- Division of Marine Science and Conservation, Nicholas School of the Environment, Duke University Marine Lab, Beaufort, NC, United States
Assessing, implementing and monitoring ecosystem restoration can be a labor intensive process, often short term (<3 years), and potentially destructive to the habitat. Advances in remote sensing technology are generating rapid, non-destructive methods for siting, executing and monitoring restoration efforts, particularly in fragile marine environments. Unoccupied aircraft systems (UAS), or drones, are a highly flexible method for accessing and remote sensing ecosystems with on-demand capabilities, greater resolution than sensors from satellites and occupied aircraft, and the ability to cover large areas quickly. With the variety of platforms and payloads available, UASs are providing a suite of tools for conservation practitioners to properly plan marine ecosystem restoration projects and evaluate their success. Both conventional and specialized sensors coupled with image processing techniques can be used to gauge impact to and recovery of entire ecological communities. For example, high-resolution, multispectral imaging allows for discernment of population changes across trophic levels, concurrent with the discrimination of species (including rare) across a landscape, and detection of vegetation stress. Structure from Motion photogrammetric processing provides centimeter-scale three-dimensional models of habitat structure to measure ecologically significant aspects like rugosity and assess their change through time. Water quality around a broad impacted area can be remotely monitored via a number of payloads before and after restoration. Additionally, specially designed payloads can be used to manually disperse seeds or materials for restoration applications without disturbing the habitat. UASs have increasing potential to reduce the costs (both time and money) associated with restoration efforts, making site assessment and long-term, broad-scale monitoring more achievable. Here we present a review of the applications of UASs in marine ecosystem restoration with an overview of the special considerations of using this technology in the marine environment.
Introduction
Much of the world’s coastal and marine habitats are threatened by climatic changes and anthropogenic modifications (Adam, 2002; Halpern et al., 2008), driving an increased focus on marine ecological conservation and restoration over the past several decades. The biogenic habitats that exist across the margins of our coastal seas (e.g., coral reefs, seagrass, saltmarsh, oyster reefs, mangroves, etc.) provide numerous benefits to the surrounding environment (Barbier et al., 2011). As the quantity and quality of these habitats have declined in the last few centuries, we are now struggling to mitigate losses in the ecosystem services they can provide (Halpern et al., 2008). Restoration of marine and coastal habitats is now occurring on many scales, spatially and temporally, and these efforts consist of a series of multifaceted stages of planning, implementation, and ultimately monitoring restored sites to determine how they respond over time. Having adequate information to guide the stages of restoration projects is essential, and accessing efficient and affordable tools to implement necessary actions during each of these phases will help ensure the success of marine ecosystem restoration (MER) projects.
Remote sensing of environments for assessment purposes, most often from orbiting satellites or occupied aircraft, has been rapidly developing in the last several decades. Remote sensing methods offer a way to monitor fragile environments, like biogenic coastal habitats, with minimal to no disturbance (Shuman and Ambrose, 2003). This is an important consideration for restoring delicate ecosystems when long term monitoring practices can have potentially lasting negative impacts on a habitat through impacts such as trampling (Goldman Martone and Wasson, 2008) (Figure 1). Traditional remote sensing methods (satellite and occupied aircraft operations) are increasingly powerful for environmental monitoring but have certain limitations including prohibitive costs, inadequate temporal resolution or timing of sensing, and insufficient spatial resolution of the data (Turner et al., 2015; Windle et al., 2019). That being said, advances in satellite sensor and data processing technologies have vastly expanded the types and quality of data products that can be generated through remote sensing while also promising increased affordability and immediacy (Pettorelli et al., 2014).
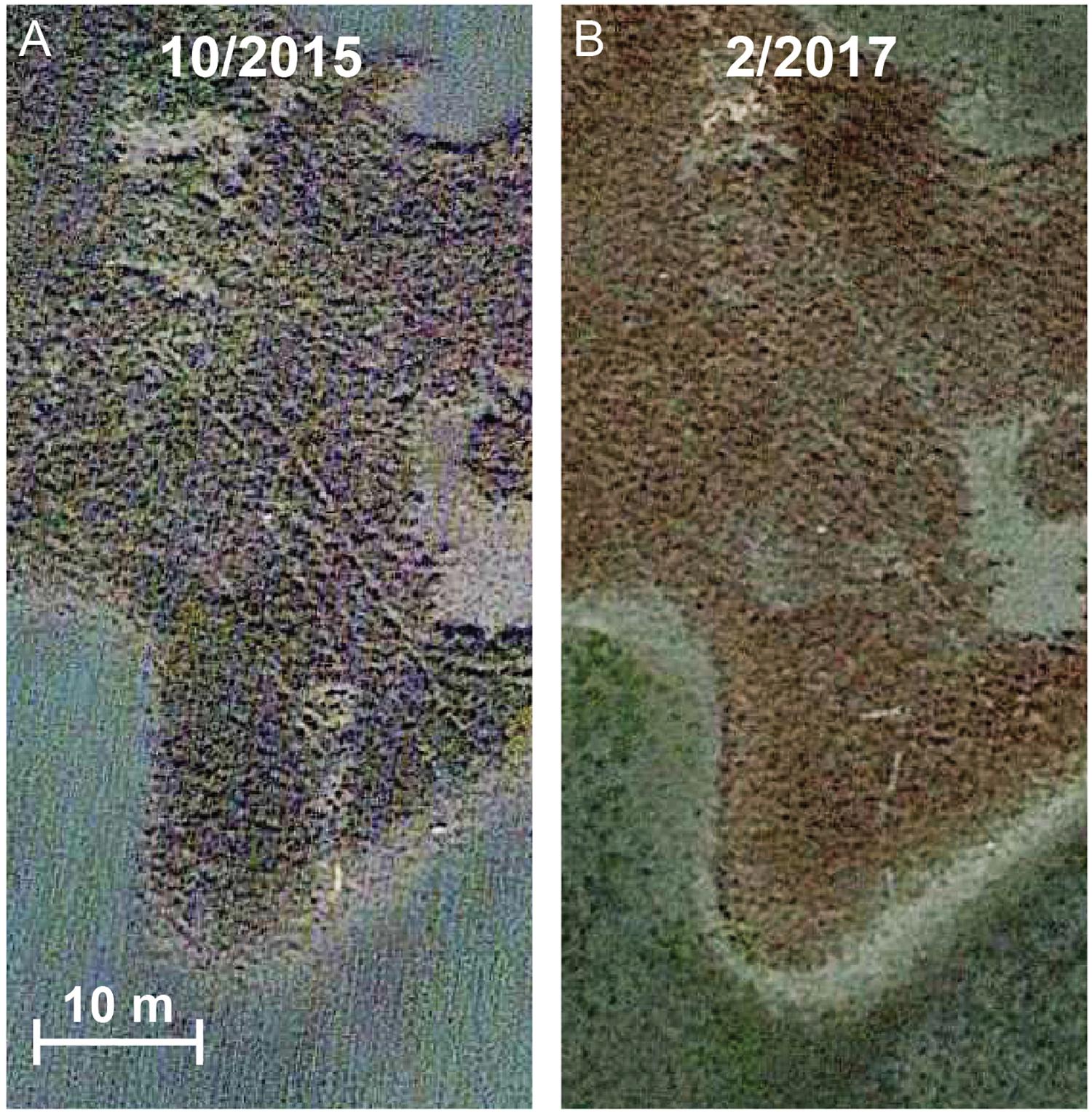
Figure 1. Vegetation sampling transects conducted in 2015 (A) in a North Carolina saltmarsh that are still visible in 2017 (B). Imagery obtained from Google Earth Pro V 7.3.3.7699 (October 25, 2015 and February 19, 2017) Middle Marsh, North Carolina, USA. 18S 351935.66 m E 3839950.94 m N. Eye alt 951 feet. Maxar Technologies 2020. [Accessed May 28, 2020].
The past decade has seen a surge of research using unoccupied aircraft systems (UAS) for conservation applications (Klemas, 2015; Marvin et al., 2016; Manfreda et al., 2018; Harris et al., 2019; Jiménez López and Mulero-Pázmány, 2019; Johnston, 2019). While these platforms are referred to with many names (e.g., drone, unmanned aerial vehicle, UAV, or remotely piloted aircraft, RPA), the aircraft itself represents one part in a system of components, which includes the ground control and communication systems between the remote pilot, aircraft, and the payload sensor on board. The range of platforms (i.e., fixed-wing, rotary-wing, and hybrid) and plethora of modular sensors (e.g., multispectral, hyperspectral, lidar, etc.) makes UAS a highly versatile tool for science and conservation missions, operating on-demand to sample at previously unobtainable temporal and spatial scales, transforming the way we can examine a range of organisms and their ecosystem interactions (Anderson and Gaston, 2013; Chabot and Bird, 2015; Pimm et al., 2015). Furthermore, developments in photogrammetric methods [e.g., Structure from Motion (SfM), spectral analysis, machine learning algorithms] allow users to extract even more information (e.g., three-dimensional structure and vegetation health) from UAS remote sensing data (Mancini et al., 2013; Ballari et al., 2016; Kalacska et al., 2017; Doughty and Cavanaugh, 2019).
As the number, complexity, and scale of coastal restoration projects grow, the application of new technological approaches, including the use of UAS-based tools, is essential. This article provides perspectives on how UAS technology is being applied to restoration tasks, and more specifically, details how they can be further integrated into important components of MER efforts. To give the reader a more comprehensive understanding of UAS capabilities and their potential applications, we first provide a brief overview of current and developing UAS technology, with reference to specific works that cover greater details of UAS equipment, operational workflows, and the data they produce. We then delve into ways UASs can be, or are, applied to various aspects of three key MER stages: planning, implementation, and monitoring (Table 1). These example applications focus primarily on how UAS-based methods can be applied to a living shoreline project, with additional examples supplied in other marine environments. Table 1 presents examples of each these use cases, providing details on the aircraft employed, it’s sensor/payload, the analytical workflow and the citation for that case study.
