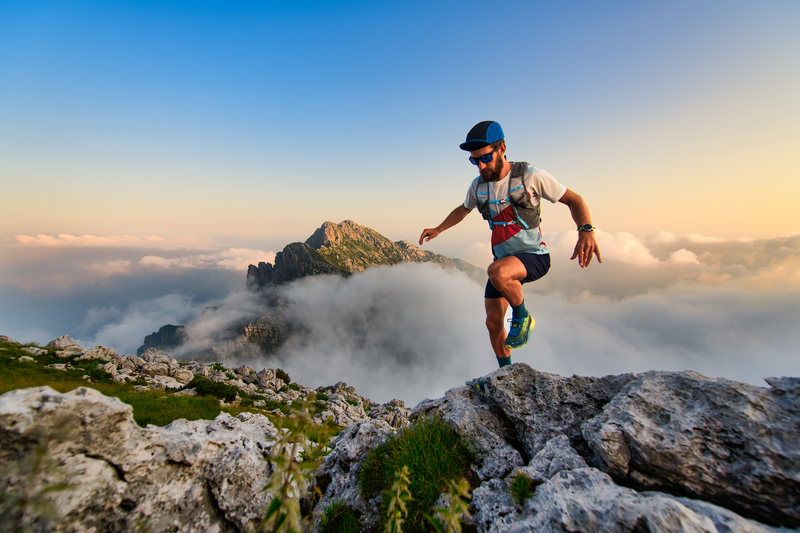
94% of researchers rate our articles as excellent or good
Learn more about the work of our research integrity team to safeguard the quality of each article we publish.
Find out more
ORIGINAL RESEARCH article
Front. Mar. Sci. , 30 June 2020
Sec. Aquatic Microbiology
Volume 7 - 2020 | https://doi.org/10.3389/fmars.2020.00472
This article is part of the Research Topic Towards a Mechanistic Understanding of Marine Microbial Ecosystems View all 10 articles
Given the biogeochemical functions of marine microbes, the structure and functional diversity of bacterioplankton communities could be regarded as bioindicators that reflect environmental quality. The habitat of the large yellow croaker (Pseudosciaena crocea) is suffering from widespread degradation, but the study of the croaker habitat is still insufficient. Here, we used 16S rRNA gene sequencing and functional prediction to investigate the surface bacterioplankton communities in an overwintering ground of P. crocea during the overwintering period (November 2018 to April 2019). Our results showed that the taxa and functions of the environmental bacterioplankton community exhibited obvious seasonality. Specifically, the bacterioplankton communities in autumn were characterized by animal parasites or symbionts, such as Acinetobacter baumannii and Moraxella, while organic substance–degrading bacteria, such as Rhodococcus, Stenotrophomonas, and Alcanivorax, dominated in spring. Moreover, phylogenetic-based mean nearest taxon distance (MNTD) analyses indicated that dispersal limitation was the most important process governing the spatiotemporal assembly of the bacterioplankton communities. The distance decay of similarity also suggested the impact of dispersal limitation on the generation of biogeographical patterns. Furthermore, variation partitioning analysis (VPA) and a partial Mantel test revealed that environmental filters, such as nutrients, temperature, and salinity, had relatively weak deterministic effects on the bacterioplankton community assembly. Overall, these findings provide a basis for understanding the theory of surface microbial community assembly in the overwintering habitat of the large yellow croaker.
Abundant fisheries resources are indispensable cornerstones for the sustainable development of the coastal economy (Tursi et al., 2015; Keen et al., 2018). However, as China’s most productive fishing area, the East China Sea has been suffering from resource degradation in recent decades due to increasing human impacts, such as overexploitation, pollutant discharge, and climate change (Wang et al., 2012). The Chinese government has implemented a series of strict fishing moratoriums and stock management systems since 1995, and the current status of most natural resources has generally improved. Despite all these programs, the wild stocks of large yellow croaker (Pseudosciaena crocea), which is known as China’s national fish, have not recovered (Liu and de Mitcheson, 2008). Evidence strongly suggests that coastal pollution, habitat degradation, and possible ecosystem changes may have compromised the potential for stock recovery (Liu and de Mitcheson, 2008). However, the habitat (including the spawning, nursery, and overwintering grounds and migration channels) of the large yellow croaker has not been sufficiently researched. According to inferred migratory routes, croaker aggregate in overwintering grounds from October to April each year (Liu and de Mitcheson, 2008; Xu and Chen, 2011; Chen and Xu, 2012). Because the low temperature may suppress croaker immune functions by influencing multiple physiological processes and thereby make the croaker susceptible to the surrounding environment (Yang et al., 2015; Liu et al., 2019), the habitat conditions of their overwintering grounds are vital for recovering their stock and supporting this marine resource.
Water microorganisms, especially planktonic bacteria, are one of the largest reserves of biodiversity and promote various ecological processes in marine ecosystems (Sunagawa et al., 2015). On the one hand, bacteria have long been used as sensitive and aggregative indicators of changes in environmental conditions due to their wide distribution, short generation time, and diverse functions (Dai et al., 2017; Yang et al., 2018). On the other hand, the bacterioplankton community includes a large number of probiotic organisms and pathogens that can directly affect fish health (Xiong et al., 2015; Hu et al., 2017). Effects of bacterioplankton on fish could also be indirect through the food chain (Kalcheva et al., 2008). For example, a microbial loop mediated by bacteria could affect the upper elements of the food chain of the croaker. Therefore, an accurate representation of the overwintering ground’s current conditions can be systematically derived by observing the marine bacterioplankton community. Given the important roles of the bacterioplankton community in the assessment of the habitat environment, it is necessary to reveal the fundamental processes that underlie biogeographic and ecological patterns of bacterioplankton diversity. However, this field of study remains a major challenge in bacterial ecology (Jiao et al., 2019). Historically, bacterial community assembly has been studied from a deterministic perspective, for example, environmental filtering and biotic interactions (Dini-Andreote et al., 2015). However, an increasing number of recent studies support the idea that stochasticity plays a predominant role in some microbial systems (Zhou et al., 2013; Chen W. D. et al., 2019), especially in the biogeographical patterns of marine bacterioplankton systems (Giovannoni and Nemergut, 2014). In fact, community assembly is affected by deterministic and stochastic processes simultaneously, but the relative effects of these two processes vary among different ecosystems. Various methods have been developed to separate the relative effects of deterministic and stochastic processes. Among them, a phylogenetic framework that uses information about phylogenetic relatedness and phylogenetic dissimilarity derived from phylogenetic trees has been widely used (Stegen et al., 2013, 2015). Using this framework will help us to reveal the potential factors underlying the spatiotemporal patterns of bacterial communities in the overwintering grounds for croaker. In addition, the focus of bacterial ecology is turning from its initial focus on pattern description toward functional profiling. The Functional Annotation of Prokaryotic Taxa (FAPROTAX), which focuses on the biogeochemical cycle functions of microorganisms, is commonly used (Louca et al., 2016). This method depends on prokaryotic functional data from culturable bacteria and species classification (OTU table) to obtain community function information and provides opportunities to understand the differences in the structure and function of bacterioplankton communities (Yan et al., 2019).
