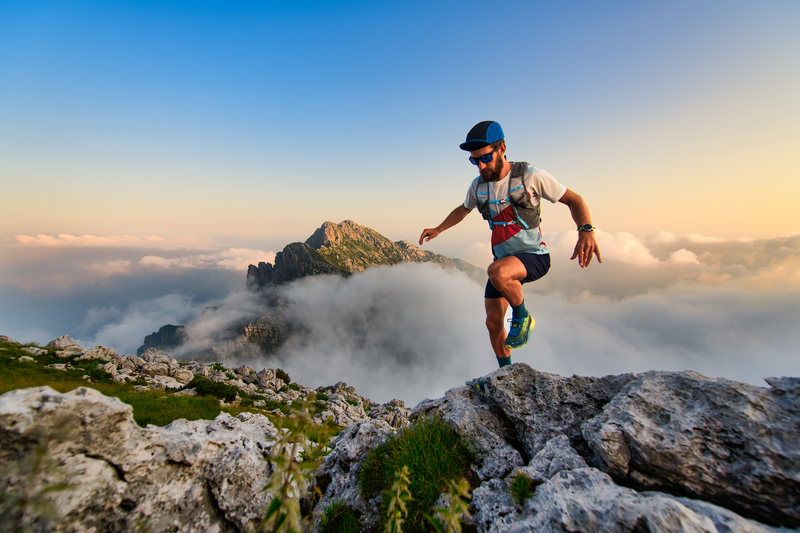
95% of researchers rate our articles as excellent or good
Learn more about the work of our research integrity team to safeguard the quality of each article we publish.
Find out more
ORIGINAL RESEARCH article
Front. Mar. Sci. , 26 March 2020
Sec. Global Change and the Future Ocean
Volume 7 - 2020 | https://doi.org/10.3389/fmars.2020.00183
This article is part of the Research Topic Biogeochemical Consequences of Climate-Driven Changes in the Arctic View all 11 articles
The transmission of ultraviolet (UVR) and photosynthetically available radiation (PAR) through sea ice is a key factor controlling under-ice phytoplankton growth in seasonally ice-covered waters. The increase toward sufficient light levels for positive net photosynthesis occurs concurrently with the sea ice melt progression in late spring when ice surface conditions shift from a relatively homogeneous high-albedo snow cover to a less reflective mosaic of bare ice and melt ponds. Here, we present a detailed dataset on the spatial and temporal progression of transmitted UVR and PAR in relation to changing quantities of snow, sea ice and melt ponds. Data were collected with a remotely operated vehicle (ROV) during the GreenEdge landfast sea ice campaign in June–July 2016 in southwestern Baffin Bay. Over the course of melt progression, there was a 10-fold increase in spatially averaged UVR and PAR transmission through the sea ice cover, reaching a maximum transmission of 31% for PAR, 7% for UVB, and 26% for UVA radiation. The depth under the sea ice experiencing spatial variability in light levels due to the influence of surface heterogeneity in snow, white ice and melt pond distributions increased from 7 ± 4 to 20 ± 6 m over our study. Phytoplankton drifting in under-ice surface waters were thus exposed to variations in PAR availability of up to 43%, highlighting the importance to account for spatial heterogeneity in light transmission through melting sea ice. Consequently, we demonstrate that spatial averages of PAR transmission provided more representative light availability estimates to explain under-ice bloom progression relative to single point irradiance measurements during the sea ice melt season. Encouragingly, the strong dichotomy between white ice and melt pond PAR transmittance and surface albedo permitted a very good estimate of spatially averaged light transmission from drone imagery of the surface and point transmittance measurements beneath different ice surface types.
In the Arctic Ocean, under-ice phytoplankton blooms can contribute significantly to spring primary production and have been documented more frequently in the last decades (e.g., Fortier et al., 2002; Mundy et al., 2009; Arrigo et al., 2014; Assmy et al., 2017; Oziel et al., 2019). During spring, surface nutrient concentrations tend to be replete and the presence of sea ice and meltwater create a low turbulence that favor the growth of diatoms (Arrigo et al., 2014; Neeley et al., 2018; Oziel et al., 2019) and the colonial haptophyte Phaeocystis pouchetti (Assmy et al., 2017). The onset of under-ice phytoplankton production is largely triggered by the seasonal increase in transmission of photosynthetically active radiation (PAR, 400–700 nm) through the ice layer to sufficient levels for positive net photosynthesis (Mundy et al., 2014). A study by Horvat et al. (2017) demonstrated that nearly 30% of the ice-covered Arctic Ocean in July permits PAR levels that are sufficient for under-ice algal blooms. However, in situ optical measurements beneath the sea ice cover are difficult due to spatial heterogeneity in light propagation caused by differences in snow depth, melt pond coverage, melt pond geometry and depth, ice thickness, and the horizontal distribution of light absorbing ice impurities (Ehn et al., 2008, 2011; Katlein et al., 2015; Light et al., 2015; Lu et al., 2016; Horvat et al., 2020).
Sea ice albedo has been widely studied showing that the decrease in light reflection is not a steady process (Fetterer and Untersteiner, 1998; Ehn et al., 2011; Perovich and Polashenski, 2012; Landy et al., 2014; Diaz et al., 2018). Snow melt and an impermeable ice layer cause surface flooding and thus a rapid decrease in the surface albedo. As melt progresses, the trapped water begins to drain toward flaws and seal breathing holes resulting in a short-term increase in regional albedo due to the emerging white ice (drained bare surface ice layer). In the last stage of melt, surface albedo further decreases with the thinning white ice layer and ice cover until ice break-up. During this surface melt progression, the initiation of melt pond formation is associated with the strongest increase in light levels at the ice bottom (Nicolaus et al., 2012; Zhang et al., 2015). However, the intensified differences in light transmittance through ponded vs. white ice combined with the lateral spreading of radiation within the ice layer create a more complex underwater light field (Frey et al., 2011; Katlein et al., 2016; Massicotte et al., 2018; Matthes et al., 2019). As shown in these studies studying the under-ice light field, vertical radiation transfer can be influenced by higher light transmittance through more transparent near-by structures causing edge effects at the ice bottom and subsurface irradiance maxima. This light attenuation discrepancy affects point measurements of light transmittance under the ice with different surface types and makes regional estimates of under-ice PAR availability for marine primary production estimates difficult. Optical measurements beneath a depth of 5 to 15 m are less affected by spatially heterogenous light transmission due to a more downward directed light propagation, which is only dependent on absorption and scattering processes within the water column (Frey et al., 2011; Katlein et al., 2015; Matthes et al., 2019). Thought from a surface perspective, Perovich (2005) defined this spatial scale of minimal variation in the propagation of solar radiation as the aggregate scale. Following this definition, the depth of spatially transmitted light independence on surface conditions is hereinafter called the “aggregate-scale depth” of light transmission. Knowledge about the impact of the surface melt progression on this depth is still limited.
To capture regional variability of light transmission through sea ice and the underlying water column, remotely operated vehicles (ROVs) equipped with different sensor arrays are more frequently used. ROVs were deployed to perform large-scale irradiance measurements beneath landfast sea ice and moving pack ice in the Arctic Ocean (Nicolaus and Katlein, 2013; Katlein et al., 2015, 2019), West Greenlandic fjord (Lund-Hansen et al., 2018), and in the Weddell Sea (Arndt et al., 2017). The minimized disturbance caused by ROV-based measurements compared to traditional core-based point-sampling methods also enables repeated operations within the same area throughout the melt season (Nicolaus et al., 2012). These measurements can be used to calculate regional estimates of under-ice PAR levels, which are needed in the investigation of the timing of under-ice phytoplankton growth. Large-scale sea-ice coverage sampling also minimizes statistical errors in primary production estimates caused by spatially heterogeneous light propagation (Massicotte et al., 2019). Hence, spatially averaged light transmission could represent a better estimate of light availability as phytoplankton cells often drift at a different rate and direction than that of the sea ice. This is particularly true for the case of a static landfast ice cover overlying a tide-influenced water column. Additionally, area-wide averages of light transmittance were found to cancel out edge effects caused by differences in ice surface reflection of melt ponds and white ice (Ehn et al., 2011; Taskjelle et al., 2017).
