- Halmos College of Natural Sciences and Oceanography, Nova Southeastern University, Dania Beach, FL, United States
The lanternfishes (Myctophidae) are a highly speciose, globally-distributed family of fishes that constitute a dominant component of the global pelagic fauna. As a vertically-migrating taxon of oceanic micronekton, myctophids play vital ecological roles in the biological carbon pump and as an important prey group for several commercially-important species. However, our knowledge of the ecology of this taxon remains incomplete, and as anthropogenic impacts continue to develop and extend into deeper waters, there is a clear need for a better understanding of its ecological role and assemblage dynamics. The aim of the present study was to examine the distribution patterns of the myctophid assemblage within a 200 km × 700 km grid of the northern Gulf of Mexico (GoM) in relation to major mesoscale hydrographic features. The 22 dominant myctophid species (>0.05% by relative abundance) were analyzed from a total of 302 trawl samples collected between January and September 2011, from 0 to 1000 m depth. Redundancy analysis (RDA) indicated that measured environmental variables and spatial patterning explained an average of 12% (range: 0–27%) of the observed variance in the myctophid assemblage. Distance-based Moran’s Eigenvector Mapping (dbMEM) and trend analysis (RDA) indicated limited significant spatial coherence within the assemblage at the scales considered. Local contribution to beta diversity scores corroborated these findings, indicating that the majority of samples were not significantly different from the mean assemblage structure. Taken together, these results suggest that the myctophid assemblage in the northern GoM is well-mixed and highly dispersed at the sub-basin scale (at least), likely the result of the interaction between vertical migration and depth-specific lateral advection. Findings such as these inform our approach to assessing impacts in a large, dynamic, pelagic ecosystems. It is essential to know over what spatial scales assessments of pelagic faunal impacts, and potential recoveries, must be based. In cases of large, spatially integrated pelagic assemblages, high dispersal rates may serve to either ameliorate the effects of a disturbance through immigration or spread the effects across a wider spatial area than the disturbance phenomenon footprint itself.
Introduction
Understanding how biodiversity is structured within ecosystems can provide insight into both the natural variability and functioning of those ecosystems and their stability in the face of natural or anthropogenic disturbance (e.g., Tilman et al., 2014). Nonetheless, there are significant gaps in our knowledge of many ecosystems, which can hinder our ability to predict future changes. This is especially true of the deep-pelagic realm, which has historically received relatively little scientific attention (Webb et al., 2010) and yet is likely to provide a wide range of essential ecosystem functions and services that are of global importance (e.g., Gjosaeter and Kawaguchi, 1980; Robinson et al., 2010; St. John et al., 2016).
One pelagic taxon of particular ecological interest is the Myctophidae, which is a highly speciose and globally-distributed family of fishes containing 251 recognized species from 32 genera (Fricke et al., 2019). Like much of the mesopelagic fauna, almost all myctophid species undertake diel vertical migrations (DVMs). While DVM behaviors are influenced by species identity, life-history stage, and environmental variables, the most common migratory pattern for myctophids involves individuals remaining at mesopelagic depths (c. 200–1000 m) during the day to avoid predation, and then migrating to epipelagic depths (c. 0–200 m) at night to feed (e.g., Gjosaeter and Kawaguchi, 1980; Sutton, 2013). DVM behaviors directly facilitate the active transport of carbon from the surface ocean to depth (e.g., Robinson et al., 2010), suggesting that myctophids and other migratory animals may play important roles in pelagic biogeochemical cycling and climate regulation. Similarly, myctophids are an important food source for numerous predators, including commercially-valuable and deep-living fish species, marine mammals and seabirds (e.g., Sutton and Hopkins, 1996; Beamish et al., 1999; Pusineri et al., 2008), and create important trophic connections between open oceans and terrestrial, coastal, and seafloor ecosystems. When one considers that the mesopelagic realm contains by far the highest biomass of fish on the planet (Kaartvedt et al., 2012; Irigoien et al., 2014) and that myctophids are once again being considered as a potential fishery resource (St. John et al., 2016), the importance of better understanding the spatial and temporal dynamics of these fishes is clear.
In recent years, there has been growing interest in identifying ecologically-meaningful predictors of deep-pelagic community structure, particularly in relation to mesoscale oceanographic features (i.e., those that occur over 10s to 100s of kilometers). The Gulf of Mexico (GoM) is a particularly interesting location to study mesoscale processes of deep-pelagic fishes, as it harbors an especially diverse myctophid assemblage (Gartner et al., 1987; Biggs and Ressler, 2001), as well as a number of relatively well-defined mesoscale oceanographic features of potential biological importance. The offshore GoM also has the potential to be heavily impacted by human activities, as was highlighted by the Deepwater Horizon oil spill in 2010, and there is therefore an urgent need to better understand the natural drivers of offshore, pelagic fauna to predict their effects on pelagic ecosystems in the future and how they may interact with anthropogenic impacts.
One of the major mesoscale oceanographic features influencing the upper circulation (<1000 m) of the GoM is the loop current (LC) and its associated eddies. The LC enters the GoM through the Yucatan Channel in the south, bringing warm, saline subtropical underwater (STUW; Rivas et al., 2005) into the GoM before exiting to the NW Atlantic through the Florida Straits (c. 750 m maximum depth). Anti-cyclonic Loop Current Eddies (LCEs) are formed from northward intrusions of the LC into the GoM, and are typically large (100s of kilometers in diameter), downwelling features associated with low surface productivity that generally persist for several months or years, and may extend to depths of several 100 m (e.g., Elliott, 1982; Biggs, 1992; Oey et al., 2003). LCEs can be identified within the GoM as regions of high sea surface height anomalies (SSHA; e.g., Zimmerman and Biggs, 1999; Jochens and DiMarco, 2008), and from temperature-salinity and temperature-depth profiles, where STUW is warmer than the surrounding water mass types below c. 200 m (Herring, 2010; Johnston et al., 2019). Over time, LCEs propagate westwards through the GoM, gradually mixing with Gulf Common Water (GCW) as they age and decay (Vukovich, 2007). Upwelling cyclonic eddies (CEs) are relatively small (10s km), transient (days–weeks) features that can form along the boundaries of LCEs (Vukovich, 2007). In the northeast GoM, cyclone-anticyclone confluences can draw productive coastal and riverine waters offshore (Biggs and Muller-Karger, 1994; Biggs and Ressler, 2001), usually following the summer rainy season when river outflows are highest (Morey et al., 2003).
In this manner, LCEs have the potential to facilitate the physical transport of fauna both between ocean basins, as well as between coastal and offshore ecosystems (e.g., Olson, 1991). However, they are also hypothesized to affect faunal distributions through local influences on primary productivity, with LCEs creating low-productivity regions, and CEs and riverine inputs creating high productivity regions respectively. In an acoustic study of the GoM, Zimmerman and Biggs (1999) reported greater abundances of mesopelagic fauna within more productive cyclonic eddies compared to less productive LCEs, and similar observations have been made for anticyclonic eddies in the Pacific Ocean (Barnett, 1984; Drazen et al., 2011). Similarly, Godo et al. (2012) reported that higher faunal biomasses were associated with an anticyclonic eddy in the Norwegian Sea that contained high-productivity coastal waters. To date, the effects of riverine waters as transient regions of enhanced productivity have not been studied for any offshore fauna in the GoM.
It is notable that most previous studies have focused on patterns of abundance and biomass, and less is known about how mesoscale features may influence biodiversity patterns within regional basins (but see Potier et al., 2014; Olivar et al., 2016). In the present study, we aim to determine the relative importance of major mesoscale environmental features and spatial processes on the beta diversity of myctophids, using data collected from the upper 1000 m of the northern GoM between a continuous January-to-September 2011 survey. For the present study, we define beta diversity as the variation in assemblage composition between samples following Legendre et al. (2005).
Materials and Methods
Data Collection and Filtering
A 10-m2 Multiple Opening-Closing Net and Environmental Sensing System (MOCNESS) was used to sample 46 locations across the northern GoM during three consecutive sampling campaigns conducted between January 2011 and September 2011 (Figures 1, 2) as part of the Offshore Nekton Sampling and Analysis Program (ONSAP; Cook et al., unpublished). Each campaign was designed to survey all stations over a period of 3 months (January–March; April–June; July–September). For the purposes of defining the spatiotemporal scales of the analyses, each campaign was considered to be a replicate survey of the northern GoM. The spatial extent was therefore c. 200 km × 700 km with a spatial grain of c. 50 km and temporal resolution of c. 3 months.
