- 1MAST-FOCUS, Liège University, Liège, Belgium
- 2European Global Ocean Observing System (EuroGOOS), Brussels, Belgium
- 3Department of Research and Development, Danish Meteorological Institute, Copenhagen, Denmark
- 4Marine Institute, Ocean Science and Information Services, Galway, Ireland
- 5ISMAR-CNR, Venice, Italy
- 6Helmholtz-Zentrum Geesthacht Centre for Materials and Coastal Research, Geesthacht, Germany
- 7Deltares, Delft, Netherlands
- 8Mechanical Engineering Department, MARETEC–Marine Environment and Technology Center, Instituto Superior Técnico, Universidade de Lisboa, Lisbon, Portugal
- 9Istituto Nazionale di Oceanografia e di Geofisica Sperimentale (OGS), Trieste, Italy
An overview of the current European capacity in terms of operational modeling of marine and coastal systems is presented. This overview is compiled from a survey conducted in 2018–2019 among members of EuroGOOS and its related network of Regional Operational Oceanographic Systems, addressing the purposes, context and technical specificities of operational modeling systems. Contributions to the survey were received from 49 organizations around Europe, which represent 104 operational model systems simulating mostly hydrodynamics, biogeochemistry and sea waves. The analysis of contributions highlights the strengths and weaknesses of the current capacity from an operational point of view, and leads to the formulation of recommendations toward the improvement of marine operational modeling services in Europe. In particular, this study highlights the heterogeneity of the European operational modeling capacity in terms of atmospheric and land boundary conditions, its limited deployment for biogeochemical phenomena, and a restricted use of data assimilation methods. In order to improve the accuracy of their simulations, model operators aim toward a further refinement of spatial resolution, and identify the quality and accessibility of forcing data and the suitability of observations for data assimilation as restricting factors. The described issues call for institutional integration efforts and promotion of good practices to homogenize operational marine model implementations, and to ensure that external forcing datasets, observation networks and process formulations and parameterizations are adequately developed to enable the deployment of high-level operational marine and coastal modeling services across Europe.
1. Introduction
Numerical ocean and coastal modeling is one of the key pillars of the operational oceanography value chain. Operational Oceanography is defined as the set of activities for the generation of products and services providing actionable information on the marine and coastal environment, designed to fulfill societal and scientific needs (Malone and Cole, 2000; Zhu, 2011; Kourafalou et al., 2015a,b; Liu et al., 2015; Schiller et al., 2018). Those activities, that range from the routine collection of in-situ and satellite ocean observations, near real time data processing and dissemination, and ocean state forecast and reanalysis, are densely interlinked. For instance, while validated models requires strong observational grounds, they can contribute to the efficient design and optimization of coastal observing systems for science and operational uses (e.g., Fujii et al., 2019).
A wide variety of operational models are currently used in European Seas, based on different computer codes and sets of parameterizations, resolving a disparate range of spatial and temporal scales, using diverse data sources as forcing and as initial and boundary conditions and relying, or not, on data assimilation methods (Bell et al., 2009; Bahurel et al., 2010; Le Traon et al., 2019). Examples of the services and products provided by such models may be found following the links given in Table 1. This diversity in model technical characteristics stems from geographical constraints and from the specific requirements of different modeling objectives. Those objectives are set according to regional and/or sectorial information needs, for instance on given phenomena of interests, or on particular temporal and spatial scales.
In view of such a heterogeneous abundance of model engines, an updated catalog of the operational models being currently used in the European Seas would be an useful tool to provide a snapshot evaluation of the current marine operational modeling capacity in Europe. Moreover, it would allow to perform a requirements and gap analysis of the existing operational modeling capacity in the European Seas from where recommendations for improvement can be drawn.
The main objective of this study was to generate an updated extensive catalog of the existing operational numerical ocean and coastal modeling capacity landscape in the European seas. In order to achieve that objective, and with a view to get a wide geographical representation, a survey on operational modeling capacities has been designed and launched through the Coastal Working Group (CWG) of the European operational oceanography community network represented by the European Global Ocean Observing System (EuroGOOS).
EuroGOOS is an international association of 44 national agencies, institutes and research organizations in 19 European countries promoting and implementing Operational Oceanography in Europe. Founded in 1994, EuroGOOS operates in the framework of the Global Ocean Observing System (GOOS) of UNESCO/IOC. EuroGOOS is achieving its main goals through a network of five Regional Operational Oceanography Systems (ROOSes), four working groups and seven networks of ocean observing platforms (task teams). Coastal operational oceanography is one of the four priority areas included in the EuroGOOS future scientific strategy (She et al., 2016). The CWG of EuroGOOS (http://eurogoos.eu/coastal-wg/) is devoted to examine and integrate the entire coastal operational oceanography value chain from coastal observations, satellite data, ocean forecasts and analysis, to products and services for a variety of users. As one of its priorities, the CWG assesses the latest operational modeling capacity in European coastal seas in order to formulate recommendations for improvement of the coastal services.
Although priorities of operational modeling and forecasting have been previously identified in institutional strategy road maps [e.g., EuroGOOS Scientific Strategy white paper (She et al., 2016) and Copernicus Marine Environment Monitoring Service (CMEMS) High Level Service Evolution Strategy (Mercator Ocean STAC, 2016)]; they were identified by leading researchers and research managers instead of operational modelers who are carrying out routine operational modeling activities at their respective organizations. By collecting inputs from the modelers who are carrying out operational modeling activities, this study will contribute to provide, from a more “operational” technical point of view, an assessment of the gaps, needs and priorities for improving operational modeling capacity in Europe.
Section 2 describes the survey and the audience of contributors. Collected contributions are presented and analyzed in section 3 and discussed in terms of gaps and requirements in section 4. Section 5 then concludes with consequent recommendation for further improvements. Reference tables for contributing institutes, models used and national and transnational data providers are listed in the Appendix.
2. Materials and Methods
An online survey on operational modeling capacity was first distributed to all the members of the CWG (representing 15 organizations around Europe) and among the chairs of the five EuroGOOS Regional Operational Oceanographic Systems (ROOS), who further forwarded the questionnaire among the modelers responsible for the operational modeling activities in their corresponding region. The EuroGOOS ROOS community, which comprises around 100 organizations including national agencies, universities, research centers, and private enterprises providing operational ocean services across Europe, offers therefore a good representation of the operational oceanography in Europe. The survey was also sent to operational modelers participating in related EU-funded projects, such as INTERREG Atlantic Area MyCOAST or H2020 ODYSSEA and shared among the CMEMS regional Monitoring and Forecasting Centers (MFCs), in such a way that the survey includes the CMEMS community models as well as other local and regional operational or pre-operational models. Although this information was not explicitly gathered, due to the technical character of the questions in the survey, it was completed most of the times by the operational modelers in each of the institutes who are responsible for the implementation (or plan to implement in the near future) of an operational numerical ocean model.
In total, the survey was sent to more than 100 contacts around Europe, granting a wide geographical coverage. It should be noted, however, that the survey does not cover all the existing operational numerical models running in Europe. For instance, the private sector has not extensively been reached, though a few private companies were contacted and effectively contributed to the survey.
The form used for the survey is provided as a Supplementary Material to the manuscript. The survey questions were based and inspired on a previous catalog of existing global, regional and coastal ocean models generated from the GOOS Regional Alliances (GRAs) performed in year 2015 (www.eurogoos.eu/models). The questionnaire was extended and systematized to ease external contributions and their analysis, and to collect sufficient information so as to access relevant technical details of the European marine modeling capacity.
The first question asked for basic information related to the organization. This section is followed by seven sections addressing important components of operational modeling capacity. These components include: model software; spatial configuration (e.g., horizontal and vertical resolution); model outputs in terms of Essential Ocean Variables (EOVs) and their purposes for Phenomenon of Interests (POIs); operational work flow (e.g., length of the forecast); data assimilation (if any, assimilation schemes and data sources); forcing and input data (lateral boundary conditions, atmospheric forcing, tidal, and land forcing); model validation and priorities for potential means of model improvements, respectively.
The authors are aware that many other questions could have been asked to make the survey more complete and exhaustive. The survey, however, was designed as a compromise between technical modeling details, for an in-depth characterization of the current model capacity, and brevity, to ease and maximize contributions to the survey so as to obtain an optimal representation of current status.
3. Results
Since the online survey was launched, in October 2018, to the date of last answer in December 2019, the questionnaire was completed by 49 institutes and organizations delivering 104 model configurations for the different European Seas (Table 1). In this section, we present the main results derived from the survey, analyzing the different modeling setups and highlighting emergent and relevant features.
