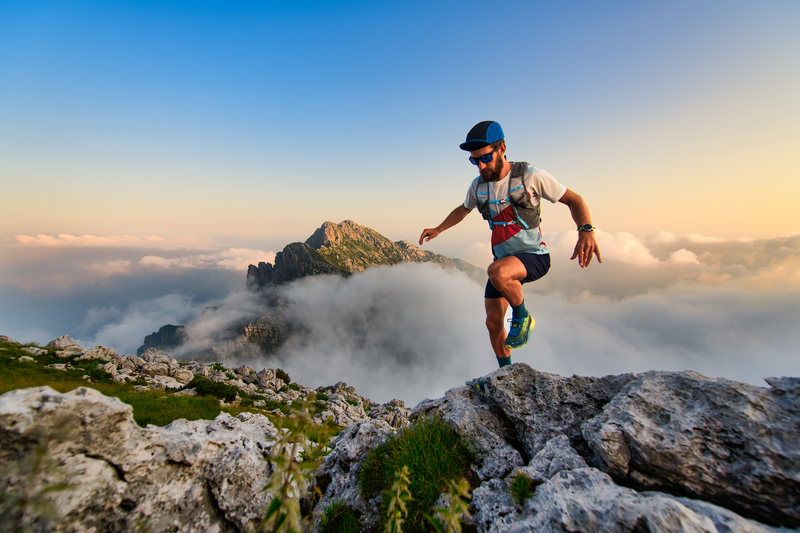
95% of researchers rate our articles as excellent or good
Learn more about the work of our research integrity team to safeguard the quality of each article we publish.
Find out more
ORIGINAL RESEARCH article
Front. Mar. Sci. , 26 May 2020
Sec. Marine Megafauna
Volume 7 - 2020 | https://doi.org/10.3389/fmars.2020.00353
This article is part of the Research Topic Small Cetacean Conservation: Current Challenges and Opportunities View all 51 articles
Globally, the exploitation of marine mammals has shifted from hunting to viewing over the last few decades. While refraining from actively killing animals may have a positive effect on marine mammal populations, whale and dolphin watching can induce changes such as displacement from preferred habitat and disruption of foraging that may also have severe fitness costs. Under some circumstances, this non-lethal disturbance may affect populations in a manner similar to directed mortality. Here, we focus on inshore dolphin populations that are known to show short-term behavioral responses to boat approaches. Long-term fitness effects have only been clearly identified in a small number of these populations, and all share certain characteristics, i.e., closed, small and food-limited. This raises the question of importance of context when considering the long-term effects of disturbance, since many dolphin populations may be open, large, and/or free from resource restriction. We explored the effect of disturbance based on the characteristics of populations using the population consequences of disturbance (PCoD) framework. PCoD was developed to link short-term changes in individual behavior and physiology to presumed long-term effects on population dynamics. To ensure our scenarios were biologically plausible, they reflected the ecological context of four well-studied populations of dolphins, Doubtful Sound, New Zealand, Sarasota Bay, United States, Durban Bay, South Africa, and Jervis Bay, Australia, in terms of their size, closure, and food resources. We found that the characteristics of the populations being disturbed are important with regards to the level of disturbance that could be tolerated. Closed populations were most sensitive, while large, open populations with no food limitation appeared to be able to withstand a higher probability of disturbance. This implies that population characteristics should be accounted for when determining the suitability of whale and dolphin watching operations in a given area.
The exploitation of wildlife has undergone a fundamental change in many parts of the world over the last few decades (Dickman, 2010). From destruction of wildlife as pests (Pimentel et al., 2005), threats to humans or livestock (Loe and Roskaft, 2004; Packer et al., 2005), trophies from safaris (Di Minin et al., 2016) or harvest for food (Weinbaum et al., 2013), there has been a significant shift to viewing rather than killing wildlife. Indisputably, refraining from actively killing animals has an immediate and measurable positive effect on a population’s survival and abundance, as well as habitats and ecosystem processes (e.g., Ripple and Beschta, 2012). However, wildlife viewing can negatively impact populations (Abd Mutalib, 2018), and can induce subtle changes such as long-term displacement from preferred habitats (Klein et al., 1995; Steckenreuter et al., 2012) or disruption of foraging (Steckenreuter et al., 2011; Pirotta et al., 2015). Other essential behaviors can also be perturbed, such as nesting or caring for offspring (Frid, 2003; McClung et al., 2004), sometimes with severe fitness costs (Gill et al., 2001; Coetzee and Chown, 2016), where fitness is defined as all intrinsic factors affecting individuals’ ability to successfully produce young. Ultimately, in some circumstances, non-lethal effects may have a similar, or even larger, influence on populations than direct mortality (Preisser et al., 2005; Zanette et al., 2011).
Across the diverse spheres of wildlife tourism, whale and dolphin watching has grown more rapidly and globally in popularity than most, and since the first decade of the 21st century, most coastal cetacean populations have been exposed to some form of whale-watching (e.g., O’Connor et al., 2009; Mallard, 2019). This growth has been driven by the recovery, and therefore the availability, of the great whales following the end of large-scale commercial whaling, their emblematic status in the developing green movement of the 1960s and 1970s, and by the popularity of dolphins arising from widespread change in attitudes in western countries through popular media and assertions regarding dolphin intelligence (Lavigne et al., 1999; Simmonds, 2011; Wearing et al., 2011). While whale-watching was initially welcomed as a more “benign” alternative to whaling, concerns have arisen that the rise of high intensity watching may negatively affect whales and dolphins (e.g., Higham et al., 2014; New et al., 2015; Rodríguez-Izquierdo et al., 2019; Sprogis et al., 2020).
Over the past two decades, numerous studies have identified short-term behavioral responses of inshore dolphin populations to boat approaches (e.g., Stensland and Berggren, 2007; Stockin et al., 2008; Senigaglia et al., 2016; Cecchetti et al., 2018). However, identification of long-term fitness effects has been less forthcoming, in part due to the difficulty of quantifying the fitness of animals that spend their entire lives at sea. Although fitness effects are difficult to measure, and analyze, they have been identified, with behavioral disturbances implicated in decreased female reproductive success and a consequent reduction in population size for at least one dolphin population (Lusseau et al., 2006).
