- 1Ocean Biogeochemistry and Ecosystems, National Oceanography Centre, Southampton, United Kingdom
- 2Imperial College London, Silwood Park, Berkshire, United Kingdom
- 3Department of Arctic and Marine Biology, UiT the Arctic University of Norway, Tromsø, Norway
- 4Department of Marine Sciences, University of Georgia, Athens, GA, United States
- 5British Oceanographic Data Centre, National Oceanography Centre, Liverpool, United Kingdom
- 6Sorbonne Université, CNRS, Laboratoire d'Océanographie de Villefranche, Villefranche-sur-Mer, France
- 7Helmholtz Centre of Polar and Marine Research, Alfred-Wegener-Institut, Bremerhaven, Germany
- 8MARUM and Faculty of Geosciences, University of Bremen, Bremen, Germany
- 9Marine Ecology, GEOMAR Helmholtz Centre for Ocean Research Kiel, Kiel, Germany
- 10Japan Agency for Marine-Earth Science and Technology, Yokosuka, Japan
- 11Federal University of Southern Bahia, Porto Seguro, Brazil
- 12Oceanography Department, University of Alaska Fairbanks, Fairbanks, AK, United States
- 13Institute of Coastal Research, Helmholtz-Zentrum Geesthacht, Geesthacht, Germany
- 14Ocean Sciences, Memorial University of Newfoundland, St. John's, NL, Canada
- 15Southern Ocean Carbon and Climate Observatory, CSIR, Stellenbosch, South Africa
- 16Department of Oceanography, Marine Research Institute, University of Cape Town, Cape Town, South Africa
- 17CSIRO Oceans and Atmosphere, Hobart, TAS, Australia
- 18Department of Oceanography, Ocean Frontier Institute, Dalhousie University, Halifax, NS, Canada
Optical particle measurements are emerging as an important technique for understanding the ocean carbon cycle, including contributions to estimates of their downward flux, which sequesters carbon dioxide (CO2) in the deep sea. Optical instruments can be used from ships or installed on autonomous platforms, delivering much greater spatial and temporal coverage of particles in the mesopelagic zone of the ocean than traditional techniques, such as sediment traps. Technologies to image particles have advanced greatly over the last two decades, but the quantitative translation of these immense datasets into biogeochemical properties remains a challenge. In particular, advances are needed to enable the optimal translation of imaged objects into carbon content and sinking velocities. In addition, different devices often measure different optical properties, leading to difficulties in comparing results. Here we provide a practical overview of the challenges and potential of using these instruments, as a step toward improvement and expansion of their applications.
Introduction
Particulate Matter in the Ocean
Life and particulate organic matter in the ocean have fundamentally shaped our planet. On the most basic level, particulate organic matter can be defined as both living and non-living matter of biological origin with a size of ≥0.2 μm in diameter, including anything from a small bacterium (0.2 μm in size) to blue whales (20 m in size; see review by Blanchard et al., 2017). Organic matter plays a crucial role in regulating marine global biogeochemical cycles and events, from the Great Oxidation Event in Earth's early history (Holland, 2006) to the sequestration of atmospheric carbon dioxide in the deep ocean (Volk and Hoffert, 1985; Heinze et al., 2015). Understanding the distribution, characteristics and dynamics of particulate matter in the ocean is hence fundamental in understanding and predicting the marine ecosystem, from food web dynamics to global biogeochemical cycles. In this review, we focus primarily on the particles and particle processes involved in the biological carbon pump within the mesopelagic zone (the region from below the productive layer to ~1,000 m depth) and on how these can be inferred from optical measurements. The technologies reviewed here can also be applied to other aquatic systems, and to problems other than the biological pump, such as the distribution and fate of microplastic in the ocean or the presence of specific organisms involved in harmful algal blooms.
The Biological Carbon Pump
The biological carbon pump describes the collection of biogeochemical processes associated with the production, sinking, and remineralization of organic carbon in the ocean (Volk and Hoffert, 1985; Giering and Humphreys, 2018). In brief, photosynthesis by microorganisms in the upper tens of meters of the water column fix inorganic carbon (any of the chemical species of dissolved carbon dioxide) into biomass. When this biomass sinks to the deep ocean, a portion of it fuels the metabolism of the organisms living there, including deep-sea fish and benthic organisms (Turner, 2015). Zooplankton play a critical role in shaping particle flux through ingestion and fragmentation of particles (e.g., Waite et al., 2000; Iversen and Poulsen, 2007; Poulsen and Iversen, 2008; Iversen et al., 2010; Giering et al., 2014; Svensen et al., 2014), production of fast-sinking fecal material (e.g., Turner, 2015; Iversen et al., 2017), and active vertical migration (e.g., Steinberg et al., 2000; Jónasdóttir et al., 2015; Kiko et al., 2017).