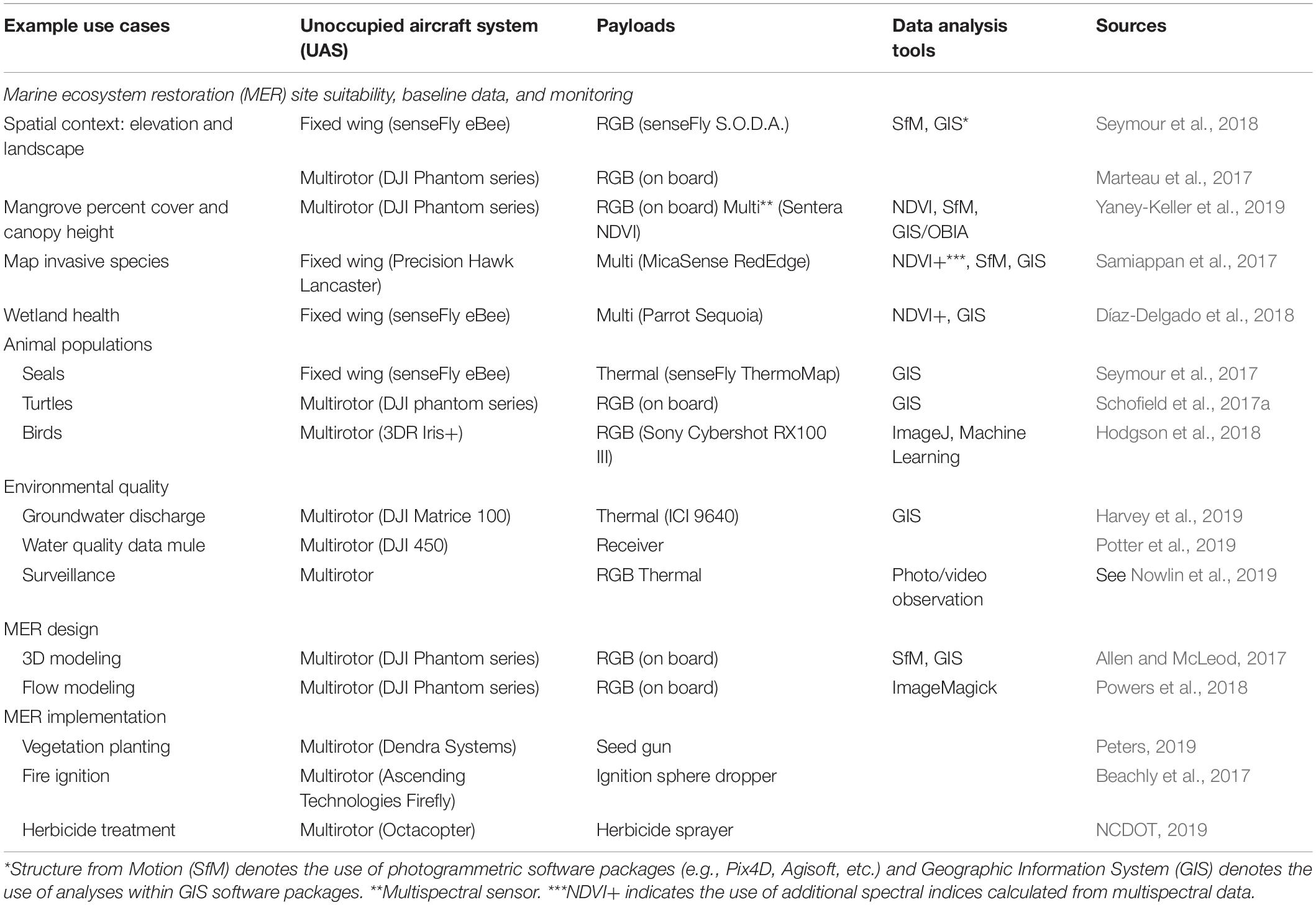
Table 1. Representative use cases with associated aircraft, payloads, and primary data analysis tools.
A Primer on Uas and Their Products
Platforms
There are a number of UAS platforms that can or have been incorporated into MER practices. Based upon their airframe configuration, these aircraft generally fit within three broad categories: rotary wing or multirotor, fixed wing, and transitional (or hybrid). The suite of aircraft distributed among these categories provide different utilities related to their maneuverability, payload capacity, flight endurance, and their launch and recovery needs. Within each category, airframes are available that encompass the spectrum of consumer-grade to professional-grade setups, with varying levels of onboard Global Navigation Satellite System (GNSS) accuracy ranging from several meters horizontal and vertical (consumer-grade) to centimeter accuracy when equipped with a survey-grade real-time kinematic (RTK) GPS (Seymour et al., 2018). Furthermore, almost all UAS are capable of autonomous operation, programmed through mission planning software. While this article focuses on how UAS are employed in MER-related activities, a more thorough discussion on UAS components, best practices and platform considerations for a broad array of missions can be found in González-Jorge et al. (2017), Joyce et al. (2018), Manfreda et al. (2018), Harris et al. (2019), and Johnston (2019).
Payloads
A variety of passive and active sensor options as well as engineered mechanical payloads enable UASs for a multitude of mission objectives. While some UASs come pre-equipped with integrated payloads, many have swappable or modular payloads, sometimes enabled through a modification kit. Passive sensors are most commonly used in restoration work, relying on solar reflectance dynamics, an organism’s thermal radiation, or non-solar generated light. Passive sensors include the basic camera RGB (Red, Green, and Blue) sensors used for digital imaging along with more complex multispectral and hyperspectral cameras that are able to obtain wide and narrow-band information across other sections of the visible and near-visible electromagnetic spectrum. While some of these multispectral sensors are geared to obtain RGB along with the wider-band spectra of near infrared and red edge, some are more directly engineered to obtain just the necessary spectral bands to calculate specific environmental health indices, such as the normalized difference vegetation index (NDVI) that compares red and near-infrared reflectance. Active sensors can include compact lidar systems and certain types of radar, like ground penetrating radar (Wu et al., 2019). Finally, mechanical payloads have been engineered to enable UASs to collect water samples (see review by Lally et al., 2019), deliver herbicides and pesticides (Rodriguez et al., 2017), and even disperse seeds (Stone, 2017).
Data Types and Uses
The variety of payload options and rapidly developing image processing technology allows for the generation of rich, temporally explicit data products that range from basic observational data to more involved environmental mapping. Basic RGB sensors provide high resolution streaming video and still photography to monitor and measure organisms and their behavior (Linchant et al., 2015; Krause et al., 2017; Gallagher et al., 2018) and determine habitats of importance. When UAS are used to conduct a mapping survey of image transects, photogrammetric software (e.g., Pix4D, Metashape, and Drone2Map) can generate high-resolution orthomosaics of study sites (Figure 2A). This can be completed with basic RGB imagery as well as with more advanced multispectral and narrow-band hyperspectral imagery. In the case of these more advanced sensors, the data products are generally in the form of reflectance indices tied to specific bands captured from 5 to 10 wider bands (10–40 nm) with multispectral to 100–1,000 narrow bands (5–15 nm) with hyperspectral, which can then be used to create false color orthomosaics or combined to produce a variety of spectral indices useful to detect specific habitat components and, in some cases, their health.
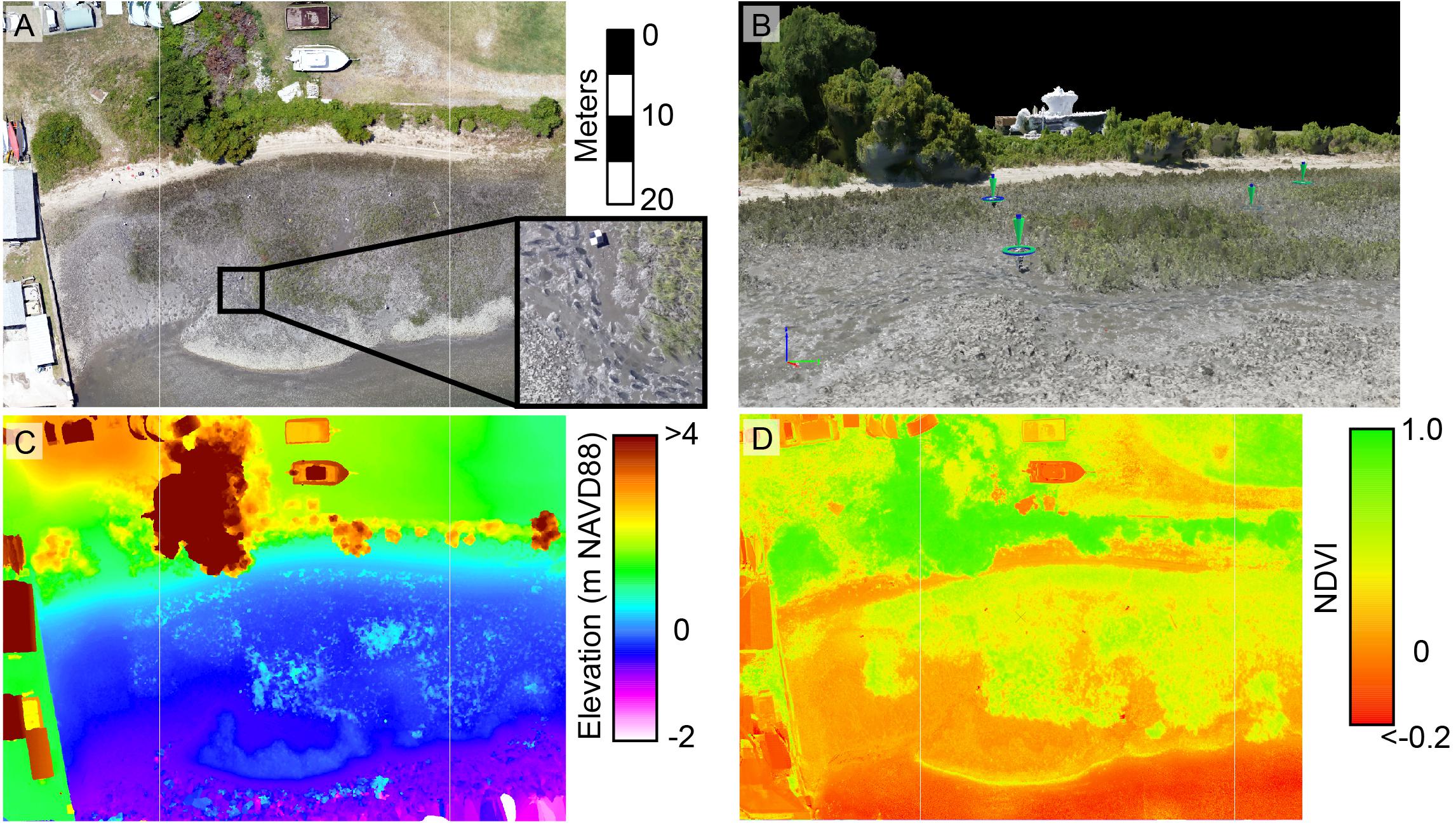
Figure 2. Stitched orthomosaic of a living shoreline (saltmarsh and oyster reef) situated on Pivers Island, North Carolina (A). Densified point cloud of the site with ground control points designated by green arrows (B). Digital elevation model of the site with water artifacts along the bottom edge (C). Normalized difference vegetation index of the site obtained from concurrent multispectral UAS imagery (D).
