- 1Coastal Research and Extension Center, Mississippi State University, Biloxi, MS, United States
- 2Mississippi-Alabama Sea Grant, Ocean Springs, MS, United States
- 3Hopkins Marine Station, Stanford University, Pacific Grove, CA, United States
- 4Gulf of Mexico Fishery Management Council, Tampa, FL, United States
- 5National Marine Fisheries Service, Southeast Fisheries Science Center, Riverside Technology, Inc., Panama City Beach, FL, United States
- 6Alabama Department of Conservation and Natural Resources, Marine Resources Division, Dauphin Island, AL, United States
- 7Department of Marine Sciences, University of South Alabama, Mobile, AL, United States
Understanding the factors that influence species’ distributions is crucial for implementing effective management and conservation practices, yet difficult for highly vagile species like sharks. Many shark species demonstrate either spatial and/or temporal sexual segregation, further confounding accurate quantification of habitat suitability. Given the importance of understanding spatiotemporal patterns in the distribution of coastal shark assemblages, we sought to quantify sex-specific abiotic factors that influence seasonal variation in a coastal shark assemblage using data from a long-term fisheries-independent bottom longline program in the northern Gulf of Mexico. Catch data (individuals/100 hooks/hour) were coupled with a suite of potentially predictive variables: surface and bottom values for temperature (°C) and salinity (psu), sea surface height (m), three-dimensional surface and bottom current velocity (u, v, w, in m/s), bottom dissolved oxygen (mg/l), depth (m), substrate grain size (mm), daylength (min), and distance from shore (km). Data were analyzed using boosted regression trees (BRT) to describe the relationships between catch data and environmental factors potentially influencing sex-specific species distribution and abundance. Between May 2006 and November 2018, we conducted 1,226 bottom longline sets and caught 13,742 individuals encompassing 67 species. The majority of the animals captured (74%) were elasmobranchs, primarily sharks. Two species from each of the following three categories were selected for further analyses: small coastal sharks (Atlantic sharpnose shark Rhizoprionodon terraenovae and blacknose shark Carcharhinus acronotus), large coastal sharks (blacktip shark C. limbatus and sandbar shark C. plumbeus), and shelf-associated sharks (smoothhound sharks, Mustelus spp. and scalloped hammerhead Sphyrna lewini). Depth and distance from shore were the strongest predictors of distribution and relative abundance, followed by longitude and bottom salinity; other factors (e.g., temperature, daylength, substrate grain size) were less predictive. For the six species examined, predictive factors were often the same for males and females, although the range of preferred values varied. Surprisingly, the importance of these predictors varied little across seasons. Collectively, our findings demonstrate that sexual segregation is the norm for sharks in the north-central Gulf of Mexico. Long-term fishery-independent monitoring to further quantify these sex-based differences in habitat use should be prioritized, particularly in light of impending climate change.
Introduction
Understanding species distributions is central to realizing their ecological roles, fundamental for developing effective conservation and management measures, and increasingly necessary in the face of imminent climate change. Examining the mechanisms underpinning species distributions can help identify critical habitats and aid in the development of habitat suitability models. For some species, habitat associations shift ontogenetically and may be sex-specific; for these species, collecting data to accurately characterize spatially and temporally shifting distributions presents a challenge, particularly given changing environmental conditions.
Sharks are highly mobile species whose distributions can be affected by a number of abiotic and environmental variables. Abiotic factors influencing shark distributions are complex, interactive, species-specific, and geographically variable (Schlaff et al., 2014). Perhaps the most common factor influencing the distribution of sharks is temperature. For example, the timing of massive seasonal migrations of blacktip shark (Carcharhinus limbatus) along the southeast coast of Florida is best predicted by water temperature, where large aggregations are only seen when water temperatures are below 25°C (Kajiura and Tellman, 2016). Similarly, residency for migratory adult lemon sharks (Negaprion brevirostris) in southeast Florida coincides with water temperatures less than 24°C (Kessel et al., 2014). While the influence of temperature, as well as salinity (e.g., Ubeda et al., 2009), dissolved oxygen (e.g., Heithaus et al., 2009), and interactions thereof (e.g., Drymon et al., 2014) are well known, other abiotic factors such as daylength (Grubbs et al., 2005) and distance from shore (Haig et al., 2018) may be important, and warrant further consideration.
Characterizing shark distributions is further complicated by tendencies toward sex-specific segregation (Springer, 1967; Sims, 2005; Wearmouth and Sims, 2008). A common type of sex-specific segregation is geographical segregation (Backus et al., 1956), defined as differences in habitat selection between sexes. This type of segregation can vary temporally; for example, females of many shark species move into discrete areas specifically for parturition (e.g., Feldheim et al., 2002). For other species, female-only aggregations have been recorded in shallow lagoons where water temperatures are 1–2°C warmer than surrounding areas (Economakis and Lobel, 1998). According to the thermal-niche hypothesis (Sims, 2005), thermal advantages, including shorter gestation and/or larger size at birth, may be conferred to pregnant females during these periods (Jirik and Lowe, 2012). In some shark fisheries, sexual segregation may lead to disproportionate removals of males or females. This has been shown for male shortfin mako (Isurus oxyrinchus) in the South Pacific Ocean (Mucientes et al., 2009) and female spiny dogfish (Squalus acanthias) in the northwest Atlantic Ocean (Rago and Sosebee, 2010). For these species, understanding the factors determining sex-specific distributions is useful for reducing harvest pressure on the over-exploited portion of the population (Dell’Apa et al., 2017; Haugen et al., 2017).
The northern Gulf of Mexico (GoM) supports a diverse assemblage of coastal sharks, and while the environmental factors underpinning the distribution within this assemblage are well-described (e.g., Drymon et al., 2013), a detailed understanding of seasonal and sex-specific drivers within this assemblage is lacking. For example, the interactive effects of temperature, salinity, and proximity to tidal inlets influence habitat use for juvenile bull (Carcharhinus leucas), blacktip, and bonnethead (Sphyrna tiburo) sharks in coastal Texas (Froeschke et al., 2010; Plumlee et al., 2018). Similarly, temperature, depth, and salinity predict habitat use for juvenile blacktip, bonnethead, finetooth (C. isodon), spinner (C. brevipinna), scalloped hammerhead (S. lewini), and Atlantic sharpnose (Rhizoprionodon terraenovae) sharks in bay systems in north Florida (Ward-Paige et al., 2014). While these studies characterize estuarine habitat use, many coastal sharks in the GoM demonstrate across-shelf movements from coastal estuaries to offshore waters. Quantifying inshore and offshore habitat use and how that may differ between males and females, is therefore a critical data gap. Given the importance of understanding spatiotemporal patterns in the distribution of coastal shark assemblages, as well as the influential mechanisms, the goals of the current study are to (1) measure seasonal variation in the northern GoM coastal shark assemblage, specifically movements into deeper water, and (2) quantify sex-specific abiotic factors that influence seasonal variation in the coastal shark assemblage.
Materials and Methods
Bottom Longline Survey
Catch data were collected as part of inshore (Drymon et al., 2010) and offshore (Powers et al., 2018) fisheries-independent bottom longline surveys conducted off the coasts of Louisiana, Mississippi and Alabama (Figure 1). For both surveys, bottom longline locations were selected using a stratified-random sampling design. Bottom longline sampling followed standardized methods described in Drymon et al. (2013). The main line consisted of 1.85 km (1 nmi) of 4 mm monofilament (545 kg test) that was set with 100 gangions. Gangions consisted of a longline snap and a 15/0 circle hook baited with Atlantic mackerel (Scomber scombrus). Each gangion was made of 3.66 m of 3 mm monofilament (320 kg test). All sets were soaked for 1 h. Once the bottom longline was retrieved, all sharks that could be boated safely were removed from the main line, unhooked, and identified to species following Castro (2010). For each individual, length (precaudal, fork, and stretch in cm), weight (in kg), sex and maturity stage (when possible) were recorded. Maturity in males was assessed following Clark and Von Schmidt (1965). Sharks were tagged either on the first dorsal fin with a plastic Rototag (Premier 1) or just below the first dorsal fin with a metal dart tag. The tag type and location depended both on species and size of shark at capture (Kohler and Turner, 2001). Catch data were converted to catch per unit effort (CPUE), expressed as individuals 100 hooks–1 hour–1.
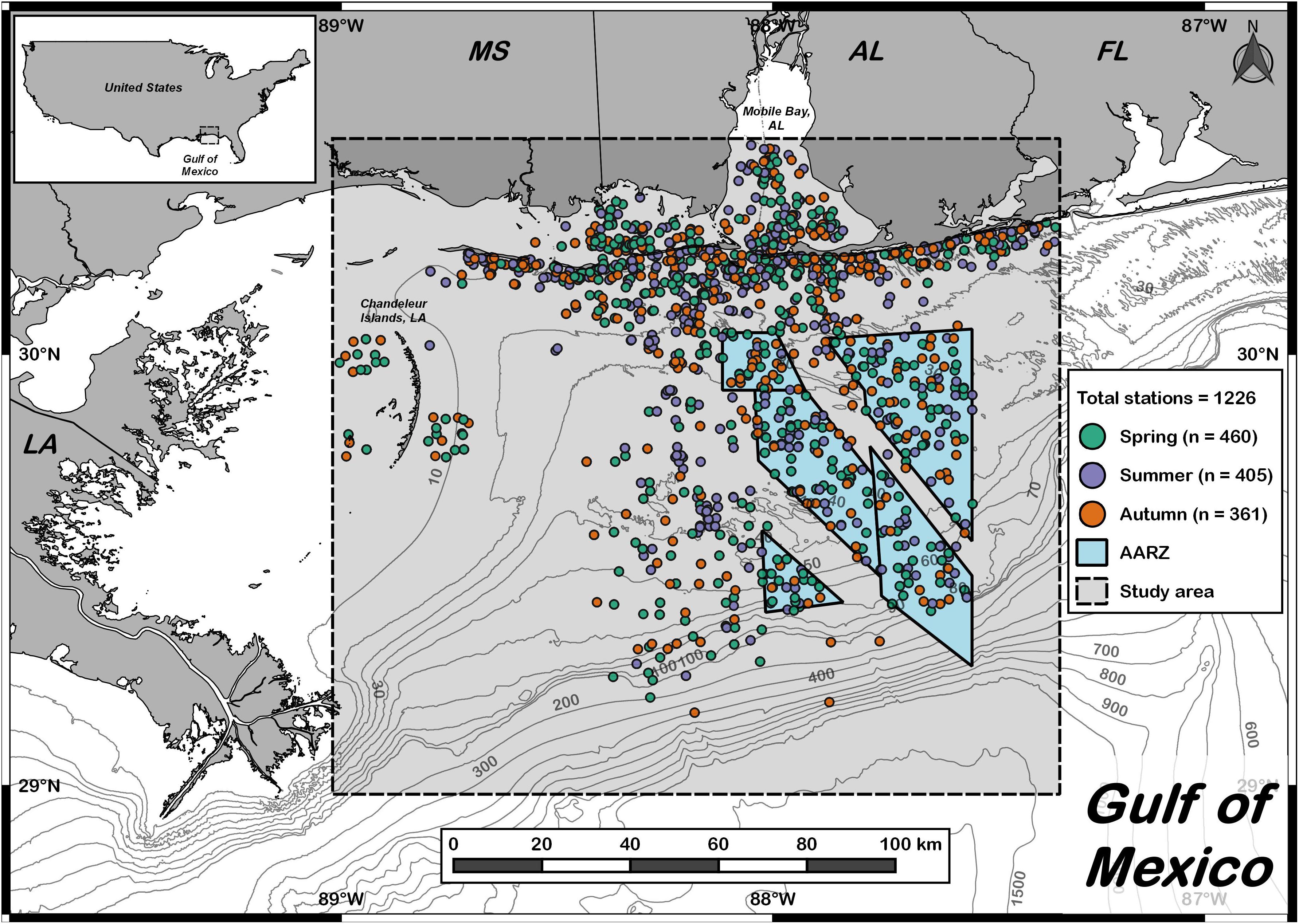
Figure 1. Study area in the northern Gulf of Mexico. Dashed line denotes the extent of the bottom longline study area. Each dot denotes an individual set made between 2006 and 2018 (n = 1226). Spring sets are shown in green (n = 460), summer sets are shown in purple (n = 405), and autumn sets are shown in orange (n = 361). Isobaths (m) indicate depth. The blue region bounded by the solid line denotes the Alabama Artificial Reef Zone (AARZ).
Environmental Data
Multiple environmental variables were examined to explain the distribution of species captured on the bottom longline during the course of this study. Temperature and salinity were explored because they have been shown to commonly influence shark distributions (Schlaff et al., 2014), particularly for coastal species (e.g., blacktip shark). Sea surface height and current velocities were examined because they are oceanographic predictors that may aid in predicting habitat suitability for species that occur primarily offshore (e.g., scalloped hammerhead). Since the species assemblage in this region included both coastal and offshore species, the variables depth and distance from shore were also considered as potential predictors. Given the distribution of sampling effort across multiple seasons (i.e., variable photoperiods), daylength was also examined as a potential predictive variable following Grubbs et al. (2005). Finally, dissolved oxygen and substrate grain size were explored because these variables are potentially relevant to this study area given episodic, large-scale hypoxia (Rabalais et al., 2002) and transitions in sediment type (Balsam and Beeson, 2003).
