- 1Antarctic Climate and Ecosystems Cooperative Research Centre, Hobart, TAS, Australia
- 2Institute for Marine and Antarctic Studies, College of Sciences and Engineering, University of Tasmania, Hobart, TAS, Australia
- 3CSIRO Oceans & Atmosphere, Hobart, TAS, Australia
- 4Centre for Marine Socioecology, University of Tasmania, Hobart, TAS, Australia
- 5Muséum nationald'Histoire naturelle, Sorbonne Universities, Départementdes milieu et peuplementsaquatiques, UMR7208 BOREA (MNHN-CNRS-UPMC-IRD-UCB), Paris, France
- 6Australian Antarctic Division, Hobart, TAS, Australia
Understanding the impacts of climate and fishing on marine systems is important for ecosystem-based management in the Southern Ocean, but can be difficult to evaluate due to patchy data in space and time. We developed the first time-dynamic food web model for the Kerguelen Plateau using Ecopath with Ecosim to explore likely drivers of change in this relatively data-poor region. The Kerguelen Plateau is located at the centre of intersecting frontal systems and is inhabited by one of the largest populations of the commercially important Patagonian toothfish. We used this model to evaluate the environmental and human drivers of food web dynamics in the region by calibrating it with French and Australian fisheries data from 1997–2018 and biomass data for the period 1986–2018. Fishing was not identified as a driver of food web dynamics within this model, which could indicate that current management strategies are sustainable. A correlation analysis with environmental parameters likely to drive food web dynamics (sea surface temperature, zonal wind, Southern Annular Mode and chlorophyll a concentration) highlighted cool sea surface temperature, higher zonal wind speeds and negative phases of the Southern Annular Mode as important drivers of change, particularly during the summer. As the Southern Ocean is predicted to warm and winds are expected to intensify under future climate change, our study illustrates the importance of considering environmental change in ecosystem management.
1. Introduction
The Commission for the Conservation of Antarctic Marine Living Resources (CCAMLR) uses an ecosystem-based approach to maintaining sustainability, therefore it is important to understand the environmental drivers of the food web (Constable et al., 2014). Food webs in the Southern Ocean are coupled to the environment at a multitude of timescales. Predator foraging for example, can occur on timescales of minutes to hours while environmental processes such as eddy dynamics can happen at monthly to yearly scales (Fulton et al., 2019). Food web dynamics themselves also vary; energy gained through phytoplankton blooms can be tracked via peaks in zooplankton biomass and seen months or years later in mid-trophic levels (e.g., fish) and Southern Ocean predators (Bannister et al., 2015; Goedegebuure et al., 2017).
The Kerguelen Plateau, located in the sub-Antarctic Indian Ocean (Figure 1), intersects with the frontal systems of the Antarctic Circumpolar Current (ACC) (Park et al., 2014). Driven by strong westerly winds, the ACC brings water of varying temperatures (0.5–3°C) to the Kerguelen Plateau (Park et al., 2014; Mintenbeck, 2017). Upwelling leads to annual phytoplankton blooms that support breeding and foraging predators (Thiers et al., 2017) and the largest fishery for Patagonian toothfish (Brooks et al., 2016).
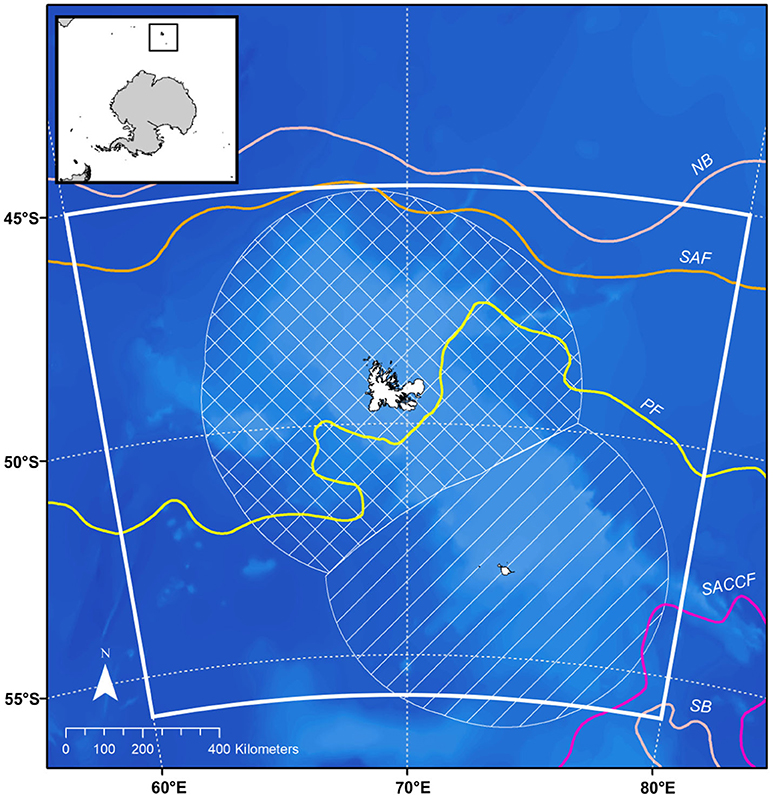
Figure 1. Map of the Kerguelen Plateau, model domain (60°–80°E, 45°–56°S) is indicated by the white box, the hatched area indicates the French EEZ, striped area indicates the Australian EEZ. Front positions and boundaries of the Antarctic Circumpolar Current (ACC) as described in Park et al. (2014), bathymetry data are from https://www.gebco.net. NB, northern boundary of the ACC; SAF, subantarctic front; PF, polar front; SACCF, southern ACC front; SB, southern boundary of the ACC.
The plateau is divided into two regions: the northern region, denoted by the hatched area in Figure 1 is a French Exclusive Economic zone (EEZ) while the southern region, denoted by the striped area in Figure 1, is an EEZ for Australia. Direct fishing for Patagonian toothfish (Dissostichus eleginoides) in the French EEZ began as a trawl fishery in 1985 and longline fishing began in 1991. The fishery has been longline only since 2001 and is active all year except late summer (February-March) to avoid seabird mortality (Duhamel et al., 2011). Australia also operates a commercial fishery from May to November in the southern region. Fishing for Patagonian toothfish in the Australian EEZ began as a trawl fishery in 1997, longline fishing began in 2003 and gradually replaced trawling. A trawl fishery for mackerel icefish (Champsocephalus gunnari) was initiated at the same time as the toothfish and continues today (from December–November). Both EEZs are located within the CCAMLR area and while Australian catch limits are agreed through consensus in CCAMLR (Mintenbeck, 2017), French catch limits are decided unilaterally.
There is limited understanding regarding how changes to the environment may impact the food web and commercial fishing (Rayfuse, 2018; Meredith et al., 2019). Sea surface temperature (SST) can affect the growth rate, population structure, prey availability, and breeding performance of predators in this region (e.g., Guinet et al., 1998; Lescroël and Bost, 2006; Pardo et al., 2017; Cristofari et al., 2018). Other observations reveal that strong westerly winds have improved foraging performance and breeding success of albatrosses in regions adjacent to the Kerguelen Plateau (Weimerskirch et al., 2012). SST and wind on the Kerguelen Plateau have been linked to large-scale climate processes such as the Southern Annular Mode (SAM) (e.g., Lovenduski and Gruber, 2005). The SAM index is an important climate driver in the Southern Ocean and is dictated by the position of strong westerly winds (Bost et al., 2015). However, the SAM has not yet been related to food web dynamics. Positive SAM phases indicate a contraction of the winds poleward and negative phases indicate an expansion of the wind belt toward the equator (Rogers et al., 2020). This strong band of wind affects SST and oceanographic processes on the Kerguelen Plateau via upwelling or downwelling (Su et al., 2020), influencing food web interactions.
Patagonian toothfish are a slow growing, long-lived species, which makes them vulnerable to over-fishing. Recruitment is influenced by the environment (such as SST) making them susceptible to future climate change (Mintenbeck, 2017). Correlating food web dynamics with climate requires consistent observations, which is difficult on the Kerguelen Plateau due to its remote location and harsh environmental conditions. Food web models are a useful medium for exploring potential climatic impacts on ecosystems and for informing ecosystem-based management practices (Fulton et al., 2019).
Ecopath with Ecosim (EwE, Christensen and Walters, 2004) is a useful tool to explore the combined environmental and human drivers of food web dynamics that are important for ecosystem-based management. EwE is suited to data-limited regions such as the Kerguelen Plateau as it estimates values where there are gaps in time-series data and uses food web interactions to generate trends for other functional groups where data are absent. Furthermore, this method can identify sensitive trophic interactions and approximates an environmental trend that can be assessed for correlation with climate trends.
In this study, we use EwE to calibrate the Ecopath model presented in Subramaniam et al. (2020) with time-series data to investigate environmental and human impacts on the Kerguelen Plateau food web.
2. Methods
2.1. Ecosim
EwE is a commonly-used software for developing food web models. The Ecopath module of EwE provides a snapshot of the food web through two master equations that balance the energy input and output of the food web (see Subramaniam et al., 2020). The balanced Ecopath model can then be calibrated to time-series data in Ecosim.
Biomass dynamics in Ecosim are described through a series of coupled simultaneous equations:
where represents the growth rate of group i during the time period t, gi is net growth efficiency (production/consumption), Fi is fishing mortality rate, Mi is other mortality rate, ei is emigration rate, Ii is immigration rate and Bi is biomass. The consumption rates Qij and Qji are calculated based on a simple “foraging arena” model while biomasses (Bi) are split into components that are vulnerable and invulnerable to predation.
The performance of the model is assessed by calculating the weighted sum of squares (SS) between the log observed data and model output (Christensen et al., 2008).
2.2. Ecosim Input and Calibration
We used the balanced Ecopath model described in Subramaniam et al. (2020) as the baseline Ecosim model. The model is comprised of 28 functional groups, organized based on similar diets and life cycles. A visual representation of this model illustrates the structure of the food web and associated trophic levels (Figure 2) and balanced model parameters are provided in Tables A1, A2. As the Kerguelen Plateau is a data-poor system, Subramaniam et al. (2020) provided an analysis of food web dynamics averaged over a period of 10 years, but also used data dating back to the late 1980s for some species. Therefore, this model can be seen as a representation of the mean state of the ecosystem from the late 1980s to 2018. A similar approach has been used for Ecosim models in other regions of the Southern Ocean (e.g., Dahood et al., 2019).
