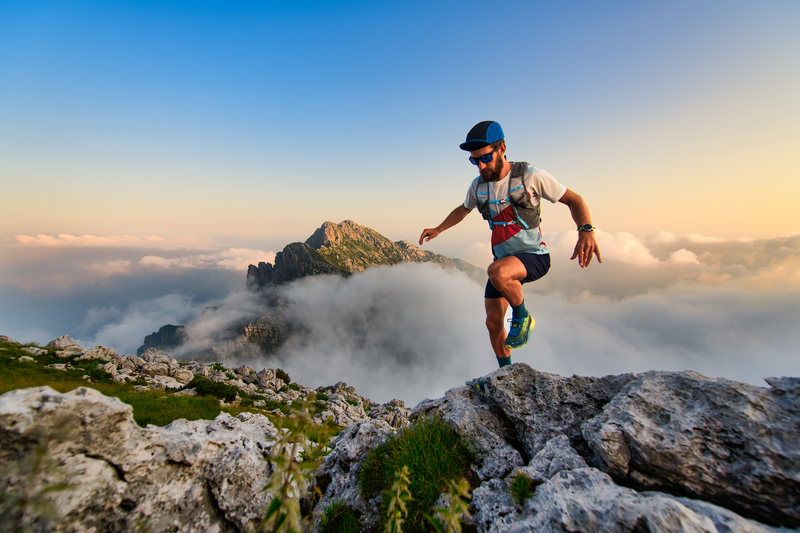
94% of researchers rate our articles as excellent or good
Learn more about the work of our research integrity team to safeguard the quality of each article we publish.
Find out more
ORIGINAL RESEARCH article
Front. Mar. Sci. , 08 July 2020
Sec. Deep-Sea Environments and Ecology
Volume 7 - 2020 | https://doi.org/10.3389/fmars.2020.00552
This article is part of the Research Topic Deep Pelagic Ecosystem Dynamics in a Highly Impacted Water Column: The Gulf of Mexico After Deepwater Horizon View all 14 articles
Oceanic diel vertical migration (DVM) constitutes the daily movement of various mesopelagic organisms migrating vertically from depth to feed in shallower waters and return to deeper water during the day. Accurate classification of taxa that participate in DVM remains non-trivial, and there can be discrepancies between methods. DEEPEND consortium (www.deependconsortium.org) scientists have been characterizing the diversity and trophic structure of pelagic communities in the northern Gulf of Mexico (nGoM). Profiling has included scientific echosounders to provide accurate and quantitative estimates of organismal density and timing as well as quantitative net sampling of micronekton. The use of environmental DNA (eDNA) can detect uncultured microbial taxa and the remnants that larger organisms leave behind in the environment. eDNA offers the potential to increase understanding of the DVM and the organisms that participate. Here we used real-time shipboard echosounder data to direct the sampling of eDNA in seawater at various time-points during the ascending and descending DVM. This approach allowed the observation of shifts in eDNA profiles concurrent with the movement of organisms in the DVM as measured by acoustic sensors. Seawater eDNA was sequenced using a high-throughput metabarcoding approach. Additionally, fine-scale acoustic data using an autonomous multifrequency echosounder was collected simultaneously with the eDNA samples and changes in organism density in the water column were compared with changes in eDNA profiles. Our results show distinct shifts in eukaryotic taxa such as copepods, cnidarians, and tunicates, over short timeframes during the DVM. These shifts in eDNA track changes in the depth of sound scattering layers (SSLs) of organisms and the density of organisms around the CTD during eDNA sampling. Dominant taxa in eDNA samples were mostly smaller organisms that may be below the size limit for acoustic detection, while taxa such as teleost fish were much less abundant in eDNA data compared to acoustic data. Overall, these data suggest that eDNA, may be a powerful new tool for understanding the dynamics and composition of the DVM, yet challenges remain to reconcile differences among sampling methodologies.
Diel vertical migration (DVM) is a well-recognized phenomenon among the world’s oceans where large numbers of fish, decapods, cephalopods, and many other species migrate vertically through the water column during crepuscular periods (Hays, 2003; Sutton et al., 2017). Generally, the animals participating in this migration will transit from deep ocean habitats (500–1000 meters) up into the epipelagic depths (0–200 meters) at night and return to the depths at dawn. Many micronekton (actively swimming organisms ∼2–20 cm length; Kloser et al., 2009) perform this daily migration in search of prey, but these migrating organisms are also important food resources for larger predators in the pelagic ecosystem. This striking vertical movement of organisms across different pelagic depths provides opportunities to study unique ecological interactions in the pelagic environment including predator-prey interactions and energy transfer between the surface and deep ocean.
Often, the DVM process is measured using echosounders to detect sound scattering layers (SSLs) in the water column. The SSLs are ubiquitous features throughout the world’s oceans and are mainly comprised of vertically migrating taxa that serve as primary prey resources for larger predators, like marine mammals (Proud et al., 2017, 2019). The SSLs are dominant biological features of these vast ecosystems and contribute importantly to the global process of carbon transport and sequestration (Irigoien et al., 2014). The depths at which SSLs occur are dynamic and often dependent on the geographic location, depth of the water column and time of day, which gives rise to well-recognized and remarkable DVM patterns (Klevjer et al., 2016).
While acoustic techniques can provide information about the dynamics of the DVM, they have a limited ability to discern the specific composition of migrating layers of organism. This gap in knowledge can be filled with towed net sampling, though a single net sampling method often cannot adequately capture all types of pelagic organisms (Milligan et al., 2018). For example, gelatinous zooplankton are not well represented in MOCHNESS sampling gear and thus may be underestimated with net sampling. One promising potential solution is the use of environmental DNA (eDNA) to detect the organisms present in the environment (Thomsen and Willerslev, 2015; Ruppert et al., 2019). eDNA consists of genetic material sampled from an environmental source rather than directly from a biological source (Thomsen and Willerslev, 2015). This sampling technique can capture all the cells that are present in the environment, which may allow for the detection and identification of larger organisms through shed biological trace remnants (cells, feces, mucus etc.). This technique when coupled with next-generation metabarcoding sequencing techniques can provide a census of the organisms present in the environment (Bucklin et al., 2016). To date, eDNA has been leveraged to profile a wide variety of environments including freshwater lakes and streams, terrestrial soils, and marine seawater and sediment (e.g., Bista et al., 2017; Cowart et al., 2018). Studies that have utilized eDNA methods in the marine environment have shown the ability to detect a variety of multicellular vertebrate and invertebrate taxa (Foote et al., 2012; Thomsen and Willerslev, 2015; Cowart et al., 2018), and complement more traditional faunal survey techniques.
