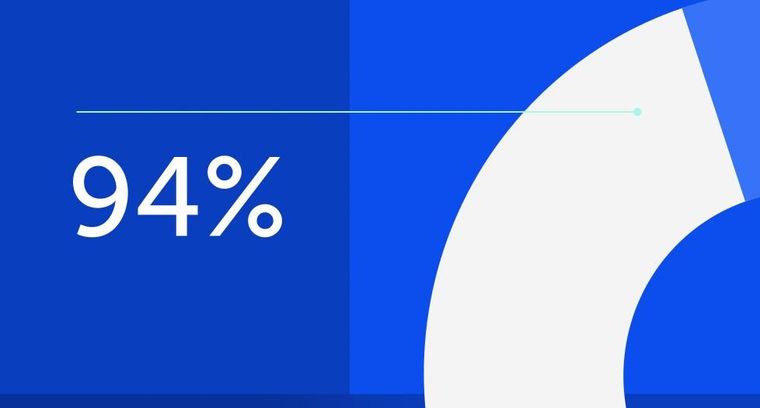
94% of researchers rate our articles as excellent or good
Learn more about the work of our research integrity team to safeguard the quality of each article we publish.
Find out more
REVIEW article
Front. Mar. Sci., 19 May 2020
Sec. Marine Ecosystem Ecology
Volume 7 - 2020 | https://doi.org/10.3389/fmars.2020.00342
Two decades have passed since the initiation of the National Oceanic and Atmospheric Administration’s research program aimed at advancing the understanding of estuary and ocean ecology of United States West Coast Pacific salmon (Oncorhynchus spp.). In this review and prospectus, we summarize key findings from this program and describe a plan for transitioning it to better support Ecosystem-Based Management (EBM). While we focus on salmon research, our approach applies to research design generally. Our path forward involves increasing understanding of ecosystem processes to improve the dependability of scenario testing under novel conditions. Over the past two decades, we developed a conceptual model for how climate, predators, prey, fisheries, and human activities influence salmon. Knowledge gaps we identified from our conceptual model include limited understanding of salmon distributions, behavior, maturation dynamics, and population dynamics, and salmon interactions with predators, competitors, and prey during winter. We consider emerging risks and vulnerabilities facing salmon and propose analysis frameworks for evaluating them. Increased predator populations, coupled with climate change, pose increasing threats to West Coast salmon and will require new strategies and actions to mitigate their negative impacts. We propose research to support the development of decision-support tools to evaluate tradeoffs associated with alternative management strategies and to inform an adaptive ecosystem management system to improve the resilience of salmon populations and salmon-dependent fisheries.
The 150-year decline of Pacific salmon (Oncorhynchus spp.) is well documented (see Nehlsen et al., 1991; Taylor, 1999). Myriad modifications to and degradation of freshwater and estuarine habitats since Europeans colonized western North America have received extensive attention for many decades now (Lichatowich, 1999). In contrast, scientific research into ocean ecology of Pacific salmon slowly began developing in the mid-20th century, and still lags far behind studies of salmon freshwater and estuary ecology (Pearcy, 1992; Beamish, 2018). With the advent of large-scale tagging programs and observations of coherent, high-amplitude fluctuations in salmon catches, abundance, productivity and survival rates that correlated to large-scale ocean climate indices (e.g., Mantua et al., 1997; Hare et al., 1999; Mueter et al., 2005; Teo et al., 2009; Kilduff et al., 2015), it became clear that much of the interannual to decadal-scale variability in salmon abundance and productivity was driven by processes in the ocean environment. In response, the National Oceanic and Atmospheric Administration (NOAA) Fisheries West Coast Science Centers proposed a coordinated plan for ocean research on Pacific salmon (Oncorhynchus spp.) to elucidate the drivers of variation in salmon abundance (Brodeur et al., 2000). The research plan was designed to inform hatchery practices, to advise development of forecasts for harvest management, and to consider ocean climate variability when evaluating restoration and recovery plans and actions. Each of the projects carried out under the ocean research plan focused on ecosystem components and how they affected salmon physiology/vital rates and subsequent salmon population dynamics. Successful outcomes include descriptions of coastwide (Weitkamp, 2010; Teel et al., 2015; Shelton et al., 2019) and local-scale distributions (Brodeur et al., 2004; Satterthwaite et al., 2013; Hassrick et al., 2016) and distributional relationships to small-scale oceanographic features (De Robertis et al., 2005; Pool et al., 2008; Brodeur and Morgan, 2016). Studies have also examined seasonal and interannual variations in diet composition (Brodeur et al., 2007a, b; Daly and Brodeur, 2015), immature salmon growth rates and condition (Brodeur et al., 2004; Beckman et al., 2008), diseases and parasites (Losee et al., 2014; Sandell et al., 2015), salmon predators (Emmett et al., 2006), environmental covariates of salmon presence or abundance (Bi et al., 2007; Pool et al., 2012; Burke et al., 2013a), and correlates of marine survival (e.g., Burke et al., 2013b; Kilduff et al., 2015).
The introduction of ecosystem considerations in stock assessment has been slow to occur in managed fisheries within the California Current Ecosystem (CCE) (Pacific Fisheries Management Council [PFMC], 2013; Haltuch et al., 2019). Using the NOAA Fisheries West Coast salmon ecology program as our case study, we provide guidance on how, where, and what to consider in our discussion of risk assessment and knowledge gaps. Improving confidence in forecasting salmon recruitment and resilience under future climate change scenarios requires identifying processes that underlie observed statistical relationships and understanding how these relationships vary by stock. The focus, therefore, of the next few decades of research should be to advise management of salmon in the context of how the ecosystem affects salmon and how ecosystem components are reliant on salmon. This will ultimately promote evidence-based approaches of strategic management to improve resilience and recovery of salmon populations and promote resilient coastal communities and fisheries. Single-population assessment maintains value for tactical management (e.g., spatial harvest control rules, hatchery release timing and abundance) but can also apply to evaluating the efficacy of chosen ecosystem-level management strategies as they apply to that species.
Successful longer-term strategic management plans will result from the accumulation of well-defined and executed tactical management actions across ecosystem components. Tactical management of salmon focuses on demographics of individual populations more than it considers their role as an integral component of ecosystems. Failure to elucidate and accommodate the complex interactions in ecosystems that affect forage, fishers, and salmon-dependent predators leads to ‘ecological surprises’ (Cury et al., 2008) that can derail successful restoration and recovery of salmon. One example is the collapse of the 2005 cohort of a California Chinook salmon population that resulted from a combination of the chronic simplification of the freshwater salmon production system, volatile ocean environmental conditions, forage variability, and increased predation on salmon (Lindley et al., 2009; Wells et al., 2017).
Over the last few decades, notable changes in the freshwater production system for salmon (e.g., dams, development, water diversions, and hatchery practices), oceanography (e.g., upwelling), trophic structure (e.g., increasing predator demand), and salmon life-history diversity have been observed in the CCE (Huber and Carlson, 2015; Satterthwaite and Carlson, 2015; Jacox et al., 2016, 2018; Holsman et al., 2018). As the CCE continues to warm, acidify, and deoxygenate in coming decades, we can expect changes in phenology of the ecosystem and distribution and productivity of salmon and their predators, competitors, forage, and fisheries. These types of changes are motivating a movement toward ecosystem approaches to fishery and environmental management. Increased appreciation of salmon fisheries and restoration efforts as coupled human-natural systems set in a dynamic environment has put a spotlight on the need for new kinds of scientific support (e.g., Richerson and Holland, 2017). Pacific salmon, with their complex life cycles, dependence on a variety of habitats, multifaceted connections with people (cultural and economic), and important role as both predators and prey in marine food webs, are perhaps most in need of this coupled human-natural system perspective.
Therefore, NOAA Fisheries and collaborating agencies have prioritized ecosystem-based approaches to manage salmon restoration and fisheries and identified a need to address a broad suite of natural and anthropogenic impacts, including those associated with climate and ocean chemistry changes (Busch et al., 2016; NOAA NW/SW Fisheries Science Centers, 2016). Understanding how salmon populations respond to a changing ecosystem, including to the impacts of climate change, could better frame salmon restoration efforts and situate scientists to provide informed advice for ecosystem-based management (EBM; e.g., fisheries, salmon restoration efforts, forage, predator recovery, hatchery management, and freshwater habitat management). We propose a salmon ocean research framework that is based on the NOAA Fisheries’ ecosystem-based fisheries management strategy (NOAA Fisheries, 2016a, b); this can benefit both Pacific salmon and ecosystem management (Figure 1A and Tables 1, 2). While the freshwater period of salmon life-history is largely beyond the scope of this proposal, the anadromous life-history of salmon requires that consideration of links between freshwater conditions and salmon dynamics following outmigration be explicitly discussed. In particular, as we explore below, salmon dynamics during the first few months at sea (e.g., survival, distributions, and growth) are not independent of freshwater conditions and management strategies.
Figure 1. (A) Modified from NOAA Fisheries (2016a) are the six tiers that should be achieved to implement ecosystem-based fishery management. We have modified the tasks within each tier to describe how a research program can provide the appropriate information for inclusion in an EBM strategy. (B) A demonstration of the sequential re-evaluation and adaptation of the process. As the results accumulate from each tier of complexity an advisory body should undertake an evaluation of the process and results and if there is a disconnect between objectives, science, models and policy advice, the practitioners should fall back a level ideally until consensus among advisory members is met regarding next steps.
Table 1. Research questions identified by consensus of authors and outside contributors that correspond to research foci indicated in the conceptual model that outlines interactions among ocean ecosystem components, processes and salmon life stages (Figure 2).
Table 2. We summarize simple, unidirectional (i.e., effects of ecosystem components on salmon) and complex, multidirectional (i.e., interactions between salmon and ecosystem components) objectives for achieving EBM.
NOAA Fisheries’ ecosystem-based fisheries management strategy is a 6-tiered approach of increasing complexity that fulfills both research and managerial needs (Figure 1A; NOAA Fisheries, 2016b). Tier 1 includes describing the system being managed and the management objectives, Tier 2 identifies research gaps, and Tier 3 is risk assessment of ecosystem state and individual ecosystem components. Tier 4 evaluates management scenario tradeoffs and Tier 5 provides advice to managers. The sixth tier is an adaptive management approach (Walters, 1986) and involves progressive re-evaluation of outcomes in response to variability in ecosystem structure, function, and management, and realigns objectives, research, and management with contemporary conditions. If re-evaluation indicated a need to modify approaches, practitioners should regress to a previous tier and rebuild from that point forward (Figure 1B).
We identify priority research objectives and approaches to improve salmon assessment and inform EBM in the CCE to promote sustainability of salmon populations, fisheries, and ecosystem components reliant on salmon. Management drivers associated with population dynamics (e.g., hatchery practices, harvest rules, habitat management, restoration and recovery actions) can be addressed as part of, and informed by, an EBM framework in the context of climate change (Figure 2). An objective of our salmon research program is to identify how ecosystem components affect salmon (Figure 2) and how salmon interact with ecosystem components (Tiers 1 and 2; Figure 1). Our ultimate goal, however, is to delineate a path toward linking research of ecosystem processes more tightly to decision making. Importantly, objectives are decided, in part, by informed ecological, economic, and societal needs and action is set by managerial bodies. Here, we argue for a research program dedicated to meeting and helping to inform objectives by providing supported actionable advice. Therefore, we identify methods to apply research findings to ecosystem-informed salmon management, and to identify consequences for the ecosystem as it is impacted by salmon.
Figure 2. Our first step in answering the question, “What is the fundamental science we need?”, was to develop a conceptual model of ecosystem components that provides a framework for process-related research questions. The conceptual model represents the unidirectional aspects of salmon ocean ecology in which we evaluate the effect of ecosystem components on salmon. First, we identified salmon-ecosystem component categories in the ocean; these are Physical Environment, Primary Production, Forage Community, Predator Species (including humans), and Salmon. Our model also includes linkages among various components that describe their relationships as Environmental Forcing, Trophic Dynamics, or Salmon Population Demographics. Next, we identified the primary management drivers for salmon ocean research and overlaid these so that they overlap the salmon-ecosystem components and linkages that affect them. Our primary management drivers include: Climate Change, Ecosystem-based Management, Salmon Restoration and Recovery, Harvest, and Hatchery Practices. Finally, we listed example research questions (#) that were suggested by the linkages (Table 1). Individual numbers represent specific research areas that should be addressed. Although estuary and river environments are identified in our framework, for the most part we focused on direct linkages with the ocean.
The bulk of this manuscript is dedicated to highlighting what is known about salmon and their role in the ecosystem (Tier 1) and what research should be prioritized to quantify the ecosystem-level dynamics interacting with salmon (Tier 2, Table 1 and Figure 2). We then outline methods for applying research findings toward assessing the risk of not achieving identified management objectives (Tier 3). We also provide directions for developing tools for evaluating the potential efficacy of management actions to address identified risks that incorporate and accommodate ecosystem-level processes (Tier 4). Our proposal is intentionally not prescriptive; individual salmon populations and coastal communities are in many ways unique, and require freedom for researchers and resource managers to tailor approaches to meet regional management objectives. We do, however, provide a template for our salmon-EBM research strategy (Table 2) and, as an example, we apply our plan to a specific research setting that addresses the interactions between specific ecosystem attributes (e.g., predation, recovery of marine predators; Box 1) and salmon recruitment.
BOX 1 | Example application. Describing major ecosystem processes that affect salmon recruitment (i.e., resulting number of sub-adults), ecosystem component interactions with salmon recruitment, and integrating Tier 2 research findings into assessment and ecosystem-based management.
Research strategies intended for simple, unidirectional aspects of salmon ocean ecology represent mechanistic relationships between ecosystem components and salmon recruitment. These relationships are applicable to assessment and management of salmon populations (identified in green on inset diagram). Hypothesized complex, multidirectional relationships include ecosystem component interactions with salmon recruitment (green and purple in the inset diagram) that are applicable to EBM. Considerations within each Tier (Figure 1) represent uncertainties. Tier 2 considerations correspond to research foci and questions that were identified in Figure 2 and Table 1. The Objectives are specific, measurable results that fill information gaps that correspond to considerations. The Tasks are the primary methodologies to achieve the objectives. Products are the ultimate outcome of the research process that is highly relevant and impactful to research and management. The inset diagram identifies major ecosystem components that affect salmon recruitment. Various components could affect different aspects of salmon recruitment. For example, increasing predator abundance (e.g., common murre) could have a direct impact on early salmon survival, whereas other components, such as southern resident killer whales, could influence salmon spawning stock abundance. Salmon recruitment variability could influence the distribution and abundance of predators directly or it could indirectly influence processes such as fishing pressure on crabs that potentially increase humpback whale entanglements with fixed gear.