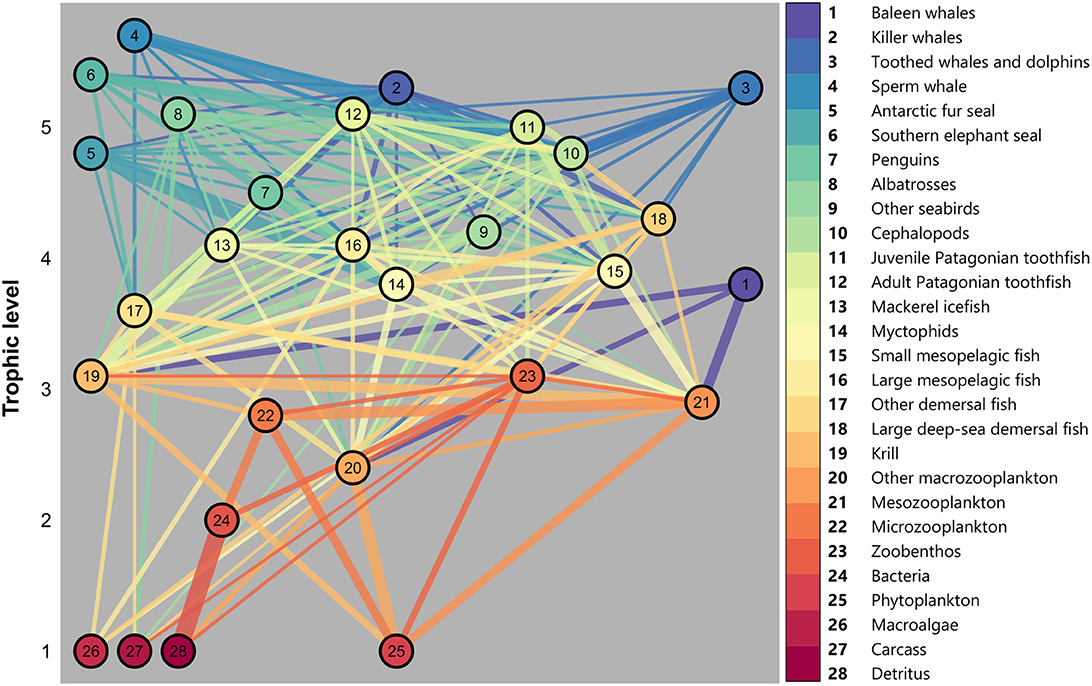
Figure 2. Energy flow through each trophic level on the Kerguelen Plateau. Node number represents functional groups listed on the right, linkages between groups represent diet of each group (colored from predator to prey) and width of linkage indicates proportion of diet (see Subramaniam et al., 2020 for details).
Trawl surveys on the Kerguelen Plateau have shown large fluctuations in the biomass of some fish species over this time period (e.g., Notothenia rossii, Duhamel et al., 2019). However, it is unclear if these fluctuations have affected food web dynamics. Other observational studies in this region suggest no major directional change over the time period considered in this model (e.g., Authier et al., 2011; Weimerskirch et al., 2018). Based on this information, the aims of our study were to investigate patterns of variability between the environment and the food web.
We used time-series data that were available and of sufficient length to calibrate the model. We used population trends for southern right whales (Bannister et al., 2015), southern elephant seals (Authier et al., 2011), black-browed albatrosses (Weimerskirch et al., 2018), and Patagonian toothfish and mackerel icefish fisheries data to parameterize the model. A full description of these data is presented in Table A3.
In the model, juvenile and adult Patagonian toothfish were partitioned as having a length less than or greater than 75 cm based on previous studies (Pruvost et al., 2005; Collins et al., 2007). Although juveniles may actually mature later in life on the Kerguelen Plateau (Yates et al., 2018), we have chosen to remain consistent with the commonly-used size separation for juveniles. Length-frequency analysis in fishery reports (CCAMLR, 2018a,b) and minimum legal catch limits (60 cm) indicate that toothfish under 75 cm are caught during the fishing season but it is unclear how much of the catch is less than 75 cm. Consultation with fisheries scientists was used to inform an estimated allocation of catch to juveniles.
Trawl fishing for Patagonian toothfish catches mostly immature fish while longline fishing catches mostly mature fish. To account for this difference in selection, time-series of nominal catch per unit effort (CPUE, kg per hook) and total catch for each season was allocated 28% to juveniles and the remainder to adults for longlines and trawl CPUE and catch was allocated to juveniles (Philippe Ziegler, Australian Antarctic Division, pers. comm). Fishing mortality was used to drive fishing activity in Ecosim using model estimated biomass for Patagonian toothfish (Table A3), this was calculated as:
Calibration of the model with time-series data was performed using the step-wise fitting procedure described in the EwE best practices guideline (Heymans et al., 2016). Previously, step-wise fitting was done manually however, an automated plug-in of this procedure was developed and recently implemented into the EwE software (Scott et al., 2016). We opted to use the automated plug-in to calibrate the model. The step-wise fitting procedure adjusts vulnerabilities and a primary production anomaly (PP anomaly) for a set of scenarios (see Figure 3).
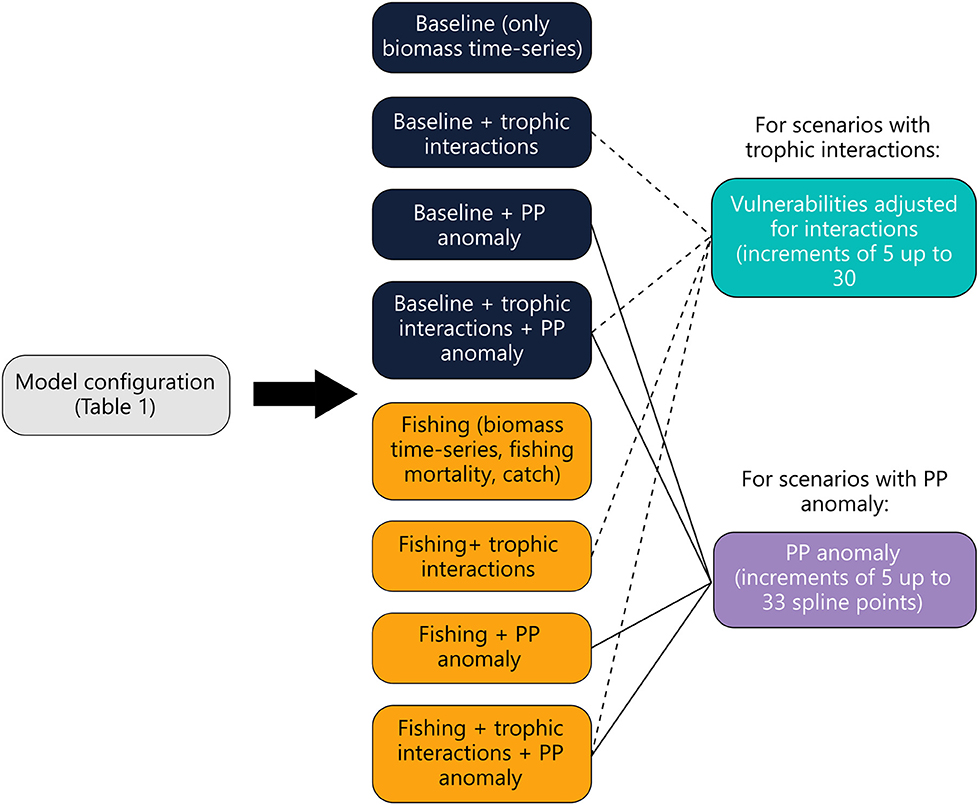
Figure 3. Diagram showing how Ecosim was used to calibrate the model. Eight scenarios were run (dark blue and yellow) for each model configuration in Table 1. Baseline scenarios (dark blue) do not include fisheries data. For scenarios including trophic interactions (blue) and PP anomalies (purple), each scenario was run by increasing the number of vulnerabilities estimated for trophic interactions and PP anomaly spline points by 5 until the maximum was reached (5, 10, 15…etc).
The vulnerability parameter describes interactions between predators and prey in the model. A value in the range 1–100 is attributed to each interaction to dictate how consumption is influenced by changes in predator and prey biomass. Values close to 1 indicate bottom-up control while values close to 100 indicate that top-down influences dominate (Christensen et al., 2008). The PP anomaly parameter represents (non-modeled) bottom-up control on the ecosystem (Scott et al., 2016), and typically represents some environmental influence on phytoplankton. For each version of the model there were a possible 172 trophic interactions that could be tuned to calibrate the model. We found that the maximum number of interactions that would elicit a noticeable change in the sum of squares was 30 interactions (or vulnerabilities). Therefore, we restricted our scenarios to a maximum of 30 vulnerabilities. Model fit using step-wise fitting is evaluated by calculating the Akaike information criterion (AIC) and penalizing for fitting too many parameters based on the number of time-series available resulting in a weighted AIC value (AICc) (Scott et al., 2016). We used a two-step approach to select the best-fitting model by first selecting the models that were statistically the best fit (had the lowest AICc values) then plotting and using a pattern oriented approach (Kramer-Schadt et al., 2007; Heymans et al., 2016).
Our data compilation resulted in biomass trends and fishing data for different periods of time. Biomass data were mostly available for the entire time period, however, fishing data were only available for shorter periods (Table A3). Additionally, due to the evolving preferences for different types of fishing gear when targeting Patagonian toothfish, there were three CPUE inputs that could be used as a proxy for toothfish biomass trends in the model. To cover potential biases resulting from data gaps, the step-wise fitting procedure was performed using four different configurations of the model (shown in Table 1). All four model configurations were very similar, only differing in the length of time calibrated or CPUE and fisheries data input.