An alternative to the difficult task of attributing specific fitness costs empirically is known as the population consequences of disturbance (PCoD) framework (New et al., 2014; Pirotta et al., 2018). This framework was developed to link short-term changes in individual behavior and physiology to potential long-term effects on population dynamics. An advantage over earlier approaches is its ability to incorporate a wide range of phenomenological, mechanistic, and hypothesized links, such as unobservable changes in individuals (e.g., New et al., 2013). The PCoD framework distinguishes disturbances that have an acute, immediate effect on vital rates (e.g., collision with a vessel, entanglement in a fishing net) from chronic disturbances (e.g., whale-watching) that alter vital rates by affecting an individual’s health, defined as all internal factors that affect homeostasis (New et al., 2013). Health is then the principal avenue through which to assess the indirect effects of whale-watching on species’ vital rates, and hence population dynamics. This approach provides us with the capacity to ask whether observed disturbance effects are invariably generalizable, i.e., whether measurable short-term disturbance will always have fitness effects, or whether there are specific characteristics of a population that make them vulnerable, or, alternatively, robust, to disturbance effects.
Small populations are inherently more vulnerable to the effects of disturbance than larger populations (O’Grady et al., 2004; Manlik et al., 2016). To date, fitness effects on dolphin populations attributable to disturbance have only been clearly identified in a few populations, all of which share certain characteristics, namely being closed to immigration and emigration, small and food-limited (Doubtful Sound dolphins, Lusseau et al., 2006; East African dolphins, Pérez-Jorge et al., 2016). This raises the question of whether the fitness effects are a function of the population or a limitation of our tools and the populations we choose to study. Large populations that are open to immigration and emigration are often less well studied, as the focus of management and conservation is often on smaller, declining populations (New et al., 2015). Larger populations may also be less sensitive to tourism effects as they are buffered by the relatively lower disturbance experienced at the individual level. Populations largely free from resource constraints, such as in Sarasota Bay, Florida, may also be able to compensate for the effects of disturbances (Lusseau, 2014; Nattrass and Lusseau, 2016), since greater food resources and overall lower exposure per capita may offset any disruption to foraging behavior.
In this article, we address whether the effect of disturbance is a function of a population’s characteristics, using stochastic simulations of four dolphin populations that represent variation across three crucial characteristics: size, food limitation, and whether a population is open or closed. Within these contexts, dolphins engage in different behaviors based upon internal drivers (e.g., physical condition) that must be satisfied. The constraints the ecological context places upon individuals’ ability to fill these needs also impacts dolphins’ response to disturbance, contributing to their ability to avoid a stressor and compensate for a negative response. Individual-based models have been used to explore the relationship between internal drivers and behavior, and have proven able to simulate realistic dynamics (e.g., New et al., 2013; Pirotta et al., 2014, 2019). Here we take advantage of an existing model for dolphin populations (New et al., 2013) and generalize it to investigate broad patterns in population response to disturbance.
We used the individual based model presented in New et al. (2013) to investigate dolphin populations’ responses to disturbance. The model allowed the intrinsic motivational state of individuals (e.g., fear) to affect their physical condition and choice of behaviors, even when such changes may not be apparent from the observed activity. Each individual in a population was defined as being either a juvenile, mother with calf, or adult, since each was assumed to affect the fission-fusion behavior observed in dolphins (e.g., Möller et al., 2006) and result in different cost-benefits between internal drivers and the behavior in which the dolphin takes part (New et al., 2013).
Individuals formed schools, whose behavior (traveling, foraging, resting, and socializing) at time t (bt) was assumed to be an observation on a true underlying activity (at). Every individual (i) within a group was assumed to be taking part in the same activity, which was itself dependent on the motivations (i.e., fear, hunger, condition, social desire) of the individual members of the group at time t (mi,t). The individuals’ motivations changed through time according to the activity in which the dolphins engaged and an effect of the season (st, warm or cold):
where A is a k by k matrix of the cost-benefit of each activity on the motivations, mi,t, ai,t–1, and d are all vectors of length k and st is a scalar. The only exception was the individuals’ condition at time t (mc,i,t), which was dependent on the individuals’ hunger (mh,i,t), thus changing at a slower rate than the other motivations (New et al., 2013).
The school’s activity at time t was chosen based on the desires (qi,t) of the individuals within the school to take part in each activity, which was a function of individual motivations and the effect of season (r). We averaged these individual desires (mt, a vector whose length is equal to the number of activities, k) and used mt to calculate the probability (pa,t) that the school would take part in a given activity.
where G is a k by k matrix indicating the strength of the relationship between motivations and activities and qi,t, pa,t, and r are all vectors of length k. The school’s activity was then chosen according to a multinomial distribution,
which gave a vector of length k where the chosen activity was equal to 1 and all other values were zero. For additional details, see New et al. (2013) and Pirotta et al. (2014).
Dolphins are social animals that live in schools whose membership and size can vary on a regular basis (Connor et al., 2000; Lusseau et al., 2003; Möller et al., 2006). Known as fission-fusion behavior, we incorporated this breaking up and reforming of schools into the model, assuming a dolphin’s decision to leave their current school was dependent on school size and overall satisfaction with levels of hunger, fear, and social desire. If fission occurred, the dolphin and the individual in their current school with whom they were most closely associated would seek to find an alternate school together.
We assumed fusion was dependent on the new school’s current activity and the level of association between schools. Association was determined by a randomly generated correlation matrix that accounted for the fact that individuals of different classes (mother with calf, adult, juvenile) will share different levels of association (e.g., mothers with calves are more likely to school together than with other adults) (Möller et al., 2006; McHugh et al., 2011; New et al., 2013).
New et al. (2013) originally built the model for the population of bottlenose dolphins (Tursiops truncatus) in the Moray Firth, United Kingdom, using a spatial map of the Firth to direct the dolphins’ movement and determine where certain behaviors (e.g., foraging) could take place, based on environmental features. However, the aim of this study was not to assess the management or conservation needs of a specific population, but rather to investigate how populations’ response to disturbance may be influenced by the broad-scale ecological contexts in which dolphins exist. Therefore, to disentangle the effects of any fine-scale environmental features from those of the populations’ characteristics, we simplified the spatial component of the model. Using an approach common in many dynamic energy budget models (e.g., Hin et al., 2019), we retained traveling as an activity with an associated cost of movement, but without any defined spatial component (location, distance).