Data for these environmental variables were obtained from multiple sources. Surface and bottom temperature (°C), surface and bottom salinity (psu), sea surface height (m), and three-dimensional surface and bottom current velocity (u, v, w in m s–1) were obtained from the Hybrid Coordinate Ocean Model (HYCOM) data server (4 km resolution). Bottom dissolved oxygen (mg l–1) was obtained from the National Oceanic and Atmospheric Administration (NOAA)1, and interpolated across ∼100 to 250 survey stations (number varied by year). Depth (m) and substrate grain size (mm) were obtained from the United States Geological Survey (USGS)2, 0.33 arc seconds, ∼10 m resolution). Daylength (min) was calculated in R (R Core Team, 2013) using code by SD3; distance from shore (km) was calculated in QGIS (Quantum GIS Development Team, 2019).
Habitat Modeling
Boosted regression trees (BRTs) were used to describe the relationships between sex-specific CPUE from the bottom longline, and environmental variables potentially influencing species’ distribution and abundance. Specifically, BRTs were fit for males and females of each species for each of three seasons (meteorological spring, summer, and autumn) resulting in six unique combinations per species. Boosted regression trees are a machine learning technique capable of fitting complex, non-linear relationships that offer predictive advantages over generalized linear or additive models (GLMs and GAMs). In particular, BRTs are relatively insensitive to multicollinearity (Abeare, 2009; Dedman et al., 2015, 2017), especially in situations where outputs from the BRT are used to predict within the range of sampled data (Dormann et al., 2013). A thorough overview of BRTs is given in Elith et al. (2008).
Preliminary analyses indicated that catch data were zero-inflated (a high proportion of zero values). Zero-inflated catch data are characteristic for many sharks that naturally occur in relatively low abundance (i.e., true zeros, Martin et al., 2005). A detailed treatment of modeling zero-inflated data is given by Maunder and Punt (2004). To properly accommodate the preponderance of zeros, a 2-step (i.e., delta or hurdle) process was chosen to model catch data. Presence/absence probability was modeled using a BRT with a binary distribution and continuous, non-zero (i.e., abundance) probability was modeled using a BRT with a Gaussian distribution. Since the catch data were also long-tailed (some instances of anomalously high catch), non-zero data were natural-log-transformed. Predictions were reverse log-transformed; the final model is a product of the binary and Gaussian BRTs (Lo et al., 1992).
Sixteen variables were considered for the BRT models (Table 1) and all models were fit using the package gbm.auto (Dedman et al., 2017) in R. Learning rate (lr), bag fraction (bf) and tree contribution (tc) are parameters that are used in concert to achieve minimum predictive error (Elith et al., 2008). These were optimized using gbm.auto for each species/season/sex model run.
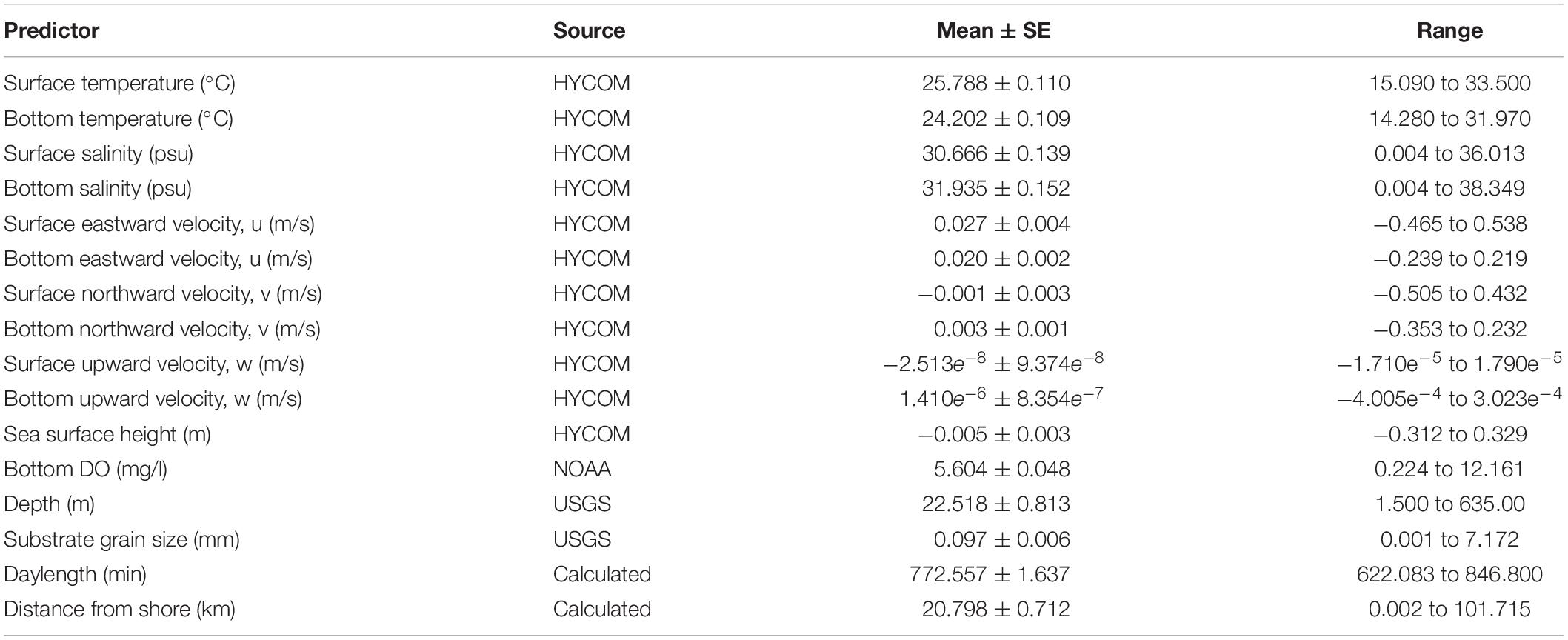
Table 1. Mean values and range for potential predictor variables included in the boosted regression trees.
Model Performance and Interpretation
The BRT modeling approach automatically partitioned the data into training and testing sets, with the ratio of these dictated by the bag fraction. Ten-fold cross-validation (CV) was then performed, with the members of the training/testing sets randomized each time. Performance metrics captured were training/testing correlation, CV deviance (and standard error (SE)) and correlation (and SE), as well as Area Under Receiver-Operator-Curve (AUC) and its CV and CV SE for the binary models (Parisien and Moritz, 2009). Final fitted functions from the binary BRT were visualized using marginal effect plots to show the effect of a variable on the response after accounting for the average effects of other model variables (Elith et al., 2008).
Habitat Suitability
The distribution of suitable habitat was predicted using the BRTs described above. Environmental data for model predictions were obtained in the same manner as above, except that HYCOM data were extracted for one representative date per season (monthly groupings per season, i.e., DJF/MAM/JJA/SON) at their native resolution of 4 km. Representative dates for environmental data were selected by ranking the absolute value of the differences of all sites’ values for all variables against the mean for those variables, then selecting a single date within each season that most closely matched those values. The BRT models later generated predictive CPUE values for each 2 km × 2 km cell, which were then mapped in QGIS (Quantum GIS Development Team, 2019) using the heatmap setting. The heatmap setting produces a color point weighted by the predicted abundance generated from the BRT. The radius of each point was set at the default of 10 mm. Using gbm.auto, we also calculated the coefficient of variation for the predicted abundance values at each 2 km × 2 km cell to represent model error. Given the large differences in predicted abundance between species, the predicted abundance values and the coefficients of variation are not evenly scaled across all seasons, sexes, and species.
Results
Bottom Longline Survey
Between May 2006 and November 2018, we conducted 1,226 bottom longline sets and caught 13,742 individuals across 67 species: 43 species of teleost, 23 species of elasmobranch, and 1 invertebrate (Supplementary Table S1). Survey effort was relatively well distributed across the three seasons sampled: spring (n = 460), summer (n = 405) and autumn (n = 361) (Figure 1). The majority of the individuals captured (74%) were elasmobranchs, primarily sharks. Atlantic sharpnose shark (n = 4,815) was the most common species, followed by blacknose shark (n = 1,532). Together, these two small coastal species represented nearly half of all individuals sampled (46%). The two most abundant large coastal sharks were blacktip shark (n = 1,207) and sandbar shark (C. plumbeus, n = 569). The two most abundant shelf-associated sharks were scalloped hammerhead (n = 209) and smoothhound sharks (Mustelus spp., n = 176). Collectively, individuals of both sexes for these six species occurred during all seasons sampled and were therefore selected for further analyses. Given the difficulty in distinguishing between three morphologically conserved species of Mustelus commonly found in the GoM (smooth dogfish M. canis, Florida smoothhound M. norrisi, and Gulf smoothhound M. sinusmexicanus, Giresi et al., 2015), NOAA Fisheries currently manages all individuals in the genus Mustelus in the GoM as a complex (SEDAR, 2015); therefore, all Mustelus will hereafter be aggregately referred to as “smoothhound sharks.”
Model Performance
Model performance was assessed for the two small coastal sharks, the two large coastal sharks, and the two shelf-associated sharks for each sex/season combination (n = 36 model configurations, Table 2). Training data AUC scores ranged from very good (0.84 for Atlantic sharpnose shark, both sexes for all seasons) to excellent (0.99 for smoothhound sharks, both sexes for all seasons) according to criteria defined in Lane et al. (2009). Cross-validated AUC scores were only slightly lower than training data scores, indicating that model overfitting was negligible (Hijmans and Elith, 2013). For most species/sex/season combinations, distributions were modeled using Gaussian BRTs; for female scalloped hammerheads and male smoothhound sharks, binary BRTs were used, as they had relatively fewer data available.
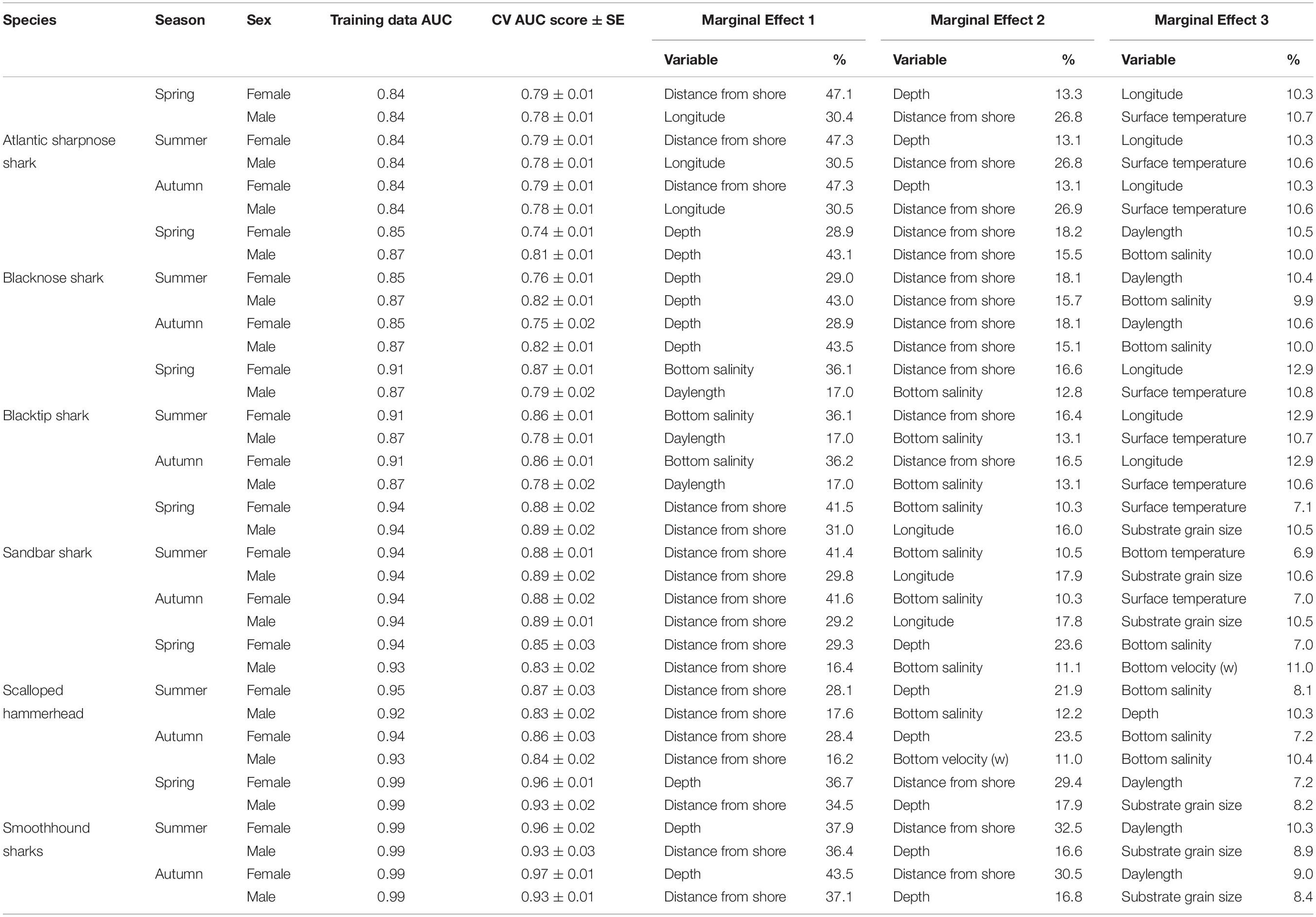
Table 2. Percent contribution of the three most influential factors identified by the boosted regression trees for Atlantic sharpnose shark, blacknose shark, blacktip shark, sandbar shark, scalloped hammerhead, and smoothhound sharks.
