- 1Laboratory of Marine Biodiversity and Ecosystems Management, Department of Marine Sciences, University of the Aegean, Mytilene, Greece
- 2Institute of Oceanography, Hellenic Centre for Marine Research, Anavyssos, Greece
The study of marine coastal food webs has a central role in the application of the integrated ecosystem approach for marine management. Changes in food webs caused by natural or anthropic drivers can lead to dramatic shifts in the overall structure and function of coastal marine ecosystems and deterioration of their services. The present review investigates the methodological approaches employed for the assessment of coastal shelf food webs at a global scale and highlights existing gaps and limitations. Out of 1652 published articles that initially met our search criteria, 880 passed the initial screening and 493 were found relevant and were fully analyzed. The information extracted included the spatiotemporal coverage of the studies; the main methodological approaches utilized for the assessment of population state variables (i.e., biomass, size, abundance) and trophic levels; the biotic components and driving factors considered; indices used to describe the structure and functioning of coastal food webs; and main knowledge gaps. Results showed that most studies have been conducted at a subnational level, mostly in the Temperate Northern Atlantic marine realm. Overall, 54% of the studies provided quantitative information on food web structure. The most common methodological approach utilized was modeling (40%), followed by non-experimental-based correlations (30%), and natural or manipulative experiments (14%). Information on population state variables was provided by 69% of the studies, while 42% employed some of the following trophic level determination techniques: stable isotopes, gut contents, fatty acids, and molecular analysis, which were either combined or used in isolation. Specific natural or human drivers were incorporated in 76% of the studies, with fishing being the most common driver. Modeling approaches included multiple indices to describe food web attributes and/or the structure and functioning of coastal shelf ecosystems. Despite the great progress achieved through the development of new tools and techniques, food web analysis still suffers from important knowledge gaps and limitations of the methodological approaches, which are extensively discussed. The present review establishes a useful knowledge base to provide guidance for future research and assessments on coastal shelf food webs, and to support ecosystem-based management.
Introduction
In the emerging era of the Anthropocene (Steffen et al., 2015), growing human pressures on marine ecosystems and their cumulative effects have caused widespread degradation of marine habitats (Hughes et al., 2017), substantial biomass declines of marine communities (Myers and Worm, 2003), local extinctions (Thibaut et al., 2014), and regime shifts (Rocha et al., 2015; Wernberg et al., 2016). Compared to the oceanic zone, coastal shelf environments are subjected to disproportionally higher cumulative pressures as they concentrate many human activities and are affected by both sea-based and land-based drivers (Hall, 2002; Micheli et al., 2013). Overfishing (Jackson et al., 2001; Sala et al., 2012), pollution (Tornero and Hanke, 2016), coastal development (Meinesz et al., 1991; Bulleri and Chapman, 2010), invasive species (Katsanevakis et al., 2014), destructive fishing practices (Saila et al., 1993; Guidetti, 2011), and climate change (Rilov, 2016) are responsible for high rates of biodiversity loss in coastal shelf ecosystems (Lotze et al., 2006; Waycott et al., 2009). Such severe impacts on marine ecosystems have raised worldwide concerns and prompted a fundamental shift in the management of marine living resources, toward a holistic and integrated approach, recognizing the full area of interactions within ecosystems (Katsanevakis et al., 2011).
The so-called ecosystem approach is globally gaining ground over conventional approaches that have traditionally focused on a single species, or ecosystem service. Aiming for sustainable, resilient populations, habitats and ecosystem functions and services, it includes a strategy for the integrated management of coastal and marine ecosystems, in order to ensure the conservation of marine resources and their sustainable use in an equitable way (SCBD, 2004; Apitz et al., 2006). To achieve this, the ecosystem approach is based on the application of appropriate scientific methodologies focused on all levels of biological organization, which encompass the essential structure, processes, functions and interactions among organisms and their environment (SCBD, 2004). Nevertheless, the complex and dynamic nature of coastal ecosystems and the absence of complete knowledge or understanding of their functioning, poses a major challenge in the successful application of this approach.
The evolved concept of ecosystem–based management (EBM) has incorporated the human component in the ecosystem, as well as the vital processes and functions that are needed to sustain the ecosystems at a healthy state. The EBM approach has thus become the primary tool to achieve the objectives of several regional and international agreements regarding the conservation of the marine environment and its sustainable use. For example, the United Nations Strategic Plan for Biodiversity (2011-2020) proposes an overarching framework based on 20 biodiversity targets (the Aichi Targets) that include both ecological and social aspects in order to reduce biodiversity loss at a global scale (Marques et al., 2014), while the European Marine Strategy Framework Directive (MSFD; Directive 2008/56/EC) focuses on 11 descriptors related to ecosystem features and human pressures in order to achieve a good environmental status in all the European Seas (EU, 2008).
For an integrated view of ecosystems, food webs are established to have a central ecological role (Odum et al., 1971). Complex interactions take place in marine ecosystems, among different food web components (Pomeroy, 1974) and these interactions can be comprehended with a holistic view of the food web, rather than separate species assessments (Cohen et al., 1993). Trophic structure of the ecosystem's components and the energy flow among them was highlighted early by ecologists (Lindeman, 1942; Odum and Odum, 1955), and various population control mechanisms were considered. Precisely, top-down control from predation and bottom up control from resource limitation were considered to affect the structure and function of coastal rocky ecosystems (Paine, 1966, 1974; Estes, 1996). Later on, it was proposed that ecosystem structure could be determined from the synergistic effect of top-down and bottom-up controls (Menge, 1992, 2000). In the meantime, another “wasp-waist” control mechanism has been described at the intermediate level, where the minority of species can transfer most of the energy between primary producers and predators, specifically in up-welling ecosystems (Rice, 1995; Bakun, 1996, 2004, 2006; Cury et al., 2000, 2004). Under certain conditions, a switch between bottom-up and top-down control can occur (Hunt and McKinnell, 2006).
Series of interactions among a trophic level that can indirectly control another trophic level have been described as trophic cascades (Hairston et al., 1960; Menge, 1995). A dynamic equilibrium takes place among trophic levels, severely affected by environmental drivers (Menge and Sutherland, 1987; Hunt and McKinnell, 2006). This is why holistic assessments of food webs are needed (Lindeman, 1942; Odum et al., 1971; Libralato et al., 2014) to investigate their dynamics and the potential for human-induced modifications, or even regime shifts through trophic cascades (Pace et al., 1999; Polis et al., 2000), due to e.g., fisheries (Pauly et al., 1998; Shannon et al., 2014), eutrophication (Armitage and Fourqurean, 2009), climate change (Ainsworth et al., 2011), pollution (Young et al., 1982), habitat alteration (Tallis, 2009; Bishop et al., 2010; Hemraj et al., 2017), and alien species (Corrales et al., 2017a).
Despite its value, a holistic assessment of the food web structure and function is not easy (Libralato et al., 2014). Often data of all trophic levels are not available (Corrales et al., 2017b; Eddy et al., 2017), cost a lot (Robinson et al., 2010) and/or are difficult to collect. Food web assessments are often restricted by knowledge gaps on species biomasses, diets, mortalities and unreported catches (Barausse et al., 2009). In cases when commercial fisheries landing data are used for the ecosystem's assessment, doubts on the reliability of fisheries statistics and available data have been expressed (Zeller and Pauly, 2007; Shannon et al., 2014). Trophic level estimations are notably difficult for omnivorous species, which are quite common in the marine environment (Pimm et al., 1991). Overall, food web studies still suffer from controversies, uncertainties, and information gaps (Bǎnaru et al., 2013; Libralato et al., 2014).
The principal aim of this study was to assess methodological approaches to analyze coastal shelf food webs, by reviewing the global literature. We review and describe the main methods applied for data collection, analysis of food webs and relevant indices. Based on the findings of this review we highlight gaps and limitations on existing methodological approaches.
Methods
A systematic, global literature review was conducted, applying the PRISMA (Preferred Reporting Items for Systematic Reviews and Meta-Analyses) methodology (Moher et al., 2010). Elsevier's Scopus database (www.scopus.com) was employed for the bibliographic search on peer-reviewed literature published between 1960 and 2018. Search criteria included at least one term between “marine or sea,” and among “benthic or coast/s or shelf or demersal,” “food web/s or trophic web/s,” and “function/s or structure/es or relation/s or interaction/s” in the title, abstract or keywords. Only published articles were considered. The search was limited to relevant subject areas (Agricultural and Biological Sciences, Environmental Science, Earth and Planetary Sciences, Biochemistry, Genetics, and Molecular Biology) and to English-language publications. The search resulted in 1639 publications. Additionally, 16 relevant publications that met the above criteria but did not show up in the search were also included in the review process. In total, 1652 publications were further analyzed, after the removal of duplicates (Figure 1). The full publication list was managed with the open-source reference management software Zotero (www.zotero.org).
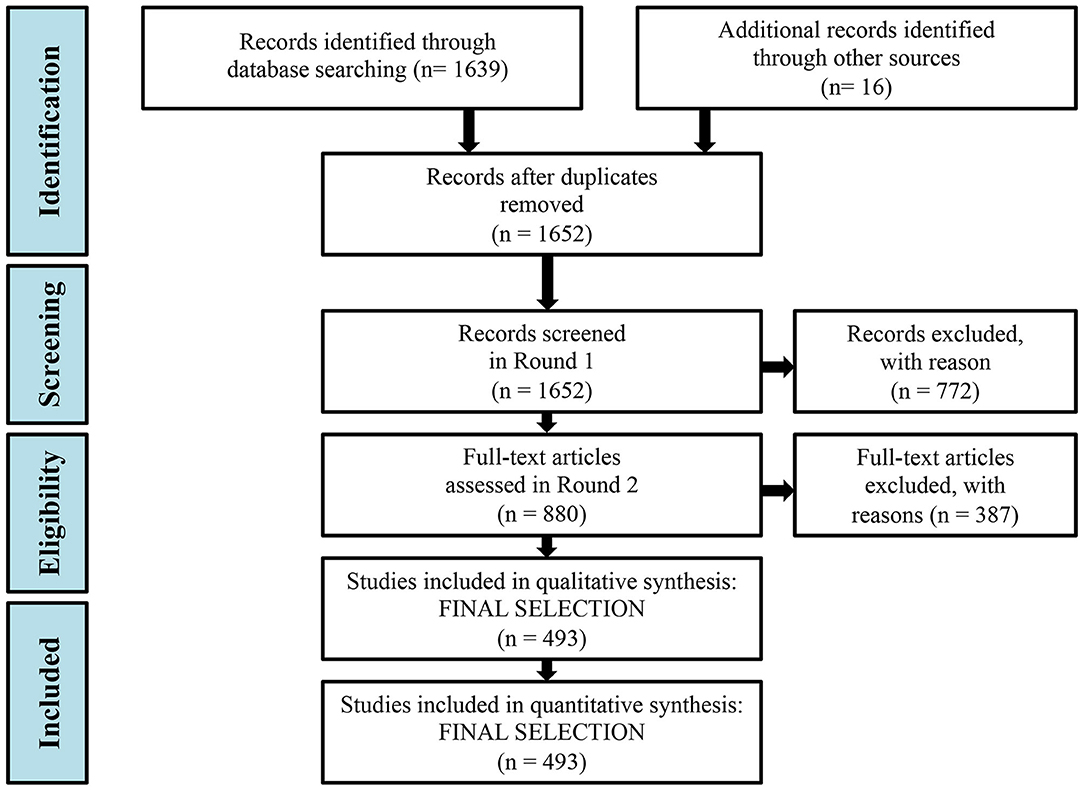
Figure 1. Flow diagram of the methodology and selection processes used in this systematic review by applying the PRISMA (Preferred Reporting Items for Systematic Reviews and MetaAnalyses) methodology (Moher et al., 2010).
Three reviewers performed two rounds of review. During the first round, papers were screened based on their titles, keywords and abstract. Papers that (1) were unrelated to food webs/trophic webs, (2) assessed only one trophic level, and thus did not properly account for trophic relations, (3) didn't study the coastal shelf, but were restricted in pelagic or bathyal ecosystems, or (4) were not marine studies, were discarded. In total, 880 articles out of 1652 remained after the initial screening. During the second round, the full text of the 880 papers was examined and 387 articles were excluded, based on the same criteria, while 493 articles remained for full analysis (Figure 1). Intercalibration among the three reviewers was achieved by means of analysis of ten common papers.
Data collected from the 493 fully reviewed papers (see Table S1 for the list of full references) included information on the spatiotemporal and geomorphological characteristics of the studies, various methodological aspects (e.g., the methodological approach followed, the type of data collected, and the analytical methods applied), the type of biotic information used (e.g., number of species, functional groups and trophic levels), the potential driving factors considered, indices used to describe the structure and functioning of coastal shelf food webs, and the highlighted gaps of knowledge mentioned. Specifically, the following information was retrieved from each paper, with each number of the following list representing one data sheet column: (1) Year of publication; (2) Name of reviewer; (3) Food web focused study: yes, no; (4) Spatial scale: subnational (covering part of a country's territorial waters or exclusive economic zone—EEZ), national (covering all territorial waters or EEZ of a country), supranational (covering marine areas of more than one country), global; (5) Continent: Europe, Asia, Africa, North America, South America, Oceania/Australia, Antarctica, more than one continents, undefined, global; (6) Country; (7) Marine biogeographic realm according to Spalding et al. (2007): Arctic, Temperate Northern Atlantic, Temperate Northern Pacific, Tropical Atlantic, Western Indo- Pacific, Central Indo-Pacific, Eastern Indo-Pacific, Tropical Eastern Pacific, Temperate South America, Temperate Southern Africa, Temperate Australasia, Southern Ocean, more than one realms, undefined; (8) Specific location: free text; (9) Bathymetric zone: only coastal shelf: 0–200 m, both coastal shelf (0–200 m) and offshore (>200 m), not specified (200-m depth was considered as the most appropriate and widely used coastal shelf boundary; Spalding et al., 2007); (10) Habitat type: sand/mud, rocky reefs, coral reefs, seagrasses, mix, undefined; (11) Years of study duration; (12) Number of seasons considered: no season, one season, two seasons, three seasons, four seasons, not clear; (13) Fieldwork included: yes, no; (14) Non-destructive methodologies used, when fieldwork included: yes (exclusively), no, both (destructive and non-destructive); (15) Main methodological approach applied to assess coastal food webs: manipulative experiment, natural experiment, non-experimental-based correlation, modeling, qualitative/conceptual, other. Manipulative experiments refer to field or laboratory experiments that include treatments/control and random selection of experimental units. In natural experiments, one of the elements of manipulative experiments is missing and the experimental units are selected by nature (i.e., not randomly). Non-experimental-based correlation refers to inference based on an observed correlation between ecosystem state variables and a specific driver, but not based on an experimental design for data collection. Modeling refers to estimates of state variables/indices derived from ecosystem models. Qualitative/conceptual studies are based on expert judgment and/or a qualitative analysis; Other studies, didn't match any of the above approaches and consisted mainly of observational studies that explored food web variables without any correlation with drivers or further modeling analysis; (16) Type of model or statistics (Ecopath, Ecopath with Ecosim, Atlantis, descriptive statistics, other); (17) If other in field 16: free text; (18) Acquisition methods for population state variables (biomass/size/abundance): literature-based sources, destructive sampling, non-destructive sampling; (19) Trophic level and/or trophic relationships determination techniques applied alone or in combination: stable isotope analysis, fatty acid analysis, gut content analysis, molecular analysis; (20) Food web reconstruction (i.e., quantitative information of the energy or carbon transfer through the food web): yes, no; (21) Scientific or common names of species studied: free text; (22) Minimum number of species studied: actual number, not mentioned; (23) Alien species studied: yes, no; (24) Scientific or common name of alien species studied: free text; (25) Quantitative food web assessment (e.g., providing biomass per trophic level): yes, no; (26) Aggregation of species into biotic functional groups (i.e., referring to groups of organisms of similar trophic level (e.g., primary producers, omnivores, piscivores): yes, no; (27) Number of biotic functional groups used; (28) Names of the different biotic functional groups used: free text; (29) Trophic level/s considered: Number, not mentioned, all trophic levels equally studied; (30) lowest trophic level studied; (31) highest trophic level studied; (32) Taxa mostly targeted: phytoplankton, algae, zooplankton, benthic invertebrates, fish, reptiles, birds, mammals, not applicable (when the study didn't focus in a single taxonomic group); (33) Driving factors considered: yes, no; (34) Categories of driving factors considered: fishing, temporal variability (mainly seasonal, inter-annual, monthly), climate change, eutrophication, pollution, habitat complexity, alien species, grazing, organic matter content/input (e.g., pelagic/coastal/terrestrial/riverine), other, more than one drivers; (35) More than one drivers and/or other drivers: free text; (36) Indices used to describe food webs: yes, no. As an “index” we considered any mathematical expression, ratio or correlation used by the authors in order to quantify key features and properties of food webs and marine ecosystems, describing characteristics of energy flow, resilience, structure, and functioning; (37) Number of indices used; (38) Names of indices used to describe food webs: free text; (39) Economic analysis performed: yes, no; (40) Knowledge gaps and future work highlighted: free text.
Descriptive statistics were used to analyze the review results using one, or combining more than one, of the aforementioned data sheet columns, and utilizing the filter option accordingly, in order to provide respective estimates. The number of studies regarding different biotic groups, taxonomic groups, and drivers was estimated separately for the different methodological approaches considered (i.e., manipulative experiment, natural experiment, non-experimental-based correlation, modeling, qualitative/conceptual, and other). Drivers were further aggregated into two distinct time periods, before and after 2004, corresponding approximately to the periods before and after the onset of the wider use of modeling approaches. QGIS was used to map the reviewed food web studies per marine realm (sensu Spalding et al., 2007). SankeyMATIC was used to create the Sankey diagram.