Unoccupied aircraft systems-generated 3D models provide accurate topographic information, which can inform efforts to restore species that are tidally dependent, as with many of our coastal biogenic habitats (i.e., oysters, saltmarsh, and mangroves). Drones equipped with lidar units can generate highly accurate 3D data of scanned environments (Resop et al., 2019), and simple overlapping imagery from most sensors can also be used to generate 3D products through the SfM process. Indeed, the advancement of SfM techniques provides for incredibly accurate (cm-scale error) 3D data products in coastal environments, especially with the incorporation of ground control practices (Seymour et al., 2018; Casella et al., 2020). From both lidar and SfM, 3D point clouds (Figure 2B and Supplementary Video S1) can be used to create digital surface models, and digital elevation models (Figure 2C) when data are georectified within a vertical datum. UAS-derived 3D modeling has been conducted in a variety of environments including shorelines (Gonçalves and Henriques, 2015; Seymour et al., 2018; Lowe et al., 2019; Seymour et al., 2019; Casella et al., 2020), several shallow-water/tidal habitats (Long et al., 2016; Ventura et al., 2016; Casella et al., 2017; Kalacska et al., 2018; Windle et al., 2019), and to study hydrological dynamics in wetlands (Capolupo et al., 2015; de Roos et al., 2018; Harvey et al., 2019).
A range of UAS data applications and post-processing software systems (e.g., ArcGIS and eCognition) can be used to extract or derive a variety of measurements and habitat condition variables that may be key for restoration efforts. Environmental health indices can be calculated using specific combinations of spectral bands, such as the NDVI mentioned previously (Figure 2D) as well as other indices that have been used in coastal vegetation health assessments (e.g., Normalized Difference Red-Edge Index, Soil-adjusted Vegetation Index, etc.). In a restoration context, these vegetation indices cannot only assess habitat health, but also help delineate habitats and differentiate species (Yaney-Keller et al., 2019). Open source software tools for UAS imagery are becoming more prevalent, enabling users to quickly conduct measurements on photographed organisms to assess their body condition and health (Torres and Bierlich, 2020). Advanced machine learning algorithms are now being widely used to classify UAS data based on spectral and 3D characteristics. Object-based image analysis (OBIA) has been used to effectively classify UAS-based maps of shallow water environments such as seagrass meadows and hard bottom habitats (Chabot et al., 2018; Ventura et al., 2018). Many of these algorithms are now established tools within geospatial software packages, but some habitat classifications may require more complex applications of machine learning such as neural networks to train a computer how to identify target habitats or metrics (Casado et al., 2015; Ridge et al., 2020). In this context, UAS can be applied to the development of training data for machine learning systems, as well as for conducting rapid validation sampling that reduces human impacts on restored systems (Gray et al., 2018). Regardless of programming complexity, increasingly active data and script sharing, facilitated through standard open source licensing, is making these tools more accessible to the general user.
Planning and Initial Monitoring
The use of UASs during the planning stages of MER projects provides heightened situational awareness of the targeted location and the larger environmental context it is embedded within. In many cases, data generated through UAS surveys of a proposed restoration site can help researchers and practitioners locate and target critical habitats (e.g., coral reefs, seagrass, mangroves, etc.) or provide maps and geospatial information needed to identify and assess specific ecosystem dynamics (predator/prey relationships) or environmental conditions (e.g., hydrological functions). With all the considerations necessary to planning, there are many avenues for incorporating UASs into this process. The major applications during project planning revolve around assessing site suitability (determining whether a location is amenable to a particular MER practice), gathering baseline data and using UAS products to guide MER design (Figure 3).
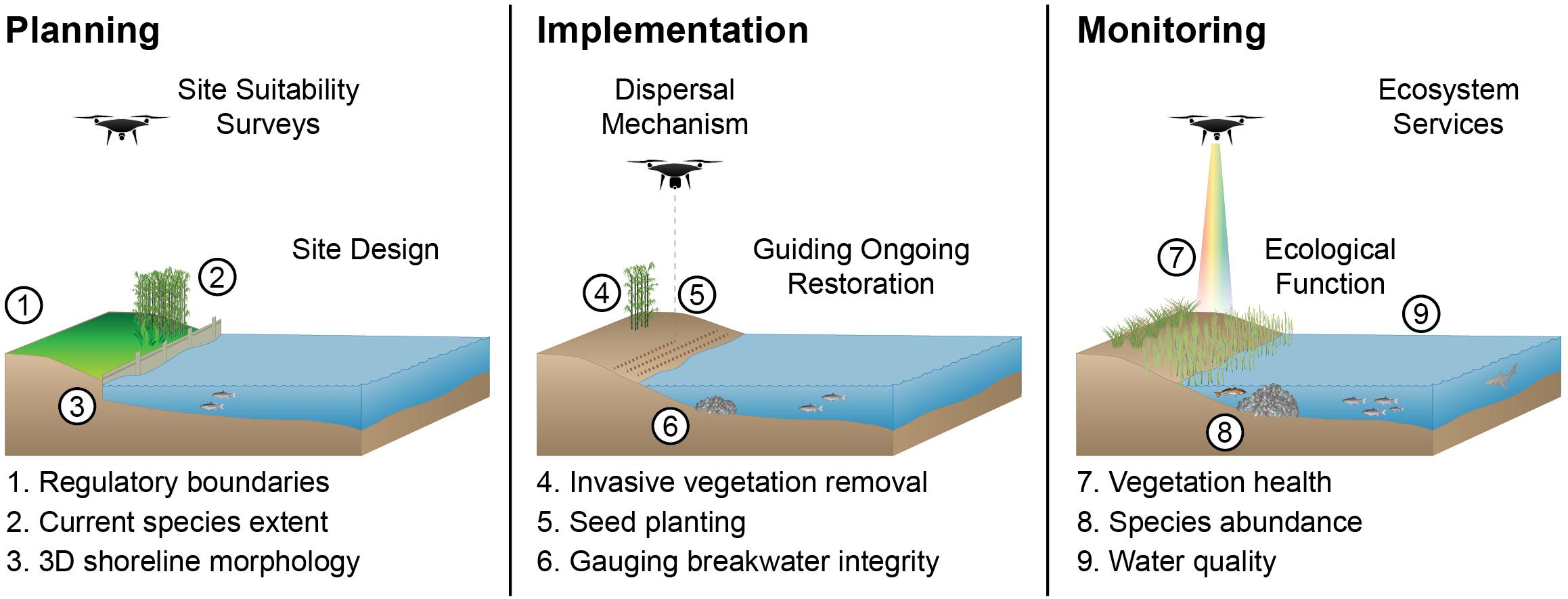
Figure 3. Synopsis of unoccupied aircraft system (UAS) applications in Marine Ecosystem Restoration (MER) projects at the three major stages with select examples provided. The Planning stage includes collecting baseline data to establish regulatory and ecological boundaries (Site Suitability) and generation of visuals and models help determine MER success criteria (Site Design). In the Implementation stage, UASs can be applied for vegetation planting, fire ignition, herbicide or pesticide treatments as well as helping direct ongoing restoration activities. During the Monitoring stage, UASs can collect data on vegetation health, wildlife abundance and behavior (Ecological Function) and data on topography, hydrology, and water quality of the surrounding landscape (Ecosystem Services). Some of the symbols for diagrams courtesy of the Integration and Application Network (ian.umces.edu/symbols).
Site Suitability and Baseline Data
From the air, users can obtain a perspective on a potential restoration site to gather data with little to no disturbance to the actual environment. With the ability to collect high resolution imagery and 3D environmental data, planners can better place a MER within the boundaries of ecological and regulatory frameworks. In this context, UAS can be applied as survey instruments to establish exactly where restoration efforts can and should be conducted, based on existing property limits or ecotones. In the example of a living shoreline, planners generally have to consider the restored wetland’s setting within the general environment (e.g., tidal range, landward boundary, etc.) as well as proximity to limiting factors, such as breakwaters to navigational channels or beds of submerged aquatic vegetation.
Accurate baseline data at restoration sites are essential to determine if MER efforts are successful. Many MER projects implement a before-after-control-impact design for assessment purposes, where obtaining pre-restoration conditions is paramount. For a living shoreline MER, this could involve mapping the current extent of specific species and their percent cover (Marcaccio et al., 2015; Husson, 2016; Yaney-Keller et al., 2019), invasive or non-native organisms (Figure 4A; Samiappan et al., 2017), and signs of degradation (Chirayath and Earle, 2016) (e.g., reef loss, marsh die off, and shoreline erosion). Similarly, Nahirnick et al. (2019) used UAS imagery to map eelgrass and were able to differentiate between eelgrass and macroalgae based on image textural differences that may not be as apparent in lower resolution imagery (>m). Additional use of specialized sensors can offer an even more detailed perspective of habitat condition through generation of health indices. NDVI can aid the classification process by using the values to isolate coastal vegetation through masking (Ballari et al., 2016), and UAS-derived NDVI values correlate strongly with aboveground biomass in a California saltmarsh (Doughty and Cavanaugh, 2019). Similarly, Díaz-Delgado et al. (2018) used UAS-based multispectral data to assess indicators of ecological integrity of wetlands in Spain. Hyperspectral sensors could be used to further discriminate species (Silvestri et al., 2003) and refine vegetation index results by removing background spectral noise in these habitats (e.g., oversaturation in dense vegetation, sediment reflection – see Hladik et al., 2013). Parsons et al. (2018) used UAS-obtained RGB and hyperspectral imagery in combination with in-water surveys to help identify areas of degradation in coral reefs. Specialized multi and hyperspectral sensors can also help determine turbidity and sediment flow (Vogt and Vogt, 2016; Díaz-Delgado et al., 2018) as well as concentrations of chlorophyll in the surface water (Kislik et al., 2018). Thermal sensors can identify groundwater discharge (Willms and Whitworth, 2016; Harvey et al., 2019), which can impact the salinity dynamics of a site or the potential input of contaminants from affected groundwater.