In the current study, we sampled eDNA during the DVM in the northern Gulf of Mexico (nGoM) to assess whether this technique captures the dynamics of this daily migration and the composition of SSLs. Sampling of eDNA was directed by shipboard acoustic sensors to sample seawater at different time-points during the DVM, and eDNA data was compared to in-situ and shipboard acoustic data to assess whether changes in eDNA communities were related to changes observed by the acoustic sensors.
Sampling of environmental DNA (eDNA) was directed by real-time multifrequency acoustic data to identify the dominant SSLs and estimate the depth of the vertically migrating organisms (micronekton). All observations described in this study were collected from a drifting research vessel (R/V Pt. Sur) during one DEEPEND cruise (DP05) where a ship-board multifrequency echosounder system characterized the depth profiles of the SSLs and directed in-situ sampling of CTD rosette outfitted with water samplers and an acoustic probe. For the cast at night, CTD 83 (Sea-Bird 911plus), was deployed within the mesopelagic zone just above the rising SSL (Table 1). It was held in that position while the SSL rose and migrated past the CTD. Seawater samples were collected throughout the DVM process. During the morning CTD cast, the SSL migrated from the surface to deeper, so CTD 84 was placed below the SSL and then held in position while the SSL moved to the same depth and then below the CTD. Water samples were taken at each time-point, similar to night casts. We also sampled in the epipelagic zone (∼90 m), but these samples were not taken during the DVM. The sampling scheme is detailed in Table 1.
Table 1. Seawater collection metadata for DEEPEND cruise DP05 which had CTD casts (nos. 83 and 84) representing collection times for eDNA.
Multifrequency acoustic backscatter data was collected with a calibrated pole-mounted echosounder system (Simrad EK60 and EK80). The transducers were mounted in an enclosed housing and suspended 2.5 m below the water surface (see Boswell et al., 2020 for additional details). Backscatter data were collected simultaneously at four frequencies (18, 38, 70, and 120 kHz). The 18, 38, and 70 kHz echosounders had sufficient power and signal to noise to permit examination of the migrating layers, however, effects of attenuation limited data quality beyond 350 m for the 120 kHz. The dominant acoustic features detected from the shipboard echosounder were examined in more detail with an autonomous echosounder (Simrad EK80 WBAT) mounted onto the bottom of the CTD rosette frame and deployed as an acoustic probe (Kubilius et al., 2015; Kloser et al., 2016). The WBAT was configured with a 70 kHz and 120 kHz transducer and was programmed to alternate in 100 ping sequences between the two transducers. The hardware limitations of the echosounder prevented simultaneous collection at both frequencies. The on-axis gain was measured by suspending a 38.1 mm tungsten carbide sphere (∼6% cobalt binder) 7 m below the CTD while probing through the water column. Calibration was performed using the Simrad Lobe program within the EK80 software (Demer et al., 2015). Sound speed profiles and absorption coefficient were computed from bin-averaged CTD data using the Ocean Toolbox (McDougall and Barker, 2011).
Raw acoustic backscatter data collected from the shipboard echosounder were imported and scrutinized in Echoview (v9) and processed following methods described by D’Elia et al. (2016) and Boswell et al. (2020). Briefly, data from the transducer face to 15 m depth were excluded from the analysis to account for nearfield effects and to eliminate surface-associated interference (e.g., bubble sweep down). Data were examined for interference from other shipboard sonar systems (intermittent or spike noise), false bottom, and background noise. False bottoms were manually excluded. Background noise was identified and removed following a modified process described by De Robertis and Higginbottom (2007). The measurements of Nautical Area Scattering Coefficient (NASC; m2 nmi–2) were derived from the echo integral in 500-m along-track x 5-m vertical bins (MacLennan et al., 2002). NASC is generally understood to be proportional to the abundance of biological scatterers and serves as a comparable index of organism biomass (Hazen et al., 2009; Zwolinski et al., 2010; Fennell and Rose, 2015). Integrated backscatter was further binned into three depth intervals: 15–200 m (epipelagic), 200–600 m (upper mesopelagic) and 600–1000 m (lower mesopelagic). We then compared the maximum NASC (m2 nmi–2) values with depth as a function of time from the 18 and 38 kHz transducer by applying a dB- difference (ΔdB18–38 = dB18–dB38) operation to highlight two categories of scatterers within the scattering layer: (1) swim bladdered fish (SBF) and (2) non-swim bladdered fish/crustaceans (NSBFC) following D’Elia et al. (2016). Swim bladdered fish targets were classified as having a ΔdB18–38 range between +3 and +12 dB while the non-swim bladdered fish/crustaceans were classified as having a ΔdB18–38 range between -14 and 0 dB. Backscatter from these two classes were integrated into 20 m (vertical) by 3 min (horizontal) cells and exported from Echoview for additional analysis.
Acoustic backscatter data from the WBAT were examined in Echoview. Given that the emphasis was to enumerate the individual scatterers within the migrating layer, we focused the analysis on the detected point targets using a single target detection algorithm in Echoview. Sequential targets which satisfied the criteria for the algorithm were tracked and identified as an individual if they had more than 3 targets across 5 consecutive pings. For point target recognition, we used a minimum uncompensated TS threshold of −75 dB and an echo length ranging from a minimum 0.7 ms to a maximum 1.50 ms. The maximum gain compensation was set to 6.0 dB with a maximum phase deviation of 0.6 degrees for both major and minor axes. Point targets within 2 to 75 m of the WBAT were included for use in analysis. During the deployment, the WBAT remained stationary at two depths during the migration of the scattering layer: ∼92 and 320 m. The acoustic data were divided into two periods and were related to the depth of the CTD during the profiling activity. The first period occurred between 16:45 and 20:00 and targeted single targets at ∼320 m depth. Data collected during this period were divided into 10-min time intervals for processing. The second period occurred between 20:00 and 20:24 and corresponded to when the CTD was positioned at ∼90 m. Data collected during this period were divided into 3-min time intervals to capture single targets at higher temporal resolution. Target strength (dB re 1 m2) distributions were derived from each interval and analyzed to examine the temporal changes in the size of detected point targets (Lurton, 2002). We conducted a Kruskal-Wallis Chi-squared test to test for significant changes among depths.
Seawater samples were collected according to the position of the CTD relative to the SSLs and at specific times using the 12 L Niskin bottle array also mounted on the CTD (Table 1). Seawater was collected to sample the eDNA for potential changes in composition of prokaryotic and eukaryotic organisms. Collections and filtering have been described by Easson and Lopez (2019). Immediately after the CTD arrived back on the surface, replicate two-liter samples (N = 3) were taken from each Niskin bottle and were filtered through sterile 0.45 υm filter membranes to remove all cells from the seawater. No organisms were directly sampled, and filters were visually inspected to ensure no organisms were visible on the filter. Filter membranes were placed in sterile tubes and frozen until analysis at NSU.
