- 1Monterey Bay Aquarium, Monterey, CA, United States
- 2Nicholas School of the Environment and Earth Sciences, Duke University, Durham, NC, United States
Southern sea otters (Enhydra lutris nereis) are currently >80% depleted with respect to both abundance and range occupancy baselines, challenging the long-term persistence of the species and the ecosystem benefits their populations might provide. From 2001 to 2018, the Monterey Bay Aquarium rescued stranded sea otter pups and reared them in captivity through a surrogacy program using non-releasable adult females. We gave 11,396 days of captive care to 56 otters, reintroduced them into the wild, and observed them over 894 total field days after release. This study describes the post-release movements of the 42 successfully released otters, quantifying their dispersal patterns and modeling environmental, demographic, and animal care influences through a machine learning framework. This random forest model specifically considers predictor variable correlation, accounts for individual and joint variable impacts, and evaluates robustness through sensitivity analyses. Heavy tailed dispersal models best explained the (n = 641) daily movements of surrogate-reared otters, and the random forest outputs ranked population demography, population growth, and El Niño most significantly. Occasionally aided by recaptures, the scale of dispersals consistently declined after release, indicating successfully released otters stabilized their movements within 3 weeks in the wild. Our results show dispersal is an important metric for measuring the success of sea otter releases and suggest environmental factors (including climate) at release sites may determine the success of reintroduction programs.
Introduction
Overexploitation during the international fur trade nearly drove the southern sea otter (Enhydra lutris nereis) to extinction in California. Hunting shrunk the population 99.8% from a statewide distribution estimated at 16,000 individuals to a single location with less than 50 otters, circa 1930 (Laidre et al., 2001; Kittinger et al., 2015). As a result of conservation management, the population has now rebounded to 2,962 individuals (Hatfield et al., 2019). While this observed recovery is encouraging, the population remains well below historical baselines. Sea otters today are estimated at <19% of their historical abundance in California, and population growth has slowed in recent years (Tinker and Hatfield, 2017; Hatfield et al., 2019). The population is now constrained to 15% of its historical range, with no significant expansion in the last 20 years (USFWS, 2015; Nicholson et al., 2018). In contrast, from 1960 to 2011 the sea otter population in southeast Alaska, though exposed to different geographic constraints to population growth, increased from 400 to 25,584 individuals (Tinker et al., 2019).
Southern sea otters are currently listed as “threatened” under the U.S. Endangered Species Act (USFWS, 2003). Risks to the continued recovery of this species stem primarily from its constrained distribution, which makes the population highly vulnerable to acute events (e.g., oil spills; USFWS, 2003) and limits the population to the carrying capacity of relatively few habitats (Tinker et al., 2013; Tinker and Hatfield, 2017). Kelp forests at the range peripheries are comparably sparse to the range center (Nicholson et al., 2018), and mortalities in these regions are dominated by white shark bites (Moxley et al., 2019). These mortalities disproportionately impact juveniles and males (Moxley et al., 2019), the demographic that typically pioneers range expansion (Lafferty and Tinker, 2014). Though the population has increased in the past, these range peripheries are losing otters (Tinker and Hatfield, 2018), and the population has declined each of the past 3 years (Hatfield et al., 2019). With limited resources throughout the range center (Tinker et al., 2008; Tinker et al., 2013), increased shark bite mortalities at the range peripheries (Tinker et al., 2016; Moxley et al., 2019), and the ongoing threats from disease, management interventions have been suggested to achieve the recovery goals defined in the federal species recovery plan (USFWS, 2003). Such interventions (e.g., reintroductions) are timely as El Niño events and a persistent marine heatwave (Di Lorenzo and Mantua, 2016) have drastically altered coastal California ecosystems and reduced kelp forest ecosystems to historical lows (Burt et al., 2018; Nicholson et al., 2018).
The Monterey Bay Aquarium (“MBA”) began rehabilitating stranded southern sea otter pups in 1984. Stranding of newborn pups occurs as a result of premature separation from the mother, though pups often strand with minimal or no injury to themselves. Consequently, orphaned pups present good candidates for reintroduction, but require prolonged captive rearing. In 2001, MBA transitioned from human-rearing to raising stranded pups using resident adult females as surrogate mothers, thus reducing exposure to humans and leading to improved reintroduction success into wild habitats (Nicholson et al., 2007; Mayer et al., 2020). Throughout the duration of this surrogacy program, captive-reared pups have been released in the greater Monterey Bay region, either in giant kelp (Macrocystis pyrifera) forests around the Monterey peninsula or more frequently in an eelgrass (Zostera marina) estuary in Elkhorn Slough. Both ecosystems are within the center of the population’s current distribution (Nicholson et al., 2018).
As a result of reintroductions, sea otter population densities in Elkhorn Slough increased and the small, local population began reproducing (Mayer et al., 2020). While these changes are viewed as positive for the larger objective of sea otter population recovery and for ecosystem restoration, sea otter foraging efficiency and prey size have decreased in this local population (Tinker and Hatfield, 2018). This has fed management concerns that Elkhorn Slough may be near capacity, and as a result, the reintroduction program is now focused on other estuary ecosystems with low-density populations. During this period, overall ecosystem health has improved in Elkhorn Slough, particularly the extent and abundance of eelgrass and water quality (Hughes et al., 2013). Sea otters are widely noted for their keystone role in kelp forests and other coastal habitats by controlling grazer populations (Estes and Palmisano, 1974; Estes and Duggins, 1995; Moxley et al., 2019) and appear to impact eelgrass communities by reducing the negative impacts of agricultural nutrient loading via initiating trophic cascades within the system (Hughes et al., 2013). Such ecosystem effects recursively improve degraded sea otter habitats which may aid in reestablishing and repopulating currently unoccupied habitats outside the current population range (Pace et al., 1999; Nicholson et al., 2018).
For sea otter reintroductions to achieve local ecosystem impacts, released animals must survive the transition from captivity to the wild (Rathbun et al., 2000; Tweed et al., 2003; van Heezik et al., 2009; Van Houtan et al., 2009). The early phase of this transition, when ecologically naïve individuals first experience novel surroundings, is often characterized by sporadic and long-distance movements. These long-distance movements may jeopardize survival by increasing metabolic demands, accelerating weight loss, intensifying risk of fatal white shark bites, and detracting from opportunities to forage, groom, or socialize at the release site (Zollner and Lima, 2005; Yeates et al., 2007; Moxley et al., 2019; Mayer et al., 2020). From a practical perspective, these movements also impede monitoring, and sometimes necessary intervention by researchers, which are both critical for evaluating and improving program success (Le Gouar et al., 2008; van Heezik et al., 2009; Adimey et al., 2016; Mayer et al., 2020). Although most individuals (34/42, 81%) who successfully transition to the wild also settle near release sites (<10 km), identifying factors that influence initial post-release movements are important to advance the efficiency and effectiveness of reintroduction programs. Understanding initial dispersal drivers may in turn aid management goals targeted at southern sea otter population recovery and restore degraded coastal ecosystems throughout the species historical range.
Materials and Methods
Sea Otter Stranding, Surrogacy, and Post-release Tracking
From 2001 to 2018, MBA staff responded to live sea otter strandings along the California coast. For the subset of cases involving dependent pups (<9 weeks old sensu Nicholson et al., 2020), MBA staff first attempted to reunite pups with their biological mothers. If this was unsuccessful, the pups were brought to MBA, assessed by a veterinarian and treated for hypothermia, dehydration, hypoglycemia, or other conditions. After several weeks of intensive care, at 8–10 weeks of age, we introduced each pup to a resident adult female sea otter to bond with and rear as her own (Nicholson et al., 2007). At 6–8 months of age, after weaning from the adoptive mother and demonstrating proficient foraging behaviors, we fitted surrogate-reared otters with color-coded flipper tags (Temple Tags, cattle ear tags) and implanted VHF radio transmitters (Advanced Telemetry Systems, model no. MM510B). Following several weeks of post-surgery supervision, we released surrogate-reared otters in Elkhorn Slough (n = 36; 2002–2017) or around the Monterey peninsula (n = 6; 2017–2018).
MBA staff and volunteers conducted daily surveys to monitor otters during the first month after release. We searched for otters from land and by boat, using VHF radio receivers (Communications Specialists Inc., R1000) with handheld directional antennae (AF Antronics, three-element folding Yagi). If unable to readily locate, a pilot (Ecoscan Resource Data) flew aerial surveys, applying a search pattern specific to an individual otter’s last known location and behavior. Once located, observers recorded the otter’s coordinates on a tablet computer and later transferred the information to a relational database. Trackers then closely monitored foraging and resting behavior to assess whether the individual was successfully transitioning to the wild (Fujii et al., in preparation), as sea otter metabolism demands productive foraging and ample rest (Ralls and Siniff, 1990; Thometz et al., 2014), unsuccessful foraging can rapidly deplete energy reserves and lead to starvation (Nicholson et al., 2018). Using spotting scopes and binoculars, observers documented distance traveled, activity budgets, prey composition, and body condition. Individuals struggling to adapt to their surroundings and exhibiting signs of stress post release (Nicholson et al., 2007) were recaptured, rehabilitated at MBA, and later re-released. A release was considered successful if after 2 weeks of monitoring, the otter was observed successfully foraging, maintaining body mass, appropriately avoiding humans, and not exhibiting overt stress behaviors.
We analyzed tracks from 42 otters reared by surrogate mothers at MBA, released, and successfully incorporated into the wild population (Nicholson et al., 2007; Mayer et al., 2020). An additional 14 otters passed through the MBA surrogacy program, and had unsuccessful releases (75% success rate, 42/56 otters); the fate of these otters included mortality (n = 9), disappearance offshore (n = 4), or transfer to accredited Association of Zoos and Aquariums facility for permanent residency (n = 1), following an “un-releasable” determination by the United States Fish & Wildlife Service (“USFWS”). Causes of mortality included starvation, disease, or wounds from other otters. For these unsuccessful releases, we had insufficient movement data (too few locations) to properly fit dispersal kernels, and therefore excluded them from our analysis.
