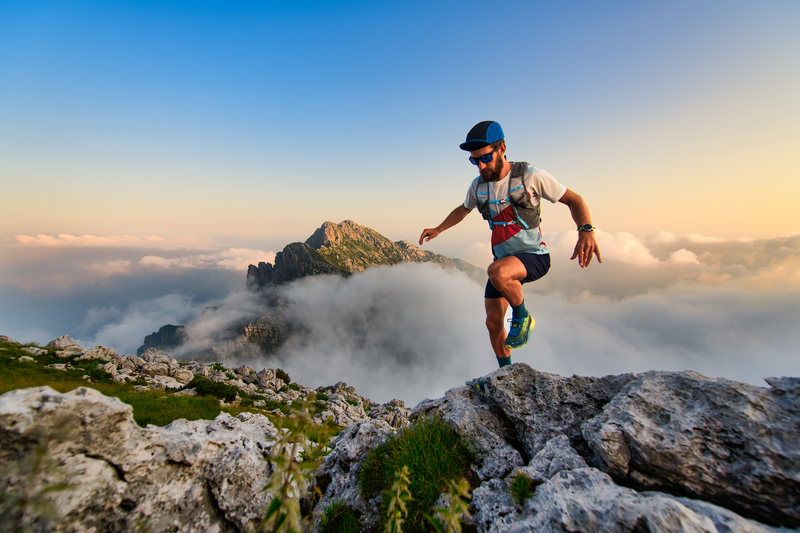
94% of researchers rate our articles as excellent or good
Learn more about the work of our research integrity team to safeguard the quality of each article we publish.
Find out more
ORIGINAL RESEARCH article
Front. Mar. Sci. , 31 July 2020
Sec. Marine Fisheries, Aquaculture and Living Resources
Volume 7 - 2020 | https://doi.org/10.3389/fmars.2020.00645
Acoustic telemetry techniques are very useful tools to monitor in detail the swimming behavior and spatial use of fish in artificial rearing environments at individual and group levels. We evaluated the feasibility of using passive acoustic telemetry to monitor fish welfare in sea-cage aquaculture at an industrial scale, characterizing for the first time the diel swimming and distribution patterns of gilthead seabream (Sparus aurata) at fine-scale. Ten fish were implanted with acoustic tags equipped with pressure and acceleration sensors, and monitored in a commercial-size sea-cage for a period of 1 month. Overall, fish exhibited clear differences in day vs. night patterns both on swimming activity and vertical distribution throughout the experiment. Space use increased at night after the implementation of structural environmental enrichment in the sea-cage. Acoustic telemetry may represent an advancement to monitor fish farming procedures and conditions, helping to promote fish welfare and product quality.
Production systems in fin-fish aquaculture, in particular intensive farming, can cause stress, pain, health problems, and even mortality at any stage of the production process (Broom, 2007). Many fish species are reared in open-sea cages during the last on-growing production stage, where they can experience stressful events by routine farming procedures and conditions, such as transport, crowding, handling, housing, high densities, feeding, and environmental stressors (Volpato et al., 2007). Behavioral studies are increasingly occupying an important place in welfare research, since behavioral responses are an early and easily observable response to adverse conditions or stressful stimulus, often specific for each stressor. Therefore, they can be used as non-invasive indicators of stress or changes in welfare (Martins et al., 2012). Common indicators of poor welfare include, for example, changes in foraging behavior, ventilatory activity, aggression, and stereotypic or abnormal behaviors. Such responses are fast and easy to observe, but they can be variable over time and difficult to quantify (Martins et al., 2012; Noble et al., 2018). Swimming behavior is a common additional indicator that can be affected by water quality, population densities, feeding regimes, and diseases; therefore, group swimming behavior and spatial distribution may be used as operational on-farm welfare indicators to evaluate hunger, stress level and general health status of fish (Martins et al., 2012; Sneddon et al., 2016; Alfonso et al., 2020a).
Acoustic telemetry techniques are very useful tools to monitor in detail the swimming behavior and spatial use of the fish in the rearing environment at both individual and group levels (Baras and Lagardère, 1995). For instance, some acoustic transmitters are equipped with sensors that provide information on the swimming depth, allowing determining the exact position of fish (Leclercq et al., 2018), or even with acceleration sensors, which provide information about swimming activity of the tagged fish (Thorstad et al., 2013; Yoshida et al., 2015; Kolarevic et al., 2016; Horie et al., 2017). Regarding farmed fish species, acoustic telemetry techniques have been previously applied to assess the swimming behavior within cages in chinook salmon Oncorhynchus tshawytscha (Cubitt et al., 2003), Atlantic cod Gadus morhua (Rillahan et al., 2009, 2011) and Atlantic salmon Salmo salar (Føre et al., 2011, 2017, 2018b; Kolarevic et al., 2016), but there are no studies about positioning on other species such as the gilthead seabream Sparus aurata. Knowledge on the complexity of the swimming trajectory, the spatial distribution, the activity rhythms, is key to assess the biological requirements and welfare status of farmed fish species and, therefore, to improve both production and management in aquaculture (Føre et al., 2011, 2018b). It is known that fish movements are correlated with energy use (e.g., muscle activity and energy expenditure of fish) (e.g., Lembo et al., 2007; Wilson et al., 2013; Wright et al., 2014; Carbonara et al., 2015; Zupa et al., 2015), which might be altered by different rearing activities, and therefore, understanding fish swimming activity and energy consumption is also essential for aquaculture management in terms of welfare and production. Fish welfare is an important issue for the industry, not only because of public perception, commercialization and acceptance of products, but also due to the benefits in terms of efficiency, quality and quantity of production (Ashley, 2007). It is therefore necessary to develop and introduce new technological systems or tools that allow the correct observation of fish welfare at a commercial scale (Føre et al., 2018a).
The aim of this study was to evaluate the feasibility of using passive acoustic telemetry techniques in commercial conditions as a potential tool to monitor fish welfare in sea-cage aquaculture. We monitored gilthead seabream juveniles implanted with acoustic transmitters equipped with pressure and acceleration sensors, in experimental sea-cages for a period of 1 month, to characterize for the first time the diel swimming and distribution patterns at a fine-scale. Moreover, we also test this tool during the inclusion of structural environmental enrichment (EE) in the sea-cage. Structural EE is a method to improve the biological functioning of captive animals by adding modifications to their environment (Näslund and Johnsson, 2016), and has been recently considered as a highly recommended tool to guarantee and improve the welfare of captive fish (Brydges and Braithwaite, 2009).