DNA was extracted using the PowerLyzer PowerSoil DNA Isolation Kit (QIAGEN) following the manufacturer’s protocol. Polymerase chain reaction (PCR) was performed following the 18S Illumina Amplicon protocol of the Earth Microbiome Project1. Sample preparation and sequencing followed the methods outlined in Easson and Lopez (2019), except that a 300 cycle Illumina MiSeq kit was used to generate paired-end 150 bp amplicons.
Bioinformatics processing was conducted in R using the DADA2 pipeline (Callahan et al., 2016; R Core Team, 2018). Initially, sequences were trimmed to remove ambiguous bases (max N = 0), sequences longer than 150 bp and shorter than 90 bp. The default ASV2 parametric error model was used to calculate sequence error rates. Next, sequences were dereplicated to infer sequence variants, paired-end reads were combined, and chimeras were removed. Once these processes finished, a sequence table of amplicon sequence variants (ASVs) was constructed. ASVs were taxonomically classified using the Silva database (release 128; Quast et al., 2015).
Statistical analysis was done in R (R Core Team, 2018) using the R packages vegan and picante (Kembel et al., 2010; Oksanen et al., 2017). Analysis was conducted separately on CTD 83 (ascending DVM at night) and CTD 84 (descending DVM descending DVM during day). ASV abundance was transformed to relative abundance so each ASV is represented as a proportion in the whole dataset for a specific sample. Initial analysis assessed eDNA community diversity (Inverse Simpson’s index) and richness (total number of unique ASVs) and compared these metrics across sampling time-points in each CTD. Beta diversity was then assessed by calculating Bray-Curtis dissimilarity among samples and a permuted multivariate analysis of variance (PERMANOVA) was used to compare beta diversity among sampling time points in each CTD.
During the day, the depth of the maximum backscatter (NASC, m2 nmi–2) occurred at a depth of ∼440 m (NASC = 58.86 m2 nmi–2) (Figure 5). The scattering layer began migrating upwards at ∼17:30 and completed the migration at 20:23, with a large amount of backscatter filling the upper 150 m of the water column (CTD 83). The downward migration began around 04:00 with the descent completing at ∼08:30 where the scattering layer settled at ∼460 m (NASC = 55.33 m2 nmi–2) (CTD 84).
Two distinct scattering layers were identified which contributed significantly to the measured water column backscatter. When the CTD was in the upper mesopelagic zone (16:45 to 20:00), the center of mass of the SBF was ∼325 m (± 97.9 m). In contrast, the center of mass for NSBFC were located an average of 407 m (± 114.3 m). When the CTD was raised to the epipelagic zone (20:00 to 20:25), the center of mass of SBF was 155 m (± 43.6 m), while NSBFC were at ∼292 m (± 217 m). Variability in the depth of the NASC maxima between SBF and NSBFC was consistent during both depth profiles.
We observed significant variability when examining the temporal patterns of target strength distributions collected at the two depths from the probe positioned within the ascending DVM. Additionally, the target strength distributions varied significantly in the distribution of scatterers within the mesopelagic layer at 320 m (p < 0.001; χ2 = 830.8, df = 17) but not within the epipelagic layer (p = 0.629; χ2 = 5.253, df = 7) (Figure 1).
Figure 1. Target strength (dB re 1 m2) density distributions during CTD cast 83 derived from the WBAT deployed at 320 m. Each distribution is approximately representative of a 12 min period where the WBAT was deployed. The asterisks indicate intervals where the water was sampled for eDNA.
A total of 3,033,134 sequence reads were recovered for the 40 samples in CTD83 and CTD84. Taxonomic classification using the Silva database (Release 128) identified 10,185 unique ASVs. Since the goal of this study was to assess detection of micronekton that are potentially vertically migrating, taxa that did not fit into this category based on taxonomic classification or had an unknown classification were excluded. Most taxa in the dataset were either unicellular (3,634 taxa) or could only be definitively classified as eukaryotes (6,326 taxa) and were thus excluded from analysis (Supplementary Table S1). The final analyzed dataset contained 92 taxa for mesopelagic CTD 83 (Supplementary Table S2), 74 taxa for mesopelagic CTD 84 (Supplementary Table S3), and 62 taxa in the epipelagic samples from both CTDs.
An exception to unicellular taxa not being involved in the DVM may be those unicellular taxa that are parasitic to vertically migrating taxa such as in the order Syndiniales, which were abundant in the current dataset (Guillou et al., 2008). Taxonomic classification of eDNA for most ASVs was only to order due to a short amplicon length (∼150 bp), limited taxonomic resolution in the Silva database, and a lack of many deep-sea organisms in taxonomic databases. In many cases, unique ASVs were detected, but these ASVs could only be classified to a higher taxonomic group. Henceforth, when individual taxa are referenced, these are unique ASVs that may be only broadly taxonomically classified.
For the ascending phase of the DVM in the mesopelagic, we observed significant shifts in eDNA diversity and composition over time (Supplementary File 1) with several groups of organisms exhibiting temporal shifts in relative abundance, suggesting variability in the structure of eukaryotic communities over time. Broad taxonomic groups such as arthropods, tunicates, annelids, cnidarians, ctenophores, and teleost fish were variable over the course of the DVM (Figure 2). Interestingly, different groups of organisms displayed peak relative abundance at different times during the DVM. For example, copepods in the class Maxillopoda appeared most abundant within the earliest sampled time-points of the DVM (at 19:00) whereas other taxa such as tunicates, ctenophores, and cnidarians appeared most abundant in the subsequent sample taken 26 min later. Teleost fish (Class Actinopterygii), which are typically considered to comprise much of the vertically migrating taxa biomass, did not display a high relative abundance in the eDNA dataset though they were consistently present (Figure 2). During the ascending DVM, gelatinous zooplankton (tunicates, ctenophores, and cnidarians) all exhibited the highest relative abundance within the middle time point of the vertically migrating band (at 19:26), however, since these organisms tend to only weakly scatter sound unless occurring in swarms (Ressler, 2002; D’Elia et al., 2016), it may be difficult to discern them in the acoustic dataset. Conversely, there is another group of gelatinous zooplankton, physonect siphonophores, that do scatter significant amounts of acoustic backscatter due to their gas-containing pneumatophore (Warren et al., 2001; Lavery et al., 2007). It is often not possible to acoustically differentiate scattering contributions from siphonophores and some swim-bladdered fish (Proud et al., 2019) which further complicates the analysis. Lastly, Annelids (Phylum Annelida) represented an abundant group in the eDNA dataset (Figure 2). Annelids in the class Clitellata exhibited an increase in relative abundance late in the ascending DVM (Supplementary Table S2).
Figure 2. Relative abundance of Annelida (A), Arthropoda (B), Hydrozoa (C), Ctenophora (D), Actinopterygii (E), and Tunicata, (F) in the eDNA community during the ascending diel vertical migration (CTD 83). Note different y-axis scales between each group.