Least Cost Paths and Dispersal Kernels
From tracking data, we calculated least cost path (“LCP”) distance between sightings spanning a 24-h period (n = 481) to generate an estimate of a sea otter’s daily distances traveled. When hampered by poor weather or other logistical challenges, we supplemented daily resights with LCP measurements representing >24-h intervals (n = 85, 13%). We also included resights with greater time spans near the end of monitoring (n = 75, 12%) after individuals had settled within a geographic area. To prevent direct as-the-crow-flies travel over land, we generated transition matrices from bathymetry and topographic data (Weatherall et al., 2015) to constrain paths to cells with <1 meter elevation. As a large portion of the area monitored during this study was within a coastal estuary, this routine accommodated the frequent intertidal habitat use that we observed. When subsequent resights were within the same 100 m transition matrix cell (4.7% resights, 30/641), we calculated a straight-line distance. All transition matrices and LCP distances were calculated using the R packages “gdistance” (Van Etten, 2017), and “raster” (Hijmans, 2017). The output from this model consisted of a chronological series of cell centroid locations and distance calculations along that path.
LCPs between consecutive sightings (day 1–2, 2–3, 3–4… n–n + 1) described daily movements which we formally analyze using dispersal kernels. For visual inspection, we plotted histograms of the daily LCP travel distances for each otter. Noticing many of the movement histograms appeared to be statistically “heavy tailed” (Atkinson et al., 2002; Reynolds and Frye, 2007; Van Houtan et al., 2007), we used the “fitdistr” function in the “MASS” package (Venables and Ripley, 2002; Ripley et al., 2020) to fit Cauchy models to the movement data for each individual. The Cauchy model is a special case of the log-Sech function (Van Houtan et al., 2007; Nathan et al., 2012) and uniquely and practically captures both near and far movements. Following previous approaches (Van Houtan et al., 2007, 2010) we also fit each otter’s movement data to Rayleigh and Gamma dispersal kernels, using the log-likelihood (LL) functions to perform maximum likelihood estimation. The corrected Akaike Information Criterion for small populations (“AICc,” Hurvich and Tsai, 1989) ranked the performance of the Cauchy, Gamma, and Rayleigh kernels facilitated by the best-fit LLs of each function.
Though movement data represent a univariate quantity (travel distance), they are derived from the bivariate coordinate (x, y) data obtained from resight locations. Therefore, the various dispersal kernels compared here represent different mechanistic families or types of two-dimensional spatial movements. The Rayleigh distribution represents Gaussian movements approximating a random walk, the Gamma distribution describes directional movement (akin to a negative exponential), where the Cauchy distribution describes a combination of short-distance or localized, as well as, long-distance movements sometimes referred to as “Lévy-flight” (Van Houtan et al., 2007, 2010). Due to its performance (see below) we extracted the scale parameter from the best-fit Cauchy model describing each individual’s post-release movements as a standardized metric to compare the initial dispersal behavior of all otters.
Modeling Drivers of Post-release Dispersal
To understand post-release movements over the study, we fit a locally weighted regression (“LOESS”) (Cleveland et al., 1988) to individual scale parameters across time. Besides being widely applied to describe non-parametric and non-linear ecological trends, the LOESS approach has value here to capture trends that may integrate environmental phenomena over time (e.g., Gagné et al., 2018b). During visual inspection, we chose a loess span of 0.6, which represented longer-term trends in dispersal without over-smoothing. Next, we selected several demographic, animal care, and environmental (including population) variables to model as drivers of post-release dispersal, defined by raw and LOESS Cauchy scale parameters. We describe below our suite of predictors and process for building and evaluating performance of our final model.
Morphometric and demographic data included individual otter body condition (ln-length/ln-weight) before release (Monson et al., 2000; Laidre et al., 2006), age at stranding, age at release, and sex. Animal care variables include number of days in the surrogacy program (release date minus stranding date), stranding and release locations, release date, surrogate mother, percent diet composed of live prey (<30 days prior to release), and the number of recaptures before successful release.
We accessed several relevant environmental variables derived using sea surface temperature (“SST”) from 2002 to 2018. We calculated the SST daily mean of hourly observations from NOAA Station #EVMC1 and #46042, located at Elkhorn Slough (36.811 N, 121.779 W) and in Monterey Bay (36.785 N, 122.398 W), respectively. Monthly El Niño conditions were described by the rank order of the multivariate ENSO index (“MEI”; Wolter and Timlin, 1993) time series. We also examined the monthly Pacific Decadal Oscillation index (“PDO”; Mantua and Hare, 2002). These metrics are established indices that characterize both regional ocean conditions and ecosystem state (Dayton and Tegner, 1990; Edwards, 2004; Lluch-Belda et al., 2005). We consider them as high resolution indicators of habitat and prey abundance not available locally. Furthermore, though particular sites within Elkhorn Slough may vary from reported values, and as released otters used habitats throughout Monterey Bay (Lindsey, 2016; see Supplementary Figure S1), these stations serve to summarize the local conditions as best as possible. Consistent with Cauchy scale smoothing, we fit a LOESS (span 0.6) to each time series and extracted the predicted values to represent longer-term environmental trends or cycles in prey resources. We added daily wind speed (Breed et al., 2017) and wave height (NOAA Station 46042, Monterey Bay, 36.785°N 122.398°W) to the model, but ultimately excluded them as they decreased model performance (see Supplementary Figure S4).
In addition to climatic variables, we include three population metrics derived from annual, range wide monitoring of the sea otter population. From these data, we used the population density, pup ratio (or the proportion of pups in the population), and 5-year trend statistics, corresponding to the year and specific location where each individual was released. Nearshore locations correspond to linear 500 m shoreline segments along the 5-fathom depth contour (extending seaward from 0 to 30 m depth). An inshore segment is separately and specifically defined for Elkhorn Slough. For the Monterey Peninsula, we calculated the mean parameter values across its shoreline segments to provide a single metric for this location. As per the standard indexing method for these data (Hatfield et al., 2019), annual sea otter counts within segments are smoothed as a 3-year average spatially across 10 km (equal to 21, 500 m bins). These smoothed counts are used to calculate population statistics within each discrete segment. We estimate the population trend by fitting a log-linear model to a 5-year time series at each 500 m segment or location (Hatfield et al., 2019). For missing data resulting from an incomplete survey in 2011, we interpolated population density and growth estimates by calculating the mean between nearest neighbor years (2010 and 2012).
Following similar studies that rank the influence of multiple potential drivers to the ecology of marine organisms (Gagné et al., 2018a,b; Becker et al., 2019), we used random forest models (“RF”; Liaw and Wiener, 2002) to test the effects of individual demographics, surrogacy practices, and environmental conditions on the dispersal of immature sea otters during their first month after release. Among machine learning algorithms, RF is well designed to describe the non-linear forms that are common in ecological data (Breiman, 2001; Hastie et al., 2009). Specifically, the entire forest generates robust predictions from an ensemble of diversified and uncorrelated trees, each considering a random subset of features and bootstrap samples of training data assembled with replacement. As the forest grows, it provides an internal unbiased estimate of the generalized error, variable importance rank, and information necessary to explore modeled feature interactions (Breiman, 2001).
Initially, we built two models with all 18 variables, using leave-one-out cross validations (“LOOCV”) to assess model sensitivity (Gagné et al., 2018a,b; Becker et al., 2019) and examine variable importance, derived from their impact to mean squared error (“MSE”) when removed (see Supplementary Figure S3). To improve model performance, we eliminated highly correlated variables (r ≥ 0.6, Wei and Simko, 2017), tuned model parameters (“mtry” and “ntree”) using a simple grid search routine with our LOOCV analysis, selected the smoothed LOESS Cauchy scale parameter as our dispersal response, and ran a second RF series incorporating only the 3 variables that reduced overall MSE (see Supplementary Figure S3). We then used partial dependency plots to examine the interactive effects of the remaining variables on sea otter dispersal. To consider uncertainty in our fitted cauchy scale parameter, we examined model performance and factor rank effects from 100 different estimates for each individual. We derived these estimates by randomly sampling from a normal distribution defined by each otter’s scale parameter and sd, then calculating the smoothed value from each iteration’s ensemble. All analyses were conducted in version 4.0.0 of the R statistical environment (R Core Team, 2018).
Lastly, we conducted a simple post hoc analysis of how time in the wild influenced the movements of released otters. Instead of comparing movements among individuals, as described above, here we categorize daily movements of all released otters (n = 42), according to their time in the wild (1, 2, 3… n days). We then fit Cauchy models to the dispersal data for each of these days post release, for otters that were released only once (n = 24), and separately for otters requiring intervention (recaptured, given further rehabilitation, and then released, n = 18). As above, we extract the scale parameter from the best-fit Cauchy model and plot the values against the time since release (or cumulative total number of days) in the wild.
Results
Post-release Tracking and Dispersal
LCP segments between daily resights show distances traveled, both within and between individuals, are statistically heavy tailed (Figure 1A). For example, otters #228 and #526 were both released in Elkhorn Slough. Otter #526 only made local movements (scale = 0.17) and ultimately took up residency in the Slough, traveling a cumulative 13 km over 11 days. Otter #228 emigrated from the Slough and settled near Año Nuevo Island (scale = 1.6), traveling a total 72 km over 10 days (Figure 1B). The Cauchy dispersal model fit the LCP distances best, achieving the lowest AICc ranking in 31 of 42 individuals (74%) and having an average δAICc value of 2.1 (see Supplementary Table S1). By comparison, the Gamma function had an average δAICc of 22, and the Rayleigh average δAICc equaled 487. As a result, we extracted the Cauchy model scale parameters as a standard metric to describe dispersal for each otter. The scale parameter ranged from 0.1 to 6.2 (Figure 1A), with the LOESS model indicating a pattern of greater values, and therefore more long-distance and sporadic post-release movements, both early and late in the study (Figure 1C). Table 1 presents summary statistics of all the raw results and dispersal model fits. Supplementary Figure S1 maps the travel paths for all otters, beyond what is displayed in Figure 1B.