For scenarios where CPUE inputs were averaged across French and Australian EEZs, only the trawl CPUE was used to approximate the trend for juveniles and 72% of longline CPUE was used for the adults. Juvenile catch and fishing mortality were averaged over gears (trawl and 28% of the longline catch for the French and Australian EEZ) and adult longline catch (72% of the longline catch for the French and Australian EEZ) and fishing mortality were averaged for these scenarios (Models 2 and 4, Table 1).
For the best fitting model scenario, Monte Carlo simulations were run within EwE to test the sensitivity of the Ecosim model to the base Ecopath model input as an additional measure to validate our approach. The Monte Carlo routine randomly selects starting biomass values for functional groups based on the coefficient of variation (CV) and calculates a new sum of squares based on the new initial values. The default CV in Ecosim is ±10% however, we tested ±20% Patagonian toothfish biomass as the starting condition for our Ecosim model. We varied toothfish biomass as there is particular uncertainty about the biomass of this group in the late 1980s (Duhamel and Hautecoeur, 2009; Duhamel et al., 2019).
We correlated the PP anomaly trend from the best fitting model with chlorophyll a, wind, sea surface temperature (SST) and the Southern Annular Mode index (SAM) taken from satellite data (sources provided in Table A4). Estimated PP anomaly and biomass trends from the model were given as monthly averages. To match the model output, climate variables were also derived from satellite data as monthly averages using the white bounding box in Figure 1. We examined correlations between the model output from the best-fitting scenario, both monthly and annual averages, and anomalies of remotely sensed data. Environmental variables were also correlated with each other to test the strength of each correlation.
Seasonal anomalies were calculated for summer (December to May of the following year) and winter (June to November of the same year) by calculating the average over the time series then subtracting this value from the seasonal (summer or winter) average for each year. Seasonal anomalies were compared to the summer and winter average biomass for each group. For each set of anomalies (monthly, annual and seasonal), a moving average was calculated in R using the “forecast” package with a 5-step moving window (Hyndman and Khandakar, 2008).
A Spearman's rank correlation was used to test for significance between model output and climate anomalies. P-values were adjusted in R using the “p.test” function and the false discovery rate method (Jafari and Ansari-Pour, 2019). We tested environmental correlations against the PP anomaly trend, phytoplankton, juvenile and adult Patagonian toothfish, other macrozooplankton and krill biomass. Due to the size and value of the Patagonian toothfish fishery, and the large-scale removal of Patagonian toothfish by fisheries on both regions of the Kerguelen Plateau, we focus on evaluating climate impacts on Patagonian toothfish in this study.
Recruitment of Patagonian toothfish is variable from year to year and most likely indirectly related to SST (Mintenbeck, 2017; Yates et al., 2018). While there have been no observational studies examining recruitment variability on the Kerguelen Plateau, studies from other regions of the Southern Ocean suggest this could be important for predicting changes to toothfish populations (e.g., Laptikhovsky and Brickle, 2005). The Patagonian toothfish displays a long life cycle: adults spawn during austral winter (May-August), eggs incubate for 3 months and hatch in pelagic waters. Juveniles inhabit pelagic waters until 13–15 months of age where they start to settle in benthic habitats (< 500 m) (Péron et al., 2016). Adults are usually found at depths greater than 600 m (Mintenbeck, 2017). Due to this long life cycle, climate impacts on recruitment may be lagged.
Successful spawning during austral winter may only be observed in the juvenile population once they start to become benthopelagic at 1+ years old (Belchier and Collins, 2008). Belchier and Collins (2008) found a 2 year lag between summer SST and successful recruitment of juvenile Patagonian toothfish. Based on this finding we tested for lagged effects up to 2 years between toothfish groups and climate variables that did not show a significant correlation in the above analysis. We moved climate variables forward (equivalent to lagging toothfish biomass) by two, three and four seasons, corresponding to a 1, 1.5 and 2 year lag, respectively. We performed a correlation analysis between the moved climate variables and toothfish biomass trends.
3. Results
Model configuration 3 demonstrated the best fit of the four model configurations under consideration (model 3, Table 1). This was unsurprising as model 3 covered the longer time period, allowing for more data points to fit to. This model covered the entire time period and incorporated all three CPUE inputs for Patagonian toothfish. The best-fitting scenarios from this model involved adjusting vulnerabilities and estimating PP anomalies. All model fits are presented in Table A5.
Model 3 was improved when trophic effects and PP anomalies were included in the model. The best-fitting scenario set vulnerabilities for 5 trophic interactions (Table A6) and 7 PP anomaly spline points (Figure A2). Importantly, the best-fitting model did not include fishing as a driver of food web dynamics. The model reproduced the general trends for toothfish groups, however, observed trends for baleen whales, southern elephant seals, albatrosses and mackerel icefish were not well-represented (Figure A1). Therefore, we sought alternative vulnerabilities for use in the model.
We chose to use alternative vulnerabilities from model 1, which had the most comprehensive data coverage. The best-fitting scenario for model 1 was achieved when only trophic effects were included (see Table A5). Similarly to model 3, the best fitting scenario for model 1 estimated vulnerabilities for 5 interactions. We replaced the vulnerabilities shown in Table A6 with those in Table 2 and ran the scenario again to calibrate the model.
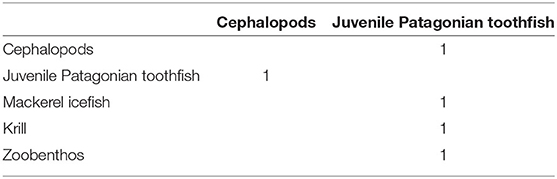
Table 2. Vulnerabilities estimated in the best-fitting scenario for model 1 that were used in model 3.
The replacement vulnerabilities produced improved estimates for Patagonian toothfish groups and mackerel icefish compared to observations (Figure 4). We also compared both model fits to a study representing population trends for predators (killer whales, toothed whales and dolphins, sperm whales, other seabirds and Antarctic fur seals) using data collected by fishery-observers (Gasco et al., 2019). Our comparison showed that replacing the vulnerabilities provided a better representation of the observer data presented in Gasco et al. (2019) (see Table A7 in Appendix).
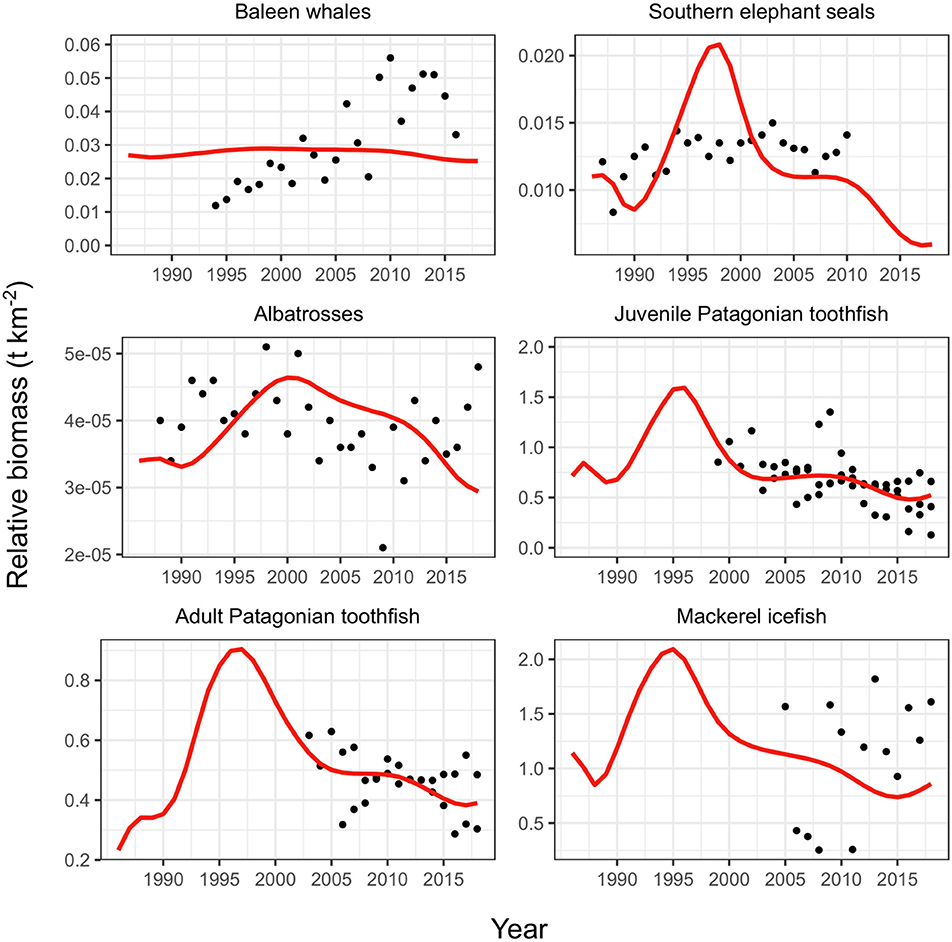
Figure 4. Fit of model to observations with replaced vulnerabilities (Table 2). Red line shows model fit, black points show observations. Toothfish observations are three CPUE inputs as described in Table 1.
Results from our sensitivity analysis indicated that while changing the initial conditions did result in increased or decreased biomass throughout the time series, this occurred in a consistent manner (i.e., more biomass at the start of the time series led to more biomass at the end of the time series). The pattern of biomass trends for both toothfish and other functional groups from this analysis show that our model was not sensitive to initial conditions (Figure 5).