The first step in achieving EBM is synthesizing what is known about the system and relating that knowledge to the associated objectives of natural resource management. Accomplishing this requires a significant collaborative effort between invested and knowledgeable community members (Table 2) (Sterling et al., 2019; Friedman et al., 2020). Here, relationships between the environment, productivity of the ecosystem and salmon dynamics may be generalized but there are significant stock-specific responses (Crozier and Zabel, 2006).
The idea that cohort strength is largely set shortly after ocean entry is a critical underlying concept (Pearcy, 1992; Beamish and Mahnken, 2001; Kilduff et al., 2014). Outmigration timing and size at estuarine and ocean entry affects subsequent growth and survival rates (Scheuerell et al., 2009; Woodson et al., 2013; Satterthwaite et al., 2014). Although larger fish may survive better during poorer ocean conditions (Woodson et al., 2013; Tucker et al., 2016), the interaction of arrival timing in the estuary with fish size can be complex (Tomaro et al., 2012) and both factors may affect ocean survival rates (Muir et al., 2006; Beacham et al., 2017). Another important concept is that life-history diversity promotes resilience to environmental variation (Schindler et al., 2010; Carlson and Satterthwaite, 2011) and current hatchery practices are reducing some life-history portfolios (Satterthwaite and Carlson, 2015; Willmes et al., 2018).
Salmon growth and condition depend on temperature and quantity and quality of available prey resources (Daly et al., 2010; Litz et al., 2017). Differences in the diets of juvenile salmon within the CCE are attributable to variability in forage availability (Brodeur et al., 2007a, b; Hertz et al., 2015) related to environmental conditions prior to, during and after salmon out-migration (Daly et al., 2013; Friedman et al., 2018). Regionally, increased upwelling or reduced downwelling in winter promotes the introduction and southward transport of nutrients to the surface layer during which potential productivity of the coastal system is set (Logerwell et al., 2003). Greater flow (more southward transport) in the California Current in spring and summer transports lipid-rich copepods from Gulf of Alaska into the northern CCE, which are associated with increased production of Chinook and coho salmon through effects on salmon prey (Peterson and Schwing, 2003).
Characterizing spatial distributions is a critical management need for informing time- and area-based management of ocean fisheries and for protecting weak or recovering stocks. Different species, stocks, and life-history types have distinct migration patterns and marine spatial distributions. Among salmonids, steelhead (Oncorhynchus mykiss) remain in coastal waters the least, with rapid westward movements almost immediately after entering the ocean, dispersing great distances from their river of origin (Hayes et al., 2012; Daly et al., 2014; Myers, 2018). By contrast, some stocks of Chinook salmon (Oncorhynchus tshawytscha) such as California Central Valley fall-run and some stocks from Puget Sound generally remain near their region of ocean entry (Weitkamp, 2010; Shelton et al., 2019). Other species and stocks, such as spring-run Chinook and coho (Oncorhynchus kisutch) salmon, exhibit distributions that vary along this spectrum from the ocean basin-scale, similar to steelhead, to more regional distributions (Trudel et al., 2009). Hassrick et al. (2016) demonstrated regional distributions are often population specific and Johnson et al. (2016) demonstrated that individual release groups of hatchery fish remain somewhat clustered together at-sea. Agent-based models incorporating salmon movement decisions and migration dynamics provide new ways to evaluate the emergent consequences of a variety of environmentally influenced behavior-related mechanisms (Byron and Burke, 2014). For example, Burke et al. (2016) found that ocean conditions, fish size, and out-migration timing all impact migration behavior, which in turn influences consumption and growth rates.
The majority of salmon mortality in the ocean seems to be due to predation (Emmett et al., 2006; Wells et al., 2017), and smaller juvenile salmon are more susceptible to higher predation rates (Holsman et al., 2010; Woodson et al., 2013; Tucker et al., 2016). Reduced forage availability or quality (e.g., lower lipid content) may reduce growth rates, size and condition of salmon (Daly et al., 2010; Wells et al., 2012), thereby increasing their vulnerability to predation, and thus their natural mortality rates. Predation also has an increased effect on salmon mortality when alternative prey is less available (Pearcy, 1992; LaCroix et al., 2009). Although starvation is a possible mortality agent, its prevalence is probably quite low; there have been very few documented cases of emaciated juvenile salmon from ocean surveys (but see Dale et al., 2017). The relationships between oceanographic conditions, forage availability, salmon density and juvenile salmon growth rates are not independent. For example, if conditions are too warm in the CCE, often associated with poor upwelling years and less lipid-rich forage (e.g., Peterson and Schwing, 2003), increased bioenergetic demand imparted on salmon can be significant enough to reduce growth even when their stomachs are fuller than during cooler periods (Daly and Brodeur, 2015).
This period in the life history of salmon is underrepresented in the literature. By autumn, juvenile salmon have outgrown the gape limits of many piscine predators (but remain susceptible to predatory marine mammals; Weise and Harvey, 2008; Chasco et al., 2017) and have become higher-trophic-level predators themselves (with the potential exclusion of sockeye salmon, Oncorhynchus nerka). Late summer and autumn also tend to be a period of higher fishing mortality of older-aged fish, which can affect abundance and demographic characteristics. Forage conditions, temperature, and nutrient transport influence growth and maturation, with faster growing fish maturing earlier (Wells et al., 2007). Substantial and variable mortality rates can affect recruitment during this period as well, especially on fish with insufficient energy reserves (considered a second critical period; Beamish and Mahnken, 2001; Beamish et al., 2004).
Our conceptual model summarizes our understanding of important drivers of Pacific salmon dynamics in the CCE and outlines specific ecosystem-related hypotheses affecting salmon (Figure 2). We embed hypothesized processes within identified managerial drivers that are critical to contemporary and future salmon management (i.e., hatchery, harvest, habitat restoration and population recovery, EBM, all with consideration of climate change). Our model is general and can be applied to different salmon populations and their many life-history types within the CCE. Building conceptual models is valuable when a diverse group of practitioners with various perspectives collaborate to arrive at consensus regarding the interpretation of current research (Harvey et al., 2016; Sterling et al., 2019). If needed, conceptual models can be further refined using data and expert opinion to derive semi-quantitative dynamic conceptual models (e.g., structural equation modeling, Wells et al., 2008; loop analysis, Dambacher et al., 2003; quantitative network models, Harvey et al., 2016; non-linear modeling, Deyle et al., 2016; Bayesian belief networks, Trifonova et al., 2017).
Addressing more complex, multidirectional aspects of the ecosystem would require a model informed by not only the simple relationships identified in Figure 2, but also ecosystem effects caused by variability in salmon dynamics and demographics. These more complex models also should be developed for the specific objectives that are identified. The inset complex, multidirectional model in Box 1 is a hypothetical example of such a model.
Although our conceptual model (Figure 2) outlines our understanding of salmon ocean ecology, it also helps identify critical knowledge gaps. This section is informed by Table 1 identifying critical questions linked directly to our conceptual model. Plans to manage individual salmon populations will likely place specific emphasis on different suites of research goals but, generally, the research priorities we provide aim to address knowledge gaps across salmon populations in the CCE. We focus largely on unidirectional aspects of the system (i.e., how the ecosystem affects salmon) but acknowledge a need to apply those findings toward quantifying direct and indirect effects salmon have on the ecosystem (complex and multidirectional aspects).
In a Web of Science search of the literature published between 2000 and 2019 we found approximately 300 papers on the topic of salmon ocean ecology in the CCE, of which half considered Chinook salmon and a quarter coho salmon. The remainder of studies represented steelhead (8%; Oncorhynchus mykiss), chum (4%; Oncorhynchus keta), pink (4%; Oncorhynchus gorbuscha), sockeye (4%; Oncorhynchus nerka), and cutthroat (1%; Oncorhynchus clarkii). Such a disparity of research across species reflects in part the different socio-economic value of different species and their current status under the Endangered Species Act. It also points to a need for more inclusive salmon research to evaluate species-specific salmon dynamics. Another broad conclusion from this literature review is that there is a major knowledge gap regarding salmon dynamics during winter, especially ocean distribution and growth, since much of this information is fishery-dependent and winter ocean fisheries are much reduced.
Management of factors with carry-over effects from freshwater rearing to ocean residence provide a potential avenue for EBM strategies to reduce the magnitude and variability of salmon mortality at sea (Gosselin et al., 2018). Factors that influence timing of and size at ocean entry cause differential early marine survival due to match-mismatch dynamics (Satterthwaite et al., 2014) and size-selective predation (Woodson et al., 2013; Tucker et al., 2016). All five management drivers identified in Figure 2 can generate carry-over effects. Hatchery practices and freshwater rehabilitation efforts to improve life-history and demographic diversity of emigrating salmon may be effective at strengthening weak populations and increasing early survival at sea. If recruitment variability can be reduced then more stable fisheries can be achieved (e.g., Brennan et al., 2019).
Transitions between freshwater, estuary, and marine residence are critical periods during which recruitment may be largely determined (Zabel and Williams, 2002; Zabel and Achord, 2004; Woodson et al., 2013; Michel, 2018). Gosselin et al. (2018) demonstrated that processes affecting freshwater rearing and out-migration influence salmon survival at sea (Figure 2, foci 1, 14). These processes include the factors freshwater flows, temperature regimes, fish density, and various hatchery practices. The abundance of smolts determines the potential for intraspecific competition in the ocean (Figure 2, foci 6, 7, Daly et al., 2012; Miller et al., 2013), and rearing conditions in freshwater also influence emigration size, emigration timing and susceptibility to predation (Figure 2, focus 8, Woodson et al., 2013; Munsch et al., 2019) as well as out-migration timing and potential mismatch with prey resources at sea (Figure 2, foci 2,3,6) (Satterthwaite et al., 2014).
In the absence of effective freshwater and estuary habitat restoration and management, variation in the timing and size at ocean entry of natural-origin smolts will likely continue to decline if freshwater systems continue to become less habitable (and thus less variable themselves) due to increasing temperatures, land and water use practices that degrade salmon habitat, and drought occurrence (Satterthwaite and Carlson, 2015). Further, increased reliance on trucking or barging fish beyond impacted habitats has exacerbated these issues by reducing life-history and phenotypic diversity (Huber and Carlson, 2015). Extending these research avenues to more species and life-history types and across the CCE can elucidate, through comparison, the generalities and spatially explicit processes affecting out-migrating salmon and provide knowledge with which to inform watershed management in the context of the ocean ecosystem.
As it relates to our identified management drivers (Figure 2), we need to know where salmon reside to determine the geographic extent of the ecosystem with which salmon interact and to develop place-based tactical and strategic management actions. All the management drivers identified in Figure 2 are dependent on quantifying dispersal and behavior. Restoration and recovery of salmon is tied to variability in mortality at sea which, as we have explored above, is tied to carry-over effects from freshwater and estuary phases of salmon life-history. However, the mechanisms underlying these dynamics are determined by spatially explicit ecosystem-level processes. Therefore, successful restoration and recovery will be achieved best through EBM-informed actions. Importantly, climate change will likely lead to trends and variability in the spatial arrangement of ecosystem processes that will need to be accounted for into the future.
While much has been learned about distribution of salmon stocks in the ocean, primarily for fished species of harvestable size during fishing periods, we have little understanding of how these patterns are generated. Advancing our knowledge of drivers of dispersal and directed swimming for fish stocks, such as gradients in local cues or larger-scale orientation and navigation mechanisms, is imperative for developing insights into habitat use and potential responses to climate trends and variability (Burke et al., 2014). Similarly, characterizing basic ecological dynamics in a spatial context, such as predation, requires an understanding of dispersal and migration behavior. There are few empirical studies that adequately describe dispersal mechanisms relative to environmental and forage conditions that would enable predictions of responses to climate scenarios (Welch et al., 2011; Travis et al., 2013; Furey et al., 2015). Agent-based models, coupled with oceanographic models, are particularly well suited for this task (Byron and Burke, 2014). Technological advances in archival tag, geolocational, and acoustic arrays provide data to inform agent-based models and should be a major focus of salmon research. Combining these observation and modeling technologies are imperative to elucidating dynamics during winter when both fishery-dependent and independent surveys are lacking.
Quantifying feeding behavior dynamics is needed to meet the goals linked to our identified management drivers (Figure 2). Improved growth following emigration to sea generally reduces early mortality (Fiechter et al., 2015) but the location of high growth potential areas (e.g., near fronts; Sato et al., 2018; Sabal et al., 2020) is also very important to modulating variability in early survival (Henderson et al., 2019). Namely, foraging behavior in response to prey-scape dynamics and the resulting local and regional distribution of salmon influence the spatial extent of ecosystem processes such as predation and fishing. While the mechanisms defining foraging behavior are out of managerial control, quantifying the processes is critical to improving restoration and recovery of salmon through improved EBM actions (e.g., potential overlap of salmon, prey and predators) (Figure 2).
Salmon tend to be trophic generalists that feed visually (Figure 2, focus 6) (Quinn, 2005). Greater growth potential and, as a consequence, earlier onset of piscivory in the ocean likely results from greater availability of suitable forage (Gross et al., 1988; Keeley and Grant, 2001). Understanding foraging ecology requires knowledge of available prey communities at spatiotemporal scales relevant to the predator (Figure 2, foci 4, 6, 5). In a system as large as the CCE, available prey varies among seasons and spatial extents, locations, and across years, and this variability can have direct effects on variability in salmon growth and survival (Friedman et al., 2018; Sabal et al., 2020). Salmon feeding behavior, such as prey selection and use of environmental cues to locate prey, and the linkage between secondary production and salmon recruitment remains poorly understood. Simplified models of foraging behavior within agent-based models for juvenile salmon have produced simulated growth rates that are consistent with observed values (Fiechter et al., 2015; Henderson et al., 2019). A reasonable assumption is that juvenile salmon make movement decisions that maximize growth and survival. Better understanding of mechanisms that control the relationship between growth at local-scale and larger-scale dispersal would increase individual-based life-cycle model realism. Field-based observations are needed to identify environmental covariates that provide a consistent set of cues for model movement rules (Burke et al., 2014, 2016). Diet selection studies relative to available prey, studies of feeding success under variable conditions, and estimates of daily rations across a range of ages and physiological states are also necessary to improve bioenergetic and foraging behavior models.