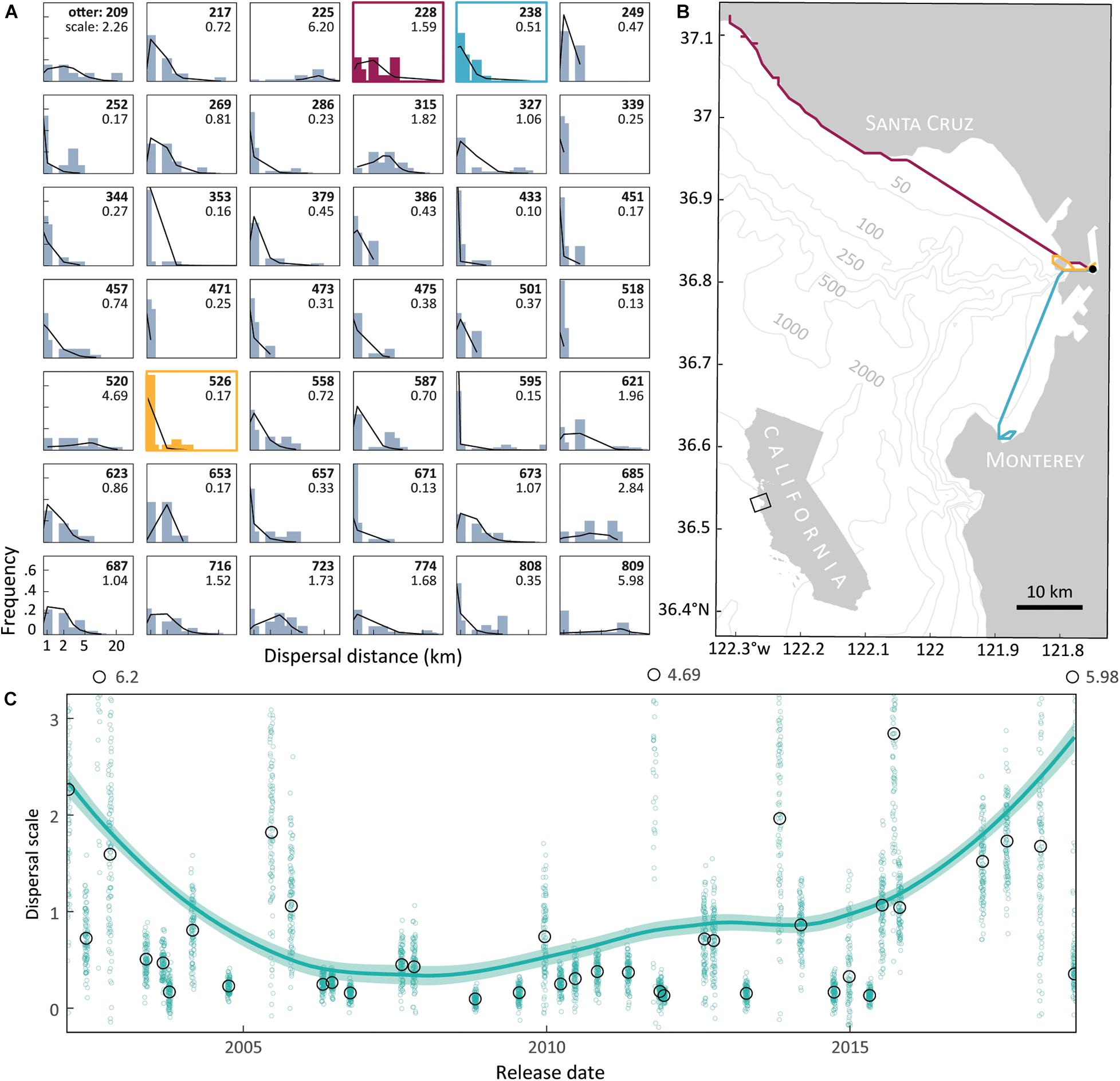
Figure 1. Surrogate-reared sea otters often moved long distances after release, especially in early and recent years. (A) Probability models fit to the dispersal frequency for 42 sea otters that were surrogate reared and then released into the wild. Movements reflect the least cost path (“LCP”) distance between observed daily resight locations, in the first month after release. Identification number and scale parameter from the best-fit Cauchy model (solid line) are labeled in each panel. This parameter is higher in animals that move greater distances. (B) Inferred daily travel routes for otters #228, #238, and #536 in Monterey Bay after their release in Elkhorn Slough estuary (filled circle). Path colors correspond to individual panels in (A), with isobaths provided. (C) Extracted scale parameter from each otter shows a pattern from 2005 to 2015 of short-distance movements, generally reflecting post-release residency in Elkhorn Slough. Black circles represent individual scale parameters (n = 42), and small green circles denote sample variation (n = 100 for each sea otter) inherent in this estimate. Solid line is the LOESS regression fit, shaded region is a 95% confidence interval.
Demographic, Animal Care, and Environmental Variables
Demographic, animal care (Figure 2) and environmental (Figure 3) factors all varied through time. The time in surrogacy (Figure 2A) and otter body condition (Figure 2D) steadily increased through the study. During this time, the live diet composition for surrogate-reared otters dropped from 80% to roughly 50% (Figure 2C). The sex ratio of surrogate-reared otters was roughly equivalent across all study years (Figure 2B) though males were more common at the outset (Figure 2A). The number of releases fluctuated, with more otters requiring recapture from 2005 to 2015 (Figure 2E).
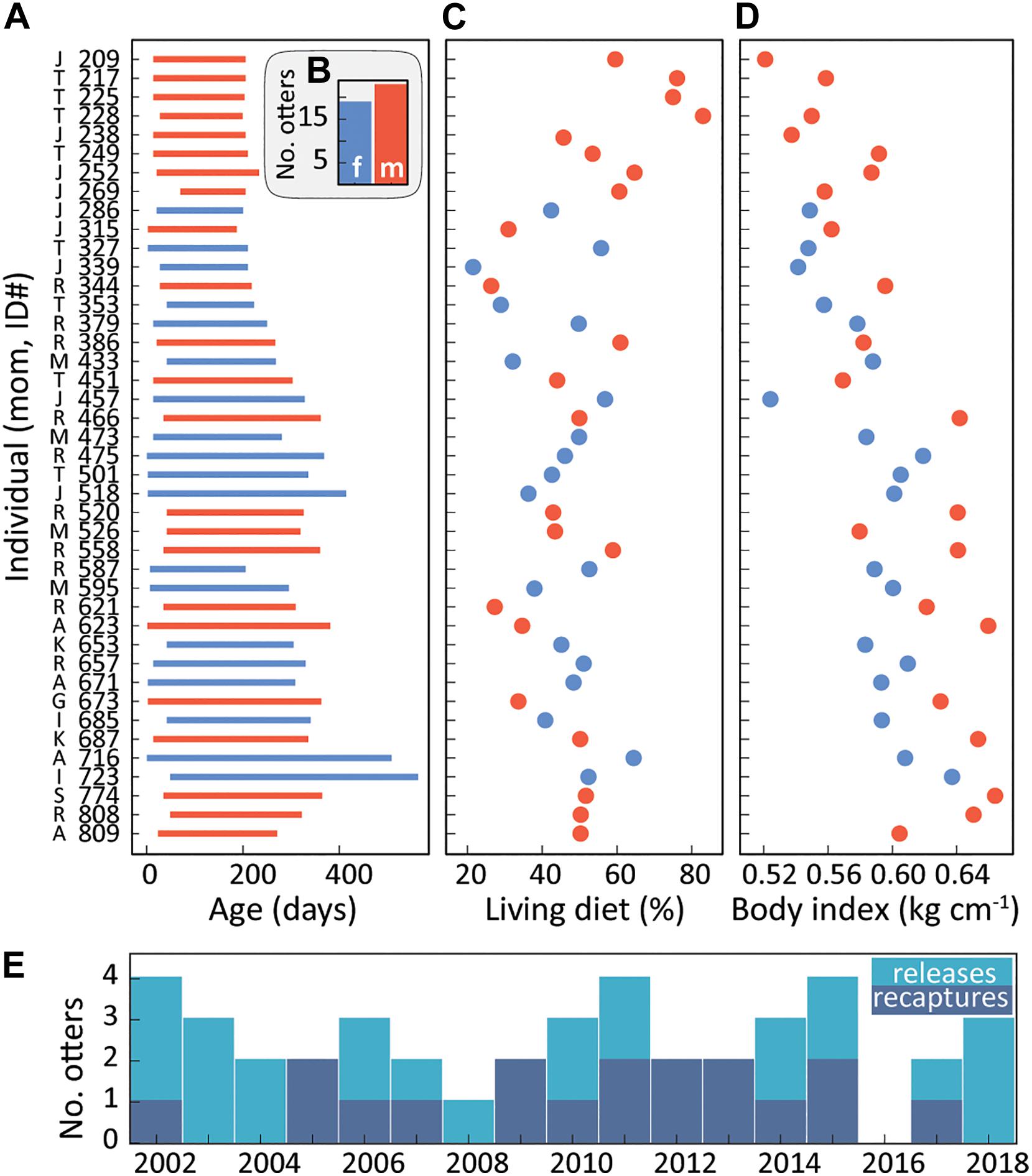
Figure 2. Key animal care metrics that may influence behavior after release. (A) Residency time in the Monterey Bay Aquarium surrogacy program, bounded by accession and release, increased over time. Y axis labels indicate surrogate mom, individual (see Figure 1A), and are constrained in (C,D). In (A–D) males are red while females are blue; (B) the cumulative sex ratio of released otters. (C) The portion of live prey in each otter’s diet in the month prior to release, potentially important for foraging success in the wild, decreased from roughly 80–50% over the study period. (D) A common index of body condition (log mass * log length– 1) recorded before release, steadily increased. (E) Eighteen of the 42 otters in this study were unsuccessful on the first release. As a result, these animals were recaptured, received additional treatment, and were subsequently re-released and successfully incorporated into the population. No otters had initial releases in 2016.