Swimming behavior and distribution of gilthead seabream in sea-cages was studied by using an acoustic telemetry system in an experimental sea-cage located in the coastal waters of Port of Andratx, belonging to the Laboratory of Marine Research and Aquaculture (LIMIA; Mallorca, Balearic Islands, Spain). The experimental sea-cage was structurally similar than those used in commercial fish production, although smaller in size, which consisted on floating plastic rings, with a fence and an anti-bird net on top, with a cylindrical-conical net-pen of 12.5 m of diameter and 6 m depth. The experiment was carried out from the 3rd-May to the 3rd-June 2019. A total of 360 seabreams were used for the experiment, of which 10 seabreams (mean length ± SE: 20.17 ± 1.09 cm; mean weight 217.58 ± 55.96 g) were tagged with “accel-tag” acoustic transmitters (Thelma Biotel Ltd., Trondheim, Norway; model ADP-LP7; output power: 146 dB; frequency: 69 kHz; diameter: 7.3 mm; length: 22.7 mm; weight in air: 2.1 g; weight in water: 1.1 g; depth range: 25 m; transmission interval: 50–70 s) equipped with a pressure sensor and a triaxial accelerometer, which provided measurements of the swimming depth (in m) and the root mean square of the three acceleration axes (in mcots–2), respectively. Fish were collected from the on-land tanks using a hand net and were anesthetized by submersion in an aqueous solution of tricaine methane sulfonate (MS-222, 75 mg L–1). Immersion period was 4 min at 15–17°C. Once anesthetized (stages 4-5; Summerfelt and Smith, 1990), the fish were photographed, measured and weighed. Then, the fish were placed with the ventral side up on a surgical table to proceed with the implantation of the transmitters. An incision (∼1 cm) was made on the ventral surface, posterior to the pelvic girdle. An “accel-tag” transmitter was introduced through the incision into the body cavity above the pelvic girdle. The incision was closed with two or three independent silk sutures. The fish were regularly sprayed with water during the surgery (handling time: 2–3 min). Before each incision, the surgical equipment was rinsed in 70% ethanol and allowed to dry. Tagged fish were placed back to the on-land tank together with the rest of the seabream for recovery, which took place in less than 5 min. No medical treatment after surgical implantation was used. This tagging procedure and technique has been successfully applied in previous studies on gilthead seabream and documented a good recovery of equilibrium and normal swimming behavior following transmitter implantation, as well as long-term survival and no mid-term stress physiological effects (Arechavala-Lopez et al., 2012; Šegvić-Bubić et al., 2018; Palstra et al., 2019; Alfonso et al., 2020b). In our experiment, tagged gilthead seabream showed 100% short-term survival rate. All processes (transport, handling, anesthesia, tagging, and recovering) were done by trained personnel, and they were approved by enabled Ethics Committee of Animal Experimentation (CEEA-103/10/18) of the University of Balearic Islands (UIB) and carried out strictly in according to the European Directive (2010/63/UE) and the Spanish Royal Decree (53/3013) regarding treatment, care and welfare of experimental animals.
After recovery, all fish (10 tagged fish + 350 untagged fish) were transferred to the experimental sea-cage, keeping them until the 6th-May for acclimation without food. After 3 days of acclimation with no feed, the fish were fed daily throughout the experiment (∼2% of body weight) with commercial food pellets (Skretting®, D-6 Alterna 3P) using automatic continuous food dispensers during the day. The swimming activity (i.e., acceleration) and vertical distribution (i.e., depth) of each fish were monitored by an acoustic receiver array, composed by three receivers (Thelma Biotel Ltd.; model TBR 700; diameter: 75 mm; length: 230 mm) positioned around the sea-cage, suspended with anchored ropes attached to the floating rings of the cage structure at 5 m deep, forming a triangle (see Supplementary Figure S1). In order to assess the position accuracy of the system, a stationary reference tag (Sync-tag; Thelma Biotel Ltd., model R-MP13; 69 kHz; code: S256; diameter: 12.7 mm; length: 33.3 mm; 597–597 s interval) was anchored at 3 m deep above one of the receivers (Supplementary Figure S1). This 1 month experiment was divided into two two-week periods: the “bare period” (6th–19th May 2019), without environmental enrichment; and the “enriched period” (21st May to 3rd–June 2019), where structural EE was added. Enrichment structures consisted of four vertical ropes (6 m length) hanging down into the net-pen with a buoy on top, forming a square in the middle of the cage at about one meter distance one from each other, and attached to the ring by two crossed horizontal ropes (Supplementary Figure S1). Receivers were equipped with temperature sensors, which provided continuous measurements of seawater temperature throughout the whole experiment.
Data from the receivers was downloaded after completion of the experiment and first inspected using the software ComPort® (Thelma Biotel), and then imported to R (R Core Team,, 2019) for all the subsequent analysis. Data was filtered to remove false detections, which are generated by collisions between acoustic signals from transmitters or due to environmental noise, and result on data corresponding to non-existing transmitters or abnormal sensor values (e.g., depth values below the maximum depth of the cage, same signal captured by several receivers but transmitting different sensor values, etc.). Then, the signals with sensor data that were simultaneously detected by different receivers were thinned to leave one record per emitted signal. Finally, data was binned into 1h intervals, for each of which the average depth and acceleration, and the interquartile range of depth (difference between the first and third quartile of depth values, used as a proxy for the vertical movement activity) were calculated. Fine-scale 3D position estimations were calculated by the manufacturer using a hyperbolic triangulation method based on the time differences of arrival, where error sensitivity of positions was estimated based on the deviation between the calculated positions and known positions (PinPoint positioning system, Thelma Biotel Ltd., Trondheim, Norway). These x,y,z positions were then used to estimate the 3D space use of each individual for each day and night period by applying a 3D kernel density estimator using the “ks” package in R (Duong, 2019). These 3D kernels densities where later used to calculate the volume size of the 50 and 95% probability contours (volumetric space use), as well as the overlap between pairs of individuals (i.e., size of the overlapped volume relative to the total joint volume of the two individuals).