Small Coastal Sharks
Atlantic sharpnose sharks of both sexes were encountered across a range of similar sizes (Figure 2A) and were captured across the extent of the study area (Figure 3A). Females were more common than males (1.1:1), which differed from the expected sex ratio (x2 = 12.95, p < 0.01). Final models for Atlantic sharpnose shark season/sex combinations had training data AUC scores of 0.84, and cross validation AUC scores ranging from 0.78 (±0.01) to 0.79 (±0.01) (Table 2). For females, distance from shore (47%), depth (13%), and longitude (10%) were the three most influential predictors of distribution, and this was consistent across seasons (Table 2). Female Atlantic sharpnose sharks were consistently distributed greater than 30 km offshore, in depths ranging from 20 to 80 m, particularly west of 88°W longitude (Figure 4A). These trends were consistent across seasons. Wide expanses of suitable habitat were predicted for female Atlantic sharpnose shark, predominantly west of 88°W. Subtle seasonal shifts in predicted habitat suitability were seen, primarily in deeper waters (Figure 5A). Coefficients of variation were highest in the deepest portion of the study region (Supplementary Figure S1A).
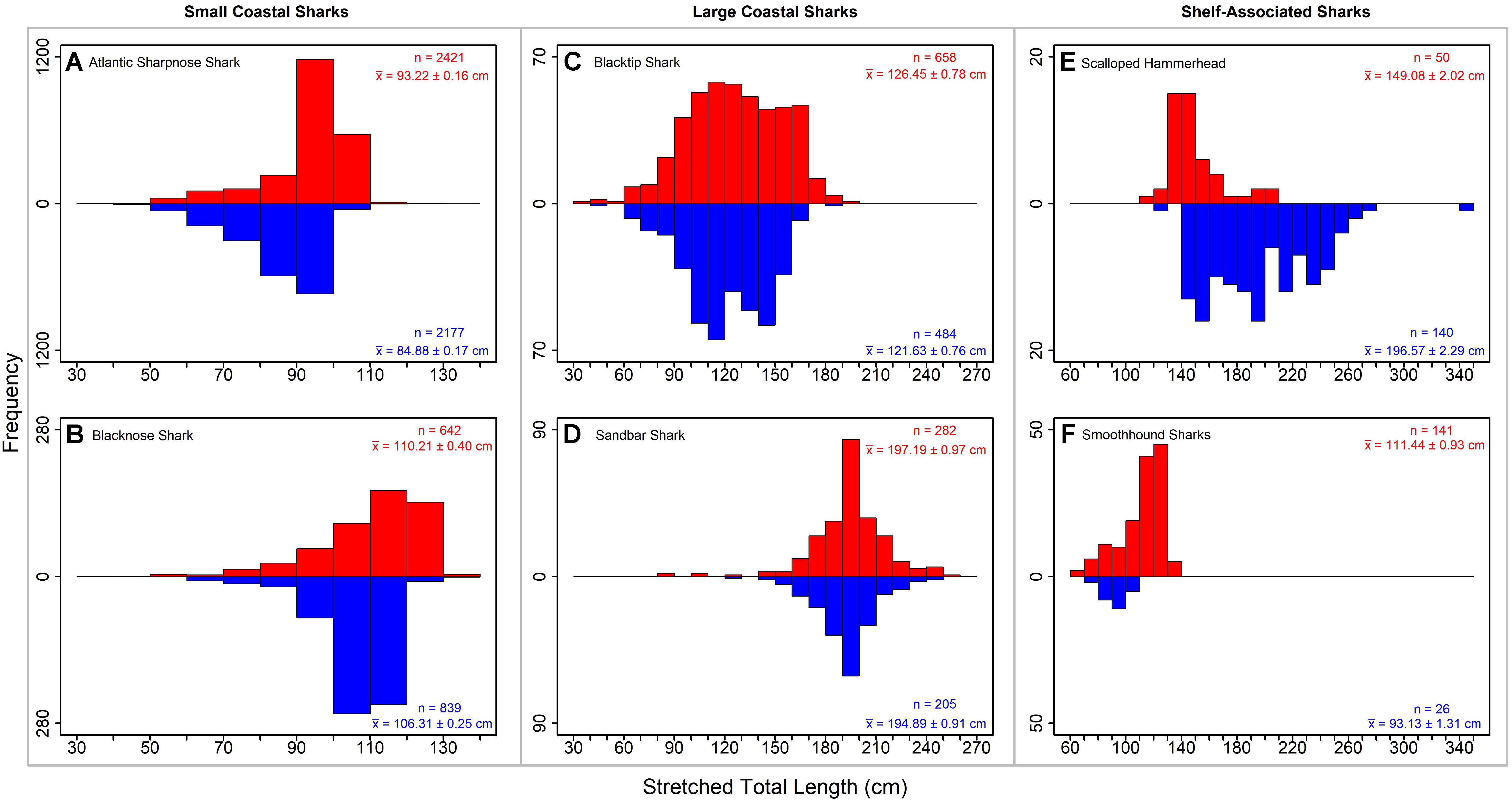
Figure 2. Length frequency plots (stretched total length in cm) for representative small coastal sharks (A) Atlantic sharpnose shark and (B) blacknose shark, large coastal sharks (C) blacktip shark and (D) sandbar shark, and shelf-associated sharks (E) scalloped hammerhead and (F) smoothhound sharks. Males are shown in blue and females are shown in red. Sample size (n) and mean (X̄ ± SE) are shown.
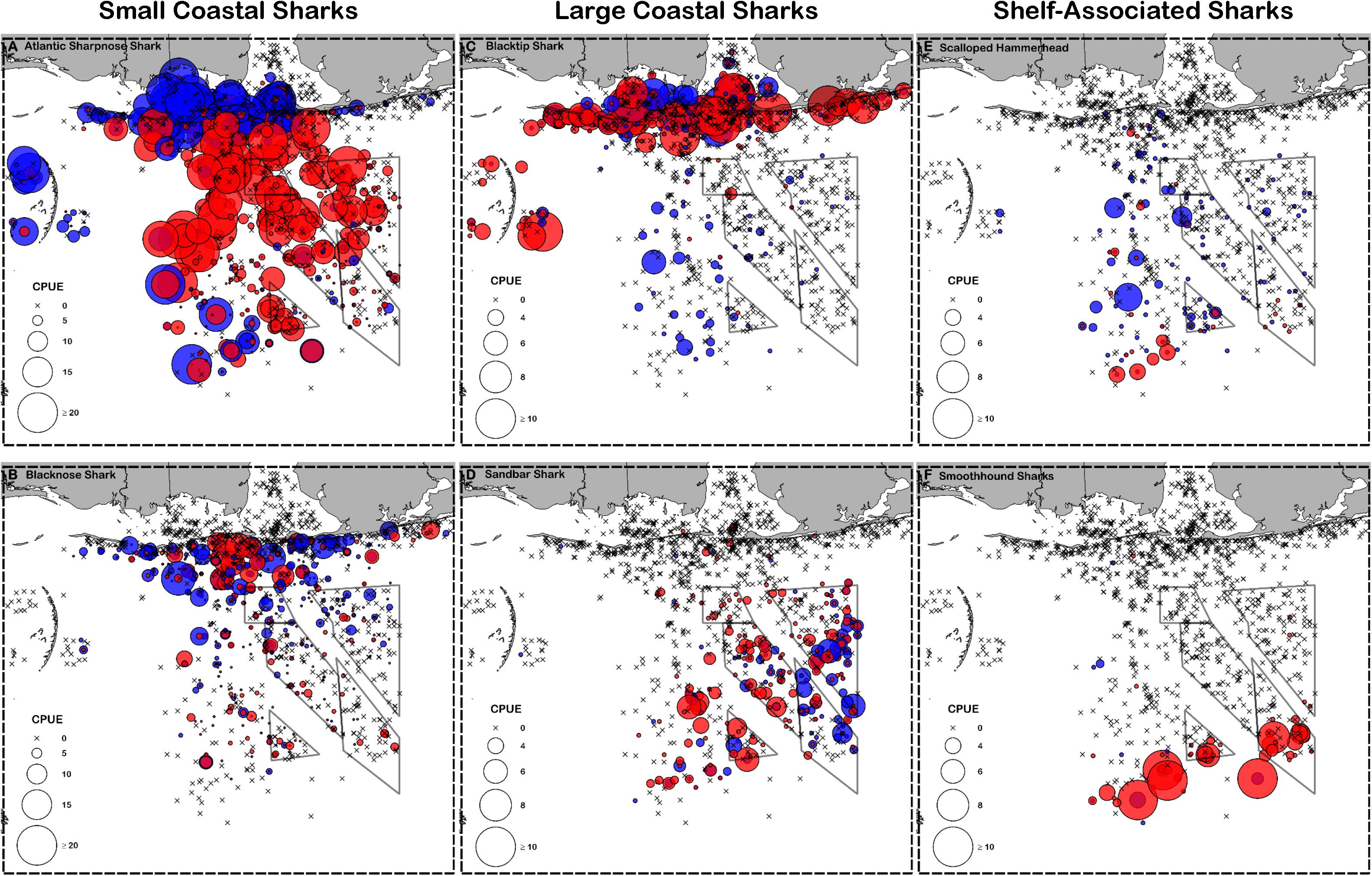
Figure 3. Catch per unit effort (CPUE, individuals 100 hooks–1 hour–1) for representative small coastal sharks (A) Atlantic sharpnose shark and (B) blacknose shark, large coastal sharks (C) blacktip shark and (D) sandbar shark, and shelf-associated sharks (E) scalloped hammerhead and (F) smoothhound sharks. Males are shown in blue and females are shown in red. Note that small coastal sharks are scaled differently than large coastal and shelf-associated sharks.
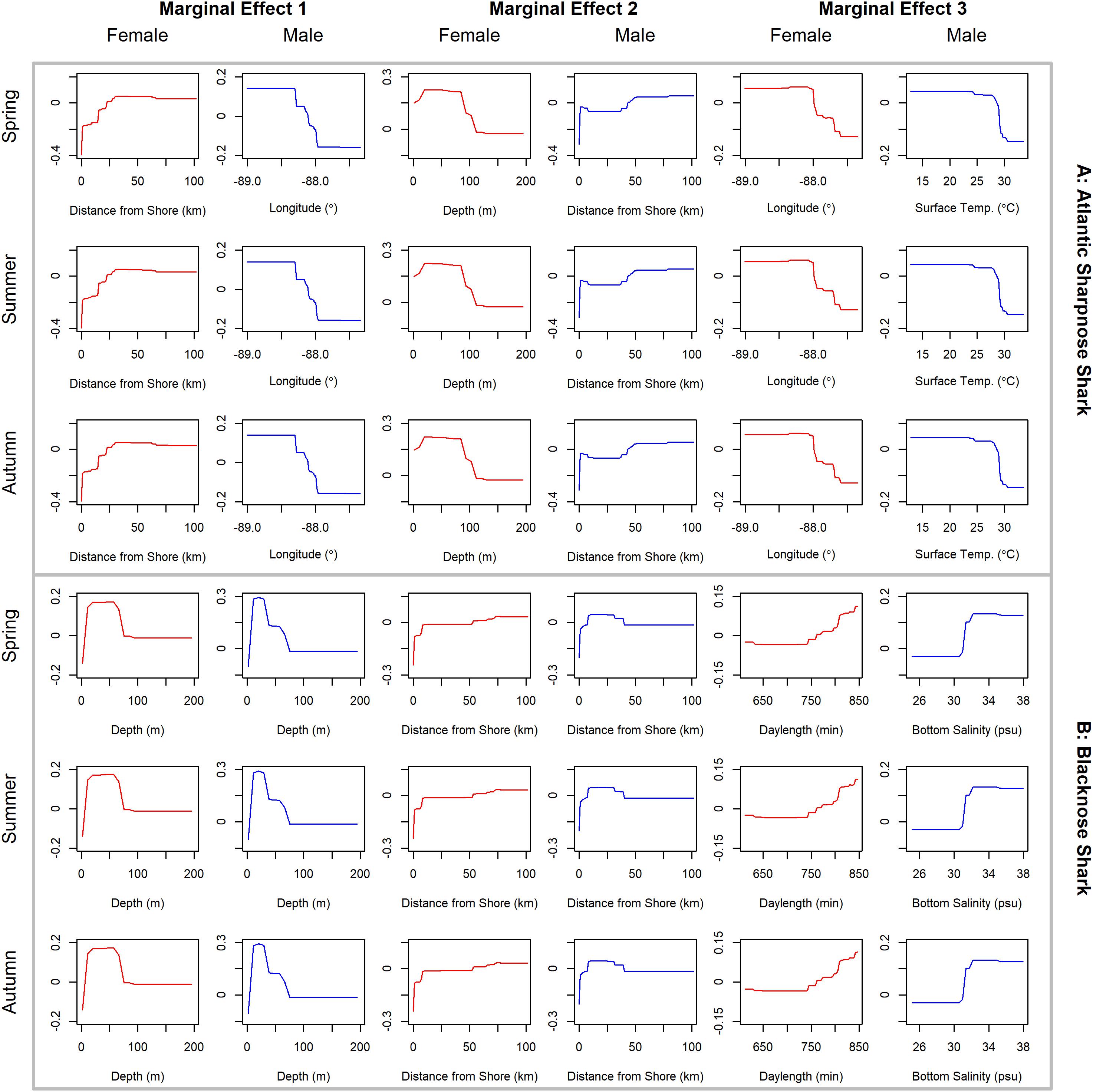
Figure 4. Line plots for the three most influential marginal effects from the binary BRT models for female (red) and male (blue) (A) Atlantic sharpnose shark and (B) blacknose shark during spring, summer and autumn.