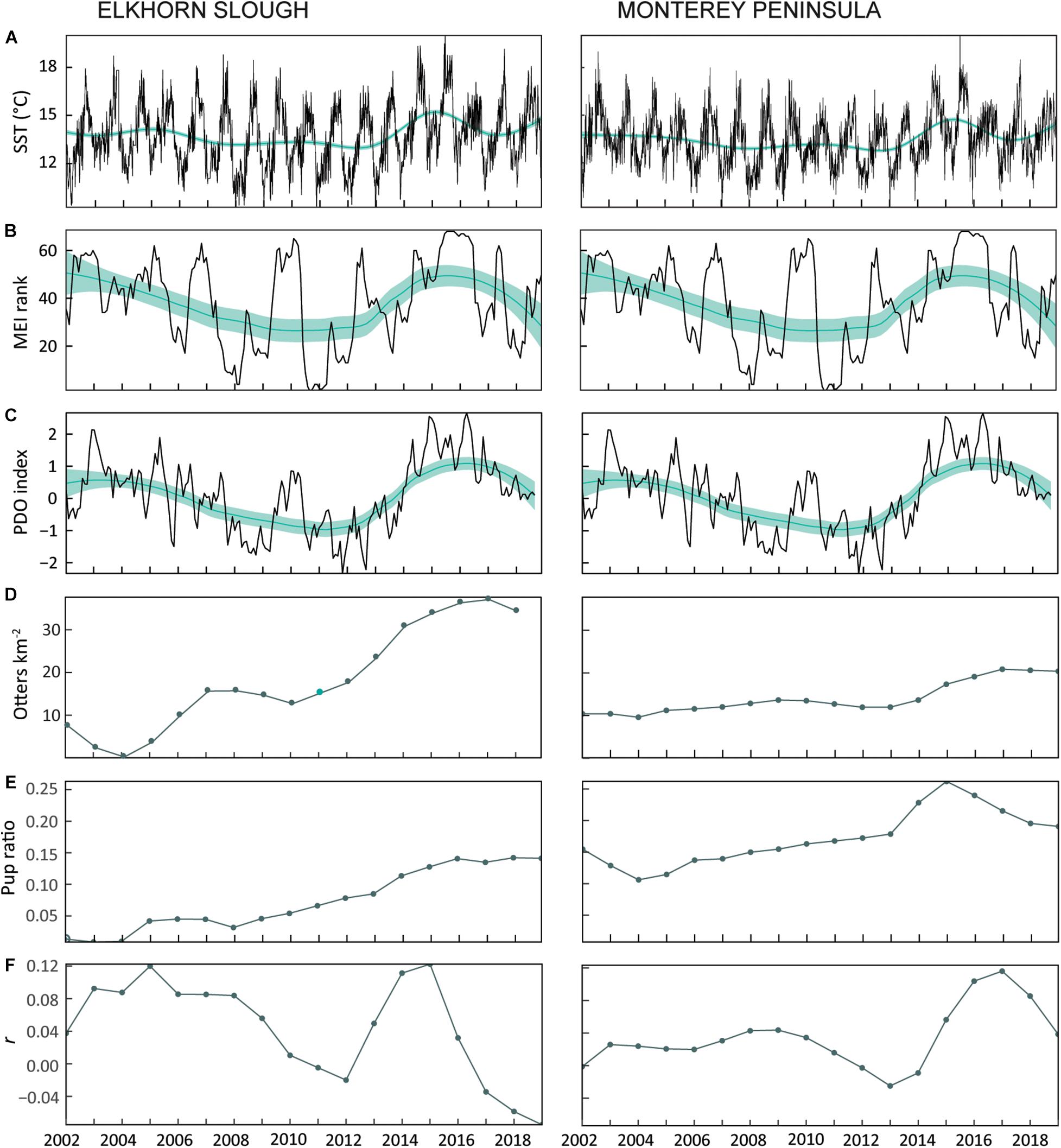
Figure 3. Environmental drivers that may influence post-release behavior. (A) Sea surface temperature (SST), (B) Multivariate ENSO index (MEI), and (C) Pacific Decadal Oscillation (PDO) show similar trends over time. Importantly, all reflect the marine heatwave event of 2014–2015. Black lines are the raw series, teal lines are a LOESS model, and shaded area is a 95% confidence interval. SST is recorded bi-hourly at NOAA stations EVMC1 (36.811 N, 121.779 W) and 46042 (36.785 N, 122.398 W) at the mouth of Elkhorn Slough and along Monterey Bay, respectively. MEI is the rank order of the monthly index, where El Niño conditions are highest and La Niña conditions lowest. Note that MEI rank and PDO index for Elkhorn Slough and Monterey Peninsula are identical because the distance between these two release locations (∼24 km) is smaller than the geographic resolution of these oceanographic parameters. (D) Surveyed sea otter population density and proportion of pups to total in Elkhorn Slough and along the Monterey Peninsula increased over the study period, while (E) population growth (r) fluctuated, showing similar trends as PDO and MEI. We used the mean of nearest neighbors to interpolate missing population density (D), pup ratio (E), and growth trend (F) values in 2011 (teal).
SST-based environmental indices showed similar temporal patterns (Figures 3A–C). The predicted LOESS values superimposed over the daily SST series indicate that temperatures peaked in 2015 and in general were higher before 2006 and after 2013, and lower between. LOESS predicted values across MEI (Figure 3B) and PDO (Figure 3C) showed similar broad trends, peaking in 2015–2016 and lowest from 2010 to 2011. There were no low MEI years or negative PDO cycles from 2014 to 2018, and consistently higher winter minimum and summer maximum temperatures. With similar features from these two indices across our time scale, during modeling we selected MEI rankings to represent oceanographic condition because ENSO events are known to have dramatic coastal influence along California. Local wild population density (Figure 3D) and pup production (Figure 3E) increased throughout the study, while population growth (Figure 3E) showed similar patterns to SST, MEI, and PDO.
Drivers of Post-release Dispersal
With satisfactory model performance (R2 = 0.87, mtry = 2, ntree = 1,500; Supplementary Figure S3), our final variables correspond well with post-release movements (Figure 4). Figure 4 plots the raw (Figure 4A) and modeled (Figure 4B) driver relationships to sea otter dispersal, as well as their interactions (Figure 4C). RF models indicate that pup ratio is the most influential variable (59% MSE increase), followed by MEI (37%), and population growth trends (21%). In both the raw and modeled relationships, dispersal increased with local pup production in the wild population. Two-way partial dependency plots (PDPs) show the effect of this factor is slightly diminished when MEI rank falls below 40 (Figure 4C) or population growth rate is above 0.08. These two factors exert opposing effects on dispersal. Regardless of MEI or local population growth rates, dispersal is highly probable when the proportion of pups to total sea otters within the release environment is greater than 0.14. When testing for effects of uncertainty introduced during scale parameter fitting, factor importance and model performance were consistent and robust (Figure 4D).
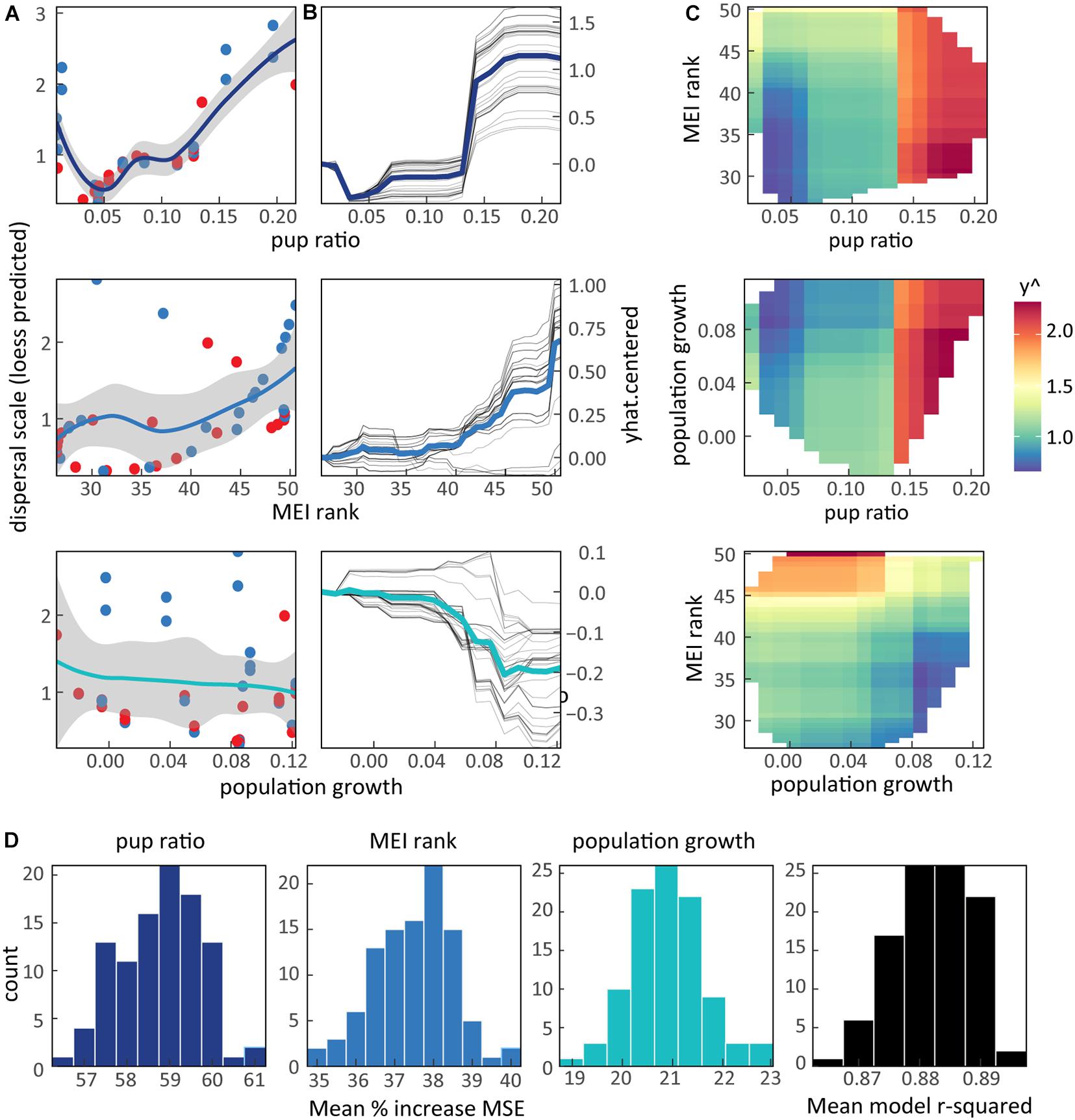
Figure 4. Environmental factors are the strongest driver of post-release dispersal. (A) Raw pair-wise comparisons, and (B) modeled individual conditional expectations (ICE) from the Random Forest model (RF) outputs for the highest ranked variables. High sea otter pup ratios within the local population and strong El Niño conditions correspond to more mobility after release. Wild population growth rate has the opposite relationship, where high r is linked to less post-release movements. (C) Two-way partial dependency plots show the interactions in the predicted impact of selected drivers on dispersal. Here dispersal increases with y^, symbolized with warm colors. Following (B), this shows that high pup ratios and MEI rank together confer the greatest movements. (D) Variable importance ranks determined by the comparative increase in MSE when each driver is removed from the model. Histograms describe variability in factor effects resulting from 100 model iterations, each drawing loess scale parameters derived from an ensemble of all study sea otters (n = 42) sampled using their individual Cauchy scale parameter and its sd. Bar colors in (D) retained from trend lines in (A,B). Overall, the RF model explains 87% of the variability in the data, and key model factors characterize social and environmental conditions at the release site.