The eDNA dataset allows us to breakdown each of these broad taxonomic groups to determine how many potential species are driving the observed dynamics (Supplementary Table S2). Previous research has noted variable vertical migration behavior within broader taxonomic groups, and eDNA techniques could help refine our understanding of these behaviors. Within our current dataset, we observed that in most cases, the main drivers of the DVM patterns (Figure 3) were due to only a few taxa in each group. For example, in Arthropoda, we observed 14 total taxa (Table 2), but only three of these taxa had much impact on the temporal variation of the group (Figure 3). Two of these taxa (ASV18, ASV19) exhibited a peak relative abundance early in the DVM, while the third taxon, ASV20, was most abundant late in the DVM. Each of these three taxa were copepods belonging to the order Calanoida. Similar to the results in Arthropoda, relatively few taxa were observed driving temporal variation in Cnidaria (3 dominant members from the order Siphonophorae), Ctenophora (1 dominant member), Tunicata (10 taxa with 2 main drivers from the family Oikopleuridae), teleost fishes (1 dominant member), and Annelids (1 dominant member).
Figure 3. Relative abundance of Annelida (A), Arthropoda (B), Hydrozoa (C), Ctenophora (D), Actinopterygii (E), and Tunicata, (F) in the eDNA community during the ascending diel vertical migration (CTD 83) broken down into individual amplicon sequence variants (ASVs) as identified by the Silva database. The dynamics of most groups were dominated by relatively few ASVs. Individual ASVs are represented by different colors in each panel and each legend lists the unique taxonomic identifier in the current dataset. ASVs with a maximum relative abundance less than 0.05% were not plotted. Note different y-axis scales between each group.
In the mesopelagic zone during the descending vertical migration of organisms, we also observed significant shifts in eDNA diversity and composition over short temporal scales (Supplementary Results). Similar to the ascending DVM in CTD 83, several broad groups of organisms were dynamic over the course of our sampling, however, the patterns were somewhat different in CTD 84. Tunicates in class Appendicularia showed a slight increase early in the vertical migration and then a gradual decrease in relative abundance over time (Figure 4). Arthropods in the class Maxillopoda (likely copepods) decreased in relative abundance until the middle of the vertical migration and then gradually increased in relative abundance. Ctenophore and Cnidarian relative abundance both peaked during the middle of the DVM. Teleost fish displayed low relative abundance in this dataset, but they were consistently present with high variability among samples. Annelids in the class Clitellata displayed a higher relative abundance late in the descending DVM (Figure 4), similar to the observed pattern in the ascending DVM (Figure 3).
Figure 4. Relative abundance of Annelida (A), Arthropoda (B), Hydrozoa (C), Ctenophora (D), Actinopterygii (E), and Tunicata, (F) in the eDNA community during the descending diel vertical migration (CTD 84).
The taxonomic composition for the descending DVM was somewhat different compared to the ascending DVM (Supplementary Table S3). In Arthropoda (18 taxa total), only ASV20, which appeared later in the DVM in the ascending phase of the DVM (CTD 83), was abundant in the descending DVM (Figure 5). Two other taxa (ASV 24 & ASV 27) in the order Calanoida and one taxon (ASV66) in the order Harpacticoida, were abundant in the descending DVM eDNA dataset. Fewer tunicate taxa were detected in the descending DVM, and we observed an absence of the two most abundant taxa from the ascending DVM. Annelids and teleost fish (Class Actinoptergii) exhibited lower richness compared to CTD83 and were comprised of only two and one unique taxa, respectively. Within the phyla Cnidaria and Ctenophora, we detected 15 and 8 unique taxa, respectively, though both were generally less abundant compared to CTD83, and were dominated by only a few taxa (Figure 5). The majority of Cnidarian taxa belonged to the order Siphonophorae (9 taxa), which includes taxa with gas inclusions (in suborder Physonectae), but taxonomic identification was only possible to order with the current sequence data.
Figure 5. Relative abundance of Annelida (A), Arthropoda (B), Hydrozoa (C), Ctenophora (D), Actinopterygii (E), and Tunicata, (F) in the eDNA community during the descending diel vertical migration (CTD 84) broken down into individual amplicon sequence variants (ASVs) as identified by the Silva database. Individual ASVs are represented by different colors in each panel and each legend lists the unique taxonomic identifier in the current dataset. ASVs with a maximum relative abundance less than 0.05% were not plotted.
Our samples in the epipelagic zone, which occurred during the DVM, represent post-migration samples for both CTD 83 (night) and CTD84 (day). The eDNA in these samples were composed of similar groups of taxa to those in mesopelagic including copepods (Class Maxillopoda), tunicates (Class Appendicularia), Annelids (Class Clitellata), and hydrozoans (Class Hydrozoa; Supplementary Figure S1). Of these six groups, Maxillopoda was the richest, containing 33 unique ASVs. Within this group, some of the same taxa were dominant (e.g., ASV18), but overall, there appeared to be more copepods in the orders Cyclopoida and Harpacticoida compared to samples from the mesopelagic zone. Cnidarians in the class Hydrozoa were less prevalent and less rich in our epipelagic samples, though some similar taxa were detected. The full results for the epipelagic taxa are summarized in Supplementary Table S4.
Studying the oceanic pelagic environment is challenging due to remoteness, depth, and the vast expanse of ocean that this zone covers. Given these fundamental challenges, cost-effective, yet comprehensive tools are needed to capture the biodiversity and dynamics of this understudied environment. Remote sensing tools such as echosounders have been utilized to capture the placement and movement (i.e., DVM) of organisms in the pelagic environment (Milligan et al., 2018), but the ability of this technology to resolve the identities of organisms is limited (D’Elia et al., 2016). The current study suggests that eDNA has the potential to fill in some of these gaps in our knowledge through its ability to resolve finer scale taxonomic identities of the organisms in this environment. Our results show that during the DVM, we are able to detect changes in the eDNA community that are concurrent with shifts in the depth of the SSLs through the migration process. The eDNA results show a complex community of arthropods, tunicates, cnidarians, ctenophores, annelids, and fish, which are all known members of the pelagic environment and in some cases are known diel vertical migrators.