Many marine mammal and seabird populations in the CCE increased substantially in recent decades, and EBM strategies to mitigate increasing predation on salmon will be necessary to improve restoration and recovery of salmon (and other) populations (De Master et al., 2001). Further, the synergy of climate change and increased predator consumptive demands require that we quantify the processes affecting salmon to better understand trade-offs involved in management strategies to achieve restoration and recovery of salmon and salmon-dependent predators. However, models to evaluate risk of failing to achieve our goals (Tier 3) and test management scenarios to evaluate outcomes of our options (Tier 4) are grossly under-parametrized in this regard (Wells et al., 2017).
Research should focus on understanding variability in trophic pathways and the impact on salmon as predator demand varies (in response to environmental forcing and changes in predator abundance). With such knowledge we can build better tools that advise management actions relevant to forage, salmon, and predators to mitigate potential impacts of predation on salmon (Chasco et al., 2017; Samhouri et al., 2017; Wells et al., 2017; Friedman et al., 2019). The dynamics that control salmon forage are similar to those that affect the forage of trophically equivalent predators (Figure 2, foci 2, 3, 4, 5, 6, 8; Friedman et al., 2018). However, when predators such as seabirds experience limited typical prey, they may increase foraging on juvenile salmon in nearshore habitats (LaCroix et al., 2009; Wells et al., 2017). Population increases in some predators, such as pinnipeds, has directly increased predation on salmon (Chasco et al., 2017; Wargo Rub et al., 2019). The need to balance trade-offs that arise when considering multiple interacting, and sometimes conflicting, objectives of managing both forage species and their predators has been recognized by the Pacific Fisheries Management Council (PFMC), which has implemented bans on development of new fisheries for krill, shortbelly rockfish (Sebastes jordani), mesopelagic species, most oceanic squids, and other forage taxa, and recognizes the need to mitigate fisheries impacts on productivity of forage species (e.g., northern anchovy Engraulis mordax, Pacific sardine Sardinops sagax) to provide adequate forage for salmon or other top-predators1 (Pacific Fisheries Management Council [PFMC], 2013) (Figure 2, foci 9, 10). We need to inform strategies resulting in synchronous recovery of predators, forage, and salmon otherwise ‘ecological surprises’ brought on by trophic imbalances and anomalous ocean conditions could increase going forward (Cury et al., 2008; Samhouri et al., 2017).
Relevant to our management drivers (Figure 2), defining tactical management actions and evaluating the success of management practices impacting restoration and recovery of salmon within their ecosystem relies on precise and accurate assessment of salmon abundance at sea and on return to the rivers to spawn. Such is true whether we are managing salmon in isolation (i.e., single-stock management) or as an integral member of the ecosystem as prey and predators.
Maturation rate and variability estimates are critical for cohort reconstructions, natural mortality estimates, and interpreting patterns in escapement at age. Currently, most salmon assessment models parametrize maturation rate estimates on assumed and constant subadult survival rates (Figure 2, foci 12, 13), but in reality, both likely vary and depend on growth, which varies according to prey availability and environment (Wells et al., 2007; Allen et al., 2017). Data from year-round, fishery-independent and fishery-dependent sampling in the ocean could be used to characterize gonad development and population demographics and also how the ocean environment influences maturation. Maturation rate variability also affects adult salmon densities in the estuary as they return to spawn, which can increase predator density in the estuary, resulting in increased adult mortality (Figure 2, foci 12, 13; Strange, 2013).
The ocean experience of returning adult salmon carries over into freshwater reproductive success (e.g., fecundity, size, and thiamine deficiency; Bigler et al., 1996; Sutherland et al., 2018). We can improve life-cycle models and other management tools by increasing our understanding of how ocean conditions affect spawning condition, fecundity, and egg viability in freshwater (Figure 2, focus 14). There is also a need to elucidate how ocean temperatures could affect timing of adult returns and condition upon arrival on the spawning grounds. Research to quantify these processes would include sampling salmon condition, age structure, and reproductive condition at sea and through the transition to freshwater and to the spawning reaches (Figure 2 foci 12, 13).
People define the management drivers that we consider important (e.g., Figure 2). In doing this we describe successful outcomes through consensus building efforts defined in Tier 1 and laid out, in part, in fisheries ecosystem plans. As integral components of the ecosystem reliant on its state, we need to elucidate our role in impacting the ecosystem, respond to variability and trends in the ecosystem, and quantify ecosystem-level processes to develop strategies to reduce the impact of perturbations on us in the future.
Coastal and river-oriented human populations reliant on salmon have experienced increasing hardships in the last several decades with reduced salmon abundance and fishing opportunities brought on by droughts, recovery of marine mammal populations, unprecedented ocean warming, variability in fishery allocations, and variability in stock distributions and composition. These adverse conditions relate to year-to-year climate extremes interacting with longer-term impacts of agriculture, hydropower, timber harvest, urbanization, hatchery production, fishing and coastal resilience, which may reinforce or attenuate ecosystem processes. Specifically, freshwater management practices need to consider the complex, multidirectional tradeoffs associated with interactions between agricultural needs, the management of water resources related to hydropower, habitat quality and quantity, and any correlated carry-over effects on salmon as they out-migrate. The interactions between these processes and coastal resilience and socioeconomics is poorly understood, likely due to their complex nature.
Collaborative process studies linked to salmon life-cycle models and in consideration of human dimensions can illustrate some of these relationships (Figure 2 foci 1, 14). To develop and carry out study designs, a transdisciplinary approach will be required inclusive of at least the resource users (e.g., agriculture, hydropower, and fisheries), watershed and fishery managers at various levels of government, hydrologists, water resource engineers, landowners, special interest groups (e.g., fishing and conservation groups), and economists (Sterling et al., 2019; Friedman et al., 2020).
At sea, salmon fisheries are linked to other fisheries in the CCE by cross-participation, meaning changes in salmon availability have potential to indirectly impact other harvested species (and vice versa) through changes in fishing behavior (Figure 2, focus 8). For example, from a vessel or fisher’s perspective, participation in commercial salmon fisheries is often coupled with participation in the (typically) winter and early spring fishery for Dungeness crab (Cancer magister), such that late season effort in that fishery may increase when salmon fishing opportunities are depressed or restricted by regulations (Thomson, 2015; Richerson and Holland, 2017). Over longer time periods, reduced access to salmon may lead fishers to continue to diversify into other fisheries by purchasing new permits (Martin, 2008). Such shifts can affect both the economy and ecosystem components directly and indirectly linked to salmon and crab, including other harvested species (Hiddink et al., 2006).
The dynamics of other fisheries also have potential to influence salmon fishing mortality and harvest regulations (Figure 2, foci 7, 8), and vice versa. For example, salmon are incidentally caught by the Pacific whiting (Merluccius productus) fishery, which is allowed to take thousands of Chinook salmon annually but has exceeded the cap several times, in some cases causing closures of the economically important Pacific whiting fishery (Figure 2, foci 7, 8). The fishery can move north to reduce risk of salmon bycatch, but doing so increases risk of Pacific Ocean perch (Sebastes alutus) and other rockfish bycatch, demonstrating the complexity of ecosystem-level fishery management (Figure 2, foci 9, 10, 12).
Ecological processes, resource user requirements, and competing interests (ecological, cultural, and socioeconomic) will need to be understood and appropriate models developed to evaluate management strategies. For example, recognizing what fishers use to buffer against fisheries fluctuations (e.g., maintaining portfolios of fishing strategies and permits; Hanna, 1992; Kasperski and Holland, 2013) and their responses to regulatory changes (e.g., Richerson et al., 2018) can help us to understand how changes in salmon and other fisheries may impact livelihoods and fishing behavior. Scaling these analyses up to the community level can elucidate how fishery dynamics and community characteristics interact, especially in a changing ecosystem context (Sethi et al., 2014; Himes-Cornell and Hoelting, 2015). The framework of community vulnerability indices established by the California Current Integrated Ecosystem Assessment (CCIEA; Breslow et al., 2013) could be leveraged to quantify socioeconomic impacts of changing salmon availability. Understanding the sociocultural and economic value of salmon in a complex ecological and social context is needed before tradeoffs can be modeled (Mangel and Dowling, 2016).
Numerous technologies have been developed that can be used to address research gaps related to distribution, stock-specific abundances, catch rates, demographics, and dynamics (see Beamish, 2018). We identify some direct applications in Table 1 relative to research foci shown in Figure 2. Genetic tools such as genetic stock identification and parentage-based tagging (Beacham et al., 2018) can identify specific populations and often hatchery of origin of individual salmon and steelhead which could inform spatially explicit harvest rules and management of freshwater habitats of origin (e.g., evaluate effectiveness of habitat restoration efforts for improving recruitment). High throughput environmental DNA is also emerging as a valuable tool for identifying presence of salmon and co-occurring species (Thomsen et al., 2012; Thomsen and Willerslev, 2015), which will improve at-sea collection and study of interactions among forage, salmon, and predators. High-quality remote sensing data such as sea surface temperatures and other physical parameters, and biotic parameters such as chlorophyll concentrations are readily available in near-real time allowing process studies to be placed in the context of their immediate and surrounding environment. Data-archiving fish tags (e.g., temperature, depth, and location) have an extended battery life and may be deployed in smaller fish, improving our potential to reconstruct the life-history of salmon through their entire ocean residence. Generally, a study examining salmon interactions with their surroundings should incorporate new tagging technologies (e.g., archival geolocation tags and satellite pop-ups), spatially explicit environmental data, and genetic markers. Statistical analyses should integrate these data streams from field studies and monitoring programs. Inter- and intra-annual spatial gaps in coverage can be mitigated with remote sensing, autonomous underwater vehicles, buoys, sail drones, and coupled ocean circulation and biophysical models (e.g., Fiechter et al., 2015; Henderson et al., 2019) available in near-real time providing estimates of local productivity and many aspects of the ocean environment.
Using functional relationships quantified by process studies, models based on states and trends across interrelated ecosystem components should be developed to evaluate risk of negative salmon responses (e.g., low recruitment and unanticipated distributional shifts) and ecosystem states (e.g., predator mortality events brought on by reduced salmon recruitment) related to natural variability and management actions. We provide advice on a number of approaches, which provide guidance on developing risk and vulnerability assessments for simple, unidirectional, and complex, multidirectional efforts (Table 2).
An integrated approach to risk analysis, based on process studies and framed in a mechanistic model, will include evaluating salmon, predators, and prey population status and drivers of that status. Ecosystem states and evaluations of short-term ecosystem risks can be examined with qualitative conceptual models (Zador et al., 2017). For example, a conceptual model of the system has been developed for Chinook salmon in Central California (Wells et al., 2016; Figure 3). Winship et al. (2015) explored the utility of incorporating metrics of environmental conditions identified in that framework into annual abundance forecasts made for Sacramento River Fall Chinook, typically the predominant contributor to ocean salmon fisheries off California and much of Oregon. Many of the indicators highlighted in that conceptual model are currently reported to fisheries managers in annual reports to the regional management council (i.e., PFMC); this is done to improve decisionmaker and stakeholder understanding of the status and trends of key physical and biological indicators throughout the CCE (Levin et al., 2009; Pacific Fisheries Management Council [PFMC], 2013; California Current Integrated Ecosystem Assessment [CCIEA], 2020). The timing of the report (in March of each year) coincides with the timing of key salmon harvest management decisions. However, the indices provided in the ecosystem status report are explicitly intended to not be formally linked to specific control rules or targets for Council-managed species. Extending the current indicator approach onto this model, for example by pairing the indices described in the report with quantitative analyses (e.g., path analysis; Fan et al., 2016), could provide managers with both qualitative and quantitative advice regarding predicted changes in salmon recruitment (Burke et al., 2013b). Deyle et al. (2013, 2016) also provide non-linear modeling examples in which environmental factors likely affecting fishes are identified, with only limited knowledge of direct or indirect mechanisms, and used to forecast recruitment.
Figure 3. The conceptual model represents the interactions within the ecosystem directly or indirectly affecting salmon survival at sea in central California (based on Wells et al., 2016). As an example of presenting risk of increased early mortality and recruitment in 2015, we have placed the state of individual indicators, previously identified as relating directly or indirectly to salmon survival onto the conceptual model and colored them according to whether they were average (yellow), below average (red), or above average (green) relative to their impact on salmon.
Assessing fish populations under different scenarios is as relevant to restoration and recovery efforts as it is to fisheries. For restoration, we evaluate the risk of failing to meet sustainability and restoration goals over time rather than overfishing risk to a cohort. For mixed-stock ocean troll fisheries like those for West Coast Chinook salmon, salmon management has a limited ability to respond to shifts in ocean and freshwater ecosystems, essentially curtailing fishing effort when assessed abundance of target and conservation stocks are forecasted to fall below conservation thresholds. These thresholds are based in part on estimates of stock productivity and carrying capacity that do not account for shifts in the ecosystem, such as changing abundances of salmon predators and prey, marine heat waves, changes in ocean circulation, and terrestrial droughts. Ignoring these changes puts salmon stocks at increased risk, but the temporal and spatial variations in these risks are rarely predictable in advance. Therefore, an important objective is to evaluate risk to salmon and associated species by examining how variability in any given ecosystem component affects other components based on findings from Tier 2 efforts.
Unidirectional ecosystem considerations (as depicted in Figure 2 and expanded on during Tier 2 efforts) can be incorporated in management decisions by including ecosystem information into assessment models (Dolan et al., 2016). Including an environmental covariate (e.g., temperature) or an adjustment to natural mortality linked to predation could potentially improve single-species assessment models by relating demographic characteristics to ecosystem variability. The successful improvement of assessments would provide a more accurate and precise estimate of abundance and, therefore, reduce risk to salmon populations (e.g., falling below escapement goals) related to the interactions between managerial practices and environmental conditions. However, process studies should inform these relationships because if they are not founded on mechanisms, they may lead to conclusions that are spurious or unstable outside of the range of conditions that have been observed (Myers, 1998; Jacox et al., 2020).
Improvements to short-term forecasts (1 year ahead of return) of potential risk of reduced salmon recruitment and productivity have been attempted by including covariates representing large and regional-scale oceanography (e.g., Logerwell et al., 2003; Haeseker et al., 2005; Rupp et al., 2012), southward transport of copepods (Peterson and Schwing, 2003), overall ecosystem state (Burke et al., 2013b), and breeding success of central-place foragers (Roth et al., 2007). Unfortunately, while these methods may be consulted for advising management qualitatively, the accuracy of results is inconsistent for implementation in tactical management models. Statistical evaluation of similar models demonstrates that inclusion of ecosystem variables has not provided sufficient improvement over current approaches that rely solely on observed salmon dynamics (e.g., Haeseker et al., 2005; Winship et al., 2015); this is a problem that is shared across a fairly broad spectrum of life-history types (Haltuch et al., 2019). There is also often instability in individual environmental drivers identified as important over time (e.g., Winship et al., 2015; Litzow et al., 2018).