Figure 5 shows the influence of time in the wild on the scale of dispersal for otters released once, and multiple times. Both series show the gradual decline of the dispersal scale parameter, over the first 2 weeks after release. Otters released just once (Figure 5A) have a gradual monotonic decline, indicating a stabilization in movements leading to residency. Otters exhibiting visible signs of stress or weight loss were recaptured, further rehabilitated, and then released again. Though they required intervention, these animals display a similar broad pattern (Figure 5B) as otters not requiring intervention, however, the series is noisier, and the scale does not decline until days 7–8. This period coincides with the first or second release during intervention and recapture.
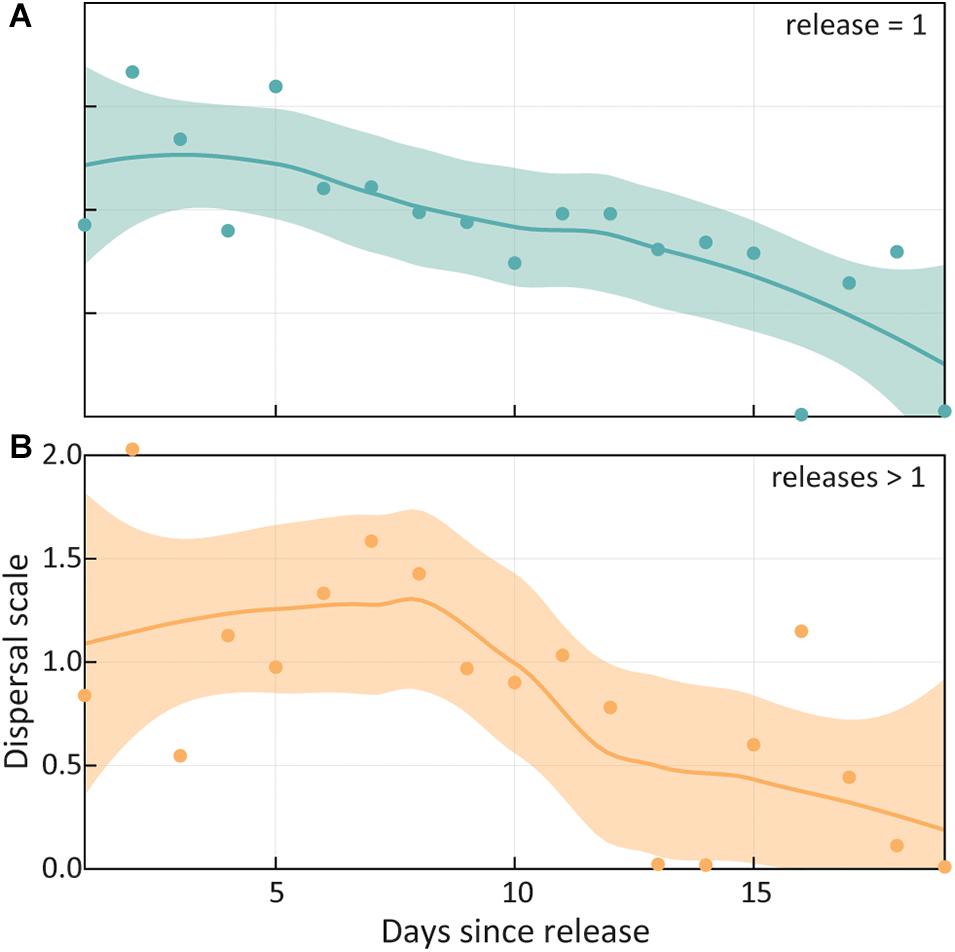
Figure 5. Dispersal scale declines as released otters acclimate to the wild environment. For sea otters (A) released once, as well as those (B) requiring recapture and re-release, the scale of movements declines over the first 3 weeks since release. Interventions for otters showing visible signs of stress after release typically occurs in their first week at liberty, and this may be reflected in the scale being constant in that period, then abruptly declining thereafter. However, both plots (with equivalent x and y axes) show the same general pattern of movement stabilization with ultimate residency (scale converging to <0.2) at the release site in a relatively short period.
Discussion
Understanding the patterns and drivers of sea otter movements after their reintroduction into the wild may be important for conservation programs aimed at expanding population and ecosystem recovery. Based on 16 years of data from 42 individuals that were surrogate reared in captivity, we found four main results. First, heavy-tailed models described sea otter movements best (Figure 1A), indicating otters dispersed near and far, and often both (Figure 1B). Second, long distance dispersals were most common early and late in the study (Figure 1C). Third, social and ecological factors at the release site (Figure 3) outweighed individual demographics and animal care variables (Figure 2) in predicting post-release movements (Figure 4D). Fourth, for individuals who exhibit high mobility after release, recapture may be an effective tool to interrupt and resolve this behavior (Figure 5).
Erratic dispersal behavior of newly released animals is common when returning naïve, rehabilitated marine mammals to the wild, but often released individuals settle into patterns typical of their wild cohorts (Moore et al., 2007). Sea otters primarily occupy small (<10 km2) home ranges within nearshore kelp forests and estuaries (Tinker et al., 2013, 2018). When young, individuals (particularly males) may demonstrate long-distance movements or exploratory behavior, roaming within large sandy basins (e.g., Monterey Bay) and along range edges, where mortality from white shark bite is high (Tinker et al., 2016; Moxley et al., 2019) but competition for forage quality is low. Early during release, a few individuals (#315, 327, 353, 379, 595; Supplementary Figure S1) strayed offshore from Elkhorn Slough into Monterey Bay, beyond maximum sea otter diving depths, indicating initial stress or flight behavior, and if prolonged, invariably required recapture (4/5 otters, 80%). By contrast, others (#217, 228, 238, 520, 621, 673, 685; Supplementary Figure S1) dispersed along the nearshore coastline later into their release, resembling exploratory behavior of wild, immature animals. Either type of dispersal may be detrimental to survival by increasing energy expenditure (Yeates et al., 2007) and exposure to white shark bites relative to sheltering near a release site when prey resources are adequate. Within release sites along the Monterey Peninsula, where the sea otter population is at equilibrium density and characterized by highly structured home ranges (Tinker et al., 2013), high female density and pup production, and male territorial behavior; all individuals (#209, 225, 723, 774, 808, 809; Supplementary Figure S1) initially dispersed between 9 and 46 km, with four permanently leaving the area, migrating northward across Monterey Bay.
Dispersal behavior of newly released sea otters corresponded most predictably with the social environment at the release site (Supplementary Figures S2, S4). Specifically, areas with high proportions of pups to total population size are typically high-density female sites where male territoriality is intense and intolerant of younger males, and competition for available prey, and mating attempts may be stressful to naïve females. Interestingly, dispersal behavior seems most modulated when the community is composed of a few females (and their pups), rather than none (Figure 4). Unregulated male areas without females may be equally unattractive to maturing individuals. Before reproduction was evident in Elkhorn Slough, releases typically resulted in some dispersal behavior. This post-release behavior stabilized with the establishment of a few male territories, which may have provided social stability and predictability adequate for recruiting young individuals to the community.