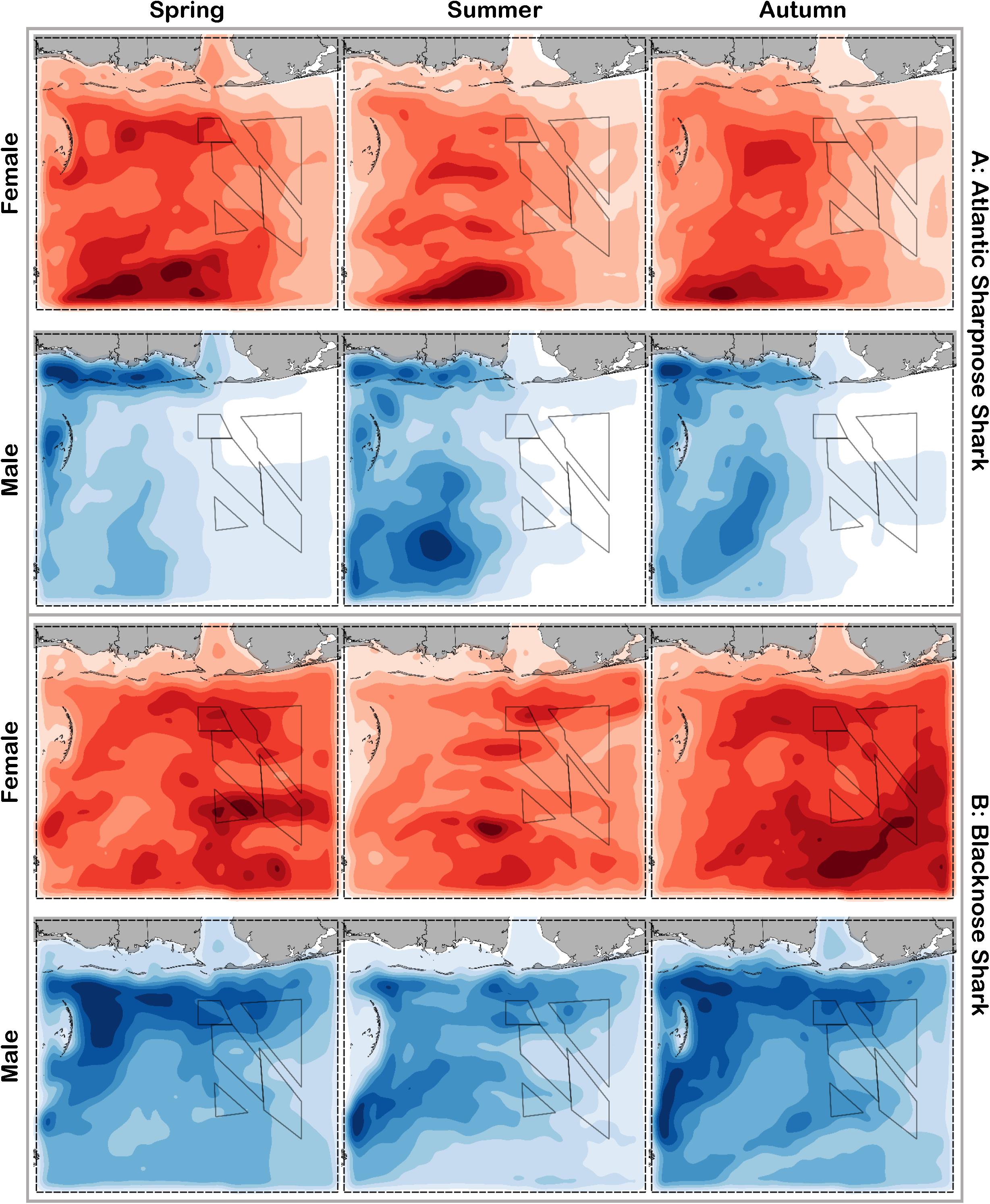
Figure 5. Predicted relative abundance from BRT models for female (red) and male (blue) (A) Atlantic sharpnose shark and (B) blacknose shark during spring, summer and autumn. Darker shades indicate higher predicted abundance, and lighter shades indicate lower predicted abundance.
The factors influencing male Atlantic sharpnose sharks were similar to those influencing females, yet the magnitude of these effects was different. The two predictors with the strongest influence for males were longitude and distance from shore, with little seasonal variation (Table 2). Like females, an east-west gradient was apparent for males, yet was even more pronounced (Figure 4A), such that relatively large areas east of 88°W longitude were predicted to be unsuitable for male Atlantic sharpnose sharks (Figure 5A). Coefficients of variation were highest for males in the western region of the study area, yet comparatively lower for males than females (Supplementary Figure S1A).
Similar to Atlantic sharpnose shark, blacknose sharks were captured across a wide range of sizes (Figure 2B), primarily in nearshore areas (Figure 3B). Females were encountered less frequently than males (0.77:1), which differed from the expected sex ratio (x2 = 26.21, p < 0.01). Final models for blacknose shark season/sex combinations had training data AUC scores ranging from 0.85 to 0.87, and cross validation AUC scores ranging from 0.74 (±0.01) to 0.82 (±0.01) (Table 2). For females, depth was the most influential predictor of abundance (29% across seasons), followed by distance from shore and daylength (Table 2). Large areas of suitable habitat were identified for female blacknose shark (Figure 5B), and coefficients of variation were highest in the fall (Supplementary Figure S1B).
Male blacknose sharks were encountered in depths shallower than female blacknose sharks (Figure 4B). Predicted suitable habitat for male blacknose sharks was less widely dispersed across the survey region but showed more seasonality relative to females (Figure 5B). The primary factors influencing male blacknose sharks were the same as those influencing females (depth and distance from shore), yet also included salinity (Table 2), with male blacknose sharks encountered primarily in waters between 30 and 33 psu (Figure 4B). Consequently, predicted suitable habitat for male blacknose sharks more closely followed the contours of the Chandeleur Islands, Louisiana and the barrier islands south of Mississippi and Alabama (Figure 5B). Coefficients of variation were relatively low for male blacknose sharks (Supplementary Figure S1B).
Large Coastal Sharks
Blacktip sharks were the most common large coastal shark, with females and males encountered across a wide range of sizes (Figure 2C), particularly in shallow waters (Figure 3C). Females were more common than males (1.36:1), which differed from the expected sex ratio (x2 = 26.51, p < 0.01). Final models for blacktip shark season/sex combinations had training data AUC scores ranging from 0.87 to 0.91 and cross validation AUC scores ranging from 0.79 (±0.02) to 0.87 (±0.01) (Table 2). For females, the three most influential predictors of abundance were bottom salinity (36%), distance from shore (16%), and longitude (13%); there was no seasonal variability in the strength of these predictors (Table 2). Female blacktip sharks preferred inshore regions with bottom salinity values between 26 and 33 psu, particularly west of 88°W longitude (Figure 6A), preferences which did not change seasonally. Predicted suitable habitat for female blacktip sharks was concentrated in the Mississippi Sound and west of the Chandeleur Islands (Figure 7A). Coefficients of variation were relatively high for females in the nearshore, western region of the study area in the spring, but not summer or autumn (Supplementary Figure S2A).
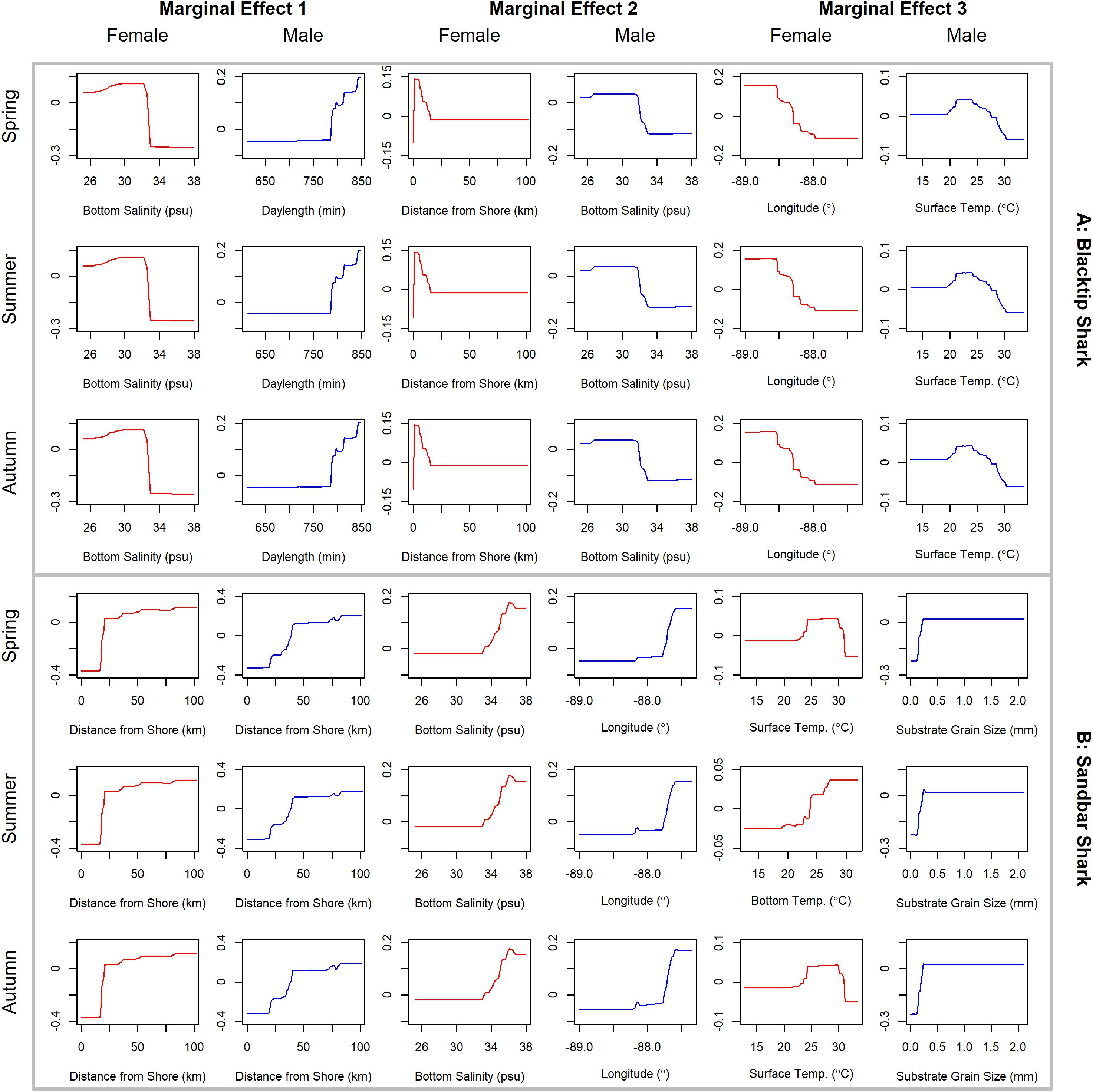
Figure 6. Line plots for the three most influential marginal effects from the binary BRT models for female (red) and male (blue) (A) blacktip shark and (B) sandbar shark during spring, summer, and autumn.