Our eDNA dataset detected many resident fauna of the pelagic environment, and while we had more resolution on organism identity compared to acoustics, we were often not able to resolve fine-scale taxonomic identities. With our short 18S rRNA fragment, we were only able to identify ∼31% of our sequences further than a kingdom classification. The presence of many unidentified sequences may represent additional biodiversity in key pelagic groups that we were unable to classify here. Although efforts have been growing, the pelagic environment is understudied relative to many other habitats (Sutton, 2013; de Vargas et al., 2015; Sieracki et al., 2019). The poor classification of many of our sequences may be due to a lack of representation of whole faunal groups or at least an absence of closely related taxa in the Silva database. These instances would lead to low taxonomic resolution in our dataset despite being able to parse individual taxa via our sequence-clustering algorithm. In addition to our classification issues, we also observed the absence or low abundance of some faunal groups that we expected to be more abundant. For example, teleost fish (Class Actinopterygii) were present, but only in low abundance in our dataset. We hypothesized that much of the biomass observed in the acoustic data was attributed to teleost fish, but this dominance is not reflected in the eDNA dataset. We also expected to observe other groups of crustaceans such as decapods in our eDNA dataset (Thomsen and Willerslev, 2015; D’Elia et al., 2016), but surprisingly these groups were absent. Potential explanations for these findings include: (1) low concentrations of eDNA from these organisms present in the environment during the short timeframe we sampled (low rate of eDNA production) or the actual low abundance of these organisms, (2) a lack of resolution in the taxonomic database to identify organisms in these taxonomic groups (organisms are present in dataset but unidentified), (3) organisms like physonect siphonophores were contributing to acoustic backscatter ascribed to teleost fish (Proud et al., 2019), and (4) issues with sequence processing methodology or the presence of inhibitors in certain cells that prevented proper sequencing of taxa in these groups. Previous studies have noted the lack of coverage of many key pelagic taxonomic groups in major databases such as NCBI and Barcode of Life Data System (BoLD) (Kvist, 2013; Cowart et al., 2018), and at present, these limitations likely limit the overall ability of 18S eDNA to finely resolve biodiversity in these hard to study environments.
We identified a diverse array of taxa in the pelagic environment that corresponds with previous faunal assemblage characterizations (Table 2). In our present dataset, sequences matching several groups of tunicates were identified, and taxonomic classification of these sequences indicated that they belonged to the groups Appendicularia (Larvaceans) and Thaliacea (Salps & Pyrosomes). Previous research has shown that members of each of these groups can alter biogeochemical cycling in the pelagic environment, through carbon export to deeper depths (Andersen, 1998). For Appendicularia, the dominant members of our eDNA dataset, this is through their contributions to marine snow via their discarded mucus houses (Barham, 1979), which can be a food source for other pelagic organisms such as copepods (Steinberg, 1995). Representative taxa such as Pyrosoma atlantica have been observed and collected via MOCNESS sampling at depth in the nGoM during DEEPEND cruises (Cook and Sutton, 2018), and this species has been identified in DVM elsewhere (Henschke et al., 2019). In addition, our eDNA dataset revealed as many as 17 Cnidarian taxa belonging to the class Hydrozoa. These hydrozoans were classified into three different orders, with Siphonophorae being the most abundant and species rich. These taxa are broadly distributed in all oceans forming crucial trophic links in the deep sea (Robison, 2004; Mapstone, 2014). Several members of this order contain gas-inclusions (sub-order Physonectae) and are therefore detectable by our acoustic sensors. Our current eDNA dataset cannot determine what proportion of the community is physonect siphonophores, but previous studies in the GoM have shown this group can be prevalent among siphonophore assemblages (Sanvicente-Añorve et al., 2007). Ctenophores (phylum Ctenophora), while generally less abundant in the eDNA dataset than tunicates or cnidarians, were consistently detected in both the ascending and descending DVM samples. Ctenophores in four orders were present in our dataset along with three taxa that could not be classified past phylum. Some species of ctenophores and siphonophores have been observed to vertically migrate when strong physical stratification of the water column is absent, thus some taxa present in our dataset may represent active migrators over the course of our experimental timeframe (Júnior et al., 2015). Copepods (Class Maxillopoda) appear as the most dominant potential migrator in our eDNA dataset, comprising nearly 20% of eDNA sequences at some time points. Copepods are abundant zooplankton members in many ocean basins, and previous research has observed variable DVM patterns within this group (Bollens and Frost, 1991; Hays et al., 2001). Across our eDNA dataset, we identified at least 20 copepod taxa, of which 13 were classified as Calanoida. The dominance of calanoid copepods is consistent with other research showing that this group is the most successful of all copepods and able to colonize all depths in the water column (e.g., Huys and Boxshall, 1991; Fosshagen et al., 2001).
The acoustic data in the present study capture the movement of mostly fish and other strong scatterers that form the dominant members of SSLs (> −60 dB). A clear migration signal can be seen for these organisms rising toward the surface displaying the characteristic DVM behavior, which co-occurs with a gradual increase in the size of organisms around the CTD (Figure 5). While our eDNA dataset does not necessarily capture an increase in these large migrators, we do see an increase in the relative abundance of copepod sequences (Figure 6) that precedes the arrival of larger acoustic targets (i.e., micronekton). Previous research has shown that calanoid copepods, the dominant copepod in our eDNA dataset, are often numerically dominant in these habitats and will migrate vertically to avoid predation (Bollens and Frost, 1989, 1991; Proud et al., 2019). While we lack direct evidence for predation avoidance in the current study, the pattern of relative abundance for two calanoid taxa (ASV18 & ASV19) in CTD 83 may suggest that these taxa are ascending to avoid vertically migrating micronekton predators. Further investigation of these dynamics is warranted, including direct sampling of these organisms, to derive any causality for the observed copepod dynamics, since other research has shown copepod vertical migration can be related to body condition (Hays et al., 2001) or environmental cues (Batchelder et al., 2002).
Figure 6. Relative abundance of copepods detected with eDNA from water samples collected at depth (arrows) relative to the deep scattering layer observed at 38 kHz. Two water collection events are displayed (red line, CTD 83 and 84) during the ascending and descending phase of the migration, respectively. The WBAT was deployed on the CTD during the ascending migration phase at 320 m and 93 m depth). The two black vertical regions in the echogram represent periods where the echosounder was not operational.
There are a few points of uncertainty in the current study that prevent us from drawing a direct relationship between the eDNA and acoustic datasets. The 18S eDNA dataset estimates a relative abundance of sequences in a volume of water, which can be difficult to relate to absolute abundances captured in the acoustic data. Additionally, we have some uncertainty as to whether our eDNA samples encompass a definitive time frame given the potential for differential residence time (i.e., how quickly DNA degrades in the pelagic environment; Rees et al., 2014; Thomsen and Willerslev, 2015) and how the presence of an organism in the environment relates to the timing of its presence in the eDNA dataset. Previous research has indicated a relatively low residence time for DNA in seawater (Dell’Anno and Corinaldesi, 2004), which would support that our eDNA dataset dynamics are capturing recent shifts in organism abundance. Despite these limitations, our data show the potential for eDNA to be widely used as a tool for monitoring pelagic biodiversity and the dynamics of the DVM. Several aspects of this study could be improved in future studies to increase the impact and resolution of eDNA data, and here we have made a few recommendations. Since the eDNA dataset captured many smaller pelagic organisms, coupling future eDNA samples with net sampling or a higher acoustic frequency that could discern smaller organisms such as copepods, would help calibrate eDNA results. These comparisons could help answer some key questions in eDNA research such as providing estimates of spatial and temporal distributions and more precise links between the presence of organisms in the environment and the presence of their DNA. Using multiple primer sets [e.g., 18S – current study, COI (fish and molluscs), 16S (crustaceans); Bernard et al., 2017; Bracken-Grissom, 2017] in order to minimize primer bias in PCR reactions and generating longer sequencing amplicons would likely help capture additional diversity in the pelagic environment. Alternatively, a non-PCR metagenomics approach would eliminate all PCR bias but would require greatly increased sequencing depth.