Resource limitation is often due to human exploitation of prey, patchy prey dynamics, or because prey occurs in locations with high perceived risk (e.g., predation), limiting the time dolphins can remain in that area (Torres and Read, 2009). Therefore, in these situations, the dolphins must move between locations to take part in different behaviors. For example, if the risk of predation is higher in foraging areas, individuals are unlikely to rest in those locations (Madin et al., 2016). Therefore, for food limited populations we modified the model to require individuals to travel for at least one-time step before switching between the other behaviors (foraging, resting, and socializing). We assumed individuals in populations without resource limitations were able to engage in any behavior without first traveling.
The probability that a school of dolphins would be disturbed on a given day was defined based upon a range of potential whale-watching intensities (e.g., Lusseau et al., 2009; Seely et al., 2017). For open populations, we assumed that only schools in the area used by tour boats were at risk of disturbance, and that 10–34% of the population were vulnerable to disturbance at any one point (Mahomed, 2008), with the exact percentage chosen stochastically each day. We also assumed all individuals within a school experienced the same external stimuli (e.g., disturbance, foraging conditions, etc.). Given that fission-fusion behavior changed the number and size of schools, we randomly selected the schools exposed to disturbance in an iterative fashion, summing the total number of individuals across all disturbed schools. When the total was equal to, or immediately greater than, the proportion of the population assumed to be vulnerable to disturbance on that day we ceased selecting schools. For the unselected schools, the risk of disturbance was zero. This process assumed there were no individual or school home ranges within the wider population, which may not always be the case.
For closed populations, we assumed the dolphins were unable to leave the general area in which tour boats were operating, but did have access to refugia (Lusseau and Higham, 2004; Steckenreuter et al., 2012). These scenarios followed a similar structure as to disturbance in the open populations with two main differences. First, we assumed that on any one day only 10–34% of the population could be in refugia, with the exact percentage chosen stochastically. Therefore, the majority of the population was always disturbed. In addition, while seeking refuge in these areas, we assumed that the dolphins foraging efficiency was reduced. We based this on the supposition that refugia are suboptimal habitat that would have a low probability of utilization in the absence of disturbance.
In the scenarios, disturbance is defined by the presence of a whale-watching vessel, and the dolphins’ fear is assumed to increase as vessels draw closer to the animals (Nowacek et al., 2001; New et al., 2013). The distance at which the dolphins detect the vessel is determined stochastically, and if the vessel is simulated as sharing the same location as the school, fear is assumed to outweigh all other motivations and the dolphins will switch from their current activity to traveling.
At the start of a simulation, we randomly assigned each individual in a population to be either a juvenile, mother with calf, or adult, as well as the level of association between individuals and the starting school membership, behavior and motivational states for each individual. Each stochastic simulation covered the time period of 1 year (365 days), with the season and its resulting effects on the model switching half way through the simulation (New et al., 2013).
To ensure that the ecological contexts being explored were biologically plausible, we chose the characteristics (size, open/closed, resource availability) of the populations to be simulated based upon four well-studied coastal dolphin populations (Tursiops spp.). The first population, small (61 individuals, Henderson, 2012), closed and food limited (Williams et al., 1993), was based upon Doubtful Sound, New Zealand (T. truncatus). The characteristics of the second closed population were taken from Sarasota Bay, Florida (T. truncatus), which is larger in size (160 individuals, Wells et al., 2015) and generally unaffected by food limitation excepting in years of red tides (Wells, 2014). The red tide years were not considered as part of the simulations for this population, since the food limitation would make the scenario too similar to that for Doubtful Sound. For a large (350 individuals, Natoli et al., 2008), open population with no food limitation (Roberts and Nieuwenhuys, 2016), we used the characteristics of the dolphins found in Durban Bay, South Africa (Tursiops aduncus). For this location there were multiple estimates of population size, including one that included over 900 individuals (Browning et al., 2014). We used and older estimate of (Natoli et al., 2008) because of the computational cost of an individual based model tracking over 900 individuals. Lastly, Jervis Bay, Australia (T. aduncus) provided the characteristics for a small (108 individuals, Möller et al., 2002), open population that is food limited (Armbrecht et al., 2014). For the sake of simplicity, we refer to the ecological context scenarios by the name of the population after which they were structured, although they are not meant to be replications of those populations.
For each of our ecological context scenarios, we investigated four disturbance regimes that were assumed to occur year-round. The baseline regime assumed the population was undisturbed, after which the probability of disturbance increased to 10, 25, or 50% for each simulated population. These values were chosen to represent realistic levels of whale-watching from around the world (e.g., Lusseau et al., 2009; Seely et al., 2017).
We used the statistical programming language R, version 3.6.0 (R Core Team, 2019) to run the separate disturbance regimes 100 times for each scenario. The code was bespoke, but made use of the packages poLCA (Lizner and Lewis, 2011) for the simulations and ggplot2 (Wickham, 2016) for plotting the figures. The results were based upon the cumulative outputs of each disturbance-context combination.
In the absence of disturbance from whale-watching vessels, the differences in activity budget and motivation across the ecological contexts were based solely upon food limitation. As we expected from the model structure, food limited populations spent more time traveling than those with readily available access to prey (Figure 1). When the chance of disturbance was 10%, we observed an increase in the time spent traveling and foraging in the activity budgets of the closed populations. We did not see a similar shift in the activity budget for an open (“Jervis Bay”) population until there was a 25% chance of disturbance, or for the largest of the four populations (350 individuals, Durban Bay) until there was a 50% chance of disturbance (Figure 1).
Figure 1. The activity time budgets for the four simulated populations (columns), Doubtful Sound (A–D), Sarasota Bay (E–H), Jervis Bay (I–L), and Durban Bay (M–P), under four different disturbance scenarios (rows): no disturbance (A,E,I,M) and a 10% (B,F,J,N), 25% (C,G,K,O), or 50% (D,H,L,P) chance of an individual being disturbed when in the area used by whale-watching vessels. The four behaviors in which the dolphins can take part are: traveling (Travel), resting (Rest), foraging (Feed), and socializing (Social).