3.1. Geographic and Sectorial Representation
Figure 1 shows the geographical domains of operational implementations included in the survey, as well as locations and names or acronyms of providing institutions, representing operational oceanographic agencies from 20 European coastal countries and Israel and Turkey (Table 1). Regional maps are also provided as Supplementary Material, which allow to identify the institution (from Table 1) operating each specific model domain.
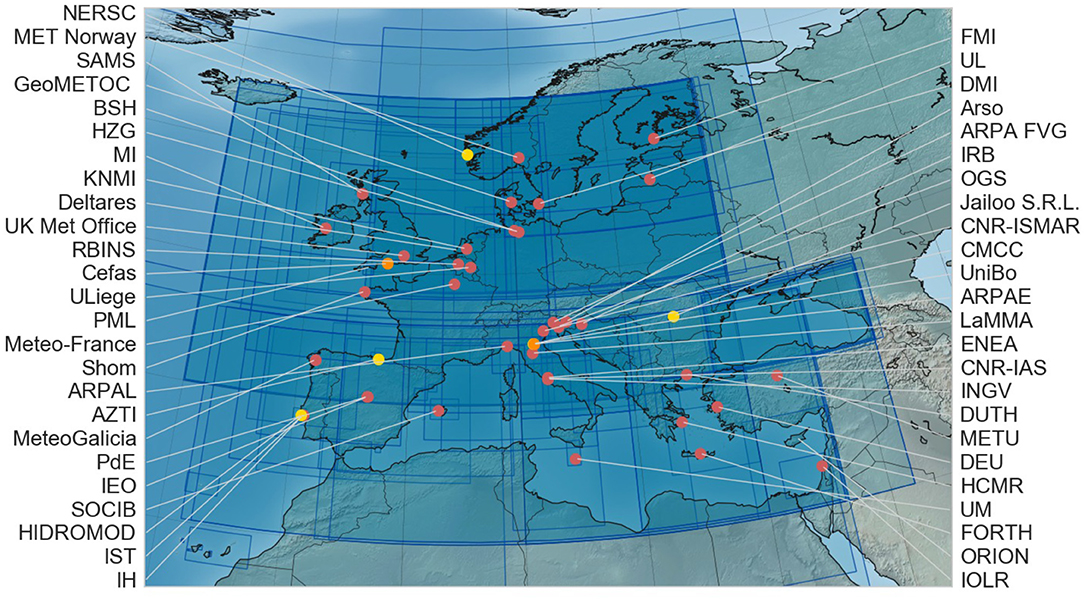
Figure 1. Model domains (blue boxes) and location of the institutes and organizations who contributed to the operational modeling capacity survey (red points denote public institutions, yellow: private, orange: mixed public/private. See Table 1 for more information on the institutes and organizations).
All EuroGOOS ROOSes are represented to a reasonable degree in the survey (Figure 2). The Black Sea is less densely covered, with no sub-regional model being reported. Only two models are reported for the Arctic ROOS, with no further models being scheduled in the coming years. Some domains pictured on the map represent multiple implementations of the same modeling system (e.g., PdE and IST use the same modeling system in several areas of Spain or Portugal). Also, some models are not defined for a fixed domain, but are instead relocatable models designed for fast, high resolution deployment upon request (e.g., for oil spills or search and rescue needs). We can also observe that some regions, especially in northern Europe, are better covered with operational ocean models than other regions as, for instance, in the south-eastern Mediterranean. Also, no detailed models are reported for the extreme northern region (e.g., Iceland, Svalbard, Greenland coasts) and some of the Baltic Sea EU countries are not represented, i.e., Estonia, Lithuania, Poland, and Sweden. While some organizations didn't complete the survey, it may also be that, despite our distribution and communication efforts, the survey did not reach all the relevant organizations in some areas.
Most contributions have been filled by the persons in charge of setting-up operational modeling systems and of issuing model results to distribution platforms. A vast majority of the respondents to the survey operate hydrodynamic models. Waves and biogeochemical models were represented to a lesser extent, while specific particle drift and sea ice models are scarce (Figure 3).
Most respondents (80%) represent public or governmental institutions, others being issued from private institutions, including both profit and non-profit ones. Data generated by the operational systems are in most cases (76%) freely available to relevant users, but are sometimes only available to restricted partners upon expressed agreements (20%) or restricted to internal use among the producing organization (4%). No case of data being only accessible at a given cost was reported to the survey which also indicates a lesser representation of private companies in the survey.
Modeling systems were distinguished by their level of operationality, which is used to present and discuss the results and is defined as follows. “Operational” models are in effective operational production and regularly provide forecasts through an online distribution platform. “Pre-operational models” are finalizing the distribution framework and are foreseen to transition toward full operational mode within the coming 2 years. “Scheduled” implementations are foreseen to transition to operational mode within the next 5 years.
3.2. Essential Ocean Variables (EOVs) and Phenomena of Interests (POIs)
In the following, EOVs or “variables” denote measurable quantities that describe some aspect of the marine state conditions (Lindstrom et al., 2012). POIs denote processes that are directly relevant for certain economic sectors or certain aspects of the regional environmental status (Malone, 2003). There exist obvious relationships between EOVs and POIs, as defined for instance by GOOS1. In this survey, however, contributors were left to their own judgement to identify the POIs' for which the data provided by their system could be relevant, based on the rationale that this should reflect their knowledge of the user community and/or institutional objectives. The EOVs (Figure 4) and POIs (Figure 5) considered are displayed for the three classes of operational model implementations.
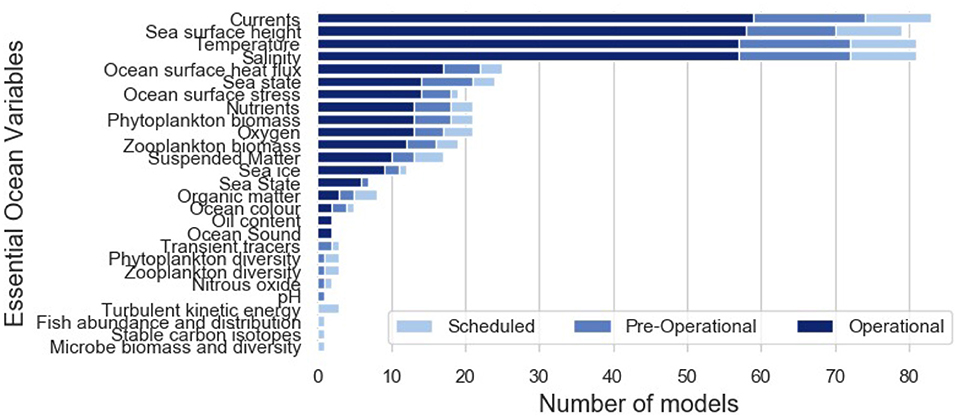
Figure 4. Number of operational, pre-operational, and scheduled model implementations providing quantitative results for each Essential Ocean Variable (EOV).
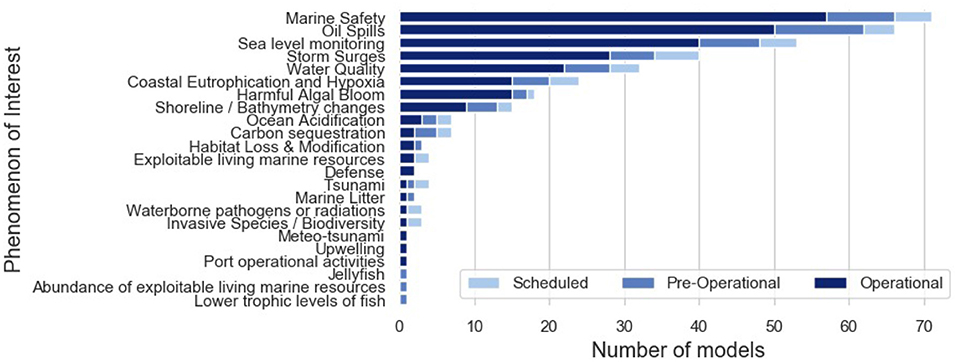
Figure 5. Number of operational, pre-operational, and scheduled model implementations providing quantitative results for each Phenomena of Interest (POI).
A first group of EOVs (currents, salinity, temperature, and sea surface height) are resolved for almost all operational models. Physical variables needed for specific applications (e.g., sea state, ocean surface stress, sea ice, surface heat fluxes) and the basic variables of biogeochemistry (oxygen, nutrients, phyto- and zoo-plankton biomasses, suspended, and organic matter) are both represented by 15–25 systems. Finally, some variables are poorly represented in the current operational context: Ocean sound, diversity of planktonic groups, fishes and microbe populations. It is notable that only a single pre-operational system reported in this survey claims to provide an operational description of pH in marine and coastal waters.