Meltwater transport and melt pond evolution at the ice surface has been described to undergo several stages throughout the sea ice spring-summer progression (Eicken et al., 2002; Polashenski et al., 2012; Landy et al., 2014). However, similar studies about the temporal increase in light transmission over the melt season are still sparse. In this paper, we hypothesize that the temporal increase in under-ice PAR and UVR levels follows the stages of melt pond evolution while the spatial heterogeneity of PAR and UVR transmission remains unchanged after the melt pond onset. We further hypothesize that including spatial heterogeneity of light transmission in the calculation of the euphotic zone depth will provide a more accurate estimate to help explain processes influencing development of an under-ice phytoplankton bloom. To quantify the increase of spectral light transmission through sea ice as a function of melt processes, a remotely operated vehicle (ROV) equipped with hyperspectral radiometers was used in June–July 2016 in Southwestern Baffin Bay. Horizontal transects and vertical profiles were repeatedly performed beneath the ice cover with changing quantities of snow, ice, melt ponds and ice algae to calculate average PAR and UVR transmittance and to investigate the interaction of increasing under-ice PAR availability and the initiation of phytoplankton growth. Simultaneously, the impact of the varying ice surface conditions on the scale of spatial variability of surface albedo and light transmission and the aggregate-scale depth are examined.
As part of the GreenEdge project in 2016, measurements of spectral irradiance and environmental parameters were performed on level landfast first-year sea ice (67° 28.784′ N, 63° 47.372′ W) near Qikiqtarjuaq, Nunavut, Baffin Bay (Figure 1). An undisturbed area east of the ice camp was chosen to repeatedly measure light transmittance through sea ice transitioning from snow-covered to shallow melt ponds and white ice surface conditions between 6 June and 2 July. Snow melt onset had already begun prior to the commencement of our study with daytime air temperatures consistently exceeding 0°C on 3 June followed by melt pond formation on 15 June (Oziel et al., 2019). Sky conditions varied between cloudy with sunny intervals, fully overcast and long periods of fog causing a decrease in incident surface PAR in June compared to the previous month [Figure 2 in Oziel et al. (2019)].
Figure 1. Location of the ice camp as part of the GreenEdge campaign in 2016 on landfast sea ice near Qikiqtarjuaq, Southern Baffin Island, NU, Canada (MODIS image, 13 June 2016).
Incoming solar irradiance at the sea ice surface, Ed(λ,0), was measured with a visible (VIS) hyperspectral radiometer (wavelength range 350–950 nm with 3.3 nm resolution over 256 channels) and a 4-channel multispectral ultraviolet (UV) radiometer (305, 325, 340, 379 nm; Satlantic HyperOCR and OCR-504 UV, respectively, Sea-Bird Scientific, United States), both with cosine collector and mounted on a tripod 1.5 m above the ice surface. Surface albedo measurements were made with a separate hyperspectral radiometer (wavelength range 320–950 nm with 3.3 nm resolution over 190 channels; Ramses-ACC, TriOS GmbH, Germany) after the under-ice light sampling. Spectral albedo, α(λ), was calculated as the ratio of five measurements of downwelling, Ed(λ,0), and upwelling, Eu(λ,0), surface irradiance measured 1 m above the ice surface:
Downwelling under-ice irradiance at 2 m water depth, Ed(λ,2), was measured using a remotely operated vehicle (ROV; SeaBotix vLBV300, United States), connected to a surface control unit through a tether cable of 300 m length (Supplementary Figure S1). The ROV was equipped with matching VIS and multispectral UV surface radiometers but calibrated for underwater deployment. All sensors were triggered synchronously, and light data was binned to one measurement per second. Also attached to the ROV was a CTD probe (SBE 49 FastCAT, Seabird, United States), an altimeter (resolution: 1 mm, VA500, Valeport, United States) to measure the distance between the vehicle and the ice bottom and a 360 degree action camera (PIXPRO SP360, Kodak, United States) to record ice bottom features. The ROV was launched and recovered through ∼1 m2 holes and moved along horizontal transects by six thrusters at an average speed of 0.5 m s–1. The average sinking speed for vertical profiles was 0.2 m s–1. An integrated camera system at the front and back of the ROV enabled under-ice navigation along guiding lines. Prior to the field deployment, the weight distribution of ROV attachments were balanced, such that the internally measured pitch and roll of the vehicle never exceeded 7° during under-ice deployments.
To increase the spatial and temporal coverage of sampling, two transects areas were designated as shown in Figure 2. The change in spectral light transmission overtime was measured continuously along one 150-m long horizontal transect, called non-destructive (ND) transect, at a water depth of 2 m. No destructive sampling occurred along the ND transect to guarantee an undisturbed ice surface melt progression throughout the sampling period. However, the first 20 m of the transect distance was not used in the data analysis to avoid artificial disturbances of the ice cover caused by the access hole and the ROV set-up procedures. To calculate spectral light transmittance, T(λ), surface and under-ice irradiance in the UV and visible spectrum were measured simultaneously during the ROV deployment. Also, along the same transect vertical profiles to a water depth of 50 m were performed at 50, 100, and 150 m distance from the access hole. To navigate the ROV consistently along the transect beneath the fully consolidated ice cover, a clear nylon fishing line was stretched taut beneath the ice through holes at the start and end of the transect and secured using ice screws. Additionally, every 10 m of the line was marked to provide a reference distance for the recorded light data. In total, the ND transect was measured eight times over four weeks. Ice draft, hI, was measured during each deployment via the ROV altimeter (mounted level with the radiometers) as the difference between the ROV depth (via the ROV CTD that was level with the radiometers) and its distance to the sea ice bottom (Supplementary Figure S1). Drilling through the sea ice cover to measure ice thickness was not performed to avoid artificial draining of the sampling area.
Figure 2. Schematic sampling set-up of under-ice ROV measurements and ice surface measurements in the two (non-destructive, destructive) transect areas.
At a near-by site, identical under-ice horizontal transects, and vertical profiles were performed to study the spatial variability in light transmission caused by the differences in sea ice surface properties. Along these destructive (D) transects, every ROV deployment was followed by surface measurements. Surface properties such as snow depth, hS, melt pond depth, hMP, and the height of drained white ice above melt pond surface, hBI, were measured with a ruler every 5 m of the D transect and after the eighth and final ROV deployment along the ND transect. Surface albedo measurements were performed every 10 m when the ice was still snow-covered. After melt ponds had formed, albedo was measured at nine locations along the transect above varying ice surface structures. In total, seven D transects were performed throughout the sampling period with varying snow depth and melt pond coverage.
Changes in surface properties and melt pond coverage within both transect areas were also documented through aerial drone (Phantom 2 Vision +, United States) surveys 90 m above the ice surface. Drone images were used to retrieve information on variability of surface brightness as proxy for surface albedo along the vehicle track. Following the procedure described in Katlein et al. (2015), pixel brightness was derived from the three RGB channels of the attached camera. The intensities of the R, G, and B channels were divided by the maximum value of 255 to gain pixel brightness from a single image of each transect. Brightness values between 0 and 1 were used in the semi-variogram analysis of the spatial variability of surface albedo.
To estimate length-weighted average albedo, , for the transect area, four replicates were measured for each surface type. Following Perovich (2005), was calculated for each transect with known melt pond coverage as
where α is the albedo and A is the area fraction for snow (S), white ice (WI) and melt ponds (MP). Under-ice irradiance data was pre-processed with the radiometers’ software ProSoft (Satlantic, United States) to perform dark corrections and immersion correction for all under-ice light measurements due to the larger refractive index of water compared to air. Recorded spectra between 320 and 700 nm were also interpolated to 1-nm steps and converted into quantum irradiance (μmol photons m–2 s–1), which is more relevant for biological studies. Irradiance in the UVB spectrum was not interpolated due to the measurement of a single wavelength (305 nm). Based on the ROV speed, the horizontal resolution of Ed(λ, 2) was between 1 and 2 m, while vertical profiles of Ed(λ,z) were calculated at 0.2-m steps from 1.6 to 50 m using linear interpolation. Spectral and PAR transmittance,T(λ)and T(PAR), respectively, as well as the diffuse vertical attenuation coefficient for downwelling irradiance, Kd(PAR), were calculated as described in Matthes et al. (2019). The vertical attenuation coefficient was calculated as the average of three vertical profiles along each horizontal transect. However, only irradiance spectra from 15 to 50 m were included to avoid the impact of variable light transmission through different surface types of the sea ice cover (Matthes et al., 2019). In order to calculate T(PAR) at the ice/water interface, Kd(PAR) was also used to extrapolate the transmittance data from 2 m water depth to the ice bottom following Ehn et al. (2011). Measured transmittance in the UV spectrum has not been corrected for light attenuation in the water due to irradiance values near the detection limit resulting in uncertainties in the calculation of Kd(UVR). Mean transmittance, , was calculated for each horizontal transect as the average of 164 to 1161 coincident measurements of surface and transmitted irradiance spectra. Additionally, to compare methods of calculating mean PAR transmittance of an area, length-weighted average transmittance, , was calculated for each D transect following Taskjelle et al. (2017)
where LP and LWI are the length of the transect covered by melt ponds or white ice, respectively, and TP and TWI are the corresponding PAR transmittances at four melt ponds and four white ice sites along the transect.