Besides the importance of “exported” organic carbon as a food source for deep ocean organisms, the biological carbon pump provides a valuable ecosystem function: Exported organic carbon transports an estimated 5–20 Gt C each year to the deep ocean (Henson et al., 2011), where some of it (~0.2–0.5 Gt C) (Guidi et al., 2015) is sequestered for several millennia. The biological carbon pump is hence of similar magnitude to current carbon emissions from fossil fuels (~10 Gt C year−1). Any changes in its magnitude caused by a warming world may have direct implications for both deep-sea organisms and atmospheric carbon dioxide concentrations (Kwon et al., 2009; Passow and Carlson, 2012).
The magnitude and efficiency (amount of carbon sequestered relative to primary production) of the biological carbon pump, hence ocean carbon storage, is partly determined by the amount of organic matter exported and the rate at which it is remineralized (i.e., the rate with which sinking organic matter is reworked and respired in the mesopelagic zone region; Kwon et al., 2009; Iversen and Ploug, 2010; Reygondeau et al., 2018). Especially particle size and composition are important parameters determining how fast a particle sinks (Ploug et al., 2008a; Iversen and Ploug, 2010), how much material it contains (Ploug et al., 2008b), and which organisms can find and utilize it (Kiørboe et al., 1999; Visser, 2001; Visser and Jackson, 2004).
Sinking particles can be phytoplankton, zooplankton, detritus, fecal pellets, or a mix of these (Simon et al., 2002; Turner, 2002, 2015). They range in size from a few micrometers to several centimeters, with particles of a diameter of >0.5 mm being referred to as “marine snow” (Alldredge and Silver, 1988). In general, particles in a fluid are thought to sink once their densities are higher than the ambient fluid, i.e., when excess densities are larger than zero. Larger individual phytoplankton cells can thus contribute to sedimentary fluxes. For example, large diatom cells and diatom chains with a diameter of >5 μm have been shown to sink at rates up to several 10 s meters per day, though this is only possible owing to the heavy ballast of a silica frustule (Waite et al., 1997a; Miklasz and Denny, 2010). Both size and density affect particle sinking velocity; for example, for sinking velocities that follow Stokes' Law, doubling the size of the particle increases the sinking speed by a factor of 4 (Moore and Villareal, 1996; Waite et al., 1997a). However, the highly porous nature of many marine particles means that they do not obey Stokes' Law because small changes in particle density (i.e., compactness) can have a large impact on their sinking velocities (Iversen and Ploug, 2010). Large sinking particles are typically of two types: (1) aggregates formed from a number of primary particles, including phytoplankton, bacteria, fecal pellets, live protozoa and zooplankton and debris, and (2) zooplankton fecal pellets, which can dominate particle flux events and sink at velocities exceeding 1,000 m d−1 (Turner, 2015).
Knowing the size, abundance, structure and composition (e.g. carbon content) of settling particles is important as these characteristics impose fundamental constraints on the biogeochemical cycling of carbon. For example, changes in climate are expected to facilitate a shift in species composition in a manner that alters the elemental composition of particulate matter, cell size and the trajectory of carbon through the food web, influencing the proportion of biomass exported to depth (Finkel et al., 2010). As such, any climate-induced change in the structure or function of phytoplankton communities is likely to alter the efficiency of the biological carbon pump, with feedbacks on the rate of climate change (Matear and Hirst, 1999; Le Quéré et al., 2007).
Autonomous Sampling on the Rise
The vastness of the ocean makes it difficult to accurately estimate the processes involved in the biological carbon pump. Remote sensing via satellites has only limited capabilities as it is restricted to the upper meters of the ocean. To date, our knowledge of the biological carbon pump is based predominantly on data collected by sediment traps (e.g., Honjo, 1996; Francois et al., 2002; Buesseler et al., 2007; Honjo et al., 2008; Fischer et al., 2016), radioactive tracers such as Thorium-234 (e.g., Buesseler et al., 2006; Waite and Hill, 2006; Roca-Martí et al., 2017), and budgets of dissolved biogeochemical tracers such as nutrients (e.g., Schlitzer, 2002; Gehlen et al., 2006). Each of these methods has yielded important insights and has its strengths and weaknesses including limited spatio-temporal coverage and/or resolution or uncertain ocean circulation. Furthermore, carbon flux estimates from these diverse methods often differ widely, and the various spatio-temporal scales of the methods complicate efforts to compare their results (Boyd and Trull, 2007).
The most direct method of measuring particle flux uses sediment traps, which collect sinking particles at a certain depth over a period of several days to months. The collected material is preserved in situ and available for biochemical analysis, including biomarkers. Sediment traps provide useful, quantitative and qualitative estimates of particle fluxes, but the small collection area of a single sediment trap (<1 m2) combined with the low number of traps that can feasibly be deployed complicates extrapolation to mesoscale and broader scale fluxes (Martin et al., 2011). Neutrally buoyant sediment traps, which were designed to overcome hydrodynamic biases and are considered the most accurate trap type (Buesseler et al., 2007), are also limited in temporal coverage to the length of a single oceanographic cruise, preventing the full characterization of seasonal and longer timescales. Full-year coverage is possible with moored sediment traps (e.g., Conte and Weber, 2014), but both spatial coverage and temporal resolution remain limited and questions of over- or under-collection due to hydrodynamic effects complicate interpretation (Yu et al., 2001). Moreover, particles are often pooled in sediment traps, making it hard to characterize the origin, size, and composition of the individual particles. An exception are gel traps, which are traps filled with a viscous, inert gel that slowly decelerates and isolates sinking particles, allowing investigation of individual particles (Jannasch et al., 1980; Waite and Nodder, 2001; Thiele et al., 2015; Flintrop et al., 2018).