Marine safety, oil spills and sea level monitoring appear as the phenomena mostly addressed by European operational models to date (>40 operational implementations). Storm surges, water quality, and eutrophication are well-considered at present (~15–25 implementations) and will benefit from an extended coverage in the coming years (~ +30–50 % within 5 years). Harmful algal blooms, shoreline/bathymetry changes and ocean acidification, are considered in some parts of Europe, but may still suffer from a restricted coverage within the next 5 years. Other POIs are only addressed by specific dedicated implementations without benefiting from current or scheduled regular spatial coverage across the European Operational modeling systems (Figure 6). It is noticeable that no operational description of biogeochemical phenomena is currently reported from Artic ROOS.
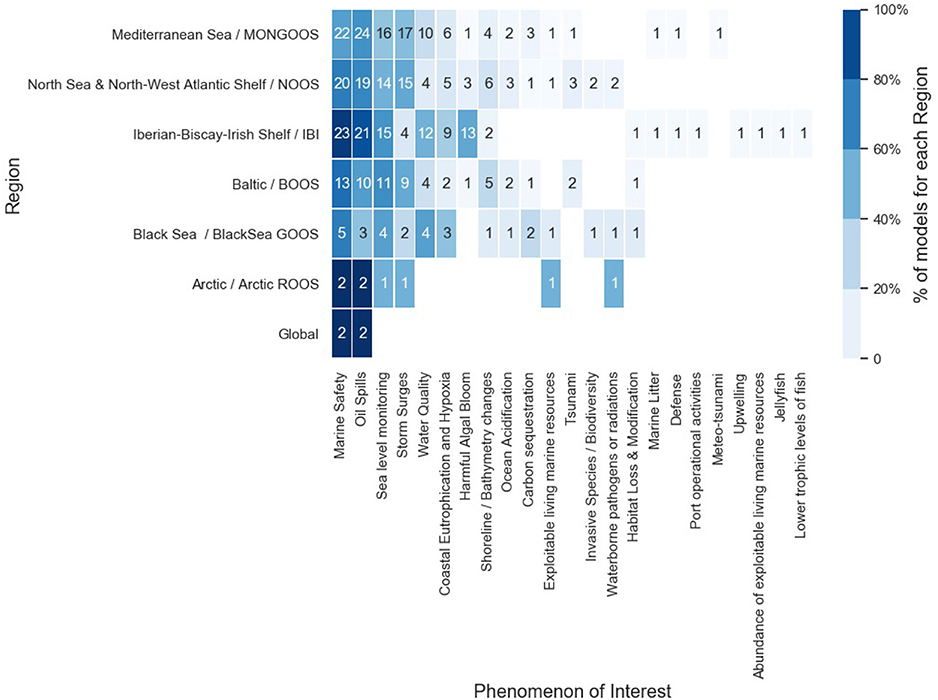
Figure 6. Relative representation of Phenomena of Interest within each particular region/ROOS. Numbers inside each box represent the total number of model implementations.
3.3. Spatial and Temporal Specifications
Figure 7 provides the range of spatial resolutions considered for the different hydrodynamic and wave models of the survey (cf. Table 2 for model references). Spatial resolutions vary over three orders of magnitude from 10 m to 10 km, with 50% of the models having a minimum resolution between 250 m and 2 km (the median resolution is 1 km). The survey specifically asked for “minimum” spatial resolution to highlight the capacities of unstructured grid models (e.g., SHYFEM, SCHISM, FVCOM) and two-way nested models (e.g., HBM, ROMS, NEMO) to focus over sub-domains of interest. For hydrodynamic models, the applications can be divided into two groups: a high resolution group and a varying resolution group. The former mainly applies to models with horizontal resolutions between 10 and 2,000 m. These models are mostly typical coastal-estuary models, e.g., HBM, SCHISM, FVCOM, SHYFEM, GETM. The latter applies to models with a wide range of horizontal resolutions between 10 m and 10 km, e.g., MOHID, HYCOM, and NEMO.
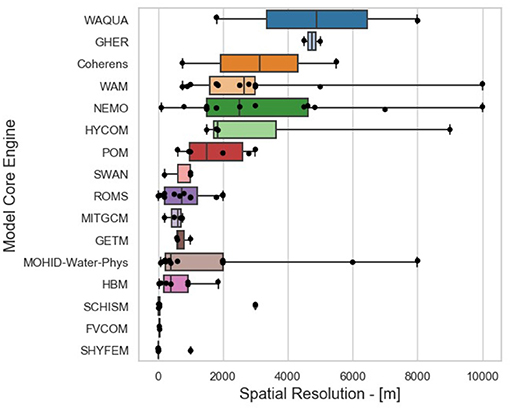
Figure 7. Spatial resolution of the ocean hydrodynamics and waves (WAM and SWAN) model implementations presented in the survey (cf. Table 2 for model references).
The geographical conditions of different European regions appear to have a naturally enhanced coastal focus in the Baltic and North Sea (Figure 8), where both spatial grid size and minimum bathymetry are the lowest (considering regional means of all models, while assigning a minimum bathymetry of 0 m for models including a wetting/drying facility).
The survey depicts an even share between different types of vertical coordinate systems, i.e., the way in which the vertical dimension is divided into distinct cells when computing simulations: between 2D (22), sigma- (32), z- (33), and hybrid- (16) coordinate systems. The median numbers of vertical layers are 26 and 41 for models using sigma and z coordinates systems, respectively. 2D models are common among implementations addressing shoreline changes, marine safety, oil spills and harmful algal bloom issues.
Regarding temporal specifications, forecasts are updated with a frequency between 6 and 24 h (75% of reported systems). Some targeted services are delivered on demand. Such “on-demand” delivery concerns only one “Operational” system, but is specified for five additional “Scheduled” systems, and can thus be seen as a developing trend. 50% of the reported systems provide forecast with at least 3 days in advance, while only 10% extends beyond 7 days in advance.
3.4. External Forcings and Boundary Conditions
Marine and coastal systems are open systems, influenced by atmospheric conditions and terrestrial runoff. Also, domains limited in space implies that oceanographic conditions should be prescribed at the lateral boundaries to enable operational simulations.
Ninety-three percent of the modeling systems are based on real-time atmospheric forcing. A large proportion (36%) relies on ECMWF (European Center for Medium Weather Forecast) products either directly or as forcing for local atmospheric models (Figure 9). Such local atmospheric models are used by 22% of the respondents, exploiting atmospheric modeling software, such as WRF (10 users), MM5 (6), Harmonie (4), HIRLAM (3), and Aladin (3). A third large group (36%) relies on regional products delivered by national meteorological institutions, depending on the region of activity (cf. Table 3). Only a small number of contributors (i.e., for ~10% of the model systems) consider a pre-processing of atmospheric forcing before their application to marine models, such as re-interpolation or filtering of land-grid influence in coastal areas, which is recommended for forecasting in coastal waters (e.g., Kara et al., 2005).
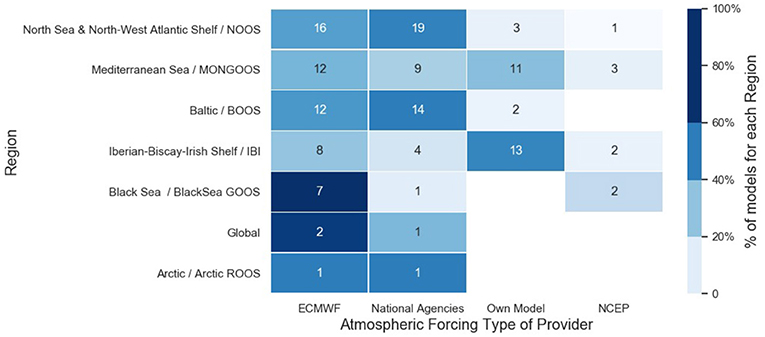
Figure 9. Type of atmospheric forcing sources considered per region. Numbers inside each box represent the total number of models.
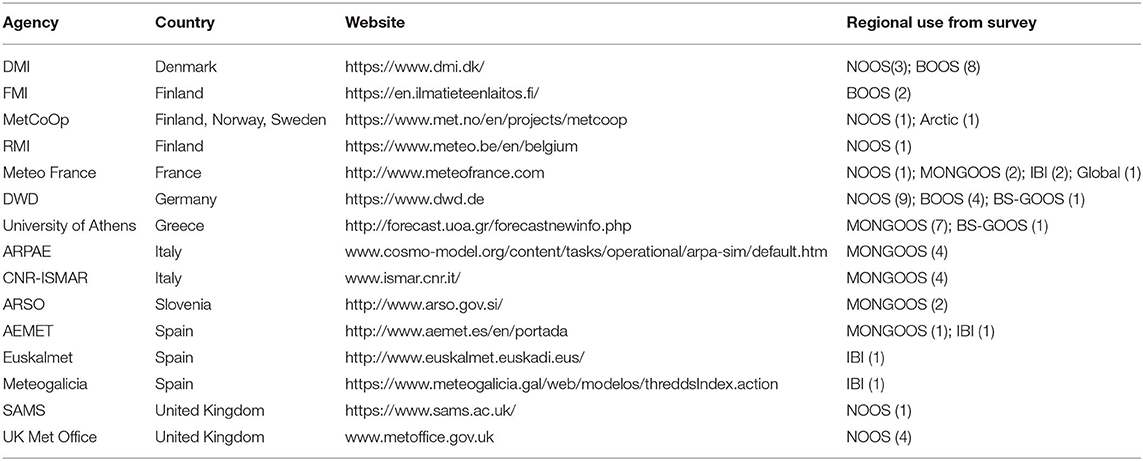
Table 3. Reference website of national providers of local atmospheric forcing mentioned in the survey.
