altered reefs. It has also been postulated that reorganisation toward a stress-tolerant coral community could increase resilience to climate change, assuming co-tolerance between local and climate change-related stressors (Côté and Darling, 2010), in line with the concept of “intrinsic resistance” (Darling and Côté, 2018). Indeed, coral richness does not translate into higher resilience to disturbances (Zhang et al., 2014). Better understanding and accounting for the role that local human impacts play in shaping benthic communities and their relationships with environmental drivers is important for developing theories, designing experiments, setting baselines, informing management, as well as optimising large-scale spatial predictive models for coral reef futures.
Here, we investigate how local human impacts affect coral reef benthic communities in the tropical Pacific. We start by classifying 62 island sites into two levels of local human impact (low versus high) using a set threshold of human population density informed by previous work (i.e., D’agata et al., 2014). We then ask whether the level of local human impact influences benthic community structure or the relationship between benthic community structure and spatio-physical explanatory drivers. Lastly, we examine the relative roles of ten biophysical parameters as drivers of benthic community structure under low versus high local human impact. We hypothesised that benthic community structure would be more related to spatio-physical drivers on reefs exposed to low local human impact compared to those with high local human impact, where we expect human-associated factors to have become more dominant. This approach allowed us to develop on the findings of Williams G.J. et al. (2015) by testing whether decoupling between reefs and biophysical drivers in the presence of humans was also detectable between different levels of local human impact.
Materials and Methods
Study Area and Sampling Design
This study intended to build on work from Williams G.J. et al. (2015) by assessing in more detail how local population density, rather than human presence/absence, potentially decouples the relationship between reef benthos and larger-scale environmental drivers. To do so, we utilised a large-scale dataset that is unique in having both site-level ecological (fish and benthic) and socio-economic data (in particular human density per reef area at the site level), which is missing from other datasets that rely on global socioeconomic estimates for human population density, or in some cases district-level surveys. Fish and benthic communities at 62 reefs within 17 different Pacific Island countries and territories were surveyed once between 2003 and 2008 (see Supplementary Figure 1 for map) as part of the Pacific Regional Oceanic and Coastal Fisheries Development Programme (PROCFish/C/CoFish) under the auspices of the Pacific Community (SPC). Importantly, all reefs were in close vicinity to, and used by, coastal human communities across a large range of intensities (e.g., relative human density ranged from 1.3 to 1705 humans km–2 reef). For site disturbance history, sampling dates and detailed sampling methodology, refer to Supplementary Table 1 and Pinca et al. (2010). Though it is important to acknowledge that the sampling programme was not originally designed in a way to address macroecological questions or aggregate beyond the state/territory level, we did our best to account for the shortcomings in the design by including additional information such as observer bias (see section “Data Analyses” for details on environmental parameters included). Furthermore, while data may not be representative of the current-day scenario, it is the trends that are important for this study. Accordingly, we are confident that despite some inevitable compromising, this gave us the best possible dataset to look at the effects of site-specific human density.
Field Surveys
Underwater surveys covered outer (fore-) reefs, with on average nine (n = 3–47) joint fish-benthic 50 m transects measured at each site. Transect data were pooled within each site. Reef fish communities were measured using the variable distance-sampling underwater visual census method along transects (described in Labrosse et al., 2002). Data on abundance and size were recorded to species-level for herbivorous fish. Counts were converted to biomass (g m–2) from established length-weight relationships (Kulbicki et al., 2005). Benthic cover data was obtained using the medium-scale approach described by Clua et al. (2006). This method is based on a semi-quantitative description of ten 25 m2 (5 × 5 m) quadrats laid down on each side of the 50 m transect (i.e., 20 replicate quadrats/500 m2 per transect). Surveyors first recorded abiotic and live coral substrates, i.e., sand, rubble, rocky slab, boulders, and hard coral − live, bleached, and long dead, with live coral divided into broad morphologies (e.g., branching, encrusting, massive). Each component was quickly estimated using a semi-quantitative scale ranging from 0 to 100% per quadrat, in units of 5%. Secondly, benthic groups (e.g., macroalgae–inclusive of calcified and fleshy types, turf algae, crustose coralline algae [CCA], sponges, cyanobacteria) growing over abiotic substrate such as long dead coral were recorded using the same semi-quantitative scale (Table 1).
Fishing grounds were initially delineated from information given by local fishers and quantified from satellite interpretations (similar to methods in Close and Hall, 2006; Léopold et al., 2014). Total reef area (km2) within each fishing ground was then derived from reef areas quantified by the Millennium Coral Reef Mapping Project from satellite images (Andréfouët et al., 2006). Socioeconomic assessments determined total population within communities with access to the fishing ground, allowing subsequent calculation of human population relative to reef area (referred to as “relative human density”). Finfish landings for each site, determined from interviews with fishers, were extrapolated to total finfish catch per year per reef area (“relative fishing pressure”) (see Pinca et al., 2010).
Data Analyses
Response Variables
As response variables, we selected the main benthic groups (i.e., those with a mean composition >10% of benthic community): dead coral (incorporating long dead coral, rubble, boulders, and pavement), live hard coral, and algal groups (differentiating turf algae, macroalgae, and CCA). We also assessed the proportional representation (within the live hard coral community) of the three most commonly observed morphological groups: branching, encrusting and massive morphologies. Pairwise relationship tests (corvif function–Zuur et al., 2009) established no collinearity among the response variables (correlation; R2 < 0.5). Table 1 provides an overview of the benthic variables and their ranges.
Model Predictors
We had to make a feasible choice of how to determine low versus high impact sites. Rather than choosing a completely arbitrary threshold, we selected one that was informed by previous findings by D’agata et al. (2014)–using boosted regression trees for the same dataset, the authors identified 25 people km–2 reef to be the threshold after which taxonomic diversity of parrotfishes significantly declined. We then ran a sensitivity analysis to demonstrate how robust our findings were (see section “Assumptions and Sensitivity Tests”). To compare reefs exposed to different disturbance regimes, we thus categorised all sites into those subject to low (i.e., <25 people km–2 reef; n = 29) and high (i.e., >25 people km–2 reef; n = 33) local human impact. Relative human density correlates with relative exploitation–i.e., relative human density was collinear (R2 = 0.8) with fishing pressure (tonnes fish km–2 reef year–1; Table 2). This threshold was also a median point and allowed similar sample sizes in each impact level (see Supplementary Figure 2). Relative human density also showed weak positive correlation with market gravity–an index combining the population size of nearby human settlements and their accessibility to reefs (Cinner and Maire, 2018; Cinner et al., 2018). However, we decided to base our study on relative human density as we had unique site-level data and we see this metric to be more directly linked to benthic communities in terms of human density-dependent sewage and agricultural run-off in addition to subsistence and artisanal fishing.
The selected biophysical predictors included a variety of factors that were either collected during field surveys or extracted from public data repositories (Table 2). Due to inherent differences in coral reefs across latitudinal scales (e.g., Hughes et al., 1999; Harriott and Banks, 2002), latitude was represented by degree distance from the equator without differentiating between north and south (0–23.9°). Degree heating weeks (DHW) data were extracted from the NOAA Coral Reef Thermal Anomaly Database (CoRTAD version 4 −Casey et al., 2012). Storm exposure was quantified from the NOAA IBTrACS-WMO data (Knapp et al., 2010a,b) within ArcMAP 10.4 (ESRI, 2011), where the number of storms (categories 1–5 on the Saffir-Simpson Hurricane Scale) passing within a 50 km radius of each site (Behrmann projection) was extracted. Storm exposure and DHW data were confined to 12 years prior to each respective site’s survey date based on the premise that remote reefs can recover from acute disturbances within this timeframe (Sheppard et al., 2008; Gilmour et al., 2013). Reef depth, estimated during field surveys, was averaged over all transects at each site. Island relief refers to each site’s geomorphology, and was classified into three categories: atoll, low-lying island, and high island based on available information (see Supplementary Table 1 for references), and authors’ knowledge. Island relief was included as a predictor due to its known influence on coral reef benthic and fish communities (Donaldson, 2002; Houk et al., 2015). Herbivorous fish from selected families (e.g., excluding herbivorous damselfish) encountered during visual surveys were classified into functional groups according to Heenan et al. (2016) (see Supplementary Table 2). Biomasses (g m–2) of the following functional groups were then incorporated as predictors: browsers, grazers, detritivores, scrapers and small excavators, and large excavators and bioeroders. Market gravity (Cinner and Maire, 2018) was extracted for each site in QGIS (QGIS Development Team, 2019) and was incorporated as a continuous predictor.
Prior to model fitting, paired plots were assessed for collinearity between model terms. Strong collinearity (R2 > −0.9) between latitude and DHW precluded their joint inclusion in subsequent models, and consequently latitude was selected because of its complete reef-specific dataset (DHW data limited to n = 55 sites). Multi-collinearity was also then tested using the generalised variance inflation factor (GVIF) function (car package−Fox and Weisberg, 2019) where values >3 suggest collinearity; as a result longitude was excluded from all models and the joint inclusion of browsers and scrapers was prohibited (i.e., individual best-fit models -see “Statistical Models” section- were constrained to contain only one or the other).