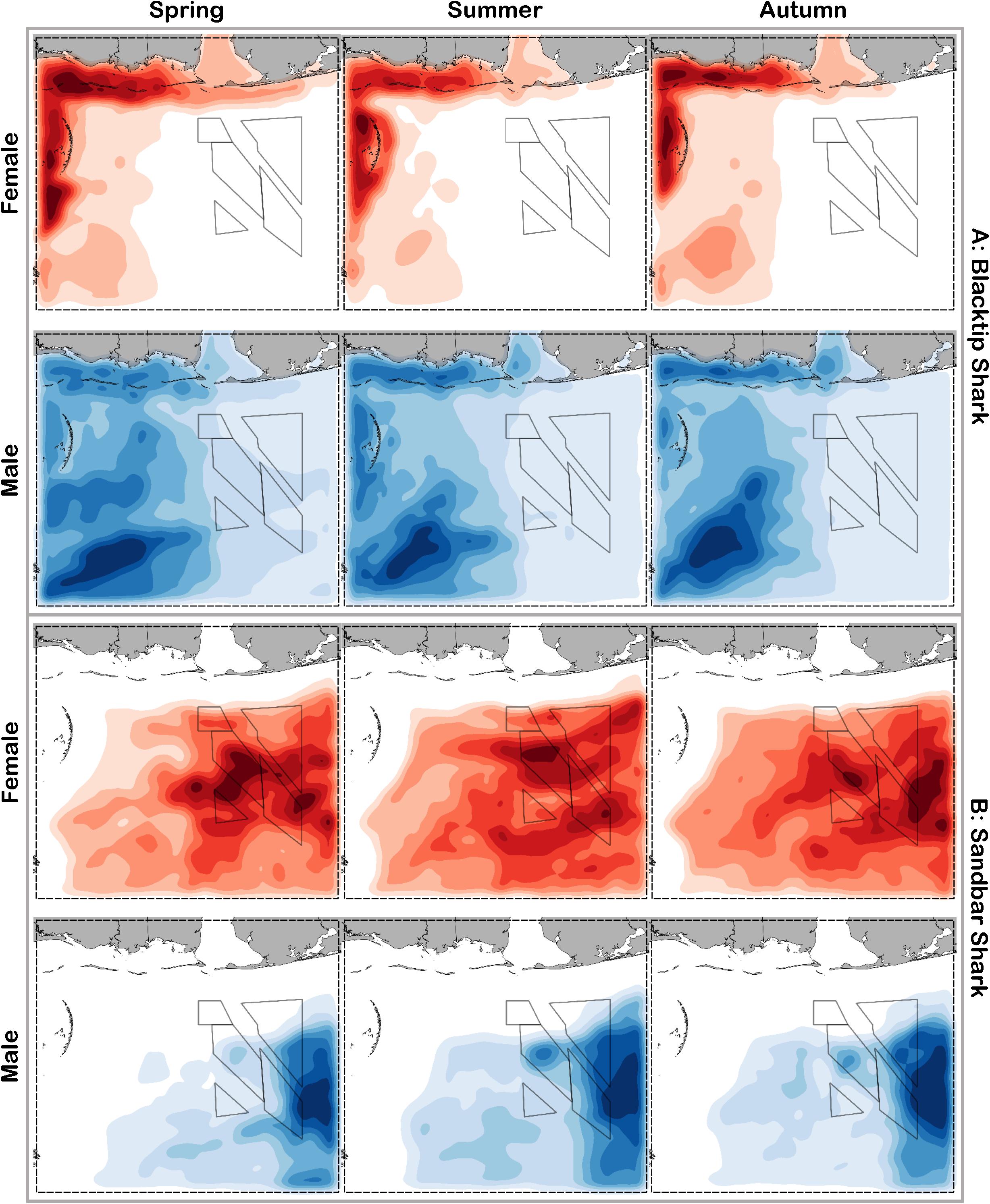
Figure 7. Predicted relative abundance from BRT models for female (red) and male (blue) (A) blacktip shark and (B) sandbar shark during spring, summer and autumn. Darker shades indicate higher predicted abundance, and lighter shades indicate lower predicted abundance.
Male blacktip shark distributions were influenced by daylength (17%), bottom salinity (13%), and surface temperature (11%) (Table 2); similar to females, no seasonal variation in the strength of these predictors was evident. Males were most abundant during periods when daylength exceeded 750 min, in waters between 26 and 33 psu, and at sea surface temperatures between 20 and 24°C (Figure 6A). Suitable habitat was predicted across the continental shelf for male blacktip sharks, particularly in the offshore waters south of Mississippi (Figure 7A). In contrast to females, coefficients of variation were highest for males during autumn and comparatively lower in spring and summer (Supplementary Figure S2A).
Sandbar sharks were the second most abundant large coastal shark. Males and females were encountered across a relatively narrow range of sizes, encompassing primarily sub-adult and adult individuals (Baremore and Hale, 2012; Figure 2D). Individuals were captured offshore in waters greater than 20 m, yet were practically absent from nearshore areas (Figure 3D). Females were encountered more often than males (1.38:1), which differed from the expected sex ratio (x2 = 12.18, p < 0.01). Final models for sandbar shark season/sex combinations had training data AUC scores of 0.94 and cross validation AUC scores ranging from 0.88 (±0.02) to 0.89 (±0.02) (Table 2). The two most influential predictors of abundance for female sandbar sharks were distance from shore (41%) and bottom salinity (10%), with little to no seasonal variation (Table 2). Females were only encountered greater than 20 km from shore, where bottom salinity was greater than 34 psu (Figure 6B). Consequently, areas with suitable habitat for female sandbar shark were predominately located past the 20 m isobath (Figure 7B). In general, coefficients of variation increased with depth (Supplementary Figure S2B).
Male sandbar shark distributions were influenced by distance from shore (∼30%), longitude (∼17%), and substrate grain size (11%) (Table 2). The relative influence of these predictors varied little across seasons. Male sandbar sharks were encountered farther offshore than females (20–45 km), almost exclusively east of 88°W longitude in areas with increasing substrate grain size (Figure 6B). Areas where suitable habitat was predicted for male sandbar sharks were restricted to the southeastern region of our sampling region (Figure 7B), and coefficients of variation were relatively small (Supplementary Figure S2B).
Shelf-Associated Sharks
Scalloped hammerheads were the most abundant shelf-associated species; males were encountered across a wider range of sizes than females (Figure 2E). Males and females were encountered broadly across the continental shelf (Figure 3E). Females were much less common than males (0.36:1), which differed from the expected sex ratio (x2 = 42.63, p < 0.01). Final models for scalloped hammerhead season/sex combinations had training data AUC scores ranging from 0.92 to 0.95 and cross validation AUC scores ranging from 0.83 (±0.02) to 0.87 (±0.03) (Table 2). Female scalloped hammerhead abundance was influenced by distance from shore (28–29%), depth (∼23%), and bottom salinity (7–8%) (Table 2), with little seasonal variation. Female scalloped hammerheads were encountered 75–85 km offshore, at depths between 50 and 100 m (Figure 8A). Suitable habitat for female scalloped hammerheads was restricted to a small core area directly south of Mobile Bay, Alabama (Figure 9A). Coefficients of variation were highest for females during summer (Supplementary Figure S3A).
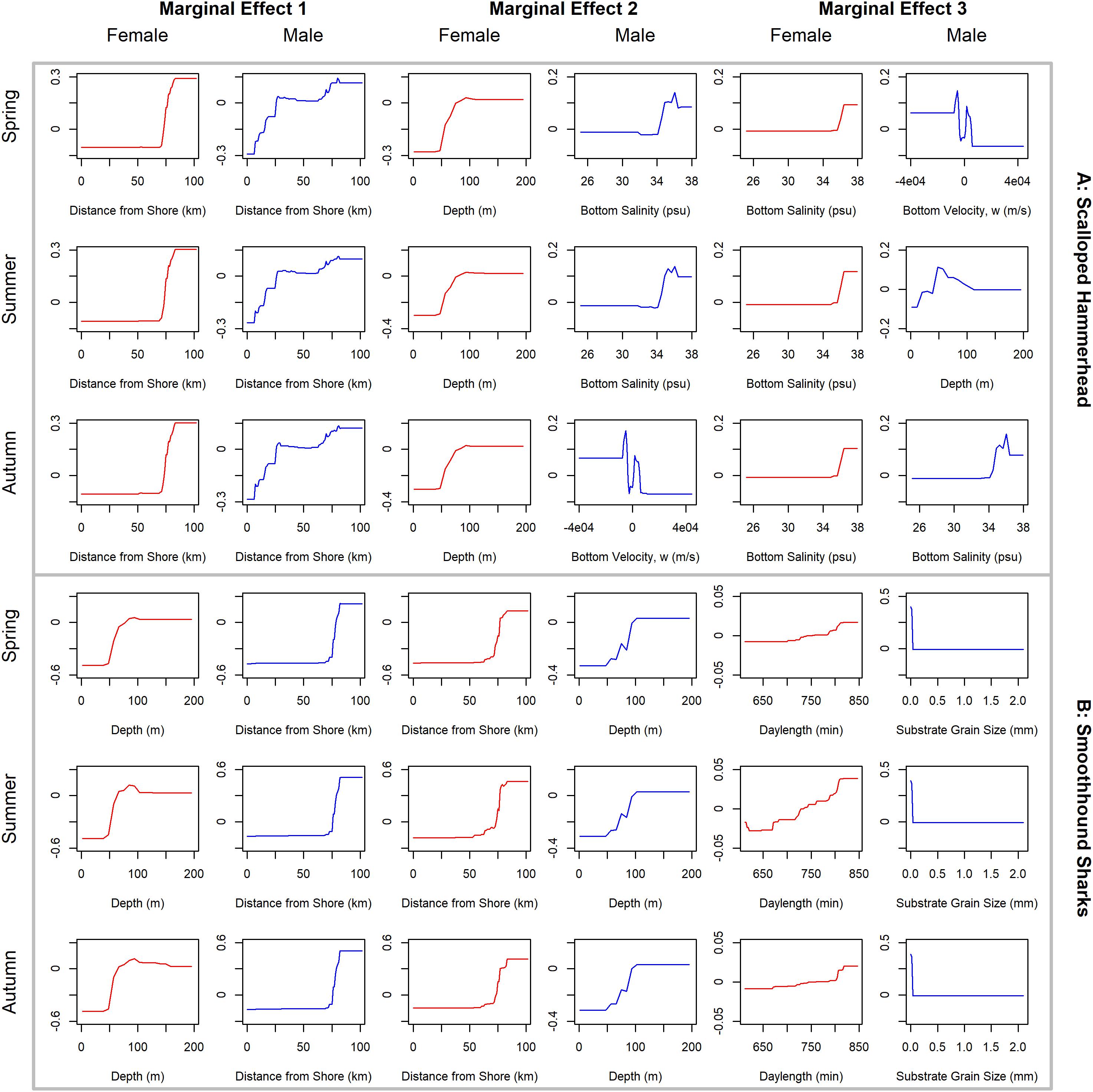
Figure 8. Line plots for the three most influential marginal effects from the binary BRT models for female (red) and male (blue) (A) scalloped hammerhead and (B) smoothhound sharks during spring, summer and autumn.