In the present study, we investigated the surface bacterioplankton communities in the overwintering grounds of P. crocea during the overwintering period using high-throughput sequencing of 16S rRNA genes, aiming at (1) revealing the spatiotemporal patterns of taxonomic and functional profiles of bacterioplankton communities in the overwintering grounds and (2) understanding the processes that underlie the observed patterns in species abundances across space and time. These results are essential for understanding microbial community assembly theory in this marine ecosystem and adding to the data on the environmental microbiome in the surface water of croaker habitat.
Surface water samples (∼2 m) were collected in sterile containers from the overwintering ground for P. crocea (Figure 1), which is located in the East China Sea during 17 November–5 December 2018 (November) and 7–30 April 2019 (April). In total, we obtained 50 water samples (25 sites × 2 time points). The samples were stored in an icebox (4°C) and immediately transported to the refrigerator at −20°C. Environmental variables of water samples, including water temperature (WT), salinity (SAL), orthophosphate (PO4), nitrite (NO2), nitrate (NO3), ammonium (NH4), silicate (SiO3), total nitrogen (TN), and total phosphorus (TP), were analyzed following the standard methods (AQSIQ, 2007) (Supplementary Table S1). Approximately 500 mL of a water sample from each site was filtered-sterilized onto one 0.2-μm pore-sized polycarbonate membrane (47 mm diameter, Millipore, Boston, MA, United States) on the sampling day. The membranes were immediately frozen at −80°C until they were needed.
Figure 1. Study area and location of sampling stations in the overwintering ground. To keep the data comparable with future studies, we keep the discontinuous numbers for site names according to the routine-monitoring project. The sampling sites remain the same for both seasons, except for November at B2 and F2 and April at B1 and F1.
Bacterioplankton DNA was extracted using a DNA extraction kit (MinkaGene Water DNA Kit). A NanoDrop One spectrophotometer was used to measure the DNA concentration and purity (Thermo Fisher Scientific, MA, United States). The V4 regions of the bacterial 16S rRNA genes were amplified (50 μL reaction volume) by thermocycling: 5 min at 94°C for initialization; 30 cycles of 30 s denaturation at 94°C, 30 s annealing at 52°C, and 30 s extension at 72°C, followed by 10 min final elongation at 72°C using the primer sets 515F (5’-GTGCCAGCMGCCGCGGTAA-3’) and 806R (5’-GGACTACNNGGGTATCTAAT-3’) with barcodes (Parada et al., 2015). One pooled sample was combined through an equimolar amount of each PCR product from each sample and sequenced using an Illumina HiSeq 2500 platform to generate 250 bp paired-end reads (Guangdong Magigene Biotechnology Co., Ltd., Guangzhou, China).
Sequenced paired-end reads were processed using USEARCH (Version 11) (Edgar, 2010). The paired reads were merged with the fastq_mergepairs function and filtered with a “maxee” value of 1.0 (Edgar, 2010). The unique sequence reads were obtained with the fastx_uniques function and then denoised using the UNOISE3 (unoise_alpha = 2 and minsize = 4 as per default settings) algorithm to correct errors and remove chimeras (Edgar, 2016). This generated a list of zero-radius operational taxonomic units (ZOTUs). A ZOTU abundance table was produced by mapping the total reads to the list of ZOTUs with the -otutab function with an identity threshold of 0.97. The representative sequences for each ZOTU were assigned to taxonomic groups using the RDP classifier within the SILVA database (release 138) clustered at 99% similarity. Raw sequences have been deposited in the NCBI Sequence Read Archive (SRA) database under the BioProject number PRJNA604077 and the accession number SRP247264. Functional profiles of the bacterial community were annotated using FAPROTAX 1.2.2 using python collapse_table.py with the rarefied ZOTU table (Louca et al., 2016).
All statistical analyses were performed in the R software environment (version 3.5.2) unless otherwise noted. To improve the normality and homoscedasticity of the data, all of the biotic data were transformed by the Hellinger transformation, and the abiotic data were normalized by a chord transformation using the decostand function (Legendre and Gallagher, 2001). The Bray–Curtis dissimilarity matrix for bacterioplankton communities was generated to identify the relationships among samples with the vegdist function. Principal coordinate analysis (PCoA) and cluster analysis were used to visualize the overall structure of the bacterioplankton communities with the hclust (method = “ward.D”) function. Permutational multivariate analysis of variance (PERMANOVA) was performed to quantitatively evaluate the contributions of seasons, latitude, and offshore distances to the variation in community structure with the adonis function. Analysis of similarity (ANOSIM) was performed to test whether there was a statistically significant difference in the community composition between the two seasons with the anosim function from the vegan package. To acquire the biomarker functions for each season, we used the randomForest (importance = TRUE, proximity = TRUE) function to generate a classification model for November and April. The biomarker functions were identified by the most important season-discriminating features using tenfold cross-validation implemented with the rfcv function (Zhang et al., 2019).
The quantification of the effects of ecological processes on bacterioplankton community assembly was performed according to Stegen et al. (2013) based on the turnover in the phylogenetic community composition and species composition. First, the observed nearest-phylogenetic-neighbor distance (βMNTDobs) as well as the null expectation of the mean nearest-phylogenetic-neighbor distance (βMNTDexp) were used to calculate β-nearest taxon index (βNTI) metrics, which quantify the phylogenetic turnover between communities (Wang et al., 2019). The relative contributions of heterogeneous and homogeneous selection were estimated as the fractions of pairwise βNTI values that were >+2 and <-2, respectively (Stegen et al., 2015). Subsequently, the outcome of the βNTI analyses in combination with a second null model referred to as a Bray–Curtis-based Raup–Crick (RCbray) (Chase et al., 2011) model was used to estimate the relative contributions of stochastic processes (Stegen et al., 2013). Specifically, a fraction of pairwise |βNTI| <2 but RCbray <-0.95 or >+0.95 indicated the relative contributions of homogenizing dispersal or dispersal limitation, respectively; a fraction of pairwise |βNTI| <2 and |RCbray| <0.95 indicated that the community assembly was not dominated by any single process (Stegen et al., 2015).