Results
Spatiotemporal Coverage of the Food Web Studies
The selection criteria were not matched for any study published before 1973, while only 29 studies matched the search terms before 2000. The publication rate of food web studies has increased substantially since 2000, reaching a maximum of 44 studies annually during 2014 and above 40 during 2017 and 2018 (Figure 2A). Relevant studies were conducted in all marine realms (sensu Spalding et al., 2007, Figure 3). However, the bulk of studies referred to the Temperate Northern Atlantic (49%), followed by the Temperate Northern Pacific (12%), and the Arctic (9%), while 5% of the studies cover more than one marine realms (Figure 3). Spatially, most studies referred to a subnational (81%) or a supranational level (16%; Figure 2B). Among all continents, 40% of the studies were conducted in Europe, 25% in North America, 12% in Asia, 7% in South America, 5% in Africa, 4% in Oceania/Australia, 2% in Antarctica, 4% of the studies were conducted in more than one continent, and 1% were global. Most studies (71%) regard ecosystems exclusively on or above the coastal shelf, 20% integrated both coastal shelf and offshore waters, while 9% referred to coastal marine ecosystems but without clarifying the studied depth zone. Only 49% of the studies defined the type of habitat investigated (Figure 2C); many of these investigate a mixture of habitat types (20%), 16% focused on sandy/muddy substrates, 9% on rocky reefs, 3% on seagrasses, and 1% on coral reefs.
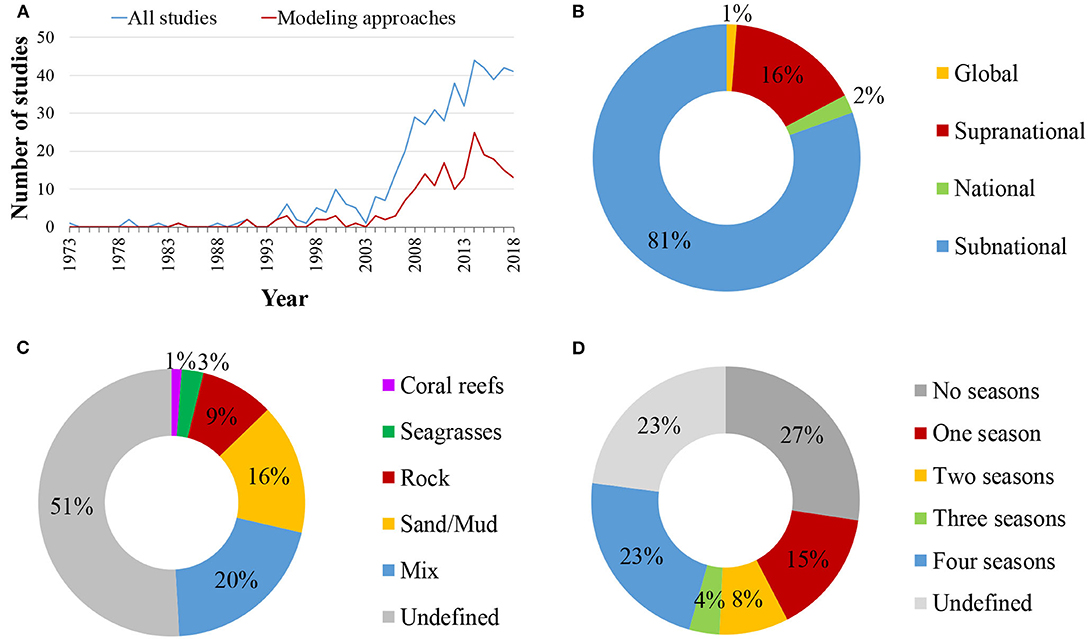
Figure 2. Spatio-temporal identity of the reviewed studies: (A) annual publication rate of coastal shelf food web studies between 1973 and 2018. Blue line: all studies, red line: studies utilizing a modeling approach; (B) spatial scale of the studies; (C) habitat types studied; (D) number of seasons accounted for.
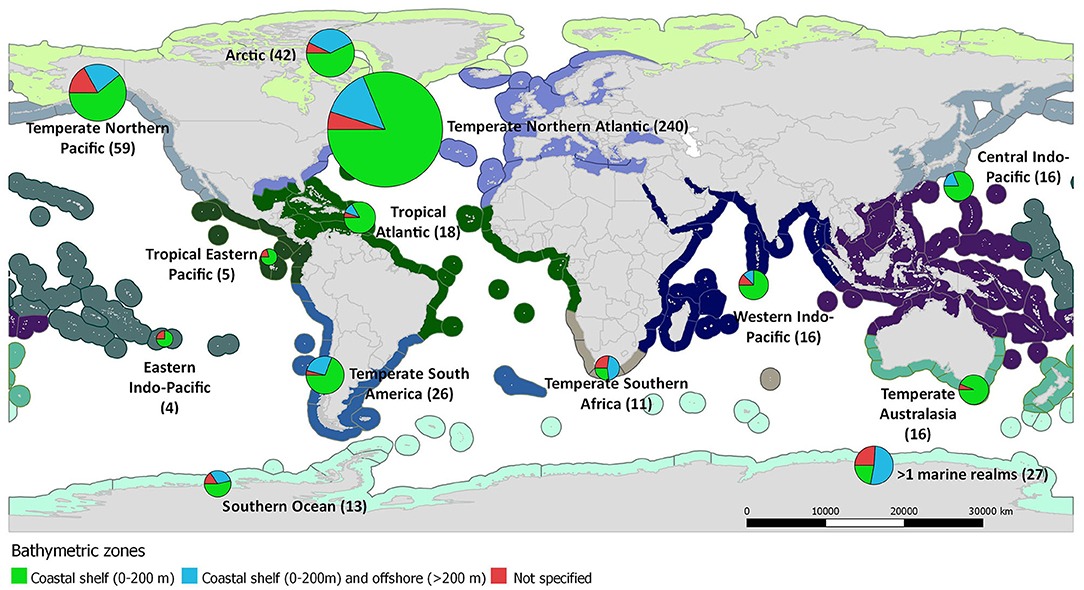
Figure 3. World map depicting the number of coastal shelf food web studies (N = 493) that have been carried out per marine realm (depicted with different color in the map; sensu Spalding et al., 2007). Pie charts represent the proportion of studies conducted per bathymetric zone.
With regards to the temporal extent, studies analyzed, simulated, modeled or predicted the food web structure over time frames, ranging from a few days to more than 400 years. Many papers referred to a time frame of <1 year (25%), 41% considered food webs over 1–10 years, 18% spanned over more than 10 years, while 17% made no mention of temporal scale. Information on the number of seasons sampled or considered in subsequent analyses can be found in half of the studies (50%; Figure 2D); of these, the majority took into account either four seasons (23%), or one season (15%). The remaining 50% either provided no information regarding seasonality (“undefined” in Figure 2D) or did not incorporate seasonality into relevant analyses (“no seasons”).
Biotic Components of the Food Web Studies
With regards to the biotic information provided in the reviewed 493 studies, 75% classified food web components into distinct biotic groups (e.g., carnivorous fish), while 25% presented information on selected species only. The number of biotic groups ranged between 1 and 135 (mean = 14.7, standard deviation = 16.9). A high number of distinct biotic groups (ranging from 11 to 135) were mostly used for the description of coastal shelf food webs in the modeling studies, while the rest food web study approaches used predominantly fewer biotic groups (Figure 4A). Specifically, many studies with experimental approaches (55% of them) and non-experimental-based correlations (26% of them) used 1–5 biotic groups. Among all the reviewed studies, 22% focused mostly on fish, 21% on benthic invertebrates and 6% on zooplankton, while a high percentage of the studies (45%) focused in more than one taxonomic group (NA in Figure 4B). Many studies with experimental approaches (45% of all of them) focused mostly on benthic invertebrates and –to a much lesser extent– on fish (Figure 4B). Of all the reviewed studies, 263 (53%) used trophic levels for the description of trophic relationships. The trophic level ranged between 1 and 5.64 (mean = 2.9, standard deviation = 0.8), and the range most commonly taken into account was 4.5 (Figure 5). Six studies included trophic levels lower than one. These are all studies using stable isotope analysis, where trophic level is calculated based on the isotopic distance of a particular dietary cluster to a chosen base reference. In these cases, values below one refer to certain primary producers and suspended particulate organic matter (Nilsen et al., 2008; Roy et al., 2015; Wing et al., 2017, 2018; Xue et al., 2017), or even some species of pelagic filter feeders or benthic surface and sub-surface deposit feeders (Bell et al., 2016).
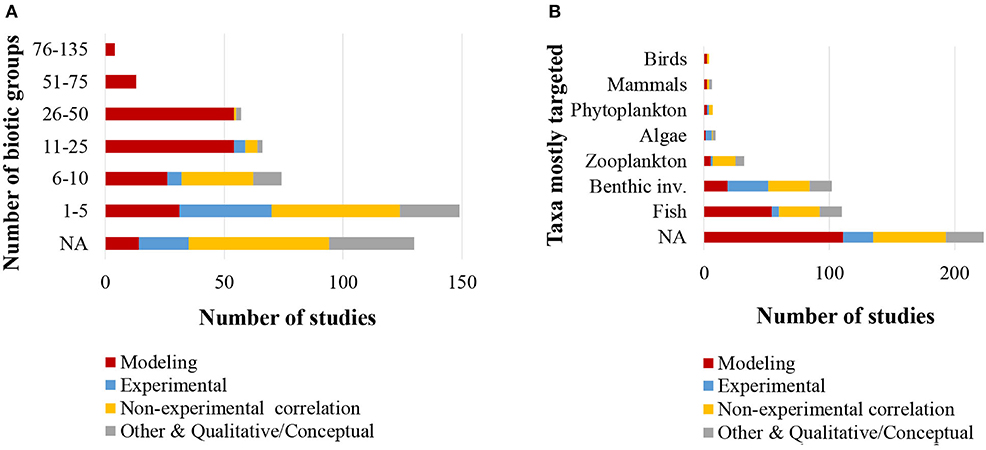
Figure 4. (A) Number of biotic groups considered (N = 493); (B) Taxonomic groups that studies focused in (N = 493). NA, Not applicable.
Methodological Aspects
Only 63% of the studies included fieldwork, while the rest 37% exclusively used literature data. The vast majority of studies that conducted fieldwork obtained data through the use of destructive data acquisition methods (81%; Figure 6A), requiring the removal of biological samples from their natural environment and the killing of marine organisms. Only 5%, employed exclusively non-destructive data acquisition methods (i.e., methods that have a minimal impact on the studied environment and the associated organisms), while only 14% used a combination of both (Figure 6A). The most common type of methodological approach employed for the assessment of coastal shelf food webs was modeling (40%; Figure 6B), followed by non-experimental-based correlation (30%), manipulative experiments (8%), natural experiments (6%), or a qualitative/conceptual type of approach (3%). Overall, 54% of the studies provided quantitative information on food web structure (Figure 6C) (e.g., biomass per trophic level), while 46% offered a qualitative description. Modeling, natural and manipulative experiments predominantly led to quantitative descriptions of food webs, non-experimental-based correlation almost equally led to both quantitative and qualitative results, while qualitative/conceptual and other types of approaches predominantly led to qualitative descriptions of food webs (Figures 6B,C).
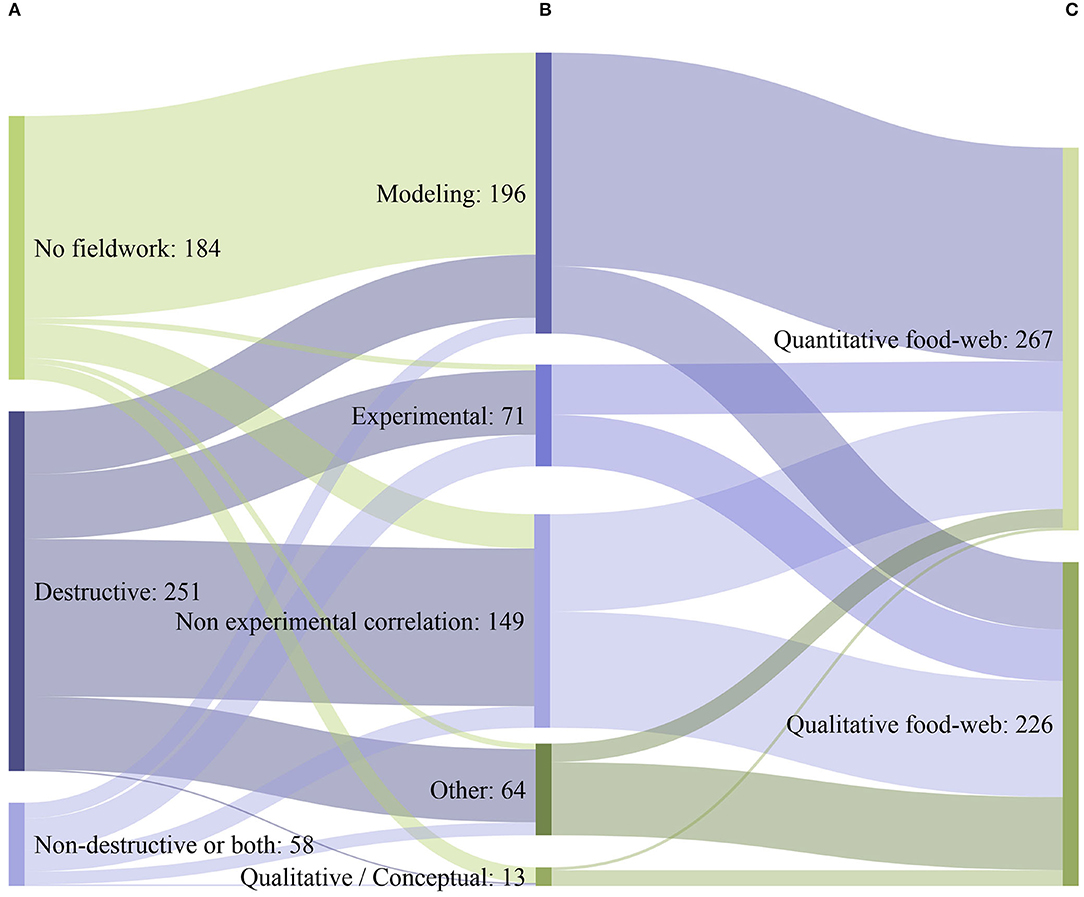
Figure 6. Sankey diagram depicting the flow between (A) the different data acquisition methods (B) the main methodological approaches used to assess marine coastal shelf food webs, (C) the type of information (Quantitative/ Qualitative) provided by the studies (N = 493). Lines and ribbons represent flows between two consecutive grouping bars only (A–B, B–C). Non-destructive or both: exclusive use of non-destructive methods alone and combined use of both destructive and non-destructive methods.
Information on population state variables (i.e., biomass, size, abundance) was provided by 340 studies (69%), of which 58% acquired data through the literature, 49% using destructive sampling techniques, and 14% through non-destructive surveys. Specifically, literature-based data included information extracted from the published scientific literature, project reports, fisheries stock assessments, national or regional statistics, fisheries landings services, open access databases (e.g., FAO-GFCM statistics; Fishbase—Froese and Pauly, 2019). Destructive sampling techniques were mostly fisheries-based, using various types of benthic and pelagic fisheries tools, such as beam trawls (Jennings et al., 2002; Brind'Amour et al., 2009; Bell et al., 2016), otter trawls (Koulouri et al., 2015; Bell et al., 2016), plankton nets (Blachowiak-Samolyk et al., 2007; Anjusha et al., 2013; Caron et al., 2017), fyke nets (Thollot et al., 1999; Bergström et al., 2016), gillnets (Di Beneditto et al., 2012; Prado et al., 2014; Donadi et al., 2017), seine nets (Faye et al., 2011), bottom dredges (Jennings et al., 2001), crab traps (Mancinelli et al., 2013), and eel traps (Heldal et al., 2018). Other particular sampling techniques included infauna sampling with VanVeen and Smith-McIntyre grabs (Soto and Escobar-Briones, 1995; Dunton et al., 2012; Whalen et al., 2013; Lovvorn et al., 2015; Tu et al., 2015; Misic et al., 2016) and USNEL box corer (Tselepides et al., 2000), scraping of rocky surfaces with chisel for the collection of algae and benthic invertebrates (Lin et al., 1999; Kroeker et al., 2011), manual hand collection of macroinvertebrates by means of SCUBA diving (Tewfik et al., 2005; O'Gorman et al., 2008; Wing et al., 2018), as well as experimental dynamite fishing (Hansen et al., 2012). On the other hand, non-destructive survey methods were much less commonly used. These methods mostly included underwater visual counts of fish and benthic invertebrates within strip transects and quadrats (plot sampling) by means of direct observation while SCUBA diving and snorkeling (e.g., Leonard et al., 1998; Albouy et al., 2010; Coll et al., 2011; Mumby et al., 2012; Sala et al., 2012) or underwater video recording (Schoenrock et al., 2018), photoquadrat sampling for the estimation of benthic cover (Edgar et al., 2011, 2017; Salomidi et al., 2016), as well as visual counts of birds from land or via aerial surveys (Kvitek and Bretz, 2005; Lassalle et al., 2011; Luczak et al., 2012) or mark-recapture bird surveys (Paiva et al., 2013). Deviating from the apparent norm of visual counts being the most commonly used set of non-destructive methods, hydroacoustics were also used by seven studies (Ainley et al., 2009; Chen et al., 2011, 2015; Pálsson et al., 2012; Paiva et al., 2013; Stauffer et al., 2015; Marsh et al., 2017) for the estimation of pelagic fish abundance. Biomass and/or abundance data were often used as basic input for ecological models reconstructing the food web under study, whereas the organisms collected via destructive methods were usually further analyzed using various techniques for the determination of trophic levels and/or trophic relationships.