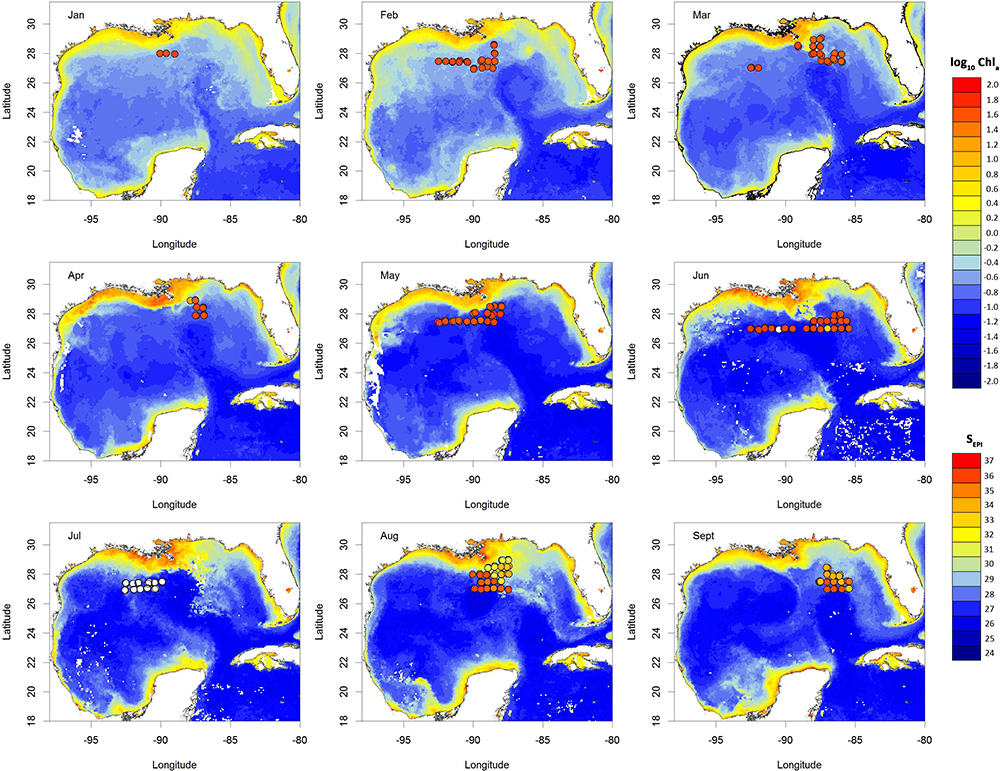
Figure 1. Maps showing the satellite-derived log10 Chla concentrations across the GoM per month. Each point is a MOCNESS sample, colored according to the SEPI values recorded by the MOCNESS sensors at the time of collection. White points indicate no MOCNESS sensor data were available. Chart datum: WGS84.
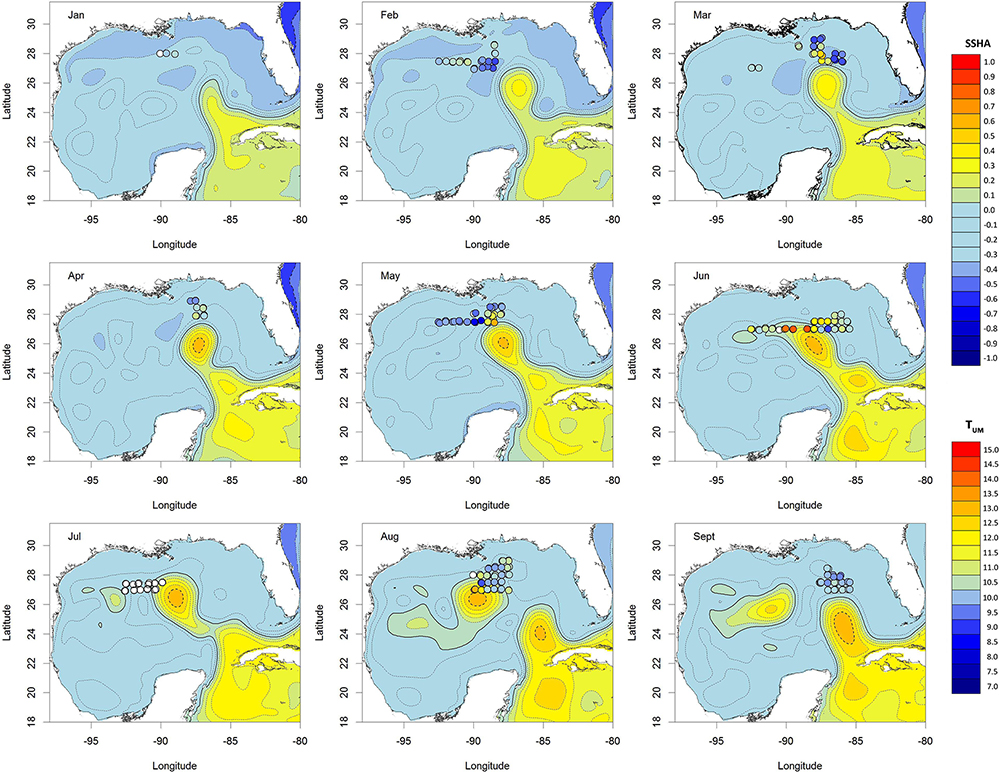
Figure 2. Maps showing satellite-derived, integrated SSHA data per month (from CMEMS). Contour lines mark 0.1 m intervals; 0 m is indicated by the solid line. Each point is a MOCNESS sample, colored according to the TUM at the time of collection. White points indicate no MOCNESS sensor data were available. Chart datum: WGS84.
Each location was visited once per campaign, during which two MOCNESS deployments were conducted, centered around solar noon and midnight. Each deployment produced five depth-stratified samples from 0 to 1500 m, with the depth ranges chosen to reflect classical ecological divisions of the pelagic ocean (Sutton, 2013) and the approximate depth (1000–1200 m) of the deep-water oil plume observed after the Deepwater Horizon oil spill (Camilli et al., 2010).
A number of quality filters were applied to the data to reduce the effects of sampling errors and random noise. Firstly, only daytime samples collected from the upper (200–600 m) and lower mesopelagic (600–1000 m), and night-time samples from the epipelagic (0–200 m) were included in the analyses. These depth strata contain the majority of the captured myctophids, and reflects their DVM behaviors (Supplementary Figure 1). Secondly, only myctophids identified to species level were retained in the dataset. Finally, myctophid counts were standardized by trawl volume (as recorded by the MOCNESS sensors) and only those species comprising > 0.5% of the total myctophid fauna were retained for further analysis.
To test the effects of mesoscale features on the myctophid assemblage composition, six physical and chemical variables were selected as indicators of either coastal and riverine inputs to the GOM, or of anti-cyclonic LCEs (Table 1). Conceptually, these spatial variables were considered to represent either “static” spatial processes with no biologically-relevant temporal component (e.g., distance from the 200 m isobath), or “dynamic” spatial processes. The values of the dynamic processes at a location represent effectively instantaneous measurements [e.g., mean temperature in the upper mesopelagic (200–600 m; TUM); minimum surface salinity (SEPI)], up to integrated monthly measures [e.g., SSHA; chlorophyll a concentration (Chla)]. Any samples for which MOCNESS sensor data were unavailable were excluded from further analysis, which included all samples collected in July 2011.
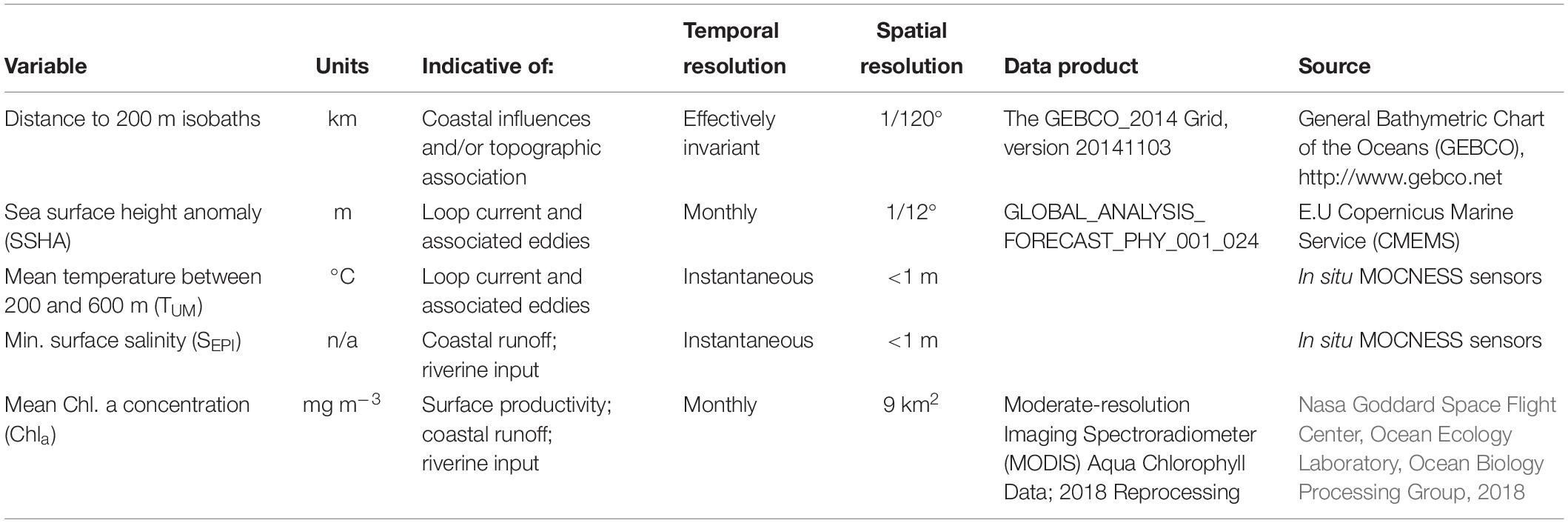
Table 1. Environmental variables selected for inclusion in the multivariate analyses and their suggested interpretation.
Community Analysis
The myctophid assemblage data were analyzed using three separate analyses: redundancy analysis (RDA; following Legendre and Legendre, 2012) to identify linear trends in assemblage composition using the Cartesian co-ordinate data; RDA to examine the effects of the mesoscale variables of interest on assemblage composition (Table 1); and distance-based Moran’s Eigenvector Maps to quantify spatial patterning within the assemblage (dbMEM; Dray et al., 2006). These three analyses were then followed by variance partitioning (Peres-Neto et al., 2006) to quantify the relative effects of the three sets of variables in shaping the myctophid beta diversity across the northern GoM. These multivariate analyses are particularly useful in that they allow spatial autocorrelation in the environmental variables and assemblage data to be explicitly accounted for Dormann et al. (2007) and partitioned within the statistical framework, reducing the risk of type 1 errors when determining the importance of the environmental conditions. Data from each depth stratum and survey campaign were analyzed separately. All analyses were conducted using R software (R Core Team, 2018) and results were considered significant at p < 0.05. Where significance was determined by permutation testing, 10000 permutations were used.