In conclusion, we have expanded the application of an established molecular ecology method (eDNA sequencing) to an important global phenomenon (DVM). We demonstrate the identification of a diverse marine community within our eDNA dataset that for certain groups may capture additional biodiversity compared to more traditional sampling methods. Our data indicate that the taxa present before the DVM (outside the SSL) differ from those present within the SSLs and after the DVM. Some dynamics within our dataset appear to mirror changes in the acoustic data suggesting eDNA metabarcoding is capturing real shifts in the composition of vertically migrating organisms, however, additional effort is needed to resolve the source of backscatter data collected at depth and the identity of organisms that are being detected in eDNA profiles during DVM activities.
Data are publicly available through the Gulf of Mexico Research Initiative Information and Data Cooperative (GRIIDC) at https://data.gulfresearchinitiative.org [CTD Data DP05: doi: 10.7266/N7GM85P1 (English et al., 2018); raw acoustic data for DP05: doi: 10.7266/N7474871 (Boswell et al., 2018; Easson, 2020); eDNA sequence data DP05: doi: 10.7266/n7-awwx-4g13].
CE, KB, and JW contributed to the conception and design of the study. JL and KB collected acoustic and seawater samples. CE generated genetic data, organized datasets, performed eDNA analysis, and constructed the initial design for the manuscript. KB, NT, and JW processed acoustic and CTD data for analysis. All authors contributed to the article and approved the submitted version.
This research was made possible by a grant from The Gulf of Mexico Research Initiative to support the consortium research entitled “Deep Pelagic Nekton Dynamics of the Gulf of Mexico” administered by Nova Southeastern University. This was contribution #200 from the Center for Coastal Oceans Research in the Institute of Water and Environment at Florida International University.
The authors declare that the research was conducted in the absence of any commercial or financial relationships that could be construed as a potential conflict of interest.
The Supplementary Material for this article can be found online at: https://www.frontiersin.org/articles/10.3389/fmars.2020.00552/full#supplementary-material
Andersen, V. (1998). “Salp and pyrosomid blooms and their importance in biogeochemical cycles,” in The Biology of Pelagic Tunicates, ed. Q. Bone (New York, NY: Oxford University Press), 125–137.
Barham, E. G. (1979). Giant larvacean houses: observations from deep submersibles. Science 205, 1129–1131. doi: 10.1126/science.205.4411.1129
Batchelder, H. P., Edwards, C. A., and Powell, T. M. (2002). Individual-based models of copepod populations in coastal upwelling regions: implications of physiologically and environmentally influenced diel vertical migration on demographic success and nearshore retention. Prog. Oceanogr. 53, 307–333. doi: 10.1016/s0079-6611(02)00035-6
Bernard, A., Weber, M., Finnegan, K., Shivji, M., and Eytan, R. (2017). DNA Sequences of the Mitochondrial Cytochrome C Oxidase I (COI) Genes From Deep Sea Fishes. Cruises DP01 and DP02 from May 2015 - August 2015. Texas, TX: Gulf of Mexico Research Initiative Information and Data Cooperative (GRIIDC).
Bista, I., Carvalho, G. R., Walsh, K., Seymour, M., Hajibabaei, M., Lallias, D., et al. (2017). Annual time-series analysis of aqueous eDNA reveals ecologically relevant dynamics of lake ecosystem biodiversity. Nat. Comm. 8:14087.
Bollens, S. M., and Frost, B. W. (1989). Zooplanktivorous fish and variable diel vertical migration in the marine planktonic copepod Calanus pacificus. Limnol. Oceanogr. 34, 1072–1083. doi: 10.4319/lo.1989.34.6.1072
Bollens, S. M., and Frost, B. W. (1991). Diel vertical migration in zooplankton: rapid individual response to predators. J. Plankton Res. 13, 1359–1365. doi: 10.1093/plankt/13.6.1359
Boswell, K., Sutton, T. T., and LaBua, S. (2018). Raw Acoustic Scattering Data of Organisms From the Water Column, Cruise DP05, April 2017 – May 2017. Texas, TX: Gulf of Mexico Research Initiative Information and Data Cooperative (GRIIDC).
Boswell, K. M., D’Elia, M., Johnston, M. W., Mohan, J. A., Warren, J. D., Wells, R. J., et al. (2020). Oceanographic structure and light levels drive patterns of sound scattering layers in a low-latitude oceanic system. Front. Mar. Sci. 7:51. doi: 10.3389/fmars.2020.00051
Bracken-Grissom, H. (2017). 16S and COI Barcoding Sequences for Crustaceans and Cephalopods in the Gulf of Mexico and Florida Straits From 2015-2016. Texas, TX: Gulf of Mexico Research Initiative Information and Data Cooperative (GRIIDC).
Bucklin, A., Lindeque, P. K., Rodriguez-Ezpeleta, N., Albaina, A., and Lehtiniemi, M. (2016). Metabarcoding of marine zooplankton: prospects, progress and pitfalls. J. Plankton Res. 38, 393–400. doi: 10.1093/plankt/fbw023
Callahan, B. J., McMurdie, P. J., Rosen, M. J., Han, A. W., Johnson, A. J. A., and Holmes, S. P. (2016). DADA2: high-resolution sample inference from illumina amplicon data. Nat. Methods 13:581. doi: 10.1038/nmeth.3869
Cook, A., and Sutton, T. (2018). Inventory of Oceanic Fauna Data Including Species, Weight, and Measurements From R/V Point Sur (Cruise DP05) in the Gulf of Mexico From 2017-05-01 to 2017-05-11. Texas, TX: Gulf of Mexico Research Initiative Information and Data Cooperative (GRIIDC).
Cowart, D. A., Murphy, K. R., and Cheng, C. H. C. (2018). Metagenomic sequencing of environmental DNA reveals marine faunal assemblages from the West Antarctic Peninsula. Mar. Genom. 37, 148–160. doi: 10.1016/j.margen.2017.11.003
De Robertis, A., and Higginbottom, I. (2007). A post-processing technique to estimate the signal-to-noise ratio and remove echosounder background noise. ICES J. Mar. Sci. 64, 1282–1291. doi: 10.1093/icesjms/fsm112
de Vargas, C., Audic, S., Henry, N., Decelle, J., Mahé, F., Logares, R., et al. (2015). Eukaryotic plankton diversity in the sunlit ocean. Science 348:126 1605.