We found that the motivational states showed a similar pattern to the activity budgets. While a 10% chance of disturbance had an effect on the motivational states of the dolphins in the closed populations, it took increasing amounts of disturbance to see a similar change in the simulations for the open populations (Figures 2–5). We did not find a substantial shift in individual condition for any of the simulated populations, despite the observed increase in fear and hunger. This was an artifact of the length of the simulations, which we only ran for 1 year. Condition changes at a slower pace than the more immediate motivations of fear, hunger or social need, so we would only expect to see a notable change in condition when the disturbance occurred over multiple years.
Figure 2. The four motivational states of condition (A,E,I,M), fear (B,F,J,N), hunger (C,G,K,O), and social need (D,H,L,P) for the simulated Doubtful Sound population under each of the four disturbance scenarios: no disturbance (A–D) and a 10% (E–H), 25% (I–L), or 50% (M–P) chance of an individual being disturbed when in the area used by whale-watching vessels. The y-axis is the cumulative count over all simulations, while the x-axis is a unit-less measure of individual motivation. Motivation is centered at zero (i.e., indifference) and can take on negative values to indicate a lack of incentive (i.e., satisfaction) and positive values to indicate strong incentive (i.e., discontent) (New et al., 2013).
Figure 3. The four motivational states of condition (A,E,I,M), fear (B,F,J,N), hunger (C,G,K,O), and social need (D,H,L,P) for the simulated Sarasota Bay population under each of the four disturbance scenarios: no disturbance (A–D) and a 10% (E–H), 25% (I–L), or 50% (M–P) chance of an individual being disturbed when in the area used by whale-watching vessels. The y-axis is the cumulative count over all simulations, while the x-axis is a unit-less measure of individual motivation. Motivation is centered at zero (i.e., indifference) and can take on negative values to indicate a lack of incentive (i.e., satisfaction) and positive values to indicate strong incentive (i.e., discontent) (New et al., 2013).
Figure 4. The four motivational states of condition (A,E,I,M), fear (B,F,J,N), hunger (C,G,K,O), and social need (D,H,L,P) for the simulated Jervis Bay population under each of the four disturbance scenarios: no disturbance (A–D) and a 10% (E–H), 25% (I–L), or 50% (M–P) chance of an individual being disturbed when in the area used by whale-watching vessels. The y-axis is the cumulative count over all simulations, while the x-axis is a unit-less measure of individual motivation. Motivation is centered at zero (i.e., indifference) and can take on negative values to indicate a lack of incentive (i.e., satisfaction) and positive values to indicate strong incentive (i.e., discontent) (New et al., 2013).
Figure 5. The four motivational states of condition (A,E,I,M), fear (B,F,J,N), hunger (C,G,K,O), and social need (D,H,L,P) for the simulated Durban Bay population under each of the four disturbance scenarios: no disturbance (A–D) and a 10% (E–H), 25% (I–L), or 50% (M–P) chance of an individual being disturbed when in the area used by whale-watching vessels. The y-axis is the cumulative count over all simulations, while the x-axis is a unit-less measure of individual motivation. Motivation is centered at zero (i.e., indifference) and can take on negative values to indicate a lack of incentive (i.e., satisfaction) and positive values to indicate strong incentive (i.e., discontent) (New et al., 2013).
The distribution of school sizes was also sensitive to the level of disturbance, but comparison of the four ecological contexts was difficult because the varying population sizes resulted in different definitions of small and large schools. However, we found that, generally, larger school sizes became less common with increasing disturbance (Figure 6).
Figure 6. The distribution of school sizes for the four simulated populations (columns), Doubtful Sound (A–D), Sarasota Bay (E–H), Jervis Bay (I–L), and Durban Bay (M–P), under four different disturbance scenarios (rows): no disturbance (A,E,I,M) and a 10% (B,F,J,N), 25% (C,G,K,O), or 50% (D,H,L,P) chance of an individual being disturbed when in the area used by whale-watching vessels (rows).
New et al.’s (2013) model had previously been extended to the population of bottlenose dolphins in Doubtful Sound (Pirotta et al., 2014), supporting the applicability of the model to populations other than that of the Moray Firth and its ability to account for a spatially constrained behavioral repertoire. The disturbance scenarios investigated in Pirotta et al. (2014) were more spatially complex than those explored here, and were specific to the tourism occurring in the area. In contrast, our goal was not to determine the effects of specific disturbances or population-specific behavioral constraints, but rather explore the role general population characteristics may have in regulating the effects of disturbance. Therefore, we generalized both the model structure, ecological contexts, and disturbance scenarios to enable us to make broad-scale comparisons, as opposed to population-specific predictions. However, useful future applications of our approach could include modeling specific disturbances for these populations, as well as exploring the potential interactions between the multiple types of disturbance to which the dolphins may be exposed (e.g., fishing pressures, commercial shipping, pollution, etc.).
Our results highlight the importance of population characteristics when considering the effect of disturbance on inshore dolphin populations. The two closed populations, which reflected the characteristics of the dolphins found in Doubtful Sound and Sarasota Bay, proved the most sensitive to disturbance, with only a 10% chance of encountering a tour boat having a notable effect on the dolphins’ behavioral time budget, motivations and school size (Figures 1–6). In contrast, the open populations, which mirrored the characteristics of the Jervis and Durban Bay dolphins, required higher probabilities of disturbance, 25 and 50%, respectively, to see the same shift in behavioral time budgets and motivations (Figures 1–5).
School size showed the least discernible differences between the disturbance scenarios (Figure 6). However, there were generally fewer larger schools when disturbance was present, regardless of the different population characteristics. Conversely, while there were fewer larger schools, they were comprised of a greater number of individuals. This is because we assumed fission-fusion at the school level was dependent on both school size and fear. We know that for all ungulate species (including cetaceans) aggregation is an anti-risk behavior, as it reduces risk per capita (Acevedo-Gutiérrez, 2002). However, there is a threshold at which the protection is no longer optimal because of the increased competition for resources and decreasing safety benefits (Lusseau et al., 2004). Therefore, the results show how individuals’ internal motivations, in this case an increase in both hunger and fear, resulted in a trade-off between protection and resource availability that was reflected in the change in school sizes.