Accelerations, vertical distribution range and size of the volumetric space use of fishes during day and night periods, as well as in presence of structural enrichment, were tested using a basic statistical model with a Bayesian approach, original by authors, which was able to deal with the non-normal distributions of the data and the non-independence between mean and variance observed in a preliminary exploration. The statistical units were the mean acceleration (acc) and the depth interquartile range (IQR) along a period of 1 h and the 3D kernel size (vol) along a day-night period. The number of observations was ∼5,000 for acc and IQR but 440 for vol (according with the experimental settings detailed above). Accordingly, hereafter, a generic response variable (y) will be used for describing this general statistical model. Provided that the main goal was to isolate the enrichment-specific effects from the potentially confounding effects of other variables, the statistical model considered three levels of variability and a number of potential effects. At the lower level, the actually observed value of y at the time slot t (yt) was assumed to be normally distributed around an expected value for the fish i at the day j, with a given standard deviation (σ) in Eq. 1:
Preliminary exploration of the data strongly suggested that all the variables considered showed different variability depending on the time of the day. Accordingly, two different values were considered for, respectively, day-time (σday–time) and night-time (σnight–time). Note that repeated observations within a day are assumed to be independent (i.e., temporal autocorrelation is assumed to be absent). The expected value was modeled as a combination of fixed and random factors in Eq. 2:
where MEAN denotes the general mean across fish and days, FISHi accounts for the specific effects of the i fish (not included in the analysis of the 3D volume) and DAYj accounts for the specific effects of the day j. Concerning the fish-specific effects, two discrete levels were considered depending whether the i fish was moving at day-time (Eq. 3) vs. night-time (Eq. 4). Within a given discrete level, the fish-specific effect was modeled as a random factor:
Note that night-time and day-time effects for the i fish are allowed to be independent. For example, a given fish may be more active during night-time, but another fish may display the opposite pattern. Preliminary versions of the model considered the putative effects of fish weight at this level, but the results obtained suggested that these effects were irrelevant. Concerning the day-level effects, a random (unexplained) variability, a temperature-related fixed affect and an enrichment-related fixed effect were considered. Moreover, as in equation 3-4, day-time and night-time were allowed to differ. However, in sake of simplicity, only the day-time equations are shown hereafter:
where βday–time was the slope of a lineal effect of temperature, Temperaturej is the average temperature of the j day (note that the mean of Temperature in a given day was substracted from each Temperaturej for ensuring zero-intercept). Finally, ENRICHMENTday–time was the effect size attributable only to the enrichment of the cage. Provided that the cage was enriched from a given day, ENRICHMENTday–time was set to zero for the days prior to enrichment. Therefore, it was specifically evaluated if ENRICHMENTday–time after the day when the cage setting was modified was (or not) larger than zero.
The parameters of the integral model (i.e., combining Eqs 1–6) have been estimated in a single analysis using a Bayesian approach implemented in a custom R script1 that runs JAGS2 for moving the MCMC chains. Provided that three response variables were analyzed, a total of three independent analyses were done. Three independent chains were run in each analysis. The convergence of the MCMC chains was assessed by visual inspection of the chains and was evaluated using the Gelman-Rubin statistic (Gelman et al., 2013) (the expected value of the Rhat statistic after convergence should be close to 1). Virtually flat priors were used: normal distribution with zero mean and a huge variance were assumed for MEAN, βday–time,βnigth–time, ENRICHMENTday–time and ENRICHMENTnigth–time. Gamma distributions (rate = 0.01, scale = 0.01) were assumed for the inverse of five squared standard deviations considered (σday.–time, σnight–time, σFishday.–time, σFishnight–time, σDay). Posterior distributions of all the model parameters were estimated by at least 30.000 valid iterations after appropriate burning and thinning (one out 10 iteration were kept). The criteria chosen to say that an effect is relevant will be when 95% of the confidence interval does not include zero (Kruschke, 2014).
A total amount of 413,858 (depth and acceleration) valid receptions (99.94% of total detections) were recorded by the receiver array set in the experimental sea-cage during the whole monitoring period. Relevant differences (95% CI includes zero) between day and night were found on two tagged seabream regarding IQR depth, but no overall pattern was observed (Figure 1A). However, there were relevant differences on IQR depth for all tagged seabream (N = 10) throughout the experimental period (95% CI includes zero), and a clear pattern can be observed between the bare and enriched periods (Figure 1B). During the bare period (i.e., before adding EE), tagged fish showed similar patterns among individuals and a clear diurnal variation in group vertical movements, swimming closer to the surface during day-time (1.27 ± 0.65 m) compared to night time (1.94 ± 0.98 m) (Figures 2A,C). The range of swimming depths of the tagged fish was positively affected by environmental enrichment during the night (95% CI does not include zero) but not during the day (95% CI includes zero) (Table 1 and Figures 2B,D). Regarding swimming acceleration, relevant differences were found on two tagged seabream between day and night periods, but no overall pattern was observed (Figure 1C). Similarly, there were relevant differences between day and night for all tagged seabream during 6 days (95% CI includes zero), though no pattern can be observed throughout the experiment (Figure 1D). Acceleration values during the bare period were similar between day (0.91 ± 0.09 m s–2) and night (0.94 ± 0.14 m s–2) periods, although showing a W-shaped pattern throughout the day with maximum mean values at night (maximum value = 2.24 m s–2) and during the afternoon (maximum value = 2.38 m s–2) (Figure 2E). Regarding the presence of structural EE, our statistical model revealed no relevant effects on swimming acceleration (95% CI includes zero), which was negatively influenced by seawater temperature during the night (95% CI includes zero) but not during the day (95% CI does not include zero) (Table 1 and Figure 2F).
Figure 1. (A) Depth IQR 95% confidence interval (CI) of individual tagged fish during all day and night periods. (B) Depth IQR 95% confidence interval (CI) of all tagged fish (N = 10) throughout the experiment (in days) during day and night periods. (C) Acceleration 95% confidence interval (CI) of individual tagged fish during all day and night periods. (D) Acceleration 95% confidence interval of all tagged fish (N = 10) throughout the experiment (in days) during day and night periods. Relevant differences are marked with on-top orange dot. Note: green dashed line represents the beginning of the experimental period with environmental enrichment (EE).
Figure 2. Daily behavioral patterns of tagged gilthead seabream (N = 10) during the experiment: overall distribution of depth values during bare (A) and enriched (B) period; IQR depth distribution during bare (C) and enriched (D) period; and overall distribution of acceleration values during bare (E) and enriched (F) period. Boxes represent the interquartile range and the inside bar is the median. The lines extend to the maximum and minimum values.
Table 1. Distribution of the main parameters “posteriors” on acceleration and depth interquartile range (IQR): the median (or percentile 50%), 95% confidence interval (percentiles 2.5 and 97.5%), the convergence index of the chains (Rhat) and the number of effective iterations (n.eff).
Regarding 3D positioning, estimated error sensitivity of positions, based on the deviation between the calculated positions and known positions, was less than 1.5 m and covered more than 95% of the sea-cage area (Supplementary Figure S1). A total of 17,127 fish locations where successfully triangulated from the detection data (1,713 ± 382 locations per fish). The volumetric space use of individuals did not show any difference between day and night times during the bare period, while significant differences were observed during the enrichment period, where tagged fish showed greater vertical distribution during the night compared to the day (Figure 3). Estimates of 3D 50% daily activity space during bare period ranged from 6.75 to 70.50 m3 at day-time and from 2.75 to 68.75 m3 at night time; while during the enriched period ranged from 3.50 to 67.50 m3 at day-time and from 2.00 to 94.87 m3 at night time (Figure 4A). Estimates of 3D 95% daily activity space estimates ranged from 34.62 to 322.62 m3 at day and from 11.00 to 315.75 m3 at night; while during the enriched period ranged from 18.00 to 301.37 m3 at day-time and from 11.87 to 360.75 m3 at night time (Figure 4B). The daily overlap at 50% activity space among pairs of seabream individuals during the bare period ranged from 0 and 0.55 at day-time and from 0 to 0.35 at night time; while during the enriched period ranged from 0 to 0.50 at day-time and from 0 to 0.42 at night time (Figure 4C). The daily overlap at 95% activity space among pairs of seabream individuals during the bare period ranged from 0.08 to 0.53 at day-time and from 0.02 to 0.53 at night time; while during the enriched period ranged from 0 to 0.52 at day-time and from 0.03 to 0.48 at night time (Figure 4D). The range of 3D kernel size of the fish was positively affected by environmental enrichment during the night (95% CI no includes zero) but not during the day (95% CI includes zero) (Table 2).