Linear regressions were performed on Bray–Curtis dissimilarity (dependent variable) versus geographical distance (independent variable) through distance-decay analysis to quantify the distance decay in the bacterioplankton community (Wang et al., 2015). The relative contributions of environmental and spatial variables were evaluated by variation partitioning analysis (VPA). First, the spatial variables were derived from geographic coordinates using both linear trend and principal coordinates of neighbor matrices (PCNM) procedures (Griffith and Peres-Neto, 2006) to capture all spatial scales with the PCNM function. Second, the environmental, trend, and PCNM variables that explained a significant (P < 0.05) amount of variation in bacterioplankton community composition were selected by a forward selection model with the forward.sel function (Blanchet et al., 2008). The significance of the selected variables was assessed with 1000 permutations by using the rda and anova.cca functions from the vegan package. Finally, variance partitioning was performed using the varpart function from the vegan package on the Hellinger-transformed bacterial data, the forward-selected environmental variables, and the forward-selected spatial variables, and the data were tested for significance with 999 permutations (Meot et al., 1998). Pairwise comparisons of environmental variables were performed using the corrplot function from the corrplot package. Partial Mantel correlations between environmental variables and bacterioplankton community composition controlled for geographic distance were computed using the mantel.partial function from the corrplot package.
The bacterioplankton communities were mainly composed by Gammaproteobacteria (mean relative abundance, 24.5%), Alphaproteobacteria (23.7%), Actinobacteria (18.1%), Bacteroidetes (11.9%), and Cyanobacteria (9.9%) during the overwintering period, but the composition of phyla/classes between the two seasons was significantly different [ANOSIM, R = 0.148, p = 0.003] (Figure 2). Similarly, the functional composition of bacterioplankton communities between two seasons was significantly different [ANOSIM, R = 0.321, p = 0.001] although they were all dominant by chemoheterotrophy (mean relative abundance, 24.7%), aerobic chemoheterotrophy (22.1%), phototrophy (6.13%), photoautotrophy (6.04%), cyanobacteria (6.00%), and oxygenic photoautotrophy (6.00%) (Figure 2). Additionally, we observed that the relative SD of functional variability was significantly lower than those of variability at different taxonomic levels (Figure 3), indicating high taxonomic variability (even at phylum level) accompanied by relatively stable functional distributions in the overwintering ground.
Figure 2. Spatial and temporal variation of taxonomy and function of surface bacterioplankton communities in the overwintering ground. (A) Phylum-level (phylum Proteobacteria is grouped into the class level) taxonomic compositions of all samples. (B) Functional characterization of all samples.
Figure 3. The relative SD of bacterioplankton taxonomic and functional variability in the overwintering ground. Different lowercase letters denote significant differences (p < 0.05) among levels based on one-way ANOVA.
To visualize the overall taxonomic and functional structure of bacterioplankton communities and their underlying driving forces, we used PCoA, Cluster in combination with PERMANOVA on Bray–Curtis dissimilarities among the samples. We found that samples in the overwintering ground display a temporal pattern of divergence along the first principal coordinate (Figures 4A,B), and this divergence was further corroborated by Cluster, where the communities from November and April formed separate clusters (Figures 4C,D). PERMANOVA of pairwise dissimilarities between bacterioplankton communities indicated that seasonal compartmentalization comprises the largest source of variation (Table 1). Although the latitude and offshore distances also showed significant effects (Table 1), the community structure did not exhibit a regular or obvious spatial pattern (Figures 4A,B).
Figure 4. The spatial and temporal patterns in the structure of bacterioplankton taxa and function. Principal coordinates analysis (PCoA) of the bacterioplankton taxa (A) and function (B) derived from the Bray–Curtis dissimilarity matrix. Hierarchical clustering of samples based on the bacterioplankton community profiles at the OTU level (C) and function (D). The ovals denote the 95% confidence ellipse.
Table 1. Quantitative effects of seasons, latitude, and offshore distances on variation in community structure (taxonomic and functional) using non-parametric permutational multivariate analysis of variance (PERMANOVA).
In view of the functional differences of the bacterioplankton community between seasons, we further explored the specific functional groups and functional genera for November and April. A model based on the random forest machine-learning algorithm was established to evaluate the importance of indicator bacterial functions. Ultimately, five bacterial functions were defined as biomarkers when considering the minimum cross-validation error of the model (Figure 5A). Of these, four bacterial functions showed higher relative abundance in April than November, and only one bacterial function showed higher relative abundance in November than April (one-way ANOVA, p < 0.001) (Figure 5A). Furthermore, we observed that the dynamics of bacterial genera that drive the biomarker functions showed significant temporal divergence (Figure 5B). For example, the genus Acinetobacter and Moraxella were abundant in the bacterioplankton communities collected in November, and the genus Rhodococcus and Stenotrophomonas were abundant in the bacterioplankton communities collected in April (Figure 5B).
Figure 5. Specific bacterial functions of two seasons. (A) Biomarker functions with higher relative abundance in two seasons. The eight biomarker functions were identified by applying the random-forest classification of the relative abundance of the bacterioplankton communities against November and April. Data bars represent means. Error bars represent SEM. (B) The spatial and temporal dynamics of bacterioplankton genera that drive the biomarker functions.
To explore the underlying driving forces of bacterioplankton community variation, we used the mean nearest taxon distance (MNTD) to quantify the relative contributions of major ecological processes that regulate the community assembly. The ecological process dominating the community assembly was dispersal limitation (accounting for 67.0–79.7%), which was relatively stable over the seasons (Figure 6). Second, 20.3–31.0% of the variation in community composition was primarily due to heterogeneous selection (Figure 6). In addition, there was still 0–3.7% of compositional variation unexplained by any ecological processes (Figure 6). These results indicate that stochastic rather than deterministic processes structured the bacterioplankton community assembly in the overwintering ground.
Figure 6. Summary of ecological processes governing the compositional variation of total bacterioplankton communities and the bacterioplankton communities collected in November and April. The percentages are given as the relative contribution of each process to the community variation as indicated by different colors.
The spatial dissimilarities in bacterioplankton taxonomic composition were significantly higher than that in functional composition (Figure 7B). Furthermore, the taxonomic composition of bacterioplankton communities collected in both November (adjusted R2 = 0.139, p < 0.001) and April (adjusted R2 = 0.040, p < 0.001) exhibit a clear distance-decay pattern in similarities (Figure 7A). This pattern was also observed in the bacterial functional composition in November (adjusted R2 = 0.142, p < 0.001) but not in April (adjusted R2 = -0.001, p = 0.395) (Figure 7A).
Figure 7. Distance-decay of similarities in taxonomy and function of bacterioplankton communities in different seasons. (A) Corrections between Bray–Curtis dissimilarities with geographic distance between sites. (B) The spatial dissimilarities in bacterioplankton taxonomic composition and functional composition.