The role of population characteristics in determining the effects of disturbance were more clearly seen in the dolphins’ activity time budgets and motivations. In particular, population size and closure appear to interact. Controlling for the effect of food limitation, population size did not seem to have a role in the effect of disturbance on the closed populations. This was likely because we assumed that all individuals outside of refugia were at the same risk regardless of population size. Given that many areas have restrictions on the number of whale-watching vessels and the hours they can operate, this assumption may be too conservative.
In contrast, population size played a role in the level of disturbance tolerated by open populations. Every dolphin school had an equal probability of being chosen as part of 10–34% of the population affected by disturbance at any time step. However, we would expect smaller populations to have fewer schools on average (Figure 6), so compared to a larger population, each school had a higher probability of being selected for exposure to disturbance. As a result, each individual in the smaller population (108 individuals, Möller et al., 2002) would be more frequently at-risk to whale-watching vessels, and thus was disturbed more often regardless of the fission-fusion rate. However, a portion of the population remained undisturbed at each time step, so regardless of their exact size, open populations still showed a lower sensitivity to disturbance than observed in the closed populations. It is possible that food limitation played a role in the smaller of the two open populations showing a lower threshold of disturbance. However, we saw a limited effect of prey availability in the closed populations, lending weight to the belief that the difference between the open populations in our simulations was due to their size.
The effect of food limitation appeared to be restricted to the activity time budgets, with food limited populations traveling more frequently than those without resource limitation. We found it surprising that prey availability did not appear to mitigate the effects of disturbance given that populations such as Sarasota Bay are known to be relatively stable, even given high levels of disturbance (Wells, 2014). This is likely a result of the model structure and implementation. The simulations covered the period of 1 year, over which we observed very little change in condition at any level of disturbance (Figures 2–5). Condition in long-lived, slow-reproducing species will change at a slower rate than the other motivations (e.g., New et al., 2013), so longer simulations, incorporating birth and death, would be needed to explore the interaction between food limitation and disturbance that resulted in consistently lost foraging opportunities. In addition, fear was the motivation that changed the most in response to disturbance (Figures 2–5), yet it had no direct effect on individuals’ condition. Given current research on the effects of stress on marine mammals (e.g., Atkinson et al., 2015; Champagne et al., 2018; Galligan et al., 2019), this aspect of the model may need to be revisited. Lastly, the lack of a spatial component to the model means that when food is limited we are unlikely to be adequately capturing the ephemeral or patchy nature of the prey distributions, potentially leading to an underestimate of the effect of disturbance on these populations. This highlights the importance of using population-specific models to predict the effect of explicitly defined disturbances when considering the use of models such as these for management or conservation purposes.
By separating the fine-scale effect of local environmental features from the broad-scale ecological context, we were able to begin exploring how population characteristics (resource availability, population size, open/closed) can interact to influence the consequences of disturbance. Crucially we found that these characteristics helped define the susceptibility to disturbance. Closed populations, being unable to avoid the disturbance, were the most sensitive, while open populations were able to withstand a higher probability of interacting with whale-watching vessels. Our results imply that population ecology plays an important role in the level of disturbance that can be tolerated and, in particular, the vulnerability of small, closed populations should be accounted for when determining the intensity of whale-watching operations for a given area.
It is unquestionable that wildlife tourism affects the activities of individual cetaceans (Senigaglia et al., 2016). However, given the difficulty in assessing how short-term changes in individual behavior affect populations in the long-term, the potential consequences of wildlife tourism are often extrapolated assuming the worst possible outcome and with little or no reference to the ecological context (e.g., Stockin et al., 2008; Parsons, 2012). It has been argued that selective pressure and removal of key individuals can severely affect population dynamics (e.g., Allendorf and Hard, 2009; Devine et al., 2012). However, tourism aimed at watching cetaceans is not deliberately targeting specific individuals within the populations to which they have access and there is evidence that wildlife tourism will not always lead to conservation concerns (Christiansen and Lusseau, 2015; Crespo et al., 2019). Therefore, while our results are consistent with population dynamics theory, it remains important to quantitatively demonstrate that the propensity for population consequences due to disturbances such as whale-watching depends on the characteristics of the population and the intensity of the disturbance. Although there are a limited number of cetacean populations for which this could be done, confirming these relationships where possible would permit more confident extrapolation for those situations that are data poor. The ability to place observed behavioral responses to whale-watching in their ecological context can help identify and prioritize those populations that will most benefit from management and conservation action. This will enable focused efforts on those populations at risk and increase successful conservation efforts.
The datasets generated for this study can be found in the FigShare repository https://figshare.com/articles/Simulations_ of_Dolphin_Behaviour_and_Motivations_in_Response_to_Dis- turbance/12298871.
RH planned for this work. LN performed the analysis and took the lead in drafting the manuscript. RH and DL provided the necessary biological context, and both contributed substantially to the writing of the manuscript.
RH supported in part by a Sitka Sound Science Centre Scientist in Residency Fellowship.
The authors declare that the research was conducted in the absence of any commercial or financial relationships that could be construed as a potential conflict of interest.
A draft of this manuscript was presented (manuscript SC66b/WW10) to the Whalewatching Sub-Committee at the 66th meeting of the Scientific Committee of the International Whaling Commission, 4–20 June 2016, in Bled, Slovenia.
Abd Mutalib, A. H. (2018). The photo frenzy phenomenon: how a single snap can affect wildlife populations. Biodiversity 19, 237–239.
Acevedo-Gutiérrez, A. (2002). Interactions between marine predators: dolphin food intake is related to number of sharks. Mar. Ecol. Prog. Ser. 240, 261–271.
Allendorf, F. W., and Hard, J. J. (2009). Human-induced evolution caused by unnatural selection through harvest of wild animals. Proc. Natl. Acad. Sci. U.S.A. 106, 9987–9994. doi: 10.1073/pnas.0901069106
Armbrecht, L. H., Roughan, M., Rossi, V., Schaeffer, A., Davies, P. L., Waite, A. M., et al. (2014). Phytoplankton composition under contrasting oceanographic conditions: upwelling and downwelling (Eastern Australia). Continent. Shelf Res. 75, 54–67.