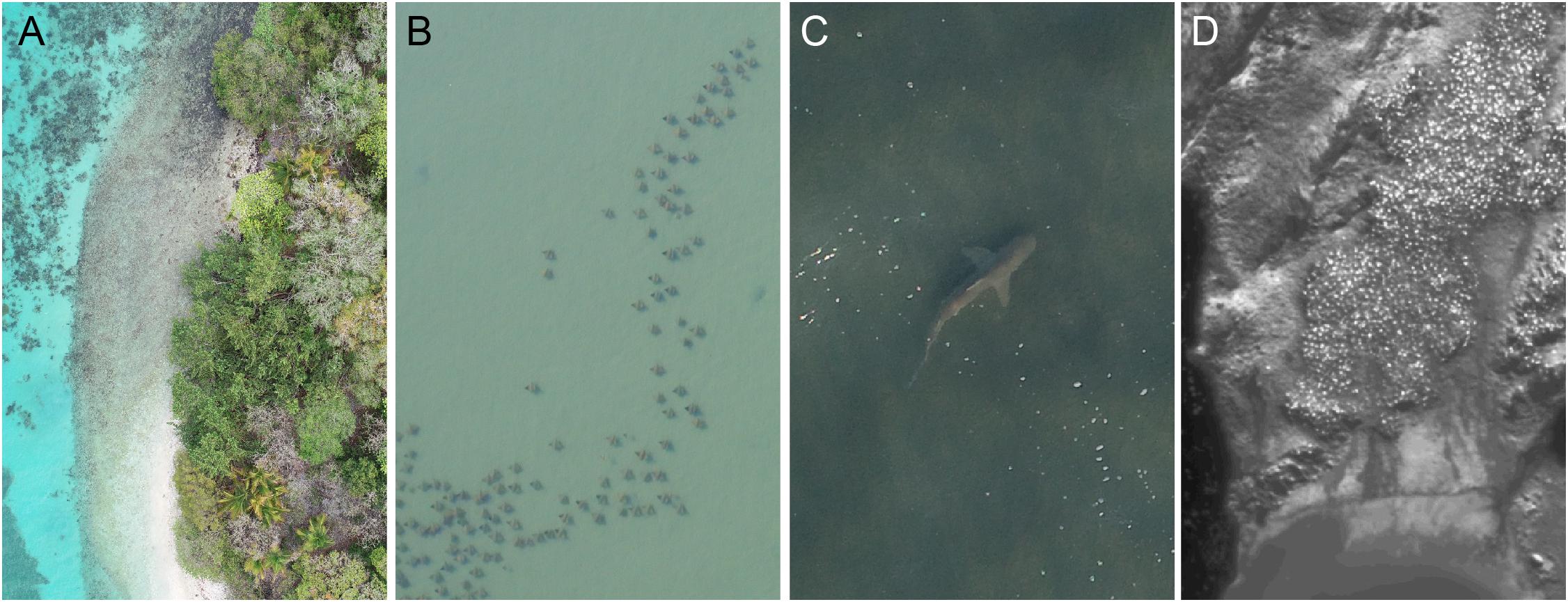
Figure 4. Examples of UAS data products capturing the presence and locations of non-native coconut palms among mangroves in Belize (A), the migration of thousands of cownose rays in a North Carolina estuary (B), shark behavior (C), and the thermal signatures (white dots) of a penguin colony (D). All images obtained by Duke University Marine Robotics and Remote Sensing Lab, collected under permit.
The use of UASs in MER is not limited to baseline habitat assessments. For example, UASs have been used to observe a variety of coastal and marine species including fish (Kiszka et al., 2016; Raoult et al., 2018; Rieucau et al., 2018; Benavides et al., 2019; Gore et al., 2019), birds (Hodgson et al., 2016, 2018; Han et al., 2017), reptiles (Elsey and Trosclair, 2016; Schofield et al., 2017a, b; Sykora-Bodie et al., 2017; Bevan et al., 2018; Rees et al., 2018), and mammals (Durban et al., 2015; Johnston et al., 2017; Krause et al., 2017; Seymour et al., 2017; Ramos et al., 2018). In some cases this is focused observation of an individual/group (Figures 4B,C), as seen with whale behavior studies (Torres et al., 2018), or orthomosaic products that can be used to enumerate congregations of organisms such as nesting birds (Figure 4D; Hodgson et al., 2016) or seal colonies (Johnston et al., 2017). UASs equipped with thermal infrared sensors can help quantify wildlife in an area, and thermal imagery has been particularly useful for examining marine mammal populations (Seymour et al., 2017) and nesting birds (Christie et al., 2016). Willms and Whitworth (2016) used UAS thermal imaging to identify temperature dynamics of spawning waters for salmon. For a projected living shoreline, these data could provide a before-restoration reference for wildlife use of the site. There continues to be major efforts ensuring that UASs for wildlife monitoring are operated at safe and non-invasive altitudes, which has produced some very promising results indicating that UASs can still collect high-resolution data without disturbing wildlife (see Arona et al., 2018 and reviews in Christie et al., 2016; Mulero-Pázmány et al., 2017).
Finally, baseline data on pollution factors at proposed restoration sites can also be facilitated with UAS. For example, UAS can be used to monitor marine debris in coastal and marine environments. UAS imagery coupled with machine learning algorithms have proven efficient at identifying and classifying litter (Martin et al., 2018), and Hengstmann et al. (2017) were able to use a UAS to geolocate marine debris and help identify the major factors influencing its distribution as part of a large beach marine litter monitoring effort in Germany. Similar work has been conducted in areas of the United States, examining marine debris dispersal across an estuarine landscape to locate hot spots for targeting clean ups and considering litter source mitigation (Newton et al., unpublished data).
Marine Ecosystem Restoration Design
Once baseline imagery is captured and processed, the maps and models generated can become instrumental for designing restoration projects. As with the living shoreline example, a restored saltmarsh may include a fringing oyster reef sill. Oysters thrive within very specific zones of the tidal range (Morris et al., 2002; Ridge et al., 2015), and the 3D models of restoration sites can help planners quickly calculate the possible extent of a MER as well as distributional guidance for vegetation and volumetric information for oyster material. Comparably, Fodrie et al. (2014) spent several days walking GPS transects across extensive sandy shoals to plan where to site their oyster restoration study, which could have been achieved in the course of just a few hours with a UAS.
Unoccupied aircraft systems-obtained data on environmental variables can help MER designers make process-driven decisions about how to structure restoration efforts. Basic RGB sensors can be used in conjunction with rhodamine applications to track flow dynamics (Powers et al., 2018) through the landscape. A UAS could be outfitted with ground penetrating radar to measure soil moisture (Wu et al., 2019), an important factor for wetland vegetation health. Soil type, quality and moisture are essential components for planning where and how to plant wetland vegetation. Understanding these dynamics can help shape a MER, allowing planners to take advantage of natural processes to maximize the success of the project.
The creation of MER site maps and model visualizations have manifold utility. The site map and 3D model could be ingested into design software for advanced viewshed analysis, rendering what the proposed project would look like when finished. These can be useful materials when approaching potential funding agencies and gaining community interest and support for restoration efforts. Finally, these initial surveys will ultimately serve as the baseline reference for establishing and eventually assessing project success metrics.
Implementation
Restoration efforts can be a massive undertaking, potentially requiring restructuring of the landscape and the deployment of materials (e.g., shell, seedlings, and corals). Considering the manual nature of many MER efforts, it is unfeasible for UASs to replace many current methods. However, UAS can assist MERs in the form of an actual dispersal mechanism for various materials (Figure 3). Within the last several years, drone-based solutions for planting trees and grasses have emerged, and are being implemented on a large scale (10s of hectares) to restore mangroves in Myanmar (Peters, 2019). In this case, a UAS was equipped with a payload that fires seed bullets, essentially biodegradable pods with a germinated seed and nutrients, into the ground. This highly successful application has resulted in the germination of millions of mangrove plants, potentially revolutionizing large scale mangrove restoration efforts. It is possible that with the right packaging of nutrients and germinated seeds this method could be expanded to other MER efforts, like saltmarsh plantings. In other dispersal methods, UASs are now being used for targeted management in the form of fire ignitions and herbicide treatments. In the case of wetland restoration, fire has been a traditional tool for inhibiting woody species (Nyman and Chabreck, 1995), and UASs have been developed with an integrated ignition sphere dispenser that is programmed to drop the spheres at specific intervals to initiate a controlled burn (Beachly et al., 2017). Some restoration efforts face encroachment by non-native species, like the common reed Phragmites australis. Restoration work in a Spartina alterniflora marsh showed that herbicide treatment of Phragmites allowed the native S. alterniflora to bounce back in under 5 years (Gratton and Denno, 2005). Recently, UASs have been outfitted with herbicide dispensers and have become part of Phragmites control initiatives in North Carolina (NCDOT, 2019). These types of uses extend beyond addressing plant species. For example, UAS can now be used to drop poison baits targeting invasive species of rodents (Island Conservation, 2019).
While some MER activities are discrete one-time efforts, many are multiyear programs where UASs can be a significant boon by guiding subsequent actions and interventions. Periodic surveying and follow up plantings, invasive or unwanted vegetation treatments, or the need to secure or augment stabilization structures like an oyster reef sill are examples of these extended use-cases. The use of multispectral and hyperspectral sensors can provide vegetation index information during MER implementation to gauge the health of the restored vegetation and identify whether or not additional actions need to be taken to ensure restoration success (e.g., fertilizer treatments, grazer removals, etc.).
Monitoring Success
The autonomous nature of UAS surveys makes for an efficient, repeatable tool for monitoring an ecosystem. Many of the UAS applications for monitoring success of MERs are extensions of the applications discussed earlier for the planning phase (Figure 3). However, the temporal context becomes even more important with restoration monitoring, since understanding how these systems are changing through- or as a result of MER efforts is essential for gauging project outcomes. Furthermore, UAS-derived data can help achieve specific monitoring metrics established by restoration funding agencies (e.g., US Fish and Wildlife Service, National Fish and Wildlife Foundation), which will serve as an added frame of reference in the sections below.
Ecological Dynamics
Subsequent UAS mapping missions using simple RGB or combined multispectral and/or hyperspectral can assess changes to areal habitat extent through time. This process can elucidate changes in the extents of the restored species in conjunction with other possible notable factors, like invasive species (Figure 4A). As an example application for meeting funding requirements, measuring percent cover by species is one of the National Fish and Wildlife Foundation’s (NFWF) primary monitoring metrics for both wetland and living shoreline restoration projects under their Coastal Resilience Fund (NFWF, 2019), and includes the total areal footprint of oyster reef restored. Under NFWF guidance, both of these restoration activities also require a metric for shoreline position (NFWF, 2019), which can be obtained from UAS-derived orthomosaics with cm-level accuracy.
Repeatable visual transects, through RGB and/or thermal infrared, can provide population estimates of organisms or behavioral insights of species across trophic levels, essentially measuring the use and ecological functions of restored habitats as compared to the pre-restoration conditions. For example, Rieucau et al. (2018) conducted UAS video transects along fringing reefs to examine habitat-dependent shark behavior, providing a baseline for possible future disruptions to that habitat. Sykora-Bodie et al. (2017) used UAS image transects perpendicular to the beach to identify and count sea turtles in areas that may result in interactions with fisheries, and Gray et al. (2019) went a step further by using a deep learning algorithm to automate sea turtle detection. These methods can be applied to understanding if a MER is benefiting local wildlife and providing desired ecosystem services.