Statistical Models
All statistical analyses were performed in R version 3.6.1 (R Development Core Team, 2019). Differences in benthic community structure between reefs exposed to low versus high local human impact level were tested using t-tests with appropriate variance structures depending on homogeneity of variance test outcomes. Due to surveyor discrepancies in recording turf algal cover, we created a random effect (bias_score) to be incorporated within turf algae models (see Supplementary Figure 3 for details). No surveyor-related discrepancies were evident for other benthic groups (see Supplementary Figure 3). To test how turf algal cover differed across the two local human impact levels we thus used a linear mixed effects model incorporating bias_score as a random effect. All hereon described models were run separately for sites predetermined to be exposed to either low or high local human impacts to explicitly test for decoupling of abiotic and biotic predictors under different disturbance regimes.
To test whether the level of local human impact influenced the relationship between benthic community structure and spatio-physical explanatory drivers, we developed a spatio-physical model (i.e., focussing on spatial and physical predictors only) that included storm exposure, reef depth, latitude, and island relief. To account for non-linear relationships between response variables and predictors, we applied generalised additive mixed effects models (GAMM) using the gamm4 (Wood and Scheipl, 2014) and lme4 packages. To account for possible spatial autocorrelation, ten unique island clusters (cluster) were identified and incorporated into models as a random effect (for details see Supplementary Figure 4). For turf algae models, bias_score was additionally included as a random effect. To avoid overfitting, the number of knots within models was limited to four. We retained the adjusted-R2 (Adj-R2) values from the model output to quantify each model’s explanatory power. Adj-R2 values for each response variable were then compared (by paired t-tests) to test overall differences in model performance in explaining benthic community structure under the two local human impact levels.
Lastly, to examine the relative roles of ten biophysical parameters as predictors of benthic community structure under low versus high local human impacts, we applied model selection techniques using the MuMIn package (Barton, 2016). From an initial model containing ten biophysical predictors (i.e., those included in the spatio-physical model as well as biomass of herbivorous fish functional groups, and market gravity–see Table 2), the dredge function was used to run all possible predictor combinations and rank models from best to worst based on Akaike weight. The function also returns a value between 0 and 1 for each predictor that reflects its relative importance (RI), representing the total Akaike weight of all models containing that predictor (i.e., higher values correspond to greater RI). Output models were restricted to comprising a maximum of four predictors. One sample (Niue) was removed from the model selection procedure due to a lack of data for market gravity (i.e., n = 61). Model selection was run separately for sites with low and high levels of local human impact, and all models incorporated the uGamm wrapper function to allow the inclusion of random effects consistent with spatio-physical model constructions. To assess incongruities between benthic communities exposed to different local human impact levels, we retained the best-fit model structures (i.e., all predictors included in models with Akaike weight >0.05) for each benthic response variable, as well as the RI of individual predictors.
Assumptions and Sensitivity Tests
All response variables were logit-transformed (appropriate for percentage data −Warton and Hui, 2011) using the car package, following adjustment using each respective variable’s minimum value >0. All model predictors were standardised (z-scores) to allow comparisons between predictors with largely varying effect sizes and numeric values (Zuur et al., 2009). Model residuals were checked for the violation of model assumptions using the gam.check function (see Supplementary Figure 5). As part of a sensitivity analysis, spatio-physical models were additionally run using ±5 and ±10 humans km–2 reef as a threshold from which to categorise “low” and “high” impact sites, with consistent outcomes observed (see Supplementary Table 3). Furthermore, we repeated the same for a range of thresholds based on human density relative to outer reef area (as opposed to full reef area), again with consistent outcomes observed (see Supplementary Table 4).
Results
Benthic Assemblages Under Contrasting Local Human Impacts
Benthic communities subject to high local human impact were associated with lower live hard coral cover (−9.2% mean absolute coverage; t-test: p = 0.002; Figure 1A). However, the relative contribution of different coral morphologies within the live hard coral community did not differ significantly with local human impact level (Figure 1B). The only algal group that differed significantly depending on the local impact level was turf algae, which was higher under high local human impact (+10.7% mean absolute coverage; linear mixed effects model: p = 0.015; Figure 1C).
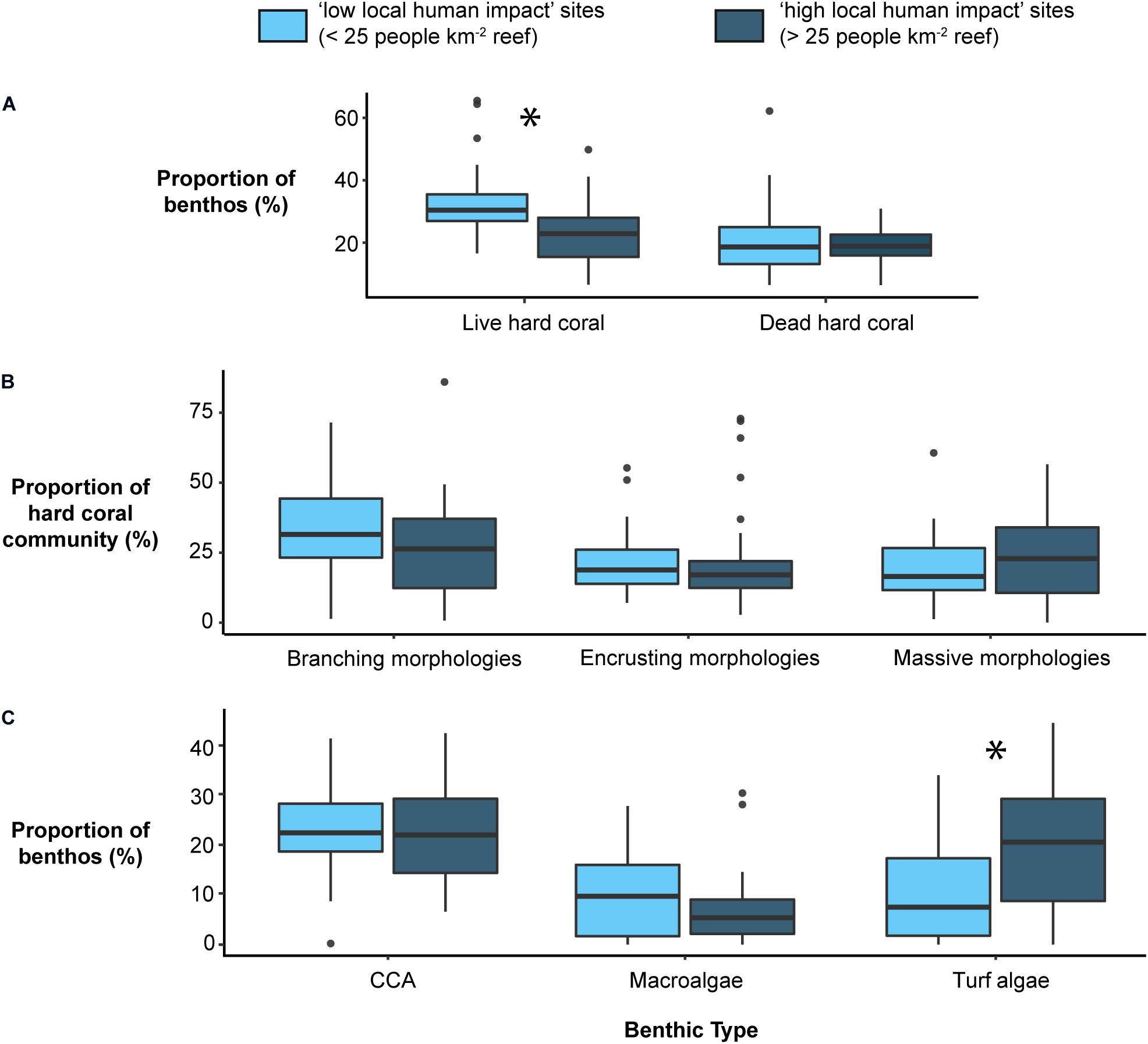
Figure 1. Boxplots detailing (A) benthic cover (%) of substrate types (from left to right; live hard coral, dead hard coral), (B) the relative proportion (%) of the three most dominant morphologies in the live hard coral community (branching, encrusting, massive), and (C) benthic cover (%) of different algal groups (CCA, macroalgae, turf algae), at sites determined to have low or high local impacts. *Indicate significant differences (p < 0.05) in cover of the respective benthic variable between local impact levels according to two-sample t-tests, or for turf algae according to linear mixed effect models incorporating bias_score as a random effect (see section “Model Predictors”).
Predictive Strength of Spatio-Physical Model
The pre-defined spatio-physical model exhibited relatively high power in explaining benthic assemblage variance at sites with low local human impacts (mean Adj-R2 ± SE; 0.35 ± 0.09; Figure 2), but model performance was severely compromised when local human impacts were high (0.10 ± 0.04; paired t-test: p = 0.01). When considering only the substrate types and dominant morphological groups (i.e., without the algal groups) the mean adjusted-R2 for sites with low local human impacts increases to 0.44 ± 0.06 but stays unchanged at sites with high local human impacts. When local human impacts were low, spatio-physical predictors explained a high proportion of the variance of live hard coral (Adj-R2 = 0.52) and macroalgae (Adj-R2 = 0.59) cover, and the relative contribution of branching (Adj-R2 = 0.64) and massive (Adj-R2 = 0.50) coral morphologies. Conversely, these variables were consistently poorly explained when local human impacts were high (Adj-R2 = 0.00, 0.26, 0.17, 0.10, respectively). No variance in turf algae was explained by this model for reefs at either local human impact level. Significant differences in the power of the spatio-physical model in explaining benthic assemblage variance between “low” and “high” impact sites held constant when the threshold was moved ±5 and 10 humans km–2 reef (i.e., paired t-test: p < 0.05; Supplementary Table 3).