The impact of varying ice surface conditions on the aggregate scale depth was investigated by plotting change in standard deviation, SD, of the three PAR measurements at each depth of the vertical profiles of each sampling day. A difference in SD below the threshold of ± 1 μmol photons m–2 s–1 was chosen to identify the depth at which the under-ice light field is no longer influenced by spatial differences in light transmission through the ice cover. Repeated irradiance measurements along the ND transect and drone pictures of the same area also enabled a spatial analysis of the change in the scale of variability in surface brightness and PAR transmittance over time. Semi-variogram statistics of these two parameters provided information about the spatial distance (lag) between the first and the next measurement that is no longer correlated with the first measurement. To investigate the length scales of spatial variability the data set, a least square fit of exponential (surface brightness) or gaussian (transmittance) theoretical variograms were used to obtain range values. The range describes the lag distance at which the model reaches 95% of the estimated semi-variance (sill) and thus measurements are spatially independent. Significant changes in the range of surface brightness, a proxy for surface albedo, and PAR transmittance were investigated using linear regression.
In June 2016, under-ice irradiance measurements commenced just after snow melt onset (Figure 3A). Melt water became visible at the ice surface on 15 June (Figure 3B), and field observations showed that the snow cover turned into large melt grain clusters. A rain event on 22 June contributed to a rapidly flooded ice surface (Figure 3C). After the rain event, air temperatures decreased leading to snowfall, freezing surface water during the night and a decline in the rate of surface melt. By 27 June, large, but shallow melt ponds had formed that were separated by an elevated and drained white ice surface cover as shown for 30 June in Figure 3D. Increased ice surface drainage led to shrinking melt pond sizes and more prominent white ice coverage in the following days (Figure 3E).
Figure 3. Areal images of sea ice surface conditions along the sampling transects on (A) 8 June, (B) 20 June, (C) 22 June, (D) 30 June, and (E) 2 July, 2016. The ROV under-ice ND transect is indicated by the purple dashed line.
Snow depth ± standard deviation decreased from 30.1 ± 6.9 cm to 6.5 ± 3.8 cm within the first sampling week leading up to the melt pond onset on 15 June (Figure 4A). The initial snow cover was characterized by a high water content forming a 7.3 ± 6.1 cm thick layer of slush at its basis on 9 June. Aerial drone surveys of the D transect showed that melt pond coverage increased from 1.7% on 16 June to a maximum of 52.5% on 22 June (Figure 4A). In the following days, melt pond coverage decreased gradually from 26.5% on 30 June to 16.8% on 2 July.
Figure 4. Time series of spatially averaged (A) snow depth (purple circles, standard deviation error bars), ice draft (black squares), melt pond coverage (red diamonds), and averaged (B) surface water salinity (blue squares), surface water temperature (orange squares), and freezing temperature of seawater for each transect at 2.4-m depth over the sampling period. Images of the ice bottom were taken at 2 m on (C) 6 June, and (D) 30 June 2016. Melt pond coverage for the transect area is highlighted for D transects (empty diamonds) and ND transect (filled diamonds) after the melt pond onset (dotted line). The shaded area highlights the difference between water and freezing temperature.
Ice draft decreased over the sampling period from average values of 1.47 ± 0.08 m to 1.24 ± 0.13 m along the ND transect (Figure 4A). An average freeboard of 0.09 ± 0.03 m was measured at a near-by area and was within the stated standard deviation of the ice draft along the ROV transects. Temperature and salinity, measured at 2.4 m water depth and averaged for each horizontal ROV transect, shows the seasonal increase in heat content (departure from freezing temperature) and melt water content (Figure 4B). Up to 13 June, measured surface water salinity and temperature remained nearly constant at 32.2 and −1.7°C, respectively. After melt pond onset salinity decreased to 31.7, while the difference between the freezing temperature of seawater at −1.7°C and the surface water temperature at −1.3°C on 2 July increased suggesting a larger energy input associated with solar radiative heating. This warming of the upper water column coincides with an alteration in sea ice bottom topography. Under-ice images taken on 6 June showed a smooth ice bottom with a brown coloration indicating the presence of ice algae and dark aggregates caught in small drainage holes (Figure 4C and Supplementary Video S1). By the beginning of July, the ice bottom appeared smoother, with larger holes and domes, and without a visible bottom coloration (Figure 4D).
Due to clear sky conditions, incoming radiation was highest in the first half of June (Table 1). The second half of June was characterized by several fog events and an increasing cloud cover, which created a diffuse surface light field and reduced surface Ed(UVA,PAR,0) until the cloud cover decreased again in the beginning of July. Daily incident radiation in the UVB spectrum, Ed(305nm,0), reached values between 1–3 mmol photons m–2 d–1 throughout the sampling period. Transmitted UVR and PAR increased with surface melt and varied along each transect (Table 1). This spatial variability further increased with the formation of melt ponds at the ice surface. Only at this late melt stage was a very low Ed(305nm,2) of 0.003 μmol photons m–2 s–1 actually measured at 2 m water depth. In the water column, diffuse vertical attenuation of PAR increased in late June, which was accompanied by an observed decrease in visibility of the guiding lines.
Table 1. Mean and standard deviation (SD) of incoming UVR and PAR at the sea ice surface, Ed(0), and at 2 m water depth, Ed(2), the daily incident UVR and PAR, and the mean vertical diffuse attenuation coefficient, Kd(PAR), for four days in 2016.
Measured surface PAR albedo as well as transmittance of PAR and four wavelengths in the UV spectrum are shown over the sampling period in Figure 5. Concurrently with the development of melt ponds, calculated length-weighted declined from 0.91 for snow-covered ice, to 0.58 after melt pond onset on 15 June (Figure 5A). As surface melt progressed, albedo variability increased until distinct melt ponds had formed. From 22 June onward, stayed relatively consistent between 0.47 and 0.53. Drone images also revealed that the prevailing landfast ice cover compromised a mosaic of smaller and larger ice floes that were frozen together (Supplementary Figure S2). This structural component had an impact on the color of melt ponds by creating brighter and darker ponds and thus causing a large range of melt pond albedo from 0.21 to 0.44.
Figure 5. Time series of (A) length-weighted mean PAR surface albedo, (B) PAR transmittance at the sea ice bottom, and (C) UVR transmittance for four wavelengths (305, 325, 340, 379 nm) at 2-m depth over the sampling period. Boxplots of PAR transmittance show median (black bar), mean (black cross), length-weighted mean (red cross) and the 25% and 75% quartiles of measurements along each D transect (gray) and the ND transect (white). Whisker length correspond to ±2.7σ, outliers are shown as blue dots. The beginning of melt stages (I – III) is highlighted as dotted lines.
Measured T(PAR) and T(UVR) at the ice bottom are presented as boxplots to display the variability of transmittance along the horizontal transect for each day (Figures 5B,C). With the shift in surface conditions from a highly reflective snow cover to a less reflective mosaic of bare ice and melt ponds, light transmission through the ice cover increased by a factor of 30. However, a continuous increase was only observed in the second and third week of June before mean PAR transmittance, , leveled off to an average of 0.23 ± 0.05 (Figure 5B). The observed seasonal progression of light transmittance was split into three stages defined by the state of ice surface melt and the corresponding change in the magnitude and spatial variability of light transmittance:
Only 0.02 ± 0.01 of incoming PAR was transmitted through the snow-covered ice and spatial variability of light transmission did not change noticeably.
Once melt water became visible in large stretches at the ice surface, increased by an order of magnitude to 0.31 on 22 June, while under-ice irradiance became increasingly variable.
A short snowfall event followed by an enhanced surface melt resulted in discrete areas of white ice and melt pond, defining stage III. PAR transmittance and its spatial variability did not increase further during this stage. In fact, measured along the ND transect decreased from 0.23 to 0.16.