To explore the deterministic mechanisms underlying community assembly, we used VPA for disentangling the relative role of environmental and spatial variables. Results of the variation partitioning showed that the drivers of bacterioplankton community composition were different between seasons and between taxonomic and functional levels (Figure 8). Specifically, the environment-related variables (pure environmental variables, environmental-trend covariation, environmental-PCNM covariation, covariation of all variables) dominated the variation of bacterioplankton taxonomic composition collected in all seasons (both November and April) and November (only November) and the variation of bacterioplankton functional composition collected in April (only April), while space-related variables (pure linear trend, pure PCNM, environmental-trend covariation, environmental-PCNM covariation, trend-PCNM covariation, covariation of all variables) dominated the variation of bacterioplankton taxonomic composition collected in April and the variation of bacterioplankton functional composition collected in all seasons and November (Figure 8). Remarkably, there was a large proportion (53.4–76.7%) of variation unexplained by the above variables. To further identify the geographic-independent environmental drivers, we correlated distance-corrected dissimilarities of taxonomic and functional community composition with those of environmental variables (Figure 9). Overall, nutrients were the strongest correlates of both taxonomic and functional composition in both seasons. Moreover, significant correlations were found between the temporal differences in community composition (between the seasons) and various environmental variables (Figure 9A), and the spatial differences in community composition (within the season) were weakly driven by environmental variables (Figures 9B,C).
Figure 8. Variation partitioning of the taxonomic and functional structure of total bacterioplankton communities (all) and the bacterioplankton communities collected in November (nov) and April (apr) by the seawater environmental variables (Env) and the spatial variables including linear trend (Trend) and PCNM variables (PCNM).
Figure 9. Environmental drivers of taxonomic and functional composition of total bacterioplankton communities (A) and the bacterioplankton communities collected in November (B) and April (C). Pairwise comparisons of environmental variables are shown with a color gradient denoting Spearman’s correlation coefficients. The taxonomic and functional composition of bacterioplankton communities was related to each environment variable by partial (geographic distance-corrected) Mantel tests. Edge width corresponds to Mantel’s r statistic for the corresponding distance correlations, and edge color denotes the statistical significance based on 9999 permutations. WT, water temperature; SAL, salinity; PO4, orthophosphate; NO2, nitrite; NO3, nitrate; NH4, ammonium; SiO3, silicate; TN, total nitrogen; TP, total phosphorus.
The habitats of the large yellow croaker are being degraded due to the increased use of coastal areas for aquaculture operations and development (Kang et al., 2018). The failed recovery of the common snook (Centropomus undecimalis) indicates that habitat degradation is considered more significant than exploitation in explaining population declines (Brennan et al., 2008), which indicates the need for the study and restoration of croaker habitat. Planktonic bacteria are generally used as a bioindicator to systematically reflect or record the conditions of the marine environment or fish habitats due to their biogeochemical significance and direct relationship with fish health (Dowle et al., 2015; Dai et al., 2017). Over the past decade, dozens of studies have characterized patterns, associations, and drivers in the bacterioplankton community in various fish habitats and elucidated similarities and differences in the bacterioplankton communities between fish farms and natural areas (Tamminen et al., 2011; Xiong et al., 2015; Jing et al., 2019). However, comprehensive information on the spatial and temporal patterns of bacterioplankton communities in the croaker habitat is scarce.
Our study systematically investigated the surface bacterioplankton communities in an overwintering ground for large yellow croakers. We found that, during the overwintering period, the dominant bacterioplankton phyla and functions remained unchanged, but their relative abundance exhibited seasonality (Figures 2, 4 and Table 1). Specifically, the bacterioplankton community in autumn was characterized by animal parasites or symbionts (Figure 5A), and Acinetobacter was the representative genus (Figure 5B). Acinetobacter baumannii, which was detected in our samples, is a virulent pathogen for many fish species that can trigger serious septicemia outbreaks (Brahmi et al., 2016; Li et al., 2017). More seriously, A. baumannii has been proven to be a serious human clinical pathogen involved in a large number of infections, including bacteremia, secondary meningitis, urinary tract infection, and ventilator-associated pneumonia (Mari-Almiralla et al., 2019). Therefore, the presence of Acinetobacter in overwintering grounds increases the latent risk of fish disease and the possibility of coinfection between fish and humans through handling or consumption (Xia et al., 2008). In addition, other functional genera found in autumn, such as Moraxella (Figure 5B), are opportunistic pathogens of fish (Walke et al., 2015). Once the environment deteriorates or suddenly changes, those bacteria might threaten the health of the croaker or other fish. In spring, many nutrient-related functional bacterioplankton were dominant (Figure 5). Rhodococcus, one of the dominant functional genera in April (Figure 5B), is generally regarded as the most versatile and efficient group of organisms that are suitable for the biodegradation of organic compounds (Larkin et al., 2005; Martínková et al., 2009). Given this characteristic, Rhodococcus has been found worldwide in eutrophic aquatic ecosystems (Wu et al., 2009; Lee et al., 2010), suggesting that it might be used as an organismal indicator of nutrient enrichment (Rowbotham and Cross, 1977). Moreover, Alcanivorax (Figure 5B), a hydrocarbon-degrading bacterium, could also be enriched in eutrophic environments and could be related to the presence of pollutants (Patel et al., 2014). Other functional genera in spring, such as Stenotrophomonas (Jia et al., 2019) and Neptunomonas (Lanfranconi et al., 2010), were also organic-degrading or nitrogen-related organisms that prefer organic-rich or eutrophic conditions. Therefore, the functional composition of the bacterioplankton community in April suggests that the nutrient level in the surface water of the habitat in spring was higher than that in the winter, which was verified by environmental data (Supplementary Table S1) and implies that this sea area has a high risk of developing eutrophication.
One key question in ocean microbial ecology is the extent to which environmental filtering and various biological interactions, on the one hand, and limited dispersal and historical contingency, on the other, are responsible for spatiotemporal patterns (Sunagawa et al., 2015). Nevertheless, the answer to this question in marine ecosystems is still controversial. For example, some studies have proposed that marine bacterioplankton communities are structured by deterministic factors (Pontarp et al., 2012; Sunagawa et al., 2015; Wang et al., 2019), and other studies have demonstrated that stochastic factors can potentially overwhelm the influences of selection on community structure (Beaton et al., 2016; Evans et al., 2017; Zhou and Ning, 2017). The latter theory is supported by our findings as dispersal limitation dominated the processes governing both the spatial and temporal scales of bacterioplankton community assembly (Figure 6). It has historically been considered that free-living bacteria are small, numerous, and easily transported and, hence, do not experience passive dispersal limitations (Cottenie, 2005; Shurin et al., 2009). However, numerous studies have recently documented that bacterial communities in water show spatial differences and reveal strong biogeographic patterns (Verreydt et al., 2012; Müller et al., 2014; Wu et al., 2018). The distance-similarity decay relationships for the bacterioplankton communities observed in our study (Figure 7) also suggest the impact of dispersal limitation on the generation of biogeographical patterns in marine bacteria (Bell, 2010; Adams et al., 2013). It is interesting to note that, even though the study area is located in the East China Sea and the geographic scale of the overwintering ground we investigated is comparatively broad, the distance-decay patterns in our study were weaker than those in other studies as the distance decay rates (the regression slopes) of our bacterioplankton communities were lower (Wang et al., 2015; Dai et al., 2017). The strong distance-decay patterns in Wang et al. (2015) and Dai et al. (2017) were mainly attributed to spatially structured environmental gradients, which essentially suggests that the spatial heterogeneity of bacterioplankton communities in their studies was structured by the deterministic heterogeneous selection of abiotic and biotic environmental conditions (Dini-Andreote et al., 2015). However, stochastic dispersal exerted a dominant role in shaping our bacterioplankton communities (Figure 6), contributed to the relatively random distribution of the bacterial community compared with that under harsh environmental filters (Evans et al., 2017), and attenuated the heterogeneity of bacterial taxa in our study. Additionally, lower or no distance-similarity decay relationships were found in bacterial functions compared with the relationships found in bacterial taxa (Figure 7). This is a universal phenomenon because functional redundancy, which means that different populations share similar or the same functions, appears to be quite high in the microbial community, especially compared with the level of functional redundancy in plant or animal communities (Delgado-Baquerizo et al., 2016; Louca et al., 2018).