Structure from Motion (SfM) technology continues to transform the role UASs can play in assessing ecosystem structure. Instead of measuring oyster reef growth through time using terrestrial lidar (Rodriguez et al., 2014; Ridge et al., 2015, 2017) UAS-derived approaches (e.g., Windle et al., 2019), could assess a greater number of oyster reefs during narrow low tide windows across larger coastal landscapes. The development of structural metrics in vegetated environments has been greatly enhanced by the addition of UAS tools. Multiple studies have used SfM products to measure canopy heights and estimate biomass in mangroves (Warfield and Leon, 2019; Yaney-Keller et al., 2019). Yaney-Keller et al. (2019) actually used a dual band NDVI sensor (no RGB) in conjunction with SfM to measure canopy heights and percent cover in mangroves, and differentiate species in the estuaries of Pacific Costa Rica. Boon et al. (2016) also combined SfM products with orthophotos to greatly enhance wetland delineation and health assessment.
Ecosystem Services
In addition to monitoring ecological recovery, UASs have a vast potential for monitoring how MER efforts benefit the surrounding landscape, both natural and anthropic. Many MER projects have implications for shoreline stabilization and coastal resilience, and assessing how adjacent landscapes are sheltered by these marginal habitats is a metric for evaluating success. One way to monitor this is through assessing elevation, a primary metric often included in restoration monitoring for wetlands and living shorelines (NFWF, 2019) as it represents the habitat’s vulnerability to – and ability to keep pace with – sea level rise. Elevations are easily obtained for non-vegetated surfaces using SfM, like oyster reefs (Windle et al., 2019) and beach environments (Seymour et al., 2018), but may need a more strategic approach in densely vegetated environments, potentially using dormant season flights, fine scale mapping (low altitude), or other references like water level. Working in a coastal wetland, Meng et al. (2017) developed a workflow to help estimate the terrain in these densely vegetated environments. While not serving as a replacement for finite sediment surface measurements like those obtained from saltmarsh Surface Elevation Tables (SETs, Cahoon, 2015), UAS-derived elevation data could help determine the overall scalability of SET measurements over a larger area. UASs have been used to track changes to barrier islands and other coastal environments over the course of months to years capturing morphological changes to the landscape (Seymour et al., 2019). Similarly, Marteau et al. (2017) used these methods to track geomorphic change of a restored riverine environment to assess restoration outcomes.
As mentioned previously, UAS payload configurations can be used to sample water quality either directly or through remotely sensed data processes to monitor how well a MER is benefiting water quality. Considering the repetitive nature of monitoring, a rising concept in UASs for environmental observation is the idea of drones as data mules (Palma et al., 2017). This relies on a coupled system with in situ sensors and a UAS equipped with data retrieval hardware that will remotely link to these sensors and download the data (Potter et al., 2019). For the purposes of MER, the deployed equipment could be a network of autonomous water quality samplers across a site (Trevathan and Johnstone, 2018). Instead of physically extracting the data from the samplers, a UAS could be flown over with a datalink to retrieve the data wirelessly, never requiring managers to have to enter the site. Potter et al. (2019) demonstrated the use of this coupled system with a network of sensors collecting water temperature, pH, and conductivity that transmitted the data to a UAS that hovered above the central node. The system could be adapted to collect any number of environmental variables and would be especially useful in remote locations that do not have existing wireless communication networks.
Another major benefit of UAS-based monitoring is the fine temporal scale afforded by its on-demand nature. A combination of sensor payloads can provide an abundance of multidimensional data over a landscape in a relatively short period of time. This readily enables the surveying of MER sites before and after storms, or other predictable disturbances, to assess impact on the MER as well the benefits a MER may be offering adjacent habitats. In the case of a living shoreline restoration project, UAS provide significant opportunities to assess how natural systems dampen storm energy compared to artificial or unstructured shorelines. Recognizing this advantage, UASs are incorporated into post-storm monitoring in coastal systems internationally (Turner et al., 2016; Seymour et al., 2019; Casella et al., 2020). Studies conducted on living shorelines to assess their resilience and shoreline protection against storm impacts (e.g., Smith et al., 2018) would benefit from the incorporation of UASs, which would expedite field sampling and reduce unintentional, but inevitable, impacts of direct sampling within the habitat (Figure 1).
Enforcement and Protection
While highly dependent upon the legal restrictions governing an area, UASs have been used as a tool to protect conservation areas. With limited personnel resources, UASs could provide a periodic mobile watchtower over a MER site to deter poaching (Mulero-Pázmány et al., 2014) and other illegal or unpermitted acts. A review of UAS use for conservation in protected areas touted the benefits of using drones for enforcement while also acknowledging that there are still some ethical considerations left to work out (Jiménez López and Mulero-Pázmány, 2019). In general, though, UAS surveying of public use of a managed land can be a viable option, and Nowlin et al. (2019) provide best practices for this use case in some coastal contexts.
Caveats
As with any developing technology, caveats to its integration emerge as we test limits and determine best practices. Unfortunately for some entities drone usage may be periodically restricted, as has been seen while governments navigate cybersecurity issues (e.g., grounding of some United States federal agency drone fleets –U.S. Department of the Interior [USDOI], 2020). While the UAS research community has begun to harmonize methods, standards, procedures, and reporting (Joyce et al., 2018; Barnas et al., 2020), there remains work to be done (Buters et al., 2019). Some key caveats for UAS MER applications are related to image acquisition methods. For example, image quality of submerged landscapes from UAS is highly dependent on a number of environmental conditions including (but not limited to) sun angle, cloud cover, surface disturbance by wind, and turbidity (Joyce et al., 2018; Nahirnick et al., 2019). This may also prove true in areas with uniform image texture (e.g., smooth sand) that may generate artifacts in the point clouds (Seymour et al., 2018). When imaging forest canopies, like mangroves, higher altitudes and more overlap may be necessary because of the greater potential for the foliage to move with even just a small amount of wind (Fraser and Congalton, 2018). NDVI assessments of certain species, like emergent vegetation, can be affected by inundation (Kearney et al., 2009) or high soil water content (Ballari et al., 2016) affecting attempts to estimate biomass (Byrd et al., 2014). In some cases, these problems can be mitigated with specific mission planning insights, like adjusting UAS altitude, orientation of acquired imagery (away from sun), or understanding the limitations of the data collected for a certain habitat. Further consideration must also be made to the cost-benefit of using UAS technology for specific applications. While costs of aircraft and sensors continue to drop, the pricing can range from $1,000 (USD) for consumer-grade to $10K–$20K (USD) for professional-grade aircraft and sensor packages, with the more advanced packages (e.g., hyperspectral, lidar, etc.) reaching $50K–$100K (USD). The software (e.g., Pix4D, Agisoft Metashape, etc.) may add another cost on the order of $1K–$5K (USD). This may make adoption of UASs cost-prohibitive for outright purchase for some restoration managers, but the data could still be obtained by contracting an institution equipped with appropriate resources. It is worth noting that many of the studies within this review used a consumer-grade drone on the order of $1,000–$2,000 (USD), indicating a lot of work can still be accomplished at that technology tier. There are also well-funded UAS and spatial analysis programs that help train and equip natural resource managers in developing regions, like the growing Flying Labs program (WeRobotics, 2020). This program has fostered the development of local UAS capacity in 27 countries spread across South America, Africa, Asia, and Europe that, in part, seek to overcome this challenge. Though technical and regulatory hurdles exist (Hardin and Jensen, 2011), progress made within the last decade to alleviate these hurdles is quite promising for the further development and application of this technology (Hardin et al., 2019).
Conclusion
As drone platform and sensor technology continues to advance, UAS-based approaches to MER will further bridge the scale between in situ sampling and remote sensing from occupied aircraft and satellites. Innovations in platforms, sensors, engineered payloads, and image processing have proven to be a valuable addition to the MER toolbox, across all stages of a project. UAS offer unparalleled flexibility in temporal and spatial resolution for environmental monitoring, enabling access to hard-to-reach areas with little to no disturbance. While UAS can be applied successfully in most restoration projects, these operations must be conducted within local legal and ethical frameworks (e.g., pilot certifications, airspace authority, and permitting) and with consideration of potential disturbance impacts to non-target species. Limitations in UAS flight time, operational conditions, and payload capacity will continue to diminish, as will airspace restrictions and other regulatory hurdles. As these challenges fade, UAS-based approaches to marine restoration projects will increase and diversify, establishing UAS as a fundamental and versatile tool for enhancing planning, implementation, and monitoring of marine restoration efforts globally.
Author Contributions
All authors listed have made a substantial, direct and intellectual contribution to the work, and approved it for publication.
Funding
Support for this article and several projects highlighted within has been provided by the North Carolina Division of Marine Fisheries (Marine Resources Fund, Grant #2017-H-068) and the Duke Bass Connections Program.
Conflict of Interest
The authors declare that the research was conducted in the absence of any commercial or financial relationships that could be construed as a potential conflict of interest.
Acknowledgments
We would like to thank the current and former members of the Marine Robotics and Remote Sensing Lab for their role in the projects highlighted within this work.
Supplementary Material
The Supplementary Material for this article can be found online at: https://www.frontiersin.org/articles/10.3389/fmars.2020.00438/full#supplementary-material
VIDEO S1 | Densified point cloud created from unoccupied aircraft system imagery (standard RGB) over a living shoreline constructed by the National Oceanic and Atmospheric Administration Beaufort Lab (Pivers Island, Beaufort, North Carolina, USA). The restored shoreline consists of planted saltmarsh and oyster reef.
References
Adam, P. (2002). Saltmarshes in a time of change. Environ. Conserv. 29, 39–61. doi: 10.1017/S0376892902000048
Allen, T., and McLeod, G. (2017). Geodesign and Sea Level Rise: Linking Coastal Flooding, Ecosystem Models, Drone Mapping, and Green Infrastructure for Coastal Resilience. Available online at: https://coastalgeotools.org/wp-content/uploads/Allen.pdf (accessed May 12, 2020).
Anderson, K., and Gaston, K. J. (2013). Lightweight unmanned aerial vehicles will revolutionize spatial ecology. Front. Ecol. Environ. 11, 138–146. doi: 10.1890/120150
Arona, L., Dale, J., Heaslip, S. G., Hammill, M. O., and Johnston, D. W. (2018). Assessing the disturbance potential of small unoccupied aircraft systems (UAS) on gray seals (Halichoerus grypus) at breeding colonies in Nova Scotia, Canada. PeerJ 6:e4467. doi: 10.7717/peerj.4467
Ballari, D., Orellana, D., Acosta, E., Espinoza, A., and Morocho, V. (2016). Uav monitoring for environmental management in galapagos islands. Int. Arch. Photogramm. Remote Sens. 41, 1105–1111. doi: 10.5194/isprsarchives-XLI-B1-1105-2016
Benavides, M. T., Fodrie, F. J., and Johnston, D. W. (2019). Shark detection probability from aerial drone surveys within a temperate estuary. J. Unmanned Veh. Syst. 8, 44–56. doi: 10.1139/juvs-2019-0002
Barbier, E. B., Hacker, S. D., Kennedy, C., Koch, E. W., Stier, A. C., and Silliman, B. R. (2011). The value of estuarine and coastal ecosystem services. Ecol. Monogr. 81, 169–193.