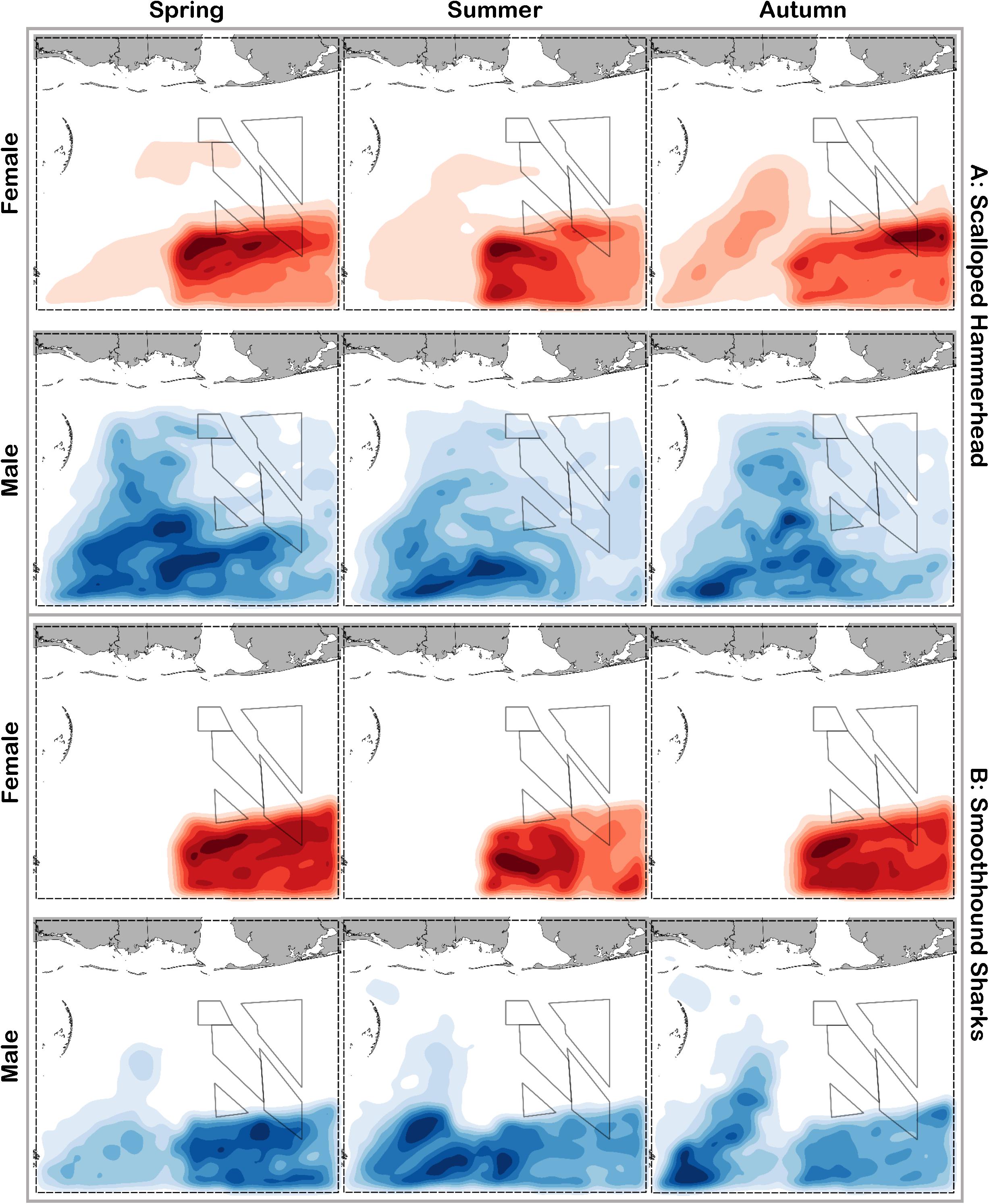
Figure 9. Predicted relative abundance from BRT models for female (red) and male (blue) (A) scalloped hammerhead and (B) smoothhound sharks during spring, summer and autumn. Darker shades indicate higher predicted abundance, and lighter shades indicate lower predicted abundance.
Like females, male scalloped hammerhead abundance was influenced by distance from shore (∼17%), as well as bottom salinity (11–12%) and bottom velocity (11%) (Table 2), with marginal seasonal variation. Male scalloped hammerheads were encountered closer to shore (15–75 km offshore) relative to females, at depths between 25 and 100 m (Figure 8A). Consequently, greater amounts of suitable habitat were predicted for male scalloped hammerheads compared to females (Figure 9A), and coefficients of variation associated with these predictions were comparatively higher, particularly in spring (Supplementary Figure S3A).
Smoothhound sharks were the second most abundant shelf-associated shark and were encountered across a range of sizes, primarily encompassing sub-adults and adults (SEDAR 39, Figure 2F). Smoothhound sharks were encountered on the continental shelf, but across a relatively narrow depth range (Figure 3F). Females were markedly more common than males (5.42:1), which differed from the expected sex ratio (x2 = 79.19, p < 0.01). Final models for smoothhound shark season/sex combinations had training data AUC scores of 0.99, and cross validation AUC scores ranging from 0.93 (±0.03) to 0.97 (±0.01) (Table 2). Female smoothhound shark abundance was influenced by depth (37–44%), distance from shore (30–33%), and daylength (7–10%) (Table 2). The relative strength of these predictors varied slightly between seasons. Female smoothhound sharks were encountered at depths between 50 and 100 m, between 75 and 85 km offshore (Figure 8B). Predicted habitat suitable for female smoothhound sharks was similar to predicted suitable habitat for female scalloped hammerheads (Figure 9B). Coefficients of variation were highest for females in the deepest portion of the study area, particularly in spring and summer (Supplementary Figure S3B).
Male smoothhound shark abundance was influenced by distance from shore (35–37%), depth (17–18%), and substrate grain size (8–9%) (Table 2). Male smoothhound sharks were encountered 75–85 km offshore, at depths ranging from 50 to 100 m (Figure 8B), similar to what was shown for females, however, larger areas of suitable habitat were predicted for males relative to females (Figure 9B). Coefficients of variation were similar between males and females (Supplementary Figure S3B).
Discussion
A diverse assemblage of sharks, dominated by the Atlantic sharpnose shark, was identified across the extent of the area we surveyed. Our findings are remarkably similar to those of Murawski et al. (2018), who identified the continental shelf of the northern GoM as the most speciose region of the Gulf and documented high relative abundance of the Atlantic sharpnose shark in this region. Our study illustrates a strong degree of sexual segregation between female and male Atlantic sharpnose sharks, as first recorded by Springer (1967). This segregation is most pronounced during spring and autumn, when adult males are abundant in the inshore waters north of the barrier islands while females inhabit offshore areas. Parsons and Hoffmayer (2005) also noted this segregation, and suggested the possibility of a reproductively-motivated summer exodus of mature male Atlantic sharpnose sharks to deeper waters for mating. Our findings support this assertion; spatial and temporal overlap between adult male and female Atlantic sharpnose sharks was only predicted offshore during the summer. Collectively, these patterns support the notion that this fast-growing species likely does not depend on discrete, inshore nurseries (Knip et al., 2010; Heupel et al., 2018); rather, mating and parturition for this species may take place in deeper waters during the summer months, as suggested by Drymon et al. (2010).
Notable differences in population structure were apparent between Atlantic sharpnose shark and the other small coastal shark examined in detail, the blacknose shark. First, the composition of the blacknose shark population differed from that of Atlantic sharpnose shark. A smaller size range of blacknose sharks was noted, and most individuals were identified as adults (Driggers et al., 2004); moreover, the sex ratio was biased in favor of males. Second, the factors best predicting distribution and abundance of blacknose sharks appear to act independently of sex, unlike the factors influencing Atlantic sharpnose shark distribution and abundance. Interestingly, males were predicted to exist in higher abundance shallower and closer to shore than females. Third, although the blacknose shark was the second most abundant species sampled in our study, its relative abundance was less than one third of the relative abundance of Atlantic sharpnose shark. This trend aligns with both fishery-dependent (Scott-Denton et al., 2011) and fishery-independent (Murawski et al., 2018) catch data; both of these datasets also suggest that blacknose sharks are even less abundant in areas outside of the northern GoM. The most recent assessment for blacknose shark was unable to determine the status of the GoM stock (SEDAR, 2011); consequently, commercial retention of blacknose sharks in the GoM is currently prohibited. Despite preliminary signs of shark recovery as indicated by increasing population trends for GoM Atlantic sharpnose shark, the relative abundance of GoM blacknose shark is declining (Peterson et al., 2017). Yet, of the six species we examined, blacknose shark possesses the largest predicted range of suitable habitat. We are therefore optimistic that ongoing efforts to rebuild this stock will not be impeded by a lack of suitable habitat.
Blacktip shark was the most abundant large coastal species observed during the survey. This species was captured across a wide range of sizes (neonate through mature individuals) and showed strong sexual segregation. Gravid females and neonates were encountered throughout the nearshore regions of the study area, in accordance with the classical shark population model proposed by Springer (1967). Despite the presence of gravid females inshore, temperature was not a significant predictor of female presence or relative abundance, suggesting that the thermal-niche hypothesis (Wearmouth and Sims, 2008) does not explain this case of sexual segregation. Instead, we speculate that gravid females may be preferentially occupying nearshore waters to provide predator refugia for neonate blacktip sharks following parturition. Surprisingly, the clear sexual segregation demonstrated by this species has not resulted in a sex-biased fishery, as has been shown for shortfin mako in the equatorial Pacific (Mucientes et al., 2009) and spiny dogfish and smooth dogfish in the northwest Atlantic (Dell’Apa et al., 2014, 2018; Haugen et al., 2017). Rather, the most recent update to the stock assessment (SEDAR, 2018) indicates that GoM blacktip sharks represent an outcome of successful fisheries management. Effective quota allocation may contribute to this accomplishment. The commercial quota for the federal GoM blacktip shark fishery is disproportionately divided between the areas east and west of 88°W longitude. The habitat suitability predictions from our BRTs are in striking agreement with this longitudinal quota delineation. Large areas of highly suitable blacktip shark habitat are predicted in the western GoM sub-region (i.e., west of 88°W longitude); in contrast, little suitable habitat is predicted in the eastern GoM sub-region (i.e., east of 88°W longitude). Accordingly, the 2019 quota for western GoM blacktip sharks is approximately ten times greater than the available quota for eastern GoM blacktip sharks. Our BRT results support the assertion that GoM blacktip shark can support sustainable fisheries when strong science-based management strategies are applied (Simpfendorfer and Dulvy, 2017).
Sandbar sharks were encountered across a relatively narrow range of sizes; most individuals were adults approximately 2 m in length. This species has been protected in the GoM since 2010, and populations in the GoM seem to be increasing as a result (Peterson et al., 2017). Our findings show very clear and interesting associations between sandbar sharks and Alabama’s Artificial Reef Zone (AARZ), where depredation (i.e., the partial or complete removal of a hooked species by a non-target species) by sandbar sharks is common in hook and line fisheries (Drymon et al., 2019). In Western Australia, depredation by sharks is higher in areas receiving higher fishing pressure, perhaps indicting learned behavior by the sharks (Mitchell et al., 2018), which may also be the case for sandbar sharks inhabiting the AARZ. Although limited, recapture data from four adult sandbar sharks tagged in the AARZ indicates strong site fidelity. Each of these individuals was recaptured less than 30 km from the location where it was originally tagged. In particular, one individual was at large for nearly 5 years after release and was recaptured only 18 km from the tagging location (Drymon unpublished data). We postulate that the strong associations of sandbar sharks with, and their possible site fidelity to, the AARZ may be driven by a factor not examined in our BRT analysis, namely red snapper (Lutjanus campechanus). The AARZ represents a regional hotspot of red snapper abundance (Karnauskas et al., 2017) and experiences heavy fishing pressure during relatively short recreational fishing seasons. The recent compression of the federal red snapper recreational fishing season has resulted in a derby-style fishery, particularly in the AARZ, where the number of anglers per day has increased in accordance with shortened seasons (Powers and Anson, 2016). Consequently, the spatially and temporally limited recreational fishery for red snapper may explain the high predicted abundance of sandbar sharks in the AARZ. This and similar competition between anglers and sharks over a shared resource (in this case, red snapper) exemplifies an emerging challenge to be addressed as shark populations continue to recover (Carlson et al., 2019).
Male scalloped hammerheads were encountered across a wider range of sizes and were approximately three times more abundant than females. Sexual segregation has previously been described for scalloped hammerheads in the GoM (Branstetter, 1987), and our results support those findings. Similar dynamics have been documented for scalloped hammerheads in the Pacific Ocean, where sexual segregation may allow faster growth among females resulting from increased prey availability in offshore waters (Klimley, 1987). Our findings are consistent with this concept, termed the “forage-selection hypothesis” (Sims, 2005), although dietary studies would be required to confirm this as the mechanism driving sexual segregation in scalloped hammerheads in the northern GoM. Despite the sexual segregation shown for scalloped hammerheads in the current study, distance from shore was the strongest predictor of presence for both sexes, with little seasonal variation. Specifically, females’ predicted suitable habitat is deeper than that of males. Habitat use determined from satellite-tagged scalloped hammerheads support these findings; females more frequently use shelf-edge (>200 m isobath) areas compared to shallower depths used by males (Wells et al., 2018). Regardless of the mechanism driving sexual segregation in scalloped hammerheads, additional efforts to understand habitat use by mature females are needed to reveal habitat areas of particular concern for this species, such as potential areas for parturition (Wells et al., 2018).
Individuals in the genus Mustelus are morphologically conserved, with three genetically distinct species known to occur across the GoM (Giresi et al., 2015). As a result, the smooth dogfish, the Gulf smoothhound and the Florida smoothhound are currently managed as a single, multi-species complex. Accordingly, our sex ratio and BRT analyses apply to all sharks in the genus Mustelus. However, our field identifications since publication of Giresi et al. (2015) demonstrate that nearly all smoothhound sharks represented in our survey are the Gulf smoothhound. Gulf smoothhound sharks are thought to be endemic to the GoM (Compagno et al., 2005) and limited data on their horizontal distribution indicates that they occur predominantly between the Florida panhandle and Louisiana (Murawski et al., 2018). In addition to this horizontal stratification, Gulf smoothhound shark also occupy specific depth ranges. Giresi et al. (2015) report the mean depth of capture for Gulf smoothhound to be 112 m, strikingly similar to the depth range predicted by the BRTs in the current study. Collectively, these habitat preferences place the Gulf smoothhound at risk; species with narrow habitat ranges (e.g., limited geographic range, depth range) are most vulnerable to the synergistic effects of climate change and overfishing (Cheung et al., 2018). In fact, Cheung et al. (2018) report that the two species at highest risk from the combined effects of overfishing and climate change are in the genus Mustelus (M. canis and M. lenticulatus). Therefore, while Gulf smoothhound sharks (and the smoothhound shark complex) are not currently overfished or experiencing overfishing (NOAA, 2019), the relatively restrictive habitat preferences identified for this species indicate that it should be monitored closely and managed in the future with a precautionary approach.