Figure 3. Horizontal and vertical representation of 3D kernel utilization distributions (KUDs) for all the tagged fish at day (left) and night (right) time, during the bare (A) and enrichment period (B). Color gradient represents percentage of space utilization for all tagged fish.
Figure 4. Distribution of volume sizes and volumes in 50 and 95% over the time, day and night, in the bare period (blue) and enrichment period (orange). Boxes represent the interquartile range and the inside bar is the median. The lines extend to the values at 1.5 times the interquartile range, while the open circles represent potential outliers.
Table 2. Distribution of the main parameters “posteriors” on 3D kernel size (95% contour): the median (or percentile 50%), 95% confidence interval (percentiles 2.5 and 97.5%), the convergence index of the chains (Rhat) and the number of effective iterations (n.eff).
The combination of a receiver array and acoustic tags has provided for the first time detailed information on the swimming behavior and distribution of gilthead seabream directly in an experimental sea-cage. There was a clear variation in vertical movements and activity, which seems to be influenced by circadian rhythms. It has been shown that farmed gilthead seabream synchronizes their locomotor activity to both light and feeding phases, two main drivers of circadian rhythms (Bégout and Lagardère, 1995; López-Olmeda et al., 2009; Sánchez et al., 2009; Montoya et al., 2010). The natural activity rhythm of this species is diurnal, though fish can change their behavior to nocturnal when fed at night (Paspatis et al., 2000; López-Olmeda et al., 2009; Montoya et al., 2010; Vera et al., 2013; Paredes et al., 2014). Previous studies have reported seasonal variations in feeding patterns of gilthead seabream, in cold periods their activity is mainly nocturnal, while in warm periods their activity is diurnal (Paspatis et al., 2000; Velázquez et al., 2004; Vera et al., 2013; Paredes et al., 2014). Arechavala-Lopez et al. (2012) found that the diurnal variation of escaped gilthead seabream seemed to be influenced by the feeding time of the farms, at dawn, since seabreams remained close to the surface during night time and descended to greater depth in the morning. A previous study showed similar daily activity patterns on farmed seabream in an experimental cage, indicating that experimental seabream may have good ability to predict and time a re-occurring event such as feeding (Palstra et al., 2019). Feeding activity of seabream depends on individual learning capacity and stress copying style (Attia et al., 2012; Castanheira et al., 2017), but also on individual feeding motivation and social organization. The gilthead seabream is a schooling species which displays social hierarchies in terms of use of space and competition for food (Montero et al., 2009; Arechavala-Lopez et al., 2019, 2020b; Oikonomidou et al., 2019), which has been shown to be an important social mechanism affected by the size of the group, as well as by the food delivery rate and method (Karplus et al., 2000; Andrew et al., 2002, 2004; Sanchez-Muros et al., 2003, 2017; Sánchez et al., 2009). Although fish swimming behavior in farming cages has been addressed in many previous studies, this is the first work describing swimming activity (i.e., accelerations), positioning and distribution of gilthead seabream inside sea-cages in such detail. It must be noted that this study was carried out in a single cage, and therefore, it is not possible to evaluate the possible variability between cages (lack of replication), but the behavioral differences between tagged individuals reveal useful information on the behavior of fish under variable conditions. Circadian rhythms have been also documented for chinook salmon (Cubitt et al., 2003), Atlantic cod (Rillahan et al., 2009, 2011) or Atlantic salmon (Føre et al., 2011, 2018b; Kolarevic et al., 2016) in sea-cages using acoustic telemetry techniques. However, these rhythms are modified when there are changes in the environment. A previous study on cod showed that adding lights to extend the day photoperiod, and increasing stocking densities, causes changes in behavior and increases swimming activity (Rillahan et al., 2011). Similarly, another study (Kolarevic et al., 2016) documented a decrease in the total activity of S. salar because of changes in in-tank dissolved oxygen levels to hyperoxic and hypoxic conditions. It has also been shown that Atlantic salmon alter their activity rhythms during challenging operations such as crowding and delousing, as well as during normal cage management in aquaculture industry. It has been suggested that these operations induced increased swimming activity without the vertical distribution of the fish being affected (Føre et al., 2018b). In our study, adding structural EE did not provoke significant changes in swimming activity, in terms of accelerations, though vertical distribution of tagged fish seemed to be modified. Nevertheless, it should be noted that rearing density in this experiment was lower than in commercial-scale cages, and given the schooling and social behavior of seabream, fish distribution and spatial use inside the cage might be different at higher densities.
Estimated error sensitivity for fish positioning in experimental cages resulted less than 1.5 m (about 0.7 m in the center of the cage), which can be enhanced in bigger commercial sea-cages given that the distance between receivers plays an important role on the time of detections arrival. It must be noticed that noise and differences in signal magnitudes may have caused small offsets on registered detection time. Similarly, the high fish densities in commercial sea-cages could have an impact on the transmission conditions in the cage as the fish may attenuate the acoustic signals (Føre et al., 2018b), but also on the behavior and physiology of seabream (Carbonara et al., 2019). Therefore, further studies about the feasibility of acoustic telemetry in aquaculture cages are needed, which must take into account those aspects that may influence both behavior of the fish and the acoustic signal transmission/reception, such as fish species and size, holding conditions (e.g., stocking densities, cage dimensions), water characteristics (e.g., temperature, salinity, turbidity), monitoring period (seasonality), location of installations (e.g., background noise due to boat traffic), as well as receiver array design and positioning. Our volumetric analysis of 3D positions successfully described the actual space utilized by tagged seabream at low densities, allowing the study of fish distribution within the environment in which they live (Simpfendorfer et al., 2012). The 3D analysis showed that seabream used different areas of the water column during night, and a wider spatial distribution range in presence of structural enrichment (in terms of volume), without increasing the overlap activity space among individuals. It should be noted that fish received the meal automatically and continuously throughout the light period and, not surprisingly, always showed a clear preference for the surface during the day. The feeding regimes employed in fish farms have a strong influence on the social interactions (Andrew et al., 2002, 2004; Ashley, 2007) and differed from feeding method used in the present study. Thus, the length of the daily feeding period may also have influenced the gilthead seabream behavior. In addition, the 3D kernels suggest that the space distribution of the fish is denser in the central part of the sea-cage, so fish remained next to the enrichment structure and often away from the net. One study (Zimmermann et al., 2012) reported that the use of enrichment in aquaculture cages of cod may reduce the incidence of escapes by decreasing the inspection behavior near the net. Since farmed fish escaping from sea-cages represent an economic loss as well as a great problem for wild fish populations (Jensen et al., 2010; Arechavala-Lopez et al., 2017), the environmental enrichment might be an alternative to diminish impacts related to escaped fish. Nevertheless, a recent study on seabream demonstrated that EE enhance cognition and exploratory behavior (Arechavala-Lopez et al., 2020a), which might lead to easier finding the holes in the net through which to escape, although further studies are needed. Given the limitations of this study due to lack of replicates it cannot be ruled out that the observed effects of structural EE on seabream are caused by other factors non-accounted for (e.g., natural variations or fish adaptation to cage environment during the second part of the experiment). A previous experimental study on juvenile gilthead seabream demonstrated that structural enrichment expands the use of the captivity space, reducing aggressiveness and interactions with the net, which results in improving overall body condition (Arechavala-Lopez et al., 2019). In fact, in agreement to our results, structures may allow fishes to be positioned without disturbing others, increasing the inter-individual distance. Considering that fin damage and erosion are substantially greater under reared conditions than in the natural environment, possibly due to the high densities, feeding protocols and aquaculture operations (Arechavala-Lopez et al., 2013), fin abrasion may be minimized by enough space availability and by avoiding stress, especially during feeding (Person-Le Ruyet and Le Bayon, 2009). However, it must be noted that different stocking densities affect the swimming behavior of seabream at individual and group level (Carbonara et al., 2019; Arechavala-Lopez et al., 2020b). Therefore, this study highlights the necessity of further studies investigating not only the potential effects of structural EE at commercial scale, but also the swimming behavior and telemetry applications in diverse aquaculture conditions and systems.