The observed large drop in measured along the ND transect on 28 June was attributed to the snowfall event. Unfortunately, surface albedo was not measured that day. Repeated measurements along this transects also showed more pronounced transmittance peaks beneath melt ponds while PAR transmittance below white ice became less variable over time (Supplementary Figure S3). These high transmittance values of discrete surface ponds became pronounced as outliers in the boxplots after the surface flooding in stage III. The larger areas of white ice transmitting less PAR compared to ponded ice also resulted in a skewed distribution and the median to be less than the calculated mean for most of the days within stage III. On the last sampling day, the variability in measured under-ice PAR levels decreased while remained unchanged at 0.20. As shown in the aerial drone image of the sampling area on 2 July (Figure 4E), more white ice had emerged at the surface due to ongoing drainage of melt ponds, leading to a drop in the melt pond coverage and a more uniform sea ice surface. It should be noted that the proposed stages of changes in are different from the stages of melt pond evolution described elsewhere (Eicken et al., 2002).
For the comparison of measured mean PAR transmittance and length-weighted average transmittance, was calculated for all D transects. To do so, T(PAR) values of 0.16 to 0.24 beneath white ice and 0.25 to 0.40 beneath ponded ice, measured along four destructive transects, were used. As shown in Figure 5B, and were not significantly different (t(12) = 0.005, p = 0.996) over the sampling period.
The increase in the transmission of one wavelength (305 nm) in the UVB spectrum and three wavelengths (325, 340, and 379 nm) in the UVA spectrum at 2 m is shown for all transects over the sampling period (Figure 5C). Beneath snow-covered sea ice in stage I, , ranged from 0.01 to 0.02, while UVB radiation was not detectable. It is noted that surface and transmitted irradiance were integrated over the UVA wavelength spectrum (320–400 nm) prior to estimating . With melt pond onset, increased to 0.26 by the end of stage II on 22 June. Also, UVB radiation was detectable beneath the ice cover with a of 0.01. In stage III, transmission of UVA radiation did not increase further, displaying a mean of 0.21 ± 0.05 for D and ND transects. However, was on average greater during stage III than stage II, reaching a mean value of 0.07 ± 0.06. During stage III, UVR transmittance remained relatively consistent, while the variability in measured under-ice UVR levels decreased. Furthermore, UVR transmission through melt ponds was twice as high than through white ice. TWI(305nm) and TMP(305nm) ranged from 0.03 to 0.08 and 0.11 to 0.14, respectively. Differences in the transmission of UVA radiation through the two surface types were less pronounced with a TWI(UVA) and TMP(UVA) between 0.16 and 0.24 and 0.22 and 0.35, respectively.
To compare measured transmittance of UVR and PAR at 2 m water depth, boxplots and the spectral shape in the 400 to 700 nm range of three sampling days (11 June, 23 June, 2 July 2016), representing different ice surface types, are shown in Figure 6. For all presented days, was smaller than due to a pronounced decrease in transmittance in the 600 to 700 nm wavelength spectrum. Differences between calculated transmittance were smallest beneath snow-covered sea ice with and of 0.2 on 11 June. During stage I, the edges ofT(λ) were much steeper with a transmittance peak at 512 nm. With melt pond formation, exceeded increasingly, while the transmittance peak of the T(λ) spectrum shifted toward the blue spectrum at 481 nm during stages II and III.
Figure 6. Measured transmittance of UVR (305, 325, 340, 379 nm) and PAR along ND transect at 2-m depth on (A) 11 June, (B) 23 June, and (C) 2 July 2016. Boxplots of UVR and PAR transmittance show median (black bar), mean (cross) and the 25% and 75% quartiles of measurements along each transect. Whisker length correspond to ± 2.7σ, outliers are shown as blue dots. Spectral irradiance in the PAR spectrum is shown for all single measurements (shaded area) along the same ND transect, and as median (black line), mean (red line), 25th/75th percentiles (dashed line) and 5th/95th percentile (dotted line).
The change in spatial variability of ice surface brightness, as a proxy for variability of (PAR), andT(PAR) at the ice bottom was investigated using semi-variances calculated for lag distances over the collected dataset (Supplementary Figure S4). Variograms of surface brightness were computed for eight horizontal transects of which aerial drone images were taken, while variograms of T(PAR) were calculated for twelve horizontal transects (Supplementary Table S1). The change in variogram range of variability in surface brightness did not follow any trend during the surface melt progression (R2 = 0.35, p = 0.15). The computed mean range throughout the sampling period was 3.9 ± 1.8 m and matched well the observed length of melt ponds between 3.7 and 4.4 m along the transects. Similar to surface brightness, no temporal trend was observed in the spatial variability of T(PAR) (R2 = 0.07, p = 0.47). However, variability in calculated length scales of T(PAR) was greater, varying between 3.9 and 7.4 m with a mean of 5.4 m.
During stage I, the ice surface was still characterized by a snow cover, such that only a small portion of the incident PAR was transmitted to the underlying water column (Figure 6A). Vertical PAR profiles were no longer influenced by differences in surface light transmission at relatively shallow water depths between 2.0 and 13.8 m (Figure 7). The influence of surface snow and melt pond distribution on the aggregate-scale depth of light transmission increased after the melt pond onset. With the occurrence of melt water at the ice surface during stage II and the formation of a more heterogenous sea ice surface during stage III, the aggregate-scale depth increased to between 14.4 and 29.0 m. This large change in depth was associated with the enhanced differences in transmittance of ponded vs. white ice. A linear regression analysis of the relationship between the aggregate scale depth and several parameters such as , melt pond coverage (%), and the mean CV of revealed only a significant negative trend of the aggregate-scale depth with (R2 = 0.64, p = 0.006). This trend was mainly driven by the large decrease in surface albedo with melt pond onset between 15 and 16 June, when we observed a steep increase in aggregate-scale depth from 8.2 to 22.6 m.
Figure 7. Aggregate scale depth of PAR transmission beneath landfast sea ice over the melt season. The dotted line states the beginning of each melt stage (I–III).
Smooth first-year ice dominated the landfast ice study site. Our observations lacked features such as pressure ridges or leads. Variogram results revealed that the 130 m transect length was more than an order of magnitude greater than the ∼4 m and ∼5 m range of α(PAR) and T(PAR) transect observations, respectively, for the typical surface features. Therefore, our spatial light transmission analysis is believed to be representative of the light available beneath landfast sea ice. Interestingly, spatial continuity in both α(PAR) and T(PAR) did not follow any temporal trend, even though their averaged ranges matched well with surface features that developed during the melt progression. For example, the 3.9 m average range for surface reflection matched the average melt pond size, whereas the range of T(PAR) was slightly larger at 5.4 m. During stage I and II, snow drifts played an important role in the scale of variability in T(PAR) as ranges of spatial variability in snow distribution patterns on FYI were similar to those of T(PAR) (Iacozza and Barber, 1999). During stage III, observed melt ponds were small in the sampling area, so that measured beneath ponds was affected by radiation propagating from the surrounding white ice patches with a mean length of 12.7 m and vice-versa (Ehn et al., 2011). Essentially, scattering by the snow and sea ice diffused the under-ice light field (Matthes et al., 2019), acting to smooth out spatial variability in light transmittance to a longer distance than that of surface melt pond size. Spatial autocorrelation analyses of PAR transmittance through mobile FYI determined even larger ranges between 7 and 30 m which were driven by variations in the ice draft originating from ridges and refrozen leads (Katlein et al., 2015; Lange et al., 2017). In our study, ice draft only varied by a few centimeters along the transect and thus it likely was not a significant factor in influencing the observed spatial variation in transmittance.
Overall, the variability of calculated PAR transmittance along the horizontal transects increased as discrepancy between surface characteristics (ponded vs. white ice) increased (Figure 5B). To investigate the relative change in variation of PAR transmittance over time, the coefficient of variation, CV (%), as the ratio of standard deviation and mean was calculated for each transect and averaged for the three melt stages. In stage I, CV of 34 ± 3% was smallest within the sampling period which is related to the small variability in snow depth and the overall low light levels at the ice bottom. The spatial variability increased with the ablation of snow and the exposure of large stretches of less reflective surface melt water. This caused a 15-fold increase in and a mean CV of 40 ± 11% in stage II and a mean CV of 43 ± 14% in stage III. However, mean CV was not significantly different between the melt stages (F2,11 = 0.876, p = 0.444).