Land forcing data are considered to be relevant for two thirds of the model systems. Systems relying on climatological and real-time land forcing are reported in equal proportions, with the latter being favored for applications targeting biogeochemical phenomena. Systems requiring real time land discharge forcing (Figure 10) usually rely on forecasts/observations provided by national agencies (cf. Table 4), the e-Hype service provided by SMHI (Lindström et al., 2010), or on their own catchment model (e.g., MOHID, TRIP, SWAT). Systems relying on climatological land forcing use published datasets (e.g., Ludwig et al., 2009; Janeković et al., 2014; van Apeldoorn and Bouwman, 2014), data from national agencies, or from the e-Hype service.
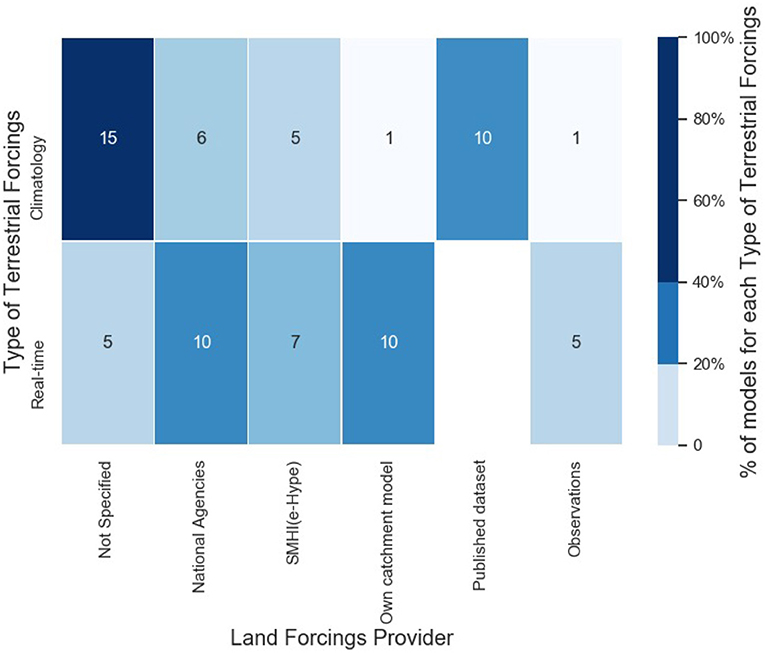
Figure 10. Type of land forcing providers for systems favoring climatological and real-time land forcings. Numbers inside each box represent the total number of models.
About half of reported systems rely on CMEMS for open boundary conditions. The second larger group relies on coarse large-scale ocean models maintained within their own institution, while others use climatologies or do not need boundary conditions at all.
The tidal dynamics is considered in all Iberian-Biscay-Irish Shelf cases, 82% of the North Sea cases, about two thirds of the Mediterranean and Baltic cases, and never in the Black Sea.
Finally, for a vast majority of cases (70%), seafloor parameterization is considered as invariant in time but variant in space. It is considered as irrelevant for 5% of the cases, while dynamic seafloor parameterization appears as a strong exception (only three cases, located in the Black, Baltic and North Seas).
3.5. Use of Observations
It is beyond the scope of this survey to enquire about the quantitative skill of the models, which would require a much more comprehensive assessment. We note however that only 20% of models provide a dynamic uncertainty together with the forecasted EOVs, which would be required for a real-time provision of confidence levels associated with the forecasts (e.g., as is usual for instance in weather forecasts). Data used for model skill assessment and validation are mainly originated from fixed platforms, remote sensing and tidal gauges (Figure 11A).
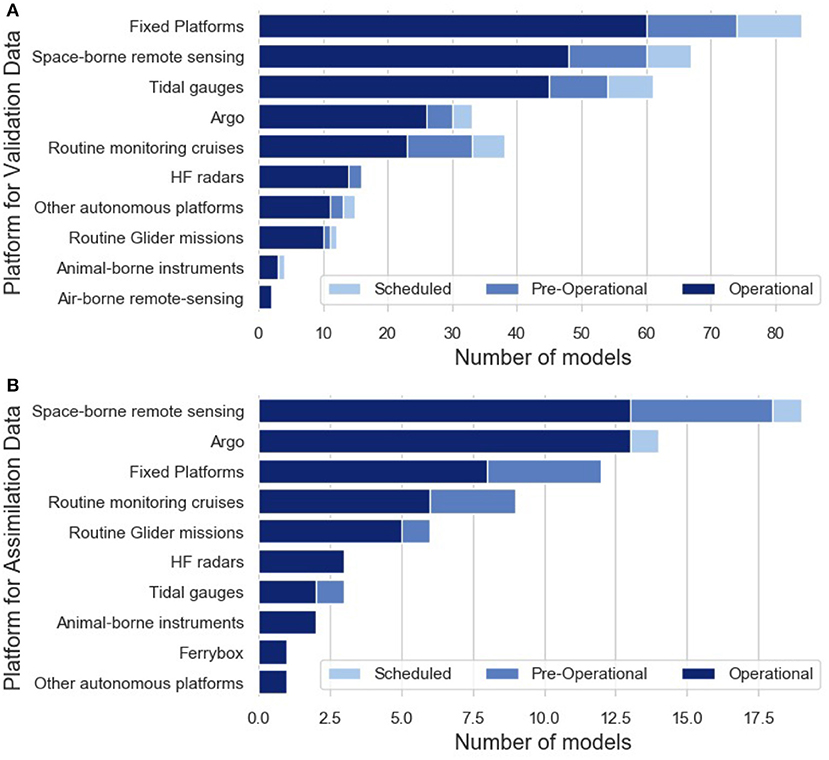
Figure 11. Observing platforms providing data used for (A) model skill assessment and validation purposes, and (B) assimilation in the operational modeling systems.
Only 24 models reported in this survey (23%) use some form of data assimilation, 8 (8%) of which only use it offline, for operational production of reanalysis. One could have expected that future implementations would make larger use of data assimilation. Instead, only 6 of the 29 reported pre-operational and scheduled systems consider data assimilation, with only one of those considering online data assimilation. Obviously, this restricted number of assimilating models calls for caution concerning the survey results on assimilation methods and exploited data sets.
Assimilating implementations exploits observations originating from space-borne remote-sensing and Argo profiling floats (Figure 11B) and from routine monitoring frameworks to a lesser extent (fixed platforms, cruises, gliders). Assimilation is largely restrained to physical variables (Figure 12), and considers primarily sea surface temperature and sea surface height (from remote sensing) as well as subsurface temperature and salinity, from Argo floats in deep waters and fixed platforms/cruises in the coastal ocean. Assimilation of ocean currents is rare (five systems, exploiting remote sensing and HF radars inputs), while assimilation of biogeochemical variables remains largely exceptional.
The methods used to implement data assimilation (Figure 13) range from simple nudging/relaxation (about 20% of assimilating systems) to variational methods (~ 40%) and ensemble methods (simplified filters and ensemble Kalman Filter, ~ 40%). It is noted that there are still planned activities for using the simple nudging/relaxation technique in the future.
3.6. Means of Improving Model Accuracy
Contributors to the survey were asked to select three top-priorities to improve the accuracy of their modeling systems. The answers show that priorities vary with the POIs of concern.
The three most common strategies envisaged to improve the accuracy of oceanographic operational models are higher quality atmospheric forcing, increasing spatial resolution, and acquiring more data for assimilation. Subsequent priorities include revising the formulation and parameterization of marine processes and enhancing land discharge forcing (Figure 14).
Systems addressing marine safety and oil spills would equally aim for increased resolution, improved atmospheric forcing and data for assimilation (Figure 15). Storm surges and sea level monitoring systems put a special emphasis on increased spatial resolution. Shoreline monitoring systems present in addition a relative emphasis on the need for improved bathymetry datasets. Contributors in charge of modeling systems addressing all biogeochemical POIs (water quality, eutrophication, carbon sequestration, acidification, harmful algal bloom, and exploitable living resources) call for an improvement of land discharge forcing as a first priority.