Measurements of natural, particle-binding radioisotopes, including Thorium-234 and Polonium-210, can be used to estimate upper-mesopelagic particle fluxes on timescales of weeks to months (e.g., Buesseler, 1998; Le Moigne et al., 2013). These estimates do not, however, provide information about the nature of the particles responsible for the measured fluxes, and rely on assumptions regarding the conversion from radioisotopes to carbon that are difficult to evaluate (Waite and Hill, 2006). Moreover, radioisotopic estimates of particle flux are complicated by potential non-steady state dynamics and physical transport (Ceballos-Romero et al., 2018). Measurements are costly, as they need to be conducted during individual oceanographic cruises, and need to be calibrated with sediment traps.
Large-scale assessments of sinking particles in the marine carbon cycle focus on measuring dissolved biogeochemical tracers such as nutrients, oxygen or pH (e.g., Schlitzer, 2002; Gehlen et al., 2006; DeVries et al., 2012; Weber et al., 2016). These tracers reflect the net production and degradation of organic material combined with processes of particle transport and circulation. Major observational programmes that use dissolved tracers include GO-SHIP (Global Ocean Ship-Based Hydrographic Investigations Program, www.go-ship.org) and SOCCOM (Southern Ocean Carbon and Climate Observations and Modeling, https://soccom.princeton.edu), which uses biogeochemical sensors on profiling floats. The use of dissolved tracers has two advantages; the sensor techniques are relatively advanced, and estimated rates are integrated over space and time thus reducing observational needs. However, these approaches are unlikely to deliver any predictive understanding of how particle fluxes will respond to environmental change as they do not identify the processes that control the sinking and transformation of particles, nor the variability on interannual timescales. The largest global effort to characterize particle fluxes more directly was the Joint Global Ocean Flux Study (JGOFS), which carried out both surveys and process studies over more than a decade (e.g., Buesseler, 2001). It made major advances in connecting surface production to mesopelagic and deep particle fluxes, primarily via biogeochemical measurements, but also by including their correlation with bulk optical properties such as light transmission (e.g., Bishop, 1999; Gardner et al., 2006) and some early applications of photographic imaging techniques (e.g., Walsh and Gardner, 1992; Diercks and Asper, 1997; Bishop et al., 2002).
Recent developments in in situ optical devices for measuring particles offer the opportunity to advance the progress that begun during JGOFS and other programmes. They are now much smaller, require much less power, and offer greater capabilities. Bulk optical property sensors, such as light transmission and scattering are available in multiple frequencies. Imaging systems have greater resolution and can be combined with image processing techniques for particle recognition to estimate particle type, size, and distribution. Optical devices for particle measurements can be used from ships (e.g., Herman, 2004; Davis et al., 2005; Picheral et al., 2010) or installed on remote platforms (e.g., moorings and Argo floats; Checkley et al., 2008; Rembauville et al., 2017). Several systems exist that can be deployed down to 6,000 m depth, and integration into standard CTD-rosettes allows for routine deployments as part of oceanographic surveys. Optical devices for measuring particles can provide high-resolution descriptions of particle abundances, sizes, and types (e.g., fecal pellet, diatom aggregate, mucous structures, zooplankton), which inform about particle origin and formation. The high-frequency spatial and temporal information collected by optical devices now allows inferring particle transformation mechanisms from observations on particle abundance and size-distributions at different water depths or from changes of their morphology over time. Imaging systems are also well-suited to investigate zooplankton-particle interactions, as they often allow for the simultaneous assessment of particles and zooplankton (e.g., Möller et al., 2012; Christiansen et al., 2018). Lastly, the use of optical devices for measuring particles has become increasingly attractive as they are continuing to become more affordable and technically more feasible.
The interpretation of optical measurements can be complicated as different optical devices generally measure distinct optical properties that are difficult to interpret and compare. A lack of standardization in data analysis algorithms further impedes the direct comparison of different datasets. These issues are exacerbated during the translation of optical particle properties into flux estimates owing to a current lack of understanding of how particle optical properties such as size and type relate to particle sinking velocity and carbon content (Kriest, 2002; Guidi et al., 2008; Iversen et al., 2010; McDonnell and Buesseler, 2010; Le Moigne et al., 2013; Laurenceau-Cornec et al., 2015a; Nowald et al., 2015; Ramondenc et al., 2016).