Prior to melt pond onset during stage I of the seasonal progression of light transmittance, measured snow depth and the corresponding low were similar to other observations on landfast and mobile FYI in the Canadian Arctic (Iacozza and Barber, 1999; Campbell et al., 2015). From the melt pond onset on 15 June, wet patches on the ice surface increased, which led to a rapid increase in until maximum transmittance values were reached during the largest spread of surface melt water on 22 June. Another study by Katlein et al. (2019) of the seasonal evolution of light transmission through mobile Arctic sea ice observed a similar increase of integrated (320–900nm) from 0.01 through melting snow-covered ice in June to 0.25 through ponded ice in August. Katlein et al. (2019) also note that spatial variability of (320–900nm) was highest after the melt pond onset. This widespread ponding in stage II due to the disappearance of snow matches as hypothesized the start of the ice ablation season described elsewhere (Eicken et al., 2002; Polashenski et al., 2012; Landy et al., 2014). In these studies, the second stage of melt pond evolution started with the complete removal of snow and an accelerated ice surface melt. Simultaneously, melt water flowed latterly toward flaws (cracks, seal breathing holes, enlarged brine drainage channels) in the ice surface while the ice was still impermeable. This led to a decrease in melt pond size and an increase in white ice patches and relates to our observed stage III of the seasonal progression of light transmittance. Also, the measured decrease in ice draft (Figure 4A), the visible change in ice bottom coloration in the ROV video footage (Figures 4C,D) and the change in shape of the transmitted PAR spectrum toward weaker attenuation at 400 and 700 nm (Figure 6) indicated a sloughing of bottom ice algae during stage I and II, which contributed to the increase in light transmission. To calculate the increase in through this process,Ed(PAR) above the ice algae layer was calculated for 15 June 2016. Following Ehn and Mundy (2013), a Kd(PAR) of 10.45 m–1 was used for the ice algae layer that corresponds to the given chlorophyll a, chl a, concentration extracted from Oziel et al. (2019). Applying Lambert-Beer’s law, the loss of the ice algal layer caused an increase of 0.02 in which corresponds to a 34% increase relative to values during stage I, but much less relative to that of stages II and III. The increase in of 0.2 due to snow melt was an order of magnitude higher. Thus, changes in the snow cover played a more important role in spatio-temporal variability of light transmission to the ice bottom compared to differences in ice algal biomass. Following the increased transmittance through the ice cover in stage II after melt pond onset was an observed rise in under-ice surface water temperature by ∼0.2°C (Figure 4B). A rapid temperature rise by ∼0.2°C was again observed in stage III concurrent with a rapid increase in phytoplankton biomass (Figure 8).
Figure 8. Change in total chlorophyll a (Tchl a) concentration (green circles) integrated over 100-m water column (Massicotte et al., 2020), and in isolume depth (z0.415), extracted from Oziel et al. (2019, white squares), as well as calculated from mean PAR transmittance (, gray squares) and calculated from and scalar irradiance using an inverse average cosine (μd) of 1.4 (blue squares) for each melt stage (I – III) at the ice camp site.
The rapid change in ice surface conditions and the resulting spatial and temporal variations in PAR transmittance through the sea ice underline the importance of continuous irradiance measurements throughout the melt season for the purpose of estimating light availability for under-ice primary production. Area-averaged PAR transmittance values account for increasing spatial variability during sea ice melt progression as also discussed in Massicotte et al. (2019), who investigated the relative error in primary production estimates when averaging a certain number of single-point under-ice light measurements at random locations. The relative error was observed to drop below 10% at 99 under-ice ROV light measurements (Table 3 in Massicotte et al., 2019). However, large scale measurements by autonomous or remotely operated vehicles are expensive and logistically challenging to deploy. Hence, length-weighted mean transmittance, , calculated from measurements beneath melt ponds and white ice combined with aerial surveys by more affordable drones (UAVs) has shown to provide an alternative (Ehn et al., 2011; Taskjelle et al., 2017). Chosen melt ponds and white ice areas for under-ice irradiance measurements should be also sufficiently large to avoid the influence of near-by surface types on the footprint of the deployed radiometer. Following equation (1) in Nicolaus et al. (2010) we calculated the irradiance sensor bottom-ice footprint size that should encapsulate 95% of incoming light measured at the sensor. We obtained a footprint range of 1 to 2 m for the corresponding range in sensor distance from the ice bottom of 0.4 to 0.8 m, as determined from the ROV-mounted altimeter. It is noted that this footprint size is likely a maximum as the equation assumes an isotropic light field, whereas Matthes et al. (2019) concluded that the under-ice light field is downward directed with a corresponding downwelling average cosine of 0.7. Regardless, with the size of melt pond and white ice patches ranging from 3.7 to 4.4 m and 7.4 to 18.0 m in our study, respectively, our ROV transmittance measurements at the center of these surface patches should not be significantly influenced by stray light from pond/ice edges. In conclusion, a lack of statistical difference between our estimates of and confirm the applicability of this UAV technique for future studies requiring characterization of spatial variability in light transmission estimates through smooth first-year sea ice. Satellite-derived melt pond fraction (Rösel and Kaleschke, 2011; Zege et al., 2015) and surface albedo (Scharien et al., 2007) from optical sensors would allow even further upscaling throughout the summer melt. Although, an application on mobile pack ice would need to include a quantitative assessment of ridges and refrozen leads to account for large variations in ice draft.
Photosynthetically available radiation availability in the upper, ice-covered water column can be estimated from incoming irradiance at the ice surface, the presented mean transmittance and the diffuse vertical attenuation coefficient, Kd(PAR). However, recorded vertical irradiance profiles to derive Kd are greatly influenced within the first meters by the previously discussed differences in light transmittance through the melting snow and ice cover (Frey et al., 2011; Massicotte et al., 2018; Matthes et al., 2019). Our results showed a deepening of the aggregate-scale depth with the formation of melt ponds. During stage I, a snow layer covered the landfast sea ice, causing light transmission to be more diffuse (Matthes et al., 2019) and small variations in the range of transmitted irradiance to the ice-water interface. The resultant mean depth at which spatial irradiance levels were no longer affected by surface variability was 7 ± 4 m. After the formation of melt pond in stage II, spatial heterogeneity of and thus the mean aggregate-scale depth increased to 20 ± 6 m. Our observations were in the same range of those reported elsewhere that fall between 5 and 15 m beneath melt pond-covered ice (Frey et al., 2011; Katlein et al., 2015; Matthes et al., 2019).
A correlation between the change in the aggregate-scale depth and sea ice surface properties as well as PAR transmittance was not identified. However, once an under-ice phytoplankton bloom develops, the scattering by phytoplankton may increase the diffusion of the heterogenous transmitted light and, hence, decrease the aggregate-scale depth. This was not observed during our study. In the sampling area, depth-integrated total chlorophyll a (Tchl a, the sum of chlorophyll a, divinyl-chlorophyll a and chlorophyllide a) concentration reached 77 mg m–2 on 1 July over the 100-m water column (Massicotte et al., 2019). This was much less than other observations of under-ice blooms with depth-integrated Tchl a concentrations ranging from 450 to 1292 mg m–2 (Fortier et al., 2002; Arrigo et al., 2014; Mundy et al., 2014). Arrigo et al. (2014) showed a 3.5-fold increase in light absorption and a 5-fold increase in scattering that was mainly attributable to phytoplankton cells (78%). Scattering by phytoplankton cells has also shown to decrease the degree of anisotropy of the downwelling under-ice radiation field in a radiative transfer model (Pavlov et al., 2017).
Radiation in the UV spectrum can inhibit the photosynthetic capacity of phytoplankton (Villafañe et al., 2004) and ice algal communities (McMinn et al., 2005). Previous optical measurements beneath Arctic landfast sea ice recorded a transmittance of 0.01 – 0.02 through bare ice and 0.19 through ponded ice in the UVA (350 – 360 nm) spectral range (Elliott et al., 2015). During our investigation, we showed that UVR transmittance increased significantly with surface melt progression, reaching levels equal to PAR transmittance with of 0.19 beneath white ice and 0.30 beneath ponded ice by the end of June. Particularly after melt pond formation, exceeded because the latter is impacted by the high absorption coefficient in the red part of the visible spectrum. However, transmittance at shorter UV wavelengths remained less than . Laboratory experiments by Perovich and Govoni (1991) demonstrated an increase in the absorption coefficient of snow and ice with decreasing wavelength within the spectrum of 250 to 400 nm, explaining the observed absence of UVB radiation during the early melt stage. However, after melt pond onset, increased to 0.05 through white ice and 0.11 through ponded ice.