Various climate indices correlated with salmon returns, such as the Pacific Decadal Oscillation (PDO) index, match variability in available longer biological time-series (Malick et al., 2015). However, these coarse indicators appear to be less correlated to salmon returns in recent years or for individual stocks. For example, the relationship between PDO and adult returns of Columbia River spring Chinook has weakened, possibly due to detrending temperature time series and the fact that cold phases of the PDO have not paired with high returns of salmon as in the past (Mantua et al., 1997). In addition, the effects of slowly changing environmental drivers on abundance will be indirectly incorporated into forecast models based on recent abundance through temporal autocorrelation, such that there may be little benefit from explicitly adding such indicators to forecast models.
One promising example in the CCE that includes environmental conditions is an ensemble modeling approach with environmental covariates (i.e., PDO, an El Niño index, sea surface height, and upwelling dynamics) used to forecast Oregon coastal coho salmon ocean abundance (Pacific Fisheries Management Council [PFMC], 2018). An ensemble approach may accommodate the shifting importance of individual environmental drivers. State-space population models incorporating multiple indicators of abundance and/or expected effects of the environment on demographic rates might also lead to improved forecasts. For example, an initial highly uncertain forecast of cohort strength might be made based on spawners and the stock-recruit relationship. This could be treated as a Bayesian prior, updated as information accumulates through estimates of juvenile outmigration, stock-specific catch rates in juvenile ocean trawl surveys, observations of environmental conditions with strongly supported modeled effects on key vital rates, and/or the returns of young age classes. Although, likely only some of these stages would be sufficiently measurable in most systems. Michielsens and Cave (2018) describe a conceptually similar approach where in-season assessment of Fraser River sockeye is improved through incorporation of updated information from test fisheries.
Many currently used salmon forecasts along the CCE rely solely on observed salmon numbers at earlier stages in the lifecycle – i.e., using spawners, smolt outmigrants, or early returns of immature fish (jacks) to predict the abundance of adults from the same cohort. This may limit the ability of environmental covariates, particularly those measured at the critical time around ocean entry and applied to sibling-based forecasts, to increase the predictive power of these short-term forecasts because they already incorporate effects of early environmental conditions on those cohorts (Satterthwaite et al., in press). A better understanding of drivers of changes in maturation rates or mortality during later years of ocean residency may have greater potential to improve forecasts than focusing on the crucial ocean entry period, because such factors would change the expected ratios of abundances at different age classes. An ecosystem perspective may be particularly important here if changes in maturation rates are driven by changes in growth rates mediated by changes in prey abundance, and if changes in mortality rates are driven by changes in the abundance of predators and/or prey.
Strategic management in support of restoration and recovery efforts requires retrospective and future ecosystem condition response evaluations. Over a longer time period, implementation of life-cycle models can provide an indication of vulnerability related to trends in habitat degradation and climate change for individual portions of the life history (Crozier et al., 2008).
Consideration must be given to developing tools for evaluating the cumulative risk that are inclusive of changes and feedbacks within the ecosystem (Holsman et al., 2017). The approaches for forecasting risk we have discussed provide an evaluation of additive risk analysis with single–pressures affecting single (or multiple) ecosystem components in succession. Pressures from the environment or predators affect individual ecosystem components, but variability in ecosystem structure and interactions and feedbacks can be important for understanding salmon survival (e.g., Buhle et al., 2009; Holsman et al., 2010) and forecasting risk of poor recruitment and lower population viability (Holsman et al., 2017). For example, timing of the fixed-gear Dungeness crab fishery may cause some fishers to extend their participation in such fisheries when salmon are less available (Richerson and Holland, 2017). Variability in the timing and effort of a fixed-gear fishery can exacerbate whale entanglements (Johnson et al., 2005; Neilson et al., 2009; Santora et al., 2020). Complex, multidirectional conceptual models based on new research findings can act initially as a framework to develop a coupled bio-physical and mechanistic model (e.g., agent-based model and end-to-end) allowing for emergent negative and positive feedbacks and cumulative risk of poor states (e.g., recruitment failure of salmon and increased mortality of seabirds) across ecosystem attributes (e.g., Scheuerell et al., 2006; Honea et al., 2016).
Once critical risks and vulnerabilities to salmon fisheries and salmon restoration efforts have been described, tools will be needed to evaluate trade-offs associated with potential management strategies, and to provide simulated output to evaluate the possibility of each of those strategies to mitigate risks. Here, we provide potential avenues for estimating trade-offs associated with management strategies. Evaluating and managing tradeoffs is an inherently complex, multidirectional application of results from Tiers 1, 2, and 3 and requires collaborations between invested and knowledgeable community members (Table 2; Jones et al., 2016). In general, simulation models that incorporate the physical environment, food-web elements (including salmon), and human dimensions will be needed. Previous studies have reviewed the variety of models used for strategic and tactical EBM advice (Hobday et al., 2011; Plagányi et al., 2011; Harvey et al., 2016; Holsman et al., 2017) and provide guidance on the utility of different modeling frameworks. Holsman et al. (2017) characterized such models as existing on a continuum of mechanistic complexity and ranging from qualitative to quantitative frameworks and suggest that a coupled modeling approach may provide an ability to rapidly explore trade-offs and prioritize species and scenarios for highly quantitative evaluations of risk and uncertainty. These range from qualitative and semi-quantitative conceptual models to fully deterministic whole-of-ecosystem models.
Conceptual models guide management decisions and risk evaluation by identifying a variety of strategic and tactical pathways including context for decisions based on explicitly delineated conceptual models and qualitative assessments of change in indicators of ecosystem state (Harvey et al., 2016; Zador et al., 2017). These approaches can be used to prioritize interactions and management scenarios to examine through quantitative model evaluations of trade-offs appropriate for tactical advice (e.g., harvest control rules) for fisheries (including but not limited to harvest control rules; Holsman et al., 2017) and strategic advice for restoration and recovery of salmon (e.g., freshwater habitat and weak stock management). Ultimately, while conceptual models provide direction and context for potential management actions, they do not provide a quantitative evaluation of tradeoffs, and thus provide limited criteria by which to evaluate risks or potential management scenarios.
Models of intermediate complexity (MICE; Plagányi et al., 2014) are useful for evaluating potential outcomes of management scenarios. MICE represent the ecosystem with inclusion of only environmental variables and taxa (often aggregated) critical to management scenarios being examined, and remaining processes are often modeled implicitly as observation or process error. MICE have both statistical fitting aspects and mechanistic components driven by hypotheses about the structure and function of ecosystems, with at least one ecological process (e.g., an interspecific interaction or spatial habitat use) represented explicitly (Plagányi et al., 2014). MICE can therefore capture mechanistic relationships as well as characterize error and uncertainty in parameter estimates. Thus, MICE provide a potentially more reliable approach to testing tactical management scenarios than purely statistically based regression approaches, while also projecting risk and accounting for uncertainty (Plagányi et al., 2014). The statistical fitting aspects of MICE can be accomplished in either a maximum likelihood or Bayesian context, in the latter case prior information can be incorporated when justified, and risk predictions can be interpreted as probabilities (conditional on model structure/assumptions and the priors). However, MICE may not be the best approach when multiple ecological processes or a large number of species all have strong effects on the output of interest.
Life-cycle models are particularly well suited to model salmon across varied habitats and can be used to evaluate risks and trade-offs associated with strategic management actions across their full life cycle (Zabel et al., 2006; Crozier et al., 2008; Scheuerell et al., 2009; Hendrix et al., 2014; Friedman et al., 2019). The models are conceptually simple stage-structured population models, typically including demographics at each stage of life for which there is abundance data (Paulik, 1973). The addition of ecosystem drivers (e.g., oceanographic and predators) with mechanistic connections to salmon recruitment (as demonstrated in Tiers 1 and 2) may provide a valuable tool for developing strategic plans for improving resilience of salmon. Life-cycle models can be formulated in any number of ways. When structured appropriately, they can accommodate the life-history variability underlying portfolio effects and sublethal effects that carry over from one habitat to another.
Currently, ocean processes other than harvest are treated simplistically in most salmon life-cycle models (e.g., Crozier et al., 2008; Hendrix et al., 2014). However, following findings resulting from Tier 2 efforts, models for many of the primary ocean processes, as suggested in Figure 2, can be improved. As an example, based on a specified conceptual model in which proximate drivers of salmon dynamics were identified from empirical studies, Friedman et al. (2019) determined from a California fall-run Chinook salmon life-cycle model that freshwater temperatures during egg incubation, rearing and outmigration flows, and marine predation were the most influential contributors toward fitting variability in escapement over 30 years. Friedman et al. (2019) then examined the outcome to salmon of potential management strategies in freshwater habitats in the context of increasing marine predation. These approaches are successful at capturing temporal aspects of salmon interactions within the ecosystem and identifying the variables and life-history periods for which salmon recruitment variability is most sensitive. However, it is evident that spatially explicit models should be included in these approaches. Growth (Fiechter et al., 2015), condition (Wells et al., 2012; Sabal et al., 2020), and at-sea survival (Wells et al., 2017; Henderson et al., 2019) vary at fine-, local-, and regional-scales. Agent-based models could be valuable modules to life-cycle modeling approaches because they model prey, salmon and predator behaviors in relationship to the dynamic environments they experience.
Spatially explicit, mechanistic models would allow us to implement agent-based model simulations under various environmental, trophic, and climate-change scenarios. One area of research that is of particular importance to agent-based models, as pointed out in our Tier 2 discussion, is the parametrization of feeding behavior which not only fuels growth but also spatiotemporal dynamics of the salmon and the spatial extent of the ecosystem with which they interact.
Agent-based models could be powerful management tools for evaluating strategies and providing strategic advice for mitigating trends and variability in the ecosystem. Such simulations could provide additional insights about specific environmental conditions (e.g., low productivity years associated with warm extremes). These simulation results may also form life-stage components of current life-cycle modeling efforts for salmon. In developing agent-based models, empirical observations should be linked to process studies to parameterize the models. Studies should focus on individual fish characteristics and rely on thorough biological (e.g., diet, otoliths, and lipid content) and environmental data that may include data derived from oceanographic model output. One concern, however, is that agent-based models are sensitive to data and assumptions going into the model (Byron and Burke, 2014; Burke et al., 2016), including propagation of errors in oceanographic model results that are used as inputs. Therefore, careful parameterization should be paired with a suite of field-based efforts to collect data or refine sensitive parameters (Table 1). Future research identified in section Tier 2 may serve the dual purpose of refining our understanding of salmon ecology in the ocean and providing improved inputs to mechanistic agent-based models.
Ecosystem models, such as Atlantis (Fulton, 2010; Kaplan et al., 2019), are useful for integrating the effects of climate, trophic, and fishery impacts and their interactions on salmon survival, restoration, and fisheries. They can be especially useful for scenario exploration and identification of sensitivities and reinforcing non-intuitive feedbacks (e.g., Kaplan et al., 2019) and for evaluating interactions within different conceptual model formulations of the ecosystem. New approaches include coupling climate-enhanced single-species, agent-based models, MICE, and ecosystem models to Regional Ocean Modeling Systems using nutrient-phytoplankton-zooplankton models (e.g., Ito et al., 2013; Fiechter et al., 2015; Woodworth-Jefcoats et al., 2015; Hermann et al., 2016; Ortiz et al., 2016). Increasingly, suites of models are being simultaneously deployed in large-scale-multimodel comparative projects focused on climate change (e.g., Tittensor et al., 2018; Future Seas2). One drawback of end-to-end models is their reliance on well-parameterized, explicit decisions about functional form or parameter values within an ecosystem that ultimately operates through myriad interactions. Generally, more complexity in a modeling approach can reduce bias but uncertainty in output increases as a result of parameter uncertainty (Collie et al., 2016).
The approach for evaluation of management scenarios should accommodate objectives, available science, and the risks and possible management actions that need to be addressed. Generally, flexibility in model structure and parameterization increases applicability toward addressing changing objectives. Along the CCE, life-cycle models are being pursued due, in part, to their flexibility to evaluate cumulative environmental and managerial pressures and their capacity to include various modeling frameworks to grow more complex as we advance our understanding of the system. MICE models provide the potential for addressing specific objectives in a relatively tractable model that is relatively easy to parameterize, and their structure can be tuned toward evaluating the efficacy of specific management strategies within a life-cycle framework. Agent-based models, in spite of their potential complexity, have been successful at capturing ecosystem dynamics in well-understood systems but they are reliant on well-defined rules for how individual agents behave/respond to the environment which are notoriously difficult to quantify. If the agent-based model’s behavioral rules or functional forms are unrealistic errors will propagate, resulting in unrealistic dynamics. However, a well-defined agent-based model could be embedded in a life-cycle model approach to capture ecosystem dynamics during particular life stages (e.g., early-life ocean component; Fiechter et al., 2015; Henderson et al., 2019) and evaluate spatiotemporal interactions between salmon and their ecosystem, including their prey and predators. While end-to-end models provide hope for an holistic and flexible approach to management scenario evaluation, their full use remains elusive because they are difficult to parameterize. Therefore, research should focus on parametrizing key elements of these model approaches (e.g., food habits, behaviors, and distribution models) because once they are well parametrized and potentially inclusive of MICE, agent-based models and other modeling subcomponents, they will be an appropriate approach for addressing multidirectional, complex objectives. However, for unidirectional, simpler objectives the simpler models will suffer less model uncertainty (Collie et al., 2016) and can be modified as objectives, risks and the ecosystem vary. In the end, we advise using the simplest model capable of addressing the objectives while maintaining flexibility. There is no single best approach, however, the life-cycle modeling framework is flexible and may include embedded models, capture spatiotemporal variability, and address life-stage specific objectives.
The vast majority of models that include ecosystem information developed in research programs rarely become central to management of fisheries, population resilience, nor the landscape or seascape (Skern-Mauritzen et al., 2016). One cause is inadequate communication with stakeholders about relevant model inputs, outputs and dynamics, and lack of convincing evidence that the new model will provide better management (Collie et al., 2016; Sterling et al., 2019). In part, this is less a matter of trust in an EBM approach but rather a vagueness and generic presentation of its application on a case-by-case basis (Lidström and Johnson, 2020). Operationalizing model results for management advice will require a clear and iterative dialog with stakeholders about uncertainties and limitations of modeling frameworks (Table 2). There are multiple reasons why environmental or socioeconomic indices could be explicitly included in an operational management model and final adoption should depend on individual management needs, structure, process, and priorities.