Prior to analysis, the myctophid count data were Hellinger transformed and converted to a Hellinger distance matrix (Legendre and Gallagher, 2001). Geographic position data (latitude and longitude) corresponding to each sample were transformed to Cartesian coordinates using the “geoXY” function in the SoDA package (Chambers, 2013), and the mesoscale variables were scaled and centered using the “scale” function. The overall relationship between the geographic distance and the Hellinger distance between samples was examined using Pearson’s correlation coefficient, and conducted separately for each of the survey periods and depth strata examined.
The analyses were then conducted in the following sequence. First, linear spatial trends in the myctophid data were identified by testing the Cartesian coordinate data against the myctophid data with the “rda” and “anova.cca” functions in the vegan package (Oksanen et al., 2017). If a significant overall effect of latitude or longitude was detected, the significant variable(s) were identified by forward selection using the double-stopping criterion described by Blanchet et al. (2008) and implemented in the “forward.sel” function in the packfor package (Dray, 2013). Any significant coordinate variable(s) were used to detrend the myctophid data prior to any further analyses. Next, RDA and forward selection were used to identify any significant effects of mesoscale variables on the (detrended) myctophid data. Variance inflation factor (VIF) scores were checked following RDA using the “VIF” function, and any variables with a score > 5 (indicating collinearity) were excluded and the model refitted.
To assess the spatial scales of variation within the data, a geographic distance matrix was generated for all sample locations by applying the Euclidean distance function “dist” to their Cartesian coordinates. The dbMEM eigenfunctions were generated from this distance matrix using the “PCNM” function in the PCNM package (Dray et al., 2015), where the truncation distance was set to the minimum distance required to connect all locations within a minimum spanning tree (here, c. 75–100 km). Moran’s I was used as the measure of spatial autocorrelation, where positive values indicate positive spatial autocorrelation and negative values indicate negative spatial autocorrelation. Since identifying positive spatial correlation was of most ecological interest, only those eigenfunctions with positive Moran’s I values were retained, and tested for overall significance using the “anova.cca” function. If the overall test indicated significant effects, forward selection was conducted as before. To identify whether the groups covaried with the observed mesoscale variables, the significant RDA axes from each group were regressed against the mesoscale variables using linear models (“lm” function).
Finally, variance partitioning was conducted with respect to any significant linear trends, significant dbMEM eigenfunctions, and significant mesoscale variables identified during the previous analyses using the “varpart” function in the vegan package.
As a complement to the spatial eigenfunction analyses, local contribution to beta diversity (LCBD; Legendre and de Caceres, 2013) scores were generated for each sample and used to identify those samples where the assemblage composition differed significantly from that of the mean assemblage. LCBD scores were generated from the Hellinger distance matrix for the myctophid data using the “LCBD.comp” function in the adespatial package (Dray et al., 2016). The resulting p-values were adjusted using the Holm correction for multiple comparisons. Linear models (LMs) were fitted to identify whether LCBD scores correlated with different environmental conditions or with Hellinger-transformed abundances of particular species. In both cases, term selection was conducted by backward selection using AIC scores as the selection criterion. Variables were retained in the model if their exclusion resulted in the AIC score increasing by ≥ 4.
Results
Environmental Setting
The physical and chemical conditions varied over the course of the sampling period, with temporal and spatial patterns evident in the data (Figures 1, 2). SEPI were typically high between January and June (January–June) with much lower values recorded later in the year (August–September). The Chla concentrations showed a similar pattern, suggesting that offshore transport of coastal and riverine water occurred during late summer 2011. The mean monthly SSHA maps indicate that a LCE began to form in February–March 2011, but that the core of the eddy remained south of the surveyed area during 2011. During April–June 2011, SSHA strongly covaried with TUM and SEPI and so was excluded from the analyses.
Community Analyses
A total of 20,953 myctophids from were collected from 302 non-empty net samples from the epipelagic (night) and mesopelagic (day), representing 18.2% of the total number of fishes captured in these depths (the numerically-dominant gonostomatid, Cyclothone spp. comprised 50.1%). The dominant taxa included in the analyses comprised 20,325 individuals from 22 species (Table 2), which represented c. 80% of all myctophids captured during 2011 by number. Some temporal variation in the rank order of the dominant species was observed through the survey period (Table 2), though species composition otherwise remained relatively consistent. All myctophids were adults, with standard lengths ranging from 7–77 mm (epipelagic; night), and 7–99 mm (mesopelagic; day).
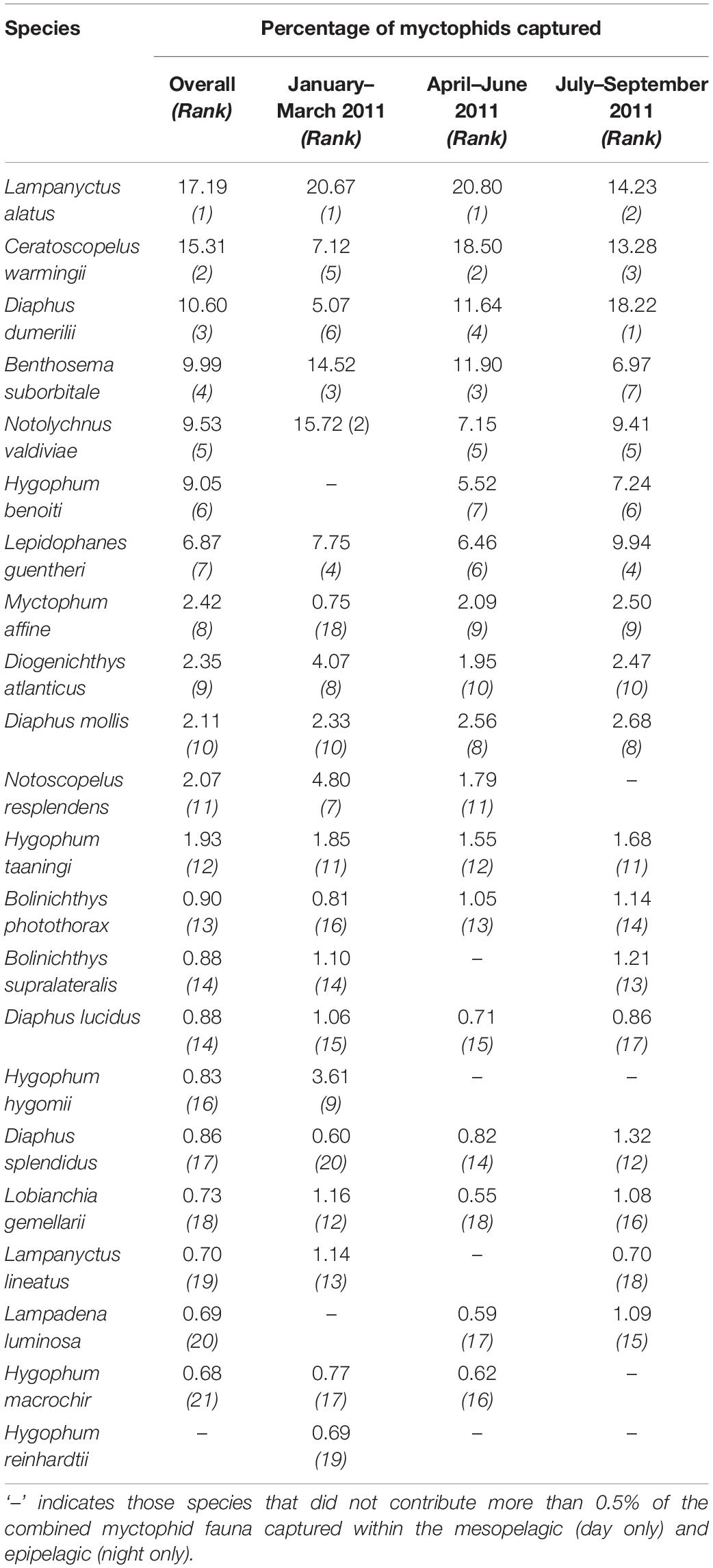
Table 2. Percentage contribution to the total myctophid assemblage captured during 2011 from each cruise series.
Epipelagic Depths (0–200 m; Night)
Between January and March 2011, the composition of myctophids between 0 and 200 m was significantly correlated with SEPI [R2 (adj.) = 0.11; p < 0.001]. A longitudinal trend in the data was identified, but did not remain significant after variance partitioning (Figure 3). No environmental variables were significantly correlated with assemblage composition between April and June (p = 0.07), though a latitudinal trend was evident [R2 (adj.) = 0.05; p = 0.01]. In August and September, myctophid assemblage composition was significantly correlated with SSHA [R2 (adj.) = 0.04; p = 0.014] and Chla concentration [R2 (adj.) = 0.03; p = 0.023]. Significant spatial patterning (dbMEM: p = 0.0001) were also evident in the data (Figure 4A). The first pattern (Axis 1) correlated significantly with SSHA [p = 0.001; R2 (adj.) = 0.29]. Both dbMEM and environmental variables remained significant following variance partitioning (Figure 3). Pearson’s correlation between Hellinger and geographic distances showed a significant positive relationship between samples collected in August and September (Table 3), indicating that samples at greater distances from each other were less similar in terms of myctophid assemblage composition. No other results were significant.