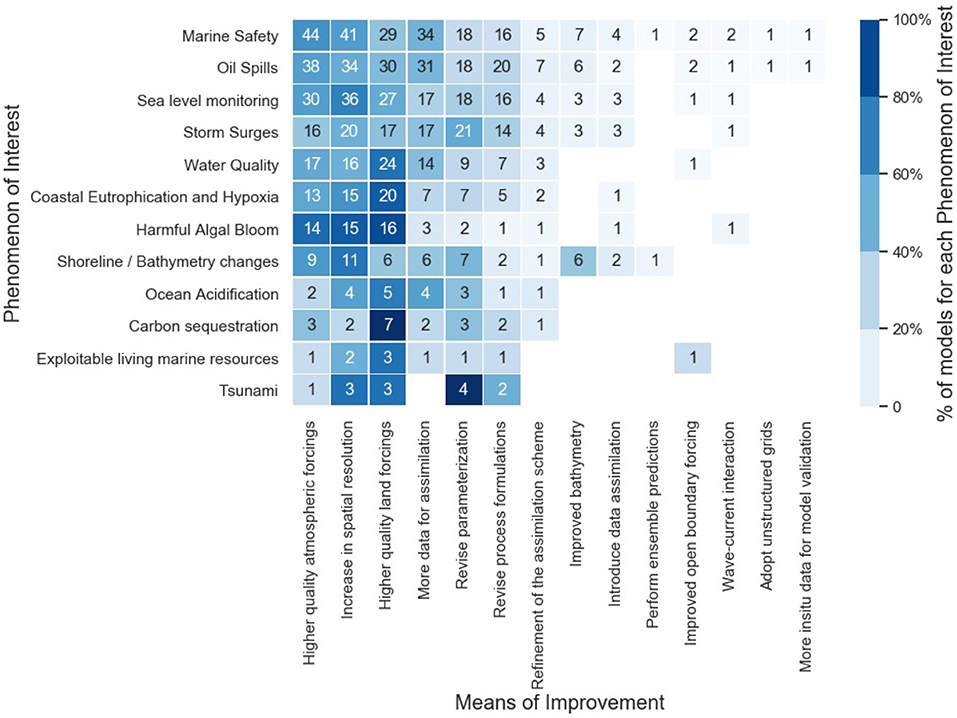
Figure 15. Priority means to improve operational ocean modeling capacity categorized per POI addressed. Numbers inside each box represent the total number of models.
4. Discussion
In this section we highlight the main strengths and gaps in the European operational modeling landscape derived from the analysis performed on the survey results.
4.1. Lack of Biogeochemical Forecasts
The survey highlights that a clear majority of the European marine and coastal systems address physical phenomena and variables, for which all European coasts benefit from some model coverage. The same can not be said for biogeochemical or biological phenomena and variables. There may be several explanations for this fact.
First, biogeochemical models are more complex, involve more parameters, and may not have reached the level of maturity as required for accurate operational predictions. This is strikingly reported concerning data assimilation, for instance. This explanation is still valid, even though in the past decade substantial efforts have been dedicated to developing and improving biogeochemical model applications with the appropriate complexity suitable for operational forecasting (Gehlen et al., 2015).
Second, the need for operational biogeochemical predictions with a few days range may currently not be as acute as for physical variables. Users concerned with ecosystem-based management (e.g., in relation with the European Marine Strategy Framework Directive and Water Framework Directive) may be more concerned with longer term products, either for environment and ecosystem assessment or fishery management at seasonal, annual and interannual scales. On the other hand, economic sectors of activity for which short-term operational biogeochemical forecasts would be relevant, may either not be developed or have not included operational oceanography in their management and operation chains, to a sufficient degree so as to induce a substantive development of biogeochemical operational modeling.
A third reason, less straightforward, is related to the fact that all models addressing biogeochemical aspects in the present survey also provide data concerning the physical state of the sea. As we climb on the complexity scale from physics to biology, “online coupled” models, which resolve ecosystem dynamics together with the basic physical variables, may be left aside in favor of “offline coupled models” implemented by downstream users of the models considered here. Those intermediaries, with private companies in particular, appear to be under-represented in this survey.
4.2. Limited Data Assimilation
The limited consideration of data assimilation is a surprising finding of the present survey. It also relates to the earlier observation that only 20% of the models provide a dynamic uncertainty complementing the operational data, which is more easily issued for assimilating systems. This result is even more surprising considering that the need for more assimilation data appears as the third priority to increase the accuracy of operational models, while very few contributors consider refinement or introduction of assimilation schemes as an improvement priority. One contributor provided a clarification, stressing that the delay between data acquisition and delivery actually prevented the use of data assimilation in their operational forecasts and reanalyses. By ticking the option “more data for assimilation,” the contributor actually wanted to stress the need for more timely and suitable data to enable the use of data assimilation in a strict operational time-schedule. Such a data requirement was also identified at European (She et al., 2016) and regional level (She, 2018; Le Traon et al., 2019).
Those comments reflect the fact that more efforts in shortening the delivery time of observations, such as from R/V measurements, and promotion of a systematic use of assimilating scheme are still needed in order to foster an European operational oceanographic network able to support the management of marine activities, which requires complementing predictions with confidence levels. Remote-sensing and autonomous in situ programs are a key element of such efforts, as highlighted by the preference for those platforms in assimilating systems (Figure 11B).
Another surprising result in regards with data assimilation is the resilient use of simple schemes, such as nudging/relaxation, while better qualified assimilation methods are well-documented and provided within open sources toolboxes (see Table 2). One could wonder, here, to which extent the need for high qualification and the limited availability of qualified personnel, play in restraining the systematic deployment of data assimilation within operational marine and coastal models.
4.3. Need to Improve External Forcing and Input Data
It appears that external forcing and boundary conditions remain one of the major issues restricting the accuracy of operational systems. This concerns both atmospheric conditions for physical systems, and terrestrial freshwater discharges and nutrients loads for biogeochemical systems. In addition, increasing spatial resolution is also perceived as a priority to enhance the accuracy of operational modeling systems. In parts, increase in spatial resolution is limited by computational means which can be addressed without specific institutional efforts (i.e., except from consolidating computational infrastructure networks). On the other hand, to be effective such an increase in spatial resolution should go along with improvements of data for coastline and bathymetry and a higher resolution of atmospheric forcing.
The survey thus highlights that the main restraining factors in providing an accurate description of the marine environment are considered to be external (data and forcings), rather than internal (processes and parameters). An obvious example is given by models of coastal biogeochemistry, which are largely driven by land inputs of nutrients and organic materials (e.g., Mészáros and El Serafy, 2018). Assessing those inputs requires hydrological approaches, land-use, and eventually, socio-economical models, but to express quantitatively those forcing in a way that is relevant for marine and coastal systems, the transformation occurring in a narrow transition zone between the land and marine domains needs a dedicated attention. More generally, it appears that interfaces between components of the earth system (ocean, atmosphere, land, cryosphere) lack the institutional support that has been built for each of those specific components.
Land forcing improvement requirement is in line with the general trend in operational oceanography, and is identified as one of the key issues by the EuroGOOS CWG. Also, the CMEMS Service Evolution Strategy R&D priorities (Version 3 June 2017) identified land forcing within their medium and long term objectives. An improved and standardized inputs of freshwater and associated river inputs of particulate and dissolved matter and homogenized river forcing approaches in global, regional and coastal models is defined as a mid-term objective. As a longer-term objective, the R&D strategy includes connection and coupling with land hydrology models. Aggregation efforts of near real time river data, adequate for coastal zone modeling, have recently increased with a dedicated section in EMODnet physics. However, neither river climatologies nor watershed models are fully satisfactory (Campuzano et al., 2016) and validated observational error estimates must also be a priority (De Mey-Frémaux et al., 2019).
Finally, while “Improved bathymetry” appears low on the list of priority means to increase the accuracy of the models (see Figure 14), it should be pointed that this option was not initially included in the list of predefined answers to the question “What would be, in your opinion, the three top means to increase the accuracy of your model.” Many authors point to the importance of accurate coastal bathymetry in studies investigating coastal oceanographic phenomena (e.g., Iglesias et al., 2014; Jacob et al., 2016; Arns et al., 2017; Liu and Guillas, 2017; Williams et al., 2017; Serafin et al., 2019). The fact that seven contributors specifically inserted this answer in the “Other” field, support without a doubt that accurate bathymetry is one of the key enablers for the development of accurate numerical models at the coastal scale.