Scope of Review
The advance of optical technology, autonomous ocean systems, and data processing power now promises a major leap in our understanding of the biological carbon pump. An important challenge now is to systematically improve the use of optical devices for measuring particles, which includes the comparison and inter-calibration of the outputs of available optical devices, as well as the collation and distribution of knowledge on how to efficiently convert optical information (abundance, size, and types of particles) into particle flux estimates. In this review, we provide an overview of the general issues that occur when trying to (1) interpret optical in situ measurements of particles in the mesopelagic zone and (2) convert these measurements into fluxes. We briefly present some of the common optical devices used for particle flux characterization in the mesopelagic zone and discuss their capabilities and limitations. A summary of currently commercially available optical devices for plankton research has been compiled by Lombard et al., (2019; Table 1), and most of these devices can be used for particle flux studies. The aim of this review is to give scientists the background needed to maximize the output of these optical devices for estimating particle flux and understanding particle dynamics.
Optical in situ Measurements of Particles
Unlike other measurements such as primary production, oxygen, salinity, or Chlorophyll a, for which there exist standard sampling and analysis protocols, there are currently no standards for optical particle sampling, data analyses and data deposition. Differences exist in data acquisition owing to the various optical devices and techniques, and data processing is often left to personal preferences, including image/signal analyses, classification and conversion algorithms. Hence, there is a great need for standardization to enable comparison of data collected by different instruments or analyzed by different scientists.
Data Acquisition: Particle Detection Methods
The size of a particle determines the detection method that is appropriate. For example, small (<2 μm) phytoplankton are much more abundant than large (>2 mm) zooplankton, so phytoplankton abundance can be measured using relatively small sample volumes. The small particle size makes detailed classification difficult as sufficient resolution of the shape at such small scales is technologically difficult. In situ characterization of small particles is therefore often restricted to estimates of abundance and biomass based on optical “bulk” properties such as transmission, backscatter, and fluorescence.
Large particles are rarer in abundance and contribute less to total biomass than small particles (Sheldon et al., 1972) and therefore require that a larger volume of water is measured. These particles have traditionally been collected with nets, bottles, or pumps, and identified visually based on shape or biochemically based on elements (e.g., diatoms via biogenic silica). The use of imaging systems that build on these classification methods is therefore convenient. Imaging can be based on photographic or holographic technology. Yet, whilst particle size and abundance can be retrieved relatively quickly from images, a more detailed classification still requires time-consuming manual identification. This step will become much faster in the coming years with the rapid advances in machine learning tools.
The decision when to use bulk water properties, like transmission, vs. imaging is fluid. The signal from an imaging system could be interpreted in a similar fashion to “bulk measurements” (i.e., looking at total frame properties rather than specific “regions of interest”), whilst anomalies in bulk signals (e.g., spikes in backscatter output) can be used to infer the size of particles (Briggs et al., 2011, 2013). “Hybrid” systems exist, such as the Laser Optical Particle Counter (LOPC; not commercially available anymore), which can combine several individual “one-pixel” photodetectors to generate two-dimensional information that can be used to investigate particle shape (Jackson and Checkley, 2011; Petrik et al., 2013).
Broadly, we can distinguish between four types of optical device: (a) single photodetectors, (b) simple photodetector arrays, (c) holographic systems, and (d) photographic systems. Box 1 explains the principles behind each of these types. Table 1 gives an overview of example devices for each type, highlighting the target range, classification level, typical sampling volumes, relevant threshold settings, and type of illumination.
Box 1. Basic principles of optical device types.
(a) Single photodetector
A single photodetector measures a bulk optical property, such as optical backscattering or beam attenuation in a volume of water. These properties are empirically correlated with particle concentration in the ocean (e.g., Bishop, 1986; Reynolds et al., 2016) and, where organic particles dominate, particulate organic carbon (POC; e.g., Gardner et al., 2001; Cetinić et al., 2012). While a single photodetector cannot distinguish individual particles within its sample volume, information about particle size can in practice be extracted from a high-resolution time series or vertical profile. This interpretation is possible because a single large particle (~>150 μm) passing through a small sample volume causes a brief jump, or “spike,” in particle concentration (Figure 1, left panel). In the mesopelagic, such particles are generally rare enough relative to the sample volumes of commercial transmissometers that their optical signals can be completely separated from the background of smaller particles (see Figure 1, right panel). Then, either their numerical concentration (Rembauville et al., 2017) or POC concentration (Briggs et al., 2011) can be calculated. When combined with a sinking velocity estimate, the latter can be converted to an estimate of POC flux. Alternatively, spike height can be correlated with particle cross-sectional area, allowing estimates of particle size. This principle has been used to estimate mean particle diameter at high particle concentrations (Briggs et al., 2013), and it could also be applied to individual spikes at lower concentrations.