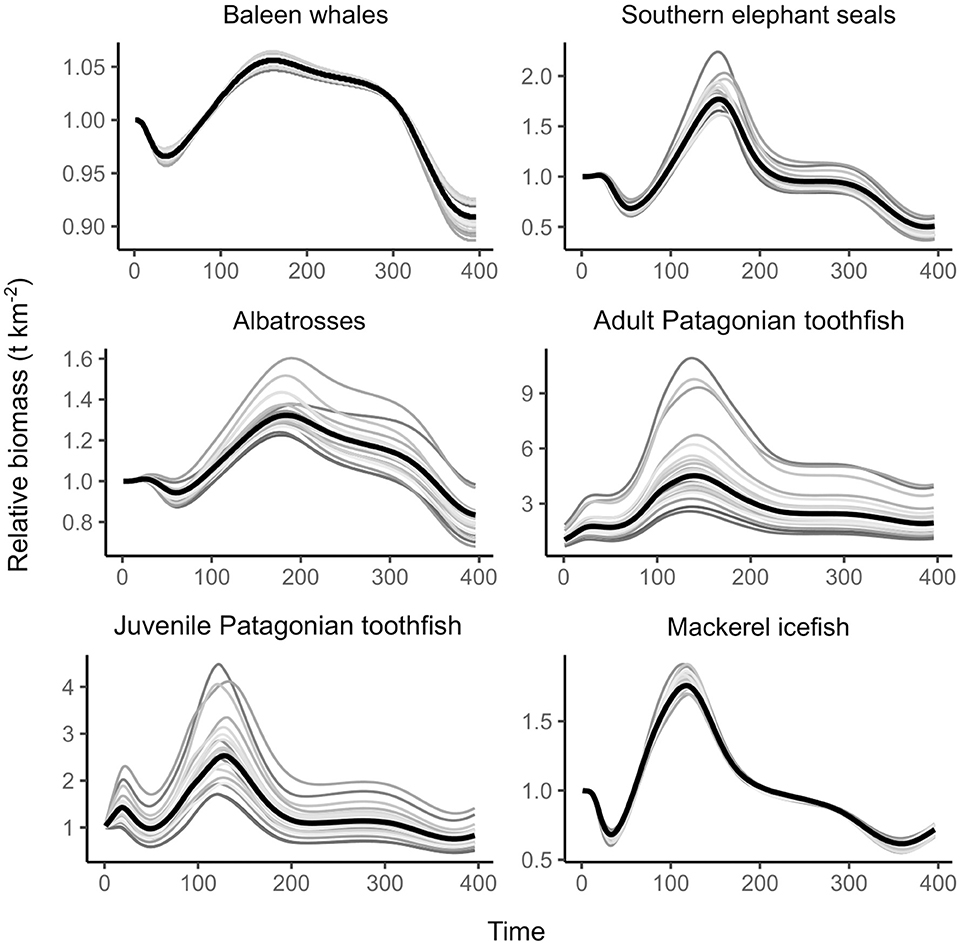
Figure 5. Results from the Monte Carlo simulations for the best-fitting model. Gray lines represent the biomass trend for each of the 20 simulations and the black lines overlay the biomass trend from the best-fitting model. Time is provided in months where January 1986 = 1.
3.1. Correlation With Environmental Variables
Correlations between environmental variables showed that SST significantly correlated with the SAM at the annual timescale and summer season while zonal wind only significantly correlated with the SAM during summer. SST and zonal were not significantly correlated with each other at any timescale (Table 3). These differing correlations support our investigation of individual variables with modeled biomass trends.
SST, zonal wind and SAM were correlated with zooplankton and toothfish biomass trends estimated by the best-fitting model. Correlations between estimated biomass and monthly anomalies for climate variables were lower than those with annual and seasonal anomalies. Annual and seasonal anomalies are more likely to be drivers of biological response over time than monthly anomalies, therefore we focus on these results for the remainder of this study. Results from correlations with monthly anomalies are presented in the Appendix (Table A8).
PP anomaly and zooplankton biomass trends correlated negatively with annual SST and SAM, and positively with annual zonal wind anomalies. Biomass increased during periods when SST was cooler than average (1990–1994 and 2005–2007, Figure 6) and declined during periods when SST was warmer than average (e.g., 1994–1999, Figure 6). SST did not appear to correlate with juvenile and adult Patagonian toothfish, however there may be a lagged effect (discussed below). Zonal wind correlated with all functional groups: estimated biomass trends increased during periods where surface wind speed was faster than average, and decreased during periods where surface wind speed was slower than average (Figure 7). PP anomaly and estimated biomass trends for phytoplankton, macrozooplankton, and krill increased during periods of negative SAM anomaly, indicating that westerly winds were expanding further north than average (Figure 8).
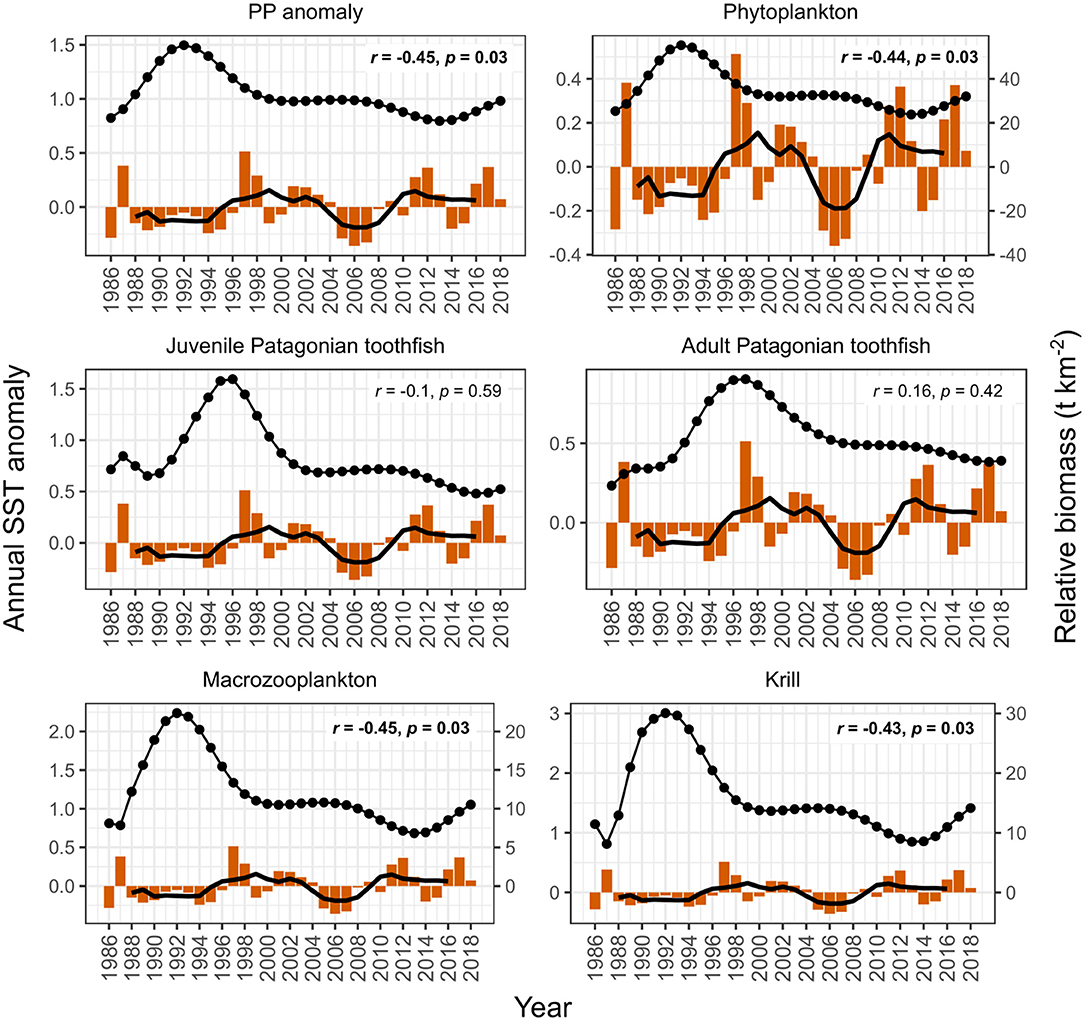
Figure 6. Comparison of model output with annual sea surface temperature (SST) anomalies (orange bars). Black points are the estimated annual biomass values for each year of the model period and black line indicates moving average used to perform correlations (relative biomass in t km−2, right axis for phytoplankton, macrozooplankton, and krill). Strength of correlations are shown by the r and p values in each plot, with significant correlations in bold.
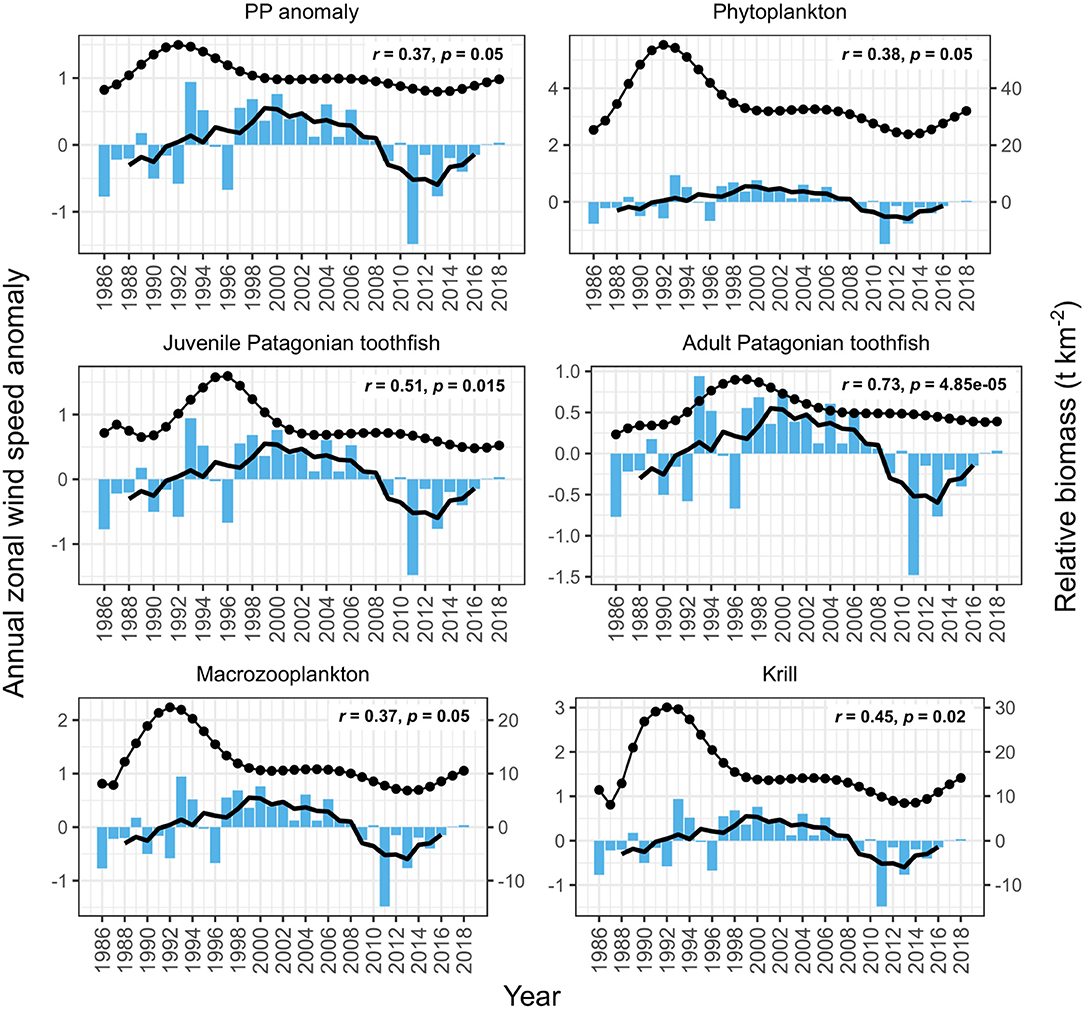
Figure 7. Comparison of model output with annual zonal wind anomalies (blue bars). Black points are the estimated annual biomass values for each year of the model period and black line indicates moving average used to perform correlations (relative biomass in t km−2, right axis for phytoplankton, macrozooplankton and krill). Strength of correlations are shown by the r and p values in each plot, with significant correlations in bold.