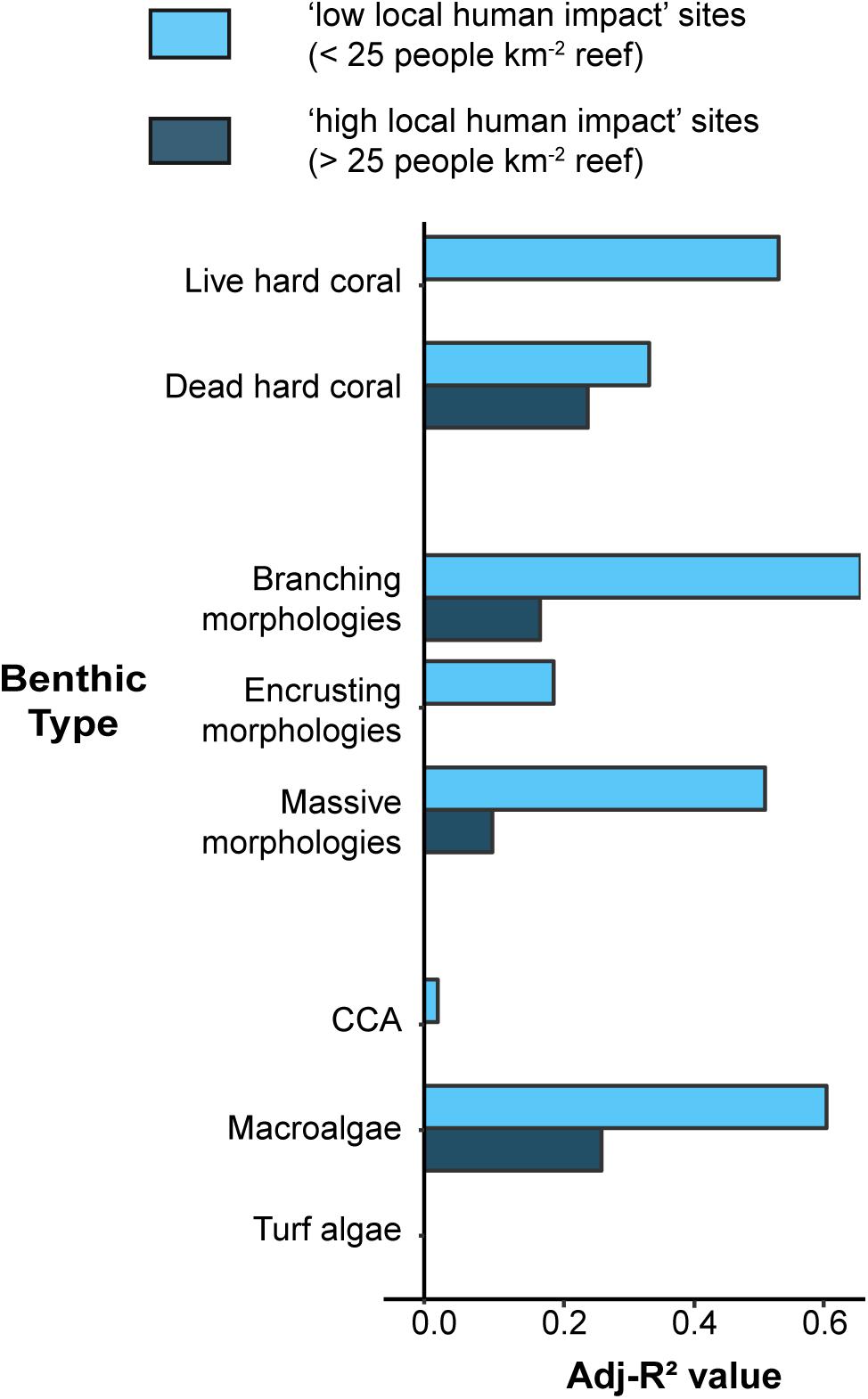
Figure 2. Explanatory power (Adj-R2) of the spatio-physical model (including the predictors storm exposure, reef depth, latitude, and island relief) in predicting individual benthic responses (live hard coral, dead hard coral, the proportion of branching, encrusting, and massive morphologies, and cover of algal groups CCA, macroalgae, and turf algae). Bars are absent where the Adj-R2 value = 0.
Relative Importance of Predictors
Best-fit models tailored for each individual benthic variable comprised distinctly different predictors depending on the level of local human impact (Figure 3A). Two of the predictors where discrepancies were most apparent were storm exposure and grazer biomass, which were only selected for best-fit models at sites with low and high local human impacts, respectively. Similarly, reef depth was selected as part of best-fit models for more response variables (live hard coral, branching morphologies, and macroalgae) at sites with low local human impacts. Further discrepancies under the two levels of local human impact were revealed by comparing the mean RI of each predictor (Figures 3B,C), as the RI trends for predictors almost reversed between low versus high local human impact sites. At sites with low local human impacts, the individual predictors with the highest RI in explaining benthic communities were storm exposure, scraper biomass, and island relief, followed by reef depth (Figure 3B). Contrastingly, apart from island relief which was on average the most important predictor, storm exposure, scraper biomass, and reef depth were among the least important predictors when local human impact was high (Figure 3C). For benthic communities exposed to high local human impact, biomass of grazers, detritivores, and browsers, as well as market gravity, ranked as the most important predictors of benthic community structure.
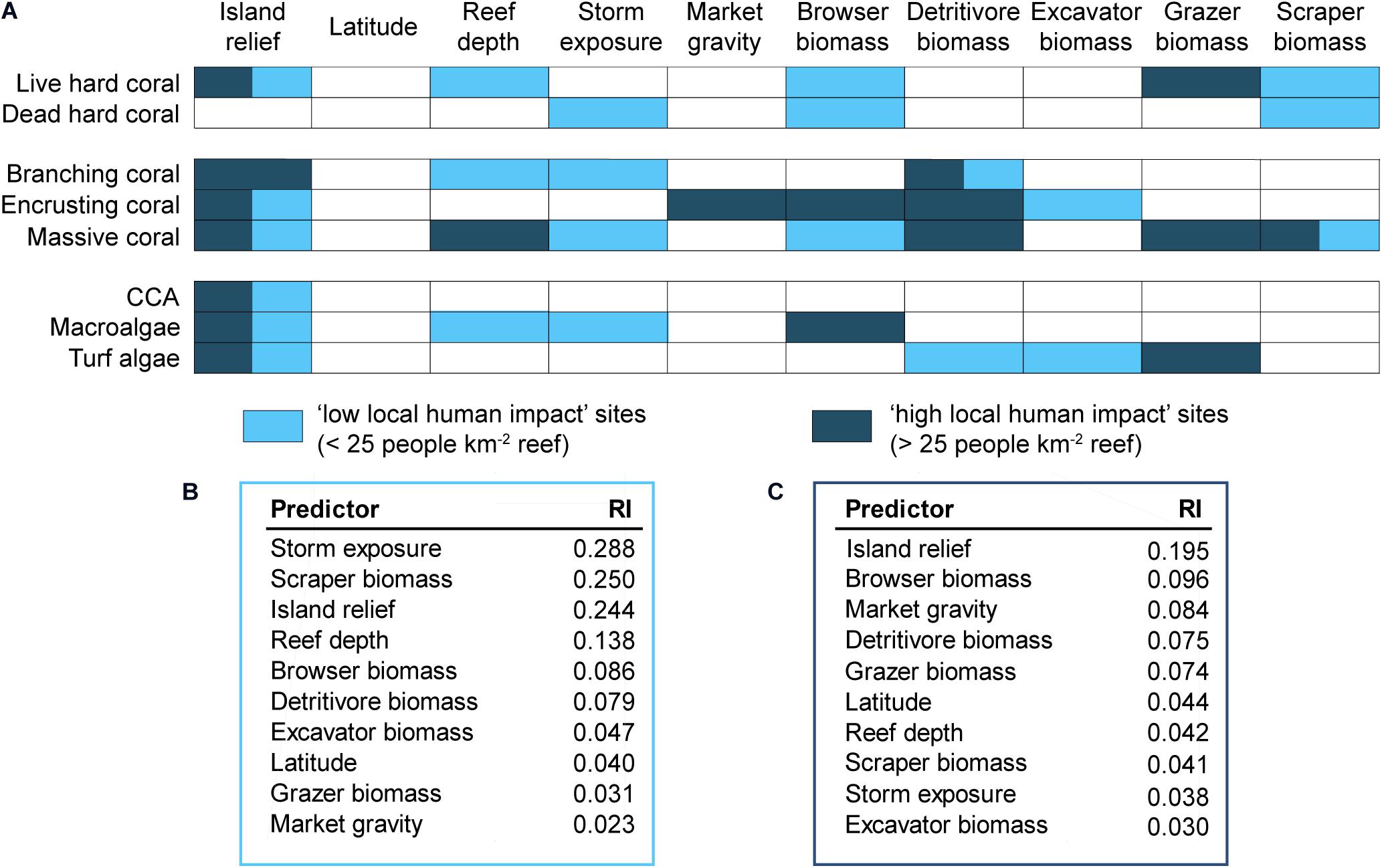
Figure 3. (A) Outcome of model selection procedures: coloured boxes indicate that the predictor was selected for best-fit models (i.e., those with Akaike weight >0.05) at sites subject to low and/or high levels of local impacts. The overall mean relative importance of each predictor (RI–based on total Akaike weight of all models including that predictor) in explaining the benthic response variables is shown separately for sites subject to (B) low and (C) high local impacts. Note: though model selection was limited to four predictors per model, all predictors included in models with Akaike weight >0.05 are shown meaning there may be more than four predictors shown within one impact level for a response variable. Model outputs for best-fit models are available in Supplementary Table 5.