Moreover, four types of trophic level and/or trophic relationship determination techniques (stable isotopes, fatty acids, gut contents, and molecular analysis) were identified in the reviewed papers (Figure 7). These techniques were deployed to define the trophic level of species and biotic groups, the trophic relationships between different food web compartments, or both. The different techniques were used either in isolation, or combined, in order to compare and/or improve the resolution of trophic relations. Out of the 206 studies (42% of all the 493 studies) that applied one of the aforementioned determination techniques, 171 (83%) used stable isotopes to trace some key element (mainly carbon—C and nitrogen—N), 19 studies applied stable isotopes in combination with lipid or fatty acid analyses, 14 used stable isotopes to validate gut contents, while one study combined stable isotopes, gut contents and fatty acid analyses. Gut contents analyses were applied in isolation in 31 studies and were combined with molecular analysis in a single study. Molecular techniques were used in isolation in two studies, while analysis of fatty acids alone was used in just one study.
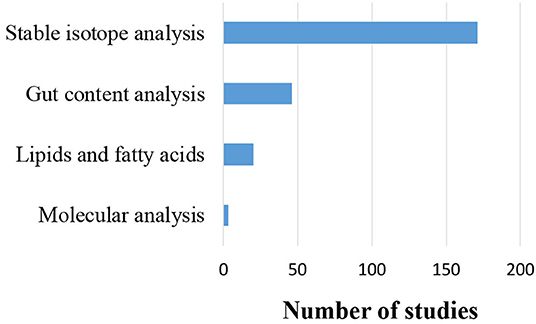
Figure 7. Number of studies that used each trophic relationship determination technique, alone or in combination with others (N = 206).
Stable isotope analysis is a method commonly used in the study of trophic relations and energy flow (see Michener and Lajtha, 2007 for a review). The method is mainly based on the use of the stable isotopes of C and N, in order to record both source of nutritional content and trophic level information. In the reviewed studies, the contribution of different sources of δ13C and δ15N to the diets of representative species or trophic groups were determined through linear mixing models based on mass balance equations (e.g., using the Isosource software; Phillips and Gregg, 2003), stable isotope mixing models (e.g., SIAR—stable isotope analysis in R, Parnell et al., 2008, 2010; IsoWeb, Kadoya et al., 2012; MixSIAR, Stock and Semmens, 2013) or other types of modeling approaches (Boecklen et al., 2011). Next to determining the number and degree of association of the different trophic levels, stable isotope analysis of stomach contents was also used to define habitat preferences, site fidelity, trophic breadth or overlap of different species (Aurioles-Gamboa et al., 2013), importance of different food sources in supporting specific trophic levels (Behringer and Butler, 2006), and describe and compare the food webs of different marine ecosystems (e.g., Cai et al., 2005). In one case, the stable isotope of δ18O was used to track freshwater contribution to the marine food webs (Bell et al., 2016), while the analysis of compound specific stable isotopic composition of specific amino acids (e.g., glutamic acid and phenylalanine) was used to define trophic position of different organisms (Chikaraishi et al., 2014).
Lipids and fatty acids are some of the most commonly used trophic markers for the study of food web dynamics. Because of their characteristics and their storage patterns in the tissues of the organisms, they are very useful tracers of trophic relationships and are considered as reliable indicators of long-term diet. Specifically, they do not degrade during digestion like other dietary nutrients, and they are stored in animal bodies in fat reservoirs, accumulating over time and representing the dietary intake over long periods of time (Iverson, 2009). In the studies of this review, fatty acid analysis has been used in two main ways: either looking for differences or changes in fatty acid composition and thus making inferences about differences or changes in diets of consumers (Koussoroplis et al., 2011; McMeans et al., 2015; Jankowska et al., 2018), or using fatty acid biomarkers to identify predator-prey relationships (McLeod and Wing, 2009; Kürten et al., 2013; McMeans et al., 2013).
The classic analysis of gut contents (including stomach, regurgitation or feces contents) is utilized to provide information on the feeding behavior of organisms, their trophic position, and the predator-prey relationships. It has been traditionally used in the study of trophic relations, as the number, size, and quantity of prey items can be assessed through direct visual observations obtained from alive or dead individuals. Direct counts and identification of prey items, provide information on the frequency of occurrence and abundance of different prey species in the gut contents, whereas, volumetric, gravimetric, or direct weighing methods give additional information on the relative contribution of each prey to the diet (Mendonça et al., 2007; Kopp et al., 2013; Oakley et al., 2014). In the reviewed studies, prey items were analyzed either individually or were aggregated into groups according to taxonomic status, functional characteristics, body size (e.g., Oakley et al., 2014), life history stage (e.g., Whitehouse et al., 2017), or association with different habitat types and depth (e.g., Giraldo et al., 2017). Similarly, the biological traits of the sampled predators, such as body or gape size, and ontogenetic shifts in feeding (e.g., Abdurahiman et al., 2010; French et al., 2013; Dunic and Baum, 2017; Hanson, 2018) were taken into account in some studies, to allow a more detailed assessment of the feeding habits of a species. The consideration of such biotic traits were sometimes also used to aggregate species into trophic guilds (non-taxonomic groups of species which exploit the same resources; Abdurahiman et al., 2010; Whitehouse et al., 2017; Hanson, 2018), estimate trophic spectra (e.g., Torruco et al., 2007), and calculate indices which were used as basic inputs in respective food web models (e.g., the consumption per biomass ratio; Ullah et al., 2018). Gut content analysis was also used to verify or complement dietary information obtained through other types of trophic level determination techniques, such as stable isotope or fatty acids analysis (e.g., Rodríguez-Graña et al., 2008; Paiva et al., 2013), to estimate bioaccumulation levels of heavy metals (e.g., McMeans et al., 2015), and to build up complex ecosystem-based models (e.g., Lassalle et al., 2011). In one study, stomach contents were subjected to molecular methods in order to obtain a finer taxonomic resolution of the prey items (Lindeque et al., 2014).
Overall, 149 studies (30% of the reviewed studies) provided a quantitative analysis through food web reconstruction, combining various data sources for the acquisition of population state variables or the determination of trophic levels and relationships. These studies are mainly based on the concept of energy or carbon transfer across the food webs, which essentially refers to the flow of organic matter from one trophic level to the next. Starting from photoautotrophic primary producers that convert solar radiation into carbon compounds through photosynthesis, energy is being transferred as organisms of the higher trophic levels consume those at the lower trophic levels. Trophic linkages are therefore assessed through the study of energy flows (Lindeman, 1942). However, such an approach requires detailed knowledge of the organisms' energy budgets, and/or nutritional composition. In the reviewed studies, this information was provided by some of the aforementioned trophic level determination techniques. For example, essential organic compounds, such as fatty acids, which can only be acquired through the assimilation of dietary components by heterotrophic organisms, were tracked down through the use of fatty acid analysis (Koussoroplis et al., 2011; McMeans et al., 2013), while protein, lipid and carbohydrate contents were estimated through several extraction and determination methods, and were converted into carbon equivalents through the application of respective conversion factors (e.g., Harriague et al., 2006). Thereof, energy budgets of individual organisms or the net energy transfer between sequential compartments within a food web were estimated using complex models which combined data on population state variables, trophic level and relationships, ecological processes (e.g., emigration or immigration), as well as the abiotic or human factors that affect them (e.g., Jennings and Collingridge, 2015). Many of these studies (N = 149) generated food web models using Ecopath with Ecosim (45%), followed by Ecopath (16%), Atlantis (3%), or various other modeling approaches (34%).
Indices and food web attributes that describe the structure and functioning of coastal shelf ecosystems were used in 65 % of the studies (the list of indices descriptions with their references is available in Table S2). These indices and descriptors focused on characteristics of energy flow within the food webs, as well as characteristics of the food web structure and other functional aspects, giving insights into the state of ecosystems, their responses to natural and human impacts and their resilience to current and/or future perturbations. Half of the studies that used indices were modeling studies. In these cases, the values of the indices and food web attributes provided were the direct output of the model used (e.g., Ecopath with Ecosim). Such commonly used indices included the total system throughput (the sum of all trophic flows in the system), which is indicative of its “ecological size” (Ulanowicz, 1980); the total primary production to total biomass ratio, which is related to ecosystem maturity (Christensen and Pauly, 1992); the system omnivory index (a measure of trophic specialization, indicating how feeding interactions are distributed among trophic levels; Christensen and Pauly, 1992); Finn's cycling index (the proportion of total system throughput recycled within the system; Finn, 1976); mean trophic level of the community (Christensen et al., 2008); mean transfer efficiency (efficiency of energy transferred from a trophic level to the next through consumption; Lindeman, 1942); keystoneness index (used to identify possible keystone species; Libralato et al., 2006); ascendency, which is a measure of the size and the organizational status of the network exchanges that occur in an ecosystem (Ulanowicz, 1980); connectance (the number of connections of any possible links between two living compartments of the food web; Gardner and Ashby, 1970), and the number of trophic links between taxa (Cohen and Briand, 1984). Indices typically used in ecological studies, such as common biodiversity indices (e.g., species richness, Shannon-Weaver index H', Simpson diversity index), were also used by various studies as descriptors of food web status. Integrated indices aiming to assess the ecological status or health of marine ecosystems were also applied in several cases, as e.g., the “ocean health index” (Halpern et al., 2012) and the “ecosystem-based quality index” (Thibaut et al., 2017).
Other Type of Information Provided
The majority of studies (76%) also included some natural or human-related driver against which the food webs were studied. Out of these studies (N = 376), 61% considered only one driver, while the remaining 39% took into account more than one drivers. A total of 53 drivers were recorded, of which 16 refer to abiotic drivers, 11 to biotic drivers, 14 to anthropogenic drivers and 12 to drivers related to global change (Table 1). The primary driver recorded in the published literature was fisheries (Figure 8A, Table 1), which was incorporated in 23% of all studies (N = 493), followed by temporal variability (15%), spatial variability (10%), species interactions (9%), climate change (8%), eutrophication (7%), and pollution (7%). The diversity of drivers used in the scientific literature has increased from a total of 22 drivers before 2004 to 51 drivers thereafter (Table 1). During these two time periods (1973–2004 and 2005–2018), fisheries (16 and 16%, respectively), temporal variability (10 and 11%), spatial variability (7 and 7%), eutrophication (4 and 5%) and other abiotic drivers (15 and 14%) have been used with the same frequency. The use of drivers linked to global change (4 and 10%), organic matter content/input (0 and 4%), and alien species (0 and 3%), shows a substantial increase during the more recent time period, while the use of other biotic drivers (15 and 9%), species interactions (9 and 6%), pollution (9 and 5%), and hydrographic conditions (6 and 3%) shows a decrease (Figure 8B). Economic analysis aiming to quantify the economic value of the food web components, their functions, or their loss, had been performed in only 2% of the reviewed articles.
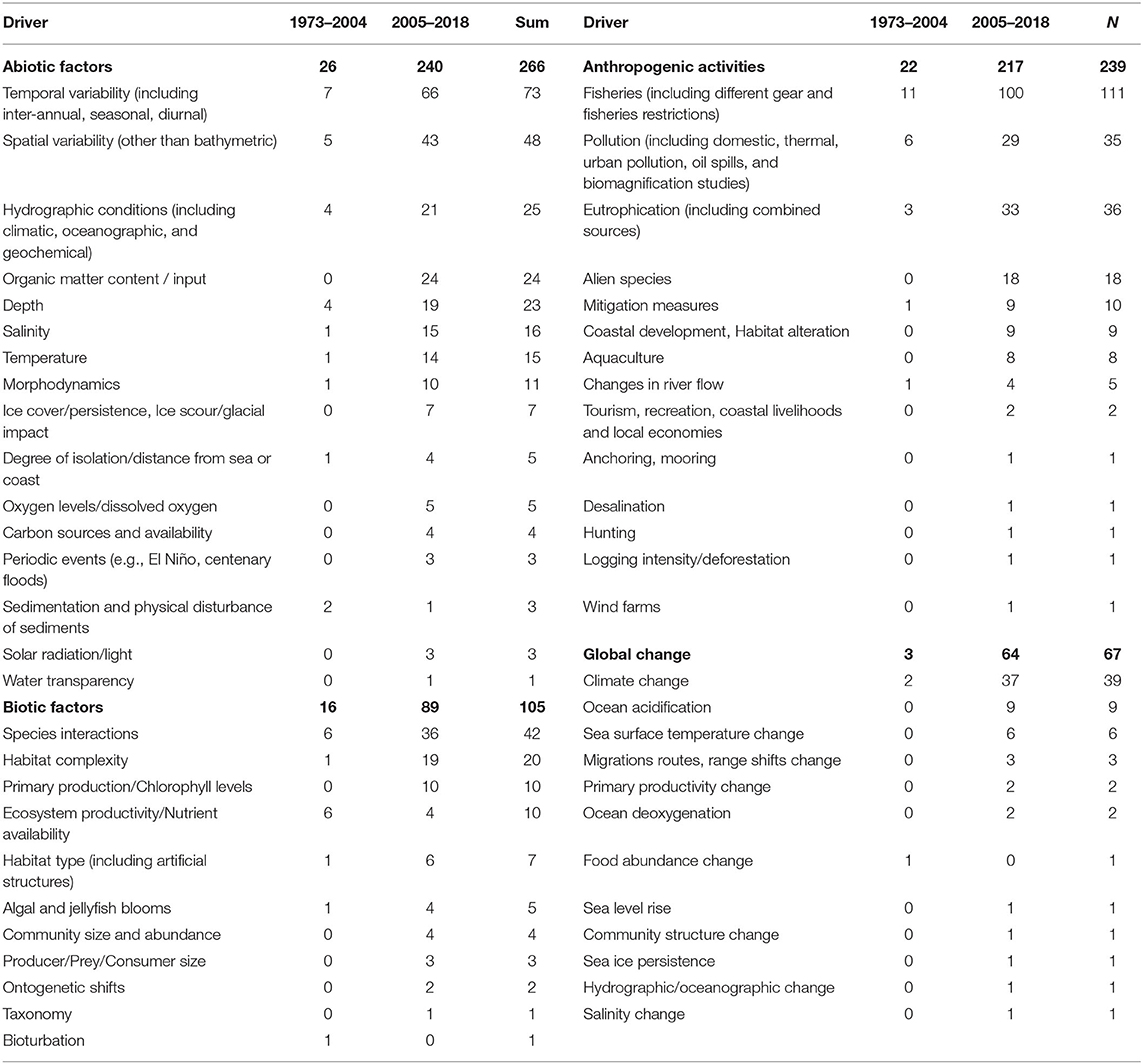
Table 1. Analytical list of all the drivers considered in the food web studies analyzed in this review.
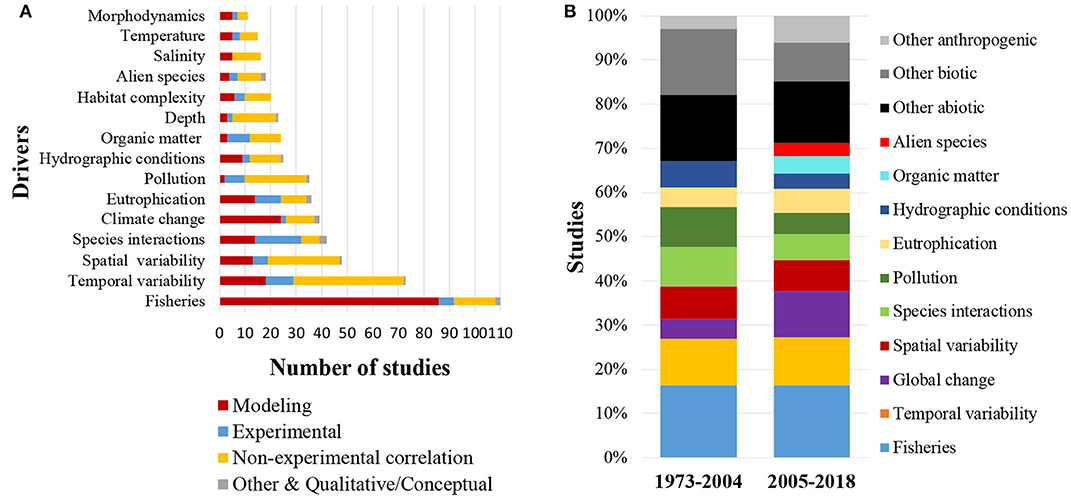
Figure 8. (A) Types of drivers most commonly recorded in the 493 published coastal shelf food web studies (B) Types of drivers most commonly used in the periods 1973–2004 and 2005–2018.