This study provides for the first time information on swimming activity and distribution of gilthead seabream inside experimental sea-cages in high detail. The results demonstrated the potential feasibility of using acoustic telemetry for monitoring fish in commercial aquaculture and therefore it might be suggested as a tool for fish farmers to know in detail the specific behavior and distribution of fish in their facilities (Baras and Lagardère, 1995). The ongoing technological advances, mostly due to improvements on tag miniaturization, battery life, software and hardware, are rapidly expanding the possibilities of using biotelemetry sensors to accurately assess fish swimming behavior and movements (Hussey et al., 2015). Electronics tag can be equipped with several kinds of environmental and physiological sensors (e.g., depth, temperature, salinity, acceleration, heart and ventilation rates, muscle activity, etc.), which help to improve the knowledge of different aspects of the fish (Cooke et al., 2016). For instance, calibrating acoustic transmitters equipped with specific sensors (e.g., accelerometers, electromyograms, heart rate) with fish energy expenditure, will help to better-understand the metabolic costs of different activities and to assess what fish do to achieve energetically optimal behavior copying with stress under farming conditions (Wilson et al., 2013; Wright et al., 2014; Carbonara et al., 2015). In addition, acoustic transmitters allow the wirelessly and real-time transmission of data to the user through hydrophone receivers (Thorstad et al., 2013), as well as real-time monitoring with cabled receivers (Føre et al., 2018a), which allow fish farmers to monitor and react almost immediately to changes in group behavior (e.g., during feeding), and extending the study period and their feasibility at fish farms. Biotelemetry sensors may also be data storage/archival tags that store data in internal storage mediums that require retrieval of the logger to access the data, limiting their applicability and battery-life. However, all this knowledge is being recently transferred to the aquaculture sector improving the farmer’s ability to monitor, control and document biological processes in fish farms (i.e., Føre et al., 2018a), but further research is still needed before being applied at commercial scale. Gilthead seabream is one of the most important fish species for Mediterranean aquaculture, so a better understanding of its circadian rhythms and behavior in on-growing sea-cages is of great importance for the industry. With this knowledge, farmers may optimize the routine activities, feeding strategies and space in the farming-cages to improve both the performance and the welfare of their fish – an interesting example where the interest of the industry and the ethical concerns around its activity walk hand in hand (Saraiva and Arechavala-Lopez, 2019). Consequently, farmers would benefit not only in terms of efficiency, quality and quantity of production, but also regarding the reputation, public perception, marketing and acceptance of products. However, it is necessary to expand the knowledge of the biological requirements for captive fish species and thus determine the optimal conditions for their cultivation. It is important to continue with this research line, studying the applicability of the acoustic telemetry in the aquaculture industry, optimizing technological monitoring tools and the structures needed for each species and farming condition, to help improving welfare status of fish in captivity.
The raw data supporting the conclusions of this article will be made available by the authors, without undue reservation, to any qualified researcher.
The animal study was reviewed and approved by the Ethics Committee of Animal Experimentation of the University of Balearic Islands (Spain) – (CEEA-103/10/18).
LM: experiment development, data analysis, result processing, and manuscript elaboration. EA and MP: data analysis, result processing, and manuscript revisions. JS: result processing and manuscript revisions. PA-L: project lead, experimental design, manuscript elaboration and revision. All authors contributed to the article and approved the submitted version.
This study belongs to “SPYFISH” project, partially funded by the Edith Maryon Foundation (Basel, Switzerland); but also received Portuguese national funds from FCT – Foundation for Science and Technology through project UID/Multi/04326/2019 as well as from the Open Philanthropy Project through the “The CAREFISH program – Improving fish welfare through stakeholder communication: from research to fish farms” led by fair-fish international association (Denens, Switzerland).
The authors declare that the research was conducted in the absence of any commercial or financial relationships that could be construed as a potential conflict of interest.
This is a contribution of the Joint Research Unit IMEDEA-LIMIA. We would like to thank the staff of LIMIA for their help and assistance during the process of the study, and for sharing their facilities and resources in Port d’Andratx. We would also like to thank Dr. Ana Roque (Institute of Agrifood Research and Technology – IRTA) and Dr. Tanja Šegvić-Bubić (Croatian Institute of Oceanography and Fisheries – IZOR) for lending us the fish and part of the telemetry equipment respectively.
The Supplementary Material for this article can be found online at: https://www.frontiersin.org/articles/10.3389/fmars.2020.00645/full#supplementary-material
FIGURE S1 | Left: Schematic representation of the experimental cage, receivers and sync-tag location, and environmental enrichment used in this study (note that the image is not to scale). Right: Heatmap of error sensitivity for fish positioning estimated in the present study, which shows the mean deviation between the calculated position and the known positions (HDOP: Heatpoint Deviation of Optimal Position; in meters). The heatmap was generated by the manufacturers (PinPoint, Thelma Biotel) as follows: (i) a wide rectangular matrix (100 × 100 points) was made around the receiver grid (50 cm between each point); (ii) For every grid point, the signal travel time to each receiver was calculated. Thereafter, a random time error of + -1 ms-1 was added to the calculated times of arrival; (iii) the simulated travel times were used to create the time difference of arrival equations and calculate the positions; (iv) error sensitivity running a simulation 20 times for every grid point (new random error for each turn); (v) the deviation between the calculated positions and known positions were stored in a new solutions matrix and plotted in the heatmap matrix.