D’Elia, M., Warren, J. D., Rodriguez-Pinto, I., Sutton, T. T., Cook, A., and Boswell, K. M. (2016). Diel variation in the vertical distribution of deep-water scattering layers in the Gulf of Mexico. Deep Sea Res. Part I Oceanogr. Res. Pap. 115, 91–102. doi: 10.1016/j.dsr.2016.05.014
Dell’Anno, A., and Corinaldesi, C. (2004). Degradation and turnover of extracellular DNA in marine sediments: ecological and methodological considerations. Appl. Environ. Microbiol. 70, 4384–4386. doi: 10.1128/aem.70.7.4384-4386.2004
Demer, D. A., Berger, L., Bernasconi, M., Bethke, E., Boswell, K., Chu, D., et al. (2015). Calibration of Acoustic Instruments. ICES Cooperative Research Report No. 326.
Easson, C. (2020). “Eukaryotic 18S rRNA Sequences as part of eDNA analyses of Diel Vertical Migration of Mixed Consortia in the Gulf of Mexico from 2016-05-03 to 2017-05-11,” in Distributed by: Gulf of Mexico Research Initiative Information and Data Cooperative (GRIIDC), Harte Research Institute (Texas A&M University–Corpus Christi). doi: 10.7266/n7-awwx-4g13
Easson, C. G., and Lopez, J. V. (2019). Environmental drivers of bacterioplankton community structure in the northern Gulf of Mexico. Front. Microb. 9:3175. doi: 10.3389/fmicb.2018.03175
English, D., Hu, C., Cook, A., and Sutton, T. (2018). Conductivity, Temperature, and Depth (CTD) Data for DEEPEND Stations, Cruise DP05, May 2017. Texas, TX: Gulf of Mexico Research Initiative Information and Data Cooperative (GRIIDC).
Fennell, S., and Rose, G. (2015). Oceanographic influences on deep scattering layers across the North Atlantic. Deep Sea Res. Part I Oceanogr. Res. Pap. 105, 132–141. doi: 10.1016/j.dsr.2015.09.002
Foote, A. D., Thomsen, P. F., Sveegaard, S., Wahlberg, M., Kielgast, J., Kyhn, L. A., et al. (2012). Investigating the potential use of environmental DNA (eDNA) for genetic monitoring of marine mammals. PLoS One 7:e41781. doi: 10.1371/journal.pone.0041781
Fosshagen, A., Boxshall, G. A., and Iliffe, T. M. (2001). The epacteriscidae, a cave-living family of calanoid copepods. Sarsia 86, 245–318. doi: 10.1080/00364827.2001.10425520
Guillou, L., Viprey, M., Chambouvet, A., Welsh, R. M., Kirkham, A. R., Massana, R., et al. (2008). Widespread occurrence and genetic diversity of marine parasitoids belonging to Syndiniales (Alveolata). Environ. Microbiol. 10, 3349–3365. doi: 10.1111/j.1462-2920.2008.01731.x
Hays, G. C. (2003). “A review of the adaptive significance and ecosystem consequences of zooplankton diel vertical migrations,” in Migrations and Dispersal of Marine Organisms, (Dordrecht: Springer), 163–170. doi: 10.1023/b:hydr.0000008476.23617.b0
Hays, G. C., Kennedy, H., and Frost, B. W. (2001). Individual variability in diel vertical migration of a marine copepod: why some individuals remain at depth when others migrate. Limnol. Oceanogr. 46, 2050–2054. doi: 10.4319/lo.2001.46.8.2050
Hazen, E. L., Friedlaender, A. S., Thompson, M. A., Ware, C. R., Weinrich, M. T., Halpin, P. N., et al. (2009). Fine-scale prey aggregations and foraging ecology of humpback whales Megaptera novaeangliae. Mar. Ecol. Prog. Ser. 395, 75–89. doi: 10.3354/meps08108
Henschke, N., Pakhomov, E. A., Kwong, L. E., Everett, J. D., Laiolo, L., Coghlan, A. R., et al. (2019). Large vertical migrations of Pyrosoma atlanticum play an important role in active carbon transport. J. Geophys. Res. Biogeo. 124, 1056–1070. doi: 10.1029/2018jg004918
Irigoien, X., Klevjer, T. A., Røstad, A., Martinez, U., Boyra, G., Acuña, J. L., et al. (2014). Large mesopelagic fishes biomass and trophic efficiency in the open ocean. Nat. Comm. 5:3271.
Júnior, M. N., Brandini, F. P., and Codina, J. C. U. (2015). Diel vertical dynamics of gelatinous zooplankton (Cnidaria, Ctenophora and Thaliacea) in a subtropical stratified ecosystem (South Brazilian Bight). PLoS One 10:e0144161. doi: 10.1371/journal.pone.0144161
Kembel, S. W., Cowan, P. D., Helmus, M. R., Cornwell, W. K., Morlon, H., Ackerly, D. D., et al. (2010). Picante: R tools for integrating phylogenies and ecology. Bioinformatics 26, 1463–1464. doi: 10.1093/bioinformatics/btq166
Klevjer, T. A., Irigoien, X., Røstad, A., Fraile-Nuez, E., Benítez-Barrios, V. M., and Kaartvedt, S. (2016). Large scale patterns in vertical distribution and behaviour of mesopelagic scattering layers. Sci. Rep. 6:19873.
Kloser, R. J., Ryan, T. E., Keith, G., and Gershwin, L. (2016). Deep-scattering layer, gas-bladder density, and size estimates using a two-frequency acoustic and optical probe. ICES J. Mar. Sci. 73, 2037–2048. doi: 10.1093/icesjms/fsv257
Kloser, R. J., Ryan, T. E., Young, J. W., and Lewis, M. E. (2009). Acoustic observations of micronekton fish on the scale of an ocean basin: potential and challenges. ICES J. Mar. Sci. 66, 998–1006. doi: 10.1093/icesjms/fsp077
Kubilius, R., Ona, E., and Calise, L. (2015). Measuring in situ krill tilt orientation by stereo photogrammetry: examples for Euphausia superba and Meganyctiphanes norvegica. ICES J. Mar. Sci. 72, 2494–2505. doi: 10.1093/icesjms/fsv077
Kvist, S. (2013). Barcoding in the dark: a critical view of the sufficiency of zoological DNA barcoding databases and a plea for broader integration of taxonomic knowledge. Mol. Phylogenet. Evol. 69, 39–45. doi: 10.1016/j.ympev.2013.05.012
Lavery, A. C., Wiebe, P. H., Stanton, T. K., Lawson, G. L., Benfield, M. C., and Copley, N. (2007). Determining dominant scatterers of sound in mixed zooplankton populations. J. Acoust. Soc. Am. 122, 3304–3326. doi: 10.1121/1.2793613
Lurton, X. (2002). An Introduction to Underwater Acoustics: Principles and Applications. Berlin: Springer Science & Business Media.