Discussion
The first food web was originally depicted by Camerano (1880), and in the following years ecologists provided different graphical representations (Pierce et al., 1912; Shelford, 1913; Petersen, 1915). More detailed descriptions of food webs (Summerhayes and Elton, 1923; Hardy, 1924), led to the development of the fundamental concepts of food chains, trophic pyramids, and trophic levels (Elton, 1927). In 1942, Lindeman examined aquatic ecosystems in an integrated manner, and introduced the aspect of trophodynamics, i.e., the dynamic processes of trophic interactions. Lindeman's approach can be considered as the cornerstone of food web analysis, and the starting point for the development of the ecosystem approach in marine ecology. Hairston et al. (1960) provided a simplified ecosystem description with the use of only three distinct trophic levels among plants, herbivores and carnivores, after which additional trophic levels were identified. Paine (1966) investigated the intertidal rocky shore community and highlighted the crucial role of predation in shaping it. By 1970s, the critical role of food webs in the thorough understanding of ecosystem processes and the integrated assessment of marine ecosystems was well acknowledged (Odum et al., 1971), while the concept of the linear food chain was gradually substituted by more complex food web analyses (Pomeroy, 1974). However, given the complexity of marine systems, the incorporation of the trophodynamic aspect in the study of marine food webs only became possible after the emergence of ecological modeling (e.g., Polovina and Ow, 1983; Baird and Ulanowicz, 1989), which largely explains the scarcity of studies on coastal food webs before the 1980s. Moreover, advances in the use of stable isotope analysis enabled the more precise description of trophic preferences of various marine species (Schoeninger and DeNiro, 1984). The fundamental theory of the representation and analysis of marine food webs progressed (Pimm, 1982), and the use of specific food web indicators enabled the assessment of ecosystem complexity in relation to stability (Pimm, 1984) and health status (Ulanowicz, 1986). It soon became obvious that changes in the ecosystem can cascade across food webs through trophic interactions (Carpenter et al., 1985), thus affecting food web structure and energy flows (Odum, 1985). Menge and Sutherland (1987) highlighted the role of environmental moderation and biotic processes (e.g., competition, predation, recruitment) as the main drivers affecting trophic structure in marine communities, while Polis et al. (1997) underlined the significance of spatial processes (e.g., movement of animals and nutrients among habitats, physical heterogeneity), in the shaping of food web dynamics.
Soon, the study of trophic relationships was used to investigate the effects of fishing on marine ecosystems (Pauly et al., 1998) and, as shown in the present review, the pressure of fisheries remains the most commonly studied driver of change in coastal shelf food webs. Over the last 20 years, scientific research on coastal shelf food webs has greatly increased, as reflected in the rising rate of relevant published literature. This is mainly due to the development of new analytical techniques and modeling tools, which are relatively easy to access and operate, and which have facilitated the more precise definition of trophic relationships and the detailed exploration of coastal shelf webs (Libralato et al., 2014). The development of the Ecopath modeling software (Christensen and Pauly, 1990) pioneered the multivariate food web analysis options, and the comparative studies among different food webs and ecosystem types (Pauly and Christensen, 1995). The development of Ecopath substantially contributed to the increasing rate of modeling studies after 2000, and based on the present review, it is currently the most common ecosystem modeling approach.
According to our analysis, food web studies have been conducted in all coastal shelf realms and continents. However, most of the studies that met our search criteria focused in the Temperate Northern Atlantic, specifically in marine areas of Europe and North America. This geographic trend can partly be explained by the provision of higher funds for marine research in Europe and North America (UNESCO Institute for Statistics, 2020), where the majority of highly ranked universities is situated (Jöns and Hoyler, 2013). Moreover, our results show that the majority of studies have investigated food webs at a subnational level or supranational level, indicating that studies tend to focus on specific water bodies rather than cover vast marine geographic areas. Nevertheless, as mentioned above, enhanced computer power and new modeling approaches have also allowed the processing of extensive time series thus facilitating the study and comparison of coastal shelf food webs over large spatial scales (i.e., at a national or even global scale; Pauly and Christensen, 1995), as well as their evolution over extensive time scales (more than 400 years) so as to forecast and project future trends in response to global change or other sources of ecosystem alteration (e.g., Hoover et al., 2013; Neira et al., 2014; Hattab et al., 2016; Eddy et al., 2017). Still, approaches that are largely driven by administrative boundaries and access to data often limit the validity of the outputs, as political boundaries commonly do not reflect meaningful ecological boundaries. Species, habitats, as well as many physical or human induced drivers of coastal food web structure (such as marine currents and pollution) move across political boundaries, thus creating strong interdependence among countries. Transboundary collaboration, data sharing and common research efforts at the ecoregional level, as opposed to unilateral uncoordinated efforts at a national or subnational scale, can lead to improved efficiency of food web assessments and ecosystem-based management (Giakoumi et al., 2013; Mazor et al., 2013; Katsanevakis et al., 2015).
All food web studies that met our search criteria focus in coastal ecosystems, but only few of them refer to a particular habitat type (specifically, sand/mud, rocky reefs, seagrasses or coral reefs). Approximately 50% of the studies do not account for habitat type, while 9% of the studies do not even mention the depth range of focus. Moreover, 50% of the studies have not incorporated seasonality into their analysis and/or do not provide sufficient details regarding the timing and the periodicity of sample collection. Food webs are known to be greatly affected by the physical environment (Briand, 1983; Shears et al., 2008), while depth, habitat type, habitat complexity, and seasonality have been identified as key components both in the structuring of coastal shelf food webs (e.g., Torruco et al., 2007; Abrantes et al., 2015; Ferriss et al., 2016), as well as in the intensity of specific human impacts that shape them, such as fisheries or eutrophication (e.g., Nilsson and Ziegler, 2007; Kritzer et al., 2016; Quillien et al., 2016). Temporal variability is generally related to changes in community composition, quantity and origin of organic matter, productivity levels, and availability of resources, as well as the size structure and ontogenetic shifts of dominant consumers (Akin and Winemiller, 2006; Tallis, 2009). On the other hand, depth and habitat type or habitat complexity are commonly linked to ontogenetic differences and changes in community structure (e.g., Shears et al., 2008; Schmidt et al., 2011; Hattab et al., 2013; Sini et al., 2019). Specifically accounting for depth, habitat type, and temporal variability is not only important for the better understanding of the food webs and the role of the multiple trophic links and pathways in the overall functioning of an ecological system, but it is also essential for the cross comparisons of datasets at different spatiotemporal scales and the prioritization of areas for conservation and management (Abrantes et al., 2015).
The vast majority of coastal shelf food web studies classified organisms into distinct biotic groups, rather than providing information on selected species only. Notably, in several cases, biotic groups were defined as “functional groups,” although the actual categorization was based on the wider taxonomic status of organisms (e.g., whales, dolphins, birds, macrobenthos), rather than on purely functional criteria. Other biotic groups were usually related to body size, age, or feeding habits (e.g., trophic or feeding guilds). Regardless the case, most studies assigned species or biotic groups into distinct trophic levels (as also reported by Libralato et al., 2014) and used different methods to define trophic levels. Investigation of trophic relations is a difficult process in marine ecosystems (Libralato et al., 2014), and as seen in our results there is still great heterogeneity in the methods of trophic level estimations (e.g., Mathews, 1993; Søreide et al., 2006).
Stable isotope analysis is currently the most extensively used method for the identification of trophic levels (present study; Vander Zanden and Rasmussen, 1999). One of its main advantages is that it allows the determination of trophic position with an increased accuracy, as stable isotopes incorporate assimilated dietary information over long periods of time (De Niro and Epstein, 1981). On the other hand, the complexity of marine food webs (Isaacs, 1973) cannot be fully captured by the stable isotope technique (Post, 2002; Boecklen et al., 2011). For example, certain food types, like detrital matter, may originate from various sources, while several species may change their feeding preferences according to size, ontogenetic changes, stochastic reasons, or simply because they are omnivores (Pimm et al., 1991). Species that act both as prey and predator can have isotopic signatures that are difficult to interpret, while, natural change in physical conditions may bring about short term seasonal shifts in the isotopic composition of the organisms and their environment. Thus, although the movement of nutrients between distinct trophic groups can be traced through the use of stable isotopes, predator-prey interactions can be identified with sufficient certainty only among specific groups of species and within rather simplified versions of ecosystems (Sousa et al., 2016).
Along with stable isotopes, lipids and fatty acids are gradually becoming increasingly common in the estimation of trophic level, as they provide reliable information on the long-term diet of organisms. However, fatty acid analyses alone cannot be used to track down the contribution of certain trophic groups within an organism's diet, especially in cases when organisms consume dietary items of different origin (e.g., ice algae vs. pelagic phytoplankton; Søreide et al., 2008). Fatty acid techniques still need to be tested and more broadly applied (Valiela, 2015).
The traditionally used gut content analysis also presents several important limitations. A main drawback is the failure to identify all prey items, especially when there are no hard structures (like otoliths, scales, beaks, carapaces, or shells). Moreover, it only provides a snapshot of the feeding habits of an individual, hence it requires large sample sizes in order to assess the seasonal and spatial variability of the dietary preferences of a species during its different life stages (Paine, 1988). This is particular problematic for species that go through ontogenetic dietary shifts, and for large piscivorous fish which consume a very wide range of prey items that are of highly variable abundance in space and time (Armstrong and Schindler, 2011). Problems related to the regurgitation of stomach contents of the predator due to stress during capture, the time of feeding vs. the time of capture of the predator, as well as the differential digestion rates of different prey items, provide extra sources of bias related to the type and abundance of prey items found in the gut contents. Most importantly, gut content techniques fail to define what prey has been assimilated into predator growth, as not all the captured prey items are eventually digested and assimilated; unassimilated material is released back to the environment in the form of unused feces (Pinnegar and Polunin, 1999). Moreover, gut content analysis requires either destructive sampling or stressful protocols for the stomach flushing (Jarman et al., 2002; Bucklin et al., 2011). For all these reasons, gut content analysis is gradually being substituted by more advanced techniques, such as stable isotopes and fatty acid analysis, and is used to supplement, compare or verify the results of the latter (e.g., Rodríguez-Graña et al., 2008; Paiva et al., 2013).
In the present review only three studies were found to use molecular tools for the determination of trophic level (Menge et al., 1999; Lindeque et al., 2014; Brannock et al., 2016). Nevertheless, molecular analysis, including DNA barcoding, is a promising new approach that is expected to advance the quality of dietary information. Samples of gut content or fecal material, containing even partially digested prey items, can provide information on the presence (Valentini et al., 2009; Jo et al., 2014), or even the frequency of occurrence (Bowser et al., 2013) of different prey items. As molecular techniques are progressing, new tools facilitate the study of ever more complex food webs (e.g., next generation sequencing; Pompanon et al., 2012), revealing the diets of fish, mammals, and seabirds (Jarman et al., 2002; Leray et al., 2013; De Barba et al., 2014). The main disadvantage of the molecular approach is that DNA sequences of dietary items may be altered or partly broken by the time they are measured (King et al., 2008). Moreover, techniques such as DNA barcoding are still quite complex and costly to apply (Valentini et al., 2009; Bucklin et al., 2011; O'Rorke et al., 2012).
All the aforementioned techniques (used in isolation or in combination) have improved the determination of trophic level and the resolution of trophic relations (Kohlbach et al., 2016, 2017). Along with these analytical methods, certain other approaches may also be used to provide qualitative supplementary information on food preferences in certain cases. For example, the structure of organs involved in the capture and consumption of prey items (e.g., Platt and Warwick, 1980), observations of feeding habits through direct observations (e.g., Paine, 1980; Kvitek and Bretz, 2005), field enclosure/exclusion experiments, and mesocosm experiments (e.g., O'Connor and Bruno, 2009; Kotta et al., 2018) may provide direct evidence on the trophic preferences of a species. However, such approaches can only be useful in a small number of studies dealing with the feeding habits of specific conspicuous marine consumers within spatiotemporally restricted environments, and thus have limited application in contemporary food web science.
Apart from trophic level determination, approximately one third of the reviewed studies provided a more elaborate quantitative analysis on the energy and/or carbon transfer through coastal shelf food webs. Such type of analysis requires detailed knowledge of the organisms' energy budgets, and/or nutritional composition usually acquired by some of the above-mentioned trophic level determination techniques. However, the estimation of the net energy transfer between trophic levels is a particularly challenging issue in the study of trophic ecology, especially when dealing with highly complex systems like the coastal shelf ecosystems. The problem arises from the fact that a significant amount of energy is lost to the environment, as the assimilated energy derived from food resources is being transferred from one trophic level to the next (Valiela, 2015). Energy is lost either through defecation of unassimilated matter, or through the use of assimilated energy for the maintenance of metabolic processes and activity levels (usually collectively referred to as respiration). The energy that is assimilated but not respired is dedicated to production, i.e., mainly growth and reproduction (Valiela, 2015). In order to estimate the net energy transfer, it is important to consider all the physiological and ecological processes involved, along with the abiotic conditions that affect them. In the majority of relevant studies this problem is partly being tackled through the use of modeling approaches (e.g., Wollrab et al., 2012; Jennings and Collingridge, 2015) and, as seen through the present review, the majority of these studies utilize modeling for the estimation of energy or carbon flow through coastal shelf food webs.
Modeling has become the primary tool in the study of marine food webs and, as mentioned above, it is partly responsible for the rapid increase of coastal food web studies in the last 20 years. It facilitates the quantitative reconstruction of food webs, since population state variables, trophic levels, trophic links, ecological processes (e.g., emigration or immigration), energy transfer estimations, as well as the abiotic or human factors that affect them can be combined within sophisticated models. It is thus the only approach that can provide an integrated analysis of whole communities within extended spatiotemporal scales, along with the respective environmental or anthropogenic drivers (Shannon et al., 2014). On the other hand, the quality of ecosystem modeling results largely depends on data availability, the uncertainty in model structure, parameter estimates and the exploration of alternative functional response hypotheses (Plagányi, 2007). Hence, modeling approaches, as well as the respective simulations and future predictions, can be of low value when the lack of sufficient data on dietary information, trophic links and ecological processes leads to low confidence in respective results (Yaragina and Dolgov, 2009; Shannon et al., 2014). Limitations of Ecopath models are also largely attributed to the inadequate inclusion of uncertainty in data inputs and model structure (Plagányi and Butterworth, 2004) and the insufficient setting of default parameters by users (Christensen et al., 2005; Plagányi, 2007).
Despite the great progress achieved, food web analysis appears to be constrained by multiple uncertainties due to the incompleteness of trophic data and the notable variability of factors that shape the structure and functioning of food webs (Valiela, 2015). Important knowledge gaps regarding population state variables, dietary information, trophic level estimation and ecological processes still exist even in well studied food webs (Link et al., 2008). Although certain groups of species, such as fish or other large prominent species, have received increased attention, there is still a great lack of quantitative information regarding the majority of marine coastal shelf organisms. This is especially true for several benthic invertebrates, such as filter feeders (Peters and Schaaf, 1991), or even for commercial demersal fish in certain areas (Barausse et al., 2009). Specifically, there is a complete lack of information on the population state variables and trophic links of infauna populations in nearly all food webs, thus adding uncertainty to the respective research outputs (Savenkoff et al., 2007). Besides the commonly highlighted lack of sufficient information regarding the highly diverse and less easily identified macro- or meiobenthic groups, abundance data of top predators are also often missing (Savenkoff et al., 2007). Moreover, dietary information for all fish, invertebrates, and top predators (such as seals, cetaceans and sharks) needs to be more complete for a thorough and robust food web analysis (Savenkoff et al., 2007; Link et al., 2008; Barausse et al., 2009; Gjoni and Basset, 2018). Results on trophic relationships are often conflicting (Båmstedt et al., 2000), as organisms commonly consume a mixture of different food sources (Valiela, 2015), while certain consumers may even change trophic level according to food availability (Tamelander et al., 2008). Correctly defining the trophic relationships according to life stage and/or size class is also essential as several marine organisms go through ontogenetic dietary shifts, and hence occupy a different ecological position in the food web (Savenkoff et al., 2007; Al-Habsi et al., 2008; Albouy et al., 2014). Likewise, several ecological processes, such as productivity, recruitment, ontogenetic shifts, migration, predation and mortality rates (Peters and Schaaf, 1991; O'Gorman et al., 2008; Albouy et al., 2014) need to be better estimated.
One of the main hurdles in correctly defining coastal shelf food webs is that marine assemblages are not autonomous entities, but open systems (Hawkins, 2004) that are subjected to numerous natural and anthropogenic drivers. When compared to offshore pelagic or bathyal food webs, coastal food webs have higher spatio-temporal variability (as the environmental conditions are more variable near the coasts), are greatly affected by land-sea interactions, and are directly exposed to numerous human stressors, all of which add further complexity to their study. This is readily evident when looking at the base of the coastal shelf food webs. Coastal systems are energetically supported by a variety of sources, including local benthic or pelagic producers, as well as allochthonous organic matter which may originate either from land via freshwater run-off or from the open sea through tidal currents and upwellings (Nielsen and Navarrete, 2004; Carlier et al., 2015; Christianen et al., 2017; Meunier et al., 2017). Moreover, the proportional contribution of the different energy sources to coastal shelf food webs may also be affected by several human-induced drivers, such as coastal development, seabed disturbance, eutrophication, and changes in river flow (Tewfik et al., 2005; Al-Habsi et al., 2008; Christianen et al., 2017; Hemraj et al., 2017). Today, it is clear that long term anthropogenic activities can cause major shifts in coastal shelf food web structure, which lead to simplified communities (Tewfik et al., 2005). It is therefore of utmost importance to accurately define anthropogenic stressors, understand their links to natural processes, and estimate the overall cumulative effects, in order to set up realistic thresholds of ecosystem resilience (Shears et al., 2008; O'Gorman et al., 2012; Giakoumi et al., 2015; Stelzenmüller et al., 2018). However, cause-effect pathways of cumulative pressures on coastal shelf food webs are often complex, involving a combination of additive, synergistic and antagonistic impacts on ecosystems (Crain et al., 2008; Stelzenmüller et al., 2018). At present, existing knowledge on the effects of cumulative natural and anthropogenic drivers on different coastal shelf food web compartments remains limited (Giakoumi et al., 2015), and constitutes one of the great challenges in marine ecosystem ecology (Borja et al., 2020). Cumulative effects assessments need to be well-framed into a risk-based framework, properly accounting for and communicating uncertainty, in order to function as operational tools that can guide management actions for protecting coastal shelf food webs (Stelzenmüller et al., 2018, 2020).