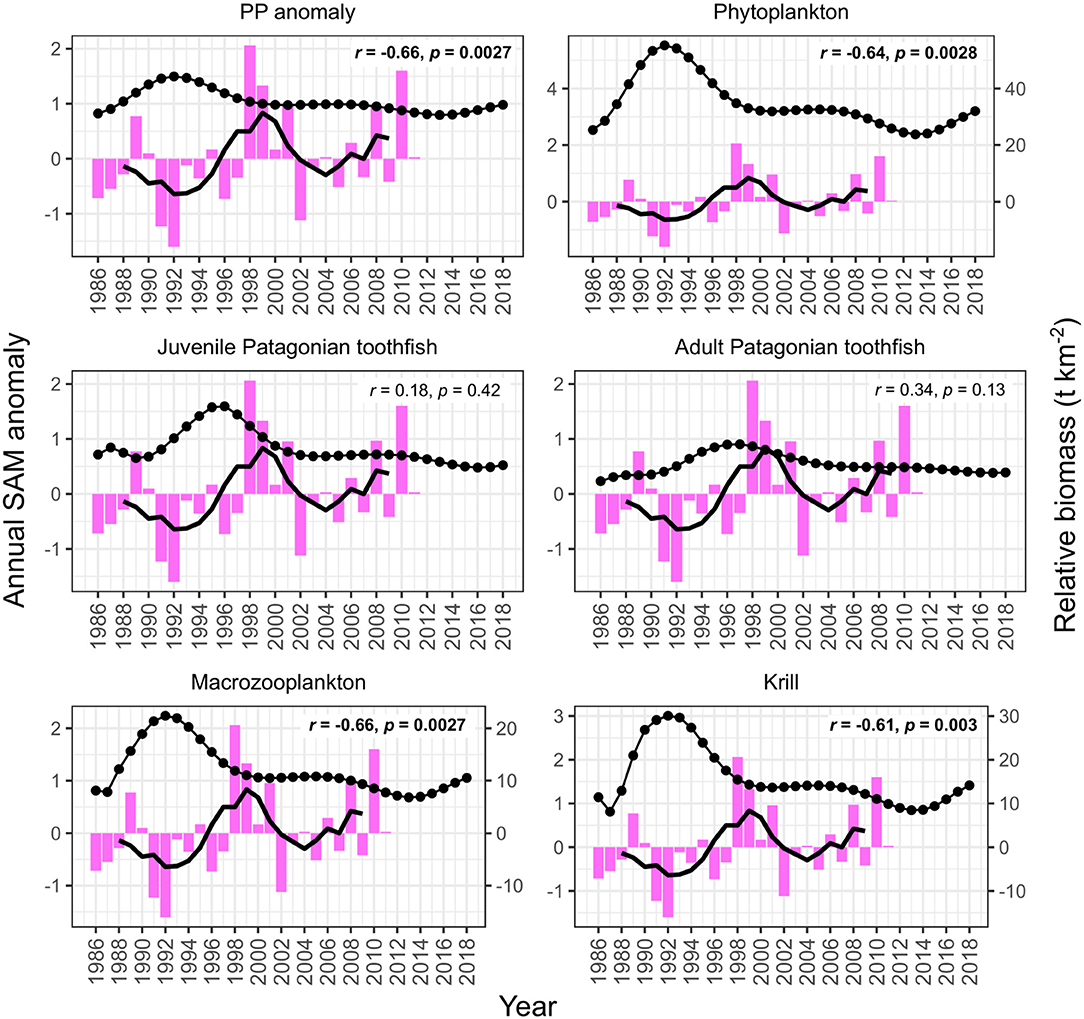
Figure 8. Model output correlations to the Southern Annular Mode (SAM) (pink bars). Black points are the estimated annual biomass values for each year of the model period and black line indicates moving average used to perform correlations (relative biomass in t km−2, right axis for phytoplankton, macrozooplankton, and krill). Strength of correlations are shown by the r and p values in each plot, with significant correlations in bold.
Summer SST correlated better with PP anomaly, phytoplankton, macrozooplankton and krill biomass trends (Table 4). Similar to annual SST, summer SST demonstrated a negative correlation with model variables. Biomass peaked during periods when summer SST was cooler than average and declined during periods when summer SST was warmer than average. Again, we found no correlation between summer SST and toothfish groups, which may be because of a lagged effect (discussed below). Estimated biomass trends were uncorrelated with winter SST (Table 4). Summer zonal wind correlated positively with biomass trends: biomass increased during periods when summer surface wind speed was faster than average and decreased during periods when summer surface wind was slower than average (Table 4). Winter wind positively correlated with only adult Patagonian toothfish (Table 4). Both summer and winter SAM exhibited a negative correlation with PP anomaly, phytoplankton, macrozooplankton and krill. In contrast, summer SAM correlated positively with adult Patagonian toothfish (Table 4).
We tested for lagged effects between juvenile Patagonian toothfish and summer SST, winter SST, winter zonal wind, summer SAM and winter SAM as they did not show a significant correlation in the above analysis. We also tested for lagged effects of adult Patagonian toothfish with summer SST, winter SST and winter SAM. We present only significant correlations in our results. Correlations appeared to be strongest at the three season and four season lag. Our results show a negative correlation between lagged juvenile Patagonian toothfish biomass and summer SST at all three seasonal lags, with the strongest correlation at the four season lag (Figure 9). Lagged juvenile biomass also correlated negatively with summer and winter SAM with strongest correlations at the three and four season lag. Lagged adult Patagonian toothfish biomass correlated negatively with summer SST at the four season lag and winter SAM at all three seasonal lags with the strongest at the three season lag (Figure 9).
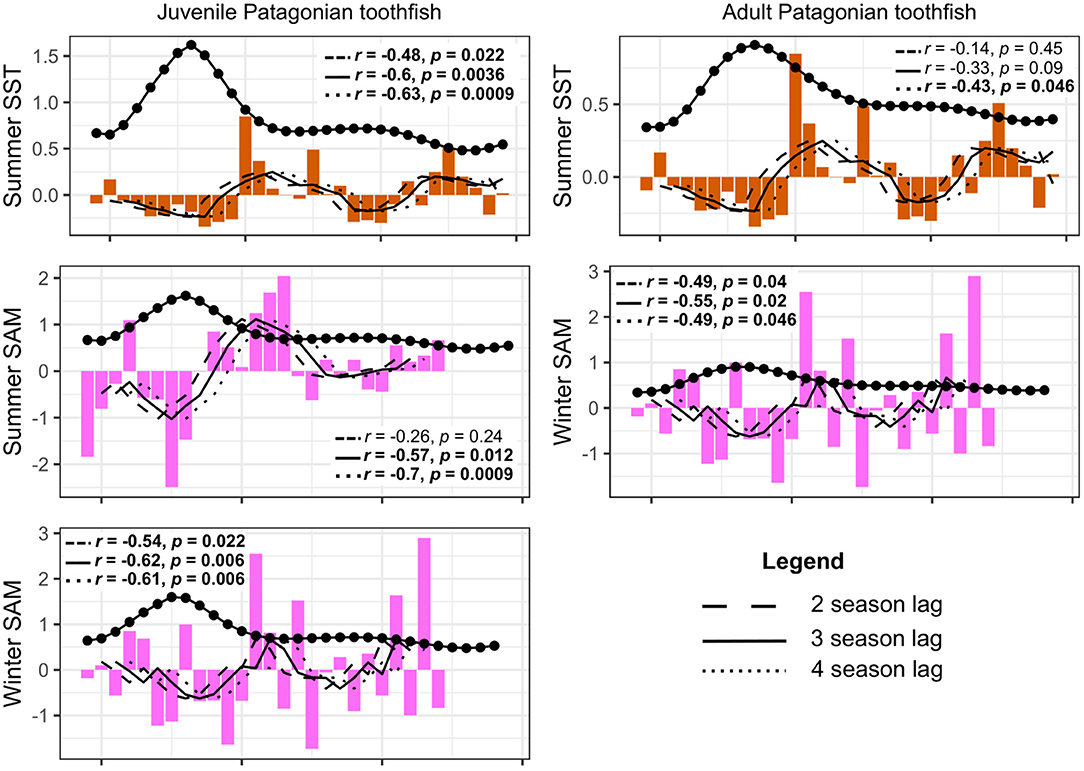
Figure 9. Lagged toothfish biomass correlations to summer SST, summer SAM and winter SAM. Biomass was lagged at two (dashed line), three (solid line), and four time-steps (dotted line), equivalent to 1, 1.5, and 2 year lags, respectively. R and p values are shown in plots with associated lagged time-step, significant correlations are shown in bold.