Barnas, A., Chabot, D., Hodgson, A., Johnston, D. W., Bird, D. M., and Ellis-Felege, S. N. (2020). A standardized protocol for reporting methods when using drones for wildlife research. J. Unmanned Veh. Syst. 8, 89–98. doi: 10.1139/juvs-2019-0011
Beachly, E., Higgins, J., Laney, C., Elbaum, S., Detweiler, C., Allen, C., et al. (2017). “A micro-UAS to start prescribed fires,” in 2016 International Symposium on Experimental Robotics, eds D. Kulić, Y. Nakamura, O. Khatib, and G. Venture (Cham: Springer International Publishing), 12–24. doi: 10.1007/978-3-319-50115-4_2
Bevan, E., Whiting, S., Tucker, T., Guinea, M., Raith, A., and Douglas, R. (2018). Measuring behavioral responses of sea turtles, saltwater crocodiles, and crested terns to drone disturbance to define ethical operating thresholds. PLoS One 13:e0194460. doi: 10.1371/journal.pone.0194460
Boon, M. A., Greenfield, R., and Tesfamichael, S. (2016). Wetland assessment using unmanned aerial vehicle (UAV) photogrammetry. Int. Arch. Photogramm. Remote Sens. Spat. Inf. Sci. ISPRS Arch. 41, 781–788. doi: 10.5194/isprsarchives-XLI-B1-781-2016
Buters, T. M., Bateman, P. W., Robinson, T., Belton, D., Dixon, K. W., and Cross, A. T. (2019). Methodological ambiguity and inconsistency constrain unmanned aerial vehicles as a silver bullet for monitoring ecological restoration. Remote Sens. 11, 1–16. doi: 10.3390/rs11101180
Byrd, K. B., O’Connell, J. L., Di Tommaso, S., and Kelly, M. (2014). Evaluation of sensor types and environmental controls on mapping biomass of coastal marsh emergent vegetation. Remote Sens. Environ. 149, 166–180. doi: 10.1016/j.rse.2014.04.003
Cahoon, D. R. (2015). Estimating relative sea-level rise and submergence potential at a coastal Wetland. Estuar. Coasts 38, 1077–1084. doi: 10.1007/s12237-014-9872-8
Capolupo, A., Pindozzi, S., Okello, C., Fiorentino, N., and Boccia, L. (2015). Photogrammetry for environmental monitoring: the use of drones and hydrological models for detection of soil contaminated by copper. Sci. Total Environ. 514, 298–306. doi: 10.1016/j.scitotenv.2015.01.109
Casado, M. R., Gonzalez, R. B., Kriechbaumer, T., and Veal, A. (2015). Automated identification of river hydromorphological features using UAV high resolution aerial imagery. Sensors 15, 27969–27989. doi: 10.3390/s151127969
Casella, E., Collin, A., Harris, D., Ferse, S., Bejarano, S., Parravicini, V., et al. (2017). Mapping coral reefs using consumer-grade drones and structure from motion photogrammetry techniques. Coral Reefs 36, 269–275. doi: 10.1007/s00338-016-1522-0
Casella, E., Drechsel, J., Winter, C., Benninghoff, M., and Rovere, A. (2020). Accuracy of sand beach topography surveying by drones and photogrammetry. Geo Mar. Lett. 40, 255–268. doi: 10.1007/s00367-020-00638-8
Chabot, D., and Bird, D. M. (2015). Wildlife research and management methods in the 21st century: where do unmanned aircraft fit in? J. Unmanned Veh. Syst. 3, 137–155. doi: 10.1139/juvs-2015-0021
Chabot, D., Dillon, C., Shemrock, A., Weissflog, N., and Sager, E. (2018). An Object-Based Image Analysis Workflow for Monitoring Shallow-Water Aquatic Vegetation in Multispectral Drone Imagery. ISPRS Int. J. Geo Inform. 7:294. doi: 10.3390/ijgi7080294
Chirayath, V., and Earle, S. A. (2016). Drones that see through waves – preliminary results from airborne fluid lensing for centimetre-scale aquatic conservation. Aquat. Conserv. Mar. Freshw. Ecosyst. 26, 237–250. doi: 10.1002/aqc.2654
Christie, K. S., Gilbert, S. L., Brown, C. L., Hatfield, M., and Hanson, L. (2016). Unmanned aircraft systems in wildlife research: current and future applications of a transformative technology. Front. Ecol. Environ. 14, 241–251. doi: 10.1002/fee.1281
de Roos, S., Turner, D., Lucieer, A., and Bowman, D. M. J. S. (2018). Using digital surface models from UAS imagery of fire damaged sphagnum peatlands for monitoring and hydrological restoration. Drones 2:45. doi: 10.3390/drones2040045
Díaz-Delgado, R., Cazacu, C., and Adamescu, M. (2018). Rapid assessment of ecological integrity for LTER wetland sites by using UAV multispectral mapping. Drones 3:3. doi: 10.3390/drones3010003
Doughty, C. L., and Cavanaugh, K. C. (2019). Mapping Coastal Wetland Biomass from High Resolution Unmanned Aerial Vehicle (UAV) Imagery. Remote Sens. 11:540. doi: 10.3390/rs11050540
Durban, J. W., Fearnbach, H., Barrett-Lennard, L. G., Perryman, W. L., and Leroi, D. J. (2015). Photogrammetry of killer whales using a small hexacopter launched at sea. J. Unmanned Veh. Syst. 3, 131–135. doi: 10.1139/juvs-2015-0020
Elsey, R. M., and Trosclair, P. L. (2016). The use of an unmanned aerial vehicle to locate alligator nests. Southeast. Nat. 15, 76–82. doi: 10.1656/058.015.0106
Fodrie, F. J., Rodriguez, A. B., Baillie, C. J., Brodeur, M. C., Coleman, S. E., Gittman, R. K., et al. (2014). Classic paradigms in a novel environment: inserting food web and productivity lessons from rocky shores and saltmarshes into biogenic reef restoration. J. Appl. Ecol. 51, 1314–1325. doi: 10.1111/1365-2664.12276
Fraser, B. T., and Congalton, R. G. (2018). Issues in Unmanned Aerial Systems (UAS) data collection of complex forest environments. Remote Sens. 10:908. doi: 10.3390/rs10060908
Gallagher, A. J., Papastamatiou, Y. P., and Barnett, A. (2018). Apex predatory sharks and crocodiles simultaneously scavenge a whale carcass. J. Ethol. 36, 205–209. doi: 10.1007/s10164-018-0543-2
Goldman Martone, R., and Wasson, K. (2008). Impacts and interactions of multiple human perturbations in a California salt marsh. Oecologia 158, 151–163. doi: 10.1007/s00442-008-1129-4
Gonçalves, J. A., and Henriques, R. (2015). UAV photogrammetry for topographic monitoring of coastal areas. ISPRS J. Photogramm. Remote Sens. 104, 101–111. doi: 10.1016/j.isprsjprs.2015.02.009
González-Jorge, H., Martínez-Sánchez, J., Bueno, M., and Arias, P. (2017). Unmanned aerial systems for civil applications: a review. Drones 1:2. doi: 10.3390/drones1010002
Gore, M., Abels, L., Wasik, S., Saddler, L., and Ormond, R. (2019). Are close-following and breaching behaviours by basking sharks at aggregation sites related to courtship? J. Mar. Biol. Assoc. U.K. 99, 681–693. doi: 10.1017/S0025315418000383
Gratton, C., and Denno, R. F. (2005). Restoration of arthropod assemblages in a Spartina salt marsh following removal of the invasive plant phragmites australis. Restor. Ecol. 13, 358–372. doi: 10.1111/j.1526-100X.2005.00045.x
Gray, P. C., Fleishman, A. B., Klein, D. J., Mckown, M. W., Bézy, V. S., Lohmann, K. J., et al. (2019). A convolutional neural network for detecting sea turtles in drone imagery. Methods Ecol. Evol. 10, 345–355. doi: 10.1111/2041-210X.13132
Gray, P. C., Ridge, J. T., Poulin, S. K., Seymour, A. C., Schwantes, A. M., Swenson, J. J., et al. (2018). Integrating drone imagery into high resolution satellite remote sensing assessments of estuarine environments. Remote Sens. 10:1257. doi: 10.3390/rs10081257
Halpern, B. S., Walbridge, S., Selkoe, K. A., Kappel, C. V., Micheli, F., D’Agrosa, C., et al. (2008). A global map of human impact on marine ecosystems. Science 319, 948–952. doi: 10.1126/science.1149345
Han, Y. G., Yoo, S. H., and Kwon, O. (2017). Possibility of applying unmanned aerial vehicle (UAV) and mapping software for the monitoring of waterbirds and their habitats. J. Ecol. Environ. 41, 1–7. doi: 10.1186/s41610-017-0040-5
Hardin, P., and Jensen, R. (2011). Small-scale unmanned aerial vehicles in environmental remote sensing: challenges and opportunities. GIScience Remote Sens. 48, 99–111. doi: 10.2747/1548-1603.48.1.99
Hardin, P. J., Lulla, V., Jensen, R. R., and Jensen, J. R. (2019). Small Unmanned Aerial Systems (sUAS) for environmental remote sensing: challenges and opportunities revisited. GIScience Remote Sens. 56, 309–322. doi: 10.1080/15481603.2018.1510088
Harris, J. M., Nelson, J. A., Rieucau, G., and Broussard, W. P. (2019). Use of drones in fishery science. Trans. Am. Fish. Soc. 148, 687–697. doi: 10.1002/tafs.10168
Harvey, M. C., Hare, D. K., Hackman, A., Davenport, G., Haynes, A. B., Helton, A., et al. (2019). Evaluation of stream and wetland restoration using UAS-based thermal infrared mapping. Water 11, 1–13. doi: 10.3390/w11081568
Hengstmann, E., Gräwe, D., Tamminga, M., and Fischer, E. K. (2017). Marine litter abundance and distribution on beaches on the Isle of Rügen considering the influence of exposition, morphology and recreational activities. Mar. Pollut. Bull. 115, 297–306. doi: 10.1016/j.marpolbul.2016.12.026
Hladik, C., Schalles, J., and Alber, M. (2013). Salt marsh elevation and habitat mapping using hyperspectral and LIDAR data. Remote Sens. Environ. 139, 318–330. doi: 10.1016/j.rse.2013.08.003
Hodgson, J. C., Baylis, S. M., Mott, R., Herrod, A., and Clarke, R. H. (2016). Precision wildlife monitoring using unmanned aerial vehicles. Sci. Rep. 6, 1–7. doi: 10.1038/srep22574
Hodgson, J. C., Mott, R., Baylis, S. M., Pham, T. T., Wotherspoon, S., Kilpatrick, A. D., et al. (2018). Drones count wildlife more accurately and precisely than humans. Methods Ecol. Evol. 9, 1160–1167. doi: 10.1111/2041-210X.12974
Husson, E. (2016). Images from unmanned aircraft systems for surveying aquatic and riparian vegetation. Acta Univ. Agric. Sueciae 115:53.