Overall, was much larger than previously reported values obtained beneath landfast sea ice in Antarctica (Trodahl and Buckley, 1990) and from radiative transfer modeling for mobile Arctic FYI (Perovich, 2006). This greater transmission highlights the potential ecological significance of UVR transmission measurements during melt season when the spring phytoplankton bloom commences underneath sea ice. Algae in melt pond and in the ice as well as phytoplankton can synthesize mycosporine-like amino acids (MAAs) that act as UV-absorbing sun protection (Uusikivi et al., 2010; Ha et al., 2012; Elliott et al., 2015; Piiparinen et al., 2015). In particular, the study by Elliott et al. (2015) showed a modulation of the MAA concentration of an under-ice phytoplankton bloom with prevailing light conditions and stage of surface melt. Our results show that a significant portion of incoming UVA irradiance, up to 26 μmol photons m–2 s–1, was transmitted during the spring melt when an under-ice bloom commenced beneath the ice cover. Incubation experiments of temperate phytoplankton assemblies in winter with radiation regimes of UVR and PAR or PAR only have shown an enhanced carbon fixation rate at UVR levels < 65 μmol photons m–2 s–1 and significantly lower fixation rates at higher UVR levels (Barbieri et al., 2002). The authors also concluded that the taxonomic composition and light history of the phytoplankton community plays an important role in the sensitivity of algal cells to UVR. More research is therefore needed on the acclimation and photoprotection of under-ice phytoplankton communities to know if our UVA levels < 26 μmol photons m–2 s–1 have an impact on the algal communities.
Several studies have identified the increase in PAR availability and water column stratification associated with melt pond onset as the trigger for under-ice phytoplankton blooms (Arrigo et al., 2014; Mundy et al., 2014; Hill et al., 2018; Oziel et al., 2019). However, the relationship between the sudden change in ice surface properties, the increase in the spatial heterogeneity of PAR transmittance, and the onset of algal growth during the spring melt are still not well understood. Figure 8 provides an overview of the measured increase in Tchl a concentration in the water column (Massicotte et al., 2020) at the ice camp site and the depth of the isolume, z0.415, where integrated PAR24h(z) = 0.415 mol photons m–2 d–1, a threshold used for positive net growth (Boss and Behrenfeld, 2010). The z0.415 was extracted from Oziel et al. (2019), and estimated from from our study using the same daily incident PAR data and Kd(PAR). It is important to note that irradiance measurements presented in Oziel et al. (2019) were taken beneath snow-covered and later white ice at the same location throughout the entire sampling period. At this site, T(PAR) increased from 0.01 to 0.09 between 6 June and 1 July, 2016. However, our ROV measurements determined ranged from 0.01 to 0.31 over the same period (Figure 5B).
As Figure 8 indicates, the initiation of the observed under-ice bloom was directly related to switches in the surface melt stage. During stage I, Tchl a concentration accumulated slowly although the z0.415 deepened from 5 m to 46 m with the exponential increase over time in PAR transmission (Figure 5B). Only after the switch to stage II, Tchl a accumulation accelerated within the mixed surface water layer to a depth of 25 m (Oziel et al., 2019). The z0.415 calculated from reached a greater maximum depth of 50 m compared to a maximum z0.415 of 31 m obtained from the white ice point. To further account for the shape of the under-ice light field, under-ice planar irradiance gained from Ed(PAR,0) and was converted to scalar irradiance by using an inverse average cosine of 1.4 (Matthes et al., 2019). Results show an even deeper maximum z0.415 of 55 m on 18 June. These differences in calculating z0.415 have large implications on the interpretation of nutrient availability for under-ice phytoplankton growth. A deeper z0.415 indicates that phytoplankton had access to a much larger nutrient pool than in the previous estimate. Indeed, Oziel et al. (2019) observed an increased nutrient drawdown at 40 m on 23 June, which also matches well with the second inflection point in Tchl a accumulation in the beginning of stage III. In the end of stage II, the z0.415 shoaled due to the increased light attenuation by the phytoplankton accumulation and remained relatively constant in stage III. By the end of the sampling period, phytoplankton Tchl a concentration leveled off at the maximum observed values of 77 mg m–2, likely as a result of the increased light attenuation by the algal cells and depleted nutrients concentrations in the surface water layer during the melt pond period (Oziel et al., 2019).
In this study, we characterized the seasonal spring progression of the transmission of UVR and PAR, and their spatial variability, in a large landfast sea ice area in southwest Baffin Bay (near the community of Qikiqtarjuaq, Nunavut) throughout the melt season in 2016. Understanding the magnitude of sunlight transmitted through the melting sea ice is of key importance to improve our understanding of the spring phytoplankton bloom. Our objectives were achieved through combined measurements of horizontal transects and vertical profiles of under-ice irradiance using a ROV, and manual and drone-based surveys of ice surface properties. This data set confirms our hypothesis of a close link between the temporal increase in under-ice PAR and UVR levels and the stages of melt pond evolution while the spatial heterogeneity of PAR and UVR transmission remained unchanged after the melt pond onset. The main findings are summarized in points 1–4 below:
1. Our study area was composed of smooth landfast sea ice. Variogram results revealed that the 130 m length of our ROV transects were more than an order of magnitude greater than the 4–5 m length scale of α(PAR) and T(PAR) transect observations for typical surface types. This indicates that our spatial light transmission measurements and statistics were representative of the light available beneath the larger landfast sea ice area.
2. With melt pond formation, spatially averaged PAR transmittance increased from 0.02 to 0.31, while variations in measured under-ice PAR levels increased to up to 43%. This exposed drifting phytoplankton cells to a wide range of light conditions and highlights the importance to accurately capture spatial heterogeneity in light transmission.
3. Melt pond onset on 15 June resulted in a steep increase in aggregate scale depth for under-ice PAR levels from 7 ± 4 to 20 ± 6 m (Figure 7). PAR profiles were found to be affected by surface variability to depths of 2.0–13.8 m during stage I prior to melt pond onset, and to depths of 14.4–29.0 m during stages II-III when melt water was visible present on the surface.
4. With progressing surface melt, exceeded due to the high absorption coefficient in the red part of the visible spectrum. Transmittance at shorter UV wavelengths remained less than . However, after melt pond onset, increased to 0.05 through white ice and 0.11 through ponded ice.
Monitoring the increasing spatial variability in transmitted light levels even under smooth, melting landfast sea ice pose challenges in this rapidly changing environment. However, continuous observations on the spatial and temporal progression of transmitted spectral irradiance in relation to the changing quantities of snow, ice and melt ponds has proven to better explain the link between the deepening of the euphotic zone accompanied by an increased nutrient accessibility and the observed increase in the Tchl a concentration in this area.
All data are accessible at the Green Edge database (http://www.obs-vlfr.fr/proof/php/GREENEDGE/x_datalist_1.php?xxop=greenedge&xxcamp=icecamp2016).
LM, SL-G, JE, and CM contributed to the conception and design of the manuscript. LM, SL-G, GV, and MB contributed to the acquisition of data. LM, SL-G, CM, and JE contributed to the analysis and interpretation of the presented data. The author and all co-authors drafted and/or revised the article and approved the submitted version for publication.
The Green Edge project is funded by the following French and Canadian programs and agencies: ANR (Contract #111112), CNES (project #131425), IPEV (project #1164), CSA, Fondation Total, ArcticNet, LEFE and the French Arctic Initiative (GreenEdge project). Further funding was provided by the Canadian Space Agency and NSERC Discovery Grants to JE and CM and ABOL-CFI to CM and JE. LM was supported by the University of Manitoba graduate student fellowship. SL-G was funded by a postdoctoral fellowship from NSERC.
The authors declare that the research was conducted in the absence of any commercial or financial relationships that could be construed as a potential conflict of interest.
This study is a contribution to the Green Edge project which was conducted under the scientific coordination of the Canada Excellence Research Chair on Remote Sensing of Canada’s new Arctic frontier and the CNRS & Université Laval Takuvik Joint International laboratory (UMI3376). We specially thank Robert Hodgson, Julien Laliberté and Henri Bitting for their help during the ROV deployment, and P. Massicotte and L. Oziel for their support in the data processing. Our field campaign was successful thanks to the contribution of J. Ferland, G. Massé, D. Christiansen-Stowe and M.-H. Forget from the Takuvik laboratory, F. Pinczon du Sel and E. Brossier from Vagabond. This project would not have been possible without the support of the Hamlet of Qikiqtarjuaq and the members of the community as well as the Inuksuit School and its Principal Jacqueline Arsenault. We further thank the two reviewers for their comments to improve this manuscript.