El Niño events have a dramatic and well-understood impact in California marine ecosystems (Storlazzi and Griggs, 2000; Lluch-Belda et al., 2005; Yoon et al., 2015; Jacox et al., 2016). It is therefore perhaps unsurprising that long-term trends in MEI corresponded with sea otter dispersal patterns after reintroduction (Figure 4). Warm water masses and increased wave energy accompanying El Niño decrease kelp cover (Steneck et al., 2003). Diseases affecting kelp forest mesopredators are also linked to warm water anomalies (Harvell et al., 2019) and kelp grazing pressure also has increased under El Niño conditions (Burt et al., 2018). As a result, ENSO and other warm water events may disrupt important sea otter foraging and shelter habitats in open ocean ecosystems. These relationships are less clear in estuary habitats where eelgrass, not kelps, are the dominant biogenic substrates. A recent study suggests environmental stressors, including climate, are important in shaping sea otter prey availability in estuaries (Hessing-Lewis et al., 2018). While our analysis provides evidence to support the role of bottom-up environmental forces on sea otter prey in estuaries, the mechanisms are unclear. Future studies may resolve how ENSO conditions may have contributed to an increase in otter movements either through the availability of preferred prey, intraspecific population interactions, or through a combination of both.
Low population growth corresponded to increased dispersal, perhaps in response to resource limitation due to crowding (Tinker et al., 2008, 2019; Tinker et al., 2013). Beyond simple carrying capacity, however, density may also affect movements through interaction with the demographic variables we assessed. As adult males often establish and defend territories (Jameson, 1989) they are less mobile (Ralls et al., 1996), where non-territorial subadult and adult males are highly mobile. Females may be more resource-driven and prioritize energetic efficiency in both foraging and movement (Thometz et al., 2016). Here, we found males showed slightly higher dispersal distances (Figure 4A and Supplementary Figure S6). However, perhaps as our released otters had not reached sexual maturity, the sex-based differences were slight. Population demographics and dynamics may together influence post-release dispersal, as competition increases for many key resources – prey, territories, and mates.
Though we present a unique and novel dataset which may hold value for endangered species reintroductions and recovery, our study has several caveats. To begin, our dispersal records are relatively modest for a machine learning modeling approach, with movement data from 42 individual otters. This sample, however, belies a considerable cumulative effort of 894 days of field tracking with an additional 11,396 days of rehabilitation in captivity (Figure 2A). To maximize our sample, we merged all successfully released otters in one category, combining otters that were released once (n = 24) or multiple (n = 18) times. Throughout the study (Figure 2E), MBA staff recaptured released otters in need of intervention to ensure successful acclimation back into the wild (see Methods). While recaptured otters, as a group, seem initially more mobile than otters not requiring intervention, both groups stabilize their movements and acclimate to the wild environment over a similar time frame (Figure 5). Recapture therefore seems to be a valuable tool to reduce energetic costs of early release and reinforce residency near the release site. Future analyses, perhaps benefiting from larger samples, may learn more about the influence of ecological and rehabilitation factors to released otters through grouped cross validations.
Besides sample size, the biotelemetry platforms we used also contribute to the limitation of data. Here we generated LCPs from resight observations, conducted daily using manual searches and VHF receivers. For three decades researchers have implanted VHF tags (Williams and Siniff, 1983; Ralls et al., 1989) to track wild sea otters, due to difficulties with external attachment applications (Garshelis and Siniff, 1983; Williams and Siniff, 1983; Ralls et al., 1989). Innovations in external transmitting tags for sea otters will automate the observation process, decreasing the logistical burden to monitoring, while providing real-time high-resolution locations multiple times a day (Hazen et al., 2012; Hays et al., 2016). Despite the limitations of our current study, daily location data were the product of an innovative and large logistical effort in rearing, tagging, and tracking surrogate-reared sea otters, and the signals identified provide an opportunity to improve the success of future releases.
In this study we successfully addressed issues which have persistently challenged endangered species reintroductions. Soft releases, or provisioning with food and temporary shelter, are commonly used when transitioning terrestrial animals from captive to wild settings (Waters, 2010; Walters et al., 2010; Imey et al., 2012; Kierulff et al., 2012). As soft releases are logistically more difficult in marine settings, we intensively monitored releases for 2 to 3 weeks and recaptured animals showing signs of stress and weight loss. This strategy likely supported our high success rate (75%) performing similar functions of a soft release, aiding the adjustment and ultimate survival of otters in the wild. Beyond transitioning to the wild, overcoming Allee effects and other small population challenges is critical for reintroductions (Pimm, 1991; Van Houtan et al., 2009). As with previous studies, we found that consistent enhancements or restocking of the wild population (Figure 2E) generated a persistent propagule pressure (Kuussaari et al., 1998; Lockwood et al., 2005), especially of females, that kept the wild population from failing. As a result, the release site changed from an aggregation of ∼20 transient males to a breeding population of >150 otters (Mayer et al., 2020). A 50-year study of 125 closed populations of terrestrial mammals indicated the benefits of restocking likely decline after populations reach 50 individuals (Van Houtan et al., 2009). Based on our findings, community structure may be more relevant than population density when establishing novel release sites. For sea otters, early seeding or even primary reintroduction of females may be the key component to creating viable and sustainable populations outside the current range.
Active reintroductions outside the current range may be necessary to restore sea otters and recover ecosystems across the California coast, and beyond. Stranding response, veterinary care, surrogacy training, and post-release tracking are all necessary for successful reintroduction, and each is logistically demanding. For this reason, understanding the factors that may drive the movements and residency of sea otters post-release are critical. Here we used existing release data to evaluate the program’s performance, in the general framework of evidence-based conservation and adaptive management (Sutherland et al., 2004; McCarthy and Possingham, 2007). Our results indicate that climatic and population factors at the release site are likely more influential than demographic or animal care dynamics in captivity and provide several practical lessons for future surrogacy rehabilitation and reintroductions.
Data Availability Statement
The datasets and scripts used in this study are available at open-access repositories at Github (https://bit.ly/3jNVUrD) and the Open Science Framework (https://osf.io/rfqeh/).
Ethics Statement
All research activities were authorized by the U.S. Fish and Wildlife Service (permits #MA186914-2 and #MA032027-2). The scope of the research outlined in this study and all protocols for use of live southern sea otters were approved by the Institutional Animal Care and Use Committee of the Monterey Bay Aquarium. Animal housing facilities complied with USDA regulations governing the welfare of marine mammals and met the Association of Zoos and Aquariums accreditation standards.
Author Contributions
KV conceived the study. SB, TN, and KV analyzed the data, generated the figures, and wrote the manuscript, with significant manuscript edits from KM. SB, TN, and KM managed and curated the data. KM, TN, and MM contributed to the design of the field tracking, captive rearing, and surrogacy reintroduction programs. All authors reviewed the final manuscript.
Funding
This research was supported by the generous donor contributions to the Monterey Bay Aquarium. The sea otter surrogacy and reintroduction programs were supported by grants from the Paul M. Angell Family Foundation, Arthur L. and Elaine V. Johnson Foundation, and the Flora Family Foundation.
Conflict of Interest
The authors declare that the research was conducted in the absence of any commercial or financial relationships that could be construed as a potential conflict of interest.
Acknowledgments
M. Staedler, J. Fujii, A. Johnson, S. Hazan, C. DeAngelo, C. Donegan, M. Young, N. Lenihan, and numerous staff and volunteers provided technical and logistical support. The Marine Mammal Center and California Department of Fish and Wildlife assisted with sea otter stranding response. D. Casper and H. Harris contributed veterinary care and expertise. B. Van Wagenen and R. Eby assisted with sea otter resights. L. Carswell provided advice and assisted with permits. The Moss Landing Harbor District and Elkhorn Slough National Estuarine Research Reserve provided field access. Jim Estes, Tim Tinker, and reviewers improved earlier versions of this manuscript.
Supplementary Material
The Supplementary Material for this article can be found online at: https://www.frontiersin.org/articles/10.3389/fmars.2020.539904/full#supplementary-material
References
Adimey, N. M., Ross, M., Hall, M., Reid, J. P., Barlas, M. E., Keith Diagne, L. W., et al. (2016). Twenty-six years of post-release monitoring of Florida manatees (Trichechus manatus latirostris): evaluation of a cooperative rehabilitation program. Aqua. Mamm. 42, 376–391. doi: 10.1578/am.42.3.2016.376
Atkinson, R., Rhodes, C., Macdonald, D., and Anderson, R. (2002). Scale-free dynamics in the movement patterns of jackals. Oikos 98, 134–140. doi: 10.1034/j.1600-0706.2002.980114.x
Becker, S. L., Brainard, R. E., and Van Houtan, K. S. (2019). Densities and drivers of sea turtle populations across Pacific coral reef ecosystems. PLoS One 14:e0214972. doi: 10.1371/journal.pone.0214972
Breed, G. A., Golson, E. A., and Tinker, M. T. (2017). Predicting animal home-range structure and transitions using a multistate Ornstein-Uhlenbeck biased random walk. Ecology 98, 32–47. doi: 10.1002/ecy.1615
Burt, J. M., Tinker, M. T., Okamoto, D. K., Demes, K. W., Holmes, K., and Salomon, A. K. (2018). Sudden collapse of a mesopredator reveals its complementary role in mediating rocky reef regime shifts. Proc. R. Soc. B Biol. Sci. 285:20180553. doi: 10.1098/rspb.2018.0553
Cleveland, W. S., Devlin, S. J., and Grosse, E. (1988). Regression by local fitting: Methods, properties, and computational algorithms. J. Econom. 37, 87–114. doi: 10.1016/0304-4076(88)90077-2
Dayton, P. K., and Tegner, M. J. (1990). Bottoms Beneath Troubled Waters: Benthic Impacts of the 1982-1984 El Niño in the Temerate Zone. Elsevier Oceanogr. Ser. 52, 433–472. doi: 10.1016/S0422-9894(08)70045-X
Di Lorenzo, E., and Mantua, N. (2016). Multi-year persistence of the 2014/15 North Pacific marine heatwave. Nat. Clim. Change 6:1042. doi: 10.1038/nclimate3082
Edwards, M. S. (2004). Estimating scale-dependency in disturbance impacts: El Niños and giant kelp forests in the northeast Pacific. Oecologia 138, 436–447. doi: 10.1007/s00442-003-1452-8
Estes, J. A., and Duggins, D. O. (1995). Sea Otters and Kelp Forests in Alaska: Generality and Variation in a Community Ecological Paradigm. Ecol. Monogr. 65, 75–100. doi: 10.2307/2937159
Estes, J. A., and Palmisano, J. F. (1974). Sea Otters: Their Role in Structuring Nearshore Communities. Science 185, 1058–1060. doi: 10.1126/science.185.4156.1058
Gagné, T. O., Hyrenbach, K. D., Hagemann, M. E., and Van Houtan, K. S. (2018b). Trophic signatures of seabirds suggest shifts in oceanic ecosystems. Sci. Adv. 4:eaao3946. doi: 10.1126/sciadv.aao3946
Gagné, T. O., Hyrenbach, K. D., Hagemann, M. E., Bass, O. L., Pimm, S. L., Macdonald, M., et al. (2018a). Seabird Trophic Position Across Three Ocean Regions Tracks Ecosystem Differences. Front. Mar. Sci. 5:317. doi: 10.17605/OSF.IO/4S9TY
Garshelis, D. L., and Siniff, D. B. (1983). Evaluation of Radio-Transmitter Attachments for Sea Otters. Wildlife Soc. Bull. 11, 378–383.