Individual relationships of best-fit predictors for each response variable further emphasised discrepancies between benthic communities and environmental predictors driven by local human impacts (for all plots see Supplementary Figure 6). A clear example of this discrepancy can be seen in coral compositional changes with increasing storm exposure. Whilst live hard coral cover remained unrelated to storm exposure under both local human impact levels (Figure 4A), the morphological composition was closely correlated with storm exposure at sites where local human impacts were low (Figures 4B–D). Specifically, with increasing storm exposure, the relative proportion of branching morphologies decreased significantly (Figure 4B), whereas encrusting (Figure 4C) and massive (Figure 4D) morphologies increased. No morphological changes with storm exposure were observed at sites with high local human impacts. Though these relationships appear primarily driven by few points at the higher end of storm exposure, they remained consistent when all reefs exposed to >10 storms were removed from the analysis–i.e., significant at “low” impact sites (branching coral p = 0.02; encrusting coral p = 0.02; massive coral p = 0.03), insignificant at “high” impact sites (all morphological growth forms p > 0.05), and no relationship for either impact level for total live hard coral cover (p > 0.05).
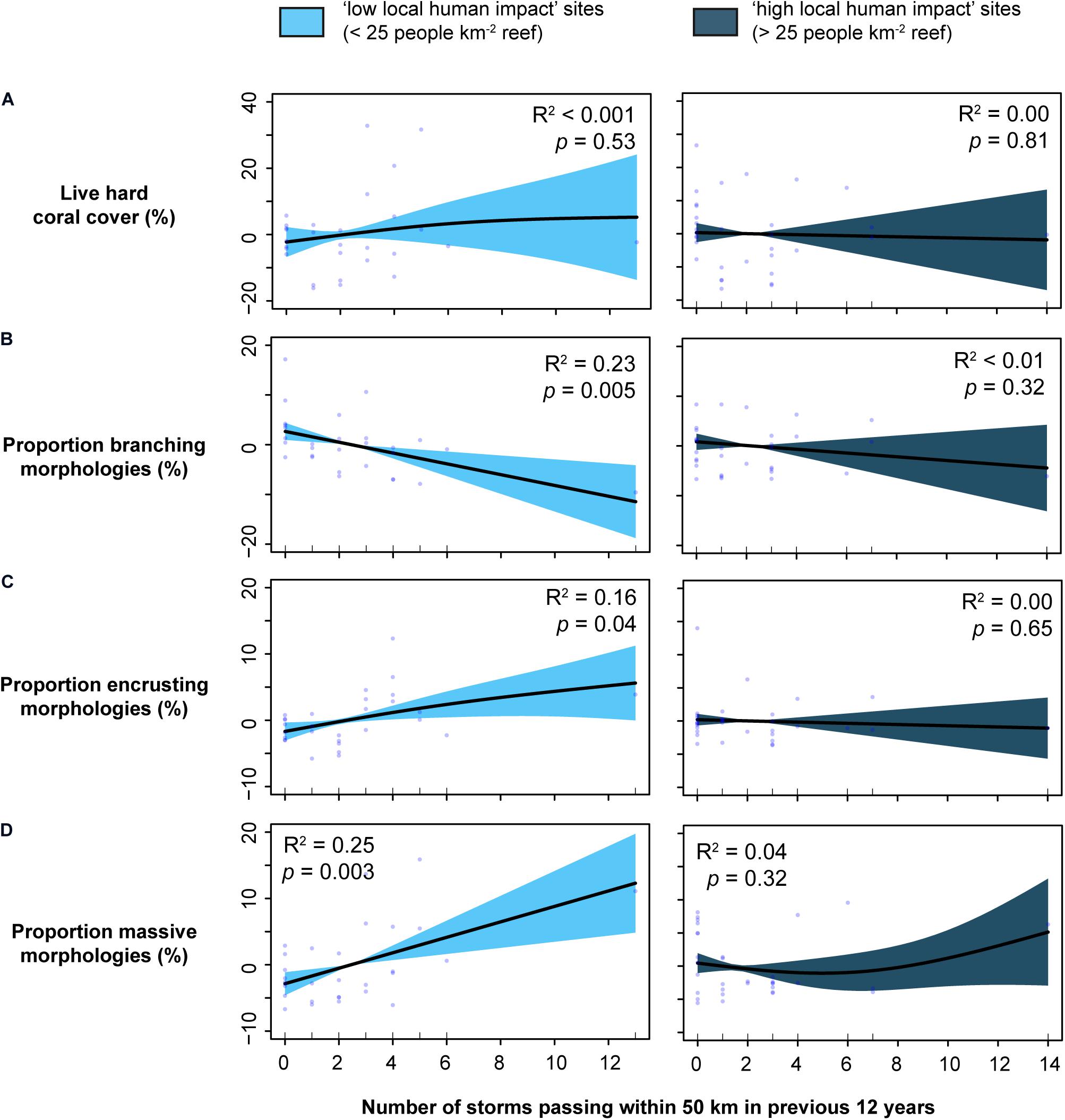
Figure 4. Smoother plots of normalised residuals from generalised additive models with 95% confidence intervals (shaded areas) to exemplify discrepancies in response-predictor relationships between local impact levels, using storm exposure as an example. The plots represent the explanatory power of storm exposure in predicting (A) live hard coral cover, and the proportion of (B) branching, (C) encrusting, and (D) massive morphologies, separated for sites with low versus high local impacts. Refer to Supplementary Figure 6 for all predictor-response plots from best-fit models.
Discussion
Under increasing climate change-associated stressors and local anthropogenic influence (Burke et al., 2011; Gattuso et al., 2015; IPCC, 2019), it is particularly important for researchers and planners to maximise the ability of models to predict ecosystem futures to allow appropriate mitigation strategies to be implemented. This study indicates that the role of local human impacts in changing coral reef ecological communities and their responses to environmental drivers should be accounted for. The results revealed that local human impacts influenced both benthic community structure and relationships with biophysical predictors. Specifically, models based on spatio-physical predictors (i.e., reef depth, latitude, storm exposure, and relief) exhibited high power at explaining benthic assemblages under low local human impacts but were strongly compromised where local human impacts were high. Importantly, these outcomes remained similar when our threshold for human impacts, informed by previous work, was reduced or increased in the frame of a sensitivity analysis. Increasing sewage input, agricultural run-off, and sedimentation are potential changes associated with increasing human densities that reduce water quality and affect benthic communities (Fabricius, 2005; Fabricius et al., 2005; Ford et al., 2017). Furthermore, as fishing removes biomass of functionally important fish species, important top-down control of some benthic organisms is lost (Bellwood et al., 2004). These localised human impacts may homogenise benthic communities by driving ecological reorganisation that favours tolerant taxa (Darling et al., 2019). Our results indicate that this homogenisation may be occurring at Pacific Island reefs that are exposed to local human impacts, leading to novel systems that react fundamentally differently and unpredictably to environmental predictors compared to reefs less influenced by humans (Williams G.J. et al., 2015). In turn, we expect that local human impacts will influence responses of coral reefs to climate change-related stressors, and that reefs close to human populations will require context-specific management approaches to maximise their future sustainability and associated critical ecosystem services (Moberg and Folke, 1999).
The results emphasise the variation in benthic assemblages that exists among Pacific Island reefs exposed to different levels of local human impact. Benthic communities were not restricted to distinct regimes dominated by either hard corals or macroalgae, supporting previous studies from the Pacific (Bruno et al., 2009; Albert et al., 2012; Jouffray et al., 2015, 2019; Smith et al., 2016). In fact, macroalgae was the least common of all the algal groups, with turf algae and CCA more prominent on these outer reefs. Reefs at sites with higher local human impacts comprised significantly more turf algae and less live hard coral. Abundant and diverse outer reef coral communities (e.g., Ellis et al., 2017) have likely retained sensitive species, facilitating measurable differences (i.e., reductions in live hard coral cover) under higher local human impacts. Higher turf algae coverage at sites with more local human impacts provides further evidence that turf algae may become the dominant benthic group on degraded Pacific Island reefs (Jouffray et al., 2015; Smith et al., 2016; Tebbett and Bellwood, 2019). This may contrast with coral reef systems in the Caribbean, where macroalgae naturally play a more dominant role (Roff and Mumby, 2012). Furthermore, a signal of local human impact may have been detected if the data had distinguished macroalgae into fleshy/frondose and calcified types (e.g., Smith et al., 2016; Cannon et al., 2019). A similar link between human population size, hard coral cover, and turf algae has also recently been reported from sites in the Indian Ocean (Brown et al., 2017).