Comparatively, environmental stressors wielded less power than stochastic processes in creating temporal and spatial differences in the bacterioplankton communities’ taxonomical and functional composition in the overwintering habitat for croaker. Our variation partitioning results showed that over 50% of the variation in the bacterioplankton community structure was unexplained by the environment-related variables (Figure 8). The lower power of the environmental variables could be explained by the restraining force of stochastic effects (Zhou and Ning, 2017); dispersal limitation can obscure the relationships between environmental variables and microbial community composition, resulting in apparently weak deterministic effects (Evans et al., 2017).
Among the purely environmental filters that were independent of spatial effects, nutrient-related variables, such as silicate, nitrate, and ammonium, could have exhibited some degree of heterogeneous selection on temporal and spatial variations in the bacterioplankton community composition in the overwintering grounds (Figure 9). The mechanism by which silicate influences the composition of the bacterioplankton community could be related to its influence on diatom growth; it may shape bacterioplankton community composition due to the tight coupling between diatoms and bacteria (Topper et al., 2010; Wang et al., 2015). The correlations of bacterioplankton community composition with nitrogenous nutrients could be attributed to the abundant biodegrading bacteria in the habitat (Figure 5), which are capable of decomposing organic substances into various inorganic nutrients (Manser et al., 2006; Li and Boyd, 2016). An equally important driver of variation in the bacterioplankton community was temperature (Figure 9); this finding is in accordance with those reported in freshwater ecosystems, oceans, and forests at the global scale (Penuelas et al., 2012; Sunagawa et al., 2015; Chen C. Y. et al., 2019). The importance of salinity in shaping the composition of the bacterioplankton community was also observed (Figure 9). Salinity may contribute to density gradients that physically separate water masses and their resident bacterial communities from near-shore to offshore areas (Lozupone and Knight, 2007; Fortunato et al., 2012) (Supplementary Table S1). Notably, more correlations were found between the temporal differences in community composition and the various environmental variables than between spatial differences and environmental variables (Figure 9); this difference may have resulted from the greater differences in environmental variables between seasons. Interestingly, the interactions among community composition and purely environmental filters were more complex and closer in spring than in autumn (Figures 9B,C). One possible explanation for this difference is that the high eutrophication level in April (Supplementary Table S1), as a long-term and continuous disturbance, could increase the importance of deterministic environmental filtering to bacterioplankton community assembly (Figure 6) (Dai et al., 2017).
Overall, we obtained a unique taxonomic and functional profile of the bacterioplankton community in the surface water of the overwintering ground and revealed the assembly mechanisms that structure it on the spatial–temporal scale. The systematic map of the surface bacterioplankton community has implied that current conditions are not conducive to the high primary productivity and the health of upper elements of the croakers’ food chain. There are, however, potential limitations to our approach that merit further discussion. First, although the above results and discussion display the patterns and processes of bacterioplankton community assembly in the overwintering grounds for large yellow croaker, samples collected from the surface water can only somewhat reflect habitat conditions for the croaker who live at moderate and lower water depths (Lü et al., 2008). Therefore, we will further study the habitat microbiome of the water masses in which croaker is located. Additionally, we did not sample replicates at the study sites, which might have limited the analysis of the within-site variability and thereby might affect the accuracy of the results of the random forest classification and the distance decay analysis to a certain extent.
All the raw sequences have been uploaded to the NCBI Sequence Read Archive (SRA) database under the BioProject number PRJNA604077 and the accession number SRP247264.
WY and Z-MZ led manuscript writing and data analysis. S-ZZ, S-HZ, and BL contributed with sequence analysis, statistical analyses, and microbiological interpretations. LZ and RN collected and processed samples. J-YZ and C-HL were responsible for organizing field sampling and inter-institution communication. All authors contributed to manuscript revision, read, and approved the submitted version.
This research was supported by the National Key Research and Development Program of China (Reference No. 2018YFC1406300), the Natural Science Foundation of Zhejiang Province (Reference No. LQ20C190003), the Department of Education Scientific Research Project of Zhejiang Province (Reference No. Y201839309), Natural Science Foundation of Ningbo (Reference Nos. 2019A610421 and 2019A610443), and the K.C. Wong Magna Fund in Ningbo University.
The authors declare that the research was conducted in the absence of any commercial or financial relationships that could be construed as a potential conflict of interest.
The authors would like to thank the colleagues from Xiamen University, Second Institute of Oceanography, and other institutions for helping on board. In addition, the authors thank Prof. Ling-feng Huang and Prof. Jian-Yu Hu from Xiamen University for their selfless sharing of environmental data.
The Supplementary Material for this article can be found online at: https://www.frontiersin.org/articles/10.3389/fmars.2020.00472/full#supplementary-material
Adams, R. I., Miletto, M., Taylor, J. W., and Bruns, T. D. (2013). Dispersal in microbes: fungi in indoor air are dominated by outdoor air and show dispersal limitation at short distances. ISME J. 7, 1262–1273. doi: 10.1038/ismej.2013.28
AQSIQ (2007). The Specification for Marine Monitoring of China-Part 4: Seawater Analysis (GB 17378.4e2007). Beijing: General administration of quality supervision, inspection and quarantine.