The shark assemblage detailed in this study straddles a unique zoogeographic province in the northern GoM. Portnoy and Gold (2012) suggest that multiple vicariant events formed a marine suture-zone in the vicinity of Mobile Bay, Alabama, which hosts at least fifteen pairs of sister taxa. It is thought that historical events created a physical barrier for sessile organisms that led to speciation of these organisms; however, these events also resulted in modern-day longitudinal differences that appear to be influencing the shark assemblage. One such difference is a gradient in sediment type. Specifically, the area south of Mobile Bay represents a transition from terrigenous sediments in the west to carbonate sediments in the east (Balsam and Beeson, 2003). This transition occurs at ∼88°W longitude and stems from a variety of other factors that influence the distribution of sharks in this assemblage in different ways. For example, the terrigenous sediments that characterize habitats west of Mobile Bay stem from increases in primary production associated with nutrient-rich outflow from Mobile Bay and the Mississippi River. The resultant increases in secondary production and forage fish biomass provide an explanation for the abundance of suitable habitat for the Atlantic sharpnose shark in areas west of Mobile Bay (Drymon et al., 2012, 2013). In addition, increased freshwater output lowers salinity, which the current study suggests increases habitat suitability for blacktip sharks. Unrelated to these abiotic factors, dense concentrations of artificial habitat occur just east of 88°W longitude in the AARZ, and may explain the high predicted concentrations for sandbar sharks, particularly males. Thus, our findings align with previous work indicating a regional zoogeographic break in the juvenile shark assemblage in this region (Bethea et al., 2015) and expand upon those findings by revealing trends in predicted habitat among adult sharks across deeper waters.
Our findings are consistent with previous studies (summarized in Wearmouth and Sims, 2008) and indicate that sexual segregation is the norm for sharks in the northern GoM. Similar to the “sexual line in the sea” demonstrated for shortfin mako in the South Pacific Ocean (Mucientes et al., 2009), the strong sexual segregation demonstrated in this study needs to be considered when forming state and federal management strategies, particularly for species like blacktip shark, which are currently managed under different quotas in the eastern and western GoM. The factors influencing relative abundance among the shark assemblage showed surprisingly little seasonal variation, suggesting that future efforts to characterize the dynamics of this shark community could take place any time of the year. These efforts would be aided by the application of acoustic and/or satellite telemetry, which would provide insights that complement traditional monitoring programs. For example, electronic tags would serve to verify habitat suitability predictions for animals outside of the range of the current study area (e.g., Lee et al., 2019). In addition, telemetry would allow investigations of long-term population dynamics, including partial migrations, which have been shown for larger, more vagile species examined in the current study (e.g., scalloped hammerhead, Hoyos-Padilla et al., 2014). Ultimately, the species- and sex-specific differences in habitat use by the shark assemblage in this region provide further evidence that long-term fishery-independent monitoring should be prioritized.
Data Availability Statement
The datasets generated for this study are available on request to the corresponding author.
Ethics Statement
All research was conducted in accordance with University of South Alabama’s Institutional Animal Care and Use Committee (IACUC) protocol 974304 (2006–2016) and the Mississippi State University Institutional Animal Care and Use Committee (IACUC) protocol 17-620 (2017–2018).
Author Contributions
JD and SP contributed to all aspects of the research including design, implementation, analysis, and writing. SD, JF, ES, and AJ contributed to data analysis and writing. AK and JM assisted with implementation of the research and writing.
Funding
Funding for this work was provided via grants from NOAA SEAMAP and the Alabama Department of Conservation and Natural Resources, Marine Resources Division through the National Fish and Wildlife Foundation, Gulf Environmental Benefits Fund.
Conflict of Interest
The authors declare that the research was conducted in the absence of any commercial or financial relationships that could be construed as a potential conflict of interest.
Acknowledgments
We would like to thank Dauphin Island Sea Lab captains Tom Guoba, Jonathan Wittmann, Rodney Collier, Willie Johnston, and Russell Wilson for keeping us safe on the vessels. Additional thanks to the numerous graduate students, technicians, and interns who assisted with field work, in particular Crystal Hightower and Trey Spearman. This manuscript was greatly improved by thoughtful comments from the two reviewers.
Supplementary Material
The Supplementary Material for this article can be found online at: https://www.frontiersin.org/articles/10.3389/fmars.2020.00035/full#supplementary-material
FIGURE S1 | Coefficient of variation of predicted relative abundance from BRT models for female (red) and male (blue) (A) Atlantic sharpnose shark and (B) blacknose shark during spring, summer and autumn. Darker shades indicate higher variance, and lighter shades indicate lower variance.
FIGURE S2 | Coefficient of variation of predicted relative abundance from BRT models for female (red) and male (blue) (A) blacktip shark and (B) sandbar shark during spring, summer and autumn. Darker shades indicate higher variance, and lighter shades indicate lower variance.
FIGURE S3 | Coefficient of variation of predicted relative abundance from BRT models for female (red) and male (blue) (A) scalloped hammerhead and (B) smoothhound sharks during spring, summer and autumn. Darker shades indicate higher variance, and lighter shades indicate lower variance.
TABLE S1 | Number, size, size range, and seasonal occurrence (denoted with X) of all species captured on bottom longline, 2016–2018.
Footnotes
- ^ https://www.ncddc.noaa.gov/hypoxia/products/2010
- ^ http://pubs.usgs.gov/ds/2006/146/basemaps/gmx_grd/gmx_grd.zip
- ^ www.github.com/SimonDedman/daylength
References
Abeare, S. (2009). Comparisons of Boosted Regression Tree, GLM and GAM Performance in the Standardization of Yellowfin Tuna Catch-Rate Data from the Gulf of Mexico Lonline (sic) Fishery. Master’s thesis, Louisiana State University, Baton Rouge, LA.
Backus, R. H., Springer, S., and Arnold, E. L. Jr. (1956). A contribution to the natural history of the white-tip shark, Pterolamiops longimanus (Poey). Deep Sea Res. 3, 178–188. doi: 10.1016/0146-6313(56)90002-8
Balsam, W. L., and Beeson, J. P. (2003). Sea-floor sediment distribution in the Gulf of Mexico. Deep Sea Res. Part I 50, 1421–1444. doi: 10.1016/j.dsr.2003.06.001
Baremore, I. E., and Hale, L. F. (2012). Reproduction of the sandbar shark in the Western North Atlantic Ocean and Gulf of Mexico. Mar. Coast. Fish. 4, 560–572. doi: 10.1080/19425120.2012.700904
Bethea, D. M., Ajemian, M. J., Carlson, J. K., Hoffmayer, E. R., Imhoff, J. L., Grubbs, R. D., et al. (2015). Distribution and community structure of coastal sharks in the northeastern Gulf of Mexico. Environ. Biol. Fishes 98, 1233–1254. doi: 10.1007/s10641-014-0355-3
Branstetter, S. (1987). Age, growth and reproductive biology of the silky shark, Carcharhinus falciformis, and the scalloped hammerhead, Sphyrna lewini, from the northwestern Gulf of Mexico. Environ. Biol. Fishes 19, 161–173. doi: 10.1007/bf00005346
Carlson, J. K., Heupel, M. R., Young, C. N., Cramp, J. E., and Simpfendorfer, C. A. (2019). Are we ready for elasmobranch conservation success? Environ. Conserv. 46, 264–266. doi: 10.1017/s0376892919000225
Cheung, W. W., Jones, M. C., Reygondeau, G., and Frölicher, T. L. (2018). Opportunities for climate-risk reduction through effective fisheries management. Glob. Change Biol. 24, 5149–5163. doi: 10.1111/gcb.14390
Clark, E., and Von Schmidt, K. (1965). Sharks of the central Gulf coast of Florida. Bull. Mar. Sci. 15, 13–83.
Compagno, L., Dando, M., and Fowler, S. (2005). A Field Guide to the Sharks of the World. Princeton, NJ: Princeton University Press.
Dedman, S., Officer, R., Brophy, D., Clarke, M., and Reid, D. G. (2015). Modelling abundance hotspots for data-poor Irish Sea rays. Ecol. Modell. 312, 77–90. doi: 10.1016/j.ecolmodel.2015.05.010
Dedman, S., Officer, R., Clarke, M., Reid, D. G., and Brophy, D. (2017). Gbm. auto: a software tool to simplify spatial modelling and Marine Protected Area planning. PLoS One 12:e0188955. doi: 10.1371/journal.pone.0188955
Dell’Apa, A., Cudney-Burch, J., Kimmel, D. G., and Rulifson, R. A. (2014). Sexual segregation of spiny dogfish in fishery-dependent surveys in Cape Cod, Massachusetts: potential management benefits. Trans. Am. Fish. 143, 833–844. doi: 10.1080/00028487.2013.869257
Dell’Apa, A., Pennino, M. G., Bangley, C. W., and Bonzek, C. (2018). A hierarchical Bayesian modeling approach for the habitat distribution of smooth dogfish by sex and season in inshore coastal waters of the US Northwest Atlantic. Mar. Coast. Fish. 10, 590–605. doi: 10.1002/mcf2.10051
Dell’Apa, A., Pennino, M. G., and Bonzek, C. F. (2017). Modeling the habitat distribution of spiny dogfish (Squalus acanthias), by sex, in coastal waters of the northeastern United States. Fish. Bull. 115, 89–100. doi: 10.7755/fb.115.1.8
Dormann, C. F., Elith, J., Bacher, S., Buchmann, C., Carl, G., Carré, G., et al. (2013). Collinearity: a review of methods to deal with it and a simulation study evaluating their performance. Ecography 36, 27–46. doi: 10.1111/j.1600-0587.2012.07348.x
Driggers, W., Carlson, J., Cullum, B., Dean, J., and Oakley, D. (2004). Age and growth of the blacknose shark, Carcharhinus acronotus, in the western North Atlantic Ocean with comments on regional variation in growth rates. Environ. Biol. Fish. 71:171. doi: 10.1007/s00244-014-0050-6
Drymon, J. M., Ajemian, M. J., and Powers, S. P. (2014). Distribution and dynamic habitat use of young bull sharks Carcharhinus leucas in a highly stratified northern Gulf of Mexico estuary. PLoS One 9:e97124. doi: 10.1371/journal.pone.0097124
Drymon, J. M., Carassou, L., Powers, S. P., Grace, M., Dindo, J., and Dzwonkowski, B. (2013). Multiscale analysis of factors that affect the distribution of sharks throughout the northern Gulf of Mexico. Fish. Bull. 111, 370–380. doi: 10.7755/fb.111.4.6
Drymon, J. M., Cooper, P. T., Powers, S. P., Miller, M. M., Magnuson, S., Krell, E., et al. (2019). Genetic identification of species responsible for depredation in commercial and recreational fisheries. N. Am. J. Fish. Manag. 39, 524–534. doi: 10.1002/nafm.10292
Drymon, J. M., Powers, S. P., and Carmichael, R. H. (2012). Trophic plasticity in the Atlantic sharpnose shark (Rhizoprionodon terraenovae) from the north central Gulf of Mexico. Environ. Biol. Fish. 95, 21–35. doi: 10.1007/s10641-011-9922-z
Drymon, J. M., Powers, S. P., Dindo, J., Dzwonkowski, B., and Henwood, T. A. (2010). Distributions of sharks across a continental shelf in the northern Gulf of Mexico. Mar. Coast. Fish. 2, 440–450. doi: 10.1577/c09-061.1
Economakis, A. E., and Lobel, P. S. (1998). Aggregation behavior of the grey reef shark, Carcharhinus amblyrhynchos, at Johnston Atoll, Central Pacific Ocean. Environ. Biol. Fish. 51, 129–139. doi: 10.1371/journal.pone.0060331
Elith, J., Leathwick, J. R., and Hastie, T. (2008). A working guide to boosted regression trees. J. Anim. Ecol. 77, 802–813. doi: 10.1111/j.1365-2656.2008.01390.x
Feldheim, K. A., Gruber, S. H., and Ashley, M. V. (2002). The breeding biology of lemon sharks at a tropical nursery lagoon. Proc. R. Soc. B 269, 1655–1661. doi: 10.1098/rspb.2002.2051
Froeschke, J., Stunz, G. W., and Wildhaber, M. L. (2010). Environmental influences on the occurrence of coastal sharks in estuarine waters. Mar. Ecol. Prog. Ser. 407, 279–292. doi: 10.3354/meps08546
Giresi, M. M., Grubbs, R. D., Portnoy, D. S., Driggers, W. B. III, Jones, L., and Gold, J. R. (2015). Identification and distribution of morphologically conserved Smoothhound Sharks in the Northern Gulf of Mexico. Trans. Am. Fish. 144, 1301–1310. doi: 10.1080/00028487.2015.1069212
Grubbs, R. D., Musick, J. A., Conrath, C. L., and Romine, J. G. (2005). “Long-term movements, migration, and temporal delineation of a summer nursery for juvenile sandbar sharks in the Chesapeake Bay region,” in Shark Nursery Grounds of the Gulf of Mexico and East Coast Waters of the United States, Vol. 50, eds C. T. McCandless, and N. E. Kohler, Jr. (Bethesda, MD: AFS), 63–86.