Island Conservation (2019). Ecological Restoration of North Seymour Island Underway Thanks to Rat Removal. Available online at: https://www.islandconservation.org/ecological-restoration-north-seymour-island-drone-rat-removal/ (accessed February 28, 2020).
Jiménez López, J., and Mulero-Pázmány, M. (2019). Drones for conservation in protected areas: present and Future. Drones 3:10. doi: 10.3390/drones3010010
Johnston, D. W. (2019). Unoccupied aircraft systems in marine science and conservation. Ann. Rev. Mar. Sci. 11, 439–463. doi: 10.1146/annurev-marine-010318-095323
Johnston, D. W., Dale, J., Murray, K. T., Josephson, E., Newton, E., and Wood, S. (2017). Comparing occupied and unoccupied aircraft surveys of wildlife populations: assessing the gray seal (Halichoerus grypus) breeding colony on Muskeget Island, USA. J. Unmanned Veh. Syst. 5, 178–191. doi: 10.1139/juvs-2017-0012
Joyce, K. E., Duce, S., Leahy, S. M., Leon, J., and Maier, S. W. (2018). Principles and practice of acquiring drone-based image data in marine environments. Mar. Freshw. Res. 70, 952–963. doi: 10.1071/MF17380
Kalacska, M., Chmura, G. L., Lucanus, O., Bérubé, D., and Arroyo-Mora, J. P. (2017). Structure from motion will revolutionize analyses of tidal wetland landscapes. Remote Sens. Environ. 199, 14–24. doi: 10.1016/j.rse.2017.06.023
Kalacska, M., Lucanus, O., Sousa, L., Vieira, T., and Arroyo-Mora, J. P. (2018). Freshwater fish habitat complexity mapping using above and underwater structure-from-motion photogrammetry. Remote Sens. 10:1912. doi: 10.3390/rs10121912
Kearney, M. S., Stutzer, D., Turpie, K., and Stevenson, J. C. (2009). The effects of tidal inundation on the reflectance characteristics of coastal marsh vegetation. J. Coast. Res. 256, 1177–1186. doi: 10.2112/08-1080.1
Kislik, C., Dronova, I., and Kelly, M. (2018). UAVs in support of algal bloom research: a review of current applications and future opportunities. Drones 2:35. doi: 10.3390/drones2040035
Kiszka, J. J., Mourier, J., Gastrich, K., and Heithaus, M. R. (2016). Using unmanned aerial vehicles (UAVs) to investigate shark and ray densities in a shallow coral lagoon. Mar. Ecol. Prog. Ser. 560, 237–242. doi: 10.3354/meps11945
Klemas, V. V. (2015). Coastal and environmental remote sensing from unmanned aerial vehicles: an overview. J. Coast. Res. 315, 1260–1267. doi: 10.2112/JCOASTRES-D-15-00005.1
Krause, D. J., Hinke, J. T., Perryman, W. L., Goebel, M. E., and LeRoi, D. J. (2017). An accurate and adaptable photogrammetric approach for estimating the mass and body condition of pinnipeds using an unmanned aerial system. PLoS One 12:e0187465. doi: 10.1371/journal.pone.0187465
Lally, H. T., O’Connor, I., Jensen, O. P., and Graham, C. T. (2019). Can drones be used to conduct water sampling in aquatic environments? A review. Sci. Total Environ. 670, 569–575. doi: 10.1016/j.scitotenv.2019.03.252
Linchant, J., Lisein, J., Semeki, J., Lejeune, P., and Vermeulen, C. (2015). Are unmanned aircraft systems (UASs) the future of wildlife monitoring? A review of accomplishments and challenges. Mamm. Rev. 45, 239–252. doi: 10.1111/mam.12046
Long, N., Millescamps, B., Guillot, B., Pouget, F., and Bertin, X. (2016). Monitoring the topography of a dynamic tidal inlet using UAV imagery. Remote Sens. 8, 1–18. doi: 10.3390/rs8050387
Lowe, M. K., Adnan, F. A. F., Hamylton, S. M., Carvalho, R. C., and Woodroffe, C. D. (2019). Assessing reef-island shoreline change using UAV-derived orthomosaics and digital surface models. Drones 3:44. doi: 10.3390/drones3020044
Mancini, F., Dubbini, M., Gattelli, M., Stecchi, F., Fabbri, S., and Gabbianelli, G. (2013). Using unmanned aerial vehicles (UAV) for high-resolution reconstruction of topography: the structure from motion approach on coastal environments. Remote Sens. 5, 6880–6898. doi: 10.3390/rs5126880
Manfreda, S., Mccabe, M. F., Miller, P. E., Lucas, R., Madrigal, V. P., Mallinis, G., et al. (2018). On the use of unmanned aerial systems for environmental monitoring. Remote Sens. 10:641. doi: 10.3390/rs10040641
Marcaccio, J. V., Markle, C. E., and Chow-Fraser, P. (2015). Unmanned aerial vehicles produce high-resolution, seasonally-relevant imagery for classifying wetland vegetation. Int. Arch. Photogramm. Remote Sens. Spat. Inf. Sci. ISPRS Arch. 40, 249–256. doi: 10.5194/isprsarchives-XL-1-W4-249-2015
Marteau, B., Vericat, D., Gibbins, C., Batalla, R. J., and Green, D. R. (2017). Application of Structure-from-Motion photogrammetry to river restoration. Earth Surf. Process. Landforms 42, 503–515. doi: 10.1002/esp.4086
Martin, C., Parkes, S., Zhang, Q., Zhang, X., McCabe, M. F., and Duarte, C. M. (2018). Use of unmanned aerial vehicles for efficient beach litter monitoring. Mar. Pollut. Bull. 131, 662–673. doi: 10.1016/j.marpolbul.2018.04.045
Marvin, D. C., Koh, L. P., Lynam, A. J., Wich, S., Davies, A. B., Krishnamurthy, R., et al. (2016). Integrating technologies for scalable ecology and conservation. Glob. Ecol. Conserv. 7, 262–275. doi: 10.1016/j.gecco.2016.07.002
Meng, X., Shang, N., Zhang, X., Li, C., Zhao, K., Qiu, X., et al. (2017). Photogrammetric UAV mapping of terrain under dense coastal vegetation: an object-oriented classification ensemble algorithm for classification and terrain correction. Remote Sens. 9, 1–23. doi: 10.3390/rs9111187
Morris, J. T., Sundareshwar, P. V., Nietch, C. T., Kjerfve, B., and Cahoon, D. R. (2002). Responses of coastal wetlands to rising sea level. Ecology 83, 2869–2877. doi: 10.1890/0012-9658(2002)083[2869:rocwtr]2.0.co;2
Mulero-Pázmány, M., Jenni-Eiermann, S., Strebel, N., Sattler, T., Negro, J. J., and Tablado, Z. (2017). Unmanned aircraft systems as a new source of disturbance for wildlife: a systematic review. PLoS One 12:e0178448. doi: 10.1371/journal.pone.0178448
Mulero-Pázmány, M., Stolper, R., Van Essen, L. D., Negro, J. J., and Sassen, T. (2014). Remotely piloted aircraft systems as a rhinoceros anti-poaching tool in Africa. PLoS One 9:e0083873. doi: 10.1371/journal.pone.0083873
Nahirnick, N. K., Hunter, P., Costa, M., Schroeder, S., and Sharma, T. (2019). Benefits and challenges of UAS imagery for eelgrass (Zostera marina) mapping in small estuaries of the canadian west coast. J. Coast. Res. 35:673. doi: 10.2112/jcoastres-d-18-00079.1
NCDOT (2019). NCDOT Using Drones for Cleaner, Safer Herbicide Spraying. North Carolina Dep. Transp. Available online at: https://www.ncdot.gov/news/press-releases/Pages/2019/2019-10-01-drone-herbicide-spray.aspx (accessed February 18, 2020).
NFWF (2019). Emergency Coastal Resilience Fund. Appendices A-B. Natl. Fish Wildl. Found. Available online at: https://www.nfwf.org/programs/emergency-coastal-resilience-fund (Accessed February 15, 2020).
Nowlin, M. B., Roady, S. E., Newton, E., and Johnston, D. W. (2019). Applying unoccupied aircraft systems to study human behavior in marine science and conservation programs. Front. Mar. Sci. 6:567. doi: 10.3389/fmars.2019.00567
Nyman, J. A., and Chabreck, R. H. (1995). Fire in Coastal Marshes: History and Recent Concerns. Available online at: http://talltimbers.org/wp-content/uploads/2014/03/NymanandChabreck1995_op.pdf (accessed February 15, 2020).
Palma, D., Zolich, A., Jiang, Y., and Johansen, T. A. (2017). Unmanned aerial vehicles as data mules: an experimental assessment. IEEE Access 5, 24716–24726. doi: 10.1109/ACCESS.2017.2769658
Parsons, M., Bratanov, D., Gaston, K. J., and Gonzalez, F. (2018). UAVs, hyperspectral remote sensing, and machine learning revolutionizing reef monitoring. Sensors 18, 1–20. doi: 10.3390/s18072026
Peters, A. (2019). These Tree-Planting Drones are Firing Seed Missiles to Restore the World’s Forests. Available online at: https://www.fastcompany.com/90329982/these-tree-planting-drones-are-firing-seed-missiles-to-restore-the-worlds-forests (accessed February 1, 2020).
Pettorelli, N., Laurance, W. F., O’Brien, T. G., Wegmann, M., Nagendra, H., and Turner, W. (2014). Satellite remote sensing for applied ecologists: opportunities and challenges. J. Appl. Ecol. 51, 839–848. doi: 10.1111/1365-2664.12261
Pimm, S. L., Alibhai, S., Bergl, R., Dehgan, A., Giri, C., Jewell, Z., et al. (2015). Emerging technologies to conserve biodiversity. Trends Ecol. Evol. 30, 685–696. doi: 10.1016/j.tree.2015.08.008
Potter, B., Valentino, G., Yates, L., Benzing, T., and Salman, A. (2019). “Environmental monitoring using a drone-enabled wireless sensor network,” in Proceedings of the 2019 Systems and Information Engineering Design Symposium (SIEDS), Charlottesville, VA, 1–6.