The spatio-physical models were weak in explaining the variance of benthic communities exposed to relatively more local human impacts. At these sites, model selection identified mostly local biotic controls or ecological features such as fish biomass and market gravity to be of highest RI. These results suggest that reefs altered by chronic local human impacts become decoupled from spatio-physical factors (Williams G.J. et al., 2015) and become more related to factors associated with human activities (e.g., market gravity) or local ecological features (e.g., biomass of functional groups of fish). Interestingly, this outcome contrasts to recent findings by Robinson et al. (2018) who did not detect decoupling at inhabited versus uninhabited reefs. Our different outcomes for decoupling could in part be explained by Robinson et al. (2018) combining (i) hard corals and CCA, and (ii) fleshy macroalgae and turf algae, each of which we found to exhibit different responses to local impacts (i.e., with higher local impacts hard coral cover decreased whereas CCA remained unchanged, turf algae increased whereas macroalgae remained unchanged). Model selection indicated that the specific spatio-physical predictors whose influence was most disrupted by local human impacts were storm exposure and reef depth. Storms can have mixed effects, benefitting reefs by alleviating thermal stress during warmer summer months but also causing physical destruction, particularly to delicate branching coral morphologies, leading to a higher proportion of more robust massive morphologies (Heron et al., 2005; Manzello et al., 2007). Accordingly, when local human impacts were low, coral communities comprised relatively less branching and more encrusting and massive morphologies at sites subject to more frequent storms. However, relationships between storm exposure and benthic assemblages were only observed at sites classified as having low local human impacts. Returning to the concept that local human impacts drive ecological reorganisation, we would expect reefs with less local human impacts to harbour a great diversity of species and morphologies, thus allowing for greater levels of ecological reorganisation in response to a stormier environment (i.e., favouring more robust morphologies). We anticipate that Pacific reefs would more likely display this phenomenon than Caribbean reefs due to a significantly larger initial species pool, with greater response diversity and functional redundancy affording a higher level of ecological insurance (Elmqvist et al., 2003; Bellwood et al., 2004; Nyström, 2006), and a loss of structurally complex coral species throughout most of the Caribbean over past decades (Alvarez-Filip et al., 2009). It would be interesting to test whether storm exposure results in more conspicuous negative impacts on coral cover (e.g., Gardner et al., 2005) in less diverse regions due to the limited capacity for ecological reorganisation to a tolerant community, even in sites with minimal local human impact. In terms of reef depth, benthic communities at sites with low local human impacts also exhibited a higher level of depth-structuring (particularly in terms of coral cover and composition, consistent with Huston, 1985) compared to those with high local human impacts. Island relief was also identified as being a strong predictor of benthic community structure (despite being found to be a weak predictor on central-western Pacific reefs −Robinson et al., 2018), and interestingly this role was maintained regardless of local human impact level. Importantly, when considering latitude–collinear with DHW/cumulative thermal stress–as an individual predictor, our results neither contradict nor confirm previous observations that local impacts exacerbate the sensitivity of coral communities to thermal stress (Wiedenmann et al., 2013; Ellis et al., 2019), instead highlighting a large variance among sites.
Thermal stress and the intensity of storms are projected to increase under future climate change scenarios (IPCC, 2019), with profound implications for coral reefs and adjacent ecosystems. Our findings suggest that while the effects of factors associated with climate change (e.g., storm exposure) on Pacific reef benthic assemblages may be reasonably well-predicted where local impacts are low, system responses become less predictable as local human impacts increase. In both marine and terrestrial systems, structural changes caused by local impacts have profoundly changed how ecosystems respond to natural stressors: for example, local stressors have affected how parts of Australia’s Great Barrier Reef have recovered from recent climate change impacts (MacNeil et al., 2019; Mellin et al., 2019) and habitat fragmentation and modification have exacerbated recent impacts of tropical and temperate forest fires (Brando et al., 2014; Alencar et al., 2015; Taylor et al., 2016). Because the effects of storms are strongly dependent not only on their intensity, but also the extent of the fetch, their frequency, and intrinsic reef properties such as topography (Lugo et al., 2000; Heron et al., 2005), this study incorporated all recorded storms (category 1–5 on the Saffir-Simpson Hurricane Scale) passing within 50 km of each site. We thus cannot deduce benthic community responses to increasing storm intensity, which is projected to occur (IPCC, 2019).
Functional groups of herbivorous fishes also emerged to be of contrasting RI depending on the level of local human impact, shifting from scrapers and small excavators at less impacted reefs to grazers at more impacted reefs. Browsers were of similar importance at reefs exposed to both local human impact levels, perhaps linked to the fact that their food source (macroalgae) remained constant regardless of local human impact level. Additional reasons for this could be that browsers have remained more resilient to fishing pressure than other herbivores, or that visual survey data does not accurately represent browser populations (for example some browser species are known to be particularly wary of divers–Kulbicki, 1998). Fish that act on turf algae and/or on surfaces available for coral settlement seem to be more sensitive to local human impacts, likely in response to benthic community shifts. Scrapers and small excavators clear substrate for calcifiers, justifying their higher RI in models focussed on less locally impacted reefs where live hard coral cover was higher and conditions for settlement and growth of juvenile corals were likely better (e.g., less nutrients, lower sedimentation). Contrastingly, grazers crop and maintain algal turfs, explaining their importance under higher local human impacts where turf algae were more dominant. These results align with findings in the Hawaiian Archipelago, where biomass of grazers and scrapers were the most important predictors of turf/macroalgal, and calcified regimes, respectively (Jouffray et al., 2015; see also Robinson et al., 2018). Though collinearity tests ruled out significant, potentially confounding relationships between biomass of different functional groups with individual abiotic predictors, it is important to acknowledge that fish communities themselves can be affected by various physical predictors (Williams I.D. et al., 2015; Samoilys et al., 2019), which could in turn influence benthic structure.
Other factors not included in these analyses are known to structure benthic assemblages, including chlorophyll, SSTs, and wave exposure (Gove et al., 2013, 2015; Williams et al., 2013; Robinson et al., 2018; Darling et al., 2019). Remotely sensed chlorophyll data captures offshore productivity, but we expected land-based input to dominate many of these reefs which are close to land, while the survey design (sometimes around the periphery of small islands/atolls) made wave exposure challenging to quantify. Additionally, wave exposure, mean SSTs, and climatological ranges can be relatively well-captured by latitude in the Pacific (Gove et al., 2013). Also, although this study goes into more detail than many similar large-scale analyses by evaluating coral growth forms, it is still limited in its ability to quantify ecological reorganisation, which would require higher resolution data (at least family or genus). This limitation can be overcome by broad-scale surveys refining the level at which hard coral communities are recorded, which will become easier with improvements in automated software tools. These results do however emphasise that even broad morphological groupings (i.e., branching, massive, encrusting morphologies) provide pertinent information on ecological changes and can improve model performance compared to when overall hard coral cover is considered (Gove et al., 2015).
Importantly, the threshold used in this study for determining low and high human impact, while informed by a previous study, was set a priori and thus does not allow defining a “carrying capacity” of human density–this would require a different survey design and analytical approach, and should be pursued in future studies. We would however suggest that although this metric is most applicable to areas where customary resource use is common practice, we anticipate that this threshold (25 humans km–2 reef) is highly relevant across the tropical Pacific (i.e., given that it is a median point in this dataset for which sites were selected due to being regionally representative of fished areas). Furthermore, the study design was not originally meant to address macroecological questions but was nonetheless the best available to study the questions we were interested in (with incorporation of appropriate secondary data on local environmental context). Again, future studies should address this by appropriate designs that allow for large-scale comparability and collect both ecological and socio-economic local data. Our findings indicate that island relief is a factor that should be considered in designing regional sampling programmes with comparable sites. Finally, we should aim to obtain context-specific information on factors associated with human population density (e.g., sewage treatment presence, farming and associated fertiliser-usage) that strengthens our ability to predict benthic communities under various levels of human population density and improve its use as a proxy of local human impact.
Our findings contribute to a better understanding of the role of local human impacts on highly diverse ecosystems such as tropical coral reefs. The results provide empirical evidence that local human impacts drive conspicuous changes in benthic community relationships with environmental predictors, with indications of ecological reorganisation. Even if decisive steps are taken to reduce fossil fuel emissions, most reefs will suffer long-term degradation from the effects of climate change by 2050, and >75% of reefs will experience annual severe bleaching (Frieler et al., 2013; van Hooidonk et al., 2016). Our results show that local human impacts can lead to increasingly unpredictable relationships between benthic communities and their physical environment, and that overlooking their role could pave the way to significant errors in future projections, potentially compromising mitigation efforts.
Data Availability Statement
The raw data supporting the conclusions of this article will be made available by the authors, without undue reservation.
Author Contributions
AF developed the study idea with close support from J-BJ, AN, BM, and SF. AF led the statistical analyses together with input from J-BJ, GW, and SF. BM and FM provided expertise on the original data. All authors provided support throughout the interpretation of the results and development of the manuscript and approve this final version.
Funding
The COFish and PROCFish-C Programmes were funded by the European Union. AF, SB, and SF were funded by the (German) Federal Ministry of Education and Research (BMBF) through the “Nachwuchsgruppen Globaler Wandel 4 + 1” (REPICORE, grant number 01LN1303A).