Alfonso, S., Sadoul, B., Cousin, X., and Bégout, M. L. (2020a). Spatial distribution and activity patterns as welfare indicators in response to water quality changes in European sea bass, Dicentrarchus labrax. Appl. Anim. Behav. Sci. 226:104974. doi: 10.1016/j.applanim.2020.104974
Alfonso, S., Zupa, W., Manfrin, A., Fiocchi, E., Dioguardi, M., Dara, M., et al. (2020b). Surgical implantation of electronic tags does not induce medium-term effect: insights from growth and stress physiological profile in two marine fish species. Anim. Biotel. 8, 1–6.
Andrew, J. E., Holm, J., Kadri, S., and Huntingford, F. A. (2004). The effect of competition on the feeding efficiency and feed handling behaviour in gilthead sea bream (Sparus aurata L.) held in tanks. Aquaculture 23, 317–331. doi: 10.1016/s0044-8486(03)00528-3
Andrew, J. E., Noble, C., Kadri, S., Jewell, H., and Huntingford, F. A. (2002). The effect of demand feeding on swimming speed and feeding responses in Atlantic salmon Salmo salar L., gilthead sea bream Sparus aurata L. and European sea bass Dicentrarchus labrax L. in sea cages. Aquacult. Res. 33, 501–507. doi: 10.1046/j.1365-2109.2002.00740.x
Arechavala-Lopez, P., Diaz-Gil, C., Saraiva, J. L., Moranta, D., Castanheira, M. F., Nuñez-Velázquez, S., et al. (2019). Effects of structural environmental enrichment on welfare of juvenile seabream (Sparus aurata). Aquac. Rep. 15:100224. doi: 10.1016/j.aqrep.2019.100224
Arechavala-Lopez, P., Caballero-Froilán, J. C., Jiménez-García, M., Capó, X., Tejada, S., Saraiva, J. L., et al. (2020a). Enriched environments enhance cognition, exploratory behaviour and brain physiological functions of Sparus aurata. Sci. Rep. 10:11252. doi: 10.1038/s41598-020-68306-6
Arechavala-Lopez, P., Nazzaro-Alvarez, J., Jardí-Pons, A., Reig, L., Carella, F., Carrassón, M., et al. (2020b). Linking stocking densities and feeding strategies with social and individual stress responses on gilthead seabream (Sparus aurata). Physiol. Behav. 213:112723. doi: 10.1016/j.physbeh.2019.112723
Arechavala-Lopez, P., Sanchez-Jerez, P., Izquierdo-Gomez, D., Toledo-Guedes, K., and Bayle-Sempere, J. T. (2013). Does fin damage allow discrimination among wild, escaped and farmed Sparus aurata (L.) and Dicentrarchus labrax (L.)? J. Appl. Ichthyol. 29, 352–357. doi: 10.1111/jai.12090
Arechavala-Lopez, P., Uglem, I., Fernandez-Jover, D., BayleSempere, J. T., and Sanchez-Jerez, P. (2012). Post-escape dispersion of farmed sea bream (Sparus aurata L.) and recaptures by local fisheries in the Western Mediterranean Sea. Fish. Res. 12, 126–135. doi: 10.1016/j.fishres.2012.02.003
Arechavala-Lopez, P., Uglem, I., Izquierdo-Gomez, D., Fernandez-Jover, D., and Sanchez-Jerez, P. (2017). Rapid dispersion of escaped meagre (Argyrosomus regius) from a coastal Mediterranean fish farm. Aquac. Res. 48, 1502–1512. doi: 10.1111/are.12986
Ashley, P. J. (2007). Fish welfare: current issues in aquaculture. Appl. Anim. Behav. Sci. 104, 199–235. doi: 10.1016/j.applanim.2006.09.001
Attia, J., Millot, S., Di-Poï, C., Bégout, M. L., Noble, C., Sanchez-Vazquez, F. J., et al. (2012). Demand feeding and welfare in farmed fish. Fish Physiol. Biochem. 38, 107–118. doi: 10.1007/978-94-007-5383-9_7
Baras, E., and Lagardère, J. P. (1995). Fish telemetry in aquaculture: review and perspectives. Aquac. Int. 3, 77–102. doi: 10.1007/bf00117876
Bégout, M. L., and Lagardère, J. P. (1995). An acoustic telemetry study of seabream (Sparus aurata L.): first results on activity rhythm effects of environmental variables and space utilization. Hydrobiology 301, 417–423. doi: 10.1007/978-94-011-0293-3_40
Broom, D. M. (2007). Welfare in relation to feelings, stress and health. REDVET Rev. Electrón. Vet. 8:12B.
Brydges, N. M., and Braithwaite, V. A. (2009). Does environmental enrichment affect the behaviour of fish commonly used in laboratory work? Appl. Anim. Behav. Sci. 118, 137–143. doi: 10.1016/j.applanim.2009.02.017
Carbonara, P., Alfonso, S., Zupa, W., Manfrin, A., Fiocchi, E., Pretto, T., et al. (2019). Behavioral and physiological responses to stocking density in sea bream (Sparus aurata): Do coping styles matter? Physiol. Behav. 212:112698. doi: 10.1016/j.physbeh.2019.112698
Carbonara, P., Scolamacchia, M., Spedicato, M. T., Zupa, W., McKinley, R. S., and Lembo, G. (2015). Muscle activity as a key indicator of welfare in farmed European sea bass (Dicentrarchus labrax L. 1758). Aquac. Res. 46, 2133–2146. doi: 10.1111/are.12369
Castanheira, M. F., Conceição, L. E., Millot, S., Rey, S., Bégout, M. L., Damsgård, B., et al. (2017). Coping styles in farmed fish: consequences for aquaculture. Rev. Aquac. 9, 23–41. doi: 10.1111/raq.12100
Cooke, S. J., Brownscombe, J. W., Raby, G. D., Broell, F., Hinch, S. G., Clark, T. D., et al. (2016). Remote bioenergetics measurements in wild fish: opportunities and challenges. Comp. Biochem. Physiol. A Mol. Integr. Physiol. 202, 23–37. doi: 10.1016/j.cbpa.2016.03.022
Cubitt, K. F., Churchill, S., Rowsell, D., Scruton, D. A., and McKinley, R. S. (2003). “3-dimensional positioning of salmon in commercial sea cages: assessment of a tool for monitoring behaviour,” in Proceedings of the fifth Conference on Fish Telemetry held in Europe Aquatic Telemetry. Advances and Applications, Ustica, 25–33.