4. Discussion
4.1. Model Evaluation
Our analysis presents the first attempt at calibrating food web dynamics on the Kerguelen Plateau with trends in observed time-series. We acknowledge that this approach to model fitting is not standard practice in the EwE community. We used this approach to cover biases in data availability and focus our interpretations on patterns of interannual variability instead of directional change. We implemented several models which reproduced the observed data with varying success. The best-fitting model reproduced observed biomass trends for juvenile Patagonian toothfish, adult Patagonian toothfish and mackerel icefish better than other model versions and reproduced observed trends for groups with no input data (see Appendix). The model also partially recreated trends for southern elephant seals and albatrosses although it could not recreate the trend for baleen whales.
Ecosim models are fitted to the data by tuning parameters (vulnerabilities and PP anomaly trends) to show which values could explain observed historical trends (Alexander et al., 2014). Consequently, the ability of the model to fit to observed data is improved with good quality data sets. In situations where data quality is poor, the model may struggle to converge on an accurate solution. The inability of the preferred model to completely represent observed trends for marine predators could be a result of data quality and the inability to incorporate all possible drivers of change. Data on population trends for baleen whales on the Kerguelen Plateau are lacking (Dréo et al., 2019). The data used to represent baleen whales was taken from an aerial survey of Australian southern right whale population. Aerial surveys are known to have large biases therefore, not much confidence was placed in the data (Marsh and Sinclair, 1989). Additionally, this trend contributed very little to the overall fit of the model. Population trends of southern elephant seals may be related to their life history and may be too complex to capture in aggregated models (Goedegebuure et al., 2018). Southern elephant seals also forage outside of the Kerguelen Plateau (Delord et al., 2014). Declines in albatrosses have been linked to demersal longline fisheries, and particularly illegal, unregulated and unreported (IUU) fisheries by-catch (Michael et al., 2019), especially during the 1990s and early 2000s (Weimerskirch et al., 2018). However, estimates of seabird mortality due to IUU are unavailable. Climatic conditions outside of the Kerguelen Plateau during winter may further contribute to observed population trends for albatrosses as they winter away from the Kerguelen Plateau (Weimerskirch et al., 2018).
Fishing was not identified as a driver of food web dynamics over this time period, indicating that in our model representation, fishing activity is currently sustainable. This is consistent with conclusions of sustainable fishing from a recent assessment of management of marine protected areas in the southern region of the Kerguelen Plateau (Brooks et al., 2019). In addition to fishing activity occurring much later into the time series, more than one gear has operated during this time. Therefore, it is possible that either the direct impacts of fishing are too subtle for the model to capture or the effects have not yet traveled through the food web. Moreover, we were unable to incorporate any fishing activity (trawl and longline) in the French EEZ prior to 2005, nor were we able to include IUU fishing activity due to data quality and/or data unavailability. Despite these limitations, the model was useful for evaluating large-scale environmental drivers of food web dynamics.
4.2. Environmental Drivers of Food Web Dynamics
Our results highlight environmental (or bottom-up control) as an important driver of food web dynamics on the Kerguelen Plateau. Peaks in phytoplankton and zooplankton biomass correlated with negative annual SST anomalies, increasing annual zonal wind speed and a negative SAM index. When seasonality was considered, phytoplankton and zooplankton biomass positively correlated with summers that exhibited cooler temperatures and higher wind speeds. Their biomasses generally correlated with negative SAM anomalies during summer and winter.
Cool surface waters combined with high wind speeds may enhance vertical mixing on the Kerguelen Plateau as strong winds can increase the depth of the mixed layer and bring cold, nutrient-rich water into the euphotic zone (Blain et al., 2007; Gille et al., 2014). This is not typical of negative SAM anomalies in the Southern Ocean (see discussion below). Observations indicate that during austral summer, cold and well-mixed waters are dominated by large phytoplankton on the Kerguelen Plateau (Quéguiner, 2013). The resulting zooplankton composition is dominated by large copepods, leading to a short, energy-efficient pathway for predators (Quéguiner, 2013; Venkataramana et al., 2019). Results from our comparison between model output and environmental variables are consistent with these observations.
Other factors such as negative wind stress curl can influence Ekman pumping and further enhance upwelling (Gille et al., 2014; Panassa et al., 2018). However, wind stress curl and Ekman pumping behave differently depending on location. South of 50°S, Ekman pumping drives upwelling while north of 50°S, Ekman pumping can induce downwelling (Gille et al., 2014). Nevertheless, wind stress curl on the Kerguelen Plateau has been useful for investigating marine heatwaves, an important climate extreme that may become more frequent in the future (Oliver et al., 2018). Wind stress curl and Ekman pumping during the winter following a marine heatwave led to downwelling, allowing warmer water to penetrate further down the water column and for the heatwave to persist longer (Su et al., 2020). Wind stress curl was not included in our study, but may be considered in future analyses with the model.
Local environmental conditions are closely linked to large-scale climate drivers (Jones et al., 2016). In our study, cold SST and periods of higher than average wind speed were related to negative SAM phases. This contrasts with other climate studies correlating negative SST anomalies with positive SAM phases across the Southern Ocean (Lovenduski and Gruber, 2005). As indicated above, the oceanography of the Kerguelen Plateau is complex and spatially heterogenous. Lovenduski and Gruber (2005) suggested that positive SAM phases in the southern Indian Ocean lead to cold upwelling in the Antarctic Zone (south of the Polar Front) and downwelling in the sub-Antarctic zone (40–50°S). Other spatial investigations of these trends found that ocean temperature on the Kerguelen Plateau was significantly correlated with the El Niño Southern Oscillation in the north and the SAM index over the whole plateau (Su et al., 2020). Future analyses may need to incorporate spatial variation in order to identify the areas where the food web will be most affected by these changes.
Food web dynamics significantly correlated with summer environmental trends and this is consistent with other studies showing strong summer environmental trends (Lovenduski and Gruber, 2005; Swart and Fyfe, 2012; Panassa et al., 2018; Fonseca and Martín-Torres, 2019; Meredith et al., 2019). Stronger correlations during summer between our model output and environmental data could be a result of the trends used to calibrate the model. Some of the observed annual trends were derived from animal counts during the breeding season in summer (e.g., Weimerskirch et al., 2018). However, other trends used in this study were not derived from counts during the breeding season (e.g., the CPUE data) and outputs from the preferred model reproduced annual trends from other studies (Gasco et al., 2019, see Appendix). Therefore, if there was any bias toward summer from the trend data, it may not be strong. Summer is an important season for the Kerguelen Plateau food web; seabirds and seals breed and forage during this time of year (Delord et al., 2014). A positive summer SAM trend has occurred over the last decade (Panassa et al., 2018; Meredith et al., 2019) and our model output suggests that continued positive SAM events could reduce the productivity of the food web, especially with regards to the Patagonian toothfish.
4.3. Environmental Impacts on the Patagonian Toothfish
Peaks in juvenile biomass correlated positively with annual and summer zonal wind. Peaks in adult biomass correlated positively with winter zonal wind anomalies and positively with summer SAM. Mixing induced by zonal wind could increase prey availability for toothfish, leading to increased biomass. The positive correlation seen with summer SAM could be indicative of downwelling on the Kerguelen Plateau, increasing prey availability for adult Patagonian toothfish located deeper into the water column. This result could be related to the catchability of toothfish rather than biomass itself as CPUE was used as a proxy for biomass. Increased prey availability can affect fish behavior and make toothfish more vulnerable to being caught (Arreguín-Sánchez, 1996; Parkes et al., 1996).
When we investigated time-lagged correlations, we found stronger correlations when we introduced a three and four season lag (equivalent to 1.5 and 2 year lag, respectively). Lagged juvenile biomass correlated negatively with summer SST and summer and winter SAM anomalies. Lagged adult biomass correlated negatively with summer SST and winter SAM at the four season lag.
Our model output produced the strongest correlation between juvenile toothfish and summer SST at the four season lag (2 year lag). This is consistent with results reported by Belchier and Collins (2008) who identified summer SST impacts on juveniles. Juvenile toothfish abundance (older than one year) in South Georgia was inversely correlated with summer SST experienced by adults prior to spawning. Belchier and Collins (2008) suggested that prey availability linked to large-scale climatic processes could be driving this variability. This is consistent with our study as lagged juvenile biomass correlated with the SAM, which has been identified as the underlying driver of prey biomass in our model.
There is no clear reason for the lagged correlations seen between adult toothfish biomass, summer SST and winter SAM. This could be related to catchability in the model. However, studies have reported toothfish showing strong year classes every 4–5 years (Laptikhovsky and Brickle, 2005) and perhaps further separation of toothfish into specific age classes may clarify these correlations. Untangling these relationships will help provide reliable estimates of life history traits that are required for effective management of the fishery (Yates et al., 2018).
5. Conclusion
Our study found that fishing was not a driver of food web dynamics and supports other recent findings of sustainable current fishing of Patagonian toothfish on the Kerguelen Plateau, with respect to effects on both toothfish biomass and food web dynamics. Our findings also highlight the importance of bottom-up processes driving the food web for the last 40 years. Cold and well-mixed waters lead to productive food web dynamics on the Kerguelen Plateau and these conditions appear to be linked to negative SAM phases, based on our model results. A continued positive SAM trend, as has been observed over the last decade, could lead to reduced prey availability and reduced predator populations. However, due to the spatial heterogeneity in oceanographic processes on the Kerguelen Plateau, more work is needed to clarify these effects. Our results also suggest that Patagonian toothfish recruitment is influenced by the environment, which is an important consideration for fisheries management, particularly if patterns of variability change under future climate change.
Data Availability Statement
The data analyzed in this study is subject to the following licenses/restrictions: All data that were not provided under a confidentiality agreement are provided in the article. The fisheries data (CPUE, catch and model estimated biomass) were provided under a confidentiality agreement and therefore cannot be made publicly available. The data are available upon request and the fishing bodies that provided the data are cited in the article. Requests to access these datasets should be directed to Clara Péron, clara.peron@mnhn.fr, Philippe Ziegler, Philippe.Ziegler@awe.gov.au.