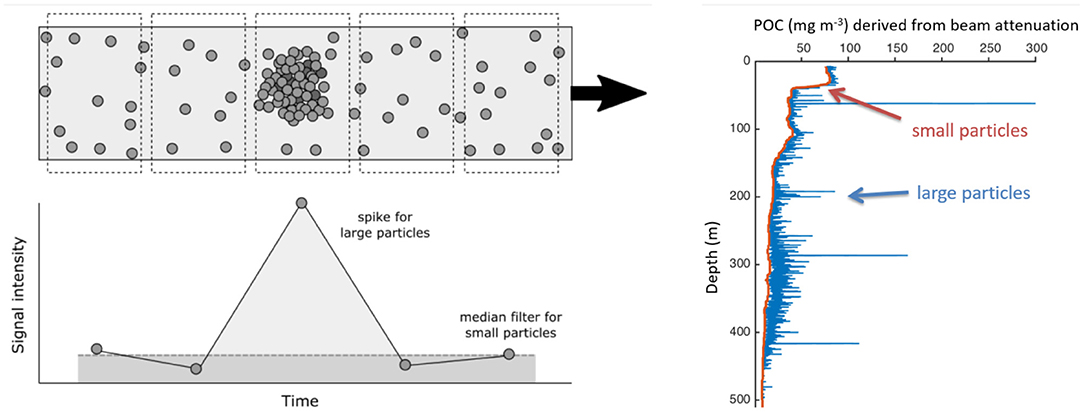
Figure 1. Working steps to derive small and large particles from single photodetectors. A median filter is fitted and assumed to be representative of small particles. Spikes are caused by large particles passing through the sampling frame.
(b) Simple photodetector array
Simple photodetector arrays use a similar principle to single photodetectors. The difference is that a number of photodetectors are arranged in a way that allows the extraction of additional particle properties. The most prominent example is the Laser Optical Plankton Recorder (LOPC; Herman, 2004). Thirty-five photodetectors (“photo-elements”) are arranged vertically and measure the absorbance of a laser sheet. As the instrument is towed through the water, particles passing through the light sheet block a portion of the light, and the receiving photo-elements register the change in voltage as digital size and transparency (Checkley et al., 2008).
Two types of particles are registered by the LOPC control software: single-element particles (SEPs) and multi-element particles (MEPs). SEPs are defined as particles occluding one or two photo elements, MEPs occlude three or more. For SEPs, only size information is recorded. For MEPs, in addition to their digital size, the occlusion of each photo-element is recorded providing information on shape and transparency.
The digital size of SEPs and MEPs is converted into equivalent spherical diameter (LOPC-ESD) using the manufacturer's calibration with black spherical beads (Herman, 2004; Checkley et al., 2008; Gaardsted et al., 2010). The LOPC-ESD is thus the diameter of a particle equivalent to the diameter of a black sphere that would block the same amount of light, which means, e.g., that a large, transparent particle can have a relatively small LOPC-ESD.
The LOPC per-se does not distinguish between particle types. However, for MEPs a separation based on transparency and/or shape can be done (Jackson and Checkley, 2011; Basedow et al., 2013, 2014) based on the ratio of LOPC-ESD to occluded diameter (the width of all photo elements occluded; Jackson and Checkley, 2011; Trudnowska et al., 2014). A small LOPC-ESD:occluded diameter ratio means that particles are transparent and/or amorphous. To relate size to organisms, correlation relationships have been determined from organism concentrations collected with plankton nets simultaneously with LOPC observations (Gaardsted et al., 2010; Ohman et al., 2012; Marcolin et al., 2015). Another prominent instrument using a simple photodetector array is the LISST (Sequoia Scientific Inc.), which measures the angular distribution of forward-scattered laser light using concentric ring detectors (Gartner et al., 2001). Additional published methods exist that also use near-forward scattering for estimating particle size distributions (Twardowski et al., 2012), These are bulk property detectors, which can be processed to estimate the size distribution of equivalent spherical particles. This method depends on different assumptions than those applied to blocking of a beam, and thus the sizes are not directly comparable to those from the LOPC or other imaging approaches.
(c) Holographic system
Holographic systems record a digital hologram of the particles in a water sample. To do so, a sample volume is illuminated with a collimated laser. As the beam hits a particle, light is scattered and interferes with the incident light of the laser beam. The resulting interference pattern is recorded by a camera (e.g., a charge-coupled device) and can be used to reconstruct a holographic image of the particle (e.g., Bochdansky et al., 2013; Talapatra et al., 2013; Nayak et al., 2018).
While this approach sounds as if the hologram would provide information on the 3D structure of each particle, this is only partially true. Particles are holographically imaged only from one side with the structure of the “backside” of the particle remaining unknown. More importantly, the 3D information is largely disregarded during the data processing routine. Rather, the holographic information is used to precisely calculate the size and position of the particle within the sample volume: The digital hologram is reconstructed providing monochrome in-focus images of each particle corrected for its position in the z-axis (i.e., accounting for the distance between particle and camera). The reconstructed images can then be analyzed using image analysis programmes in the same way as used for images by photographic devices (Graham and Nimmo Smith, 2010; Davies et al., 2015).
The advantage of the holographic systems is that the true size of each particle is known regardless of its position in space, and, owing to the method, reconstructed images exhibit strong edges that facilitate edge detection routines (Benson and French, 2007; Graham and Nimmo Smith, 2010). Moreover, holographic systems exhibit a depth of field (e.g., 7 cm at 5 μm resolution) that cannot be achieved with most lens-based system. The main disadvantage of holographic systems is the creation of unwanted interference (fringe) patterns producing noise that makes automated image analysis difficult. Commercially available holographic systems include the LISST-Holo (Sequoia Scientific, USA) and the HoloSea (4Deep, Canada).