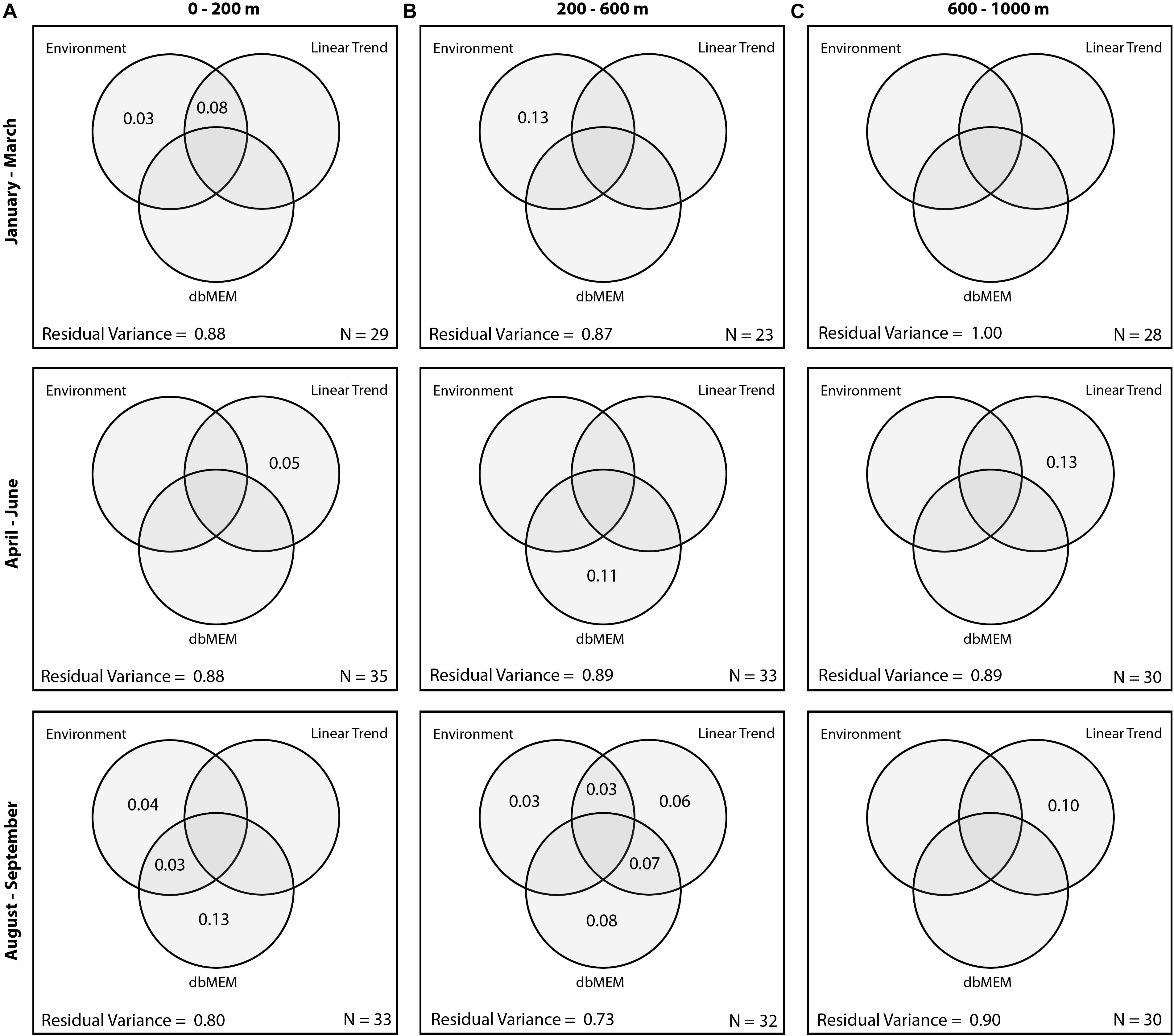
Figure 3. Venn diagrams showing the relative variance explained by significant environmental and spatial variables at (A) 0–200 m (Night); (B) 200–600 m (Day); (C) 600–1000 m (Day).
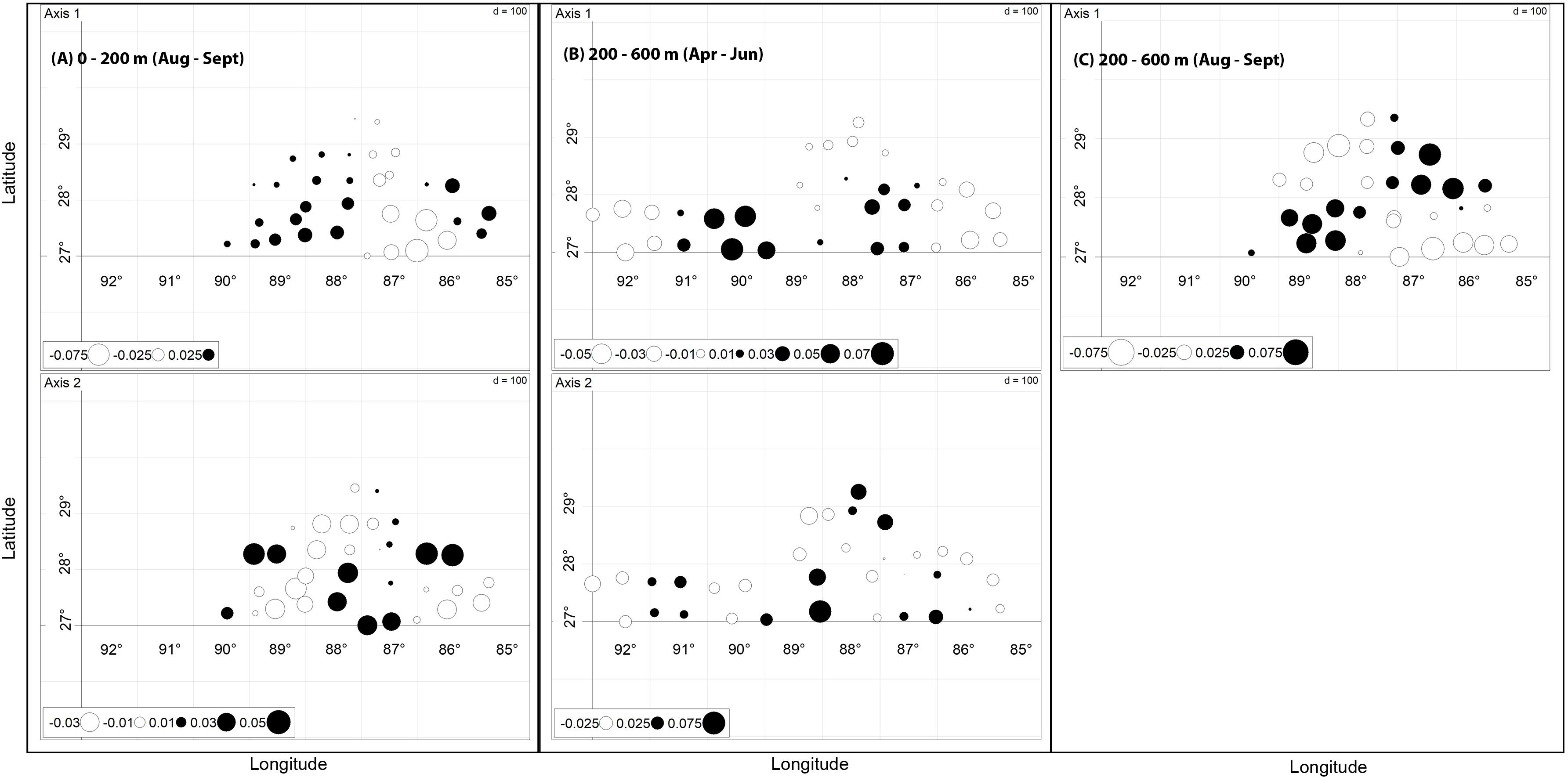
Figure 4. dbMEMs showing significant correlation to myctophid assemblage composition following RDA in (A) 0–200 m (August–September); (B) 200–600 m (April–June); (C) 200–600 m (August–September). In (A,B) two significant axes were identified; in (C) only a single significant axis was identified. Circle color and size represents the values of the eigenvalues that model positive spatial correlation and indicate the scales of spatial patterning in the assemblage data.