Føre, M., Alfredsen, J. A., and Grønningsćter, A. (2011). Development of two telemetry-based systems for monitoring the feeding behaviour of Atlantic salmon (Salmo salar L.) in aquaculture sea-cages. Comp. Electron. Agric. 76, 240–251. doi: 10.1016/j.compag.2011.02.003
Føre, M., Frank, K., Dempster, T., Alfredsen, J. A., and Høy, E. (2017). Biomonitoring using tagged sentinel fish and acoustic telemetry in commercial salmon aquaculture: a feasibility study. Aquac. Eng. 78, 163–172. doi: 10.1016/j.aquaeng.2017.07.004
Føre, M., Frank, K., Norton, T., Svendsen, E., Alfredsen, J. A., Dempster, T., et al. (2018a). Precision fish farming: a new framework to improve production in aquaculture. Biosyst. Eng. 173, 176–193. doi: 10.1016/j.biosystemseng.2017.10.014
Føre, M., Svendsen, E., Alfredsen, J. A., Uglem, I., Bloecher, N., Sveier, H., et al. (2018b). Using acoustic telemetry to monitor the effects of crowding and delousing procedures on farmed Atlantic salmon (Salmo salar). Aquaculture 495, 757–765. doi: 10.1016/j.aquaculture.2018.06.060
Gelman, A., Carlin, J. B., Stern, H. S., Dunson, D. B., Vehtari, A., and Rubin, D. B. (2013). Bayesian Data Analysis. Boca Raton, FL: Chapman and Hall/CRC.
Horie, J., Mitamura, H., Ina, Y., Mashino, Y., Noda, T., Moriya, K., et al. (2017). Development of a method for classifying and transmitting high-resolution feeding behavior of fish using an acceleration pinger. Anim. Biotel. 5:12.
Hussey, N. E., Kessel, S. T., Aarestrup, K., Cooke, S. J., Cowley, P. D., Fisk, A. T., et al. (2015). Aquatic animal telemetry: a panoramic window into the underwater world. Science 348:6240.
Jensen, Ø, Dempster, T., Thorstad, E. B., Uglem, I., and Fredheim, A. (2010). Escapes of fishes from Norwegian sea-cage aquaculture: causes, consequences and prevention. Aquac. Environ. Interact. 1, 71–83. doi: 10.3354/aei00008
Karplus, I., Popper, D., and Goldan, O. (2000). The effect of food competition and relative size of group members on growth of juvenile gilthead sea bream, Sparus aurata. Fish Physiol. Biochem. 22, 119–123.
Kolarevic, J., Aas-Hansen, Ø, Espmark, Å, Baeverfjord, G., Terjesen, B. F., and Damsgård, B. (2016). The use of acoustic acceleration transmitter tags for monitoring of Atlantic salmon swimming activity in recirculating aquaculture systems (RAS). Aquac. Eng. 72, 30–39. doi: 10.1016/j.aquaeng.2016.03.002
Kruschke, J. (2014). Doing Bayesian Data Analysis: A Tutorial with R, JAGS, and Stan. Cambridge, MA: Academic Press.
Leclercq, E., Zerafa, B., Brooker, A. J., Davie, A., and Migaud, H. (2018). Application of passive-acoustic telemetry to explore the behaviour of ballan wrasse (Labrus bergylta) and lumpfish (Cyclopterus lumpus) in commercial Scottish salmon sea-pens. Aquaculture 495, 1–12. doi: 10.1016/j.aquaculture.2018.05.024
Lembo, G., Carbonara, P., Scolamacchia, M., Spedicato, M. T., and McKinley, R. S. (2007). “Use of muscle activity indices as a relative measure of well-being in cultured sea bass Dicentrarchus labrax (Linnaeus, 1758),” in Developments in Fish Telemetry, eds A. L. Moore, B. R. Quintella, P. R. Almeida, and M. J. Costa, (Dordrecht: Springer), 271–280. doi: 10.1007/978-1-4020-6237-7_25
López-Olmeda, J. F., Montoya, A., Oliveira, C., and Sánchez-Vázquez, F. J. (2009). Synchronization to light and restricted-feeding schedules of behavioral and humoral daily rhythms in gilthead sea bream (Sparus aurata). Chronobiol. Int. 26, 1389–1408. doi: 10.3109/07420520903421922
Martins, C. I. M., Galhardo, L., Noble, C., Damsgård, B., Spedicato, M. T., Zupa, W., et al. (2012). Behavioural indicators of welfare in farmed fish. Fish Physiol. Biochem. 38, 17–41. doi: 10.1007/978-94-007-5383-9_3
Montero, D., Lalumera, G., Izquierdo, M. S., Caballero, M. J., Saroglia, M., and Tort, L. (2009). Establishment of dominance relationships in gilthead sea bream Sparus aurata juveniles during feeding: effects on feeding behaviour, feed utilization and fish health. J. Fish Biol. 74, 790–805. doi: 10.1111/j.1095-8649.2008.02161.x
Montoya, A., López-Olmeda, J. F., Yúfera, M., Sánchez-Muros, M. J., and Sánchez-Vázquez, F. J. (2010). Feeding time synchronises daily rhythms of behaviour and digestive physiology in gilthead seabream (Sparus aurata). Aquaculture 306, 315–321. doi: 10.1016/j.aquaculture.2010.06.023
Näslund, J., and Johnsson, J. I. (2016). Environmental enrichment for fish in captive environments: effects of physical structures and substrates. Fish Fish. 17, 1–30. doi: 10.1111/faf.12088
Noble, C., Gismervik, K., Iversen, M. H., Kolarevic, J., Nilsson, J., Stien, L. H., et al. (2018). Welfare Indicators for Farmed Atlantic Salmon: Tools for Assessing Fish Welfare. Tromsø: Nofima.
Oikonomidou, E., Batzina, A., and Karakatsouli, N. (2019). Effects of food quantity and distribution on aggressive behaviour of gilthead seabream and European seabass. Appl. Anim. Behav. Sci. 213, 124–130. doi: 10.1016/j.applanim.2019.02.010
Palstra, A., Arechavala-Lopez, P., and Roque, A. (2019). “Accelerometry of seabream in a seacage: effects of flow conditioning on activity patterns, growth and robustness,” in Proceedings of the Book of Abstracts of the Aquaculture Europe 2019 Congress, Berlin, 1088–1089.
Paredes, J. F., Vera, L. M., Martinez-Lopez, F. J., Navarro, I., and Sánchez-Vázquez, F. J. (2014). Circadian rhythms of gene expression of lipid metabolism in Gilthead Sea bream liver: synchronisation to light and feeding time. Chronobiol. Int. 31, 613–626. doi: 10.3109/07420528.2014.881837
Paspatis, M., Maragoudaki, D., and Kentouri, M. (2000). Self-feeding activity patterns in gilthead sea bream (Sparus aurata), red porgy (Pagrus pagrus) and their reciprocal hybrids. Aquaculture 190, 389–401. doi: 10.1016/s0044-8486(00)00409-9
Person-Le Ruyet, J., and Le Bayon, N. (2009). Effects of temperature, stocking density and farming conditions on fin damage in European sea bass (Dicentrarchus labrax). Aquat. Liv. Res. 22, 349–362. doi: 10.1051/alr/2009047
R Core Team, (2019). R: A Language and Environment for Statistical Computing. Vienna: R Foundation for Statistical Computing.