Atkinson, S., Crocker, D., Houser, D., and Mashburn, K. (2015). Stress physiology in marine mammals: how well do they fit the terrestrial model? J. Compar. Physiol. B 185, 463–486. doi: 10.1007/s00360-015-0901-0
Browning, N. E., Cockcroft, V. G., and Worthy, G. A. J. (2014). Resource partitioning among South African delphinids. J. Exp. Mar. Biol. Ecol. 457, 15–21.
Cecchetti, A., Stockin, K. A., Gordon, J., and Azevedo, J. M. N. (2018). Short-term effects of tourism on the behaviour of common dolphins (Delphinus delphis). J. Mar. Biol. Assoc. U.K. 98, 1187–1196.
Champagne, C. D., Kellar, N. M., Trego, M. L., Delehanty, B., Boonstra, R., Wasser, S. K., et al. (2018). Comprehensive endocrine response to acute stress in the bottlenose dolphin from serum, blubber and feces. Gen. Comp. Endocrinol. 266, 178–193. doi: 10.1016/j.ygcen.2018.05.015
Christiansen, F., and Lusseau, D. (2015). Linking behaviour to vital rates to measure the effects of non-lethal disturbance to wildlife. Conserv. Lett. 8, 424–431.
Coetzee, B. W., and Chown, S. L. (2016). A meta-analysis of human disturbance impacts on Antarctic wildlife. Biol. Rev. 91, 578–596. doi: 10.1111/brv.12184
Connor, R. C., Wells, R. S., Mann, J., and Read, A. J. (2000). “The bottlenose dolphin: social relationships in a fission-fusion society,” in Cetacean Societies: Field Studies of Dolphins and Whales, eds J. Mann, R. C. Conner, P. L. Tyack, and H. Whitehead, (Chicago: University of Chicago Press), 91–126.
Crespo, E. A., Pedraza, S. N., Dans, S. L., Svendsen, G. M., Degrati, M., and Coscarella, M. (2019). The southwestern Atlantic southern right whale, Eubalaena australis, population is growing but at a decelerated rate. Mar. Mamm. Sci. 35, 93–107.
Devine, J. A., Wright, P. J., Pardoe, H. E., Heino, M., and Fraser, D. J. (2012). Comparing rates of contemporary evolution in life-history traits for exploited fish stocks. Can. J. Fish. Aquat. Sci. 69, 1105–1120.
Di Minin, E., Leader-Williams, N., and Bradshaw, C. J. (2016). Banning trophy hunting will exacerbate biodiversity loss. Trends Ecol. Evol. 31, 99–102. doi: 10.1016/j.tree.2015.12.006
Dickman, A. J. (2010). Complexities of conflict: the importance of considering social factors for effectively resolving human–wildlife conflict. Anim. Conserv. 13, 458–466.
Frid, A. (2003). Dall’s sheep responses to overflights by helicopter and fixed-wing aircraft. Biol. Conserv. 110, 387–399.
Galligan, T. M., Balmer, B. C., Schwacke, L. H., Bolton, J. L., Quigley, B. M., Rosel, P. E., et al. (2019). Examining the relationships between blubber steroid hormones and persistent organic pollutants in common bottlenose dolphins. Environ. Pollut. 249, 982–991. doi: 10.1016/j.envpol.2019.03.083
Gill, J. A., Norris, K., and Sutherland, W. J. (2001). Why behavioural responses may not reflect the population consequences of human disturbance. Biol. Conserv. 97, 265–268.
Henderson, S. D. (2012). Habitat Use, Reproduction and Survival: A Comparative Study of Bottlenose Dolphins in Doubtful Sound and Dusky Sound. PhD Thesis. Dunedin, New Zealand: University of Otago.
Higham, J., Bejder, L., and Williams, R. (eds) (2014). Whale-Watching Sustainable Tourism and Management. Cambridge: Cambridge University Press.
Hin, V., Harwood, J., and de Roos, A. M. (2019). Bio-energetic modelling of medium-sized cetaceans shows high sensitivity to disturbance in seasons of low resource supply. Ecol. Appl. 29:e01903. doi: 10.1002/eap.1903
Klein, M. L., Humphrey, S. R., and Percival, H. F. (1995). Effects of ecotourism on distribution of waterbirds in a wildlife refuge. Conserv. Biol. 9, 1454–1465.
Lavigne, D. M., Scheffer, V. B., Kellert, S. R. (1999). “The evolution of North American attitudes toward marine mammals,” in Conservation and Management of Marine Mammals, eds Montgomery, S. Jr., and Reeves, R. R., (Washington DC: Smithsonian).
Lizner, D. A., and Lewis, J. (2011). poLCA: and R package for polytomous variable latent class analysis. J. Statist. Softw. 42, 1–29. doi: 10.1111/jocn.13904
Loe, J., and Roskaft, E. (2004). Large carnivores and human safety – a review. Ambio 33, 283–288. doi: 10.1579/0044-7447-33.6.283
Lusseau, D. (2014). “Ecological constraints and the propensity for population consequences of whale-watching disturbances,” in Whalewatching and Sustainable Tourism, eds J. E. S. Higham and L. Bejder, (Cambridge: CUP), 229–241.
Lusseau, D., Bain, D. E., Williams, R., and Smith, J. C. (2009). Vessel traffic disrupts the foraging behaviour of southern resident killer whales Orcinus orca. Endang. Spec. Res. 6, 211–221.
Lusseau, D., and Higham, J. E. S. (2004). Managing the impacts of dolphin-based tourism through the definition of critical habitats: the case of bottlenose dolphins (Tursiops spp.) in Doubtful Sound, New Zealand. Tourism Manage. 25, 657–667.
Lusseau, D., Schneider, K., Boisseau, O. J., Haase, P., Slooten, E., and Dawson, S. M. (2003). The bottlenose dolphin community of Doubtful Sound features a large proportion of long-lasting associations – can geographic isolation explain this unique trait? Behav. Ecol. Sociobiol. 54, 396–405.