Powers, C., Hanlon, R., and Schmale, D. G. (2018). Tracking of a fluorescent dye in a freshwater lake with an unmanned surface vehicle and an unmanned aircraft system. Remote Sens. 10:81. doi: 10.3390/rs10010081
Ramos, E. A., Maloney, B., Magnasco, M. O., and Reiss, D. (2018). Bottlenose dolphins and Antillean manatees respond to small multi-rotor unmanned aerial systems. Front. Mar. Sci. 5:316. doi: 10.3389/fmars.2018.00316
Raoult, V., Tosetto, L., and Williamson, J. (2018). Drone-based high-resolution tracking of aquatic vertebrates. Drones 2:37. doi: 10.3390/drones2040037
Rees, A. F., Avens, L., Ballorain, K., Bevan, E., Broderick, A. C., Carthy, R. R., et al. (2018). The potential of unmanned aerial systems for sea turtle research and conservation: a review and future directions. Endanger. Species Res. 35, 81–100. doi: 10.3354/esr00877
Resop, J. P., Lehmann, L., and Hession, W. C. (2019). Drone laser scanning for modeling riverscape topography and vegetation: comparison with traditional aerial lidar. Drones 3:35. doi: 10.3390/drones3020035
Ridge, J. T., Gray, P. C., Windle, A. E., and Johnston, D. W. (2020). Deep learning for coastal resource conservation: automating detection of shellfish reefs. Remote Sens. Ecol. Conserv. 1–10. doi: 10.1002/rse2.134
Ridge, J. T., Rodriguez, A. B., and Fodrie, F. J. (2017). Salt marsh and fringing oyster reef transgression in a shallow temperate estuary: implications for restoration, conservation and blue carbon. Estuar. Coasts 40, 1013–1027. doi: 10.1007/s12237-016-0196-8
Ridge, J. T., Rodriguez, A. B., Joel Fodrie, F., Lindquist, N. L., Brodeur, M. C., Coleman, S. E., et al. (2015). Maximizing oyster-reef growth supports green infrastructure with accelerating sea-level rise. Sci. Rep. 5:14785. doi: 10.1038/srep14785
Rieucau, G., Kiszka, J. J., Castillo, J. C., Mourier, J., Boswell, K. M., and Heithaus, M. R. (2018). Using unmanned aerial vehicle (UAV) surveys and image analysis in the study of large surface-associated marine species: a case study on reef sharks Carcharhinus melanopterus shoaling behaviour. J. Fish Biol. 93, 119–127. doi: 10.1111/jfb.13645
Rodriguez, A. B., Fodrie, F. J., Ridge, J. T., Lindquist, N. L., Theuerkauf, E. J., Coleman, S. E., et al. (2014). Oyster reefs can outpace sea-level rise. Nat. Clim. Chang. 4, 493–497. doi: 10.1038/NCLIMATE2216
Rodriguez, R., Leary, J. J. K., and Jenkins, D. M. (2017). “Enhancing invasive species control with unmanned aerial systems and herbicide ballistic technology,” in Proceedings of the 2017 ASABE Annu. Int. Meet, Mânoa, 1–5.
Samiappan, S., Turnage, G., Hathcock, L. A., and Moorhead, R. (2017). Mapping of invasive phragmites (common reed) in Gulf of Mexico coastal wetlands using multispectral imagery and small unmanned aerial systems. Int. J. Remote Sens. 38, 2861–2882. doi: 10.1080/01431161.2016.1271480
Schofield, G., Katselidis, K. A., Lilley, M. K. S., Reina, R. D., and Hays, G. C. (2017a). Detecting elusive aspects of wildlife ecology using drones: new insights on the mating dynamics and operational sex ratios of sea turtles. Funct. Ecol. 31, 2310–2319. doi: 10.1111/1365-2435.12930
Schofield, G., Papafitsoros, K., Haughey, R., and Katselidis, K. (2017b). Aerial and underwater surveys reveal temporal variation in cleaning-station use by sea turtles at a temperate breeding area. Mar. Ecol. Prog. Ser. 575, 153–164. doi: 10.3354/meps12193
Seymour, A. C., Dale, J., Hammill, M., Halpin, P. N., and Johnston, D. W. (2017). Automated detection and enumeration of marine wildlife using unmanned aircraft systems (UAS) and thermal imagery. Sci. Rep. 7, 1–10. doi: 10.1038/srep45127
Seymour, A. C., Ridge, J. T., Newton, E., Rodriguez, A. B., and Johnston, D. W. (2019). Geomorphic response of inlet barrier islands to storms. Geomorphology 339, 127–140. doi: 10.1016/j.geomorph.2019.04.021
Seymour, A. C., Ridge, J. T., Rodriguez, A. B., Newton, E., Dale, J., and Johnston, D. W. (2018). Deploying fixed wing unoccupied aerial systems (UAS) for coastal morphology assessment and management. J. Coast. Res. 34, 704–717. doi: 10.2112/JCOASTRES-D-17-00088.1
Shuman, C. S., and Ambrose, R. F. (2003). A comparison of remote sensing and ground-based methods for monitoring wetland restoration success. Restor. Ecol. 11, 325–333. doi: 10.1046/j.1526-100X.2003.00182.x
Silvestri, S., Marani, M., and Marani, A. (2003). Hyperspectral remote sensing of salt marsh vegetation, morphology and soil topography. Phys. Chem. Earth 28, 15–25. doi: 10.1016/S1474-7065(03)00004-4
Smith, C. S., Puckett, B., Gittman, R. K., and Peterson, C. H. (2018). Living shorelines enhanced the resilience of saltmarshes to Hurricane Matthew (2016). Ecol. Appl. 28, 871–877. doi: 10.1002/eap.1722
Stone, E. (2017). Drones Spray Tree Seeds From the Sky to Fight Deforestation. Available online at: https://www.nationalgeographic.com/news/2017/11/drones-plant-trees-deforestation-environment/ (accessed February 1, 2020).
Sykora-Bodie, S. T., Bezy, V., Johnston, D. W., Newton, E., and Lohmann, K. J. (2017). Quantifying nearshore sea turtle densities: applications of unmanned aerial systems for population assessments. Sci. Rep. 7, 1–7. doi: 10.1038/s41598-017-17719-x
Torres, L. G., Nieukirk, S. L., Lemos, L., and Chandler, T. E. (2018). Drone up! Quantifying whale behavior from a new perspective improves observational capacity. Front. Mar. Sci. 5:319. doi: 10.3389/fmars.2018.00319
Torres, W., and Bierlich, K. (2020). MorphoMetriX: a photogrammetric measurement GUI for morphometric analysis of megafauna. J. Open Source Softw. 5:1825. doi: 10.21105/joss.01825
Trevathan, J., and Johnstone, R. (2018). Smart environmental monitoring and assessment. Sensors 18:2248. doi: 10.3390/s18072248
Turner, I. L., Harley, M. D., and Drummond, C. D. (2016). UAVs for coastal surveying. Coast. Eng. 114, 19–24. doi: 10.1016/j.coastaleng.2016.03.011
Turner, W., Rondinini, C., Pettorelli, N., Mora, B., Leidner, A. K., Szantoi, Z., et al. (2015). Free and open-access satellite data are key to biodiversity conservation. Biol. Conserv. 182, 173–176. doi: 10.1016/j.biocon.2014.11.048
U.S. Department of the Interior [USDOI] (2020). Order No. 3379: Temporary Cessation of Non-Emergency Unmanned Aircraft Systems Fleet Operations. Washington, DC: U.S. Department of the Interior.
Ventura, D., Bonifazi, A., Gravina, M. F., Belluscio, A., and Ardizzone, G. (2018). Mapping and classification of ecologically sensitive marine habitats using unmanned aerial vehicle (UAV) imagery and Object-Based Image Analysis (OBIA). Remote Sens. 10, 1–23. doi: 10.3390/rs10091331
Ventura, D., Bruno, M., Jona Lasinio, G., Belluscio, A., and Ardizzone, G. (2016). A low-cost drone based application for identifying and mapping of coastal fish nursery grounds. Estuar. Coast. Shelf Sci. 171, 85–98. doi: 10.1016/j.ecss.2016.01.030
Vogt, M. C., and Vogt, M. E. (2016). Near-Remote Sensing of Water Turbidity Using Small Unmanned Aircraft Systems. Environ. Pract. 18, 18–31. doi: 10.1017/S1466046615000459
Warfield, A. D., and Leon, J. X. (2019). Estimating mangrove forest volume using terrestrial laser scanning and UAV-derived structure-from-motion. Drones 3:32. doi: 10.3390/drones3020032
WeRobotics (2020). The Flying Labs Network Builds on Local Expertise for Local Solutions. Available online at: https://flyinglabs.org/ (accessed May 12, 2020).
Willms, T., and Whitworth, G. (2016). Mapping of critical summer thermal refuge habitats for Chinook salmon, Coho salmon, steelhead and bull trout in the Nicola River Watershed - 2016. Habitat Steward. Progr. Species Risk 3, 2–8.
Windle, A. E., Poulin, S. K., Johnston, D. W., and Ridge, J. T. (2019). Rapid and accurate monitoring of intertidal Oyster Reef Habitat using unoccupied aircraft systems and structure from motion. Remote Sens. 11:2394. doi: 10.3390/rs11202394
Wu, K., Rodriguez, G. A., Zajc, M., Jacquemin, E., Clément, M., De Coster, A., et al. (2019). A new drone-borne GPR for soil moisture mapping. Remote Sens. Environ. 235:111456. doi: 10.1016/j.rse.2019.111456
Keywords: UAS, drones, marine ecosystem, restoration, conservation, living shoreline
Citation: Ridge JT and Johnston DW (2020) Unoccupied Aircraft Systems (UAS) for Marine Ecosystem Restoration. Front. Mar. Sci. 7:438. doi: 10.3389/fmars.2020.00438
Received: 09 March 2020; Accepted: 19 May 2020;
Published: 12 June 2020.
Edited by:
Tjisse Van Der Heide, Royal Netherlands Institute for Sea Research (NIOZ), NetherlandsReviewed by:
Ana B. Bugnot, The University of Sydney, AustraliaMatthew David Tietbohl, King Abdullah University of Science and Technology, Saudi Arabia
Copyright © 2020 Ridge and Johnston. This is an open-access article distributed under the terms of the Creative Commons Attribution License (CC BY). The use, distribution or reproduction in other forums is permitted, provided the original author(s) and the copyright owner(s) are credited and that the original publication in this journal is cited, in accordance with accepted academic practice. No use, distribution or reproduction is permitted which does not comply with these terms.
*Correspondence: Justin T. Ridge, justin.ridge@duke.edu