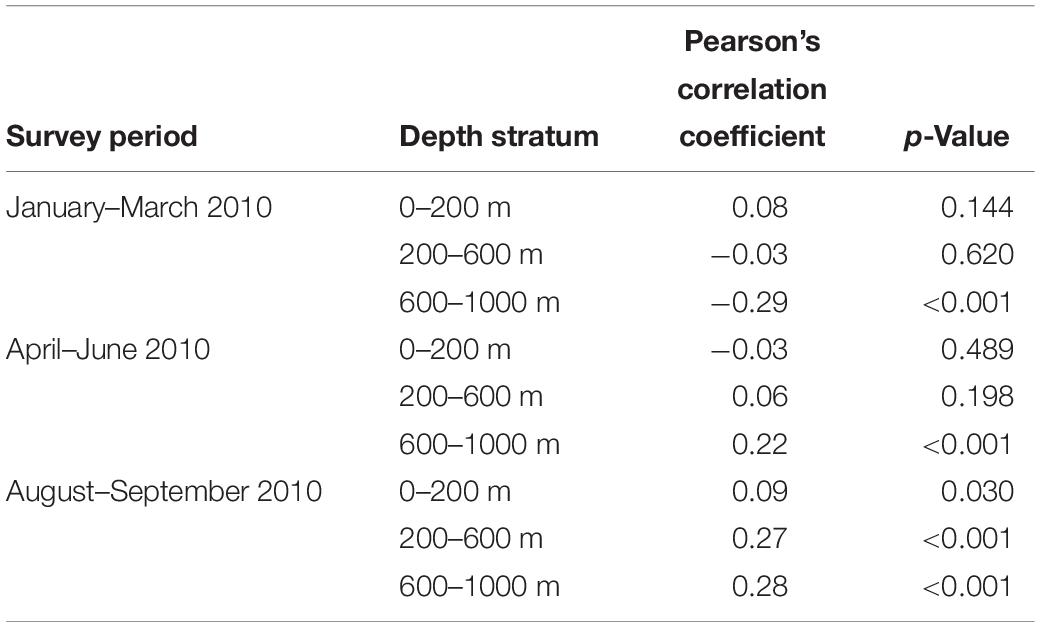
Table 3. Summary of the correlation between Hellinger distance (dissimilarity) and geographic (Euclidian) distance for samples collected from each survey period and depth stratum.
Upper Mesopelagic Depths (200–600 m; Day)
Between January and March 2011, the composition of myctophids between 200 and 600 m was significantly correlated with both TUM [R2 (adj.) = 0.07; p = 0.01] and SSHA [R2 (adj.) = 0.06; p = 0.001]. Significant spatial patterning was evident in April–June [dbMEM: R2 (adj.) = 0.11; p = 0.04; Figure 4B]. The finer-scale pattern was significantly correlated with SEPI (p = 0.005) and TUM (p = 0.002; overall adj. R2 = 0.33). In August and September, myctophid assemblage composition was significantly correlated with a latitudinal trend [R2 (adj.) = 0.05; p = 0.013], and with SSHA [R2 (adj.) = 0.08; p = 0.012] and distance to the 200 m isobath [R2 (adj.) = 0.06; p = 0.019]. A marginally significant spatial pattern (dbMEM: p = 0.045) was also evident in the data (Figure 4C), which correlated significantly with SSHA [p = 0.004, R2 (adj.) = 0.28], Chla concentration (p = 0.040) and TUM [p = 0.047; overall adj. R2 (adj.) = 0.27]. All dbMEM, latitudinal trend and environmental variables remained significant following variance partitioning (Figure 3). Pearson’s correlation between Hellinger and geographic distances showed a significant positive relationship between samples collected in August and September (Table 3), indicating that samples at greater distances from each other were less similar in terms of myctophid assemblage composition. No other results were significant.
Lower Mesopelagic Depths (600–1000 m; Day)
Myctophid assemblage composition in lower mesopelagic depths were significantly correlated with latitude [R2 (adj.) = 0.13; p < 0.001] between April and June, and with both latitude [R2 (adj.) = 0.055; p = 0.01] and longitude [R2 (adj.) = 0.095; p = 0.001] in August and September (Figure 3). Pearson’s correlation between Hellinger and geographic distances showed significant relationships between samples collected in all survey periods, with a negative correlation observed between January and March, and positive correlations between April and September (Table 3). No other results were significant.
Local Contribution to Beta Diversity (LCBD)
Local contribution to beta diversity scores indicated that five samples from epipelagic depths, and four samples from upper mesopelagic depths were significantly different to the other samples within each survey period (Figure 5). One additional sample from the upper mesopelagic was marginally significant (p = 0.056). No samples from lower mesopelagic depths were significantly different to the others (Figure 5). LMs indicated that LCBD scores correlated negatively with SSHA only (Supplementary Table 1). Samples with significantly different LCBD scores occurred with SSHA was in the range −0.37 and −0.13 m, compared with a range of −0.37 to 0.45 m for the remaining samples. LMs also indicated that high LCBD scores were correlated with lower relative abundances of the seven most abundant myctophid species, plus Notoscopelus resplendens, Hygophum taaningi, and Lampanyctus lineatus (Supplementary Table 2).
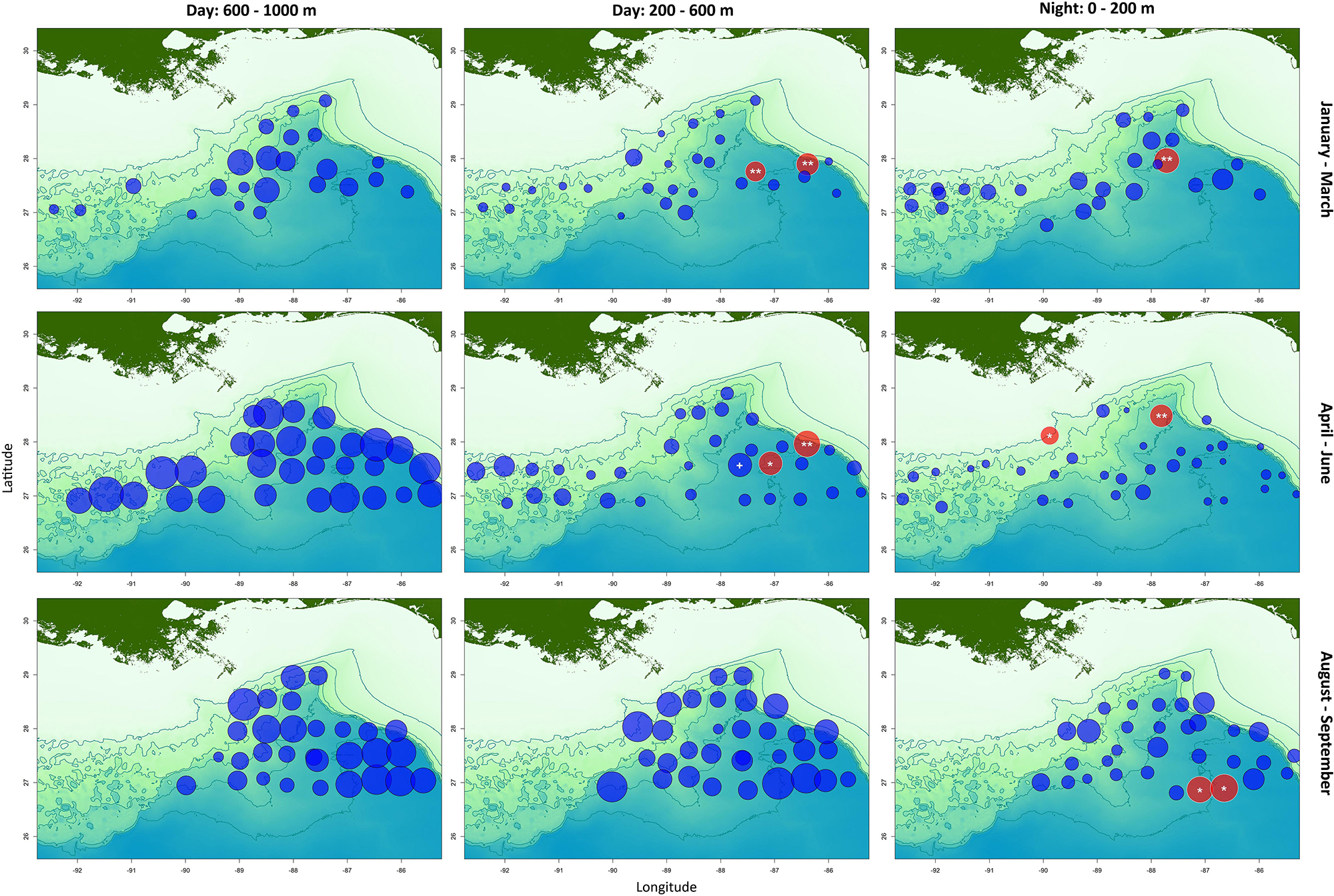
Figure 5. Local contribution to beta diversity (LCBD) scores of each sample, subdivided by depth band and cruise program. Red circles indicate samples that are significantly different to the mean community; blue circles are samples that are not significantly different. The radii of the circles correspond to the LCBD scores. The significance (with Holm correction) is indicated as: +p < 0.1; *p < 0.05; **p < 0.01.
Discussion
This study examined the spatial distributions of the myctophid assemblage in the northern Gulf of Mexico over a 9-month period in 2011 in relation to Loop Current origin water and a summer plume of low-salinity coastal and riverine runoff. Overall, the myctophid assemblage composition showed limited horizontal structuring at the spatial and temporal scales considered, suggesting that discrete, geographically maintained assemblages are not maintained in the northern Gulf, and that the greater assemblage can be treated as a single unit. The dbMEM and RDA analyses indicated that environmental and spatial variables together explained an average of 12% (range: 0–27%) of the variance in the myctophid beta diversity (i.e., the variation in assemblage composition between samples) over the 9-month survey period, but that the significant environmental variables and spatial patterns varied with both water depth and time of year. We found no evidence to suggest that Loop Current eddies contained a unique myctophid fauna compared to the rest of the GoM. While relatively little is known about the biodiversity patterns of mesopelagic fauna in general, these findings are similar to those reported in other oceans when examined at the sub-ecoregion (sensu Spalding et al., 2007; Sutton et al., 2017) level. For example, Olivar et al. (2016) determined that physical and chemical variables explained only 10% of the variance in myctophid composition across the Atlantic, Indian and Pacific Oceans, with 17% of the variance explained by spatial variables.