(d) Photographic system
Photographic systems have become the preferred choice for observing in situ particles, likely because the resulting images are relatively easy to interpret owing to their similarity to those of traditional microscopic analyses. Accordingly, there is a wide range of devices commercially available or custom-made by various oceanic research groups. Typically, photographic systems are composed of four main units: (1) a light source, (2) a camera, (3) a computer or controlling unit often with additional environmental sensors, and (4) a power supply. However, these devices vary in their specifications in terms of unit arrangements/housing, sampling volume, maximum sampling frequency, illumination type, magnification, and particle size range (Table 1).
Illumination techniques are manifold with large differences in light source and direction. Particles can be illuminated from the front, one side (P-Cam; Lampitt and Iversen, unpublished), two sides (UVP; Picheral et al., 2010), all sides (LOKI; Schmid et al., 2016), or from the back (VPR, ISIIS; Davis et al., 2005). The light sources vary from simple scattered light (P-Cam) to laser sheets (SIPPER; Samson et al., 2004) and collimated LED beams (UVP, ISIIS; Table 1). Some devices illuminate particles using a single wavelength, which results in monochrome images. In these cases, the wavelength is often in the red spectrum (i.e., wavelengths that cannot be detected by most aquatic organisms) though the choice varies from device to device (e.g., ISIIS uses blue light). Illumination using full-spectra light (white) has the advantage that imaged particles are polychrome. Inclusion of color has been shown to produce much higher classification accuracy with automated routines than monochrome images (Saminsky and Gallager, 2018). A disadvantage of colored imaging is the potential need for calibrating the colors (for example, using a reference scale) particularly if multiple data sets are to be combined. Additional disadvantages include light contamination at the ocean surface (potentially leading to increased signal noise and requiring intercalibration even within a single vertical profile; Lindsay et al., 2014), chromatic aberration, and potential changes in zooplankton behavior (attraction or avoidance) or zooplankton colouration (Mori and Lindsay, 2008). How the choice of the light source affects the ability to combine datasets from different devices has, to our knowledge, not yet been explored. For example, comparing images from a device that uses blue light with those imaged using red light may give different estimates of size and abundance of green-tinted phytodetritus.
The particle size range captured by a device is dependent on the camera resolution and, if applicable, additional magnifying lenses. Higher resolution and/or magnification allows the capture of more detailed images and hence better identification of smaller particles. On the flipside, higher magnification often comes hand-in-hand with a decreased field of view and sampling volume. This trade-off may mean that larger (hence rarer) organisms have little probability of being imaged, may be only partly imaged, or may not physically fit in the sampling frame. Devices that allow more detailed imaging often capture very small volumes (potentially <1 mL per sample).
The target range of each device is therefore unique and needs to be fully evaluated. This evaluation includes determination of the minimum and maximum particle size that can be detected both qualitatively and quantitatively. There are several options to determine this size range. For example, a particle could be defined as an object with more pixels than the background noise (typically 4–20 pixels per particle) or an object with sufficient pixels to “identify” the particle (may require >100 pixels per particle). Alternatively, the minimum size-class can be set according to the particle size-spectra. Assuming that two small particles make one big particle, the abundance of small particles should increase to the square. Hence, when the particle number no longer increases with decreasing size in a log frequency vs. log size plot, the particle size is likely below detection. Likewise, the upper end of the linear relationship in a log-log plot indicates the maximum size that can be quantitatively assessed with the given device. The upper size limit of quantitative detection is often constrained by the low concentrations of larger particles relative to the total sample volume. The uncertainty in the concentration of large particles increases with decreasing counts of those particles in their respective size bins.
Data Processing: Sizing
Once a suitable device has been chosen and optical particle measurements have been acquired, the next step is often to determine particle size. Individual particle measurements are especially useful for studying sinking particles because bulk measurements can be dominated by small, non-sinking particles. Nonetheless, bulk optical measurements can provide some amount of particle size information.
Single photodetectors do not provide direct particle size estimates, yet spike height (i.e., the high-frequency variability in the beam attenuation or backscattering signal) can be used as an indicator of particle size (Briggs et al., 2013; Box 1a). Further, the spectral slope of the beam attenuation coefficient (Boss et al., 2001) and the spectral slope of the backscattering coefficient (Slade and Boss, 2015) can be used to estimate mean particle diameter and the slope of the particle size distributions. Currently, in situ validation of these methods is either limited or conflicting (Reynolds et al., 2016), so they should be used with caution without further validation.
For some devices, size is an explicit characteristic based on the assumptions of the method. For example, both the LISST-Holo and the LOPC report the equivalent spherical diameter (ESD) of detected objects without the user having to decide on the detection method. The advantage when size is provided as a standard output is that comparison of observations collected by different users and/or different devices of the same type are fairly straightforward. The user bias on how to interpret the data at face value is removed. The disadvantage is that users are often unaware of the assumptions and limitations of the device and the exact definition of the size parameter. For example, the “ESD” provided by LISST-Holo is based on the pixels of the reconstructed particle. The area of the particle (in pixels) is used to find the diameter of a circle with the equivalent area (Box 2). The size of a particle is hence derived from a 2D image, similar to more traditional photographic imaging, with the advantage that all particles are in focus and size can be determined much more precisely (Graham and Nimmo Smith, 2010). For the LOPC, the light attenuated by a particle is converted into ESD using the manufacturer's calibration with black spherical beads (Herman, 2004). The LOPC-ESD is thus the diameter of a black sphere that attenuates an equivalent fraction of light, which means that a large, transparent particle can have a relatively small LOPC-ESD.