Author Contributions
RS was responsible for conceptualization, methodology, writing, editing, data visualization, and project administration. JM-T was responsible for conceptualization, methodology, reviewing, editing, and supervision. SC was responsible for conceptualization, reviewing, editing, and supervision. CP provided the data. PZ provided data and advice on methodology. KS was responsible for conceptualization, reviewing, editing, and supervision. All authors contributed to the article and approved the submitted version.
Funding
This study was supported by Australian Government's Cooperative Research Centres Programme through the Antarctic Climate and Ecosystems Cooperative Research Centre, the Institute for Marine and Antarctic Studies (IMAS) through the Australian Antarctic Science Programme (AAS 4347) and through the IMAS and University of Tasmania (UTAS) Tasmanian Graduate Research Scholarship.
Conflict of Interest
The authors declare that the research was conducted in the absence of any commercial or financial relationships that could be construed as a potential conflict of interest.
Acknowledgments
The authors would like to thank the Muséum national d'Histoire naturelle for providing the fisheries data for the French EEZ and the Australian Fisheries Management Association for providing fisheries data for the Australian EEZ. We would also like to thank the reviewers for their helpful comments on the development of this manuscript and Ben Raymond for his help with R. The following packages were used to generate the figures presented in this paper: ggplot2 (Wickham, 2016), RColorBrewer (Neuwirth, 2014), and gridExtra (Auguie, 2017). This study was supported by Australian Government's Cooperative Research Centres Programme through the Antarctic Climate and Ecosystems Cooperative Research Centre, the Institute for Marine and Antarctic Studies (IMAS) through the Australian Antarctic Science Programme (AAS 4347) and through the IMAS and University of Tasmania (UTAS) Tasmanian Graduate Research Scholarship.
Supplementary Material
The Supplementary Material for this article can be found online at: https://www.frontiersin.org/articles/10.3389/fmars.2020.00641/full#supplementary-material
References
Alexander, K. A., Heymans, J. J., Magill, S., Tomczak, M. T., Holmes, S. J., and Wilding, T. A. (2014). Investigating the recent decline in gadoid stocks in the west of Scotland shelf ecosystem using a foodweb model. ICES J. Mar. Sci. 72, 436–449. doi: 10.1093/icesjms/fsu149
Arreguín-Sánchez, F. (1996). Catchability: a key parameter for fish stock assessment. Rev. Fish Biol. Fish. 6, 221–242. doi: 10.1007/BF00182344
Auguie, B. (2017). gridExtra: Miscellaneous Functions for “Grid” Graphics. R package version 2.3. Available online at: https://CRAN.R-project.org/package=gridExtra
Authier, M., Delord, K., and Guinet, C. (2011). Population trends of female elephant seals breeding on the Courbet Peninsula, îles kerguelen. Polar Biol. 34, 319–328. doi: 10.1007/s00300-010-0881-1
Bannister, J. L., Hammond, P. S., and Double, M. C. (2015). Population Trend in Right Whales Off Southern Australia 1993-2015. International Whaling Commission, SC/66b/BRG/09.
Belchier, M., and Collins, M. A. (2008). Recruitment and body size in relation to temperature in juvenile Patagonian toothfish (Dissostichus eleginoides) at south Georgia. Mar. Biol. 155, 493–503. doi: 10.1007/s00227-008-1047-3
Blain, S., Queguiner, B., Armand, L., Belviso, S., Bombled, B., Bopp, L., et al. (2007). Effect of natural iron fertilization on carbon sequestration in the southern ocean. Nature 446, 1070–1074. doi: 10.1038/nature05700
Bost, C. A., Cotté, C., Terray, P., Barbraud, C., Bon, C., Delord, K., et al. (2015). Large-scale climatic anomalies affect marine predator foraging behaviour and demography. Nat. Commun. 6:8220. doi: 10.1038/ncomms9220
Brooks, C. M., Crowder, L. B., Curran, L. M., Dunbar, R. B., Ainley, D. G., Dodds, K. J., et al. (2016). Science-based management in decline in the southern ocean. Science 354:185. doi: 10.1126/science.aah4119
Brooks, C. M., Epstein, G., and Ban, N. C. (2019). Managing marine protected areas in remote areas: the case of the Subantarctic heard and Mcdonald islands. Front. Mar. Sci. 6:631. doi: 10.3389/fmars.2019.00631
CCAMLR (2018a). Fishery Report 2018: Dissotichus eleginoides Kerguelen islands, French EEZ (division 58.5.1). Report, Commission for the Conservation of Antarctic Marine Living Resources.
CCAMLR (2018b). Fishery Report 2018: Dissotichus eleginoides Heard island, Australian EEZ (division 58.5.2). Report, Commission for the Conservation of Antarctic Marine Living Resources.
Christensen, V., and Walters, C. J. (2004). Ecopath with Ecosim: Methods, capabilities and limitations. Ecol. Modell. 172, 109–139. doi: 10.1016/j.ecolmodel.2003.09.003
Christensen, V., Walters, C. J., Pauly, D., and Forrest, R. (2008). Ecopath with Ecosim Version 6 User Guide. Lenfest Ocean Futures Project, 1–235.
Collins, M. A., Ross, K. A., Belchier, M., and Reid, K. (2007). Distribution and diet of juvenile Patagonian toothfish on the south Georgia and shag rocks shelves (southern ocean). Mar. Biol. 152, 135–147. doi: 10.1007/s00227-007-0667-3
Constable, A. J., Melbourne-Thomas, J., Corney, S. P., Arrigo, K. R., Barbraud, C., Barnes, D. K. A., et al. (2014). Climate change and southern ocean ecosystems I: how changes in physical habitats directly affect marine biota. Glob. Change Biol. 20, 3004–3025. doi: 10.1111/gcb.12623
Cristofari, R., Liu, X., Bonadonna, F., Cherel, Y., Pistorius, P., Le Maho, Y., et al. (2018). Climate-driven range shifts of the king penguin in a fragmented ecosystem. Nat. Clim. Change 8, 245–251. doi: 10.1038/s41558-018-0084-2
Dahood, A., Watters, G. M., and de Mutsert, K. (2019). Using sea-ice to calibrate a dynamic trophic model for the western Antarctic peninsula. PLoS ONE 14:e0214814. doi: 10.1371/journal.pone.0214814
Delord, K., Barbraud, C., Bost, C., Cherel, Y., Guinet, C., and Weimerskirch, H. (2014). Atlas of Top Predators From French Southern Territories in the Southern Indian Ocean. CEBC-CNRS.
Dréo, R., Bouffaut, L., Leroy, E., Barruol, G., and Samaran, F. (2019). Baleen whale distribution and seasonal occurrence revealed by an ocean bottom seismometer network in the western Indian ocean. Deep Sea Res. II Top. Stud. Oceanogr. 161, 132–144. doi: 10.1016/j.dsr2.2018.04.005
Duhamel, G., and Hautecoeur, M. (2009). Biomass, abundance and distribution of fish in the Kerguelen islands EEZ (CCAMLR statistical division 58.5.1). CCAMLR Sci. 16, 1–32. Available online at: https://www.ccamlr.org/en/publications/science_journal/ccamlr-science-volume-16/ccamlr-science-volume-161-32
Duhamel, G., Péron, C., Sinègre, R., Chazeau, C., and Gasco, N. (2019). “Important readjustments in the biomass and distribution of groundfish species in the northern part of the Kerguelen plateau and skiff bank,” in The Kerguelen Plateau: Marine Ecosystem and Fisheries. Proceedings of the Second Symposium, Proceedings of the Second Symposium, eds D. Welsford, J. Dell, and G. Duhamel (Hobart, TAS), 135–184.
Duhamel, G., Pruvost, P., Bertignac, M., Gasco, N., and Hautecoeur, M. (2011). “Major fishery events in Kerguelen islands: Notothenia rossi, Champsocephalus gunnari, Dissostichus eleginoides-current distribution and status of stocks,” in The Kerguelen Plateau: Marine Ecosystem and Fisheries, eds G. Duhamel and D. C. Welsford (Hobart, TAS: Société française d'ichtyologie), 275–286.
Fonseca, R., and Martín-Torres, J. (2019). High-resolution dynamical downscaling of re-analysis data over the Kerguelen islands using the WRF model. Theoret. Appl. Climatol. 135, 1259–1277. doi: 10.1007/s00704-018-2438-0
Fulton, E. A., Blanchard, J. L., Melbourne-Thomas, J., Plagányi, E. E., and Tulloch, V. J. D. (2019). Where the ecological gaps remain, a modelers' perspective. Front. Ecol. Evol. 7:424. doi: 10.3389/fevo.2019.00424
Gasco, N., Tixier, P., Delord, K., Guinet, C., and Duhamel, G. (2019). “Scientific observation program around Kerguelen island, a ship-of-opportunity for bird and mammal data collection,” in The Kerguelen Plateau: Marine Ecosystem and Fisheries. Proceedings of the Second Symposium, Proceedings of the Second Symposium, eds D. Welsford, J. Dell, and G. Duhamel (Hobart, TAS), 365–379.