Researchers need to bring their findings from this proposed program to management and the public to help inform objectives, paths forward, and actions. Including researchers, managers, fishers and other stakeholders during objective scoping, risk analysis, management scenario evaluation, and implementation can go a long way toward alleviating concerns of a lack of transparency and poor representation, and can ultimately reduce conflict. An inclusive research, modeling, and policy process can facilitate positive relationships and bring future managerial advice into action. There is a good example from the Great Lakes that demonstrated this approach. A binational management body, the Lake Erie Committee, developed an inclusive advisory committee of fishery managers, researchers, and stakeholders to develop successful policies to manage Lake Erie walleye (Sander vitreous) (Jones et al., 2016). Prior development of the advisory board, concerns over a lack of transparency and trust led to conflict between fishery sectors, researchers, and managers. A stakeholder-engaged process used Structured Decision Making and guidance from an advisory committee to define objectives, manage expectations, and successfully develop management actions (e.g., harvest control rules).
Within the central CCE, an example of ecosystem-level research successfully informing management resulted from Santora et al. (2020). That research demonstrated that persistent ocean-warming resulted in habitat compression, causing decline in upwelling habitat and altering the distribution and abundance of forage species and subsequently the shoreward distribution shift of humpback whales, leading to higher co-occurrence with Dungeness crab fishing gear and record numbers of entanglements. Importantly, the synthesis by Santora et al. (2020) was conducted in partnership with a diverse stakeholder group to ensure that ecosystem science helped to guide considerations for developing recommendations to manage the crab fishery to mitigate the whale entanglement problem. In response, the California Department of Fish and Wildlife convened the California Dungeness Crab Fishing Gear Working Group in partnership with California Ocean Protection Council and National Marine Fisheries Service to apply an ecosystem perspective for assessing entanglement risk to wildlife and socio-economic impacts to the fishery. This working group examines risk of entanglements throughout the fishing season and advises Dungeness crab fishery timing and extent in the context of ocean-climate and ecosystem conditions.
California Current Ecosystem salmon management would similarly benefit from stakeholder consensus building and better availability of relevant ecosystem-level information. As we have tried to make clear, the path between variability in any given ecosystem component and the response of salmon, fisheries, and predator recovery can be circuitous (Figure 2). By association, relaying information to stakeholders and decisions makers can be complex and often confusing. For example, when an unusually strong marine heat wave warmed the ocean off the West Coast from late 2014 to 2016, the effects reverberated through the marine ecosystem (Cavole et al., 2016). One of the telltale changes was in the copepod community which subsequently determined the quality of the northern CCE food web (Peterson and Schwing, 2003). Instead of cool-water energy-rich copepods from the north that promote a rich prey base that helps fish grow quickly, leaner more southern copepods with less energy began to dominate (Bond et al., 2015; Cavole et al., 2016). That left young salmon facing tougher odds for survival in the ocean. In the following years, salmon returns fell to some of their lowest levels in a decade and a series of Federal Fishery Disaster declarations for the West Coast ensued. This reduction in salmon fisheries can consequently increase effort in fixed-gear fisheries through cross-participation, which then can increase whale entanglements (Santora et al., 2020). However, even with such a comprehensive understanding of the system, the vast majority of models that include such ecosystem information developed in research programs rarely become central to management (Skern-Mauritzen et al., 2016). A fundamental challenge is that the information can be so complex that the problem lies in the understanding, alternatively the message can be loud and clear but does not result in a behavior change due to inadequate motivation.
Good communication begins by appreciating that there are multiple participants - senders and receivers. Each brings to the conversation their own history, language, perspective, and needs. These influence how effectively information is translated from one person to another. That is, a researcher may think the information was conveyed clearly and understandably, while the manager may be utterly confused by the jargon or technical details. This is why it is critical to consider any interaction as a dialog built on an appreciation that people are entering into the conversation from different points with varying expectations. This evolving dialog can be guided and adapted with Structured Decision Making (Conroy and Peterson, 2013).
To help promote better communication, one question researchers ask is “What information do stakeholders need and when do they need it?” Resource managers have an important job. The information demands are great, and management errors could lead to fish stock collapses and economic damage to coastal communities. The barriers for using new or unfamiliar information under such circumstances can be high. Incorporating new inputs into the existing, well-established management processes takes time and trust. The information should be presented in a way that is understandable and clearly expresses the inherent uncertainties and limitations of the information. For example, the discussion can be rooted in a mutually agreed upon conceptual model of the system and goals (e.g., Figure 2).
It is also important when the information is presented. Assimilating new information is difficult enough under the best of circumstances. Often, managers and stakeholders are asked to take in new information under pressure; during the management process when a decision must be made. In such a case, the manager is not in discovery or learning mode. There is simply no time to adequately process complex information and ask pertinent questions. Instead, one consideration could be providing opportunities to share information outside the normal business of the management process and away from the pressure of decision-making and judgment. Most stakeholders involved in resource management are not technical experts. Given this unfamiliarity, they will need an opportunity to learn in a structured way. That is, rather than dumping all the information on them at once, consideration should be given to distributing information in understandable chunks that slowly build knowledge and confidence over time. Such a format provides the space for questions and honest exchanges of concerns, fears, and perspectives.
The results of an ocean research program should provide guidance to enable adaptive management of salmon fisheries, improve resilience of salmon populations and the ecosystem, and protect and rebuild endangered and threatened populations so that salmon can be maintained at desired levels in the face of changes in the ecosystem. Adaptive management requires predictions about how systems will respond to management actions, evaluation of the predictions following management against observations of the actual system response, and adjustments of the prediction tools and management strategies as more is learned from observing the system response.
As researchers accumulate findings through the EBM process it is important to re-evaluate and adapt (Tier 6) the objectives, science, risks, and outcomes such that their advice matches ecological, societal, and managerial needs. Figure 1B demonstrates the iterative nature of re-evaluation and adaptation in the context of the six tiers of complexity. As work progresses toward more integrated products, those products (e.g., research findings, risk assessment, and actionable managerial advice) should be re-evaluated and, if at any point in the process they run counter to current objectives, science, risks or realistic managerial actions, practitioners should step back in the process and realign efforts from that point forward. The sequence of re-evaluation and adaptation increases in complexity from identifying objectives to advising management (Figure 1B). The flow can be shown as a series of questions to consider. For example, after completing Tier 1 efforts: Are the objectives and current understanding consistent with what is known and agreeable to any new members of an advisory body? Onward: Are new or unconsidered knowledge gaps in our understanding related to objectives being addressed? Are we addressing the most important risks related to our objectives in the context of our understanding of the system? Do we have confidence in results from our management scenario evaluations and their capacity to address observed risks associated with our objectives? And finally, are the results of the process resulting in actionable advice and if so, is it being used? At all points during this re-evaluation the advisory body of stakeholders, managers, and modelers ideally should be looped into discussions and be party to decisions continuing forward (Jones et al., 2016).
Monitoring ocean processes is important to provide indications of current or pending issues that may require adapting the research direction and/or approach. A robust monitoring system paired with process studies will aid in identifying variability and trends in system structure and function (Link et al., 2015; Busch et al., 2016; Sigler et al., 2016). Climate change has been linked to instability of the monitored environment, productivity, and trophic pathways (Sydeman et al., 2013; Jacox et al., 2016, 2018; Holsman et al., 2018). For example, the early 2000s were associated with the greatest modern-era escapement numbers recorded for some California Chinook salmon stocks but by 2007 the Central Valley Fall-run Chinook salmon population collapsed (Lindley et al., 2009). Increasing predation on salmon from predators seeking alternative prey during a period of delayed upwelling was the proximate cause of recent poor salmon survival (Wells et al., 2017; Friedman et al., 2019), but a long-term decline of life-history variation linked to freshwater habitat degradation, harvest and hatchery practices increased the susceptibility of salmon to poor ocean conditions (Lindley et al., 2009). Importantly, in the last few decades a number of predator populations have recovered to a point that they must be considered in an ecosystem approach to managing salmon (Chasco et al., 2017). In an effort to reduce predation on salmon, culling of sea lions and piscivorous birds has been proposed and/or implemented along the CCE notably in the Columbia River estuary (Roby et al., 2005; Wargo Rub et al., 2019). However, research findings resulting from our proposed program may provide the information needed to accommodate synchronous recovery of predators, forage, and salmon (Samhouri et al., 2017). As contemporary climate trends continue in concert with the increased abundance of predators and a varying forage base, science to support resilience of salmon populations and their ecosystem will rely on re-evaluating conceptual models of the system, identification of new research gaps, and development of well-parameterized contemporary operational models for advising management.
Over the last two decades a great deal of research has been dedicated to quantifying ecology of salmon at sea. Much of this work was performed with the goal of improving tactical management of salmon, largely focusing on assessment model improvements. For salmon in the CCE only one assessment model currently includes a measure of ocean conditions (i.e., Oregon coastal coho salmon; Pacific Fisheries Management Council [PFMC], 2018). Where our last two decades has failed to consistently improve on salmon assessment beyond the inclusion of salmon demographics and observed dynamics, we have succeeded at identifying a number of mechanisms in the ocean that are complicated and non-intuitive, but have dramatic effects on mortality of salmon. For example, predation impacts on salmon may be more significant under conditions of poor ocean productivity (e.g., low upwelling), during which predation can be the dominant determinant of later adult salmon abundance.
Individual salmon populations, coastal communities, and regional fisheries represent unique situations requiring latitude to tailor approaches to meet identified objectives. We provide general guidance on how to do that (Tables 1, 2) and, for specificity, we provide an example application in Box 1. Some generalities, however, can be summarized: (1) purpose-built conceptual models representing the contemporary understanding of the ecosystem are useful for developing a research program and applying its results to management, (2) we have a lack of understanding of some salmon behavior, their dynamics during winter, and the vast majority of research efforts have been dedicated to Chinook and coho salmon with little focused on remaining species, (3) improvements should continue to be made on salmon assessment models through research on maturation rates and environmental drivers, and importantly, risk assessments should be cumulative across ecosystem components and consider variability in the ecosystem structure and trophic pathways, (4) models to evaluate potential tradeoffs associated with imagined management scenarios should be tailored to specific goals and models should only be as complex and necessary (Collie et al., 2016), and (5) well-designed interactive communication and consideration of the full suite of relevant opinions is a requirement in successfully adopting the findings of a research program into management (Sterling et al., 2019). Finally, we need to acknowledge that the ecosystem is not static, the variability in its structure and function must be considered, and the outcome of all Tiers in EBM must be re-evaluated and adapted as needed. As climate trends continue to affect freshwater habitats and ocean conditions, and as both predator populations and their consumptive demands change (Chasco et al., 2017), it is likely the ecosystem that exists today will be significantly different in the coming decades. Our proposed approach attempts to situate us to accommodate this change.
BW, DH, BB, and RB provided the underlying concepts and framework. Remaining authors represented specific areas of knowledge (i.e., JS ecosystem oceanography. JF ecosystem-based management. NM oceanography. WS and MM population assessment. SK and FD communications. RZ, SL, and KF life-cycle modeling. KR human dimensions).
The authors declare that the research was conducted in the absence of any commercial or financial relationships that could be construed as a potential conflict of interest.
We thank C. Harvey, K. Holsman, L. Krasnow, J. Link, and M. Malick and reviewers for constructive comments and review of earlier drafts.
Allen, S. D., Satterthwaite, W. H., Hankin, D. G., Cole, D. J., and Mohr, M. S. (2017). Temporally varying natural mortality: sensitivity of a virtual population analysis and an exploration of alternatives. Fish. Res. 185, 185–197.
Beacham, T. D., Neville, C. M., Tucker, S., and Trudel, M. (2017). Is there evidence for biologically significant size-selective mortality of coho salmon during the first winter of marine residence? Trans. Am. Fish. Soc. 146, 395–407.
Beacham, T. D., Wallace, C., Macconnachie, C., Jonsen, K., Mcintosh, B., Candy, J. R., et al. (2018). Population and individual identification of Chinook salmon in British Columbia through parentage-based tagging and genetic stock identification with single nucleotide polymorphisms. Can. J. Fish. Aquat. Sci. 75, 1096–1105. doi: 10.1111/eva.12711
Beamish, R. J. (2018). The Ocean Ecology of Pacific Salmon and Trout. Bethesda, MD: American Fisheries Society.
Beamish, R. J., and Mahnken, C. (2001). A critical size and period hypothesis to explain natural regulaiton of salmon abudnace and the linkage to climate and climate change. Prog. Oceanogr. 49, 423–435.
Beamish, R. J., Mahnken, C., and Neville, C. M. (2004). Evidence that reduced early marine growth is associated with lower marine survival of Coho salmon. Trans. Am. Fish. Soc. 133, 26–33.
Beckman, B. R., Gadberry, B., Parkins, P., and Larsen, D. A. (2008). The effect of yakima river spring chinook salmon sire life history type on emergence timing and size of progeny. Trans. Am. Fish. Soc. 137, 1285–1291.
Bi, H., Ruppel, R. E., and Peterson, W. T. (2007). Modeling the pelagic habitat of salmon off the Pacific Northwest (USA) coast using logistic regression. Mar. Ecol. Prog. Ser. 336, 249–265.
Bigler, B. S., Welch, D. W., and Helle, J. H. (1996). A review of size trends among North Pacific salmon (Oncorhynchus spp.). Can. J. Fish. Aquat. Sci. 53, 455–465.
Bond, N. A., Cronin, M. F., Freeland, H., and Mantua, N. (2015). Causes and impacts of the 2014 warm anomaly in the NE Pacific. Geophys. Res. Lett. 42, 3414–3420.
Brennan, S. R., Schindler, D. E., Cline, T. J., and Walsworth, T. E. (2019). Shifting habitat mosaics and fish production across river basins. Science 364, 783–785. doi: 10.1126/science.aav4313
Breslow, S., Holland, D., Levin, P., Norman, K., Poe, M., Thomson, C., et al. (2013). “Human dimensions of the CCIEA: a summary of concepts, methods, indicators, and assessments,” in The California Current Integrated Ecosystem Assessment: Phase III Report, eds C. J. Harvey, C. J. Garfiled, N. Hazen, and G. D. Williams, (Silver Spring, MD: NOAA).
Brodeur, R. D., Boehlert, G. W., Casillas, E., Eldridge, M. B., Helle, J. H., Peterson, W. T., et al. (2000). A coordinated research plan for estuarine and ocean research on Pacific salmon. Fisheries 25, 7–16.
Brodeur, R. D., Daly, E. A., Schabetsberger, R. A., and Mier, K. L. (2007a). Interannual and interdecadal variability in juvenile coho salmon (Oncorhynchus kisutch) diets in relation to environmental changes in the northern California Current. Fish. Oceanogr. 16, 395–408.