Haig, J. A., Lambert, G. I., Sumpton, W. D., Mayer, D. G., and Werry, J. M. (2018). Habitat features influence catch rates of near-shore bull shark (Carcharhinus leucas) in the Queensland shark control program, Australia 1996-2012. Estuar. Coast. Shelf. Sci. 200, 289–300. doi: 10.1016/j.ecss.2017.11.013
Haugen, J. B., Curtis, T. H., Fernandes, P. G., Sosebee, K. A., and Rago, P. J. (2017). Sexual segregation of spiny dogfish (Squalus acanthias) off the northeastern United States: implications for a male-directed fishery. Fish. Res. 193, 121–128. doi: 10.1016/j.fishres.2017.04.007
Heithaus, M. R., Delius, B. K., Wirsing, A. J., and Dunphy-Daly, M. M. (2009). Physical factors influencing the distribution of a top predator in a subtropical oligotrophic estuary. Limnol. Oceanogr. 54, 472–482. doi: 10.4319/lo.2009.54.2.0472
Heupel, M. R., Kanno, S., Martins, A. P., and Simpfendorfer, C. A. (2018). Advances in understanding the roles and benefits of nursery areas for elasmobranch populations. Mar. Fresh. Res. 70, 897–907.
Hijmans, R. J., and Elith, J. (2013). Species Distribution Modeling with R. R CRAN Project. Vienna: R Foundation for Statistical Computing.
Hoyos-Padilla, M. E., Ketchum, J. T., Klimley, A. P., and Galván-Magaña, F. (2014). Ontogenetic migration of a female scalloped hammerhead Sphyrna lewini in the Gulf of California. Anim. Biotelemetry 2, 1–9.
Jirik, K. E., and Lowe, C. G. (2012). An elasmobranch maternity ward: female round stingrays Urobatis halleri use warm, restored estuarine habitat during gestation. J. Fish. Biol. 80, 1227–1245. doi: 10.1111/j.1095-8649.2011.03208.x
Kajiura, S. M., and Tellman, S. L. (2016). Quantification of massive seasonal aggregations of blacktip sharks (Carcharhinus limbatus) in Southeast Florida. PLoS One 11:e0150911. doi: 10.1371/journal.pone.0150911
Karnauskas, M., Walter, J. F. III, Campbell, M. D., Pollack, A. G., Drymon, J. M., and Powers, S. (2017). Red snapper distribution on natural habitats and artificial structures in the northern Gulf of Mexico. Mar. Coast. Fish. 9, 50–67. doi: 10.1080/19425120.2016.1255684
Kessel, S. T., Chapman, D. D., Franks, B. R., Gedamke, T., Gruber, S. H., Newman, J. M., et al. (2014). Predictable temperature-regulated residency, movement and migration in a large, highly mobile marine predator (Negaprion brevirostris). Mar. Ecol. Prog. Ser. 514, 175–190. doi: 10.3354/meps10966
Klimley, A. P. (1987). The determinants of sexual segregation in the scalloped hammerhead shark, Sphyrna lewini. Environ. Biol. Fishes 18, 27–40. doi: 10.1007/bf00002325
Knip, D. M., Heupel, M. R., and Simpfendorfer, C. A. (2010). Sharks in nearshore environments: models, importance, and consequences. Mar. Ecol. Prog. Ser. 402, 1–11. doi: 10.3354/meps08498
Kohler, N. E., and Turner, P. A. (2001). Shark tagging: a review of conventional methods and studies. Environ. Biol. Fishes 60, 191–224. doi: 10.1016/bs.mcb.2018.11.005
Lane, J. Q., Raimondi, P. T., and Kudela, R. M. (2009). Development of a logistic regression model for the prediction of toxigenic Pseudo-nitzschia blooms in Monterey Bay. Calif. Mar. Ecol. Prog. Ser. 383, 37–51. doi: 10.3354/meps07999
Lee, K. A., Smoothey, A. F., Harcourt, R. G., Roughan, M., Butcher, P. A., and Peddemors, V. M. (2019). Environmental drivers of abundance and residency of a large migratory shark, Carcharhinus leucas, inshore of a dynamic boundary current. Mar. Ecol. Prog. Ser. 622, 121–137. doi: 10.3354/meps13052
Lo, N. C. H., Jacobson, L. D., and Squire, J. L. (1992). Indices of relative abundance from fish spotter data based on delta-lognormal models. Can. J. Fish. Aquat. Sci. 49, 2515–2526. doi: 10.1139/f92-278
Martin, T. G., Wintle, B. A., Rhodes, J. R., Kuhnert, P. M., Field, S. A., Low-Choy, S. J., et al. (2005). Zero tolerance ecology: improving ecological inference by modelling the source of zero observations. Ecol. Lett. 8, 1235–1246. doi: 10.1111/j.1461-0248.2005.00826.x
Maunder, M. N., and Punt, A. E. (2004). Standardizing catch and effort data: a review of recent approaches. Fish. Res. 70, 141–159. doi: 10.1016/j.fishres.2004.08.002
Mitchell, J. D., McLean, D. L., Collin, S. P., Taylor, S., Jackson, G., Fisher, R., et al. (2018). Quantifying shark depredation in a recreational fishery in the Ningaloo Marine Park and Exmouth Gulf, Western Australia. Mar. Ecol. Prog. Ser. 587, 141–157. doi: 10.3354/meps12412
Mucientes, G. R., Queiroz, N., Sousa, L. L., Tarroso, P., and Sims, D. W. (2009). Sexual segregation of pelagic sharks and the potential threat from fisheries. Biol. Lett. 5, 156–159. doi: 10.1098/rsbl.2008.0761
Murawski, S. A., Peebles, E. B., Gracia, A., Tunnell, J. W. Jr., and Armenteros, M. (2018). Comparative abundance, species composition, and demographics of continental shelf fish assemblages throughout the Gulf of Mexico. Mar. Coast. Fish. 10, 325–346. doi: 10.1002/mcf2.10033
NOAA (2019). 2018 Stock Assessment and Fishery Evaluation Report (SAFE) for Atlantic Highly Migratory Species. Silver Spring: NOAA,Google Scholar
Parisien, M. A., and Moritz, M. A. (2009). Environmental controls on the distribution of wildfire at multiple spatial scales. Ecol. Monogr. 79, 127–154. doi: 10.1890/07-1289.1
Parsons, G. R., and Hoffmayer, E. R. (2005). Seasonal changes in the distribution and relative abundance of the Atlantic sharpnose shark Rhizoprionodon terraenovae in the north central Gulf of Mexico. Copeia 2005, 914–920. doi: 10.1643/0045-8511(2005)005%5B0914:scitda%5D2.0.co;2
Peterson, C. D., Belcher, C. N., Bethea, D. M., Driggers, W. B. III, Frazier, B. S., and Latour, R. J. (2017). Preliminary recovery of coastal sharks in the south-east United States. Fish Fish. 18, 845–859. doi: 10.1111/faf.12210
Plumlee, J. D., Dance, K. M., Matich, P., Mohan, J. A., Richards, T. M., TinHan, T. C., et al. (2018). Community structure of elasmobranchs in estuaries along the northwest Gulf of Mexico. Estuar. Coast. Shelf Sci. 204, 103–113. doi: 10.1016/j.ecss.2018.02.023
Portnoy, D. S., and Gold, J. R. (2012). Evidence of multiple vicariance in a marine suture-zone in the Gulf of Mexico. J Biogeogr. 39, 1499–1507. doi: 10.1111/j.1365-2699.2012.02699.x
Powers, S. P., and Anson, K. (2016). Estimating recreational effort in the Gulf of Mexico red snapper fishery using boat ramp cameras: reduction in federal season length does not proportionally reduce catch. N. Am. J. Fish. Manag. 36, 1156–1166. doi: 10.1080/02755947.2016.1198284
Powers, S. P., Drymon, J. M., Hightower, C. L., Spearman, T., Bosarge, G. S., and Jefferson, A. (2018). Distribution and age composition of red snapper across the inner continental shelf of the north-central Gulf of Mexico. Trans. Am. Fish. 147, 791–805. doi: 10.1002/tafs.10081
R Core Team (2013). R: A Language and Environment for Statistical Computing. Vienna: R Foundation for Statistical Computing.
Rabalais, N. N., Turner, R. E., and Wiseman, W. J. (2002). Gulf of Mexico hypoxia, a.k.a. “The Dead Zone”. Annu. Rev. Ecol. Evol. Syst. 33, 235–263. doi: 10.1146/annurev.ecolsys.33.010802.150513
Rago, P. J., and Sosebee, K. A. (2010). Biological Reference Points for Spiny Dogfish. Silver Spring: NOAA.
Schlaff, A. M., Heupel, M. R., and Simpfendorfer, C. A. (2014). Influence of environmental factors on shark and ray movement, behaviour and habitat use: a review. Rev. Fish Biol. Fisher. 24, 1089–1103. doi: 10.1007/s11160-014-9364-8
Scott-Denton, E., Cryer, P. F., Gocke, J. P., Harrelson, M. R., Kinsella, D. L., Pulver, J. R., et al. (2011). Descriptions of the US Gulf of Mexico reef fish bottom longline and vertical line fisheries based on observer data. Mar. Fish. Rev. 73, 1–26.
SEDAR (2015). HMS Gulf of Mexico Smoothhound Complex. 39 Final Stock Assessment Report (SAR). SEDAR.
SEDAR (2018). HMS Gulf of Mexico Blacktip Shark Update. 29 Update Stock Assessment Report (SAR). SEDAR.
Simpfendorfer C. A., and Dulvy, N. K. (2017). Bright spots of sustainable shark fishing. Curr. Biol. 27, R97–R98. doi: 10.1016/j.cub.2016.12.017
Sims D. W. (2005). “Differences in habitat selection and reproductive strategies of male and female sharks,” in Sexual Segregation in Vertebrates: Ecology of the Two Sexes, ed. K. Ruckstuhl, (Cambridge, MA: Cambridge University Press), 127–147. doi: 10.1017/cbo9780511525629.009
Springer S. (1967). “Social organization of shark populations,” in Sharks, Skates and Rays, eds P. W. Gilbert, R. F. Mathewson, and D. P. Rall, (Baltimore, MD: John Hopkins Press), 149–174.
Ubeda, A. J., Simpfendorfer, C. A., and Heupel, M. R. (2009). Movements of bonnetheads, Sphyrna tiburo, as a response to salinity change in a Florida estuary. Environ. Biol. Fishes 84, 293–303. doi: 10.1093/jhered/esv030
Ward-Paige, C. A., Britten, G. L., Bethea, D. M., and Carlson, J. K. (2014). Characterizing and predicting essential habitat features for juvenile coastal sharks. Mar. Ecol. 36, 419–431. doi: 10.1111/maec.12151
Wearmouth, V. J., and Sims, D. W. (2008). Sexual segregation in marine fish, reptiles, birds and mammals: behaviour patterns, mechanisms and conservation implications. Adv. Mar. Biol. 54, 107–170. doi: 10.1016/S0065-2881(08)00002-3
Keywords: sexual segregation, boosted regression trees, BRT, fisheries-independent monitoring, bottom longline, elasmobranch, habitat suitability, species distribution modeling
Citation: Drymon JM, Dedman S, Froeschke JT, Seubert EA, Jefferson AE, Kroetz AM, Mareska JF and Powers SP (2020) Defining Sex-Specific Habitat Suitability for a Northern Gulf of Mexico Shark Assemblage. Front. Mar. Sci. 7:35. doi: 10.3389/fmars.2020.00035
Received: 08 November 2019; Accepted: 20 January 2020;
Published: 06 February 2020.
Edited by:
Michele Thums, Australian Institute of Marine Science (AIMS), AustraliaReviewed by:
Vinay Udyawer, Australian Institute of Marine Science (AIMS), AustraliaJonathan M. Werry, Griffith University, Australia
Copyright © 2020 Drymon, Dedman, Froeschke, Seubert, Jefferson, Kroetz, Mareska and Powers. This is an open-access article distributed under the terms of the Creative Commons Attribution License (CC BY). The use, distribution or reproduction in other forums is permitted, provided the original author(s) and the copyright owner(s) are credited and that the original publication in this journal is cited, in accordance with accepted academic practice. No use, distribution or reproduction is permitted which does not comply with these terms.
*Correspondence: J. M. Drymon, bWFyY3VzLmRyeW1vbkBtc3N0YXRlLmVkdQ==