Box 2. Equivalent Spherical Diameter (ESD) and Equivalent Circular Diameter (ECD).
ECD is calculated by rearranging the area of a particle into a circle and calculating its diameter (Figure 2). ECD and corresponding volume (V) can be calculated by excluding holes (ECDexcl and Vexcl) or including holes (ECDincl and Vincl). Depending on the porosity of the particle, ECDexcl and Vexcl can be substantially smaller than ECDincl and Vincl (respectively, 89 and 69% in our example). It is noteworthy that Vexcl might still overestimate the solid volume of a porous particle because of open spaces in the particle that are not visible in an image.
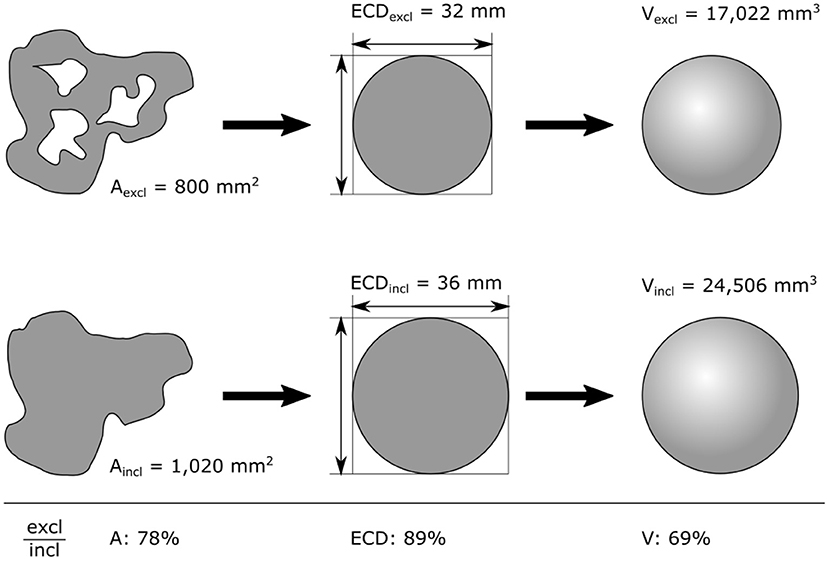
Figure 2. Working steps to derive equivalent circular diameter (ECD) and volume (V) from the 2D image of a particle. Both metrics can be derived from the imaged area (A). Care has to be taken whether holes are excluded (excl; top row) or included (incl; bottom row). Percentages at the bottom highlight the resulting differences of our example (ratio between excluded over included).
ECD is often used synonymously with the equivalent spherical diameter (ESD). While ECD and ESD are similar and may be the same, they are practically calculated differently with the former being derived from a 2D object and the latter from a 3D object. Different assumptions are used to allow this conversion, and these should be stated clearly.
For particles with known geometry, e.g., cylindrical fecal pellets or certain phytoplankton, the transformation from the image area to the particle volume can take advantage of this knowledge, and can even be corrected for the likely orientation of the particles relative to the camera (Ebersbach and Trull, 2008; Ebersbach et al., 2011).
For imaging devices (holographic or photographic systems), particle detection and subsequent sizing rely heavily on background subtraction, threshold settings (Giering and Hosking, in review), edge detection, and segmentation algorithms. The ultimate choice is often left to the user, introducing operator bias on a very basic level before any further analysis is carried out. Next, the user needs to decide on a metric to report size. As mentioned above, ESD is often the preferred metric, though many others exist (equivalent circular diameter (ECD), equivalent circular perimeter diameter, Feret diameter, length and width). Note that many reported ESDs are based on ECDs as most in situ systems work with particle area and not volume. Last, when ESD and ECD are calculated, a decision has to be made whether to include or exclude possible holes in the imaged particle, which can dramatically influence the final size estimate (Box 2).
Besides the difficulties in algorithm and metric choices, there are technical and practical issues for each of the different devices to capture the real size of a particle. The concept of size is in principle simple, however, in reality very complicated because of the complex shapes of marine particles, such as copepods with their legs and antennae, twisted diatom chains with their spines, radiolarians with delicate spikes, aggregates and feeding structures with complex shapes and empty cavities, or exopolymer particles, which are often undetected owing to their transparency. Not all imaging systems can resolve the necessary detail to capture such complex structures. Moreover, most imaging devices take a 2D image, which can substantially misrepresent the true size and shape of the original 3D particle.