Beaton, E. D., Stevenson, B. S., King-Sharp, K. J., Stamps, B. W., Nunn, H. S., and Stuart, M. (2016). Local and regional diversity reveals dispersal limitation and drift as drivers for groundwater bacterial communities from a fractured granite formation. Front. Microbiol. 7:1933. doi: 10.3389/fmicb.2016.01933
Bell, T. (2010). Experimental tests of the bacterial distance–decay relationship. ISME J. 4, 1357–1365. doi: 10.1038/ismej.2010.77
Blanchet, F. G., Legendre, P., and Borcard, D. (2008). Forward selection of explanatory variables. Ecology 89, 2623–2632. doi: 10.1890/07-0986.1
Brahmi, S., Touati, A., Cadiere, A., Djahmi, N., Pantel, A., Sotto, A., et al. (2016). First description of two sequence type 2 Acinetobacter baumannii isolates carrying OXA-23 carbapenemase in Pagellus acarne fished from the Mediterranean Sea near Bejaia, Algeria. Antimicrob. Agents Chemother. 60, 2513–2515. doi: 10.1128/Aac.02384-15
Brennan, N. P., Walters, C. J., and Leber, K. M. (2008). Manipulations of stocking magnitude: addressing density-dependence in a juvenile cohort of common snook (Centropomus undecimalis). Rev. Fish. Sci. 16, 215–227. doi: 10.1080/10641260701689022
Chase, J. M., Kraft, N. J. B., Smith, K. G., Vellend, M., and Inouye, B. D. (2011). Using null models to disentangle variation in community dissimilarity from variation in alpha-diversity. Ecosphere 2:24. doi: 10.1890/Es10-00117.1
Chen, C. Y., He, R. Q., Cheng, Z. Y., Han, M. Z., Zha, Y. G., Yang, P. S., et al. (2019). The seasonal dynamics and the influence of human activities on campus outdoor microbial communities. Front. Microbiol. 10:1579. doi: 10.3389/fmicb.2019.01579
Chen, J. J., and Xu, Z. L. (2012). Analysis of population division and geographical isolation of Larimichthys crocea in the East China Sea and Yellow Sea. J. Fish. China 19, 310–320. doi: 10.3724/sp.j.1118.2012.00310
Chen, W. D., Ren, K. X., Isabwe, A., Chen, H. H., Liu, M., and Yang, J. (2019). Stochastic processes shape microeukaryotic community assembly in a subtropical river across wet and dry seasons. Microbiome 7:138. doi: 10.1186/s40168-019-0749-8
Cottenie, K. (2005). Integrating environmental and spatial processes in ecological community dynamics. Ecol. Lett. 8, 1175–1182. doi: 10.1111/j.1461-0248.2005.00820.x
Dai, W. F., Zhang, J. J., Tu, Q. C., Deng, Y., Qiu, Q. F., and Xiong, J. B. (2017). Bacterioplankton assembly and interspecies interaction indicating increasing coastal eutrophication. Chemosphere 177, 317–325. doi: 10.1016/j.chemosphere.2017.03.034
Delgado-Baquerizo, M., Giaramida, L., Reich, P. B., Khachane, A. N., Hamonts, K., Edwards, C., et al. (2016). Lack of functional redundancy in the relationship between microbial diversity and ecosystem functioning. J. Ecol. 104, 936–946. doi: 10.1111/1365-2745.12585
Dini-Andreote, F., Stegen, J. C., van Elsas, J. D., and Salles, J. F. (2015). Disentangling mechanisms that mediate the balance between stochastic and deterministic processes in microbial succession. Proc. Natl. Acad. Sci. U.S.A. 112, E1326–E1332. doi: 10.1073/pnas.1414261112
Dowle, E., Pochon, X., Keeley, N., and Wood, S. A. (2015). Assessing the effects of salmon farming seabed enrichment using bacterial community diversity and high-throughput sequencing. FEMS Microbiol. Ecol. 91:fiv089. doi: 10.1093/femsec/fiv089
Edgar, R. C. (2010). Search and clustering orders of magnitude faster than BLAST. Bioinformatics 26, 2460–2461. doi: 10.1093/bioinformatics/btq461
Edgar, R. C. (2016). UNOISE2: improved error-correction for Illumina 16S and ITS amplicon sequencing. bioRxiv [Preprint]. doi: 10.1101/081257
Evans, S., Martiny, J. B. H., and Allison, S. D. (2017). Effects of dispersal and selection on stochastic assembly in microbial communities. ISME J. 11, 176–185. doi: 10.1038/ismej.2016.96
Fortunato, C. S., Herfort, L., Zuber, P., Baptista, A. M., and Crump, B. C. (2012). Spatial variability overwhelms seasonal patterns in bacterioplankton communities across a river to ocean gradient. ISME J. 6, 554–563. doi: 10.1038/ismej.2011.135
Giovannoni, S., and Nemergut, D. (2014). Microbes ride the current. Science 345, 1246–1247. doi: 10.1126/science.1259467
Griffith, D. A., and Peres-Neto, P. R. (2006). Spatial modeling in ecology: the flexibility of eigenfunction spatial analyses. Ecology 87, 2603–2613. doi: 10.1890/0012-9658(2006)87[2603:smietf]2.0.co;2
Hu, X. J., Wen, G. L., Cao, Y. C., Gong, Y. X., Li, Z. J., He, Z. L., et al. (2017). Metabolic and phylogenetic profiles of microbial communities from a mariculture base on the Chinese Guangdong coast. Fish. Sci. 83, 465–477. doi: 10.1007/s12562-017-1073-5
Jia, Y., Zhou, M., Chen, Y., Lou, J., and Hu, Y. (2019). Carbon selection for nitrogen degradation pathway by Stenotrophomonas maltophilia: based on the balances of nitrogen, carbon and electron. Bioresour. Technol. 294:122114. doi: 10.1016/j.biortech.2019.122114
Jiao, S., Xu, Y., Zhang, J., and Lu, Y. (2019). Environmental filtering drives distinct continental atlases of soil archaea between dryland and wetland agricultural ecosystems. Microbiome 7:15. doi: 10.1186/s40168-019-0630-9
Jing, X. Y., Gou, H. L., Gong, Y. H., Ji, Y. T., Su, X. L., Zhang, J., et al. (2019). Seasonal dynamics of the coastal bacterioplankton at intensive fish-farming areas of the Yellow Sea, China revealed by high-throughput sequencing. Mar. Pollut. Bull. 139, 366–375. doi: 10.1016/j.marpolbul.2018.12.052
Kalcheva, H. V., Nikolova, L. N., Terziyski, D. I., Stoeva, A. S., Pehlivanov, L. Z., and Kalchev, R. K. (2008). Relationships between bacterioplankton, zooplankton and environmental factors in fertilized and non-fertilized carp fishponds. Acta Zool. Bulgarica Suppl. 2, 175–184.