Conflict of Interest
The authors declare that the research was conducted in the absence of any commercial or financial relationships that could be construed as a potential conflict of interest.
The reviewer KO declared a past collaboration with several of the authors J-BJ, AN, GW, and SF to the handling editor.
Acknowledgments
We would like to thank the Pacific Community survey team, in particular Samasoni Sauni, Pierre Boblin, Ribanataake Awira, Laurent Vigliola and Silvia Pinca, as well as the fisheries officers across the PICTs who assisted with logistics and data collection. Data collection was made possible by the support of in-country Fisheries Divisions, as well as the chiefs and people of all local communities at the surveyed sites. The research reported in this manuscript contributes to the Programme on Ecosystem Change and Society (www.pecs-science.org).
Supplementary Material
The Supplementary Material for this article can be found online at: https://www.frontiersin.org/articles/10.3389/fmars.2020.571115/full#supplementary-material
References
Albert, S., Dunbabin, M., Skinner, M., Moore, B., and Grinham, A. (2012). “Benthic shift in a Solomon Islands’ lagoon: corals to cyanobacteria,” in Proceedings of the 12th International Coral Reef Symposium, Cairns, Australia, 9-13 July 2012, Cairns, 1–5.
Alencar, A. A., Brando, P. M., Asner, G. P., and Putz, F. E. (2015). Landscape fragmentation, severe drought, and the new Amazon forest fire regime. Ecol. Appl. 25, 1493–1505. doi: 10.1890/14-1528.1
Alvarez-Filip, L., Dulvy, N. K., Gill, J. A., Côté, I. M., and Watkinson, A. R. (2009). Flattening of Caribbean coral reefs: region-wide declines in architectural complexity. Proc. R. Soc. B Biol. Sci. 276, 3019–3025. doi: 10.1098/rspb.2009.0339
Andréfouët, S., Muller-Karger, F. E., Robinson, J. A., Kranenburg, C. J., Torres-Pulliza, D., Spraggins, S. A., et al. (2006). “Global assessment of modern coral reef extent and diversity for regional science and management applications: a view from space,” in Proceedings of 10th International Coral Reef Symposium, Okinawa, 1732–1745.
Ban, S. S., Graham, N. A. J., and Connolly, S. R. (2014). Evidence for multiple stressor interactions and effects on coral reefs. Glob. Change Biol. 20, 681–697. doi: 10.1111/gcb.12453
Barton, K. (2016). MuMIn: Multi-Model Inference. R Package Version 1.15.6. Available online at: https://CRAN.R-project.org/package=MuMIn (accessed April 15, 2020).
Bellwood, D. R., Hughes, T. P., Folke, C., and Nyström, M. (2004). Confronting the coral reef crisis. Nature 429, 827–833. doi: 10.1038/nature02691
Brando, P. M., Balch, J. K., Nepstad, D. C., Morton, D. C., Putz, F. E., Coe, M. T., et al. (2014). Abrupt increases in Amazonian tree mortality due to drought–fire interactions. Proc. Natl. Acad. Sci. U.S.A. 111, 6347–6352. doi: 10.1073/pnas.1305499111
Brewer, T. D., Cinner, J. E., Fisher, R., Green, A., and Wilson, S. K. (2012). Market access, population density, and socioeconomic development explain diversity and functional group biomass of coral reef fish assemblages. Glob. Environ. Change 22, 399–406. doi: 10.1016/j.gloenvcha.2012.01.006
Brown, K. T., Bender-Champ, D., Bryant, D. E. P., Dove, S., and Hoegh-Guldberg, O. (2017). Human activities influence benthic community structure and the composition of the coral-algal interactions in the central Maldives. J. Exp. Marine Biol. Ecol. 497, 33–40. doi: 10.1016/j.jembe.2017.09.006
Browne, N. K., Smithers, S. G., and Perry, C. T. (2013). Spatial and temporal variations in turbidity on two inshore turbid reefs on the Great Barrier Reef, Australia. Coral Reefs 32, 195–210. doi: 10.1007/s00338-012-0965-1
Bruno, J. F., Sweatman, H., Precht, W. F., Selig, E. R., and Schutte, V. G. W. (2009). Assessing evidence of phase shifts from coral to macroalgal dominance on coral reefs. Ecology 90, 1478–1484. doi: 10.1890/08-1781.1
Bruno, J. F., and Valdivia, A. (2016). Coral reef degradation is not correlated with local human population density. Sci. Rep. 6:29778. doi: 10.1038/srep29778
Burke, L. M., Reytar, K., Spalding, M., and Perry, A. (2011). Reefs at Risk Revisited. Washington, DC: World Resources Institute.
Cannon, S. E., Donner, S. D., Fenner, D., and Beger, M. (2019). The relationship between macroalgae taxa and human disturbance on central Pacific coral reefs. Marine Pollut. Bull. 145, 161–173. doi: 10.1016/j.marpolbul.2019.05.024
Casey, K. S., Selig, E. R., and Foti, G. (2012). The Coral Reef Temperature Anomaly Database (CoRTAD) Version 4 - Global, 4 km Sea Surface Temperature and Related Thermal Stress Metrics for 1981-10-31 to 2010-12-31 (NODC Accession 0087989). Version 2.2. Silver Spring, MD: National Oceanographic Data Center, NOAA.
Cinner, J. E., Maire, E., Huchery, C., MacNeil, M. A., Graham, N. A. J., Mora, C., et al. (2018). Gravity of human impacts mediates coral reef conservation gains. Proc. Natl. Acad. Sci. U.S.A. 115, E6116–E6125. doi: 10.1073/pnas.1708001115
Cinner, J., and Maire, E. (2018). Global Gravity of Coral Reefs Spatial Layer. Queensland: James Cook University.
Close, C. H., and Hall, G. B. (2006). A GIS-based protocol for the collection and use of local knowledge in fisheries management planning. J. Environ. Manage. 78, 341–352. doi: 10.1016/j.jenvman.2005.04.027
Clua, E., Legendre, P., Vigliola, L., Magron, F., Kulbicki, M., Sarramegna, S., et al. (2006). Medium scale approach (MSA) for improved assessment of coral reef fish habitat. J. Exp. Marine Biol. Ecol. 333, 219–230. doi: 10.1016/j.jembe.2005.12.010
Côté, I. M., and Darling, E. S. (2010). Rethinking ecosystem resilience in the face of climate change. PLoS Biol. 8:e1000438. doi: 10.1371/journal.pbio.1000438
D’agata, S., Mouillot, D., Kulbicki, M., Andréfouët, S., Bellwood, D. R., Cinner, J. E., et al. (2014). Human-mediated loss of phylogenetic and functional diversity in coral reef fishes. Curr. Biol. 24, 555–560. doi: 10.1016/j.cub.2014.01.049
Darling, E. S., and Côté, I. M. (2018). Seeking resilience in marine ecosystems. Science 359, 986–987. doi: 10.1126/science.aas9852
Darling, E. S., McClanahan, T. R., Maina, J., Gurney, G. G., Graham, N. A. J., Januchowski-Hartley, F., et al. (2019). Social–environmental drivers inform strategic management of coral reefs in the Anthropocene. Nat. Ecol. Evol. 3, 1341–1350. doi: 10.1038/s41559-019-0953-8
Donaldson, T. J. (2002). High islands versus low islands: a comparison of fish faunal composition of the Palau islands. Environ. Biol. Fishes 65, 241–248. doi: 10.1023/A:1020067931910
Ellis, J., Anlauf, H., Kürten, S., Lozano-Cortés, D., Alsaffar, Z., Cúrdia, J., et al. (2017). Cross shelf benthic biodiversity patterns in the Southern Red Sea. Sci. Rep. 7:437. doi: 10.1038/s41598-017-00507-y
Ellis, J. I., Jamil, T., Anlauf, H., Coker, D. J., Curdia, J., Hewitt, J., et al. (2019). Multiple stressor effects on coral reef ecosystems. Glob. Change Biol. 25, 4131–4146. doi: 10.1111/gcb.14819
Elmqvist, T., Folke, C., Nyström, M., Peterson, G., Bengtsson, J., Walker, B., et al. (2003). Response diversity, ecosystem change, and resilience. Front. Ecol. Environ. 1:488–494. doi: 10.2307/3868116
Fabricius, K., De’ath, G., McCook, L., Turak, E., and Williams, D. (2005). Changes in algal, coral and fish assemblages along water quality gradients on the inshore Great Barrier Reef. Marine Pollut. Bull. 51, 384–398. doi: 10.1016/j.marpolbul.2004.10.041
Fabricius, K. E. (2005). Effects of terrestrial runoff on the ecology of corals and coral reefs: review and synthesis. Marine Pollut. Bull. 50, 125–146. doi: 10.1016/j.marpolbul.2004.11.028
Ferrario, F., Beck, M. W., Storlazzi, C. D., Micheli, F., Shepard, C. C., and Airoldi, L. (2014). The effectiveness of coral reefs for coastal hazard risk reduction and adaptation. Nature Commun. 5:3794. doi: 10.1038/ncomms4794
Ford, A. K., Hoytema, N. V., Moore, B. R., Pandihau, L., Wild, C., and Ferse, S. C. A. (2017). High sedimentary oxygen consumption indicates that sewage input from small islands drives benthic community shifts on overfished reefs. Environ. Conserv. 44, 405–411. doi: 10.1017/S0376892917000054
Fox, J., and Weisberg, S. (2019). An R Companion to Applied Regression, 3rd Edn. Thousand Oaks CA: Sage Publications.