Brodeur, R. D., Daly, E. A., Sturdevant, M. V., Miller, T. W., Moss, J. H., et al. (2007b). “Regional comparisons of juvenile salmon feeding in coastal marine waters off the west coast of North America,” in Ecology of Juvenile Salmon in the Northeast Pacific Ocean: Regional Comparisons, eds C. B. Grimes, R. D. Brodeur, L. J. Haldorson, and S. M. McKinnell, (Bethesda, MD: American Fisheries Society, Symposium), 57.
Brodeur, R. D., Fisher, J. P., Teel, D. J., Emmett, R. L., Casillas, E., and Miller, T. W. (2004). Juvenile salmonid distribution, growth, condition, origin, and environmental and species associations in the Northern California Current. Fish. Bull. 102, 25–46.
Brodeur, R. D., and Morgan, C. A. (2016). Influence of a Coastal Riverine Plume on the Cross-shelf Variability in Hydrography, Zooplankton, and Juvenile Salmon Diets. Estuar. Coasts 39, 1183–1198.
Buhle, E. R., Holsman, K. K., Scheuerell, M. D., and Albaugh, A. (2009). Using an unplanned experiment to evaluate the effects of hatcheries and environmental variation on threatened populations of wild salmon. Biol. Conserv. 142, 2449–2455.
Burke, B. J., Anderson, J. J., and Baptista, A. M. (2014). Evidence for multiple navigational sensory capabilities of Chinook salmon. Aquat. Biol. 20, 77–90.
Burke, B. J., Anderson, J. J., Miller, J. A., Tomaro, L., Teel, D. J., Banas, N. S., et al. (2016). Estimating behavior in a black box: how coastal oceanographic dynamics influence yearling Chinook salmon marine growth and migration behaviors. Environ. Biol. Fishes 99, 671–686.
Burke, B. J., Liermann, M. C., Teel, D. J., and Anderson, J. J. (2013a). Environmental and geospatial factors drive juvenile Chinook salmon distribution during early ocean migration. Can. J. Fish. Aquat. Sci. 70, 1167–1177.
Busch, D. S., Griffis, R., Link, J., Abrams, K., Baker, J., Brainard, R. E., et al. (2016). Climate science strategy of the US national marine fisheries service. Mar. Policy 74, 58–67.
Burke, B. J., Peterson, W. T., Beckman, B. R., Morgan, C., Daly, E. A., and Litz, M. (2013b). Multivariate models of adult Pacific salmon returns. PLoS One 8:e54134. doi: 10.1371/journal.pone.0054134
Byron, C. J., and Burke, B. J. (2014). Salmon ocean migration models suggest a variety of population-specific strategies. Rev. Fish. Biol. Fish. 24, 737–756.
California Current Integrated Ecosystem Assessment [CCIEA] (2020). California Current Ecosystem Status Report, 2020. Report to the Pacific Fishery Management Council. Available online at: https://www.pcouncil.org/documents/2020/02/g-1-a-ieateam-report-1.pdf/
Carlson, S. M., and Satterthwaite, W. H. (2011). Weakened portfolio effect in a collapsed salmon population complex. Can. J. Fish. Aquat. Sci. 68, 1579–1589.
Cavole, L. M., Demko, A. M., Diner, R. E., Giddings, A., Koester, I., Pagniello, C. M., et al. (2016). Biological impacts of the 2013–2015 warm-water anomaly in the Northeast Pacific: Winners, losers, and the future. Oceanography 29, 273–285.
Chasco, B. E., Kaplan, I. C., Thomas, A. C., Acevedo-Gutiérrez, A., Noren, D. P., Ford, M. J., et al. (2017). Competing tradeoffs between increasing marine mammal predation and fisheries harvest of Chinook salmon. Sci. Rep. 7:15439. doi: 10.1038/s41598-017-14984-8
Collie, J. S., Botsford, L. W., Hastings, A., Kaplan, I. C., Largier, J. L., Livingston, P. A., et al. (2016). Ecosystem models for fisheries management: finding the sweet spot. Fish Fish. 17, 101–125.
Conroy, M. J., and Peterson, J. T. (2013). Decision Making in Natural Resource Management: A Structured, Adaptive Approach. Hoboken, NJ: John Wiley & Sons.
Crozier, L., and Zabel, R. W. (2006). Climate impacts at multiple scales: evidence for differential population responses in juvenile Chinook salmon. J. Anim. Ecol. 75, 1100–1109. doi: 10.1111/j.1365-2656.2006.01130.x
Crozier, L. G., Zabel, R. W., and Hamlett, A. F. (2008). Predicting differential effects of climate change at the population level with life-cycle models of spring Chinook salmon. Glob. Change Biol. 14, 236–249.
Cury, P. M., Shin, Y. J., Planque, B., Durant, J. M., Fromentin, J. M., Kramer-Schadt, S., et al. (2008). Ecosystem oceanography for global change in fisheries. Trends Ecol. Evol. 23, 338–346. doi: 10.1016/j.tree.2008.02.005
Dale, K. E., Daly, E. A., and Brodeur, R. D. (2017). Interannual variability in the feeding and condition of subyearling Chinook salmon off Oregon and Washington in relation to fluctuating ocean conditions. Fish. Oceanogr. 26, 1–16.
Daly, E. A., Auth, T. D., Brodeur, R. D., and Peterson, W. T. (2013). Winter ichthyoplankton biomass as a predictor of early summer prey fields and survival of juvenile salmon in the northern California Current. Mar. Ecol. Prog. Ser. 484, 203–217.
Daly, E. A., Benkwitt, C. E., Brodeur, R. D., Litz, M. N. C., and Copeman, L. A. (2010). Fatty acid profiles of juvenile salmon indicate prey selection strategies in coastal marine waters. Mar. Biol. 157, 1975–1987.
Daly, E. A., and Brodeur, R. D. (2015). Warming ocean conditions relate to increased trophic requirements of threatened and endangered salmon. PLoS One 10:e0144066. doi: 10.1371/journal.pone.0144066
Daly, E. A., Brodeur, R. D., Fisher, J. P., Weitkamp, L. A., Teel, D. J., and Beckman, B. R. (2012). Spatial and trophic overlap of marked and unmarked Columbia River Basin spring Chinook salmon during early marine residence with implications for competition between hatchery and naturally produced fish. Environ. Biol. Fishes 94, 117–134.
Daly, E. A., Scheurer, J. A., Brodeur, R. D., Weitkamp, L. A., Beckman, B. R., and Miller, J. A. (2014). Juvenile Steelhead Distribution, Migration, Feeding, and Growth in the Columbia River Estuary, Plume, and Coastal Waters. Mar. Coast. Fish. 6, 62–80.
Dambacher, J. M., Luh, H. K., Li, H. W., and Rossignol, P. A. (2003). Qualitative stability and ambiguity in model ecosystems. Am. Nat. 161, 876–888. doi: 10.1086/367590
De Master, D. P., Fowler, C. W., Perry, S. L., and Richlen, M. F. (2001). Predation and competition: the impact of fisheries on marine-mammal populations over the next one hundred years. J. Mammal. 82, 641–651.
De Robertis, A., Morgan, C. A., Schabetsberger, R. A., Zabel, R. W., Brodeur, R. D., Emmett, R. L., et al. (2005). Columbia River plume fronts. II. Distribution, abundance, and feeding ecology of juvenile salmon. Mar. Ecol. Prog. Ser. 299, 33–44.
Deyle, E. R., Fogarty, M., Hsieh, C. H., Kaufman, L., Maccall, A. D., Munch, S. B., et al. (2013). Predicting climate effects on Pacific sardine. Proc. Natl. Acad. Sci. U.S.A. 110, 6430–6435. doi: 10.1073/pnas.1215506110
Deyle, E. R., May, R. M., Munch, S. B., and Sugihara, G. (2016). Tracking and forecasting ecosystem interactions in real time. Proc. R. Soc. B Biol. Sci. 283:20152258. doi: 10.1098/rspb.2015.2258
Dolan, T. E., Patrick, W. S., and Link, J. S. (2016). Delineating the continuum of marine ecosystem-based management: a US fisheries reference point perspective. ICES J. Mar. Sci. 73, 1042–1050.
Emmett, R. L., Krutzikowsky, G. K., and Bentley, P. (2006). Abundance and distribution of pelagic piscivorous fishes in the Columbia River plume during spring/early summer 1998-2003: Relationship to oceanographic conditions, forage fishes, and juvenile salmonids. Prog. Oceanogr. 68, 1–26.
Fan, Y., Chen, J., Shirkey, G., John, R., Wu, S. R., Park, H., et al. (2016). Applications of structural equation modeling (SEM) in ecological studies: an updated review. Ecol. Proc. 5:19.
Fiechter, J., Huff, D. D., Martin, B. T., Jackson, D. W., Edwards, C. A., Rose, K., et al. (2015). Environmental conditions impacting juvenile Chinook salmon growth off central California: An ecosystem model analysis. Geophys. Res. Lett. 42, 2910–2917.
Friedman, W. R., Halpern, B. S., McLeod, E., Beck, M. W., Duarte, C. M., Kappel, C. V., et al. (2020). Research priorities for achieving healthy marine ecosystems and human communities in a changing climate. Front. Mar. Sci. 7:5. doi: 10.3389/fmars.2020.00005
Friedman, W. R., Martin, B. T., Wells, B. K., Warzybok, P., Michel, C., Danner, E. M., et al. (2019). Modeling composite effects of marine and freshwater processes on migratory species. Ecosphere 10:e02743. doi: 10.1002/ecs2.2743
Friedman, W. R., Santora, J. A., Schroeder, I. D., Huff, D. D., Brodeur, R. D., Field, J. C., et al. (2018). Environmental and geographic relationships among salmon forage assemblages along the continental shelf of the California Current. Mar. Ecol. Prog. Ser. 596, 181–198.
Furey, N. B., Vincent, S. P., Hinch, S. G., and Welch, D. W. (2015). Variability in migration routes influences early marine survival of juvenile salmon smolts. PLoS One 10:e0139269. doi: 10.1371/journal.pone.0139269
Gosselin, J. L., Zabel, R. W., Anderson, J. J., Faulkner, J. R., Baptista, A. M., and Sandford, B. P. (2018). Conservation planning for freshwater-marine carryover effects on Chinook salmon survival. Ecol. Evol. 8, 319–332. doi: 10.1002/ece3.3663
Gross, M. R., Coleman, R. M., and Mcdowall, R. M. (1988). Aquatic productivity and the evolution of diadromous fish migration. Science 239, 1291–1293. doi: 10.1126/science.239.4845.1291
Haeseker, S. L., Peterman, R. M., Su, Z. M., and Wood, C. C. (2005). Retrospective evaluation of preseason forecasting models for pink salmon. N. Am. J. Fish. Manage. 25, 897–918.
Haltuch, M. A., Brooks, E. N., Brodziak, J., Devine, J. A., Johnson, K. F., Klibansky, N., et al. (2019). Unraveling the recruitment problem: a review of environmentally-informed forecasting and management strategy evaluation. Fish. Res. 217, 98–216.
Hanna, S. S. (1992). Interactions between shellfish and groundfish fisheries on the west coast: implications for system management. J. Shellfish Res. 11, 133–141.
Hare, S. R., Mantua, N. J., and Francis, R. C. (1999). Inverse production regimes: Alaska and West Coast Pacific salmon. Fisheries 24, 6–14.
Harvey, C. J., Reum, J. C. P., Poe, M. R., Williams, G. D., and Kim, S. J. (2016). Using conceptual models and qualitative network models to advance integrative assessments of marine ecosystems. Coast. Manag. 44, 486–503.
Hassrick, J. L., Henderson, M. J., Huff, D. D., Sydeman, W. J., Sabal, M. C., Harding, J. A., et al. (2016). Early ocean distribution of juvenile Chinook salmon in an upwelling ecosystem. Fish. Oceanogr. 25, 133–146.
Hayes, S. A., Bond, M. H., Wells, B. K., Hanson, C. V., Jones, A. W., and MacFarlane, R. B. (2012). “Using archival tags to infer habitat use of central California steelhead and coho salmon,” in Advances in Fish Tagging and Marking Technology, Vol. 76, eds J. R. McKenzie, B. Parsons, A. C. Seitz, R. K. Kopf, M. Mesa, and Q. Phelps (Bethesda, MD: American Fisheries Society), 560.
Henderson, M., Fiechter, J., Huff, D. D., and Wells, B. K. (2019). Spatial variability in ocean-mediated growth potential is linked to Chinook salmon survival. Fish. Oceanogr. 28, 334–344.
Hendrix, N., Criss, A., Danner, E., Greene, C. M., Imaki, H., Pike, A., et al. (2014). Life Cycle Modeling Framework for Sacramento River Winter-Run Chinook Salmon. NOAA Tech. Memo. NMFS-SWFSC-530. (Silver Spring, MD: NOAA).
Hermann, A. J., Gibson, G. A., Bond, N. A., Curchitser, E. N., Hedstrom, K., Cheng, W., et al. (2016). Projected future biophysical states of the Bering Sea. Deep Sea Res. Part II 134, 30–47.
Hertz, E., Trudel, M., Brodeur, R. D., Daly, E. A., Eisner, L., and Farley, E. V. Jr., et al. (2015). Continental-scale variability in the feeding ecology of juvenile Chinook salmon along the coastal Northeast Pacific Ocean. Mar. Ecol. Prog. Ser. 537, 247–263.
Hiddink, J. G., Hutton, T., Jennings, S., and Kaiser, M. J. (2006). Predicting the effects of area closures and fishing effort restrictions on the production, biomass, and species richness of benthic invertebrate communities. ICES J. Mar. Sci. 63, 822–830.
Himes-Cornell, A., and Hoelting, K. (2015). Resilience strategies in the face of short- and long-term change: out-migration and fisheries regulation in Alaskan fishing communities. Ecol. Soc. 20:9.
Hobday, A. J., Smith, A. D. M., Stobutzki, I. C., Bulman, C., Daley, R., Dambacher, J. M., et al. (2011). Ecological risk assessment for the effects of fishing. Fish. Res. 108, 372–384.
Holsman, K., Hollowed, A., Ito, S., Bograd, S., Hazen, E., King, J., et al. (2018). “Climate change impacts, vulnerabilities and adaptations: North Pacific and Pacific Arctic marine fisheries,” in Impacts of Climate Change on Fisheries and Aquaculture: Synthesis of Current Knowledge, Adaptation and Mitigation Options, Vol. 627, eds M. Barange, T. Bahri, M. C. M. Beveridge, K. L. Cochrane, S. Funge-Smith, and F. Poulain, (Rome: FAO Fisheries and Aquaculture Technical Paper).