Whereas, the effects of certain drivers have been more extensively and regularly investigated throughout the years (e.g., fisheries, spatial and temporal variability), others remain much less studied and understood (e.g., species interactions, climate change, habitat alterations). The increased use of drivers related to upcoming threats in the more recent literature, such as those linked to global change, alien species and other anthropogenic drivers, is partly due to the advancements of modeling approaches, but also reflects the need to provide future predictions that will allow human communities to adapt to the changing conditions (Lawler et al., 2010; Essl et al., 2020). Although understanding the effects of such threats is critical in order to propose effective management strategies and impact mitigation actions, at present there is a low level of certainty both with regards to the extent of certain threats (e.g., climate change and alien species) and to the nature and magnitude of their present and future impacts on coastal shelf food webs (Lawler et al., 2010; Ainsworth et al., 2011; Giakoumi et al., 2015). Besides, the interaction among different drivers (e.g., the impacts of alien species are driven by other drivers such as climate change, eutrophication and pollution; Essl et al., 2020) further complicates assessments of cumulative effects on coastal shelf food webs.
In order to reduce biodiversity loss and the deterioration of coastal shelf food webs, quantitative knowledge on community state variables and trophic links needs to be constantly updated, while the respective effects of the various environmental processes and anthropogenic stressors need to be better defined and quantified. This will facilitate a better understanding of ecological processes and the overall functioning of coastal shelf systems, which will enable more accurate estimations of ecosystem resilience and predictions of potential impacts, and will contribute to more informed and effective management.
Finally, given the limitation of modeling approaches when studying data-deprived food webs and ecosystems, systematic model-independent data should be used to reinforce ecosystem modeling results (Coll et al., 2009). From then on, further insights to food web changes could be achieved through the dynamic simulations and comparison to other past or present food webs (Coll et al., 2008).
Data Availability Statement
The original contributions presented in the study are included in the article/Supplementary Material, further data inquiries can be directed to the corresponding author/s.
Author Contributions
SK contributed in the conception, design and coordination of the study, and the discussion. EK, MS, and YI equally participated in the paper screening during the review. EK contributed in the design and first analysis, wrote the first draft of introduction and methods and contributed to the drafting of the discussion. MS carried out most of the analysis, prepared graphs and figures, wrote first draft for the results and contributed to the drafting of the discussion. YI reviewed the indices, contributed to the results and the preparation of figures. All authors have contributed to manuscript revision, and have read and approved the submitted version.
Funding
This research is carried out/funded in the context of the project ‘Development of innovative methods for the study of marine food webs' (MIS 5004302) under the call for proposals ‘Supporting researchers with an emphasis on new researchers' (EDULLL 34). The project is co-financed by Greece and the European Union (European Social Fund - ESF) by the Operational Programme Human Resources Development, Education and Lifelong Learning 2014-2020.
Conflict of Interest
The authors declare that the research was conducted in the absence of any commercial or financial relationships that could be construed as a potential conflict of interest.
Supplementary Material
The Supplementary Material for this article can be found online at: https://www.frontiersin.org/articles/10.3389/fmars.2020.00636/full#supplementary-material
References
Abdurahiman, K. P., Nayak, T. H., Zacharia, P. U., and Mohamed, K. S. (2010). Trophic organization and predator-prey interactions among commercially exploited demersal finfishes in the coastal waters of the southeastern Arabian Sea. Estuar. Coast. Shelf. S. 87, 601–610. doi: 10.1016/j.ecss.2010.03.002
Abrantes, K. G., Barnett, A., Baker, R., and Sheaves, M. (2015). Habitat-specific food webs and trophic interactions supporting coastal-dependent fishery species: an Australian case study. Rev. Fish. Biol. Fisher. 25, 337–363. doi: 10.1007/s11160-015-9385-y
Ainley, D. G., Dugger, K. D., Ford, R. G., Pierce, S. D., Reese, D. C., Brodeur, R. D., et al. (2009). Association of predators and prey at frontal features in the California Current: competition, facilitation, and co-occurrence. Mar. Ecol. Prog. Ser. 389, 271–294. doi: 10.3354/meps08153
Ainsworth, C. H., Samhouri, J. F., Busch, D. S., Cheung, W. W. L., Dunne, J., and Okey, T. A. (2011). Potential impacts of climate change on Northeast Pacific marine foodwebs and fisheries. ICES J. Mar. Sci. 68, 1217–1229. doi: 10.1093/icesjms/fsr043
Akin, S., and Winemiller, K. O. (2006). Seasonal variation in food web composition and structure in a temperate tidal estuary. Estuar. Coast. 29, 552–567. doi: 10.1007/BF02784282
Albouy, C., Mouillot, D., Rocklin, D., Culioli, J. M., and Le Loc'h, F. (2010). Simulation of the combined effects of artisanal and recreational fisheries on a Mediterranean MPA ecosystem using a trophic model. Mar. Ecol. Prog. Ser. 412, 207–221. doi: 10.3354/meps08679
Albouy, C., Velez, L., Coll, M., Colloca, F., Le Loc'h, F., and Mouillot, D. (2014). From projected species distribution to food-web structure under climate change. Glob. Change Biol. 20, 730–741. doi: 10.1111/gcb.12467
Al-Habsi, S. H., Sweeting, C. J., Polunin, N. V. C., and Graham, N. A. J. (2008). δ15N and δ13C elucidation of size-structured food webs in a western arabian sea demersal trawl assemblage. Mar. Ecol. Prog. Ser. 353, 55–63. doi: 10.3354/meps07167
Anjusha, A., Jyothibabu, R., Jagadeesan, L., Mohan, A. P., Sudheesh, K., Krishna, K., et al. (2013). Trophic efficiency of plankton food webs: observations from the Gulf of Mannar and the Palk Bay, Southeast Coast of India. J. Marine Syst. 115, 40–61. doi: 10.1016/j.jmarsys.2013.02.003
Apitz, S. E., Elliott, M., Fountain, M., and Galloway, T. S. (2006). European environmental management: Moving to an ecosystem approach. Integr. Environ. Asses. 2, 80–85. doi: 10.1002/ieam.5630020114
Armitage, A. R., and Fourqurean, J. W. (2009). Stable isotopes reveal complex changes in trophic relationships following nutrient addition in a coastal marine ecosystem. Estuar. Coast. 32, 1152–1164. doi: 10.1007/s12237-009-9219-z
Armstrong, J. B., and Schindler, D. E. (2011). Excess digestive capacity in predators reflects a life of feast and famine. Nature 476, 84–87. doi: 10.1038/nature10240
Aurioles-Gamboa, D., Rodríguez-Pérez, M. Y., Sánchez-Velasco, L., and Lavín, M. F. (2013). Habitat, trophic level, and residence of marine mammals in the Gulf of California assessed by stable isotope analysis. Mar. Ecol. Prog. Ser. 488, 275–290. doi: 10.3354/meps10369
Båmstedt, U., Gifford, D. J., Irigoien, X., Atkinson, A., and Roman, M. (2000). “Feeding” in ICES Zooplankton Methodological Manual, eds R. Harris, P. Wiebe, J. Lenz, H. R. Skjødal, and M. Huntley (New York, NY: Academic Press), 297–399. doi: 10.1016/B978-012327645-2/50009-8
Baird, D., and Ulanowicz, R. E. (1989). The seasonal dynamics of the Chesapeake Bay ecosystem. Ecol. Monogr. 59, 329–364. doi: 10.2307/1943071
Bakun, A. (1996). “Patterns in the Ocean: Ocean Processes and Marine Population Dynamics. University of California Sea Grant, San Diego, CA, USA,” in Cooperation With Centro de Investigaciones Biológicas de Noroeste (La Paz, Baja California Sur, Mexico), 323.
Bakun, A. (2004). “Chapter 24 – REgime shifts” in The Sea (Volume 13), eds A.R., Robinson, J., McCarthy, and B. J., Rothschild (Cambridge: Harvard University Press), 971–1018.
Bakun, A. (2006). Wasp-waist populations and marine ecosystem dynamics: navigating the “predator pit” topographies. Prog. Oceanogr. 68, 271–288. doi: 10.1016/j.pocean.2006.02.004
Bǎnaru, D., Mellon-Duval, C., Roos, D., Bigot, J. –L., Souplet, A., Jadaud, A., et al. (2013). Trophic structure in the Gulf of Lions marine ecosystem (north-western Mediterranean Sea) and fishing impacts. J. Mar. Syst. 111–112, 45–68. doi: 10.1016/j.jmarsys.2012.09.010
Barausse, A., Duci, A., Mazzoldi, C., Artioli, Y., and Palmeri, L. (2009). Trophic network model of the Northern Adriatic Sea: Analysis of an exploited and eutrophic ecosystem. Estuar. Coast. Shelf. S. 83, 577–590. doi: 10.1016/j.ecss.2009.05.003
Behringer, D. C., and Butler, M. J. (2006). Stable isotope analysis of production and trophic relationships in a tropical marine hard-bottom community. Oecologia 148, 334–341. doi: 10.1007/s00442-006-0360-0
Bell, L. E., Bluhm, B. A., and Iken, K. (2016). Influence of terrestrial organic matter in marine food webs of the Beaufort Sea shelf and slope. Mar. Ecol. Prog. Ser. 550, 1–24. doi: 10.3354/meps11725
Bergström, L., Karlsson, M., Bergström, U., Pihl, L., and Kraufvelin, P. (2016). Distribution of mesopredatory fish determined by habitat variables in a predator-depleted coastal system. Mar. Biol. 163:201. doi: 10.1007/s00227-016-2977-9
Bishop, M. J., Coleman, M. A., and Kelaher, B. P. (2010). Cross-habitat impacts of species decline: response of estuarine sediment communities to changing detrital resources. Oecologia 163, 517–525. doi: 10.1007/s00442-009-1555-y
Blachowiak-Samolyk, K., Kwasniewski, S., Dmoch, K., Hop, H., and Falk-Petersen, S. (2007). Trophic structure of zooplankton in the Fram Strait in spring and autumn 2003. Deep-Sea Res. Pt. II 54, 2716–2728. doi: 10.1016/j.dsr2.2007.08.004
Boecklen, W. J., Yarnes, C. T., Cook, B. A., and James, A. C. (2011). On the use of stable isotopes in trophic ecology. Annu. Rev. Ecol. Evol. Syst. 42, 411–440. doi: 10.1146/annurev-ecolsys-102209-144726
Borja, A., Andersen, J. H., Arvanitidis, C. D., Basset, A., Buhl-Mortensen, L., Carvalho, S., et al. (2020). Past and future grand challenges in marine ecosystem ecology. Front. Mar. Sci. 7:362. doi: 10.3389/fmars.2020.00362
Bowser, A. K., Diamond, A. W., and Addison, J. A. (2013). From puffins to plankton: a DNA-based analysis of a seabird food chain in the Northern Gulf of Maine. PLoS ONE 8:e83152. doi: 10.1371/journal.pone.0083152
Brannock, P. M., Wang, L., Ortmann, A. C., Waits, D. S., and Halanych, K. M. (2016). Genetic assessment of meiobenthic community composition and spatial distribution in coastal sediments along northern Gulf of Mexico. Mar. Environ. Res. 119, 166–175. doi: 10.1016/j.marenvres.2016.05.011
Briand, F. (1983). Environmental control of food web structure. Ecology 64, 253–263. doi: 10.2307/1937073
Brind'Amour, A., Rouyer, A., and Martin, J. (2009). Functional gains of including non-commercial epibenthic taxa in coastal beam trawl surveys: a note. Cont. Shelf Res. 29, 1189–1194. doi: 10.1016/j.csr.2009.01.003
Bucklin, A., Steinke, D., and Blanco-Bercial, L. (2011). DNA barcoding of marine metazoa. Annu. Rev. Mar. Sci. 3, 471–508. doi: 10.1146/annurev-marine-120308-080950
Bulleri, F., and Chapman, M. G. (2010). The introduction of coastal infrastructure as a driver of change in marine environments. J. Appl. Ecol. 47, 26–35. doi: 10.1111/j.1365-2664.2009.01751.x
Cai, D., Li, H., Tang, Q., and Sun, Y. (2005). Establishment of trophic continuum in the food web of the Yellow Sea and East China Sea ecosystem: Insight from carbon and nitrogen stable isotopes. Sci. China Ser. C 48, 531–539. doi: 10.1360/04yc0136
Camerano, L. (1880). Dell' equilibrio dei viventi mercè la reciproca distruzione. Att. Real. Acc. Sci. Torino 15, 393–414.
Carlier, A., Chauvaud, L., van der Geest, M., Le Loc'h, F., Le Duff, M., Vernet, M., et al. (2015). Trophic connectivity between offshore upwelling and the inshore food web of Banc d'Arguin (Mauritania): New insights from isotopic analysis. Estuar. Coast. Shelf. S. 165, 149–158. doi: 10.1016/j.ecss.2015.05.001
Caron, D. A., Connell, P. E., Schaffner, R. A., Schnetzer, A., Fuhrman, J. A., Countway, P. D., et al. (2017). Planktonic food web structure at a coastal time-series site: I. Partitioning of microbial abundances and carbon biomass. Deep Sea Res. Pt. I 121, 14–29. doi: 10.1016/j.dsr.2016.12.013
Carpenter, S. R., Kitchell, J. F., and Hodgson, J. R. (1985). Cascading trophic interactions and lake productivity: Fish predation and herbivory can regulate lake ecosystems. BioScience 35, 634–639. doi: 10.2307/1309989
Chen, Z., Xu, S., and He, P. (2011). An ecological model of the artificial ecosystem (northern Hangzhou Bay, China): analysis of ecosystem structure and fishing impacts. Helgoland Mar. Res. 65, 217–231. doi: 10.1007/s10152-010-0218-z
Chen, Z., Xu, S., and Qiu, Y. (2015). Using a food-web model to assess the trophic structure and energy flows in Daya Bay, China. Cont. Shelf Res. 111, 316–326. doi: 10.1016/j.csr.2015.08.013
Chikaraishi, Y., Steffan, S. A., Ogawa, N. O., Ishikawa, N. F., Sasaki, Y., Tsuchiya, M., et al. (2014). High-resolution food webs based on nitrogen isotopic composition of amino acids. Ecol. Evol. 4, 2423–2449. doi: 10.1002/ece3.1103
Christensen, V., and Pauly, D. (1992). ECOPATH II—a software for balancing steady-state ecosystem models and calculating network characteristics. Ecol. Model. 61, pp.169–185. doi: 10.1016/0304-3800(92)90016-8
Christensen, V., Walters, C., and Pauly, D. (2005). Ecopath With Ecosim: A User's Guide. Vancouver: Fisheries Centre of University of British Columbia.
Christensen, V., Walters, C., Pauly, D., and Forrest, R. (2008). Ecopath With Ecosim 6: A User's guide. Vancouver: Fisheries Centre, University of British Columbia.
Christensen, V, and Pauly, D. (1990). A Draft Guide to the ECOPATH II Program (ver. 1.0). Manila: ICLARM Software 6, ICLARM.
Christianen, M. J. A., Middelburg, J. J., Holthuijsen, S. J., Jouta, J., Compton, T. J., van der Heide, T., et al. (2017). Benthic primary producers are key to sustain the Wadden Sea food web: stable carbon isotope analysis at landscape scale. Ecology 98, 1498–1512. doi: 10.1002/ecy.1837
Cohen, J. E., Beaver, R. A., Cousins, S. H., DeAngelis, D. L., Goldwasser, L., Heong, K. L., et al. (1993). Improving food webs. Ecology 74, 252–258. doi: 10.2307/1939520
Cohen, J. E., and Briand, F. (1984). Trophic links of community food webs. Proc. Natl. Acad. Sci. U.S.A. 81, 4105–4109. doi: 10.1073/pnas.81.13.4105
Coll, M., Lotze, H. K., and Romanuk, T. N. (2008). Structural degradation in Mediterranean Sea food webs: testing ecological hypotheses using stochastic and mass-balance modelling. Ecosystems 11, 939–960. doi: 10.1007/s10021-008-9171-y
Coll, M., Palomera, I., and Tudela, S. (2009). Decadal changes in a NW Mediterranean Sea food web in relation to fishing exploitation. Ecol. Model. 220, 2088–2102. doi: 10.1016/j.ecolmodel.2009.04.049
Coll, M., Schmidt, A., Romanuk, T., and Lotze, H. K. (2011). Food web structure of seagrass communities across different spatial scales and human impacts. PLoS ONE 6:e22591. doi: 10.1371/journal.pone.0022591
Corrales, X., Coll, M., Ofir, E., Piroddi, C., Goren, M., Edelist, D., et al. (2017b). Hindcasting the dynamics of an eastern Mediterranean marine ecosystem under the impacts of multiple stressors. Mar. Ecol. Prog. Ser. 580, 17–36. doi: 10.3354/meps12271
Corrales, X., Ofir, E., Coll, M., Goren, M., Edelist, D., Heymans, J. J., et al. (2017a). Modeling the role and impact of alien species and fisheries on the Israeli marine continental shelf ecosystem. J. Mar. Syst. 170, 88–102. doi: 10.1016/j.jmarsys.2017.02.004
Crain, C. M., Kroeker, K., and Halpern, B. S. (2008). Interactive and cumulative effects of multiple human stressors in marine systems. Ecol. Lett. 11, 1304–1315. doi: 10.1111/j.1461-0248.2008.01253.x
Cury, P., Bakun, A., Crawford, R. J. M., Jarre-Teichmann, A., Quinones, R. A., Shannon, L. J., et al. (2000). Small pelagics in upwelling systems: patterns of interaction and structural changes in “wasp-waist” ecosystems. ICES J. Mar. Sci. 210, 603–618. doi: 10.1006/jmsc.2000.0712
Cury, P., Fréon, P., Moloney, C. L., Shannon, L., and Shin, Y.-J. (2004). “Processes and patterns of interactions in marine fish populations: an ecosystem perspective,” in The Sea (Volume 13), eds A. R. Robinson, J. McCarthy, and B. J. Rothschild (Cambridge: Harvard University Press) 475–533.