Within the upper 600 m, the results suggested significant, albeit weak, effects of variables associated with Loop Current eddies and low-salinity coastal water between January and March, and August and September. It is notable that the Loop Current eddy-associated variables were not significant between April and June, when the strongest Loop Current eddy conditions occurred within the survey region (Figure 2). Higher LCBD scores were associated with lower SSHA values, which can be indicative of cyclonic eddies (Vukovich, 2007). As upwelling features, cyclonic eddies would contain cooler water than the surrounding Gulf Common Water however, which was not evident from the TUW data. It may be that the discrepancy reflects the temporal scales measured by each metric, where the monthly-averaged SSHA could have provided an indication of previous oceanographic conditions (or the formation of new conditions) at each sample site that were not detected in situ, but which nonetheless influenced faunal distributions enough for a residual (or pre-emptive) effect to be detected. However, given the relatively small number of samples (N = 9) identified as having a significantly different faunal composition, it is possible that these findings could simply reflect the patchy distributions of micronekton in the water column (e.g., Benoit-Bird and Au, 2003) and further, targeted study of these features is needed.
In the lower mesopelagic zone (600–1000 m), patterns of beta diversity were simpler and only significantly structured by linear spatial gradients, which explained 10–13% of the variation in species composition, though significant positive and negative correlations between Hellinger distance and geographic distance were identified in all samples collected from 600 to 1000 m. The LCBD analyses however suggested that the myctophid assemblage below 600 m was homogeneous across the northern GoM at the time of the study. Circulation patterns within the GoM are believed to comprise of an upper layer and a lower layer that become effectively decoupled from each other at c. 800–1200 m depth (Hamilton and Lugo-Fernandez, 2001). While further work is needed, it is nonetheless possible that the transition from an upper- to lower-level circulation pattern may explain the different spatial patterns observed in the lower mesopelagic samples compared to those collected above 600 m, where the influence of Loop Current origin waters is strongest. Taken together, our findings indicate that the myctophid assemblage within the upper 1000 m of the GoM showed only weak horizontal structuring over the study period at the spatial (75–700 km) and temporal (<3 months) scales considered.
Significant broad-scale spatial structuring was identified between April and September (0–600 m) that was not fully explained by the measured environmental variables. While it is important not to over-interpret the patterns observed from a small number of dbMEMs (Legendre and Legendre, 2012), there are several possible explanations that may account for some of the observed spatial structuring, including unmeasured or “historic” environmental conditions, or biotic and stochastic processes that are not under environmental control (Legendre and Legendre, 2012). Current speed and direction were not included in the present study for example, but convergence or divergence fronts could feasibly explain some additional portion of the variance observed in the assemblage structure by physically aggregating or dispersing the fauna, respectively (Olson et al., 1994; Potier et al., 2014). Similarly, better understanding of circulatory patterns in the deep GoM may also provide insight into the spatial structuring of the micronekton in lower mesopelagic and bathypelagic (>1000 m) depths. It is also possible that the myctophid fauna were less affected by their immediate conditions than by a set of conditions that they encountered at some earlier point in time, leading to detection of a residual effect. Biotic responses may include inter-specific interactions such as competition for resources (e.g., with other zooplanktivorous organisms (e.g., engraulid fishes) that may be seasonally abundant), or predator-prey relationships, which are likely to be important in structuring mesopelagic assemblages at fine spatial (meters) and temporal (minutes–hours) scales (Benoit-Bird and McManus, 2014; Koslow et al., 2014). Alternatively, the observed differences in rank dominance of myctophid species between sampling campaigns may be indicative of underlying seasonal changes in the myctophid assemblage related to their underlying population dynamics for example.
One explanation for the weak horizontal structuring within the myctophid assemblage is the high dispersal potential of pelagic organisms. With few hard barriers to dispersal, mobile pelagic organisms have relatively unrestricted access to all parts of their environment (given sufficient time; Heino et al., 2015). In a recent review, Gaither et al. (2016) highlighted that most circumtropical fishes are pelagic and deep-living (i.e., occurring below 200 m), with myctophids comprising 17% of all known circumtropical species. Gaither et al. (2016) suggested that highly dispersed taxa tended to be those with highly mobile adult stages and which are habitat generalists (or prefer widely-distributed habitats), and that passive transport of planktonic pelagic species and larvae by oceanic currents is also likely to promote dispersal (Gaither et al., 2016; Allen et al., 2018). Both larval and adult myctophids are pelagic, and while their small size may preclude long-distance swimming, their ability to undertake DVMs and onshore-offshore migrations (Benoit-Bird and Au, 2006) indicates that they are capable of actively choosing favorable environmental conditions at scales of at least 100–1000s m, and of tolerating a wide range of environmental conditions associated with changing depth (e.g., temperature, salinity, pressure, light levels).
Changing vertical distributions may also play an important role in promoting horizontal dispersal. Ontogenetic changes in vertical distributions are common amongst myctophids, with many species having non-migratory, epipelagic larval and juvenile stages, with adults moving into progressively deeper waters as they age (e.g., Badcock and Merrett, 1976; Gartner et al., 2008). Adult myctophids also typically perform DVMs to feed and reproduce in the surface waters, but the proportions of individuals migrating on a given day can be highly variable (Watanabe et al., 1999) and may be influenced by environmental (Badcock and Merrett, 1976; Linkowski, 1996; Ekau et al., 2010; Drazen et al., 2011) or biotic (e.g., Angel and Pugh, 2000) conditions. Additionally, since the speed and direction of water currents in the upper 1000 m of the GoM vary with depth (Jochens and DiMarco, 2008), vertically-migrating species have the potential to be passively moved relatively large horizontal distances, at different speeds and in different directions depending on their preferred vertical distribution at any given life-history stage. All these traits could promote dispersal, and lead to a more homogeneous assemblage than would be observed if species were structured only by environmental variables (Heino et al., 2015), or if species maintained geographic integrity via active station-keeping. In a recent circumglobal study, Villarino et al. (2018) came to a similar conclusion regarding the relative dispersal potential of myctophid fishes, where passive drift was hypothesized to dominate their distribution patterns at small sizes, with vertical migration rather than horizontal movements dominating their dispersal behaviors as adults.
While the results presented here indicate that myctophid distributions are not directly driven by environmental parameters at the scales studied, it is possible that some drivers may not be detected by the methods used in this study. By using Hellinger distances in the analyses, we implicitly define “assemblage composition” as the relative abundance of species within a given sample. Changes in the relative abundance species within samples are accounted for, but differences in absolute abundance between samples are not. In the GoM, Loop Current eddies have been previously associated with a lower abundance and biomass of pelagic fauna (if not explicitly myctophids; Zimmerman and Biggs, 1999; Wells et al., 2017), but these differences would not be detected by the methods used here, and will require further study to quantify.
Assuming that high dispersal and mixing rates drive myctophid assemblage distributions within the northern GoM, it is possible to make some inferences about how offshore assemblages may be impacted by stressors and disturbance events. Within the GoM, such information is particularly useful for understanding any potential impacts from the Deepwater Horizon oil spill on the deep-pelagic fauna, given the lack of pre-spill data for the region, and in predicting how offshore ecosystems may be impacted by future spills. For example, metacommunity theory suggests that assemblages with high dispersal rates are likely to be more resilient because individuals can recolonize local areas rapidly after a disturbance event (Heino, 2013). However, the extent to which “local areas” or refugia might exist within the pelagic realm is unclear. Physical and chemical boundaries (e.g., pycnoclines) may preclude the mixing of spilled oil to some extent, but these are not hard barriers to micronekton movements. If a disturbance is detectable by the fauna, but not immediately lethal, mobile individuals may be able to move away from the impacted area before it has a major impact on their health. Such avoidance behavior has been observed in mobile demersal fishes exposed to oil spills in other regions (Elmgren et al., 1983; Law and Kelly, 2004), but it is unclear how well micronekton would be able to actively avoid impacted areas, particularly on the scale of DWHOS. In open ecosystems like those in the pelagic realm, local or point-source disturbances could have a greater footprint than they might in more enclosed ecosystems. It is also possible that high dispersal rates in a pelagic system could lead to increased rates of lethal or sub-lethal exposure than would be expected in more static or enclosed ecosystems, since a greater number of individuals could move into (and out of) the disturbed area over the duration of the event (Heino, 2013; Heino et al., 2015).
The present study makes use of an exceptionally large dataset of deep-pelagic fishes, and provides the first spatially-explicit analysis of myctophid beta diversity patterns in the GoM. While these findings represent a snapshot view of the conditions and fauna during a single year, they nonetheless provide novel insights into how deep-pelagic biodiversity is structured and provide support for the idea that myctophid assemblages are well-mixed and highly dispersed. We anticipate that these findings will provide a useful basis upon which to build further analyses examining seasonal and inter-annual changes in the abundance and biodiversity patterns of deep-pelagic fishes, as well as further exploration of the importance of biotic interactions and behavioral choices amongst the fauna. Given the importance of myctophids in delivering vital ecosystem services such as carbon sequestration from the surface ocean to depth, and as prey for numerous commercially and ecologically important species (e.g., St. John et al., 2016), we anticipate that these findings will also provide valuable, necessary scientific data upon which to build reliable offshore management strategies to protect the functioning and resilience of deep-pelagic ecosystems.
Data Availability Statement
The faunal data used in this study are publicly available through the Gulf of Mexico Research Initiative Information & Data Cooperative (GRIIDC) at https://data.gulfresearchinitiative.org (10.7266/N7VX0DK2). Additional data were sourced from the E.U. Copernicus Marine Service (GLOBAL_ANALYSIS_ FORECAST_PHY_001_024), the GEBCO_2014 Grid (version 20141103), and NASA’s Ocean Biology Processing Group (10.5067/AQUA/MODIS/L3M/CHL/2018).