Harvell, C. D., Montecino-Latorre, D., Caldwell, J. M., Burt, J. M., Bosley, K., Keller, A., et al. (2019). Disease epidemic and a marine heat wave are associated with the continental-scale collapse of a pivotal predator (Pycnopodia helianthoides). Sci. Adv. 5:eaau7042. doi: 10.1126/sciadv.aau7042
Hastie, T., Tibshirani, R., and Friedman, J. (eds). (2009). “Overview of supervisd learning,” in The elements of statistical learning, (Berlin: Springer), 9–41. doi: 10.1007/978-0-387-84858-7_2
Hatfield, B. B., Yee, J. L., Kenner, M. C., and Tomoleoni, J. A. (2019). California sea otter (Enhydra lutris nereis) census results. Spring 2019. USGS Data Ser. 1118:20. doi: 10.3133/ds1118
Hays, G. C., Ferreira, L. C., Sequeira, A. M., Meekan, M. G., Duarte, C. M., Bailey, H., et al. (2016). Key questions in marine megafauna movement ecology. Trends Ecol. Evol. 31, 463–475. doi: 10.1016/j.tree.2016.02.015
Hazen, E. L., Maxwell, S. M., Bailey, H., Bograd, S. J., Hamann, M., Gaspar, P., et al. (2012). Ontogeny in marine tagging and tracking science: technologies and data gaps. Mar. Ecol. Prog. Ser. 457, 221–240. doi: 10.3354/meps09857
Hessing-Lewis, M., Rechsteiner, E. U., Hughes, B. B., Tinker, M. T., Monteith, Z. L., Olson, A. M., et al. (2018). Ecosystem features determine seagrass community response to sea otter foraging. Mar. Poll. Bull. 134, 134–144. doi: 10.1016/j.marpolbul.2017.09.047
Hughes, B. B., Eby, R., Van Dyke, E., Tinker, M. T., Marks, C. I., Johnson, K. S., et al. (2013). Recovery of a top predator mediates negative eutrophic effects on seagrass. Proc. Natl. Acad. Sci. 110, 15313–15318. doi: 10.1073/pnas.1302805110
Hurvich, C. M., and Tsai, C. L. (1989). Regression and time series model selection in small samples. Biometrika 76, 297–307. doi: 10.1093/biomet/76.2.297
Imey, N. A. D., Mignucci-Giannoni, A. A., Auil-Gomez, N. E., Da Silvaa, V. M., Alvite, C. M. S. D. C., Morales-Vela, B., et al. (2012). Manatee rescue, rehabilitation, and release efforts as a tool for species conservation. Florida, FL: University Press of Florida Press.
Jacox, M. G., Hazen, E. L., Zaba, K. D., Rudnick, D. L., Edwards, C. A., Moore, A. M., et al. (2016). Impacts of the 2015–2016 El Niño on the California Current System: Early assessment and comparison to past events. Geophys. Res. Lett. 43, 7072–7080. doi: 10.1002/2016GL069716
Jameson, R. J. (1989). Movements, home range, and territories of male sea otter off central California. Mar. Mamm. Sci. 5, 159–172. doi: 10.1111/j.1748-7692.1989.tb00330.x
Kierulff, M., Ruiz−Miranda, C., De Oliveira, P. P., Beck, B., Martins, A., Dietz, J., et al. (2012). The G olden lion tamarin Leontopithecus rosalia: a conservation success story. Int. Zoo Yearbook 46, 36–45. doi: 10.1111/j.1748-1090.2012.00170.x
Kittinger, J. N., Mcclenachan, L., Gedan, K. B., and Blight, L. K. (2015). Marine historical ecology in conservation: Applying the past to manage for the future. California, CA: University of California Press. doi: 10.1525/california/9780520276949.001.0001
Kuussaari, M., Saccheri, I., Camara, M., and Hanski, I. (1998). Allee effect and population dynamics in the Glanville fritillary butterfly. New Jersey, NJ: John Wiley and Sons, 384–392. doi: 10.2307/3546980
Lafferty, K. D., and Tinker, T. M. (2014). Sea otters are recolonizing southern California in fits and starts. Ecosphere 5, 1–11. doi: 10.1890/ES13-00394.1
Laidre, K. L., Estes, J. A., Tinker, M. T., Bodkins, J., Monson, D., and Schneider, K. S. (2006). Patterns of growth and body condition in sea otters from the Aleutian archipelago before and after the recent population decline. J. Anim. Ecol. 75, 978–989. doi: 10.1111/j.1365-2656.2006.01117.x
Laidre, K. L., Jameson, R. J., and Demaster, D. P. (2001). An estimation of carrying capacity for sea otters along the California coast. Mar. Mamm. Sci. 17, 294–309. doi: 10.1111/j.1748-7692.2001.tb01272.x
Le Gouar, P., Robert, A., Choisy, J. P., Henriquet, S., Lecuyer, P., Tessier, C., et al. (2008). Roles of survival and dispersal in reintroduction success of Griffon vulture (Gyps fulvus). Ecol. Appl. 18, 859–872. doi: 10.1890/07-0854.1
Lindsey, J. K. (2016). Estuarine habitat use by the California sea otter (Enhydra lutris nereis). California, CA: University of San Jose.
Lluch-Belda, D., Lluch-Cota, D. B., and Lluch-Cota, S. E. (2005). Changes in marine faunal distributions and ENSO events in the California Current. Fisher. Oceanogr. 14, 458–467. doi: 10.1111/j.1365-2419.2005.00347.x
Lockwood, J. L., Cassey, P., and Blackburn, T. (2005). The role of propagule pressure in explaining species invasions. Trends Ecol. Evol. 20, 223–228. doi: 10.1016/j.tree.2005.02.004
Mantua, N., and Hare, S. (2002). The Pacific Decadal Oscillation. J. Oceanogr. 58, 35–44. doi: 10.1023/A:1015820616384
Mayer, K. A., Tinker, M. T., Nicholson, T. E., Murray, M. J., Johnson, A. B., Deangelo, C., et al. (2020). Surrogate rearing a keystone species to enhance population and ecosystem restoration. Oryx 53, 1–11. doi: 10.1017/s0030605319000346
McCarthy, M. A., and Possingham, H. P. (2007). Active adaptive management for conservation. Conserv. Biol. 21, 956–963. doi: 10.1111/j.1523-1739.2007.00677.x
Monson, D. H., Estes, J. A., Bodkin, J. L., and Siniff, D. B. (2000). Life history plasticity and population regulation in sea otters. Oikos 90, 457–468. doi: 10.1034/j.1600-0706.2000.900304.x
Moore, M., Early, G., Touhey, K., Barco, S., Gulland, F., and Wells, R. (2007). Rehabilitation and release of marine mammals in the United States: risks and benefits. Mar. Mamm. Sci. 23, 731–750. doi: 10.1111/j.1748-7692.2007.00146.x
Moxley, J. H., Nicholson, T. E., Van Houtan, K. S., and Jorgensen, S. J. (2019). Non-trophic impacts from white sharks complicate population recovery for sea otters. Ecol. Evol. 9, 6378–6388. doi: 10.1002/ece3.5209
Nathan, R., Klein, E., Robledo-Arnuncio, J. J., and Revilla, E. (2012). “Disperal kernels,” in Dispersal ecology and evolution, eds T. G. Benton, J. Clobert, M. Baguette, and J. M. Bullock (Oxford: Oxford University Press), 187–210. doi: 10.1093/acprof:oso/9780199608898.003.0015
Nicholson, T. E., Mayer, K. A., Staedler, M. M., and Johnson, A. B. (2007). Effects of rearing methods on survival of released free-ranging juvenile southern sea otters. Biol. Conserv. 138, 313–320. doi: 10.1016/j.biocon.2007.04.026
Nicholson, T. E., Mayer, K. A., Staedler, M. M., Fujii, J. A., Murray, M. J., Johnson, A. B., et al. (2018). Gaps in kelp cover may threaten the recovery of California sea otters. Ecography 41, 1751–1762. doi: 10.1111/ecog.03561
Nicholson, T. E., Mayer, K. A., Staedler, M. M., Gagne, T. O., Murray, M. J., Young, M., et al. (2020). Robust age estimation of southern sea otters from multiple morphometrics. Ecol. Evol. 10, 8592–8609. doi: 10.1002/ece3.6493
Pace, M. L., Cole, J. J., Carpenter, S. R., and Kitchell, J. F. (1999). Trophic cascades revealed in diverse ecosystems. Trends Ecol. Evol. 14, 483–488. doi: 10.1016/s0169-5347(99)01723-1
R Core Team (2018). R: A language and environment for statistical computing. R Foundation for Statistical Computing. Vienna: R Core Team.