MacLennan, D. N., Fernandes, P. G., and Dalen, J. (2002). A consistent approach to definitions and symbols in fisheries acoustics. ICES J. Mar. Sci. 59, 365–369. doi: 10.1006/jmsc.2001.1158
Mapstone, G. M. (2014). Global diversity and review of Siphonophorae (Cnidaria: Hydrozoa). PLoS One 9:e87737. doi: 10.1371/journal.pone.0087737
McDougall, T. J., and Barker, P. M. (2011). Getting started with TEOS-10 and the Gibbs Seawater (GSW) oceanographic toolbox. SCOR/IAPSO WG 127, 1–28.
Milligan, R. J., Bernard, A. M., Boswell, K. M., Bracken-Grissom, H. D., D’Elia, M. A., DeRada, S., et al. (2018). The application of novel research technologies by the deep pelagic nekton dynamics of the Gulf of Mexico (DEEPEND) Consortium. Mar. Technol. Soc. J. 52, 81–86. doi: 10.4031/mtsj.52.6.10
Oksanen, J., Blanchet, F. G., Friendly, M., Kindt, R., Legendre, P., McGlinn, D., et al. (2017). Vegan: Community Ecology Package. R package version 2.4–5.
Proud, R., Cox, M. J., and Brierley, A. S. (2017). Biogeography of the global ocean’s mesopelagic zone. Curr. Biol. 27, 113–119. doi: 10.1016/j.cub.2016.11.003
Proud, R., Handegard, N. O., Kloser, R. J., Cox, M. J., and Brierley, A. S. (2019). From siphonophores to deep scattering layers: uncertainty ranges for the estimation of global mesopelagic fish biomass. ICES J. Mar. Sci. 76, 718–733. doi: 10.1093/icesjms/fsy037
Quast, C., Pruesse, E., Gerken, J., Schweer, T., Yilmaz, P., Peplies, J., et al. (2015). “SILVA databases,” in Encyclopedia of Metagenomics: Genes, Genomes and Metagenomes: Basics, Methods, Databases and Tools, ed. K. E. Nelson (Berlin: Springer). 626–635.
R Core Team (2018). R: A Language and Environment for Statistical Computing. Vienna: R Foundation for Statistical Computing.
Rees, H. C., Maddison, B. C., Middleditch, D. J., Patmore, J. R., and Gough, K. C. (2014). The detection of aquatic animal species using environmental DNA–a review of eDNA as a survey tool in ecology. J. Appl. Ecol. 51, 1450–1459. doi: 10.1111/1365-2664.12306
Ressler, P. H. (2002). Acoustic backscatter measurements with a 153 kHz ADCP in the northeastern Gulf of Mexico: determination of dominant zooplankton and micronekton scatterers. Deep Sea Res. Part I Oceanogr. Res. Pap. 49, 2035–2051. doi: 10.1016/S0967-0637(02)00117-6
Robison, B. H. (2004). Deep pelagic biology. J. Exp. Mar. Biol. Ecol. 300, 253–272. doi: 10.1016/j.jembe.2004.01.012
Ruppert, K. M., Kline, R. J., and Rahman, M. S. (2019). Past, present, and future perspectives of environmental DNA (eDNA) metabarcoding: a systematic review in methods, monitoring, and applications of global eDNA. Glob. Ecol. Conserv. 17: e00547. doi: 10.1016/j.gecco.2019.e00547
Sanvicente-Añorve, L., Alba, C., Alatorre, M. A., and Flores-Coto, C. (2007). Cross-shelf and vertical distribution of siphonophore assemblages under the influence of freshwater outflows in the southern Gulf of Mexico. Hydrobiologia 586, 69–78. doi: 10.1007/s10750-006-0492-6
Sieracki, M. E., Poulton, N. J., Jaillon, O., Wincker, P., de Vargas, C., Rubinat-Ripoll, L., et al. (2019). Single cell genomics yields a wide diversity of small planktonic protists across major ocean ecosystems. Sci. Rep. 9, 1–11.
Steinberg, D. K. (1995). Diet of copepods (Scopalatum vorax) associated with mesopelagic detritus (giant larvacean houses) in Monterey Bay, California. Mar. Biol. 122, 571–584. doi: 10.1007/bf00350679
Sutton, T. T. (2013). Vertical ecology of the pelagic ocean: classical patterns and new perspectives. J. Fish. Biol. 83, 1508–1527. doi: 10.1111/jfb.12263
Sutton, T. T., Clark, M. R., Dunn, D. C., Halpin, P. N., Rogers, A. D., Guinotte, J., et al. (2017). A global biogeographic classification of the mesopelagic zone. Deep Sea Res. Part I Oceanog. Res. Pap. 126, 85–102. doi: 10.1016/j.dsr.2017.05.006
Thomsen, P. F., and Willerslev, E. (2015). Environmental DNA–An emerging tool in conservation for monitoring past and present biodiversity. Biol. Conserv. 183, 4–18. doi: 10.1016/j.biocon.2014.11.019
Warren, J. D., Stanton, T. K., Benfield, M. C., Wiebe, P. H., Chu, D., and Sutor, M. (2001). In situ measurements of acoustic target strengths of gas-bearing siphonophores. ICES J. Mar. Sci. 58, 740–749. doi: 10.1006/jmsc.2001.1047
Keywords: pelagic, Gulf of Mexico, eDNA, acoustics, DEEPEND
Citation: Easson CG, Boswell KM, Tucker N, Warren JD and Lopez JV (2020) Combined eDNA and Acoustic Analysis Reflects Diel Vertical Migration of Mixed Consortia in the Gulf of Mexico. Front. Mar. Sci. 7:552. doi: 10.3389/fmars.2020.00552
Received: 30 August 2019; Accepted: 16 June 2020;
Published: 08 July 2020.
Edited by:
Øyvind Fiksen, University of Bergen, NorwayReviewed by:
Luis Manuel Bolaños, Oregon State University, United StatesCopyright © 2020 Easson, Boswell, Tucker, Warren and Lopez. This is an open-access article distributed under the terms of the Creative Commons Attribution License (CC BY). The use, distribution or reproduction in other forums is permitted, provided the original author(s) and the copyright owner(s) are credited and that the original publication in this journal is cited, in accordance with accepted academic practice. No use, distribution or reproduction is permitted which does not comply with these terms.
*Correspondence: Cole G. Easson, Y2dlYXNzb244NkBnbWFpbC5jb20=
Disclaimer: All claims expressed in this article are solely those of the authors and do not necessarily represent those of their affiliated organizations, or those of the publisher, the editors and the reviewers. Any product that may be evaluated in this article or claim that may be made by its manufacturer is not guaranteed or endorsed by the publisher.
Research integrity at Frontiers
Learn more about the work of our research integrity team to safeguard the quality of each article we publish.