4.4. Need to Revise Parameterization and Process Formulations
As can be noted in Figure 14, revised parameterization and revised process formulations have been identified within the top 6 of the means of improvement. This should not be surprising since these recommendations are directly linked to the increase in spatial resolution that also scored a high ranking. Increase in spatial resolution offers an opportunity to model more explicitly sub-grid processes, which previously had to be parameterized. It also calls for a need to revise process formulations, for example those associated with small scale turbulent mixing, which thanks to increased resolution can now be resolved rather than being parameterized. Clearly, a recommendation for model developers can be drawn for the continuation of their efforts on further development of the models toward improving their capacity to represent coastal phenomena. In fact, recent developments in Europe saw the implementation of non-hydrostatic and non-Boussinesq formulations (Auclair et al., 2018) and revision of the parameterization of subgrid processes for more coastal applications (Holt et al., 2017; Graham et al., 2018).
4.5. Coastal Operational Modeling: Fitness for Purpose?
It is clear that enhancing the social and economic benefits of marine operational modeling requires a focus on the coastal zone. Reaching a strict definition of what requirements a modeling setup should fulfill to claim a “coastal label” is not straightforward and will not be attempted here. However, a number of points of attention can be highlighted from the present survey regarding the fitness of the current European modeling capacity for the purpose of addressing coastal concerns.
The required improvement of near-real time land discharge, nutrient and organic load has already been mentioned in section 4.3, and is particularly acute for the coastal regions.
The same section calls for an improvement of atmospheric forcing in general terms, that can be further specified for the coastal context. Although several works highlight the need for dedicated post-processing for a realistic representation of atmospheric conditions in the vicinity of the coasts, such post-processing is only considered by 10% of contributors. Examples given for such processing includes re-interpolation, masking of land-point data, multiple nested simulations of local atmospheric models, and suppression of land roughness for sea domains.
Section 4.2 describes the need to enforce the delivery chain between near-real time observation and model operators to foster the use of data assimilation. This need is particularly acute for the coastal domain, not covered by standard free-floating autonomous platforms and where many remote-sensing products are challenged (Malthus and Mumby, 2003; Bouffard et al., 2010). Tide Gauge, routine glider missions, HF radars, dedicated space-borne remote sensing algorithms, as well as emerging animal-borne observation programs (e.g., Harcourt et al., 2019; March et al., 2019) can be considered as part of the solution for enhancing the operationality of data assimilation in the coastal domain, but the deployment of affordable observation networks characterizing subsurface physical and biogeochemical characteristics in the coastal domain still deserves dedicated attention.
Finally, the relative influence of the sea floor on the system dynamics increases near the coast. While evident for biogeochemical POIs (e.g., Grall and Chauvaud, 2002; Capet et al., 2016), this statement also concerns sea state and hydrodynamics (Holland and Elmore, 2008). The current survey indicates that dynamical sea floors are rare exceptions in the European modeling landscape. It is likely that, as the focus of operational models narrows on the coastal domain, the inclusion of coupled benthic-pelagic interactions and dynamics representation of seafloor constituents will require dedicated attention.
5. Conclusions and Recommendations
Here, we draw a number of recommendations, and proposed actions, to foster the marine and coastal operational modeling capacity in Europe based on the previous discussions.
1. Although great integration efforts in operational oceanography have been made within ROOSes and EuroGOOS communities, the survey shows clear inhomogeneity in operational modeling capacity. The quality of atmospheric and land forcing and the nature of their origin (i.e., local models, national agencies, …) is quite different from region to region. Some global models are applied at very high resolution while there already exist many high quality coastal models embedding refined parameterization for fine scale processes. Very simple assimilation schemes are still used, while the source codes of better appropriated methods are openly available. Furthermore, there is a lack of common quality standards in European operational ocean modeling community (although this is already the case in CMEMS community), while only 20% of the systems provide dynamic uncertainty estimate. A large diversity of hydrodynamic models and data assimilation schemes reflects a less integrated use of resources. The quality of marine operational modeling should be enhanced at a faster pace by promoting code-sharing, modular architecture and transferability as lead principles in the development of the model core engines, while preserving a diversity of numerical approaches tailored for specific regions or information needs.
Also, above inhomogeneity calls for more integration and adoption of best practices within models and data assimilation systems. An overarching reference could be found, for instance, in the Ocean Best Practices repository (https://www.oceanbestpractices.net/), whose usage could be promoted within operational oceanography networks. Finally, automatic model inter-comparison procedures or multi-model ensemble analyses, to be conducted at regional level (e.g., Barnard et al., 1997), would implicitly promote integration of development efforts delivered by individual institutions. Such multi-model considerations are largely justified by the important geographical overlap between different model domains (Figure 1).
2. An exhaustive coverage of the European coasts with operational models providing information on biogeochemical phenomena remains to be consolidated to enable a proper monitoring of the Marine Strategy Framework Directive (MSFD) and the Water Framework Directive (WFD), and to enable the deployment of economic sectors that could benefit from such operational services. The most restricting aspects regarding this objective lie in the lack of dedicated services for real time land discharge forcing and the lack of near real-time observations to build biogeochemical assimilating systems.
Enforcing collaborations between atmosphere, land, marine and societal research communities still constitutes an important objective to homogenize and enhance the quality of operational products at those domain's interfaces. It appears, however, as particularly acute and urgent to enable proper operational services characterizing coastal and near-shore biogeochemistry. The findings of this survey therefore provide important recommendations for the upcoming Coastal Zone monitoring product (Copernicus Land Monitoring Service, CLMS, 2019) and stress that programs/services addressing land/sea interfaces directly and aiming to build an integrated real-time land forcing service contribute to improve marine operational services as a whole.
Building biogeochemical assimilating systems requires to identify bottlenecks in the provision of near-real time quality-controlled datasets that best constrain the uncertainties of biogeochemical predictions. First, data collection initiatives, such as EMODnet (http://www.emodnet.eu/) and CMEMS INSTAC (e.g., http://www.marineinsitu.eu) may prioritize explicitly near-real time biogeochemical data set, and work out their operational diffusion. It is expected that the fast expansion of the Bio-Argo network (Roemmich et al., 2019) will alleviate part of this issue, at least in the open sea. Second, data acquisition efforts should be tailored to efficiently constrain the major sources of uncertainties for biogeochemical products that are most relevant to users. In this regard, a first step should be to define user requirements in terms of biogeochemical EOVs and to initiate and facilitate the uptake of operational products by offshore and coastal economic actors (e.g., aquaculture, tourism, offshore energy, etc…). To stimulate the downstream use of marine biogeochemical products would help in identifying gaps in terms of operational services, i.e., in the provision of specific EOVs and/or aiming for particular sub-regional model implementations.
3. Data assimilation improves and enforces the service quality through generating more accurate products and dynamical uncertainty assessment. However, data assimilation is only implemented for 23% of the surveyed models, and remains exceptional in biogeochemical systems.
A bottleneck is identified, that the lack of an adequate delivery of observations still restricts a systematic exploitation of data assimilation capacity in operational marine modeling modeling systems. It thus seems that further efforts are required to enable an operational access to those data sets and to addresses the scientific and technical challenge of their inclusion within operational assimilating systems. Beyond remote-sensing and in situ autonomous monitoring program, assimilation techniques should be further applied to promote a more systematic use of other near-real time observations platforms (e.g., HF radars, ferrybox, and tide gauges) via impact studies using Observing System Simulation Experiments (OSSEs) (Oke et al., 2015; She et al., 2017) and/or Observing System Experiments (OSEs) (She, 2018; Fujii et al., 2019; Le Traon et al., 2019). OSE-type approaches are important tools to promote the use of assimilation technique and helps in designing optimized operational forecasting systems. In particular, estuarine and straits dynamics that are largely dominated by tides and their interaction with buoyancy forcing, provide a new challenge to integrating observations and modeling (Pein et al., 2016). It is important, of course, to highlight that routine monitoring programs and in-situ sampling remains essential for model validation and calibration. This is particularly relevant to initiate new model implementations in less covered sub-regions (e.g., southern Mediterranean Sea or Black Sea, cf. Figure 1).
It is also appealing that very basic assimilation methods are still used, while more adapted methods are openly available (see references in Table 2). A factor potentially restricting the uptake of assimilation practices lie in the high-level technical profiles required to operate and implement assimilation systems. Capacity building, through formation or allocation of additional human resources, should probably be envisaged within institutions to deploy the step enhancement of operational services that would stem from a systematic use of data assimilation. In a longer term perspective, a promotion of ocean modeling and data assimilation in early career scientist programs, summer schools, national and European master programs is strongly advised and should be promoted through EU or national funding mechanisms for research and innovation networks.