Gille, S. T., Carranza, M. M., Cambra, R., and Morrow, R. (2014). Wind-induced upwelling in the Kerguelen plateau region. Biogeosciences 11, 6389–6400. doi: 10.5194/bg-11-6389-2014
Goedegebuure, M., Melbourne-Thomas, J., Corney, S. P., Hindell, M. A., and Constable, A. J. (2017). Beyond big fish: the case for more detailed representations of top predators in marine ecosystem models. Ecol. Modell. 359, 182–192. doi: 10.1016/j.ecolmodel.2017.04.004
Goedegebuure, M., Melbourne-Thomas, J., Corney, S. P., McMahon, C. R., and Hindell, M. A. (2018). Modelling southern elephant seals mirounga leonina using an individual-based model coupled with a dynamic energy budget. PLoS ONE 13:e0194950. doi: 10.1371/journal.pone.0194950
Guinet, C., Chastel, O., Koudil, M., Durbec Jean, P., and Jouventin, P. (1998). Effects of warm sea-surface temperature anomalies on the blue petrel at the Kerguelen islands. Proc. R. Soc. Lond. Ser. B Biol. Sci. 265, 1001–1006. doi: 10.1098/rspb.1998.0390
Heymans, J. J., Coll, M., Link, J. S., Mackinson, S., Steenbeek, J., Walters, C., and Christensen, V. (2016). Best practice in ecopath with ecosim food-web models for ecosystem-based management. Ecol. Modell. 331, 173–184. doi: 10.1016/j.ecolmodel.2015.12.007
Hyndman, R. J., and Khandakar, Y. (2008). Automatic time series forecasting: the forecast package for R. J. Stat. Softw. 26, 1–22. doi: 10.18637/jss.v027.i03
Jafari, M., and Ansari-Pour, N. (2019). Why, when and how to adjust your p values? Cell J. 20, 604–607. doi: 10.22074/cellj.2019.5992
Jones, J. M., Gille, S. T., Goosse, H., Abram, N. J., Canziani, P. O., Charman, D. J., et al. (2016). Assessing recent trends in high-latitude southern hemisphere surface climate. Nat. Clim. Change 6, 917–926. doi: 10.1038/nclimate3103
Kramer-Schadt, S., Revilla, E., Wiegand, T., and Grimm, V. (2007). Patterns for parameters in simulation models. Ecol. Modell. 204, 553–556. doi: 10.1016/j.ecolmodel.2007.01.018
Laptikhovsky, V., and Brickle, P. (2005). The Patagonian toothfish fishery in Falkland islands' waters. Fish. Res. 74, 11–23. doi: 10.1016/j.fishres.2005.04.006
Lescroël, A., and Bost, C.-A. (2006). Recent decrease in gentoo penguin populations at Iles Kerguelen. Antarct. Sci. 18, 171–174. doi: 10.1017/S0954102006000198
Lovenduski, N. S., and Gruber, N. (2005). Impact of the southern annular mode on southern ocean circulation and biology. Geophys. Res. Lett. 32:L11603. doi: 10.1029/2005GL022727
Marsh, H., and Sinclair, D. F. (1989). Correcting for visibility bias in strip transect aerial surveys of aquatic fauna. J. Wildlife Manage. 53, 1017–1024. doi: 10.2307/3809604
Meredith, M., Sommerkorn, M., Cassotta, S., Derksen, C., Ekaykin, A., Hollowed, A., et al. (2019). “Polar Regions,” in IPCC Special Report on the Ocean and Cryosphere in a Changing Climate, eds H.-O. Pörtner, D.C. Roberts, V. Masson-Delmotte, P. Zhai, M. Tignor, E. Poloczanska, K. Mintenbeck, A. Alegría, M. Nicolai, A. Okem, J. Petzold, B. Rama, and N. M. Weyer (The Intergovernmental Panel on Climate Change), 203–320.
Michael, P., Wilcox, C., Barbraud, C., Delord, K., de Grissac, S., and Weimerskirch, H. (2019). “Future resilience scaled by surveillance: high sensitivity of albatross to regional illegal, unreported and unregulated fishing effort,” in The Kerguelen Plateau: Marine Ecosystem and Fisheries. Proceedings of the Second Symposium, Proceedings of the Second Symposium, eds D. Welsford, J. Dell, and G. Duhamel (Hobart, TAS), 341–353.
Mintenbeck, K. (2017). Impacts of Climate Change on the Southern Ocean. John Wiley & Sons Ltd. doi: 10.1002/9781119154051.ch20
Neuwirth, E. (2014). RColorBrewer: ColorBrewer Palettes. R package version 1.1-2. Available online at: https://CRAN.R-project.org/package=RColorBrewer
Oliver, E. C. J., Donat, M. G., Burrows, M. T., Moore, P. J., Smale, D. A., Alexander, L. V., et al. (2018). Longer and more frequent marine heatwaves over the past century. Nat. Commun. 9:1324. doi: 10.1038/s41467-018-03732-9
Panassa, E., Völker, C., Wolf-Gladrow, D., and Hauck, J. (2018). Drivers of interannual variability of summer mixed layer depth in the southern ocean between 2002 and 2011. J. Geophys. Res. Oceans 123, 5077–5090. doi: 10.1029/2018JC013901
Pardo, D., Jenouvrier, S., Weimerskirch, H., and Barbraud, C. (2017). Effect of extreme sea surface temperature events on the demography of an age-structured albatross population. Philos. Trans. R. Soc. B Biol. Sci. 372:1723. doi: 10.1098/rstb.2016.0143
Park, Y.-H., Durand, I., Kestenare, E., Rougier, G., Zhou, M., d'Ovidio, F., et al. (2014). Polar front around the Kerguelen islands: an up-to-date determination and associated circulation of surface/subsurface waters. J. Geophys. Res. Oceans 119, 6575–6592. doi: 10.1002/2014JC010061
Parkes, G., Moreno, C., Pilling, G., and Young, Z. (1996). Use of the Leslie stock depletion model for the assessment of local abundance of Patagonian toothfish (Dissostichus eleginoides). CCAMLR Sci. 3, 55–77.
Péron, C., Welsford, D. C., Ziegler, P., Lamb, T. D., Gasco, N., Chazeau, C., et al. (2016). Modelling spatial distribution of Patagonian toothfish through life-stages and sex and its implications for the fishery on the Kerguelen plateau. Prog. Oceanogr. 141, 81–95. doi: 10.1016/j.pocean.2015.12.003
Pruvost, P., Duhamel, G., and Palomares, M. L. D. (2005). “An ecosystem model of the Kerguelen islands' EEZ,” in Modeling Antarctic Marine Ecosystems. Fisheries Centre Research Reports, eds M. L. D. Palomares, P. Pruvost, T. J. Pitcher, and D. Pauly (Vancouver, BC: The Fisheries Centre, University of British Columbia), 40–64.
Quéguiner, B. (2013). Iron fertilization and the structure of planktonic communities in high nutrient regions of the southern ocean. Deep Sea Res. II Top. Stud. Oceanogr. 90, 43–54. doi: 10.1016/j.dsr2.2012.07.024
Rayfuse, R. (2018). Climate change and Antarctic fisheries: ecosystem management in CCAMLR. Ecol. Law Q. 45:53. doi: 10.15779/Z381834271
Rogers, A., Frinault, B., Barnes, D., Bindoff, N., Downie, R., Ducklow, H., et al. (2020). Antarctic futures: An assessment of climate-driven changes in ecosystem structure, function, and service provisioning in the southern ocean. Annu. Rev. Mar. Sci. 12, 87–120. doi: 10.1146/annurev-marine-010419-011028
Scott, E., Serpetti, N., Steenbeek, J., and Heymans, J. J. (2016). A stepwise fitting procedure for automated fitting of ecopath with ecosim models. SoftwareX 5, 25–30. doi: 10.1016/j.softx.2016.02.002
Su, Z., Pilo, G. S., Corney, S. P., Holbrook, N. J., Mori, M., and Ziegler, P. (2020). Characterising marine heatwaves in the Kerguelen plateau region. Front. Mar. Sci. [Epub ahead of print].
Subramaniam, R. C., Corney, S. P., Swadling, K. M., and Melbourne-Thomas, J. (2020). Exploring ecosystem structure and function of the northern Kerguelen plateau using a mass-balanced food web model. Deep Sea Res. II Top. Stud. Oceanogr. 174:104787. doi: 10.1016/j.dsr2.2020.104787
Swart, N. C., and Fyfe, J. C. (2012). Observed and simulated changes in the southern hemisphere surface westerly wind-stress. Geophys. Res. Lett. 39:L16711. doi: 10.1029/2012GL052810
Thiers, L., Delord, K., Bost, C.-A., Guinet, C., and Weimerskirch, H. (2017). Important marine sectors for the top predator community around Kerguelen archipelago. Polar Biol. 40, 365–378. doi: 10.1007/s00300-016-1964-4
Venkataramana, V., Anilkumar, N., Naik, R. K., Mishra, R. K., and Sabu, P. (2019). Temperature and phytoplankton size class biomass drives the zooplankton food web dynamics in the Indian ocean sector of the southern ocean. Polar Biol. 42, 823–829. doi: 10.1007/s00300-019-02472-w
Weimerskirch, H., Delord, K., Barbraud, C., Le Bouard, F., Ryan, P. G., Fretwell, P., et al. (2018). Status and trends of albatrosses in the French southern territories, western Indian ocean. Polar Biol. 41, 1963–1972. doi: 10.1007/s00300-018-2335-0
Weimerskirch, H., Louzao, M., de Grissac, S., and Delord, K. (2012). Changes in wind pattern alter albatross distribution and life-history traits. Science 335:211. doi: 10.1126/science.1210270
Wickham, H. (2016). ggplot2: Elegant Graphics for Data Analysis. New York, NY: Springer-Verlag. doi: 10.1007/978-3-319-24277-4_9
Keywords: Ecosim, environmental drivers, ecosystem change, Kerguelen Plateau, Patagonian toothfish fishery
Citation: Subramaniam RC, Melbourne-Thomas J, Corney SP, Alexander K, Péron C, Ziegler P and Swadling KM (2020) Time-Dynamic Food Web Modeling to Explore Environmental Drivers of Ecosystem Change on the Kerguelen Plateau. Front. Mar. Sci. 7:641. doi: 10.3389/fmars.2020.00641
Received: 07 May 2020; Accepted: 13 July 2020;
Published: 11 September 2020.
Edited by:
Marta Coll, Institute of Marine Sciences (CSIC), SpainReviewed by:
Konstantinos Tsagarakis, Hellenic Centre for Marine Research (HCMR), GreeceBrett W. Molony, CSIRO Oceans and Atmosphere (O&A), Perth, Australia
Copyright © 2020 Subramaniam, Melbourne-Thomas, Corney, Alexander, Péron, Ziegler and Swadling. This is an open-access article distributed under the terms of the Creative Commons Attribution License (CC BY). The use, distribution or reproduction in other forums is permitted, provided the original author(s) and the copyright owner(s) are credited and that the original publication in this journal is cited, in accordance with accepted academic practice. No use, distribution or reproduction is permitted which does not comply with these terms.
*Correspondence: Roshni C. Subramaniam, roshni.subramaniam@utas.edu.au