Kang, B., Liu, M., Huang, X. X., Li, J., Yan, Y. R., Han, C. C., et al. (2018). Fisheries in Chinese seas: what can we learn from controversial official fisheries statistics? Rev. Fish Biol. Fish. 28, 503–519. doi: 10.1007/s11160-018-9518-1
Keen, M. R., Schwarz, A. M., and Wini-Simeon, L. (2018). Towards defining the Blue Economy: practical lessons from pacific ocean governance. Mar. Policy 88, 333–341. doi: 10.1016/j.marpol.2017.03.002
Lanfranconi, M. P., Bosch, R., and Nogales, B. (2010). Short-term changes in the composition of active marine bacterial assemblages in response to diesel oil pollution. Microb. Biotechnol. 3, 607–621. doi: 10.1111/j.1751-7915.2010.00192.x
Larkin, M. J., Kulakov, L. A., and Allen, C. C. R. (2005). Biodegradation and Rhodococcus – masters of catabolic versatility. Curr. Opin. Biotechnol. 16, 282–290. doi: 10.1016/j.copbio.2005.04.007
Lee, Y. K., Ahn, C. Y., Kim, H. S., and Oh, H. M. (2010). Cyanobactericidal effect of Rhodococcus sp isolated from eutrophic lake on Microcystis sp. Biotechnol. Lett. 32, 1673–1678. doi: 10.1007/s10529-010-0350-5
Legendre, P., and Gallagher, E. D. (2001). Ecologically meaningful transformations for ordination of species data. Oecologia 129, 271–280. doi: 10.1007/s004420100716
Li, J., Cao, J. L., Wang, X., Liu, N., Wang, W. M., and Luo, Y. (2017). Acinetobacter pittii, an emerging new multi-drug resistant fish pathogen isolated from diseased blunt snout bream (Megalobrama amblycephala Yih) in China. Appl. Microbiol. Biotechnol. 101, 6459–6471. doi: 10.1007/s00253-017-8392-4
Li, Y. L., and Boyd, C. E. (2016). Laboratory tests of bacterial amendments for accelerating oxidation rates of ammonia, nitrite and organic matter in aquaculture pond water. Aquaculture 460, 45–58. doi: 10.1016/j.aquaculture.2016.03.050
Liu, C., Shen, W. L., Hou, C. C., Gao, X. M., Wang, Q. F., Wu, X. F., et al. (2019). Low temperature-induced variation in plasma biochemical indices and aquaglyceroporin gene expression in the large yellow croaker Larimichthys crocea. Sci. Rep. 9:2717. doi: 10.1038/s41598-018-37274-3
Liu, M., and de Mitcheson, Y. S. (2008). Profile of a fishery collapse: why mariculture failed to save the large yellow croaker. Fish Fish. 9, 219–242. doi: 10.1111/j.1467-2979.2008.00278.x
Louca, S., Parfrey, L. W., and Doebeli, M. (2016). Decoupling function and taxonomy in the global ocean microbiome. Science 353, 1272–1277. doi: 10.1126/science.aaf4507
Louca, S., Polz, M. F., Mazel, F., Albright, M. B. N., Huber, J. A., O’Connor, M. I., et al. (2018). Function and functional redundancy in microbial systems. Nat. Ecol. Evol. 2, 936–943. doi: 10.1038/s41559-018-0519-1
Lozupone, C. A., and Knight, R. (2007). Global patterns in bacterial diversity. Proc. Natl. Acad. Sci. U.S.A. 104, 11436–11440. doi: 10.1073/pnas.0611525104
Lü, H., Xu, J., and Vander Haegen, G. (2008). Supplementing marine capture fisheries in the East China Sea: sea ranching of Prawn Penaeus orientalis, restocking of Large Yellow Croaker Pseudosciaena crocea, and cage culture. Rev. Fish. Sci. 16, 366–276. doi: 10.1080/10641260701678207
Manser, R., Gujer, W., and Siegrist, H. (2006). Decay processes of nitrifying bacteria in biological wastewater treatment systems. Water Res. 40, 2416–2426. doi: 10.1016/j.watres.2006.04.019
Mari-Almiralla, M., Cosgaya, C., Pons, M. J., Nemec, A., Ochoa, T. J., Ruiz, J., et al. (2019). Pathogenic Acinetobacter species including the novel Acinetobacter dijkshoorniae recovered from market meat in Peru. Int. J. Food Microbiol. 305:108248. doi: 10.1016/j.ijfoodmicro.2019.108248
Martínková, L., Uhnáková, B., Pátek, M., Nešvera, J., and Křen, V. (2009). Biodegradation potential of the genus Rhodococcus. Environ. Int. 35, 162–177. doi: 10.1016/j.envint.2008.07.018
Meot, A., Legendre, P., and Borcard, D. (1998). Partialling out the spatial component of ecological variation: questions and propositions in the linear modelling framework. Environ. Ecol. Stat. 5, 1–27. doi: 10.1023/A:1009693501830
Müller, A. L., de Rezende, J. R., Hubert, C. R. J., Kjeldsen, K. U., Lagkouvardos, I., Berry, D., et al. (2014). Endospores of thermophilic bacteria as tracers of microbial dispersal by ocean currents. ISME J. 8, 1153–1165. doi: 10.1038/ismej.2013.225
Parada, A. E., Needham, D. M., and Fuhrman, J. A. (2015). Every base matters: assessing small subunit rRNA primers for marine microbiomes with mock communities, time series and global field samples. Environ. Microbiol. 18, 1403–1414. doi: 10.1111/1462-2920.13023
Patel, V., Munot, H., Shouche, Y. S., and Madamwar, D. (2014). Response of bacterial community structure to seasonal fluctuation and anthropogenic pollution on coastal water of Alang–Sosiya ship breaking yard, Bhavnagar, India. Bioresour. Technol. 161, 362–370. doi: 10.1016/j.biortech.2014.03.033
Penuelas, J., Rico, L., Ogaya, R., Jump, A. S., and Terradas, J. (2012). Summer season and long-term drought increase the richness of bacteria and fungi in the foliar phyllosphere of Quercus ilex in a mixed Mediterranean forest. Plant Biol. 14, 565–575. doi: 10.1111/j.1438-8677.2011.00532.x
Pontarp, M., Canback, B., Tunlid, A., and Lundberg, P. (2012). Phylogenetic analysis suggests that habitat filtering is structuring marine bacterial communities across the globe. Microb. Ecol. 64, 8–17. doi: 10.1007/s00248-011-0005-7
Rowbotham, T. J., and Cross, T. (1977). Ecology of rhodococcus coprophilus and associated actinomycetes in fresh water and agricultural habitats. J. Gen. Microbiol. 100, 231–240. doi: 10.1099/00221287-100-2-231
Shurin, J. B., Cottenie, K., and Hillebrand, H. (2009). Spatial autocorrelation and dispersal limitation in freshwater organisms. Oecologia 159, 151–159. doi: 10.1007/s00442-008-1174-z
Stegen, J. C., Lin, X. J., Fredrickson, J. K., Chen, X. Y., Kennedy, D. W., Murray, C. J., et al. (2013). Quantifying community assembly processes and identifying features that impose them. ISME J. 7, 2069–2079. doi: 10.1038/ismej.2013.93
Stegen, J. C., Lin, X. J., Fredrickson, J. K., and Konopka, A. E. (2015). Estimating and mapping ecological processes influencing microbial community assembly. Front. Microbiol. 6:370. doi: 10.3389/fmicb.2015.00370
Sunagawa, S., Coelho, L. P., Chaffron, S., Kultima, J. R., Labadie, K., Salazar, G., et al. (2015). Structure and function of the global ocean microbiome. Science 348:1261359. doi: 10.1126/science.1261359
Tamminen, M., Karkman, A., Corander, J., Paulin, L., and Virta, M. (2011). Differences in bacterial community composition in Baltic Sea sediment in response to fish farming. Aquaculture 313, 15–23. doi: 10.1016/j.aquaculture.2011.01.020
Topper, B., Larsen, A., Thingstad, T. F., Thyrhaug, R., and Sandaa, R. A. (2010). Bacterial community composition in an Arctic phytoplankton mesocosm bloom: the impact of silicate and glucose. Polar Biol. 33, 1557–1565. doi: 10.1007/s00300-010-0846-4
Tursi, A., Maiorano, P., Sion, L., and D’Onghia, G. (2015). Fishery resources: between ecology and economy. Rendiconti Lincei Sci. Fisiche E Nat. 26, 73–79. doi: 10.1007/s12210-014-0372-3
Verreydt, D., De Meester, L., Decaestecker, E., Villena, M.-J., Van Der Gucht, K., Vannormelingen, P., et al. (2012). Dispersal-mediated trophic interactions can generate apparent patterns of dispersal limitation in aquatic metacommunities. Ecol. Lett. 15, 218–226. doi: 10.1111/j.1461-0248.2011.01728.x
Walke, S., Kashte, S., Parwe, N., and Chavan, S. (2015). Opportunistic pathogens in the mucous of skin, gills, fins and mouth of Labeo Rohita. Int. J. Fish. Aquat. Stud. 3, 169–172.