De Barba, M, Miquel, C, Boyer, F., Mercier, C., Rioux, D., and Coissac, E. (2014), DNA metabarcoding multiplexing validation of data accuracy for diet assessment: application to omnivorous diet. Mol. Ecol. Resour. 14, 306–323. doi: 10.1111/1755-0998.12188.
De Niro, M. J., and Epstein, J. E. (1981). Influence of diet on the distribution of nitrogen isotopes in animals. Geochim. Cosmochim. Acta 45, 341–351. doi: 10.1016/0016-7037(81)90244-1
Di Beneditto, A. P. M., Bittar, V. T., Camargo, P. B., Rezende, C. E., and Kehrig, H. A. (2012). Mercury and nitrogen isotope in a marine species from a tropical coastal food web. Arch. Environ. Con. Tox. 62, 264–271. doi: 10.1007/s00244-011-9701-z
Donadi, S., Austin, Å. N., Bergström, U., Eriksson, B. K., Hansen, J. P., Jacobson, P., et al. (2017). A cross-scale trophic cascade from large predatory fish to algae in coastal ecosystems. P. Roy. Soc. B Biol. Sci. 284:45. doi: 10.1098/rspb.2017.0045
Dunic, J. C., and Baum, J. K. (2017). Size structuring and allometric scaling relationships in coral reef fishes. J. Anim. Ecol. 86, 577–589. doi: 10.1111/1365-2656.12637
Dunton, K. H., Schonberg, S. V., and Cooper, L. W. (2012). Food web structure of the Alaskan nearshore shelf and estuarine lagoons of the Beaufort Sea. Estuar. Coast. 35, 416–435. doi: 10.1007/s12237-012-9475-1
Eddy, T. D., Lotze, H. K., Fulton, E. A., Coll, M., Ainsworth, C. H., Neves, J. A., et al. (2017). Ecosystem effects of invertebrate fisheries. Fish Fish. 18, 40–53. doi: 10.1111/faf.12165
Edgar, G. J., Banks, S. A., Bessudo, S., Cortés, J., Guzmán, H. M., Henderson, S., et al. (2011). Variation in reef fish and invertebrate communities with level of protection from fishing across the Eastern Tropical Pacific seascape. Global Ecol. Biogeogr. 20, 730–743. doi: 10.1111/j.1466-8238.2010.00642.x
Edgar, G. J., Stuart-Smith, R. D., Thomson, R. J., and Freeman, D. J. (2017). Consistent multi-level trophic effects of marine reserve protection across northern New Zealand. PLoS ONE 12:e0177216. doi: 10.1371/journal.pone.0177216
Essl, F., Lenzner, B., Bacher, S., Bailey, S., Capinha, C., Daehler, C., et al. (2020). Drivers of future alien species impacts: an expert-based assessment. Glob. Change Biol. doi: 10.1111/gcb.15199. [Epub ahead of print].
Estes, J. A. (1996). “The influence of large, mobile predators in aquatic food webs: examples from sea otters and kelp forests,” in Aquatic Predators and their Prey, eds S. Greenstreet and M. Tasker (London: Blackwell), 65–72.
EU (2008). Directive 2008/56/EC of the European Parliament and of the Council of 17 June 2008 Establishing a Framework for Community Action in the field of Marine Environmental Policy (Marine Strategy Framework Directive). Official Journal of the European Union 164, 19-40.
Faye, D., de Morais, L. T., Raffray, J., Sadio, O., Thiaw, O. T., and Le Loc'h, F. (2011). Structure and seasonal variability of fish food webs in an estuarine tropical marine protected area (Senegal): evidence from stable isotope analysis. Est. Coast. Shelf S. 92, 607–617. doi: 10.1016/j.ecss.2011.02.017
Ferriss, B. E., Reum, J. C. P., McDonald, P. S., Farrell, D. M., and Harvey, C. J. (2016). Evaluating trophic and non-trophic effects of shellfish aquaculture in a coastal estuarine foodweb. ICES J. Mar. Sci. 73, 429–440. doi: 10.1093/icesjms/fsv173
Finn, J. T. (1976). Measures of ecosystem structure and function derived from analysis of flows. J. Theor. Biol. 56, 363–380. doi: 10.1016/S0022-5193(76)80080-X
French, B., Clarke, K. R., Platell, M. E., and Potter, I. C. (2013). An innovative statistical approach to constructing a readily comprehensible food web for a demersal fish community. Estuar. Coast. Shelf. S. 125, 43–56. doi: 10.1016/j.ecss.2013.03.014
Gardner, M. R., and Ashby, W. R. (1970). Connectance of large dynamic (cybernetic) systems: critical values for stability. Nature 228, 784–784. doi: 10.1038/228784a0
Giakoumi, S., Halpern, B. S., Michel, L. N., Gobert, S., Sini, M., Boudouresque, C.-F., et al. (2015). Towards a framework for assessment and management of cumulative human impacts on marine food webs. Conserv. Biol. 29, 1228–1234. doi: 10.1111/cobi.12468
Giakoumi, S., Sini, M., Gerovasileiou, V., Mazor, T., Beher, J., Possingham, H. P., et al. (2013). Ecoregion-based conservation planning in the Mediterranean: dealing with large-scale heterogeneity. PLoS ONE 8:e76449. doi: 10.1371/journal.pone.0076449
Giraldo, C., Ernande, B., Cresson, P., Kopp, D., Cachera, M., Travers-Trolet, M., et al. (2017). Depth gradient in the resource use of a fish community from a semi-enclosed sea. Limnol. Oceanogr. 62, 2213–2226. doi: 10.1002/lno.10561
Gjoni, V., and Basset, A. (2018). A cross-community approach to energy pathways across lagoon macroinvertebrate guilds. Estuar. Coast 41, 2433–2446. doi: 10.1007/s12237-018-0422-7
Guidetti, P. (2011). The destructive date mussel fishery and the persistence of barrens in Mediterranean rocky reefs. Mar. Pollut. Bull. 62, 691–695. doi: 10.1016/j.marpolbul.2011.01.029
Hairston, N. G., Smith, F. E., and Slodbodkin, L. B. (1960). Community structure, population control and competition. Am. Nat. 94, 421–425. doi: 10.1086/282146
Hall, S. J. (2002). The continental shelf benthic ecosystem: current status, agents for change and future prospects. Environ. Conserv. 29, 350–374 doi: 10.1017/S0376892902000243
Halpern, B. S., Longo, C., Hardy, D., McLeod, K. L., Samhouri, J. F., Katona, S. K., et al. (2012). An index to assess the health and benefits of the global ocean. Nature 488, 615–620. doi: 10.1038/nature11397
Hansen, J. P., Wikström, S. A., and Kautsky, L. (2012). Taxon composition and food-web structure in a morphometric gradient of Baltic sea land-uplift bays. Boreal Environ. Res. 17, 1–19.
Hanson, J. M. (2018). Feeding interactions between fishes in a coastal ecosystem in the southern Gulf of St. Lawrence Atlantic Canada. T. A. Fish. Soc. 147, 61–78. doi: 10.1002/tafs.10021
Hardy, A. C. (1924). The herring in relation to its animate environment. 1. The food and feeding habits of the herring with special reference to the east coast of England. Fish. Invest. London 7, 1–53.
Harriague, A. C., Gaozza, L., Montella, A., and Misic, C. (2006). Benthic communities on a sandy Ligurian beach (NW Mediterranean). Hydrobiologia 571, 383–394. doi: 10.1007/s10750-006-0264-3
Hattab, T., Ben Rais Lasram, F., Albouy, C., Romdhane, M. S., Jarboui, O., Halouani, G., et al. (2013). An ecosystem model of an exploited southern Mediterranean shelf region (Gulf of Gabes, Tunisia) and a comparison with other Mediterranean ecosystem model properties. J. Marine Syst. 128, 159–174. doi: 10.1016/j.jmarsys.2013.04.017
Hattab, T., Leprieur, F., Ben Rais Lasram, F., Gravel, D., Loc'h, F. L., and Albouy, C. (2016). Forecasting fine-scale changes in the food-web structure of coastal marine communities under climate change. Ecography 39, 1227–1237. doi: 10.1111/ecog.01937
Hawkins, S. J. (2004). Scaling up: the role of species and habitat patches in functioning of coastal ecosystems. Aquat. Conserv. 14, 217–219. doi: 10.1002/aqc.637
Heldal, H. E., Bogstad, B., Dolgov, A. V., Gwynn, J. P., and Teien, H. C. (2018). Observations of biota in Stepovogo Fjord, Novaya Zemlya, a former dumping site for radioactive waste. Polar Biol. 41, 115–124. doi: 10.1007/s00300-017-2175-3
Hemraj, D. A., Hossain, A., Ye, Q., Qin, J. G., and Leterme, S. C. (2017). Anthropogenic shift of planktonic food web structure in a coastal lagoon by freshwater flow regulation. Sci. Rep. 7:44441. doi: 10.1038/srep44441
Hoover, C., Pitcher, T., and Christensen, V. (2013). Effects of hunting, fishing and climate change on the Hudson Bay marine ecosystem: II. Ecosystem model future projections. Ecol. Model. 264, 143–156. doi: 10.1016/j.ecolmodel.2013.01.010
Hughes, T. P., Barnes, M. L., Bellwood, D. R., Cinner, J. E., Cumming, G. S., Jackson, J. B. C., et al. (2017). Coral reefs in the anthropocene. Nature 546, 82–90. doi: 10.1038/nature22901
Hunt, J. G. L., and McKinnell, S. (2006). Interplay between top-down, bottom-up, and waspwaist control in marine ecosystems. Prog. Oceanogr. 68, 115–124. doi: 10.1016/j.pocean.2006.02.008
Isaacs, J. D. (1973). Potential trophic biomasses and trace-substance concentrations in unstructured marine food webs. Mar. Biol. 22, 97–104. doi: 10.1007/BF00391775
Iverson, S. J. (2009). “Tracing aquatic food webs using fatty acids: from qualitative indicators to quantitative determination,” in Lipids in Aquatic Ecosystems, eds M. T. Arts et al. (New York, NY: Springer), 281–308 doi: 10.1007/978-0-387-89366-2_12
Jackson, J. B. C., Kirby, M. X., Berger, W. H., Bjorndal, K. A., Botsford, L. W., Bourque, B. J., et al. (2001). Historical overfishing and the recent collapse of coastal ecosystems. Science 293, 629–638 doi: 10.1126/science.1059199
Jankowska, E., De Troch, M., Michel, L. N., Lepoint, G., and Włodarska-Kowalczuk, M. (2018). Modification of benthic food web structure by recovering seagrass meadows, as revealed by trophic markers and mixing models. Ecol. Indic. 90, 28–37. doi: 10.1016/j.ecolind.2018.02.054
Jarman, S. N., Gales, N. J., Tierney, M., Gill, P. C., and Elliott, N. G. A. (2002). DNA-based method for identification of krill species and its application to analysing the diet of marine vertebrate predators. Mol. Ecol. 11, 2679–2690. doi: 10.1046/j.1365-294X.2002.01641.x
Jennings, S., and Collingridge, K. (2015). Predicting consumer biomass, size-structure, production, catch potential, responses to fishing and associated uncertainties in the world's marine ecosystems. PLoS ONE 10:e0133794. doi: 10.1371/journal.pone.0133794
Jennings, S., Pinnegar, J. K., Polunin, N. V., and Warr, K. J. (2001). Impacts of trawling disturbance on the trophic structure of benthic invertebrate communities. Mar. Ecol. Prog. Ser. 213, 127–142. doi: 10.3354/meps213127
Jennings, S., Pinnegar, J. K., Polunin, N. V., and Warr, K. J. (2002). Linking size-based and trophic analyses of benthic community structure. Mar. Ecol. Prog. Ser. 226, 77–85. doi: 10.3354/meps226077
Jo, H., Gim, J.-A., Jeong, K.-S., Kim, H.-S., and Joo, G.-J. (2014). Application of DNA barcoding for identification of freshwater carnivorous fish diets: Is number of prey items dependent on size class for Micropterus salmoides? Ecol. Evol. 4, 219–229. doi: 10.1002/ece3.921
Jöns, H., and Hoyler, M. (2013). Global geographies of higher education: the perspective of world university rankings. Geoforum 46, 45–59. doi: 10.1016/j.geoforum.2012.12.014
Kadoya, T., Osada, Y., and Takimoto, G. (2012). IsoWeb: a Bayesian isotope mixing model for diet analysis of the whole food web. PLoS ONE 7:e41057. doi: 10.1371/journal.pone.0041057
Katsanevakis, S., Levin, N., Coll, M., Giakoumi, S., Shkedi, D., Mackelworth, P., et al. (2015). Marine conservation challenges in an era of economic crisis and geopolitical instability: the Mediterranean Sea case. Mar. Policy 51, 31–39. doi: 10.1016/j.marpol.2014.07.013
Katsanevakis, S., Stelzenmüller, V., South, A., Sørensen, T. K., Jones, P. J. S., Kerr, S., et al. (2011). Ecosystem-based marine spatial management: review of concepts, policies, tools, and critical issues. Ocean Coast. Manage. 54, 807–820. doi: 10.1016/j.ocecoaman.2011.09.002
Katsanevakis, S., Wallentinus, I., Zenetos, A., Leppäkoski, E., Çinar, M. E., Oztürk, B., et al. (2014). Impacts of marine invasive alien species on ecosystem services and biodiversity: a pan-European review. Aquat. Invasions 9, 391–423. doi: 10.3391/ai.2014.9.4.01
King, R. A., Read, D. S., Traugott, M., and Symondson, W. O. (2008). Molecular analysis of predation: a review of best practice for DNA-based approaches. Mol. Ecol. 17, 947–963. doi: 10.1111/j.1365-294X.2007.03613.x
Kohlbach, D., Graeve, M., Lange, B. A., David, C., Peeken, I., and Flores, H. (2016). The importance of ice algae-produced carbon in the central Arctic Ocean ecosystem: Food web relationships revealed by lipid and stable isotope analyses. Limnol. Oceanogr. 61, 2027–2044. doi: 10.1002/lno.10351
Kohlbach, D., Schaafsma, F. L., Graeve, M., Lebreton, B., Lange, B. A., David, C., et al. (2017). Strong linkage of polar cod (Boreogadus saida) to sea ice algae-produced carbon: Evidence from stomach content, fatty acid and stable isotope analyses. Prog. Oceanogr. 152, 62–74. doi: 10.1016/j.pocean.2017.02.003
Kopp, D., Le Bris, H., Grimaud, L., Nérot, C., and Brind'Amour, A. (2013). Spatial analysis of the trophic interactions between two juvenile fish species and their preys along a coastal-estuarine gradient. J. Sea Res. 81, 40–48. doi: 10.1016/j.seares.2013.03.013
Kotta, J., Wernberg, T., Jänes, H., Kotta, I., Nurkse, K., Pärnoja, M., et al. (2018). Novel crab predator causes marine ecosystem regime shift. Sci. Rep. 8:4956. doi: 10.1038/s41598-018-23282-w
Koulouri, P., Dounas, C., Arvanitidis, C., Koutsoubas, D., Tselepides, A., and Eleftheriou, A. (2015). A field experiment on trophic relations within the benthic boundary layer (BBL) over an oligotrophic continental shelf. Estuar. Coast. Shelf S. 164, 392–407. doi: 10.1016/j.ecss.2015.07.029
Koussoroplis, A. M., Bec, A., Perga, M. E., Koutrakis, E., Bourdier, G., and Desvilettes, C. (2011). Fatty acid transfer in the food web of a coastal Mediterranean lagoon: evidence for high arachidonic acid retention in fish. Estuar. Coast. Shelf. S. 91, 450–461. doi: 10.1016/j.ecss.2010.11.010
Kritzer, J. P., DeLucia, M.-B., Greene, E., Shumway, C., Topolski, M. F., Thomas-Blate, J., et al. (2016). The importance of benthic habitats for coastal fisheries, BioScience 66, 274–284. doi: 10.1093/biosci/biw014
Kroeker, K. J., Micheli, F., Gambi, M. C., and Martz, T. R. (2011). Divergent ecosystem responses within a benthic marine community to ocean acidification. Proc. Natl. Acad. Sci. U.S.A. 108, 14515–14520. doi: 10.1073/pnas.1107789108
Kürten, B., Frutos, I., Struck, U., Painting, S. J., Polunin, N. V., and Middelburg, J. J. (2013). Trophodynamics and functional feeding groups of North Sea fauna: a combined stable isotope and fatty acid approach. Biogeochemistry 113, 189–212. doi: 10.1007/s10533-012-9701-8
Kvitek, R., and Bretz, C. (2005). Shorebird foraging behavior, diet, and abundance vary with harmful algal bloom toxin concentrations in invertebrate prey. Mar. Ecol. Prog. Ser. 293, 303–309. doi: 10.3354/meps293303