Author Contributions
TS designed and conducted the surveys presented here, and identified the myctophid specimens. RM conducted the data analyses, prepared the figures, and wrote the first manuscript draft. Both authors reviewed and edited the final draft.
Funding
This research was funded in part by the NOAA Office of Response and Restoration and in part by a grant from the Gulf of Mexico Research Initiative (GoMRI).
Conflict of Interest
The authors declare that the research was conducted in the absence of any commercial or financial relationships that could be construed as a potential conflict of interest.
Acknowledgments
We would like to thank A. Cook for her assistance and data management during both the ONSAP program. We also thank K. Bowen, K. Lord, L. Malarkey, M. Novotny, A. Pickard, and N. Pruzinsky for assistance with quantitative sample processing. We would also like to thank the crew and scientific staff of the M/V Meg Skansi and R/V Point Sur.
Supplementary Material
The Supplementary Material for this article can be found online at: https://www.frontiersin.org/articles/10.3389/fmars.2020.00015/full#supplementary-material
References
Allen, R. M., Metaxas, A., and Snelgrove, P. V. R. (2018). Applying movement ecology to marine animals with complex life cycles. Ann. Rev. Mar. Sci. 10, 19–42. doi: 10.1146/annurev-marine-121916-063134
Angel, M. V., and Pugh, P. R. (2000). Quantification of diel vertical migration by micronektonic taxa in the northeast Atlantic. Hydrobiologia 40, 161–179. doi: 10.1007/978-94-017-1982-7_16
Badcock, J., and Merrett, N. R. (1976). Midwater fishes in the eastern north Atlantic–1. Prog. Oceanogr. 7, 3–58. doi: 10.1016/0079-6611(76)90003-3
Barnett, M. A. (1984). Mesopelagic fish zoogeography in the central tropical and sub-tropical Pacific-ocean – species composition and structure at representative locations in 3 ecosystems. Mar. Biol. 82, 199–208. doi: 10.1007/bf00394103
Beamish, R. J., Leask, K. D., Ivanov, O. A., Balanov, A. A., Orlov, A. M., and Sinclair, B. (1999). The ecology, distribution, and abundance of midwater fishes of the Subarctic Pacific gyres. Prog. Oceanogr. 43, 399–442. doi: 10.1016/s0079-6611(99)00017-8
Benoit-Bird, K. J., and Au, W. W. L. (2003). Spatial dynamics of a nearshore, micronekton sound-scattering layer. ICES J. Mar. Sci. 60, 899–913. doi: 10.1016/S1054-3139(03)00092-4
Benoit-Bird, K. J., and Au, W. W. L. (2006). Extreme diel horizontal migrations by a tropical nearshore resident micronekton community. Mar. Ecol. Prog. Ser. 319, 1–14. doi: 10.3354/meps319001
Benoit-Bird, K. J., and McManus, M. A. (2014). A critical time window for organismal interactions in a pelagic ecosystem. PLoS One 9:e97763. doi: 10.1371/journal.pone.0097763
Biggs, D. C. (1992). Nutrients, plankton, and productivity in a warm-core ring in the western Gulf of Mexico. J. Geophys. Res. Oceans 97, 2143–2154.
Biggs, D. C., and Muller-Karger, F. E. (1994). Ship and satellite-observations of chlorophyll stocks in interacting cyclone-anticyclone eddy pairs in the western Gulf of Mexico. J. Geophys. Res. Oceans 99, 7371–7384.
Biggs, D. C., and Ressler, P. H. (2001). Distribution and abundance of phytoplankton, zooplankton, ichthyoplankton, and micronekton in the deepwater Gulf of Mexico. Gulf Mex. Sci. 19, 7–29.
Blanchet, F. G., Legendre, P., and Borcard, D. (2008). Forward selection of explanatory variables. Ecology 89, 2623–2632. doi: 10.1890/07-0986.1
Camilli, R., Reddy, C. M., Yoerger, D. R., Van Mooy, B. A. S., Jakuba, M. V., Kinsey, J. C., et al. (2010). Tracking hydrocarbon plume transport and biodegradation at Deepwater Horizon. Science 330, 201–204. doi: 10.1126/science.1195223
Chambers, J. M. (2013). Functions and Examples for Software for Data Analysis. R Package Version 1.0-6.
Dormann, C. F., Mcpherson, J. M., Araujo, M. B., Bivand, R., Bollinger, J., Carl, G., et al. (2007). Methods to account for spatial autocorrelation in the analysis of species distributional data: a review. Ecography 30, 609–628. doi: 10.1111/j.2007.0906-7590.05171.x
Dray, S. (2013). Packfor: Forward Selection with Permutation (Canoco p.46). R Package Version 0.0-8/r109. Available at: https://R-Forge.R-project.org/projects/sedar/ (accessed December 17, 2019).
Dray, S., Blanchet, G., Borcard, D., Guenard, G., Jombart, T., and Larocque, G. (2016). Adespatial: Multivariate Multiscale Spatial Analysis, R Package Version 0.0-7. Available at: https://CRAN.R-project.org/package=adespatial (accessed December 17, 2019).
Dray, S., Legendre, P., Borcard, D., and Blanchet, G. (2015). PCNM Spatial Eigenfunctions. Available at: http://r-forge.r-project.org/R/?group_id=195 (accessed June 9, 2016).
Dray, S., Legendre, P., and Peres-Neto, P. R. (2006). Spatial modelling: a comprehensive framework for principal coordinate analysis of neighbour matrices (PCNM). Ecol. Modell. 196, 483–493. doi: 10.1016/j.ecolmodel.2006.02.015
Drazen, J. C., De Forest, L. G., and Domokos, R. (2011). Micronekton abundance and biomass in Hawaiian waters as influenced by seamounts, eddies, and the moon. Deep Sea Res. Part I Oceanogr. Res. Pap. 58, 557–566. doi: 10.1016/j.dsr.2011.03.002
Ekau, W., Auel, H., Poertner, H. O., and Gilbert, D. (2010). Impacts of hypoxia on the structure and processes in pelagic communities (zooplankton, macro-invertebrates and fish). Biogeosciences 7, 1669–1699. doi: 10.5194/bg-7-1669-2010
Elliott, B. A. (1982). Anticyclonic rings in the Gulf of Mexico. J. Phys. Oceanogr. 12, 1292–1309. doi: 10.1175/1520-0485(1982)012<1292:aritgo>2.0.co;2
Elmgren, R., Hansson, S., Larsson, U., Sundelin, B., and Boehm, P. D. (1983). The Tsesis oil-spill – acute and long-term impact on the benthos. Mar. Biol. 73, 51–65. doi: 10.1007/bf00396285
Fricke, R., Eschmeyer, W. N., and Van der Laan, R. (eds) (2019). Eschmeyer’s Catalog of Fishes: Genera, Species, References. Electronic Version. Available at: http://researcharchive.calacademy.org/research/ichthyology/catalog/fishcatmain.asp (accessed July 20, 2019).
Gaither, M. R., Bowen, B. W., Rocha, L. A., and Briggs, J. C. (2016). Fishes that rule the world: circumtropical distributions revisited. Fish Fish. 17, 664–679. doi: 10.1111/faf.12136
Gartner, J. V. Jr., Sulak, K. J., Ross, S. W., and Necaise, A. M. (2008). Persistent near-bottom aggregations of mesopelagic animals along the North Carolina and Virginia continental slopes. Mar. Biol. 153, 825–841. doi: 10.1007/s00227-007-0855-1
Gartner, J. V., Hopkins, T. L., Baird, R. C., and Milliken, D. M. (1987). The lanternfishes (pisces, myctophidae) of the eastern Gulf of Mexico. Fis. Bull. 85, 81–98.
Gjosaeter, J., and Kawaguchi, K. (1980). A Review of the World Resources of Mesopelagic Fish Fao Fisheries Technical Paper No. 193. Rome: Food and Agriculture Organization of the United Nations, 1–151.
Godo, O. R., Samuelsen, A., MacAulay, G. J., Patel, R., Hjollo, S. S., and Horne, J. (2012). Mesoscale eddies are oases for higher trophic marine life. PLoS One 7:e30161. doi: 10.1371/journal.pone.0030161
Hamilton, P., and Lugo-Fernandez, A. (2001). Observations of high speed deep currents in the northern Gulf of Mexico. Geophys. Res. Lett. 28, 2867–2870. doi: 10.1029/2001gl013039
Heino, J. (2013). The importance of metacommunity ecology for environmental assessment research in the freshwater realm. Biol. Rev. 88, 166–178. doi: 10.1111/j.1469-185X.2012.00244.x
Heino, J., Melo, A. S., Siqueira, T., Soininen, J., Valanko, S., and Mauricio Bino, L. (2015). Metacommunity organisation, spatial extent and dispersal in aquatic systems: patterns, processes and prospects. Freshw. Biol. 60, 845–869. doi: 10.1111/fwb.12533
Herring, H. J. (2010). Gulf of Mexico Hydrographic Climatology and Method of Synthesizing Subsurface Profiles From the Satellite Sea Surface Height Anomaly. Report No. 122. Silver Spring, MD: United States Department of Commerce National Oceanographic and Atmospheric Administration.