Frieler, K., Meinshausen, M., Golly, A., Mengel, M., Lebek, K., Donner, S. D., et al. (2013). Limiting global warming to 2 °C is unlikely to save most coral reefs. Nature Clim. Change 3, 165–170. doi: 10.1038/nclimate1674
Gardner, T. A., Côté, I. M., Gill, J. A., Grant, A., and Watkinson, A. R. (2005). Hurricanes and caribbean coral reefs: impacts, recovery patterns, and role in long-term decline. Ecology 86, 174–184. doi: 10.1890/04-0141
Gattuso, J.-P., Magnan, A., Billé, R., Cheung, W. W. L., Howes, E. L., Joos, F., et al. (2015). Contrasting futures for ocean and society from different anthropogenic CO2 emissions scenarios. Science 349:aac4722. doi: 10.1126/science.aac4722
Gilmour, J. P., Smith, L. D., Heyward, A. J., Baird, A. H., and Pratchett, M. S. (2013). Recovery of an isolated coral reef system following severe disturbance. Science 340, 69–71. doi: 10.1126/science.1232310
Gove, J. M., Williams, G. J., McManus, M. A., Clark, S. J., Ehses, J. S., and Wedding, L. M. (2015). Coral reef benthic regimes exhibit non-linear threshold responses to natural physical drivers. Marine Ecol. Prog. Series 522, 33–48. doi: 10.3354/meps11118
Gove, J. M., Williams, G. J., McManus, M. A., Heron, S. F., Sandin, S. A., Vetter, O. J., et al. (2013). Quantifying climatological ranges and anomalies for pacific coral reef ecosystems. PLoS One 8:e61974. doi: 10.1371/journal.pone.0061974
Graham, N. A., Cinner, J. E., Norström, A. V., and Nyström, M. (2014). Coral reefs as novel ecosystems: embracing new futures. Curr. Opin. Environ. Sustain. 7, 9–14. doi: 10.1016/j.cosust.2013.11.023
Hamilton, R. J., Giningele, M., Aswani, S., and Ecochard, J. L. (2012). Fishing in the dark-local knowledge, night spearfishing and spawning aggregations in the Western Solomon Islands. Biol. Conserv. 145, 246–257. doi: 10.1016/j.biocon.2011.11.020
Harriott, V., and Banks, S. (2002). Latitudinal variation in coral communities in eastern Australia: a qualitative biophysical model of factors regulating coral reefs. Coral Reefs 21, 83–94. doi: 10.1007/s00338-001-0201-x
Heenan, A., Hoey, A. S., Williams, G. J., and Williams, I. D. (2016). Natural bounds on herbivorous coral reef fishes. Proc. R. Soc. B Biol. Sci. 283:20161716. doi: 10.1098/rspb.2016.1716
Henderson, C. J., Gilby, B. L., Schlacher, T. A., Connolly, R. M., Sheaves, M., Maxwell, P. S., et al. (2020). Landscape transformation alters functional diversity in coastal seascapes. Ecography 43, 138–148. doi: 10.1111/ecog.04504
Heron, S., Morgan, J., Eakin, M., and Skirving, W. (2005). “Hurricanes and their effects on coral reefs,” in Status of Caribbean Coral Reefs after Bleaching and Hurricanes in 2005, eds C. Wilkinson and D. Souter (Townsville: Global Coral Reef Monitoring Network and Reef and Rainforest Research Centre), 31–36.
Hoegh-Guldberg, O., Mumby, P. J., Hooten, A. J., Steneck, R. S., Greenfield, P., Gomez, E., et al. (2007). Coral reefs under rapid climate change and ocean acidification. Science 318, 1737–1742. doi: 10.1126/science.1152509
Houk, P., Camacho, R., Johnson, S., McLean, M., Maxin, S., Anson, J., et al. (2015). The micronesia challenge: assessing the relative contribution of stressors on coral reefs to facilitate science-to-management feedback. PLoS One 10:e0130823. doi: 10.1371/journal.pone.0130823
Hughes, T. P., Anderson, K. D., Connolly, S. R., Heron, S. F., Kerry, J. T., Lough, J. M., et al. (2018). Spatial and temporal patterns of mass bleaching of corals in the Anthropocene. Science 359, 80–83. doi: 10.1126/science.aan8048
Hughes, T. P., Baird, A. H., Dinsdale, E. A., Moltschaniwskyj, N. A., Pratchett, M. S., Tanner, J. E., et al. (1999). Patterns of recruitment and abundance of corals along the Great Barrier Reef. Nature 397, 59–63. doi: 10.1038/16237
Huston, M. A. (1985). Patterns of species diversity on coral reefs. Annu. Rev. Ecol. Syst. 16, 149–177. doi: 10.1146/annurev.es.16.110185.001053
IPCC (2019). “Summary for policymakers,” in IPCC Special Report on the Ocean and Cryosphere in a Changing Climate, eds H.-O. Pörtner, D. C. Roberts, V. Masson-Delmotte, P. Zhai, M. Tignor, E. Poloczanska, et al. (Geneva: IPCC).
Jouffray, J.-B., Nyström, M., Norström, A. V., Williams, I. D., Wedding, L. M., Kittinger, J. N., et al. (2015). Identifying multiple coral reef regimes and their drivers across the Hawaiian archipelago. Philos. Trans. R. Soc. B Biol. Sci. 370:20130268. doi: 10.1098/rstb.2013.0268
Jouffray, J.-B., Wedding, L. M., Norström, A. V., Donovan, M. K., Williams, G. J., Crowder, L. B., et al. (2019). Parsing human and biophysical drivers of coral reef regimes. Philos. Trans. R. Soc. B Biol. Sci. 286:20182544. doi: 10.1098/rspb.2018.2544
Knapp, K. R., Applequist, S., Howard, J. D., Kossin, J. P., Kruk, M., and Schreck, C. (2010a). NCDC International Best Track Archive for Climate Stewardship (IBTrACS) Project, Version 3. Silver Spring, MD: NOAA National Centers for Environmental Information. doi: 10.7289/V5NK3BZP
Knapp, K. R., Kruk, M. C., Levinson, D. H., Diamond, H. J., and Neumann, C. J. (2010b). The international best track archive for climate stewardship (IBTrACS). Bull. Amer. Meteor. Soc. 91, 363–376. doi: 10.1175/2009BAMS2755.1
Kulbicki, M. (1998). How the acquired behaviour of commercial reef fishes may influence the results obtained from visual censuses. J. Exp. Marine Biol. Ecol. 222, 11–30. doi: 10.1016/S0022-0981(97)00133-0
Kulbicki, M., Guillemot, N., and Amand, M. (2005). A general approach to length-weight relationships for New Caledonian lagoon fishes. Cybium 29, 235–252.
Labrosse, P., Kulbicki, M., and Ferraris, J. (2002). ). Underwater Visual Fish Census Surveys: Proper use and Implementation. Noumea, New Caledonia: Secretariat of the Pacific Community (SPC).
Léopold, M., Guillemot, N., Rocklin, D., and Chen, C. (2014). A framework for mapping small-scale coastal fisheries using fishers’ knowledge. ICES J. Mar. Sci. 71, 1781–1792. doi: 10.1093/icesjms/fst204
Lugo, A. E., Rogers, C. S., and Nixon, S. W. (2000). Hurricanes, coral reefs and rainforests: resistance, ruin and recovery in the caribbean. AMBIO J. Hum. Environ. 29, 106–114. doi: 10.1579/0044-7447-29.2.106
MacNeil, M. A., Mellin, C., Matthews, S., Wolff, N. H., McClanahan, T. R., Devlin, M., et al. (2019). Water quality mediates resilience on the Great Barrier Reef. Nature Ecol. Evol. 3, 620–627. doi: 10.1038/s41559-019-0832-3
Maire, E., Cinner, J., Velez, L., Huchery, C., Mora, C., D’agata, S., et al. (2016). How accessible are coral reefs to people? A global assessment based on travel time. Ecol. Lett. 19, 351–360. doi: 10.1111/ele.12577
Manzello, D. P., Brandt, M., Smith, T. B., Lirman, D., Hendee, J. C., and Nemeth, R. S. (2007). Hurricanes benefit bleached corals. Proc. Natl. Acad. Sci. U.S.A. 104, 12035–12039. doi: 10.1073/pnas.0701194104
McWilliam, M., Pratchett, M. S., Hoogenboom, M. O., and Hughes, T. P. (2020). Deficits in functional trait diversity following recovery on coral reefs. Philos. Trans. R. Soc. B Biol. Sci. 287:20192628. doi: 10.1098/rspb.2019.2628
Mellin, C., Matthews, S., Anthony, K. R. N., Brown, S. C., Caley, M. J., Johns, K. A., et al. (2019). Spatial resilience of the Great Barrier Reef under cumulative disturbance impacts. Glob. Change Biol. 25, 2431–2445. doi: 10.1111/gcb.14625
Moberg, F., and Folke, C. (1999). Ecological goods and services of coral reef ecosystems. Ecol. Econ. 29, 215–233. doi: 10.1016/S0921-8009(99)00009-9
Morgan, K. M., Perry, C. T., Smithers, S. G., Johnson, J. A., and Daniell, J. J. (2016). Evidence of extensive reef development and high coral cover in nearshore environments: implications for understanding coral adaptation in turbid settings. Sci. Rep. 6:29616. doi: 10.1038/srep29616
Morse, N., Pellissier, P., Cianciola, E., Brereton, R., Sullivan, M., Shonka, N., et al. (2014). Novel ecosystems in the Anthropocene: a revision of the novel ecosystem concept for pragmatic applications. Ecol. Soc. 19:12. doi: 10.5751/ES-06192-190212
Mumby, P. J. (2017). Embracing a world of subtlety and nuance on coral reefs. Coral Reefs 36, 1003–1011. doi: 10.1007/s00338-017-1591-8
Norström, A. V., Nyström, M., Jouffray, J.-B., Folke, C., Graham, N. A., Moberg, F., et al. (2016). Guiding coral reef futures in the Anthropocene. Front. Ecol. Environ. 14:490–498. doi: 10.1002/fee.1427
Nyström, M. (2006). Redundancy and response diversity of functional groups: implications for the resilience of coral reefs. ambi 35, 30–35. doi: 10.1579/0044-7447-35.1.30
Pinca, S., Kronen, M., Friedman, K., Magron, F., Chapman, L., Tardy, E., et al. (2010). Regional Assessment Report: Profiles and Results from Survey Work at 63 Sites across 17 Pacific Island Countries and Territories. Noumea: Pacific Regional Oceanic and Coastal Fisheries Development Programme (PROCFish/C/CoFish).