Lassalle, G., Lobry, J., Le Loc'h, F., Bustamante, P., Certain, G., Delmas, D., et al. (2011). Lower trophic levels and detrital biomass control the Bay of Biscay continental shelf food web: Implications for ecosystem management. Prog. Oceanogr. 91, 561–575. doi: 10.1016/j.pocean.2011.09.002
Lawler, J. J., Tear, T. H., Shaw, M. R., Gonzalez, P., Kareiva, P., et al. (2010). Resource management in a changing and uncertain climate. Front. Ecol. Environ. 8:146. doi: 10.1890/070146
Leonard, G. H., Levine, J. M., Schmidt, P. R., and Bertness, M. D. (1998). Flow-driven variation in intertidal community structure in a Maine estuary. Ecology 79, 1395–1411. doi: 10.1890/0012-9658(1998)079[1395:FDVIIC]2.0.CO;2
Leray, M., Yang, J. Y., Meyer, C. P., Mills, S. C., Agudelo, N., Ranwez, V., et al. (2013). A new versatile primer set targeting a short fragment of the mitochondrial COI region for metabarcoding metazoan diversity: application for characterizing coral reef fish gut contents. Front. Zool. 10:34. doi: 10.1186/1742-9994-10-34
Libralato, S., Christensen, V., and Pauly, D. (2006). A method for identifying keystone species in food web models. Ecol. Model. 195, 153–171. doi: 10.1016/j.ecolmodel.2005.11.029
Libralato, S., Pranovi, F., Stergiou, K. I., and Link, J. (2014). Trophodynamics in marine ecology: 70 years after Lindeman. Mar. Ecol. Prog. Ser. 512, 1–7. doi: 10.3354/meps11033
Lin, H. J., Shao, K. T., Kuo, S. R., Hsieh, H. L., Wong, S. L., Chen, I. M., et al. (1999). A trophic model of a sandy barrier lagoon at Chiku in southwestern Taiwan. Estuar. Coast. Shelf. S. 48, 575–588. doi: 10.1006/ecss.1998.0457
Lindeman, R. L. (1942). The trophic-dynamic aspect of ecology. Ecology 23, 399–417. doi: 10.2307/1930126
Lindeque, P. K., Dimond, A., Harmer, R. A., Parry, H. E., Pemberton, K. L., and Fileman, E. S. (2014). Feeding selectivity of bivalve larvae on natural plankton assemblages in the western English Channel. Mar. Biol. 162, 291–308. doi: 10.1007/s00227-014-2580-x
Link, J., Overholtz, W., O'Reilly, J., Green, J., Dow, D., Palka, D., et al. (2008). The Northeast U.S. continental shelf Energy Modeling and Analysis exercise (EMAX): ecological network model development and basic ecosystem metrics. J. Mar. Syst. 74, 453–474. doi: 10.1016/j.jmarsys.2008.03.007
Lotze, H. K., Lenihan, H. S., Bourque, B. J., Bradbury, R. H., Cooke, R. G., Kay, M. C., et al. (2006). Depletion, degradation, and recovery potential of Estuaries and Coastal Seas. Science 312, 1806–1809. doi: 10.1126/science.1128035
Lovvorn, J. R., Jacob, U., North, C. A., Kolts, J. M., Grebmeier, J. M., Cooper, L. W., et al. (2015). Modeling spatial patterns of limits to production of deposit-feeders and ectothermic predators in the northern Bering Sea. Est. Coast. Shelf S. 154, 19–29. doi: 10.1016/j.ecss.2014.12.020
Luczak, C., Beaugrand, G., Lindley, J. A., Dewarumez, J. M., Dubois, P. J., and Kirby, R. R. (2012). North Sea ecosystem change from swimming crabs to seagulls. Biol. Lett. 8, 821–824. doi: 10.1098/rsbl.2012.0474
Mancinelli, G., Carrozzo, L., Costantini, M. L., Rossi, L., Marini, G., and Pinna, M. (2013). Occurrence of the Atlantic blue crab Callinectes sapidus Rathbun, 1896 in two Mediterranean coastal habitats: temporary visitor or permanent resident? Est. Coast. Shelf S. 135, 46–56. doi: 10.1016/j.ecss.2013.06.008
Marques, A., Pereira, H. M., Krug, C., Leadley, P. W., Visconti, P., Januchowski-Hartley, S. R., et al. (2014). A framework to identify enabling and urgent actions for the 2020 Aichi Targets. Basic Appl. Ecol. 15, 633–638. doi: 10.1016/j.baae.2014.09.004
Marsh, J. M., Mueter, F. J., Iken, K., and Danielson, S. (2017). Ontogenetic, spatial and temporal variation in trophic level and diet of Chukchi Sea fishes. Deep Sea Res. Pt. II 135, 78–94. doi: 10.1016/j.dsr2.2016.07.010
Mathews, C. P. (1993). “Productivity and energy flows at all trophic levels in the River Thames, England: Mark 2,” in Trophic Models of Aquatic Ecosystems, Vol. 26, eds V. Christensen and D. Pauly (ICLARM Conference Proceedings), 390.
Mazor, T., Possingham, H. P., and Kark, S. (2013). Collaboration among countries in marine conservation can achieve substantial efficiencies. Divers. Distrib. 19, 1380–1393. doi: 10.1111/ddi.12095
McLeod, R. J., and Wing, S. R. (2009). Strong pathways for incorporation of terrestrially derived organic matter into benthic communities. Estuar. Coast. Shelf. S. 82, 645–653. doi: 10.1016/j.ecss.2009.02.025
McMeans, B. C., Arts, M. T., and Fisk, A. T. (2015). Impacts of food web structure and feeding behavior on mercury exposure in Greenland sharks (Somniosus microcephalus). Sci. Total Environ. 509–510, 216–225. doi: 10.1016/j.scitotenv.2014.01.128
McMeans, B. C., Rooney, N., Arts, M. T., and Fisk, A. T. (2013). Food web structure of a coastal Arctic marine ecosystem and implications for stability. Mar. Ecol. Prog. Ser. 482, 17–28. doi: 10.3354/meps10278
Meinesz, A., Lefevre, J. R., and Astier, J. M. (1991). Impact of coastal development on the infralittoral zone along the southeastern Mediterranean shore of continental France. Mar. Pollut. Bull. 23, 343–347. doi: 10.1016/0025-326X(91)90698-R
Mendonça, V. M., Raffaelli, D., Boyle, P., and Emes, C. (2007). The ecological role of overwintering fish in the food web of the Culbin Sands lagoon ecosystem, NE Scotland: Identifying major trophic links and testing effects of the fish Pomatoschistus microps (Pallas) on benthic invertebrates. Sci. Mar. 71, 649–660. doi: 10.3989/scimar.2007.71n4649
Menge, B. A. (1992). Community regulation: under what conditions are bottom-up factors important on rocky shores? Ecology 73, 755–765. doi: 10.2307/1940155
Menge, B. A. (1995). Indirect effects in marine intertidal interaction webs: patterns and importance. Ecol. Monogr. 65, 21–74. doi: 10.2307/2937158
Menge, B. A. (2000). Top-down and bottom-up community regulation in marine rocky intertidal habitats. J. Exp. Mar. Biol. Ecol. 250, 257–289. doi: 10.1016/S0022-0981(00)00200-8
Menge, B. A., Daley, B. A., Lubchenco, J., Sanford, E., Dahlhoff, E., Halpin, P. M., et al. (1999). Top-down and bottom-up regulation of New Zealand rocky intertidal communities. Ecol. Mongr. 69, 297–230. doi: 10.1890/0012-9615(1999)069[0297:TDABUR]2.0.CO;2
Menge, B. A., and Sutherland, J. P. (1987). Community regulation: variation in disturbance, competition, and predation in relation to environmental stress and recruitment. Am. Nat. 130, 730–757. doi: 10.1086/284741
Meunier, C. L., Liess, A., Andersson, A., Brugel, S., Paczkowska, J., Rahman, H., et al. (2017). Allochthonous carbon is a major driver of the microbial food web – A mesocosm study simulating elevated terrestrial matter runoff. Mar. Environ. Res. 129, 236–244. doi: 10.1016/j.marenvres.2017.06.008
Micheli, F., Halpern, B. S., Walbridge, S., Ciriaco, S., Ferretti, F., Fraschetti, S., et al. (2013). Cumulative human impacts on Mediterranean and Black Sea marine ecosystems: assessing current pressures and opportunities. PLoS ONE 8:e79889. doi: 10.1371/journal.pone.0079889
Michener, R. H., and Lajtha, K., (eds.). (2007). Stable Isotopes in Ecology and Environmental Science. 2nd Edn. Oxford: Blackwell. doi: 10.1002/9780470691854
Misic, C., Gaozza, L., Petrillo, M., and Harriague, A. C. (2016). The allochthonous material input in the trophodynamic system of the shelf sediments of the Gulf of Tigullio (Ligurian Sea, NW Mediterranean). Mar. Environ. Res. 120, 9–19. doi: 10.1016/j.marenvres.2016.07.001
Moher, D., Liberati, A., Tetzlaff, J., and Altman, D. G. (2010). Preferred reporting items for systematic reviews and meta-analyses: the PRISMA statement. Int. J. Surg. 8, 336–341. doi: 10.1016/j.ijsu.2010.02.007
Mumby, P. J., Steneck, R. S., Edwards, A. J., Ferrari, R., Coleman, R., Harborne, A. R., et al. (2012). Fishing down a Caribbean food web relaxes trophic cascades. Mar. Ecol. Prog. Ser. 445, 13–24. doi: 10.3354/meps09450
Myers, R., and Worm, B. (2003). Rapid worldwide depletion of predatory fish communities. Nature 423, 280–283. doi: 10.1038/nature01610
Neira, S., Moloney, C., Shannon, L. J., Christensen, V., Arancibia, H., and Jarre, A. (2014). Assessing changes in the southern Humboldt in the 20th century using food web models. Ecol. Model. 278, 52–66. doi: 10.1016/j.ecolmodel.2014.01.003
Nielsen, K. J., and Navarrete, S. A. (2004). Mesoscale regulation comes from the bottom-up: Intertidal interactions between consumers and upwelling. Ecol. Lett. 7, 31–41 doi: 10.1046/j.1461-0248.2003.00542.x
Nilsen, M., Pedersen, T., Nilssen, E. M., and Fredriksen, S. (2008). Trophic studies in a high-latitude fjord ecosystem - A comparison of stable isotope analyses (δ13C and δ15N) and trophic-level estimates from a mass-balance model. Can. J. Fish. Aquat. Sci. 65, 2791–2806. doi: 10.1139/F08-180
Nilsson, P., and Ziegler, P. (2007). Spatial distribution of fishing effort in relation to seafloor habitats in the Kattegat, a GIS analysis. Aquat. Conserv. 17, 421–440. doi: 10.1002/aqc.792
Oakley, J. W., Simons, J., and Stunz, G. W. (2014). Spatial and habitat-mediated food web dynamics in an oyster-dominated estuary. J. Shellfish Res. 33, 841–855. doi: 10.2983/035.033.0319
O'Connor, M. I., and Bruno, J. F. (2009). Predator richness has no effect in a diverse marine food web. J. Anim. Ecol. 78, 732–740. doi: 10.1111/j.1365-2656.2009.01533.x
Odum, E. P. (1985). Trends expected in stressed ecosystems. BioScience 35, 419–422. doi: 10.2307/1310021
Odum, E. P., and Odum, H. T. (1955). Trophic structure and productivity of a windward coral reef community on Eniwetok Atoll. Ecol. Monogr. 25, 291–320. doi: 10.2307/1943285
Odum, E. P., Odum, H. T., and Andrews, J. (1971). Fundamentals of Ecology. Philadelphia, PA: Saunders.
O'Gorman, E. J., Enright, R. A., and Emmerson, M. C. (2008). Predator diversity enhances secondary production and decreases the likelihood of trophic cascades. Oecologia 158, 557–567. doi: 10.1007/s00442-008-1165-0
O'Gorman, E. J., Fitch, J. E., and Crowe, T. P. (2012). Multiple anthropogenic stressors and the structural properties of food webs. Ecology 93, 441–448. doi: 10.1890/11-0982.1
O'Rorke, R., Lavery, S., and Jeffs, A. (2012). PCR enrichment techniques to identify the diet of predators. Mol. Ecol. Resour. 12, 5–17. doi: 10.1111/j.1755-0998.2011.03091.x
Pace, M. L., Cole, J. E., Carpenter, S. R., and Kitchell, J. F. (1999). Trophic cascades revealed in diverse ecosystems. Trends Ecol. Evol. 14, 483–488. doi: 10.1016/S0169-5347(99)01723-1
Paine, R. T. (1966). Food web complexity and species diversity. Am. Nat. 100, 65–75. doi: 10.1086/282400
Paine, R. T. (1974). Intertidal community structure: experimental studies on the relationship between a dominant competitor and its principal predator. Oecologia 15, 93–120. doi: 10.1007/BF00345739
Paine, R. T. (1980). Food webs: linkage, interaction strength, and community infrastructure. J. Anim. Ecol. 49, 667–685. doi: 10.2307/4220
Paine, R. T. (1988). Habitat suitability and local population persistence of the sea palm Postelsia palmaeformis. Ecology 69, 1787–1794. doi: 10.2307/1941157
Paiva, V. H., Geraldes, P., Marques, V., Rodríguez, R., Garthe, S., and Ramos, J. A. (2013). Effects of environmental variability on different trophic levels of the North Atlantic food web. Mar. Ecol. Prog. Ser. 477, 15–28. doi: 10.3354/meps10180
Pálsson, O. K., Gislason, A., Guð*finnsson, H. G., Gunnarsson, B., Olafsdóttir, S. R., Petursdottir, H., et al. (2012). Ecosystem structure in the Iceland Sea and recent changes to the capelin (Mallotus villosus) population. ICES J. Mar. Sci. 69, 1242–1254. doi: 10.1093/icesjms/fss071
Parnell, A., Inger, R., Bearhop, S., and Jackson, A. L. (2008). SIAR: Stable Isotope Analysis in R. Available online at: http://cran.r-project.org/web/packages/siar/index.html. (accessed March 20 2020).
Parnell, A. C., Inger, R., Bearhop, S., and Jackson, A. L. (2010). Source partitioning using stable isotopes: coping with too much variation. PLoS ONE 5:e9672. doi: 10.1371/journal.pone.0009672
Pauly, D., and Christensen, V. (1995). Primary production required to sustain global fisheries. Nature 374, 255–257. doi: 10.1038/374255a0
Pauly, D., Christensen, V., Dalsgaard, J., Froese, R., and Torres, F. J. (1998). Fishing down marine food webs. Science 279, 860–863. doi: 10.1126/science.279.5352.860
Peters, D. S., and Schaaf, W. E. (1991). Empirical model of the trophic basis for fishery yield in coastal waters of the Eastern USA. Trans. Am. Fish. Soc. 120, 459–473.
Petersen, C. G. J. (1915). A preliminary result of the investigations on the valuation of the sea. Report of the Danish Biological Station
Phillips, D. L., and Gregg, J. W. (2003). Source partitioning using stable isotopes: coping with too many sources. Oecologia, 136, 2361–2269. doi: 10.1007/s00442-003-1218-3
Pierce, W. D., Cushman, R. A., and Hood, C. E. (1912). The insect enemies of the cotton boll weevil. U.S. Dep. Agric. Bureau Entomol. Bull. 100:941. doi: 10.5962/bhl.title.64941
Pimm, S. L. (1984). The complexity and stability of ecosystems. Nature 307, 321–326. doi: 10.1038/307321a0
Pimm, S. L., Lawton, J. H., and Cohen, J. E. (1991). Food web patterns and their consequences. Nature 350, 669–674. doi: 10.1038/350669a0
Pinnegar, J. K., and Polunin, N. V. C. (1999). Differential fractionation of δ13C and δ15N among fish tissues: implications for the study of trophic interactions. Funct. Ecol. 13, 225–231. doi: 10.1046/j.1365-2435.1999.00301.x
Plagányi, É. E. (2007). Models for an Ecosystem Approach to Fisheries. Rome: FAO Fisheries Technical Paper.
Plagányi, É. E., and Butterworth, D. S. (2004). A critical look at the potential of Ecopath with Ecosim to assist in practical fisheries management. Afr. J. Mar. Sci. 26, 261–288. doi: 10.2989/18142320409504061
Platt, H. M., and Warwick, R. M. (1980). “The significance of free-living nematodes to the littoral ecosystem,” in The Shore Environment. Volume 2. Ecosystems, eds J. H. Price, D. E. G. Irvine, and W. F. Farnham (Cambridge: Academic Press), 729–759.
Polis, G. A., Anderson, W. B., and Holt, R. D. (1997). Toward an integration of landscape ecology and food web ecology: the dynamics of spatially subsidized food webs. Annu. Rev. Ecol. Syst. 28, 289–316. doi: 10.1146/annurev.ecolsys.28.1.289
Polis, G. A., Sears, A. L. W., Huxel, G. R., Strong, D. R., and Maron, J. (2000). When is a trophic cascade a trophic cascade? Trends Ecol. Evol. 15, 473–475. doi: 10.1016/S0169-5347(00)01971-6
Polovina, J. J., and Ow, M. D. (1983). ECOPATH: A User's Manual and Program Listings. Honolulu: NOAA, National Marine Fisheries Service, Honolulu Admin Rep 23.