Holsman, K., Samhouri, J., Cook, G., Hazen, E., Olsen, E., Dillard, M., et al. (2017). An ecosystem-based approach to marine risk assessment. Ecosyst. Health Sustain. 3:e01256.
Holsman, K. K., Mcdonald, P. S., Barreyro, P. A., and Armstrong, D. A. (2010). Restoration through eradication? Removal of an invasive bioengineer restores some habitat function for a native predator. Ecol. Appl. 20, 2249–2262. doi: 10.1890/09-1168.1
Honea, J. M., Mcclure, M. M., Jorgensen, J. C., and Scheuerell, M. D. (2016). Assessing freshwater life-stage vulnerability of an endangered Chinook salmon population to climate change influences on stream habitat. Clim. Res. 71, 127–137.
Huber, E. R., and Carlson, S. M. (2015). Temporal Trends in Hatchery Releases of Fall-Run Chinook Salmon in California’s Central Valley. San Fran. Est. Wat. Sci. 13:23.
Ito, S., Okunishi, T., Kishi, M. J., and Wang, M. Y. (2013). Modelling ecological responses of Pacific saury (Cololabis saira) to future climate change and its uncertainty. ICES J. Mar. Sci. 70, 980–990.
Jacox, M. G., Alexander, M. A., Mantua, N. J., Scott, J. D., Hervieux, G., Webb, R. S., et al. (2018). Forcing of multiyear extreme ocean temperatures that impacted California Current living marine resources in 2016. Bull. Am. Meteor. Soc. 99, S27–S33.
Jacox, M. G., Alexander, M. A., Siedlecki, S., Chen, K., Kwon, Y.-O., Brodie, S., et al. (2020). Seasonal-to-interannual prediction of North American coastal marine ecosystems: Forecast methods, mechanisms of predictability, and priority developments. Prog. Oceanogr. 183:102307.
Jacox, M. G., Hazen, E. L., Zaba, K. D., Rudnick, D. L., Edwards, C. A., Moore, A. M., et al. (2016). Impacts of the 2015-2016 El Nino on the California Current System: early assessment and comparison to past events. Geophys. Res. Lett. 43, 7072–7080.
Johnson, A., Salvador, G., Kenney, J., Robbins, J., Landry, S., and Clapham, P. (2005). Fishing gear involved in entanglements of right and humpback whales. Mar. Mamm. Sci. 21, 635–645. doi: 10.1111/cobi.12590
Johnson, R. C., Garza, J. C., Macfarlane, R. B., Grimes, C. B., Phillis, C. C., Koch, P. L., et al. (2016). Isotopes and genes reveal freshwater origins of Chinook salmon Oncorhynchus tshawytscha aggregations in California’s coastal ocean. Mar. Ecol. Prog. Ser. 548, 181–196.
Jones, M. L., Catalano, M. J., Peterson, L. K., and Berger, A. M. (2016). “Stakeholder-centered development of a harvest control rule for Lake Erie walleye Sander vitreus,” in Management Science in Fisheries, eds C. T. T. Edwards, and D. J. Dankel, (Oxford: Routledge), 163–183.
Kaplan, I. C., Francis, T. B., Punt, A. E., Koehn, L. E., Curchitser, E., Hurtado-Ferro, F., et al. (2019). A multi-model approach to understanding the role of Pacific sardine in the California Current food web. Mar. Ecol. Prog. Ser. 61, 307–321.
Kasperski, S., and Holland, D. S. (2013). Income diversification and risk for fishermen. Proc. Natl. Acad. Sci. U.S.A. 110, 2076–2081. doi: 10.1073/pnas.1212278110
Keeley, E. R., and Grant, J. W. A. (2001). Prey size of salmonid fishes in streams, lakes, and oceans. Can. J. Fish. Aquat. Sci. 58, 1122–1132. doi: 10.1111/jfb.12321
Kilduff, D. P., Botsford, L. W., and Teo, S. L. H. (2014). Spatial and temporal covariability in early ocean survival of Chinook salmon (Oncorhynchus tshawytscha) along the west coast of North America. ICES J. Mar. Sci. 71, 1671–1682.
Kilduff, D. P., Di Lorenzo, E., Botsford, L. W., and Teo, S. L. H. (2015). Changing central Pacific El Niños reduce stability of North American salmon survival rates. Proc. Natl. Acad. Sci. U.S.A. 112, 10962–10966.
LaCroix, J. J., Wertheimer, A. C., Orsi, J. A., Sturdevant, M. V., Fergusson, E. A., and Bond, N. A. (2009). A top-down survival mechanism during early marine residency explains coho salmon year-class strength in southeast Alaska. Deep Sea Res. Part II 56, 2560–2569.
Levin, P. S., Fogarty, M. J., Murawski, S. A., and Fluharty, D. (2009). Integrated ecosystem assessments: developing the scientific basis for ecosystem-based management of the ocean. PLoS Biol. 7:e14. doi: 10.1371/journal.pbio.1000014
Lichatowich, J. (1999). Salmon without Rivers: A History of the Pacific Salmon Crisis. New York, NY: Island Press.
Lidström, S., and Johnson, A. F. (2020). Ecosystem-based fisheries management: a perspective on the critique and development of the concept. Fish Fish. 21, 216–222.
Lindley, S. T., Grimes, C. B., Mohr, M. S., Peterson, W., Stein, J., Anderson, J. T., et al. (2009). What Caused the Sacramento River Fall Chinook Stock Collapse? NOAA Tech. Memo. NOAA-TM-NMFS-SWFSC-447. (Silver Spring, MD: NOAA).
Link, J. S., Griffis, R., and Busch, S. (eds) (2015). NOAA Fisheries Climate Science Strategy. NOAA Tech. Memo. NMFS-F/SPO-155.(Silver Spring, MD: NOAA).
Litz, M. N. C., Miller, J. A., Copeman, L. A., Teel, D. J., Weitkamp, L. A., Daly, E. A., et al. (2017). Ontogenetic shifts in the diets of juvenile Chinook Salmon: new insight from stable isotopes and fatty acids. Environ. Biol. Fishes 100, 337–360.
Litzow, M. A., Ciannelli, L., Puerta, P., Wettstein, J. J., Rykaczewski, R. R., and Opiekun, M. (2018). Non-stationary climate-salmon relationships in the Gulf of Alaska. Proc. R. Soc. B Biol. Sci. 285:20181855. doi: 10.1098/rspb.2018.1855
Logerwell, E. A., Mantua, N., Lawson, P. W., Francis, R. C., and Agostini, V. N. (2003). Tracking environmental processes in the coastal zone for understanding and predicting Oregon coho (Oncorhynchus kisutch) marine survival. Fish. Oceanogr. 12, 554–568.
Losee, J. P., Miller, J. A., Peterson, W. T., Teel, D. J., and Jacobson, K. C. (2014). Influence of ocean ecosystem variation on trophic interactions and survival of juvenile coho and Chinook salmon. Can. J. Fish. Aquat. Sci. 71, 1747–1757.
Malick, M. J., Cox, S. P., Peterman, R. M., Wainwright, T. C., and Peterson, W. T. (2015). Accounting for multiple pathways in the connections among climate variability, ocean processes, and coho salmon recruitment in the Northern California Current. Can. J. Fish. Aquat. Sci. 72, 1552–1564.
Mangel, M., and Dowling, N. A. (2016). Reference points for optimal yield: a framework for assessing economic, conservation, and sociocultural tradeoffs in ecosystem-based fishery management. Coast. Manag. 44, 517–528.
Mantua, N. J., Hare, S. R., Zhang, Y., Wallace, J. M., and Francis, R. C. (1997). A Pacific interdecadal climate oscillation with impacts on salmon production. Bull. Am. Meteor. Soc. 78, 1069–1079.
Michel, C. J. (2018). Decoupling outmigration from marine survival indicates outsized influence of streamflow on cohort success for California’s Chinook salmon populations. Can. J. Fish. Aquat. Sci. 76, 1398–1410.
Michielsens, C. G. J., and Cave, J. D. (2018). In-season assessment and management of salmon stocks using a Bayesian time–density model. Can. J. Fish. Aquat. Sci. 76, 1073–1085.
Miller, J. A., Teel, D. J., Baptista, A., and Morgan, C. A. (2013). Disentangling bottom-up and top-down effects on survival during early ocean residence in a population of Chinook salmon (Oncorhynchus tshawytscha). Can. J. Fish. Aquat. Sci. 70, 617–629.
Mueter, F. J., Pyper, B. J., and Peterman, R. M. (2005). Relationships between coastal ocean conditions and survival rates of northeast Pacific salmon at multiple lags. Trans. Am. Fish. Soc. 134, 105–119.
Muir, W. D., Marsh, D. M., Sandford, B. P., Smith, S. G., and Williams, J. G. (2006). Post-hydropower system delayed mortality of transported Snake river stream-type Chinook salmon: Unraveling the mystery. Trans. Am. Fish. Soc. 135, 1523–1534.
Munsch, S. H., Greene, C. M., Johnson, R. C., Satterthwaite, W. H., Imaki, H., and Brandes, P. L. (2019). Warm, dry winters truncate timing and size distribution of seaward-migrating salmon across a large, regulated watershed. Ecol. Appl. 29:e01880. doi: 10.1002/eap.1880
Myers, K. W. (2018). “Ocean ecology of steelhead,” in The Ocean Ecology of Pacific Salmon and Trout, ed. R. Beamish, (Bethesda, MD: American Fisheries Society).
Myers, R. A. (1998). When Do Environment–recruitment Correlations Work? Rev. Fish. Biol. Fish. 8, 285–305.
Nehlsen, W., Williams, J. E., and Lichatowich, J. A. (1991). Pacific Salmon at the Crossroads - Stocks at Risk from California, Oregon, Idaho, and Washington. Fisheries 16, 4–21.
Neilson, J. L., Straley, J. M., Gabriele, C. M., and Hills, S. (2009). Non-lethal entanglement of humpback whales (Megaptera novaeangliae) in fishing gear in northern Southeast Alaska. J. Biogeogr. 36, 452–464.
NOAA Fisheries (2016a). Ecosystem-Based Fisheries Management Policy of the National Marine Fisheries Service, National Oceanic and Atmospheric Administration. NOAA Fisheries Policy Directive 01-120. (Silver Spring, MD: NOAA).
NOAA Fisheries (2016b). NOAA Fisheries Ecosystem Based Fisheries Management Road Map, National Oceanic and Atmospheric Administration. NOAA Fisheries Service Instruction 01-120-01. (Silver Spring, MD: NOAA).
NOAA NW/SW Fisheries Science Centers, (2016). Western Regional Action Plan (WRAP), NOAA Fisheries Climate Science Strategy. U.S. Department of Commerce, NOAA Technical Memorandum NMFS-SWFSC-565. (Silver Spring, MD: NOAA).
Ortiz, I., Aydin, K., Hermann, A. J., Gibson, G. A., Punt, A. E., Wiese, F. K., et al. (2016). Climate to fish: Synthesizing field work, data and models in a 39-year retrospective analysis of seasonal processes on the eastern Bering Sea shelf and slope. Deep Sea Res. Part II 134, 390–412.
Pacific Fisheries Management Council [PFMC], (2013). Pacific Coast Fishery Ecosystem Plan for the U.S. Portion of the California Current Large Marine Ecosystem. Portland, OR: Pacific Fisheries Management Council.
Pacific Fisheries Management Council [PFMC], (2018). Preseason Report 1: Stock Abundance Analysis and Environmental Assessment Part 1 for 2018 Ocean Salmon Fishery Regulations. Portland, OR: Pacific Fisheries Management Council.
Paulik, G. J. (1973). Studies of the possible form of the stock-recruitment curve. Rapp. P.V Reun. Cons. Perm. Int. Explor. Mer. 164, 302–315.
Pearcy, W. G. (1992). Ocean Ecology of North Pacific Salmonids. Seattle, WA: Washington Sea Grant Program.
Peterson, W. T., and Schwing, F. B. (2003). A new climate regime in northeast pacific ecosystems. Geophys. Res. Lett. 30, 4175–4180. doi: 10.1073/pnas.1113806109
Plagányi, E. E., Punt, A. E., Hillary, R., Morello, E. B., Thebaud, O., Hutton, T., et al. (2014). Multispecies fisheries management and conservation: tactical applications using models of intermediate complexity. Fish Fish. 15, 1–22.
Plagányi, É. E., Bell, J. D., Bustamante, R. H., Dambacher, J. M., Dennis, D. M., Dichmont, C. M., et al. (2011). Modelling climate-change effects on Australian and Pacific aquatic ecosystems: a review of analytical tools and management implications. Mar. Freshw. Res. 62, 1132–1147.
Pool, S. S., Brodeur, R. D., Goodman, N. L., and Daly, E. A. (2008). Abundance, distribution, and feeding patterns of juvenile coho salmon (Oncorhynchus kisutch) in the Juan de Fuca Eddy. Estuar. Coast. Shelf Sci. 80, 85–94.
Pool, S. S., Reese, D. C., and Brodeur, R. D. (2012). Defining marine habitat of juvenile Chinook salmon, Oncorhynchus tshawytscha, and coho salmon, O. kisutch, in the northern California Current System. Environ. Biol. Fishes 93, 233–243.
Quinn, T. P. (2005). The Behavior and Ecology of Pacific Salmon and Trout. Bethesda, MD: American Fisheries Society.
Richerson, K., and Holland, D. S. (2017). Quantifying and predicting responses to a US West Coast salmon fishery closure. ICES J. Mar. Sci. 74, 2364–2378.
Richerson, K., Leonard, J., and Holland, D. S. (2018). Predicting the economic impacts of the 2017 West Coast salmon troll ocean fishery closure. Mar. Policy 95, 142–152.
Roby, D. D., Collis, K., and Lyons, D. E. (2005). Conservation and Management for Fish-Eating Birds and Endangered Salmon. USDA Forest Service Gen. Tech. Rep. PSW-GTR-191. Washington, DC: USDA.
Roth, J. E., Mills, K. L., and Sydeman, W. J. (2007). Chinook salmon (Oncorhynchus tshawytscha) - seabird covariation off central California and possible forecasting applications. Can. J. Fish. Aquat. Sci. 64, 1080–1090.
Rupp, D. E., Wainwright, T. C., Lawson, P. W., and Peterson, W. T. (2012). Marine environment-based forecasting of coho salmon (Oncorhynchus kisutch) adult recruitment. Fish. Oceanogr. 21, 1–19.