QGIS Development Team (2019). QGIS Geographic Information System. Open Source Geospatial Foundation Project. Available online at: http://qgis.osgeo.org (accessed August 21, 2020).
R Development Core Team (2019). R: A Language and Environment for Statistical Computing. Vienna: R Foundation for Statistical Computing.
Riegl, B., Berumen, M., and Bruckner, A. (2013). Coral population trajectories, increased disturbance and management intervention: a sensitivity analysis. Ecol. Evol. 3, 1050–1064. doi: 10.1002/ece3.519
Robinson, J. P. W., Williams, I. D., Yeager, L. A., McPherson, J. M., Clark, J., Oliver, T. A., et al. (2018). Environmental conditions and herbivore biomass determine coral reef benthic community composition: implications for quantitative baselines. Coral Reefs 37, 1157–1168. doi: 10.1007/s00338-018-01737-w
Roff, G., and Mumby, P. J. (2012). Global disparity in the resilience of coral reefs. Trends Ecol. Evol. 27, 404–413. doi: 10.1016/j.tree.2012.04.007
Rogers, A., Blanchard, J. L., and Mumby, P. J. (2018). Fisheries productivity under progressive coral reef degradation. J. Appl. Ecol. 55, 1041–1049. doi: 10.1111/1365-2664.13051
Rogers, C. (1990). Responses of coral reefs and reef organisms to sedimentation. Mar. Ecol. Prog. Ser. 62, 185–202. doi: 10.3354/meps062185
Samoilys, M. A., Halford, A., and Osuka, K. (2019). Disentangling drivers of the abundance of coral reef fishes in the Western Indian Ocean. Ecol. Evol. 9, 4149–4167. doi: 10.1002/ece3.5044
Sandin, S. A., Smith, J. E., DeMartini, E. E., Dinsdale, E. A., Donner, S. D., Friedlander, A. M., et al. (2008). Baselines and degradation of coral reefs in the Northern Line Islands. PLoS One 3:e1548. doi: 10.1371/journal.pone.0001548
Schoepf, V., Stat, M., Falter, J. L., and McCulloch, M. T. (2015). Limits to the thermal tolerance of corals adapted to a highly fluctuating, naturally extreme temperature environment. Sci. Rep. 5:17639. doi: 10.1038/srep17639
Sheppard, C., Harris, A., and Sheppard, A. L. S. (2008). Archipelago-wide coral recovery patterns since 1998 in the Chagos Archipelago, central Indian Ocean. Mar. Ecol. Prog. Ser. 362, 109–117. doi: 10.3354/meps07436
Smith, J. E., Brainard, R., Carter, A., Grillo, S., Edwards, C., Harris, J., et al. (2016). Re-evaluating the health of coral reef communities: baselines and evidence for human impacts across the central Pacific. Proc. R. Soc. B 283:20151985. doi: 10.1098/rspb.2015.1985
Steffen, W., Crutzen, P. J., and McNeill, J. R. (2007). The Anthropocene: are humans now overwhelming the great forces of nature? Ambio 36, 614–621. doi: 10.2307/25547826
Taylor, A. H., Trouet, V., Skinner, C. N., and Stephens, S. (2016). Socioecological transitions trigger fire regime shifts and modulate fire–climate interactions in the Sierra Nevada, USA, 1600–2015 CE. Proc. Natl. Acad. Sci. U.S.A. 113, 13684–13689. doi: 10.1073/pnas.1609775113
Tebbett, S. B., and Bellwood, D. R. (2019). Algal turf sediments on coral reefs: what’s known and what’s next. Mar. Pollut. Bull. 149:110542. doi: 10.1016/j.marpolbul.2019.110542
van Hooidonk, R., Maynard, J., Tamelander, J., Gove, J., Ahmadia, G., Raymundo, L., et al. (2016). Local-scale projections of coral reef futures and implications of the Paris Agreement. Sci. Rep. 6:39666. doi: 10.1038/srep39666
van Woesik, R., Sakai, K., Ganase, A., and Loya, Y. (2011). Revisiting the winners and the losers a decade after coral bleaching. Mar. Ecol. Prog. Ser. 434, 67–76. doi: 10.3354/meps09203
Warton, D. I., and Hui, F. K. C. (2011). The arcsine is asinine: the analysis of proportions in ecology. Ecology 92, 3–10. doi: 10.1890/10-0340.1
Wiedenmann, J., D’Angelo, C., Smith, E. G., Hunt, A. N., Legiret, F.-E., Postle, A. D., et al. (2013). Nutrient enrichment can increase the susceptibility of reef corals to bleaching. Nature Clim. Change 3, 160–164. doi: 10.1038/nclimate1661
Williams, G. J., Gove, J. M., Eynaud, Y., Zgliczynski, B. J., and Sandin, S. A. (2015). Local human impacts decouple natural biophysical relationships on Pacific coral reefs. Ecography 38, 751–761. doi: 10.1111/ecog.01353
Williams, G. J., Graham, N. A. J., Jouffray, J.-B., Norström, A. V., Nyström, M., Gove, J. M., et al. (2019). Coral reef ecology in the Anthropocene. Funct. Ecol. 33, 1014–1022. doi: 10.1111/1365-2435.13290
Williams, G. J., Smith, J. E., Conklin, E. J., Gove, J. M., Sala, E., and Sandin, S. A. (2013). Benthic communities at two remote Pacific coral reefs: effects of reef habitat, depth, and wave energy gradients on spatial patterns. PeerJ 1:e81. doi: 10.7717/peerj.81
Williams, I. D., Baum, J. K., Heenan, A., Hanson, K. M., Nadon, M. O., and Brainard, R. E. (2015). Human, oceanographic and habitat drivers of central and Western pacific coral reef fish assemblages. PLoS One 10:e0120516. doi: 10.1371/journal.pone.0120516
Wood, S., and Scheipl, F. (2014). gamm4: Generalized Additive Mixed Models Using mgcv and lme4. R Package Version 0.2–3. Available online at: https://cran.r-project.org/package=gamm4
Woodhead, A. J., Hicks, C. C., Norström, A. V., Williams, G. J., and Graham, N. A. J. (2019). Coral reef ecosystem services in the Anthropocene. Funct. Ecol. 33, 1023–1034. doi: 10.1111/1365-2435.13331
Zhang, S. Y., Speare, K. E., Long, Z. T., McKeever, K. A., Gyoerkoe, M., Ramus, A. P., et al. (2014). Is coral richness related to community resistance to and recovery from disturbance? PeerJ 2:e308. doi: 10.7717/peerj.308
Keywords: climate change, reef degradation, ecological reorganisation, ecological homogenisation, generalised additive models, model selection
Citation: Ford AK, Jouffray J-B, Norström AV, Moore BR, Nugues MM, Williams GJ, Bejarano S, Magron F, Wild C and Ferse SCA (2020) Local Human Impacts Disrupt Relationships Between Benthic Reef Assemblages and Environmental Predictors. Front. Mar. Sci. 7:571115. doi: 10.3389/fmars.2020.571115
Received: 09 June 2020; Accepted: 31 August 2020;
Published: 21 October 2020.
Edited by:
Cristina Linares, University of Barcelona, SpainReviewed by:
Kirsten L. L. Oleson, University of Hawai‘i at Mānoa, United StatesNadine Schubert, University of Algarve, Portugal
Copyright © 2020 Ford, Jouffray, Norström, Moore, Nugues, Williams, Bejarano, Magron, Wild and Ferse. This is an open-access article distributed under the terms of the Creative Commons Attribution License (CC BY). The use, distribution or reproduction in other forums is permitted, provided the original author(s) and the copyright owner(s) are credited and that the original publication in this journal is cited, in accordance with accepted academic practice. No use, distribution or reproduction is permitted which does not comply with these terms.
*Correspondence: Amanda K. Ford, amandakford@hotmail.com