The Supplementary Material for this article can be found online at: https://www.frontiersin.org/articles/10.3389/fmars.2020.00183/full#supplementary-material
Arndt, S., Meiners, K. M., Ricker, R., Krumpen, T., Katlein, C., and Nicolaus, M. (2017). Influence of snow depth and surface flooding on light transmission through Antarctic pack ice. J. Geophys. Res. Oceans 122, 2108–2119. doi: 10.1002/2016JC012325
Arrigo, K. R., Perovich, D. K., Pickart, R. S., Brown, Z. W., van Dijken, G. L., Lowry, K. E., et al. (2014). Phytoplankton blooms beneath the sea ice in the Chukchi Sea. Deep Sea Res. Part II Top. Stud. Oceanogr. 105, 1–16.
Assmy, P., Fernández-Méndez, M., Duarte, P., Meyer, A., Randelhoff, A., Mundy, C. J., et al. (2017). Leads in Arctic pack ice enable early phytoplankton blooms below snow-covered sea ice. Sci. Rep. 7:40850. doi: 10.1038/srep40850
Barbieri, E. S., Villafanñ, V. E., and Helbling, E. W. (2002). Experimental assessment of UV effects on temperate marine phytoplankton when exposed to variable radiation regimes. Limnol. Oceanogr. 47, 1648–1655. doi: 10.4319/lo.2002.47.6.1648
Boss, E., and Behrenfeld, M. (2010). In situ evaluation of the initiation of the North Atlantic phytoplankton bloom. Geophys. Res. Lett. 110:18603.
Campbell, K., Mundy, C. J., Barber, D. G., and Gosselin, M. (2015). Characterizing the sea ice algae chlorophyll a–snow depth relationship over Arctic spring melt using transmitted irradiance. J. Mar. Syst. 147, 76–84.
Diaz, A., Ehn, J., Landy, J., Else, B., Campbell, K., and Papakyriakou, T. (2018). The energetics of extensive meltwater flooding of level Arctic Sea Ice. J. Geophys. Res. Oceans 123, 8730–8748.
Ehn, J. K., and Mundy, C. J. (2013). Assessment of light absorption within highly scattering bottom sea ice from under-ice light measurements: Implications for Arctic ice algae primary production. Limnol. Oceanogr. 58, 893–902.
Ehn, J. K., Mundy, C. J., Barber, D. G., Hop, H., Rossnagel, A., and Stewart, J. (2011). Impact of horizontal spreading on light propagation in melt pond covered seasonal sea ice in the Canadian Arctic. J. Geophys. Res. Oceans 116:C00G02.
Ehn, J. K., Papakyriakou, T. N., and Barber, D. G. (2008). Inference of optical properties from radiation profiles within melting landfast sea ice. J. Geophys. Res. Oceans 113:C09024. doi: 10.1029/2007JC004656
Eicken, H., Krouse, H. R., Kadko, D., and Perovich, D. K. (2002). Tracer studies of pathways and rates of meltwater transport through Arctic summer sea ice. J. Geophys. Res. Oceans 107, SHE 22-1–SHE 22-20. doi: 10.1029/2000JC000583
Elliott, A., Mundy, C. J., Gosselin, M., Poulin, M., Campbell, K., and Wang, F. (2015). Spring production of mycosporine-like amino acids and other UV-absorbing compounds in sea ice-associated algae communities in the Canadian Arctic. Mar. Ecol. Prog. Ser. 541, 91–104. doi: 10.3354/meps11540
Fetterer, F., and Untersteiner, N. (1998). Observations of melt ponds on Arctic sea ice. J. Geophys. Res. Oceans 103, 24821–24835.
Fortier, M., Fortier, L., Michel, C., and Legendre, L. (2002). Climatic and biological forcing of the vertical flux of biogenic particles under seasonal Arctic sea ice. Mar. Ecol. Prog. Ser. 225, 1–16.
Frey, K. E., Perovich, D. K., and Light, B. (2011). The spatial distribution of solar radiation under a melting Arctic sea ice cover. Geophys. Res. Lett. 38:L22501.
Ha, S.-Y., Kim, Y.-N., Park, M.-O., Kang, S.-H., Kim, H., and Shin, K.-H. (2012). Production of mycosporine-like amino acids of in situ phytoplankton community in Kongsfjorden, Svalbard, Arctic. J. Photochem. Photobiol. B 114, 1–14. doi: 10.1016/j.jphotobiol.2012.03.011
Hill, V. J., Light, B., Steele, M., and Zimmerman, R. C. (2018). Light availability and phytoplankton growth beneath Arctic sea ice: Integrating observations and modeling. J. Geophys. Res. Oceans 123, 3651–3667.
Horvat, C., Flocco, D., Rees Jones, D. W., Roach, L., and Golden, K. M. (2020). The effect of melt pond geometry on the distribution of solar energy under first-year Sea ice. Geophys. Res. Lett. 47:e2019GL085956. doi: 10.1029/2019GL085956
Horvat, C., Jones, D. R., Iams, S., Schroeder, D., Flocco, D., and Feltham, D. (2017). The frequency and extent of sub-ice phytoplankton blooms in the Arctic Ocean. Sci. Adv. 3:e1601191. doi: 10.1126/sciadv.1601191
Iacozza, J., and Barber, D. G. (1999). An examination of the distribution of snow on sea-ice. Atmos. Ocean 37, 21–51. doi: 10.1080/07055900.1999.9649620
Katlein, C., Arndt, S., Belter, H. J., Castellani, G., and Nicolaus, M. (2019). Seasonal evolution of light transmission distributions through Arctic sea ice. J. Geophys. Res. Oceans 124, 5418-5435. doi: 10.1029/2018JC014833
Katlein, C., Arndt, S., Nicolaus, M., Perovich, D. K., Jakuba, M. V., Suman, S., et al. (2015). Influence of ice thickness and surface properties on light transmission through Arctic sea ice. J. Geophys. Res. Oceans 120, 5932–5944.
Katlein, C., Perovich, D. K., and Nicolaus, M. (2016). Geometric effects of an inhomogeneous sea ice cover on the under ice light field. Front. Earth Sci. 4:6. doi: 10.3389/feart.2016.00006
Landy, J., Ehn, J., Shields, M., and Barber, D. (2014). Surface and melt pond evolution on landfast first-year sea ice in the Canadian Arctic Archipelago. J. Geophys. Res. Oceans 119, 3054–3075.
Lange, B. A., Katlein, C., Castellani, G., Fernández-Méndez, M., Nicolaus, M., Peeken, I., et al. (2017). Characterizing Spatial Variability of Ice Algal Chlorophyll a and Net Primary Production between Sea Ice Habitats Using Horizontal Profiling Platforms. Front. Mar. Sci. 4:349. doi: 10.3389/fmars.2017.00349
Light, B., Perovich, D. K., Webster, M. A., Polashenski, C., and Dadic, R. (2015). Optical properties of melting first-year Arctic sea ice. J. Geophys. Res. Oceans 120, 7657–7675.
Lu, P., Leppäranta, M., Cheng, B., and Li, Z. (2016). Influence of melt-pond depth and ice thickness on Arctic sea-ice albedo and light transmittance. Cold Reg. Sci. Technol. 124, 1–10. doi: 10.1016/j.coldregions.2015.12.010
Lund-Hansen, L. C., Juul, T., Eskildsen, T. D., Hawes, I., Sorrell, B., Melvad, C., et al. (2018). A low-cost remotely operated vehicle (ROV) with an optical positioning system for under-ice measurements and sampling. Cold Reg. Sci. Technol. 151, 148–155. doi: 10.1016/j.coldregions.2018.03.017
Massicotte, P., Amiraux, R., Amyot, M.-P., Archambault, P., Ardyna, M., Arnaud, L., et al. (2020). Green Edge ice camp campaigns: understanding the processes controlling the under-ice Arctic phytoplankton spring bloom. Earth Syst. Sci. Data Discuss. 12, 151–176.