Sabal, M., Hazen, E. L., Bograd, S. J., MacFarlane, R. B., Schroeder, I. D., Hayes, S. A., et al. (2020). The California Current seascape influences juvenile salmon foraging ecology at multiple scales. Mar. Ecol. Prog. Ser. 63, 159–173.
Samhouri, J. F., Stier, A. C., Hennessey, S. M., Novak, M., Halpern, B. S., and Levin, P. S. (2017). Rapid and direct recoveries of predators and prey through synchronized ecosystem management. Nat. Ecol. Evol. 1:0068. doi: 10.1038/s41559-016-0068
Sandell, T. A., Teel, D. J., Fisher, J., Beckman, B., and Jacobson, K. C. (2015). Infections by Renibacterium salmoninarum and Nanophyetus salmincola Chapin are associated with reduced growth of juvenile Chinook salmon, Oncorhynchus tshawytscha (Walbaum), in the Northeast Pacific Ocean. J. Fish Dis. 38, 365–378. doi: 10.1111/jfd.12243
Santora, J. A., Mantua, N. J., Schroeder, I. D., Field, J. C., Hazen, E. L., Bograd, S. J., et al. (2020). Habitat compression and ecosystem shifts as potential links between marine heatwave and record whale entanglements. Nat. Commun. 11:536. doi: 10.1038/s41467-019-14215-w
Sato, M., Barth, J. A., Benoit-Bird, K. J., Pierce, S. D., Cowles, T. J., Brodeur, R. D., et al. (2018). Coastal upwelling fronts as a boundary for planktivorous fish distributions. Mar. Ecol. Prog. Ser. 595, 171–186.
Satterthwaite, W., Carlson, S., Allen-Moran, S., Vincenzi, S., Bograd, S., and Wells, B. (2014). Match-mismatch dynamics and the relationship between ocean-entry timing and relative ocean recoveries of Central Valley fall run Chinook salmon. Mar. Ecol. Prog. Ser. 511, 237–248.
Satterthwaite, W. H., Andrews, K. S., Burke, B. J., Gosselin, J. L., Greene, C. M., Harvey, C. J., et al. (in press). Ecological thresholds in forecast performance for key United States West Coast Chinook salmon stocks. ICES J. Mar. Sci. fsz189. doi: 10.1093/icesjms/fsz189
Satterthwaite, W. H., and Carlson, S. M. (2015). Weakening portfolio effect strength in a hatchery-supplemented Chinook salmon population complex. Can. J. Fish. Aquat. Sci. 72, 1860–1875.
Satterthwaite, W. H., Mohr, M. S., O’Farrell, M. R., and Wells, B. K. (2013). A comparison of temporal patterns in the ocean spatial distribution of California’s Central Valley Chinook salmon runs. Can. J. Fish. Aquat. Sci. 70, 574–584.
Scheuerell, M. D., Hilborn, R., Ruckelshaus, M. H., Bartz, K. K., Lagueux, K. M., Haas, A. D., et al. (2006). The Shiraz model: a tool for incorporating anthropogenic effects and fish-habitat relationships in conservation planning. Can. J. Fish. Aquat. Sci. 63, 1596–1607.
Scheuerell, M. D., Zabel, R. W., and Sandford, B. P. (2009). Relating juvenile migration timing and survival to adulthood in two species of threatened Pacific salmon (Oncorhynchus spp.). J. Appl. Ecol. 46, 983–990.
Schindler, D. E., Hilborn, R., Chasco, B., Boatright, C. P., Quinn, T. P., Rogers, L. A., et al. (2010). Population diversity and the portfolio effect in an exploited species. Nature 465, 609–612. doi: 10.1038/nature09060
Sethi, S. A., Reimer, M., and Knapp, G. (2014). Alaskan fishing community revenues and the stabilizing role of fishing portfolios. Mar. Policy 48, 134–141.
Shelton, A. O., Satterthwaite, W. H., Ward, E. J., Feist, B. E., and Burke, B. (2019). Using hierarchical models to estimate stock-specific and seasonal variation in ocean distribution, survivorship, and aggregate abundance of fall run Chinook salmon. Can. J. Fish. Aquat. Sci. 76, 95–108.
Sigler, M., Hollowed, A., Holsman, K., Zador, S., Haynie, A., Himes-Cornell, A., et al. (2016). Alaska regional action plan for the Southeastern Bering Sea: NOAA Fisheries Climate Science Strategy. NOAA Tech. Memo. NMFS-AFSC-336. (Silver Spring, MD: NOAA)Google Scholar
Skern-Mauritzen, M., Ottersen, G., Handegard, N. O., Huse, G., Dingsør, G. E., Stenseth, N. C., et al. (2016). Ecosystem processes are rarely included in tactical fisheries management. Fish Fish. 17, 165–175.
Sterling, E. J., Zellner, M., Jenni, K. E., Leong, K., Glynn, P. D., Bendor, T. K., et al. (2019). Try, try again: lessons learned from success and failure in participatory modeling. Elementa 7:13.
Strange, J. S. (2013). Factors influencing the behavior and duration of residence of adult Chinook salmon in a stratified estuary. Environ. Biol. Fishes 96, 225–243.
Sutherland, W. J., Butchart, S. H., Connor, B., Culshaw, C., Dicks, L. V., Dinsdale, J., et al. (2018). 2018 horizon scan of emerging issues for global conservation and biological diversity. Trends Ecol. Evol. 33, 47–58. doi: 10.1016/j.tree.2017.11.006
Sydeman, W. J., Santora, J. A., Thompson, S. A., Marinovic, B., and Lorenzo, E. D. (2013). Increasing variance in North Pacific climate relates to unprecedented ecosystem variability off California. Glob. Change Biol. 19, 1662–1675. doi: 10.1111/gcb.12165
Taylor, J. E. III (1999). Making Salmon: An Environmental History of the Northwest Fisheries Crisis. Seattle, WA: University of Washington Press.
Teel, D. J., Burke, B. J., Kuligowski, D. R., Morgan, C. A., and Van Doornik, D. M. (2015). Genetic identification of Chinook salmon: stock-specific distributions of juveniles along the Washington and Oregon coasts. Mar. Coast. Fish. 7, 274–300.
Teo, S. L. H., Botsford, L. W., and Hastings, A. (2009). Spatio-temporal covariability in coho salmon (Oncorhynchus kisutch) survival, from California to southeast Alaska. Deep Sea Res. Part II 56, 2570–2578.
Thomsen, P. F., Kielgast, J., Iversen, L. L., Moller, P. R., Rasmussen, M., and Willerslev, E. (2012). Detection of a diverse marine fish fauna using environmental DNA from seawater samples. PLoS One 7:e41732. doi: 10.1371/journal.pone.0041732
Thomsen, P. F., and Willerslev, E. (2015). Environmental DNA - An emerging tool in conservation for monitoring past and present biodiversity. Biol. Conserv. 183, 4–18.
Tittensor, D. P., Eddy, T. D., Lotze, H. K., Galbraith, E. D., Cheung, W., Barange, M., et al. (2018). A protocol for the intercomparison of marine fishery and ecosystem models: Fish-MIP v1.0. Geosci. Model Dev. 11, 1421–1442.
Tomaro, L. M., Teel, D. J., Peterson, W. T., and Miller, J. A. (2012). When is bigger better? Early marine residence of middle and upper Columbia River spring Chinook salmon. Mar. Ecol. Prog. Ser. 452, 237–252.
Travis, J. M. J., Delgado, M., Bocedi, G., Baguette, M., Barton, K., Bonte, D., et al. (2013). Dispersal and species’ responses to climate change. Oikos 122, 1532–1540. doi: 10.1016/j.ympev.2016.12.021
Trifonova, N., Maxwell, D., Pinnegar, J., Kenny, A., and Tucker, A. (2017). Predicting ecosystem responses to changes in fisheries catch, temperature, and primary productivity with a dynamic Bayesian network model. ICES J. Mar. Sci. 74, 1334–1343.
Trudel, M., Fisher, J., Orsi, J. A., Morris, J. F. T., Thiess, M. E., Sweeting, R. M., et al. (2009). Distribution and Migration of Juvenile Chinook Salmon Derived from Coded Wire Tag Recoveries along the Continental Shelf of Western North America. Trans. Am. Fish. Soc. 138, 1369–1391.
Tucker, S., Hipfner, J. M., and Trudel, M. (2016). Size- and condition-dependent predation: a seabird disproportionately targets substandard individual juvenile salmon. Ecology 97, 461–471. doi: 10.1890/15-0564.1
Walters, C. J. (1986). Adaptive Management of Renewable Resources. Basingstoke: Macmillan Publishers Ltd.
Wargo Rub, A. M. W., Som, N. A., Henderson, M. J., Sandford, B. P., Van Doornik, D. M., Teel, D. J., et al. (2019). Changes in adult Chinook salmon (Oncorhynchus tshawytscha) survival within the lower Columbia River amid increasing pinniped abundance. Can. J. Fish. Aquat. Sci. 76, 1862–1873.
Weise, M. J., and Harvey, J. T. (2008). Temporal variability in ocean climate and California sea lion diet and biomass consumption: implications for fisheries management. Mar. Ecol. Prog. Ser. 373, 157–172.
Weitkamp, L. A. (2010). Marine Distributions of Chinook Salmon from the West Coast of North America Determined by Coded Wire Tag Recoveries. Trans. Am. Fish. Soc. 139, 147–170.
Welch, D. W., Melnychuk, M. C., Payne, J. C., Rechisky, E. L., Porter, A. D., Jackson, G. D., et al. (2011). In situ measurement of coastal ocean movements and survival of juvenile Pacific salmon. Proc. Natl. Acad. Sci. U.S.A. 108, 8708–8713. doi: 10.1073/pnas.1014044108
Wells, B. K., Field, J. C., Thayer, J. A., Grimes, C. B., Bograd, S. J., Sydeman, W. J., et al. (2008). Untangling the relationships among climate, prey and top predators in an ocean ecosystem. Mar. Ecol. Prog. Ser. 364, 15–29.
Wells, B. K., Grimes, C. B., and Waldvogel, J. B. (2007). Quantifying the effects of wind, upwelling, curl, sea surface temperature and sea level height on growth and maturation of a California Chinook salmon (Oncorhynchus tshawytscha) population. Fish. Oceanogr. 16, 363–382.
Wells, B. K., Santora, J. A., Field, J. C., Macfarlane, R. B., Marinovic, B. B., and Sydeman, W. J. (2012). Population dynamics of Chinook salmon Oncorhynchus tshawytscha relative to prey availability in the central California coastal region. Mar. Ecol. Prog. Ser. 457, 125–137.
Wells, B. K., Santora, J. A., Henderson, M. J., Warzybok, P., Jahncke, J., Bradley, R. W., et al. (2017). Environmental conditions and prey-switching by a seabird predator impact juvenile salmon survival. J. Mar. Syst. 174, 54–63.
Wells, B. K., Santora, J. A., Schroeder, I. D., Mantua, N., Sydeman, W. J., Huff, D. D., et al. (2016). Marine ecosystem perspectives on Chinook salmon recruitment: a synthesis of empirical and modeling studies from a California upwelling system. Mar. Ecol. Prog. Ser. 552, 271–284.
Willmes, M., Hobbs, J. A., Sturrock, A. M., Bess, Z., Lewis, L. S., Glessner, J. J. G., et al. (2018). Fishery collapse, recovery, and the cryptic decline of wild salmon on a major California river. Can. J. Fish. Aquat. Sci. 75, 1836–1848.
Winship, A. J., O’Farrell, M. R., Satterthwaite, W. H., Wells, B. K., and Mohr, M. S. (2015). Expected future performance of salmon abundance forecast models with varying complexity. Can. J. Fish. Aquat. Sci. 72, 557–569.
Woodson, L. E., Wells, B. K., Weber, P. K., Macfarlane, R. B., Whitman, G. E., and Johnson, R. C. (2013). Size, growth, and origin-dependent mortality of juvenile Chinook salmon Oncorhynchus tshawytscha during early ocean residence. Mar. Ecol. Prog. Ser. 487, 163–175.
Woodworth-Jefcoats, P. A., Polovina, J. J., Howell, E. A., and Blanchard, J. L. (2015). Two takes on the ecosystem impacts of climate change and fishing: Comparing a size-based and a species-based ecosystem model in the central North Pacific. Prog. Oceanogr. 138, 533–545.
Zabel, R. W., and Achord, S. (2004). Relating size of individuals to juvenile survival within and among closely-related populations of chinook salmon. Ecology 85, 795–806.
Zabel, R. W., Scheuerell, M. D., McClure, M. M., and Williams, J. G. (2006). The interplay between climate variability and density dependence in the population viability of Chinook salmon. Conserv. Biol. 20, 190–200. doi: 10.1111/j.1523-1739.2005.00300.x
Zabel, R. W., and Williams, J. G. (2002). Selective mortality in chinook salmon: What is the role of human disturbance? Ecol. Appl. 12, 173–183.
Keywords: California Current Ecosystem, Chinook, steelhead, coho, ecosystem models, life-cycle models, management strategies
Citation: Wells BK, Huff DD, Burke BJ, Brodeur RD, Santora JA, Field JC, Richerson K, Mantua NJ, Fresh KL, McClure MM, Satterthwaite WH, Darby F, Kim SJ, Zabel RW and Lindley ST (2020) Implementing Ecosystem-Based Management Principles in the Design of a Salmon Ocean Ecology Program. Front. Mar. Sci. 7:342. doi: 10.3389/fmars.2020.00342
Received: 16 October 2019; Accepted: 23 April 2020;
Published: 19 May 2020.
Edited by:
Michael Arthur St. John, Technical University of Denmark, DenmarkReviewed by:
Steve Macdonald, Department of Fisheries and Oceans, CanadaCopyright © 2020 Wells, Huff, Burke, Brodeur, Santora, Field, Richerson, Mantua, Fresh, McClure, Satterthwaite, Darby, Kim, Zabel and Lindley. This is an open-access article distributed under the terms of the Creative Commons Attribution License (CC BY). The use, distribution or reproduction in other forums is permitted, provided the original author(s) and the copyright owner(s) are credited and that the original publication in this journal is cited, in accordance with accepted academic practice. No use, distribution or reproduction is permitted which does not comply with these terms.
*Correspondence: Brian K. Wells, YnJpYW4ud2VsbHNAbm9hYS5nb3Y=
Disclaimer: All claims expressed in this article are solely those of the authors and do not necessarily represent those of their affiliated organizations, or those of the publisher, the editors and the reviewers. Any product that may be evaluated in this article or claim that may be made by its manufacturer is not guaranteed or endorsed by the publisher.
Research integrity at Frontiers
Learn more about the work of our research integrity team to safeguard the quality of each article we publish.