Ralls, K., and Siniff, D. B. (1990). Time Budgets and Activity Patterns in California Sea Otters. J. Wildlife Manage. 54, 251–259. doi: 10.2307/3809038
Ralls, K., Eagle, T. C., and Siniff, D. B. (1996). Movement and spatial use patterns of California sea otters. Can. J. Zool. 74, 1841–1849. doi: 10.1139/z96-207
Ralls, K., Siniff, D., Williams, T., and Kuechle, V. (1989). An intraperitoneal radio transmitter for sea otters. Mar. Mamm. Sci. 5, 376–381. doi: 10.1111/j.1748-7692.1989.tb00349.x
Rathbun, G. B., Hatfield, B. B., and Murphey, T. G. (2000). Status of translocated sea otters at San Nicolas Island. California. Southwestern Naturalist 45, 322–328. doi: 10.2307/3672835
Reynolds, A. M., and Frye, M. A. (2007). Free-Flight Odor Tracking in Drosophila Is Consistent with an Optimal Intermittent Scale-Free Search. PLoS One 2:e354. doi: 10.1371/journal.pone.0000354
Ripley, B., Venables, B., Bates, D. M., Hornik, K., Gebhardt, A., Firth, D., et al. (2020). Package ‘mass’. Cran R package Version 7.3-53.
Steneck, R. S., Graham, M. H., Bourque, B. J., Corbett, D., Erlandson, J. M., Estes, J. A., et al. (2003). Kelp forest ecosystems: biodiversity, stability, resilience and future. Environ. Conserv. 29, 436–459. doi: 10.1017/s0376892902000322
Storlazzi, C. D., and Griggs, G. B. (2000). Influence of El Niño–Southern Oscillation (ENSO) events on the evolution of central California’s shoreline. Geol. Soc. Am. Bull. 112, 236–249. doi: 10.1130/0016-7606(2000)112<236:IOENOE>2.0.CO;2
Sutherland, W. J., Pullin, A. S., Dolman, P. M., and Knight, T. M. (2004). The need for evidence-based conservation. Trends Ecol. Evol. 19, 305–308. doi: 10.1016/j.tree.2004.03.018
Thometz, N. M., Staedler, M. M., Tomoleoni, J., Bodkin, J. L., Bentall, G. B., and Tinker, M. T. (2016). Trade-offs between energy maximization and parental care in a central place forager, the sea otter. Behav. Ecol. 27, 1552–1566. doi: 10.1093/beheco/arw089
Thometz, N. M., Tinker, M. T., Staedler, M. M., Mayer, K. A., and Williams, T. M. (2014). Energetic demands of immature sea otters from birth to weaning: implications for maternal costs, reproductive behavior and population-level trends. J. Exp. Biol. 217, 2053–2061. doi: 10.1242/jeb.099739
Tinker, M. T., Jessup, D., Staedler, M., Murray, M., Miller, M., Burgess, T. et al. (2013). Sea Otter Population Biology at Big Sur and Monterey California: Investigating the Consequences of Resource Abundance and Anthropogenic Stressors for Sea Otter Recovery. Final Report to the California Coastal Conservancy and the U.S. Fish and Wildlife Service.California, CA: University of California Santa Cruz.
Tinker, M. T., and Hatfield, B. B. (2017). California sea otter (Enhydra letris nereis) census results, spring 2017. Reston, VA: U.S. Geological Survey. doi: 10.3133/ds1067
Tinker, M. T., and Hatfield, B. B. (2018). California sea otter (Enhydra letris nereis) census results, spring 2018. Reston, VA: U.S. Geological Survey.
Tinker, M. T., Bentall, G., and Estes, J. A. (2008). Food limitation leads to behavioral diversification and dietary specialization in sea otters. Proc. Natl. Acad. Sci. 105, 560–565. doi: 10.1073/pnas.0709263105
Tinker, M. T., Espinosa, S. M., Staedler, M. M., Tomoleoni, J. A., Fujii, J., Eby, R., et al. (2018). The population status and ecology of sea otters in Elkhorn Slough, California. Final Report for California Coastal Conservancy and U.S. Fish and Wildlife Service. Washington, D.C: U.S. Fish and Wildlife Service.
Tinker, M. T., Gill, V. A., Esslinger, G. G., Bodkin, J., Monk, M., Mangel, M., et al. (2019). Trends and Carrying Capacity of Sea Otters in Southeast Alaska. J. Wildlife Manage. 83, 1073–1089. doi: 10.1002/jwmg.21685
Tinker, M. T., Hatfield, B. B., Harris, M. D., and Ames, J. A. (2016). Dramatic increase in sea otter mortality from white sharks in California. Mar. Mamm. Sci. 32, 309–326. doi: 10.1111/mms.12261
Tweed, E. J., Foster, J. T., Woodworth, B. L., Oesterle, P., Kuehler, C., Lieberman, A. A., et al. (2003). Survival, dispersal, and home-range establishment of reintroduced captive-bred puaiohi. Myadestes palmeri. Biol. Conserv. 111, 1–9. doi: 10.1016/s0006-3207(02)00175-1
USFWS (2003). Final revised recovery plan for the southern sea otter (Enhydra lutris nereis). Washington, D.C: U.S. Fish and Wildlife Service.
USFWS (2015). Southern sea otter (Enhydra lutris nereis) 5-year review: summary and evaluation. Ventura, CA: Ventura Fish and Wildlife Office.
Van Etten, J. (2017). R Package gdistance: Distances and Routes on Geographical Grids. J. Statist. Soft. 76, 1–21. doi: 10.18637/jss.v076.i13
van Heezik, Y., Maloney, R. F., and Seddon, P. J. (2009). Movements of translocated captive-bred and released Critically Endangered kaki (black stilts. Himantopus novaezelandiae) and the value of long-term post-release monitoring. Oryx 43, 639–647. doi: 10.1017/s0030605309990081
Van Houtan, K. S., Bass, O. L., Lockwood, J., and Pimm, S. L. (2010). Importance of estimating dispersal for endangered bird management. Conserv. Lett. 3, 260–266. doi: 10.1111/j.1755-263x.2010.00108.x
Van Houtan, K. S., Halley, J. M., Van Aarde, R. J., and Pimm, S. L. (2009). Achieving success with small, translocated mammal populations. Conserv. Lett. 2, 254–262. doi: 10.1111/j.1755-263x.2009.00081.x
Van Houtan, K. S., Pimm, S. L., Halley, J. M., Bierregaard, R. O., and Lovejoy, T. E. (2007). Dispersal of Amazonian birds in continuous and fragmented forest. Ecol. Lett. 10, 219–229. doi: 10.1111/j.1461-0248.2007.01004.x
Venables, W. N. R., and Ripley, B. D. (2002). Modern Applied Statistics with S, 4th Edn. New York: Springer. doi: 10.1007/978-0-387-21706-2
Walters, J. R., Derrickson, S. R., Michael Fry, D., Haig, S. M., Marzluff, J. M., and Wunderle, J. M. Jr. (2010). Status of the California Condor (Gymnogyps californianus) and efforts to achieve its recovery. Auk 127, 969–1001. doi: 10.1525/auk.2010.127.4.969
Waters, S. (2010). Swift fox Vulpes velox reintroductions: a review of release protocols. Int. Zoo Yearbook 44, 173–182. doi: 10.1111/j.1748-1090.2009.00091.x
Weatherall, P., Marks, K. M., Jakobsson, M., Schmitt, T., Tani, S., Arndt, J. E., et al. (2015). A new digital bathymetric model of the world’s oceans. Earth Space Sci. 2, 331–345. doi: 10.1002/2015EA000107
Wei, T., and Simko, V. (2017). R package “corrplot”: Visualization of a Correlation Matrix (Version 0.84).
Williams, T. D., and Siniff, D. B. (1983). Surgical implantation of radiotelemetry devices in the sea otter. J. Am. Vet. Med. Assoc. 183, 1290–1291.
Wolter, K., and Timlin, M. S. (1993). “Monitoring ENSO in COADS with a seasonally adjusted principle component index,” in Proceedings of the 17th Climate Diagnostics. (Oklahoma: University of Oklahoma), 52–57.
Yeates, L. C., Williams, T. M., and Fink, T. L. (2007). Diving and foraging energetics of the smallest marine mammal, the sea otter (Enhydra lutris). J. Exp. Biol. 210, 1960–1970. doi: 10.1242/jeb.02767
Yoon, J.-H., Wang, S. S., Gillies, R. R., Kravitz, B., Hipps, L., and Rasch, P. J. (2015). Increasing water cycle extremes in California and in relation to ENSO cycle under global warming. Nat. Commun. 6:8657. doi: 10.1038/ncomms9657
Keywords: reintroduction (release), ecosystem restoration, surrogacy, random forest (bagging) and machine learning, dispersal, heavy tailed data, endangered species, population recovery
Citation: Becker SL, Nicholson TE, Mayer KA, Murray MJ and Van Houtan KS (2020) Environmental Factors May Drive the Post-release Movements of Surrogate-Reared Sea Otters. Front. Mar. Sci. 7:539904. doi: 10.3389/fmars.2020.539904
Received: 02 March 2020; Accepted: 21 October 2020;
Published: 12 November 2020.
Edited by:
Rowan Trebilco, Centre for Marine Socioecology, AustraliaReviewed by:
Juan Carlos Villaseñor-Derbez, University of California, Santa Barbara, United StatesDaniel Okamoto, Florida State University, United States
Copyright © 2020 Becker, Nicholson, Mayer, Murray and Van Houtan. This is an open-access article distributed under the terms of the Creative Commons Attribution License (CC BY). The use, distribution or reproduction in other forums is permitted, provided the original author(s) and the copyright owner(s) are credited and that the original publication in this journal is cited, in accordance with accepted academic practice. No use, distribution or reproduction is permitted which does not comply with these terms.
*Correspondence: Teri E. Nicholson, dG5pY2hvbHNvbkBtYmF5YXEub3Jn
†These authors have contributed equally to this work