4. Increasing spatial resolution is perceived as a priority to enhance the accuracy of operational modeling systems. But to increase spatial resolution can not effectively enhance simulation accuracy if this effort is not complemented with improvements of coastline, bathymetry and atmospheric inputs to model setups. For instance, a consensus on best practices in the preparation of external atmospheric forcing for their usage in the coastal domain seems to deserve attention from the European marine modeling community.
In addition, higher spatial resolution and narrower focus on the coastal zone increase the relevance of specific processes that are roughly formulated and parameterized in large scale models. Increasing spatial resolution therefore calls for sustaining research and development activities addressing those processes through dedicated integrated field sampling and model sensitivity analyses.
5. Finally, to monitor the development of European Operational Oceanography and highlights points of attention, large-angle capacity survey, such as proposed here should be pursued, and evaluated against user demands. The overview provided in this manuscript might be affected by the moderate number of participants. In particular, private sectors and systems operating downstream of traditional marine modeling systems appears to be under-represented, as well as some regions and countries. Yet, to our knowledge, it constitutes the most comprehensive operational modeling survey available to date in the European region and has stated a methodology of assessment for the operational modeling capacity which can be used in future surveys. In the perspective to address those gaps and update the present overview by including more operational groups and models already existing in Europe, but which are not represented in the present study, EuroGOOS, through its Coastal Working Group, may envisage a regular update of the current survey every 2–3 years. We invite readers interested to contribute to a next similar assessment or willing to share suggestions in this regard, to contact the corresponding author.
Data Availability Statement
The datasets generated for this study are available on request to the corresponding author.
Author Contributions
AC and VF initiated and constructed the survey, analyzed the answers, and coordinated the manuscript collaborative writing. JSh, TD, GU, JSt, GE, LM, LU, GN, and FC contributed to discussion of the results and building of the concluding recommendations. GE chairs the EuroGOOS Coastal Working Group, of which all co-authors are members. All authors contributed to the drafting and revision of the manuscript.
Funding
AC benefits from a Post-doctoral Researcher grant of the F.R.S.-FNRS. FC was supported by the INTERREG Atlantic Area Project MyCOAST (EAPA 285/2016) co-funded by the ERDF (EU).
Conflict of Interest
The authors declare that the research was conducted in the absence of any commercial or financial relationships that could be construed as a potential conflict of interest.
The reviewer DM declared a past co-authorship with one of the authors GN to the handling editor.
Acknowledgments
We thank Patrick Gorringe (SMHI, Sweden) for providing the authors with a first draft of the model questionnaire on which the survey used in this study was initially based. The authors would also like to thank all people who took their time to answer the model capacity survey and provided valuable inputs. We also thank the two reviewers for their insightful comments that improved the manuscript.
Supplementary Material
The Supplementary Material for this article can be found online at: https://www.frontiersin.org/articles/10.3389/fmars.2020.00129/full#supplementary-material
Data Sheet 1. Form used for the survey.
Figure S1. Regional map - Black Sea.
Figure S2. Regional map - Baltic (BOOS).
Figure S3. Regional map - Iberian Biscay Irish Shelf (IBI).
Figure S4. Regional map - Mediterranean Sea (MONGOOS).
Figure S5. Regional map - North Sea & North-West Atlantic Shelf (NOOS).
Figure S6. Regional map - Arctic ROOS.
Footnotes
References
Arns, A., Dangendorf, S., Jensen, J., Talke, S., Bender, J., and Pattiaratchi, C. (2017). Sea-level rise induced amplification of coastal protection design heights. Sci. Rep. 7:40171. doi: 10.1038/srep40171
Auclair, F., Benshila, R., Debreu, L., Ducousso, N., Dumas, F., Marchesiello, P., et al. (2018). Some Recent Developments around the CROCO Initiative for Complex Regional to Coastal Modeling. Hambourg. 1–47.
Bahurel, P., Adragna, F., Bell, M., Jacq, F., Johannessen, J. A., She, J., et al. (2010). “Ocean monitoring and forecasting core services, the european MyOcean Example,” in Proceedings of OceanObs'09: Sustained Ocean Observations and Information for Society, Vol. 1, eds J. Hall, D. E. Harrison, and D. Stammer (Venice: ESA Publication WPP-306), 21–25. doi: 10.5270/OceanObs09.pp.02
Barnard, S., Barnier, B., Beckmann, A., Böning, C. W., Coulibaly, M., DeCuevas, D., et al. (1997). DYNAMO: Dynamics of North Atlantic Models: Simulation and Assimilation With High Resolution Models. Technical Report, Institute of Oceanography, University of Kiel, Kiel, Germany.
Bell, M., Lefèbvre, M., Le Traon, P.-Y., Smith, N., and Wilmer-Becker, K. (2009). GODAE: the global ocean data assimilation experiment. Oceanography 22, 14–21. doi: 10.5670/oceanog.2009.62
Bouffard, J., Pascual, A., Ruiz, S., Faugère, Y., and Tintoré, J. (2010). Coastal and mesoscale dynamics characterization using altimetry and gliders: a case study in the balearic sea. J. Geophys. Res. 115:79. doi: 10.1029/2009JC006087
Campuzano, F., Brito, D., Juliano, M., Fernandes, R., de Pablo, H., and Neves, R. (2016). Coupling watersheds, estuaries and regional ocean through numerical modelling for western iberia: a novel methodology. Ocean Dyn. 66, 1745–1756. doi: 10.1007/s10236-016-1005-4
Capet, A., Meysman, F. J. R., Akoumianaki, I., Soetaert, K., and Grégoire, M. (2016). Integrating sediment biogeochemistry into 3D oceanic models: a study of benthic-pelagic coupling in the black sea. Ocean Model. 101, 83–100. doi: 10.1016/j.ocemod.2016.03.006
CLMS (2019). Coastal Zone Monitoring—On Land. Available online at: https://land.copernicus.eu/user-corner/technical-library/coastal-zone-monitoring (accessed September 10, 2019).
De Mey-Frémaux, P., Ayoub, N., Barth, A., Brewin, R., Charria, G., Campuzano, F., et al. (2019). Model-observations synergy in the coastal ocean. Front. Mar. Sci. 6:436. doi: 10.3389/fmars.2019.00436
Fujii, Y., Rémy, E., Zuo, H., Oke, P., Halliwell, G., Gasparin, F., et al. (2019). Observing system evaluation based on ocean data assimilation and prediction systems: on-going challenges and a future vision for designing and supporting ocean observational networks. Front. Mar. Sci. 6:417. doi: 10.3389/fmars.2019.00417
Gehlen, M., Barciela, R., Bertino, L., Brasseur, P., Butenschön, M., Chai, F., et al. (2015). Building the capacity for forecasting marine biogeochemistry and ecosystems: recent advances and future developments. J. Oper. Oceanogr. 8, s168–s187. doi: 10.1080/1755876X.2015.1022350
Graham, J. A., O'Dea, E., Holt, J., Polton, J., Hewitt, H. T., Furner, R., et al. (2018). Amm15: a new high-resolution nemo configuration for operational simulation of the european north/west shelf. Geosci. Model Dev. 11, 681–696. doi: 10.5194/gmd-11-681-2018
Grall, J., and Chauvaud, L. (2002). Marine eutrophication and benthos: the need for new approaches and concepts. Glob. Change Biol. 8, 813–830. doi: 10.1046/j.1365-2486.2002.00519.x
Harcourt, R., Sequeira, A. M. M., Zhang, X., Roquet, F., Komatsu, K., Heupel, M., et al. (2019). Animal-Borne telemetry: an integral component of the ocean observing toolkit. Front. Mar. Sci. 6:326. doi: 10.3389/fmars.2019.00326
Holland, K. T., and Elmore, P. A. (2008). A review of heterogeneous sediments in coastal environments. Earth Sci. Rev. 89, 116–134. doi: 10.1016/j.earscirev.2008.03.003
Holt, J., Hyder, P., Ashworth, M., Harle, J., Hewitt, H. T., Liu, H., et al. (2017). Prospects for improving the representation of coastal and shelf seas in global ocean models. Geosci. Model Dev. 10, 499–523. doi: 10.5194/gmd-10-499-2017
Iglesias, O., Lastras, G., Souto, C., Costa, S., and Canals, M. (2014). Effects of coastal submarine canyons on tsunami propagation and impact. Mar. Geol. 350, 39–51. doi: 10.1016/j.margeo.2014.01.013
Jacob, B., Stanev, E., and Zhang, Y. (2016). Local and remote response of the north sea dynamics to morphodynamic changes in the wadden sea. Ocean Dyn. 66, 671–690. doi: 10.1007/s10236-016-0949-8
Janeković, I., Mihanović, H., Vilibić, I., and Tudor, M. (2014). Extreme cooling and dense water formation estimates in open and coastal regions of the adriatic sea during the winter of 2012. J. Geophys. Res. C Oceans 119, 3200–3218. doi: 10.1002/2014JC009865
Kara, A. B., Hurlburt, H. E., and Wallcraft, A. J. (2005). Black sea mixed layer sensitivity to various wind and thermal forcing products on climatological time scales. J. Clim. 18, 5266–5293. doi: 10.1175/JCLI3573R2.1
Kourafalou, V., Mey, P. D., Hénaff, M. L., Charria, G., Edwards, C., He, R., et al. (2015a). Coastal ocean forecasting: system integration and evaluation. J. Oper. Oceanogr. 8, 137–146. doi: 10.1080/1755876X.2015.1022336
Kourafalou, V., Mey, P. D., Staneva, J., Ayoub, N., Barth, A., Chao, Y., et al. (2015b). Coastal ocean forecasting: science foundation and user benefits. J. Oper. Oceanogr. 8, s147–s167. doi: 10.1080/1755876X.2015.1022348
Le Traon, P.-Y., Reppucci, A., Alvarez Fanjul, E., Aouf, L., Behrens, A., Belmonte, M., et al. (2019). From observation to information and users: the copernicus marine service perspective. Front. Mar. Sci. 6:234. doi: 10.3389/fmars.2019.00234
Lindstrom, E., Gunn, J., Fischer, A., McCurdy, A., and Glover, L. K. Members of Task Team for an Integrated Framework for Sustained Ocean Observing (2012). A Framework for Ocean Observing. Technical report, IOC.