Pomeroy, L. R. (1974). The ocean's food web: a changing paradigm. Bioscience 24, 499–504. doi: 10.2307/1296885
Pompanon, F., Deagle, B. E., Symondson, W. O. C., Brown, D. S., Jarman, S. N., and Taberlet, P. (2012). Who is eating what: diet assessment using next generation sequencing. Mol. Ecol. 21, 1931–1950, doi: 10.1111/j.1365-294X.2011.05403.x
Post, D. M. (2002). Using stable isotopes to estimate trophic position; models, methods, and assumptions. Ecology 83, 703–718. doi: 10.1890/0012-9658(2002)083[0703:USITET]2.0.CO;2
Prado, P., Vergara, C., Caiola, N., and Ibáñez, C. (2014). Influence of salinity regime on the food-web structure and feeding ecology of fish species from Mediterranean coastal lagoons. Est. Coast. Shelf S. 139, 1–10. doi: 10.1016/j.ecss.2013.12.013
Quillien, N., Nordström, M. C., Schaal, G., Bonsdorff, E., and Grall, J. (2016). Opportunistic basal resource simplifies food web structure and functioning of a highly dynamic marine environment. J. Exp. Mar. Biol. Ecol. 477, 92–102. doi: 10.1016/j.jembe.2016.01.010
Rice, J. (1995). Food web theory, marine food webs, and what climate change may do to northern fish populations in climate change and northern fish populations. Can. Spe. Publicat. Fisher. Aquatic Sci. 121, 561–568.
Rilov, G. (2016). Multi-species collapses at the warm edge of a warming sea. Sci. Rep. 6:36897. doi: 10.1038/srep36897
Robinson, L., Greenstreet, S., Reiss, H., Callaway, R., Craeymeersch, J., De, B.oois, et al. (2010). Length-weight relationships of 216 North Sea benthic invertebrates and fish. J. Mar. Biol. Assoc. 90, 95–104. doi: 10.1017/S0025315409991408
Rocha, J., Yletyinen, J., Biggs, R., Blenckner, T., and Peterson, G. (2015). Marine regime shifts: drivers and impacts on ecosystems services. Philos. Trans. R. Soc. B 370:0273. doi: 10.1098/rstb.2013.0273
Rodríguez-Graña, L., Calliari, D., Conde, D., Sellanes, J., and Urrutia, R. (2008). Food web of a SW Atlantic shallow coastal lagoon: spatial environmental variability does not impose substantial changes in the trophic structure. Mar. Ecol. Prog. Ser. 362, 69–83. doi: 10.3354/meps07401
Roy, V., Iken, K., Gosselin, M., Tremblay, J.-E., Bélanger, S., and Archambault, P. (2015). Benthic faunal assimilation pathways and depth-related changes in food-web structure across the Canadian Arctic. Deep-Sea Res. Pt. I 102, 55–71. doi: 10.1016/j.dsr.2015.04.009
Saila, S. B., Kocic, V. L., and McManus, J. W. (1993). Modelling the effects of destructive fishing practices on tropical coral reefs. Mar. Ecol. Prog. Ser. 94, 51–60. doi: 10.3354/meps094051
Sala, E., Ballesteros, E., Dendrinos, P., Di Franco, A., Ferretti, F., Foley, D., et al. (2012). The structure of Mediterranean rocky reef ecosystems across environmental and human gradients, and conservation implications. PLoS ONE 7:e32742. doi: 10.1371/journal.pone.0032742
Salomidi, M., Giakoumi, S., Gerakaris, V., Issaris, Y., Sini, M., and Tsiamis, K. (2016). Setting an ecological baseline prior to the bottom-up establishment of a marine protected area in Santorini Island, Aegean Sea. Medit. Mar. Sci. 17, 720–737. doi: 10.12681/mms.1802
Savenkoff, C., Swain, D. P., Hanson, J. M., Castonguay, M., Hammill, M. O., Bourdages, H., et al. (2007). Effects of fishing and predation in a heavily exploited ecosystem: comparing periods before and after the collapse of groundfish in the southern Gulf of St. Lawrence (Canada). Ecol. Model. 204, 115–128. doi: 10.1016/j.ecolmodel.2006.12.029
SCBD (2004). The Ecosystem Approach, (CBD Guidelines) Montreal: Secretariat of the Convention on Biological Diversity, 50.
Schmidt, A. L., Coll, M., Romanuk, T. N., and Lotze, H. K. (2011). Ecosystem structure and services in eelgrass Zostera marina and rockweed Ascophyllum nodosum habitats. Mar. Ecol. Prog. Ser. 437, 51–68. doi: 10.3354/meps09276
Schoeninger, M. J., and DeNiro, M. J. (1984). Nitrogen and carbon isotopic composition of bone collagen from marine and terrestrial animals, Geochim. Cosmochim. Ac. 48, 625–639. doi: 10.1016/0016-7037(84)90091-7
Schoenrock, K. M., Vad, J., Muth, A., Pearce, D. M., Rea, B. R., Schofield, J. E., et al. (2018). Biodiversity of kelp forests and coralline algae habitats in southwestern Greenland. Diversity 10:117. doi: 10.3390/d10040117
Shannon, L., Coll, M., Bundy, A., Gascuel, D., Heymans, J. J., Kleisner, K., et al. (2014). Trophic level-based indicators to track fishing impacts across marine ecosystems. Mar. Ecol. Prog. Ser. 512, 115–140. doi: 10.3354/meps10821
Shears, N. T., Babcock, R. C., and Salomon, A. K. (2008). Context-dependent effects of fishing: variation in trophic cascades across environmental gradients. Ecol. Appl. 18, 1860–1873. doi: 10.1890/07-1776.1
Shelford, V. E. (1913). Animal Communities in Temperate America as Illustrated in the Chicago Region. University of Chicago Press, Chicago, Illinois, USA. Reprint 1937 with bibliographic addenda. Reprint 1977. New York, NY: Arno Press. doi: 10.5962/bhl.title.7231
Sini, M., Vatikiotis, K., Thanopoulou, Z., Katsoupis, C., Maina, I., Kavadas, S., et al. (2019). Small-scale coastal fishing shapes the structure of shallow rocky reef fish in the aegean sea. Front. Mar. Sci. 6:599. doi: 10.3389/fmars.2019.00599
Søreide, J. E., Falk-Petersen, S., Hegseth, E. N., Hop, H., Carroll, M. L., Hobson, K. A., et al. (2008). Seasonal feeding strategies of Calanus in the high-Arctic Svalbard region. Deep Sea Res. Pt. II 55, 2225–2244. doi: 10.1016/j.dsr2.2008.05.024
Søreide, J. E., Hop, H., Carroll, M. C., Falk-Petersen, S., and Hegseth, E. N. (2006). Seasonal food web structures and sympagic-pelagic coupling in the European Arctic revealed by stable isotopes and a two-source food web model, Prog. Oceanogr. 71, 59–87. doi: 10.1016/j.pocean.2006.06.001
Soto, L. A., and Escobar-Briones, E. (1995). Coupling mechanisms related to benthic production in the SWGulf of Mexico. In:Biology and Ecology of Shallow Coastal Waters: Proceedings of the 28th European Marine Biology Symposium Iraklio: Olsen & Olsen. 233–42
Sousa, L., Xavier, R., Costa, V., Humphries, N. E., Trueman, C., Rosa, R., et al. (2016). DNA barcoding identifies a cosmopolitan diet in the ocean sunfish. Sci. Rep. 6:28762. doi: 10.1038/srep28762
Spalding, M. D., Fox, H. E., Allen, G. R., Davidson, N., Ferdana, Z. A., Finlayson, M., et al. (2007). Marine ecoregions of the world: a bioregionalization of coastal and shelf areas. Bioscience 57, 573–583. doi: 10.1641/B570707
Stauffer, B. A., Miksis-Olds, J., and Goes, J. I. (2015). Cold regime interannual variability of primary and secondary producer community composition in the Southeastern Bering Sea. PLoS ONE 10:e0131246. doi: 10.1371/journal.pone.0131246
Steffen, W., Broadgate, W., Deutsch, L., Gaffney, O., and Ludwig, C. (2015). The trajectory of the Anthropocene: The great acceleration. Anthropocene Rev. 2, 81–98. doi: 10.1177/2053019614564785
Stelzenmüller, V., Coll, M., Cormier, R., Mazaris, A. D., Pascual, M., Loiseau, C., et al. (2020). Operationalizing risk-based cumulative effect assessments in the marine environment. Sci. Total Environ. 724:138118. doi: 10.1016/j.scitotenv.2020.138118
Stelzenmüller, V., Coll, M., Mazaris, A. D., Giakoumi, S., Katsanevakis, S., Portman, M., et al. (2018). A risk-based approach to cumulative effect assessments for marine management. Sci. Total Environ. 612, 1132–1140. doi: 10.1016/j.scitotenv.2017.08.289
Stock, B. C., and Semmens, B. X. (2013). MixSIAR GUI User Manual, Version 1.0. Available online at: https://github.com/brianstock/MixSIAR/releases.
Summerhayes, V., and Elton, C. (1923). Contributions to the ecology of Spitsbergen and Bear Island. J. Ecol. 11, 214–216. doi: 10.2307/2255863
Tallis, H. (2009). Kelp and rivers subsidize rocky intertidal communities in the Pacific Northwest (USA). Mar. Ecol. Prog. Ser. 389, 85–96. doi: 10.3354/meps08138
Tamelander, T., Reigstad, M., Hop, H., Carroll, M. L., and Wassmann, P. (2008). Pelagic and sympagic contribution of organic matter to zooplankton and vertical export in the Barents Sea marginal ice zone. Deep-Sea Res. Pt. II 55, 2330–2339. doi: 10.1016/j.dsr2.2008.05.019
Tewfik, A., Rasmussen, J. B., and McCann, K. S. (2005). Anthropogenic enrichment alters a marine benthic food web. Ecology 86, 2726–2736. doi: 10.1890/04-1537
Thibaut, T., Blanfun,é, A., Boudouresque, C. F., Personnic, S., Ruitton, S., Ballesteros, E., et al. (2017). An ecosystem-based approach to assess the status of Mediterranean algae-dominated shallow rocky reefs. Mar. Pollut. Bull. 117, 311–329. doi: 10.1016/j.marpolbul.2017.01.029
Thibaut, T., Blanfune, A., Boudouresque, C., and Verlaque, M. (2014). Decline and local extinction of Fucales in French Riviera: the harbinger of future extinctions? Medit. Mar. Sci. 16, 206–224. doi: 10.12681/mms.1032
Thollot, P., Kulbicki, M., and Harmelin-Vivien, M. (1999). Trophic analysis and food webs of mangrove fish assemblages from New Caledonia. CR. Acad. Sci. III-Vie 7, 607–619. doi: 10.1016/S0764-4469(00)88531-5
Tornero, V., and Hanke, G. (2016). Chemical contaminants entering the marine environment from sea-based sources: a review with a focus on European seas. Mar. Pollut. Bull. 112, 17–38. doi: 10.1016/j.marpolbul.2016.06.091
Torruco, D., Chávez, E. A., and González, A. (2007). Spatio-temporal variation of the structural organization of demersal communities in the southwestern Gulf of Mexico. Rev. Biol. Trop. 55, 509–536. doi: 10.15517/rbt.v55i2.6028
Tselepides, A., Papadopoulou, K. N., Podaras, D., Plaiti, W., and Koutsoubas, D. (2000). Macrobenthic community structure over the continental margin of Crete (South Aegean Sea, NE Mediterranean). Prog. Oceanogr. 46, 401–428. doi: 10.1016/S0079-6611(00)00027-6
Tu, K. L., Blanchard, A. L., Iken, K., and Horstmann-Dehn, L. (2015). Small-scale spatial variability in benthic food webs in the northeastern Chukchi Sea. Mar. Ecol. Prog. Ser. 528, 19–37. doi: 10.3354/meps11216
Ulanowicz, R. E. (1980). A hypothesis on the development of natural communities. J. Theor. Biol. 85, 223–245. doi: 10.1016/0022-5193(80)90019-3
Ulanowicz, R. E. (1986). Growth and Development: Ecosystems Phenomenology. New York, NY: Springer. doi: 10.1007/978-1-4612-4916-0
Ullah, H., Nagelkerken, I., Goldenberg, S. U., and Fordham, D. A. (2018). Climate change could drive marine food web collapse through altered trophic flows and cyanobacterial proliferation. PLoS Biol. 16:e2003446. doi: 10.1371/journal.pbio.2003446
UNESCO Institute for Statistics (2020). How Much Does Your Country Invest in RandD? Available online at: http://uis.unesco.org/apps/visualisations/research-and-development-spending/ (accessed February 28 2020).
Valentini, A., Pompanon, F., and Taberlet, P. (2009). DNA barcoding for ecologists. Trends Ecol. Evol. 24, 110–117, doi: 10.1016/j.tree.2008.09.011
Valiela, I. (2015). Marine Ecological Processes. 3rd Edn. New York, NY: Springer Science and Business Media LLC. doi: 10.1007/978-0-387-79070-1
Vander Zanden, M. J., and Rasmussen, J. B. (1999). Primary consumer δ13C and δ15N and the trophic position of aquatic consumers. Ecology 80, 1395–1404. doi: 10.1890/0012-9658(1999)080[1395:PCCANA]2.0.CO;2
Waycott, M., Duarte, C. M., Carruthers, T. J., Orth, R. J., Dennison, W. C., Olyarnik, S., et al. (2009). Accelerating loss of seagrasses across the globe threatens coastal ecosystems. Proc. Natl. Acad. of Sci. U.S.A. 106, 12377–12381. doi: 10.1073/pnas.0905620106
Wernberg, T., Bennett, S., Babcock, R. C., Bettignies, T. D., Cure, K., Depczynski, M., et al. (2016). Climate driven regime shift of a temperate marine ecosystem. Science 353, 169–172. doi: 10.1126/science.aad8745
Whalen, M. A., Duffy, J. E., and Grace, J. B. (2013). Temporal shifts in top-down vs. bottom-up control of epiphytic algae in a seagrass ecosystem. Ecology 94, 510–520. doi: 10.1890/12-0156.1
Whitehouse, G. A., Buckley, T. W., and Danielson, S. L. (2017). Diet compositions and trophic guild structure of the eastern Chukchi Sea demersal fish community. Deep-Sea Res. Pt. II 135, 95–110. doi: 10.1016/j.dsr2.2016.03.010
Wing, S. R., Leichter, J. J., Wing, L. C., Stokes, D., Genovese, S. J., McMullin, R. M., et al. (2018). Contribution of sea ice microbial production to Antarctic benthic communities is driven by sea ice dynamics and composition of functional guilds. Glob. Change Biol. 24, 3642–3653. doi: 10.1111/gcb.14291
Wing, S. R., Wing, L. C., Shatova, O. A., and Van Hale, R. (2017). Marine micronutrient vectors: Seabirds, marine mammals and fishes egest high concentrations of bioactive metals in the subantarctic island ecosystem. Mar. Ecol. Prog. Ser. 563, 13–23. doi: 10.3354/meps11978
Wollrab, S., Diehl, S., and De Roos, A. M. (2012). Simple rules describe bottom-up and top-down control in food webs with alternative energy pathways. Ecol. Lett. 15, 935–946. doi: 10.1111/j.1461-0248.2012.01823.x
Xue, X., Xue, J., Liu, W., Adams, D. H., and Kannan, K. (2017). Trophic magnification of parabens and their metabolites in a subtropical marine food web. Envir. Sci. Tech. 51, 780–789. doi: 10.1021/acs.est.6b05501
Yaragina, N., and Dolgov, A. (2009). Ecosystem structure and resilience—A comparison between the Norwegian and the Barents Sea. Deep-Sea Res. Pt. II 56, 2141–2153. doi: 10.1016/j.dsr2.2008.11.025
Young, D. R., Mearns, A. J., Schafer, H. A., Hershelman, C. P., and Gossett, R. W. (1982). “Pollutant flow through the marine food web,” in Final report on Grant PFR-7715376 to the National Science Foundation, Southern California Coastal Water Research Project Authority.
Keywords: food webs, ecosystem approach, methodological approaches, data collection, trophic level, modeling, human pressures, ecosystem-based management
Citation: Kytinou E, Sini M, Issaris Y and Katsanevakis S (2020) Global Systematic Review of Methodological Approaches to Analyze Coastal Shelf Food Webs. Front. Mar. Sci. 7:636. doi: 10.3389/fmars.2020.00636
Received: 30 March 2020; Accepted: 13 July 2020;
Published: 31 August 2020.
Edited by:
Angel Borja, Technological Center Expert in Marine and Food Innovation (AZTI), SpainReviewed by:
Chris Harvey, National Oceanic and Atmospheric Administration (NOAA), United StatesMauro Sinopoli, University of Naples Federico II, Italy
Copyright © 2020 Kytinou, Sini, Issaris and Katsanevakis. This is an open-access article distributed under the terms of the Creative Commons Attribution License (CC BY). The use, distribution or reproduction in other forums is permitted, provided the original author(s) and the copyright owner(s) are credited and that the original publication in this journal is cited, in accordance with accepted academic practice. No use, distribution or reproduction is permitted which does not comply with these terms.
*Correspondence: Eleni Kytinou, ZWt5dGlub3VAZ21haWwuY29t