Wang, K., Hu, H. J., Yan, H. Z., Hou, D. D., Wang, Y. T., Dong, P. S., et al. (2019). Archaeal biogeography and interactions with microbial community across complex subtropical coastal waters. Mol. Ecol. 28, 3101–3118. doi: 10.1111/mec.15105
Wang, K., Ye, X. S., Chen, H. P., Zhao, Q. F., Hu, C. J., He, J. Y., et al. (2015). Bacterial biogeography in the coastal waters of northern Zhejiang, East China Sea is highly controlled by spatially structured environmental gradients. Environ. Microbiol. 17, 3898–3913. doi: 10.1111/1462-2920.12884
Wang, L., Shi, X. F., Su, Y. Q., Meng, Z. N., and Lin, H. R. (2012). Loss of genetic diversity in the cultured stocks of the Large Yellow Croaker, Larimichthys crocea, revealed by microsatellites. Int. J. Mol. Sci. 13, 5584–5597. doi: 10.3390/ijms13055584
Wu, G. F., Liu, J., and Ye, Z. X. (2009). Characterization of phosphobacteria isolated from eutrophic aquatic ecosystems. Microbiology 78, 769–775. doi: 10.1134/S0026261709060149
Wu, W. X., Lu, H. P., Sastri, A., Yeh, Y. C., Gong, G. C., Chou, W. C., et al. (2018). Contrasting the relative importance of species sorting and dispersal limitation in shaping marine bacterial versus protist communities. ISME J. 12, 485–494. doi: 10.1038/ismej.2017.183
Xia, L., Xiong, D. M., Gu, Z. M., Xu, Z., Chen, C. F., Xie, J., et al. (2008). Recovery of Acinetobacter baumannii from diseased channel catfish (Ictalurus punctatus) in China. Aquaculture 284, 285–288. doi: 10.1016/j.aquaculture.2008.07.038
Xiong, J. B., Chen, H. P., Hu, C. J., Ye, X. S., Kong, D. J., and Zhang, D. M. (2015). Evidence of bacterioplankton community adaptation in response to long-term mariculture disturbance. Sci. Rep. 5:15274. doi: 10.1038/srep15274
Xu, Z. L., and Chen, J. J. (2011). Analysis of migratory route of Larimichthys crocea in the East China Sea and Yellow Sea. J. Fish. China 35, 429–437.
Yan, D. B., Xia, P. H., Song, X., Lin, T., and Cao, H. P. (2019). Community structure and functional diversity of epiphytic bacteria and planktonic bacteria on submerged macrophytes in Caohai Lake, southwest of China. Ann. Microbiol. 69, 933–944. doi: 10.1007/s13213-019-01485-4
Yang, B. Y., Wang, C. C., Hu, H. H., Tu, Y. Q., Han, D., Zhu, X. M., et al. (2015). Repeated handling compromises the immune suppression and improves the disease resistance in overwintering channel catfish (Ictalurus punctatus). Fish Shellfish Immunol. 47, 418–428. doi: 10.1016/j.fsi.2015.09.010
Yang, W., Zhu, J., Zheng, C., Qiu, H., Zheng, Z., and Lu, K. (2018). Succession of bacterioplankton community in intensive shrimp (Litopenaeus vannamei) aquaculture systems. Aquaculture 497, 200–213. doi: 10.1016/j.aquaculture.2018.07.053
Zhang, J. Y., Liu, Y. X., Zhang, N., Hu, B., Jin, T., Xu, H. R., et al. (2019). NRT1.1B is associated with root microbiota composition and nitrogen use in field-grown rice. Nat. Biotechnol. 37, 676–684. doi: 10.1038/s41587-019-0104-4
Zhou, J., and Ning, D. (2017). Stochastic community assembly: does it matter in microbial ecology? Microbiol. Mol. Biol. Rev. 81:e00002-17. doi: 10.1128/MMBR.00002-17
Keywords: large yellow croaker, surface water, planktonic bacteria, community assembly, dispersal limitation, FAPROTAX
Citation: Yang W, Zheng S-Z, Zhou S-H, Zhao L, Zhu J-Y, Lukwambe B, Nicholaus R, Li C-H and Zheng Z-M (2020) Structure and Functional Diversity of Surface Bacterioplankton Communities in an Overwintering Habitat for Large Yellow Croaker, Pseudosciaena crocea, of the Southern East China Sea. Front. Mar. Sci. 7:472. doi: 10.3389/fmars.2020.00472
Received: 27 January 2020; Accepted: 27 May 2020;
Published: 30 June 2020.
Edited by:
Karoline Faust, KU Leuven, BelgiumReviewed by:
Bernd Wemheuer, University of New South Wales, AustraliaCopyright © 2020 Yang, Zheng, Zhou, Zhao, Zhu, Lukwambe, Nicholaus, Li and Zheng. This is an open-access article distributed under the terms of the Creative Commons Attribution License (CC BY). The use, distribution or reproduction in other forums is permitted, provided the original author(s) and the copyright owner(s) are credited and that the original publication in this journal is cited, in accordance with accepted academic practice. No use, distribution or reproduction is permitted which does not comply with these terms.
*Correspondence: Zhong-Ming Zheng, emhlbmd6aG9uZ21pbmdAbmJ1LmVkdS5jbg==
Disclaimer: All claims expressed in this article are solely those of the authors and do not necessarily represent those of their affiliated organizations, or those of the publisher, the editors and the reviewers. Any product that may be evaluated in this article or claim that may be made by its manufacturer is not guaranteed or endorsed by the publisher.
Research integrity at Frontiers
Learn more about the work of our research integrity team to safeguard the quality of each article we publish.