Lusseua, D., Williams, R., Wilson, B., Grellier, K., Barton, T. R., Hammond, P. S., et al. (2004). Parallel influence of climate on the behaviour of Pacific killer whales and Atlantic bottlenose dolphins. Ecol. Lett. 7, 1068–1076. doi: 10.1111/j.1461-0248.2004.00669.x
Lusseau, D., Wilson, B., Hammond, P. S., Grellier, K., Durban, J. W., Parsons, K. M., et al. (2006). Quantifying the influence of sociality on population structure in bottlenose dolphins. J. Anim. Ecol. 75, 14–24. doi: 10.1111/j.1365-2656.2005.01013.x
Madin, E. M., Dill, L. M., Ridlon, A. D., Heithaus, M. R., and Warner, R. R. (2016). Human activities change marine ecosystems by altering predation risk. Glob. Change Biol. 22, 44–60. doi: 10.1111/gcb.13083
Mahomed, M. (2008). Behaviour of Bottlenose Dolphins: Inference for Dolphin Tourism off Durban, South Africa. Thesis, Master of Science. University of Kwazulu-Natal, Kwazulu-Natal.
Mallard, G. (2019). Regulating whale watching: a common agency analysis. Ann. Tourism Res. 76, 191–199.
Manlik, O., McDonald, J. A., Mann, J., Raudino, H. C., Bejder, L., Krützen, M., et al. (2016). The relative importance of reproduction and survival for the conservation of two dolphin populations. Ecol. Evol. 6, 3496–3512. doi: 10.1002/ece3.2130
McClung, M. R., Seddon, P. J., Massaro, M., and Setiawan, A. N. (2004). Nature-based tourism impacts on yellow-eyed penguins Megadyptes antipodes: does unregulated visitor access affect fledging weight and juvenile survival? Biol. Conserv. 119, 279–285.
McHugh, K. A., Allen, J. B., Barleycorn, A. A., and Wells, R. S. (2011). Natal philopatry, ranging behavior, and habitat selection of juvenile bottlenose dolphins in Sarasota Bay, Florida. J. Mammal. 92, 1298–1313.
Möller, L. M., Allen, S. J., and Harcourt, R. G. (2002). Group characteristics, site fidelity and seasonal abundance of bottlenose dolphins (Tursiops aduncus) in Jervis Bay and Port Stephens, south-eastern Australia. Austr. Mammol. 24, 11–22.
Möller, L. M., Beheregaray, L. B., Allen, S. J., and Harcourt, R. G. (2006). Association patterns and kinship in female Indo-Pacific bottlenose dolphins (Tursiops aduncus) of southeastern Australia. Behav. Ecol. Sociobiol. 61, 109–117.
Natoli, A., Peddemors, V. M., and Hoelzel, A. R. (2008). Population structure of bottlenose dolphins (Tursiops aduncus) impacted by bycatch along the east coast of South Africa. Conserv. Genet. 9, 627–636.
Nattrass, S., and Lusseau, D. (2016). Using resilence to predict the effects of disturbance. Sci. Rep. 6:25539. doi: 10.1038/srep25539
New, L. F., Clark, J. S., Costa, D. P., Fleishman, E., Hindell, M. A., Klanjscek, T., et al. (2014). Estimating the long-term fitness of southern elephant seals on the basis of short-term measures of behaviour. Mar. Ecol. Prog. Ser. 496, 99–108.
New, L. F., Hall, A. J., Harcourt, R., Kaufman, G., Parsons, E. C. M., Pearson, H. C., et al. (2015). The modelling and assessment of whale-watching impacts. Ocean Coastal Manage. 115, 10–16.
New, L. F., Harwood, J., Thomas, L., Donovan, C., Clark, J. S., Hastie, G., et al. (2013). Modeling the biological significance of behavior change in coastal bottlenose dolphins in response to disturbance. Funct. Ecol. 27, 314–322.
Nowacek, S., Wells, R., and Solow, A. R. (2001). Short-term effects of boat traffic on bottlenose dolphins, Tursiops truncatus, in Sarasota Bay, Florida. Mar. Mammal Sci. 17, 673–688.
O’Connor, S., Campbell, R., Cortez, H., and Knowles, T. (2009). Whale Watching Worldwide: Tourism Numbers, Expenditures and Economic Benefits. Yarmouth MA: International Fund for Animal Welfare, 2695.
O’Grady, J. J., Reed, D. H., Brook, B. W., and Frankham, R. (2004). What are the best correlates of predicted extinction risk? Biol. Conserv. 118, 513–520.
Packer, C., Ikanda, D., Kissui, B., and Kushnir, H. (2005). Lion attacks on humans in Tanzania. Nature 436, 927–928.
Parsons, E. C. M. (2012). The negative impacts of whale-watching. J. Mar. Biol. 2012:9. doi: 10.1155/2012/807294
Pérez-Jorge, S., Gomes, I., Hayes, K., Corti, G., Louzao, M., Genovart, M., et al. (2016). Effects of nature-based tourism and environmental drivers on the demography of a small dolphin population. Biol. Conserv. 197, 200–208.
Pimentel, D., Zuniga, R., and Morrison, D. (2005). Update on the environmental and economic costs associated with alien-invasive species in the United States. Ecol. Econ. 52, 273–288.
Pirotta, E., Booth, C., Costa, D., Fleishman, E., Kraus, S., Lusseau, D., et al. (2018). Understanding the population consequences of disturbance. Ecol. Evol. 8, 9934–9946.
Pirotta, E., Mangel, M., Costa, D., Goldbogen, J., Harwood, J., Hin, V., et al. (2019). Anthropogenic disturbance in a changing environment: modelling lifetime reproductive success to predict the consequences of multiple stressors on a migratory population. Oikos 128, 1340–1357.
Pirotta, E., Merchant, N. D., Thompson, P. M., Barton, T. R., and Lusseau, D. (2015). Quantifying the effect of boat disturbance on bottlenose dolphin foraging activity. Biol. Conserv. 181, 82–89.
Pirotta, E., New, L., Harwood, J., and Lusseau, D. (2014). Activities, motivations and disturbance: fitting a state-space model to bottlenose dolphin behavioural data in Doubtful Sound, New Zealand. Ecol. Modell. 282, 44–58.