Lindström, G., Pers, C., Rosberg, J., Strömqvist, J., and Arheimer, B. (2010). Development and testing of the HYPE (hydrological predictions for the environment) water quality model for different spatial scales. Hydrol. Res. 41, 295–319. doi: 10.2166/nh.2010.007
Liu, X., and Guillas, S. (2017). Dimension reduction for gaussian process emulation: an application to the influence of bathymetry on tsunami heights. SIAM/ASA J. Uncertain. Quantif. 5, 787–812. doi: 10.1137/16M1090648
Liu, Y., Kerkering, H., and Weisberg, R. H., (eds.). (2015). “Chapter 1–introduction to coastal ocean observing systems,” in Coastal Ocean Observing Systems, (Boston, MA: Academic Press), 1–10.
Ludwig, W., Dumont, E., Meybeck, M., and Heussner, S. (2009). River discharges of water and nutrients to the Mediterranean and Black sea: major drivers for ecosystem changes during past and future decades? Prog. Oceanogr. 80, 199–217. doi: 10.1016/j.pocean.2009.02.001
Malone, T. C. (2003). The coastal module of the global ocean observing system (GOOS): an assessment of current capabilities to detect change. Mar. Policy 27, 295–302. doi: 10.1016/S0308-597X(03)00043-5
Malone, T. C., and Cole, M. (2000). Toward a global scale coastal ocean observing system. Oceanography 13, 7–11. doi: 10.5670/oceanog.2000.48
Malthus, T. J., and Mumby, P. J. (2003). Remote sensing of the coastal zone: an overview and priorities for future research. Int. J. Remote Sens. 24, 2805–2815. doi: 10.1080/0143116031000066954
March, D., Boehme, L., Tintoré, J., Vélez-Belchi, P. J., and Godley, B. J. (2019). Towards the integration of animal-borne instruments into global ocean observing systems. Glob. Change Biol. 26, 586–596. doi: 10.1111/gcb.14902
Mercator Ocean STAC (2016). CMEMS High Level Service Evolution Strategy. Technical report, Mercator Ocean.
Mészáros, L., and El Serafy, G. (2018). Setting up a water quality ensemble forecast for coastal ecosystems: a case study of the southern North Sea. J. Hydroinform. 20, 846–863. doi: 10.2166/hydro.2018.027
Oke, P. R., Larnicol, G., Jones, E. M., Kourafalou, V., Sperrevik, A. K., Carse, F., et al. (2015). Assessing the impact of observations on ocean forecasts and reanalyses: part 2, regional applications. J. Oper. Oceanogr. 8, s63–s79. doi: 10.1080/1755876X.2015.1022080
Pein, J. U., Grayek, S., Schulz-Stellenfleth, J., and Stanev, E. V. (2016). On the impact of salinity observations on state estimates in EMS estuary. Ocean Dyn. 66, 243–262. doi: 10.1007/s10236-015-0920-0
Roemmich, D., Alford, M. H., Claustre, H., Johnson, K., King, B., Moum, J., et al. (2019). On the future of argo: a global, full-depth, multi-disciplinary array. Front. Mar. Sci. 6:439. doi: 10.3389/fmars.2019.00439
Schiller, A., Mourre, B., Drillet, Y., and Brassington, G. (2018). “An overview of operational oceanography,” in New Frontiers in Operational Oceanography, eds E. Chassignet, A. Pascual, J. Tintoré, and J. Verron (GODAE Ocean View), 1–26. doi: 10.17125/gov2018.ch01
Serafin, K. A., Ruggiero, P., Barnard, P. L., and Stockdon, H. F. (2019). The influence of shelf bathymetry and beach topography on extreme total water levels: linking large-scale changes of the wave climate to local coastal hazards. Coast. Eng. 150, 1–17. doi: 10.1016/j.coastaleng.2019.03.012
She, J. (2018). “Assessment of baltic sea observations for operational oceanography,” in Proceedings of EuroGOOS 8th Conference, eds E. Buch, V. Fernández, D. Eparkhina, P. Gorringe, and G. Nolan (Bergen), 79–87.
She, J., Allen, I., Buch, E., Crise, A., Johannessen, J. A., Le Traon, P.-Y., et al. (2016). Developing european operational oceanography for blue growth, climate change adaptation and mitigation, and ecosystem-based management. Ocean Sci. 12, 953–976. doi: 10.5194/os-12-953-2016
She, J., Buch, E., and Nolan, G. (2017). Report on Lessons Learned From OSSE Experiments in Support of the Definition of Requirements to an in-situ Observing System. Technical Report report D2.4.1, EuroGOOS-CMEMS.
van Apeldoorn, D., and Bouwman, L. (2014). D4.6: SES Land-Based Runoff and Nutrient Load Data (1980–2000). Technical report, Policy-Oriented Marine Environmental Research in the Southern EUropean Seas FP7 Project.
Williams, C. N., Cornford, S. L., Jordan, T. M., Dowdeswell, J. A., Siegert, M. J., Clark, C. D., et al. (2017). Generating synthetic fjord bathymetry for coastal greenland. Cryosphere 11, 363–380. doi: 10.5194/tc-11-363-2017
Zhu, J. (2011). “Overview of regional and coastal systems,” in Operational Oceanography in the 21st Century, eds A. Schiller and G. B. Brassington (Dordrecht: Springer Netherlands), 413–439.
Appendix
In order to promote integration in the European Marine Operational Modeling community, and to acknowledge actors of this community having contributed to the present survey, we provide here the lists of:
• Institutions and organizations having contributed to the survey (Table 1).
• Modeling softwares used within the implementations reported to the present survey. Given references provide access to user manuals, community websites or reference publications (Table 2).
• National and trans-national agencies providing local atmospheric forcing services and their usage in the different regions (Table 3).
• National and trans-national agencies providing local land discharge forcing services and their usage in the different regions (Table 4).
Keywords: operational oceanography, ocean modeling, ocean observations, coastal observations, marine services, coastal modeling, users requirements
Citation: Capet A, Fernández V, She J, Dabrowski T, Umgiesser G, Staneva J, Mészáros L, Campuzano F, Ursella L, Nolan G and El Serafy G (2020) Operational Modeling Capacity in European Seas—An EuroGOOS Perspective and Recommendations for Improvement. Front. Mar. Sci. 7:129. doi: 10.3389/fmars.2020.00129
Received: 28 October 2019; Accepted: 17 February 2019;
Published: 03 March 2020.
Edited by:
Anna Milena Zivian, Ocean Conservancy, United StatesReviewed by:
Yoshikazu Sasai, Japan Agency for Marine-Earth Science and Technology, JapanDavid March, Centre for Ecology and Conservation, University of Exeter, United Kingdom
Copyright © 2020 Capet, Fernández, She, Dabrowski, Umgiesser, Staneva, Mészáros, Campuzano, Ursella, Nolan and El Serafy. This is an open-access article distributed under the terms of the Creative Commons Attribution License (CC BY). The use, distribution or reproduction in other forums is permitted, provided the original author(s) and the copyright owner(s) are credited and that the original publication in this journal is cited, in accordance with accepted academic practice. No use, distribution or reproduction is permitted which does not comply with these terms.
*Correspondence: Vicente Fernández, vicente.fernandez@eurogoos.eu