Rillahan, C., Chambers, M., Howell, W. H., and Watson, W. H. III (2009). A self-contained system for observing and quantifying the behavior of Atlantic cod, Gadus morhua, in an offshore aquaculture cage. Aquaculture 293, 49–56. doi: 10.1016/j.aquaculture.2009.04.003
Rillahan, C., Chambers, M. D., Howell, W. H., and Watson, W. H. III (2011). The behavior of cod (Gadus morhua) in an offshore aquaculture net pen. Aquaculture 310, 361–368. doi: 10.1016/j.aquaculture.2010.10.038
Sánchez, J. A., López-Olmeda, J. F., Blanco-Vives, B., and Sánchez-Vázquez, F. J. (2009). Effects of feeding schedule on locomotor activity rhythms and stress response in sea bream. Physiol. Behav. 98, 125–129. doi: 10.1016/j.physbeh.2009.04.020
Sanchez-Muros, M. J., Corchete, V., Suarez, M. D., Cardenete, G., Gomez-Milan, E., and De la Higuera, M. (2003). Effect of feeding method and protein source on Sparus aurata feeding patterns. Aquaculture 224, 89–103. doi: 10.1016/s0044-8486(03)00211-4
Sanchez-Muros, M. J., Sánchez, B., Barroso, F. G., Toniolo, M., Trenzado, C. E., and Rus, A. S. (2017). Effects of rearing conditions on behavioural responses, social kinetics and physiological parameters in gilthead sea bream Sparus aurata. Appl. Anim. Behav. Sci. 197, 120–128. doi: 10.1016/j.applanim.2017.08.004
Saraiva, J. L., and Arechavala-Lopez, P. (2019). Welfare of fish—no longer the elephant in the room. Fishes 4:39. doi: 10.3390/fishes4030039
Šegvić-Bubić, T., Arechavala-Lopez, P., Vučić, I., Talijančić, I., Grubišić, L., Žužul, I., et al. (2018). Site fidelity of farmed gilthead seabream Sparus aurata escapees in a coastal environment of the Adriatic Sea. Aquac. Environ. Inter. 10, 21–34. doi: 10.3354/aei00251
Simpfendorfer, C. A., Olsen, E. M., Heupel, M. R., and Moland, E. (2012). Three-dimensional kernel utilization distributions improve estimates of space use in aquatic animals. Can. J. Fish. Aquatic Sci. 69, 565–572. doi: 10.1139/f2011-179
Sneddon, L. U., Wolfenden, D. C., and Thomson, J. S. (2016). “Stress management and welfare,” in Fish Physiology, eds C. B. Schreck, L. Tort, A. P. Farrell, and C. J. Brauner, (Academic Press), 463–539.
Summerfelt, R. C., and Smith, L. S. (1990). “Methods for fish biology,” in Anesthesia, Surgery and Related Techniques, eds C. B. Schreck, and P. B. Moyle, (Bethesda, MD: American Fisheries Society), 213–272.
Thorstad, E. B., Rikardsen, A. H., Alp, A., and Økland, F. (2013). The use of electronic tags in fish research - an overview of fish telemetry methods. Turk. J. Fish. Aquat. Sci. 13, 881–896.
Velázquez, M., Zamora, S., and Martínez, F. J. (2004). Influence of environmental conditions on demand-feeding behaviour of gilthead seabream (Sparus aurata). J. Appl. Ichthyol. 20, 536–541. doi: 10.1111/j.1439-0426.2004.00613.x
Vera, L. M., Negrini, P., Zagatti, C., Frigato, E., Sanchez-Vazquez, F. J., and Bertolucci, C. (2013). Light and feeding entrainment of the molecular circadian clock in a marine teleost (Sparus aurata). Chronobiol. Int. 30, 649–661. doi: 10.3109/07420528.2013.775143
Volpato, G. L., Goncalves-de-Freitas, E., and Fernandes-de-Castilho, M. (2007). Insights into the concept of fish welfare. Dis. Aquat. Org. 75, 165–117.
Wilson, S. M., Hinch, S. G., Eliason, E. J., Farrell, A. P., and Cooke, S. J. (2013). Calibrating acoustic acceleration transmitters for estimating energy use by wild adult Pacific salmon. Comp. Biochem. Physiol. A Mol. Integr. Physiol. 164, 491–498. doi: 10.1016/j.cbpa.2012.12.002
Wright, S., Metcalfe, J. D., Hetherington, S., and Wilson, R. (2014). Estimating activity-specific energy expenditure in a teleost fish, using accelerometer loggers. Mar. Ecol. Progr. Ser. 496, 19–32. doi: 10.3354/meps10528
Yoshida, N., Mitamura, H., Okamoto, H., and Arai, N. (2015). Measurement of activity for sit-and-wait predator, red-spotted grouper, using acoustic acceleration transmitter. J. Adv. Mar. Sci. Technol. Soc. 21, 1–5.
Zimmermann, E. W., Purchase, C. F., and Fleming, I. A. (2012). Reducing the incidence of net cage biting and the expression of escape-related behaviors in Atlantic cod (Gadus morhua) with feeding and cage enrichment. Appl. Anim. Behav. Sci. 14, 71–78. doi: 10.1016/j.applanim.2012.07.009
Keywords: accelerometers, daily activity, 3D positioning, spatial distribution, open-sea cages, aquaculture management
Citation: Muñoz L, Aspillaga E, Palmer M, Saraiva JL and Arechavala-Lopez P (2020) Acoustic Telemetry: A Tool to Monitor Fish Swimming Behavior in Sea-Cage Aquaculture. Front. Mar. Sci. 7:645. doi: 10.3389/fmars.2020.00645
Received: 26 March 2020; Accepted: 14 July 2020;
Published: 31 July 2020.
Edited by:
Morten Omholt Alver, Norwegian University of Science and Technology, NorwayReviewed by:
Pierluigi Carbonara, COISPA Tecnologia & Ricerca, ItalyCopyright © 2020 Muñoz, Aspillaga, Palmer, Saraiva and Arechavala-Lopez. This is an open-access article distributed under the terms of the Creative Commons Attribution License (CC BY). The use, distribution or reproduction in other forums is permitted, provided the original author(s) and the copyright owner(s) are credited and that the original publication in this journal is cited, in accordance with accepted academic practice. No use, distribution or reproduction is permitted which does not comply with these terms.
*Correspondence: Pablo Arechavala-Lopez, cGFibG9AZmFpci1maXNoLm5ldA==
Disclaimer: All claims expressed in this article are solely those of the authors and do not necessarily represent those of their affiliated organizations, or those of the publisher, the editors and the reviewers. Any product that may be evaluated in this article or claim that may be made by its manufacturer is not guaranteed or endorsed by the publisher.
Research integrity at Frontiers
Learn more about the work of our research integrity team to safeguard the quality of each article we publish.