Massicotte, P., Bécu, G., Lambert-Girard, S., Leymarie, E., and Babin, M. (2018). Estimating underwater light regime under spatially heterogeneous Sea Ice in the Arctic. Appl. Sci. 8:2693. doi: 10.3390/app8122693
Massicotte, P., Peeken, I., Katlein, C., Flores, H., Huot, Y., Castellani, G., et al. (2019). Sensitivity of phytoplankton primary production estimates to available irradiance under heterogeneous sea-ice conditions. J. Geophys. Res. Oceans 124, 5436–5450. doi: 10.1029/2019JC015007
Matthes, L. C., Ehn, J. K., Girard, S. L., Pogorzelec, N. M., Babin, M., and Mundy, C. J. (2019). Average cosine coefficient and spectral distribution of the light field under sea ice: implications for primary production. Elem Sci Anth. 7:25. doi: 10.1525/elementa.363
McMinn, A., Ashworth, C., and Ryan, K. (2005). Growth and Productivity of Antarctic Sea Ice Algae under PAR and UV Irradiances. Bot. Mar. 42, 401–407. doi: 10.1515/BOT.1999.046
Mundy, C. J., Gosselin, M., Ehn, J., Gratton, Y., Rossnagel, A., Barber, D. G., et al. (2009). Contribution of under-ice primary production to an ice-edge upwelling phytoplankton bloom in the Canadian Beaufort Sea. Geophys. Res. Lett. 36:L17601.
Mundy, C. J., Gosselin, M., Gratton, Y., Brown, K., Galindo, V., Campbell, K., et al. (2014). Role of environmental factors on phytoplankton bloom initiation under landfast sea ice in Resolute Passage, Canada. Mar. Ecol. Prog. Ser. 497, 39–49.
Neeley, A. R., Harris, L. A., and Frey, K. E. (2018). Unraveling Phytoplankton Community Dynamics in the Northern Chukchi Sea Under Sea-Ice-Covered and Sea-Ice-Free Conditions. Geophys. Res. Lett. 45, 7663–7671. doi: 10.1029/2018GL077684
Nicolaus, M., Hudson, S. R., Gerland, S., and Munderloh, K. (2010). A modern concept for autonomous and continuous measurements of spectral albedo and transmittance of sea ice. Cold Reg. Sci. Technol. 62, 14–28. doi: 10.1016/j.coldregions.2010.03.001
Nicolaus, M., and Katlein, C. (2013). Mapping radiation transfer through sea ice using a remotely operated vehicle (ROV). Cryosphere 7, 763–777. doi: 10.5194/tc-7-763-2013
Nicolaus, M., Katlein, C., Maslanik, J., and Hendricks, S. (2012). Changes in Arctic sea ice result in increasing light transmittance and absorption. Geophys. Res. Lett. 39:L24501.
Oziel, L., Massicotte, P., Randelhoff, A., Ferland, J., Vladoiu, A., Lacour, L., et al. (2019). Environmental factors influencing the seasonal dynamics of spring algal blooms in and beneath sea ice in western Baffin Bay. Elem. Sci. Anth. 7:34. doi: 10.1525/elementa.372
Pavlov, A. K., Taskjelle, T., Kauko, H. M., Hamre, B., Stephen, H. R., Assmy, P., et al. (2017). Altered inherent optical properties and estimates of the underwater light field during an Arctic under-ice bloom of Phaeocystis pouchetii. J. Geophys. Res. Oceans 122, 4939–4961. doi: 10.1002/2016JC012471
Perovich, D. K. (2005). On the aggregate-scale partitioning of solar radiation in Arctic sea ice during the Surface Heat Budget of the Arctic Ocean (SHEBA) field experiment. J. Geophys. Res. Oceans 110:C03002. doi: 10.1029/2004JC002512
Perovich, D. K. (2006). The interaction of ultraviolet light with Arctic sea ice during SHEBA. Ann. Glaciol. 44, 47–52. doi: 10.3189/172756406781811330
Perovich, D. K., and Govoni, J. W. (1991). Absorption coefficients of ice from 250 to 400 nm. Geophys. Res. Lett. 18, 1233–1235. doi: 10.1029/91GL01642
Perovich, D. K., and Polashenski, C. (2012). Albedo evolution of seasonal Arctic sea ice. Geophys. Res. Lett. 39:L08501. doi: 10.1029/2012GL051432
Piiparinen, J., Enberg, S., Rintala, J.-M., Sommaruga, R., Majaneva, M., Autio, R., et al. (2015). The contribution of mycosporine-like amino acids, chromophoric dissolved organic matter and particles to the UV protection of sea-ice organisms in the Baltic Sea. Photochem. Photobiol. Sci. 14, 1025–1038. doi: 10.1039/c4pp00342j
Polashenski, C., Perovich, D., and Courville, Z. (2012). The mechanisms of sea ice melt pond formation and evolution. J. Geophys. Res. Oceans 117:C01001. doi: 10.1371/journal.pone.0107452
Rösel, A., and Kaleschke, L. (2011). Comparison of different retrieval techniques for melt ponds on Arctic sea ice from Landsat and MODIS satellite data. Ann. Glaciol. 52, 185–191.
Scharien, R. K., Yackel, J. J., Granskog, M. A., and Else, B. G. T. (2007). Coincident high resolution optical-SAR image analysis for surface albedo estimation of first-year sea ice during summer melt. Remote Sens. Environ. 111, 160–171. doi: 10.1016/j.rse.2006.10.025
Taskjelle, T., Hudson, S. R., Granskog, M. A., and Hamre, B. (2017). Modelling radiative transfer through ponded first-year Arctic sea ice with a plane-parallel model. Cryosphere 11, 2137–2148.
Trodahl, H. J., and Buckley, R. G. (1990). Enhanced ultraviolet transmission of Antarctic sea ice during the austral spring. Geophys. Res. Lett. 17, 2177–2179. doi: 10.1029/GL017i012p02177
Uusikivi, J., Vähätalo, A. V., Granskog, M. A., and Sommaruga, R. (2010). Contribution of mycosporine-like amino acids and colored dissolved and particulate matter to sea ice optical properties and ultraviolet attenuation. Limnol. Oceanogr. 55, 703–713. doi: 10.4319/lo.2010.55.2.0703
Villafañe, V. E., Barbieri, E. S., and Helbling, E. W. (2004). Annual patterns of ultraviolet radiation effects on temperate marine phytoplankton off Patagonia, Argentina. J. Plankton Res. 26, 167–174. doi: 10.1093/plankt/fbh011
Zege, E., Malinka, A., Katsev, I., Prikhach, A., Heygster, G., Istomina, L., et al. (2015). Algorithm to retrieve the melt pond fraction and the spectral albedo of Arctic summer ice from satellite optical data. Remote Sens. Environ. 163, 153–164.
Zhang, J., Ashjian, C., Campbell, R., Spitz, Y. H., Steele, M., and Hill, V. (2015). The influence of sea ice and snow cover and nutrient availability on the formation of massive under-ice phytoplankton blooms in the Chukchi Sea. Deep Sea Res. Part II Top. Stud. Oceanogr. 118, 122–135. doi: 10.1016/j.dsr2.2015.02.008
Keywords: Arctic sea ice, radiative transfer, PAR, UVR, transmittance, spatial variability, ROV, under-ice phytoplankton bloom
Citation: Matthes LC, Mundy CJ, L.-Girard S, Babin M, Verin G and Ehn JK (2020) Spatial Heterogeneity as a Key Variable Influencing Spring-Summer Progression in UVR and PAR Transmission Through Arctic Sea Ice. Front. Mar. Sci. 7:183. doi: 10.3389/fmars.2020.00183
Received: 13 December 2019; Accepted: 09 March 2020;
Published: 26 March 2020.
Edited by:
Robyn E. Tuerena, The University of Edinburgh, United KingdomReviewed by:
Lars Chresten Lund-Hansen, Aarhus University, DenmarkCopyright © 2020 Matthes, Mundy, L.-Girard, Babin, Verin and Ehn. This is an open-access article distributed under the terms of the Creative Commons Attribution License (CC BY). The use, distribution or reproduction in other forums is permitted, provided the original author(s) and the copyright owner(s) are credited and that the original publication in this journal is cited, in accordance with accepted academic practice. No use, distribution or reproduction is permitted which does not comply with these terms.
*Correspondence: Lisa C. Matthes, bWF0dGhlc2xAbXl1bWFuaXRvYmEuY2E=
Disclaimer: All claims expressed in this article are solely those of the authors and do not necessarily represent those of their affiliated organizations, or those of the publisher, the editors and the reviewers. Any product that may be evaluated in this article or claim that may be made by its manufacturer is not guaranteed or endorsed by the publisher.
Research integrity at Frontiers
Learn more about the work of our research integrity team to safeguard the quality of each article we publish.