Irigoien, X., Klevjer, T. A., Rostad, A., Martinez, U., Boyra, G., and Acuna, J. L. (2014). Large mesopelagic fishes biomass and trophic efficiency in the open ocean. Nat. Commun. 5:3271. doi: 10.1038/ncomms4271
Jochens, A. E., and DiMarco, S. F. (2008). Physical oceanographic conditions in the deepwater Gulf of Mexico in summer 2000–2002. Deep Sea Res. II 55, 2541–2554. doi: 10.1016/j.dsr2.2008.07.003
Johnston, M. W., Milligan, R. J., Easson, C. G., Derada, S., English, D. C., and Penta, B. (2019). An Empirically-validated method for characterizing pelagic habitats in the Gulf of Mexico using ocean model data. Limnol. Oceanogr. Methods 17, 362–375.
Kaartvedt, S., Staby, A., and Aksnes, D. L. (2012). Efficient trawl avoidance by mesopelagic fishes causes large underestimation of their biomass. Mar. Ecol. Prog. Ser. 456, 1–6. doi: 10.3354/meps09785
Koslow, J. A., Davison, P., Lara-Lopez, A., and Ohman, M. D. (2014). Epipelagic and mesopelagic fishes in the southern California current system: ecological interactions and oceanographic influences on their abundance. J. Mar. Syst. 138, 20–28. doi: 10.1016/j.jmarsys.2013.09.007
Law, R. J., and Kelly, C. (2004). The impact of the “Sea Empress” oil spill. Aquat. Living Resour. 17, 389–394. doi: 10.1051/alr:2004029
Legendre, P., Borcard, D., and Peres-Neto, P. R. (2005). Analyzing beta diversity: partitioning the spatial variation of community composition data. Ecol. Monogr. 75, 435–450. doi: 10.1890/05-0549
Legendre, P., and de Caceres, M. (2013). Beta diversity as the variance of community data: dissimilarity coefficients and partitioning. Ecol. Lett. 16, 951–963. doi: 10.1111/ele.12141
Legendre, P., and Gallagher, E. D. (2001). Ecologically meaningful transformations for ordination of species data. Oecologia 129, 271–280. doi: 10.1007/s004420100716
Linkowski, T. B. (1996). Lunar rhythms of vertical migrations coded in otolith microstructure of North Atlantic lanternfishes, genus Hygophum (Myctophidae). Mar. Biol. 124, 495–508. doi: 10.1007/bf00351031
Morey, S. L., Schroeder, W. W., O’Brien, J. J., and Zavala-Hidalgo, J. (2003). The annual cycle of riverine influence in the eastern Gulf of Mexico basin. Geophys. Res. Lett. 30:1867.
Nasa Goddard Space Flight Center, Ocean Ecology Laboratory, Ocean Biology Processing Group (2018). Moderate-Resolution Imaging Spectroradiometer (MODIS) Aqua Chlorophyll Data 2018 Reprocessing. Greenbelt, MD: NASA (OB.DAAC). doi: 10.5067/AQUA/MODIS/L3M/CHL/2018
Oey, L. Y., Lee, H. C., and Schmitz, W. J. (2003). Effects of winds and Caribbean eddies on the frequency of loop current eddy shedding: a numerical model study. J. Geophys. Res. Oceans 108:3324.
Oksanen, J., Blanchet, G., Friendly, M., Kindt, R., Legendre, P., McGlinn, D. et al. (2017). vegan: Community Ecology Package. R Package Version 2.4-2. Available at: https://CRAN.R-project.org/package=vegan
Olivar, M. P., Gonzalez-Gordillo, J. I., Salat, J., Chust, G., Cozar, A., Hernandez-Leon, S., et al. (2016). The contribution of migratory mesopelagic fishes to neuston fish assemblages across the Atlantic. Indian Pac. Oceans Mar. Freshw. Res. 67, 1114–1127.
Olson, D. B., Hitchcock, G. L., Mariano, A. J., Ashjian, C. J., Peng, G., Nero, R. W., et al. (1994). Life on the edge: marine life and fronts. Oceanography 7, 52–60. doi: 10.1016/j.marenvres.2018.09.010
Peres-Neto, P. R., Legendre, P., Dray, S., and Borcard, D. (2006). Variation partitioning of species data matrices: estimation and comparison of fractions. Ecology 87, 2614–2625. doi: 10.1890/0012-9658(2006)87%5B2614:vposdm%5D2.0.co;2
Potier, M., Bach, P., Menard, F., and Marsac, F. (2014). Influence of mesoscale features on micronekton and large pelagic fish communities in the Mozambique channel. Deep Sea Res. Part II Top. Stud. Oceanogr. 100, 184–199. doi: 10.1016/j.dsr2.2013.10.026
Pusineri, C., Chancollon, O., Ringelstein, J., and Ridoux, V. (2008). Feeding niche segregation among the Northeast Atlantic community of oceanic top predators. Mar. Ecol. Prog. Ser. 361, 21–34. doi: 10.3354/meps07318
R Core Team (2018). R: A Language and Environment for Statistical Computing. Vienna: R Foundation for Statistical Computing.
Rivas, D., Badan, A., and Ochoa, J. (2005). The ventilation of the deep Gulf of Mexico. J. Phys. Oceanogr. 35, 1763–1781. doi: 10.1175/jpo2786.1
Robinson, C., Steinberg, D. K., Anderson, T. R., Aristegui, J., Carlson, C. A., Frost, J. R., et al. (2010). Mesopelagic zone ecology and biogeochemistry – a synthesis. Deep Sea Res. Part II Top. Stud. Oceanogr. 57, 1504–1518. doi: 10.1016/j.dsr2.2010.02.018
Spalding, M. D., Fox, H. E., Allen, G. R., Davidson, N., Ferdaña, Z. A., Finlayson, M., et al. (2007). Marine ecoregions of the world: a bioregionalization of coastal and shelf areas. Bioscience 57, 573–583. doi: 10.1641/b570707
St. John, M. A., Borja, A., Chust, G., Heath, M., Grigorov, I., and Mariani, P. (2016). A dark hole in our understanding of marine ecosystems and their services: perspectives from the mesopelagic community. Front. Mar. Sci. 3:31. doi: 10.3389/fmars.2016.00031
Sutton, T. T. (2013). Vertical ecology of the pelagic ocean: classical patterns and new perspectives. J. Fish Biol. 83, 1508–1527. doi: 10.1111/jfb.12263
Sutton, T. T., Clark, M. R., Dunn, D. C., Halpin, P. N., Rogers, A. D., and Guinotte, J. (2017). A global biogeographic classification of the mesopelagic zone. Deep Sea Res. Part 1 Oceanogr. Res. Pap. 126, 85–102. doi: 10.1016/j.dsr.2017.05.006
Sutton, T. T., and Hopkins, T. L. (1996). Trophic ecology of the stomiid (Pisces: Stomiidae) fish assemblage of the eastern Gulf of Mexico: strategies, selectivity and impact of a top mesopelagic predator group. Mar. Biol. 127, 179–192. doi: 10.1007/bf00942102
Tilman, D., Isbell, F., and Cowles, J. M. (2014). “Biodiversity and ecosystem functioning,” in Annual Review of Ecology, Evolution, and Systematics, Vol 45, ed. D. J. Futuyma (Palo Alto, CA: Annual Review).
Villarino, E., Watson, J. R., Jonsson, B., Gasol, J. M., Salazar, G., and Acinas, S. G. (2018). Large-scale ocean connectivity and planktonic body size. Nat. Commun. 9:142. doi: 10.1038/s41467-017-02535-8
Vukovich, F. M. (2007). Climatology of ocean features in the Gulf of Mexico using satellite remote sensing data. J. Phys. Oceanogr. 37, 689–707. doi: 10.1175/jpo2989.1
Watanabe, H., Moku, M., Kawaguchi, K., Ishimaru, K., and Ohno, A. (1999). Diel vertical migration of myctophid fishes (Family Myctophidae) in the transitional waters of the western North Pacific. Fish. Oceanogr. 8, 115–127. doi: 10.1046/j.1365-2419.1999.00103.x
Webb, T. J., Vanden Berghe, E., and O’Dor, R. (2010). Biodiversity’s big wet secret: the global distribution of marine biological records reveals chronic under-exploration of the deep pelagic ocean. PLoS One 5:e1022. doi: 10.1371/journal.pone.0010223
Wells, R. J. D., Rooker, J. R., Quigg, A., and Wissel, B. (2017). Influence of mesoscale oceanographic features on pelagic food webs in the Gulf of Mexico. Mar. Biol. 164:92.
Keywords: beta diversity, deep sea, micronekton, Myctophidae, spatial analysis
Citation: Milligan RJ and Sutton TT (2020) Dispersion Overrides Environmental Variability as a Primary Driver of the Horizontal Assemblage Structure of the Mesopelagic Fish Family Myctophidae in the Northern Gulf of Mexico. Front. Mar. Sci. 7:15. doi: 10.3389/fmars.2020.00015
Received: 31 July 2019; Accepted: 09 January 2020;
Published: 12 February 2020.
Edited by:
Ana Colaço, Marine Research Institute (IMAR), PortugalReviewed by:
Xabier Irigoien, King Abdullah University of Science and Technology, Saudi ArabiaVerena Wang, The University of Southern Mississippi, United States
Copyright © 2020 Milligan and Sutton. This is an open-access article distributed under the terms of the Creative Commons Attribution License (CC BY). The use, distribution or reproduction in other forums is permitted, provided the original author(s) and the copyright owner(s) are credited and that the original publication in this journal is cited, in accordance with accepted academic practice. No use, distribution or reproduction is permitted which does not comply with these terms.
*Correspondence: Rosanna J. Milligan, Ui5NaWxsaWdhbkBub3ZhLmVkdQ==