Preisser, E. L., Bolnick, D. I., and Benard, M. F. (2005). Scared to death? The effects of intimidation and consumption in predator-prey interactions. Ecology 86, 501–509.
R Core Team, (2019). R: A Language and Environment for Statistical Computing. Vienna: R Foundation for Statistical Computing.
Ripple, W. J., and Beschta, R. L. (2012). Trophic cascades in yellowstone: the first 15 years after wolf reintroduction. Biol. Conserv. 145, 205–213.
Roberts, M. J., and Nieuwenhuys, C. (2016). Observations and mechanisms of upwelling in the northern KwaZulu-Natal Bight, South Africa. Afr. J. Mar. Sci. 38(Suppl. 1), S43–S63.
Rodríguez-Izquierdo, E., Miquelajaureguli, Y., Padilla, P., and Bojórquez-Tapia, L. A. (2019). Modelling approach for crafting environmental regulations under deep uncertainty: whale watching in Ojo de liebre, Mexico. Ecol. Modell. 408:108731. doi: 10.1016/j.ecolmodel.2019.108731
Seely, E., Osborne, R. E., Koski, K., and Larson, S. (2017). Soundwatch: eighteen years of monitoring whale watch vessel activities in the Salish Sea. PLoS ONE 12:e0189764. doi: 10.1371/journal.pone.0189764
Senigaglia, V., Christiansen, F., Bejder, L., Gendron, D., Lundquist, D., Noren, D. P., et al. (2016). Meta-analysis of whale-watching impact studies: comparisons of cetacean responses to disturbance. Mar. Ecol. Prog. Ser. 542, 251–263.
Simmonds, M. P. (2011). “The British and the whales,” in Whales and Dolphins: Cognition, Culture, Conservation and Human Perceptions, eds P. Brakes and M. P. Simmonds, (New York: Routledge), 56–75.
Sprogis, K. R., Bejder, L., Hanf, D., and Christiansen, F. (2020). Behavioural responses of migrating humpback whales to swim-with-whale activities in the Ningaloo Marine Park, Western Australia. J. Exp. Mar. Biol. Ecol. 522, 151254.
Steckenreuter, A., Harcourt, R., and Möller, L. (2011). Distance does matter: close approaches by boats impede feeding and resting behaviour of Indo-Pacific bottlenose dolphins. Wildl. Res. 38, 455–463. doi: 10.1016/j.jenvman.2011.11.002
Steckenreuter, A., Möller, L., and Harcourt, R. (2012). How does Australia’s largest dolphin-watching industry affect the behaviour of a small and resident population of Indo-Pacific bottlenose dolphins? J. Environ. Manage. 97, 14–21. doi: 10.1016/j.jenvman.2011.11.002
Stensland, E., and Berggren, P. (2007). Behavioural changes in female Indo-Pacific bottlenose dolphins in response to boat-based tourism. Mar. Ecol. Prog. Ser. 332, 225–234.
Stockin, K. A., Lusseau, D., Binedell, V., Wiseman, N., and Orams, M. B. (2008). Tourism effects the behavioural budget of the common dolphin Delphinus sp. in the Hauraki Gulf, New Zealand. Mar. Mamm. Sci. 355, 287–295.
Torres, L. G., and Read, A. J. (2009). Where to catch a fish? The influence of foraging tactics on the ecology of bottlenose dolphins (Tursiops truncatus) in Florida Bay, Florida. Mar. Mamm. Sci. 25, 797–815.
Wearing, S., Buchmann, A., and Jobberns, C. (2011). Free Willy: the whale-watching legacy. Worldwide Hospital. Tourism Themes 3, 127–140.
Weinbaum, K. Z., Brashares, J. S., Golden, C. D., and Getz, W. M. (2013). Searching for sustainability: are assessments of wildlife harvests behind the times? Ecol. Lett. 16, 99–111. doi: 10.1111/ele.12008
Wells, R. S. (2014). “Social structure and life history of bottlenose dolphins near Sarasota Bay, Florida: insights from four decades and five generations,” in Primates and Cetaceans: Field Research and Conservation of Complex Mammalian Societies, eds J. Yamigiwa and L. Karczmarski, (Tokyo: Springer), 149–172.
Wells, R. S., Allen, J. B., Lovewell, G., Gorzelany, J., Delynn, R. E., Fauquier, D. A., et al. (2015). Carcass-recovery rates for resident bottlenose dolphins in Sarasota Bay, Florida. Mar. Mamm. Sci. 31, 355–368.
Williams, J. A., Dawson, S. M., and Slooten, E. (1993). The abundance and distribution of bottlenosed dolphins (Tursiops truncatus) in Doubtful Sound, New Zealand. Can. J. Zool. 71, 2080–2088.
Keywords: disturbance, human-wildlife interactions, sustainable tourism, Tursiops, whale-watching
Citation: New L, Lusseau D and Harcourt R (2020) Dolphins and Boats: When Is a Disturbance, Disturbing? Front. Mar. Sci. 7:353. doi: 10.3389/fmars.2020.00353
Received: 24 January 2020; Accepted: 27 April 2020;
Published: 26 May 2020.
Edited by:
Alastair Martin Mitri Baylis, South Atlantic Environmental Research Institute, Falkland IslandsReviewed by:
Phil J. Bouchet, University of St. Andrews, United KingdomCopyright © 2020 New, Lusseau and Harcourt. This is an open-access article distributed under the terms of the Creative Commons Attribution License (CC BY). The use, distribution or reproduction in other forums is permitted, provided the original author(s) and the copyright owner(s) are credited and that the original publication in this journal is cited, in accordance with accepted academic practice. No use, distribution or reproduction is permitted which does not comply with these terms.
*Correspondence: Leslie New, bGVzbGllLm5ld0B3c3UuZWR1
Disclaimer: All claims expressed in this article are solely those of the authors and do not necessarily represent those of their affiliated organizations, or those of the publisher, the editors and the reviewers. Any product that may be evaluated in this article or claim that may be made by its manufacturer is not guaranteed or endorsed by the publisher.
Research integrity at Frontiers
Learn more about the work of our research integrity team to safeguard the quality of each article we publish.