For many devices with a relatively large depth of field, such as the Video Plankton Recorder, the z-position of a particle is unknown, meaning that the true size is unknown: A small particle close to the camera can have the same apparent size as a large particle further away (Figure 3). This imprecision is, however, likely averaged out over sufficient data provided that particles are illuminated consistently regardless of their z-position; i.e., small particles are as likely detected close to the camera than further away. For shadowgraph systems, the imaging of silhouettes using collimated light ensures that a particle is always imaged at the same size, regardless of how close it is to the camera (Cowen and Guigand, 2008; Ohman et al., 2019). Alternatively, a telecentric lens configuration can be used. In particle-rich environments, overlapping of objects might become an additional problem for devices with a large depth of field (Figure 3). Problems might also arise when only a small part of a larger particle is captured or illuminated, leading to a potential underestimation of particle size. Diffusion of light as it travels through the water from the particle to the sensor can cause a “halo” effect in which small particles appear larger than they really are. This effect can be corrected for with a size specific conversion factor between pixels and size (Picheral et al., 2010).
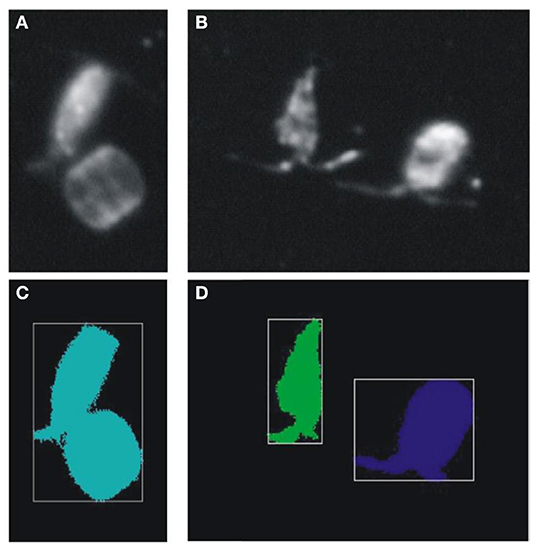
Figure 3. 2D representations of 3D particles in a 3D environment cause problems for particle recognition, sizing, and identification. (A,B) Original images taken by the Video Plankton Recorder (VPR). In both cases, it is not clear whether the two particles have the same size or appear of similar size because they are positioned at different distances from the camera. (C,D) Particles detected by the image processing routine. Besides the aforementioned sizing issue, the particle detection algorithm fails to separate the copepod and diatom in (A), and does not resolve the antennae of the copepods in (B). Images: KO Möller.
Estimating the absolute size of a particle using optical devices will likely always remain a challenge owing to the technical limitations and overall assumptions of the different methods. A big step forward to making data more comparable is increased transparency and standardization in data acquisition, analysis and description. We therefore recommend the following:
1. Instrument. The determination of size can be influenced by the type of device and/or its current configuration. Thus, to help end-users put data into context, we recommend that the instrument name and serial number are clearly reported. To reduce ambiguity, this should ideally be accompanied by an identifier from a standardized list, such as the SeaVox Device Catalog1. Furthermore, relevant technical details should be explicitly mentioned, such as illumination type and frequency, sampling volume and frequency, and relevant calibrations.
2. Image resolution. The final estimated size of particles is partly determined by the resolution of the image: A higher resolution allows not only the detection of smaller particles but also the description of more complex shapes. The resulting size estimate may vary significantly. A clear description of the image resolution, pixel size, and particle detection criteria (i.e., minimum and maximum particle size, see also Box 1d) will help to compare datasets.
3. Image/signal processing. All details of image or signal processing should be reported and the code made available (e.g., via GitHub). Image and signal processing steps may include background subtraction, noise reduction, dilation and erosion techniques, object recognition, and segmentation.
4. Thresholding and edge detection (for holographic and photographic systems). The most appropriate threshold (e.g., for black and white images the gray-scale value for the transformation of the image into a black-white binary field representing background and particle, which are used to calculate particle statistics) or edge detection algorithm should be determined. This could be done by using calibration beads or real aggregates and plankton of known size. Uncertainties should be clearly stated in the methods. Alternatively, a sensitivity analysis using a range of thresholds or algorithms and their effect on estimated particle size should be carried out.
5. Size metric. The metric used for describing size should be clearly stated. We recommend using ESD/ECD as this is the most widely used metric (Box 2).
6. Data deposition and sharing. (For a detailed discussion, see section data deposition and sharing). Particles imaged at high enough resolution to allow identification (generally ~30 × 30 px) should be saved as separate “vignettes” (images of individual particles extracted from the frame) and made publicly available to allow future image-based analyses. A unique identifier or hashtag could be assigned to the particle. In addition, a file in text-format containing the measurements on all individual particles (e.g., the parameters given by image analyses programmes such as imageJ, MatLab's Imaging Processing Tool Box, or the plugins for Python's image analyses) should be provided.
Data Processing: Classification
The size and abundance of particles is useful information and sufficient for many applications (e.g., identification of changes in particle populations with depth and its possible links to disaggregation and flux attenuation; Stemmann et al., 2004a,b; Jouandet et al., 2011; Kiko et al., 2017). sTo fully understand ecosystem processes, however, the identity of the particles is key: e.g., whether it is an amorphous aggregate or an individual zooplankton. Several devices have been designed specifically for quantification and identification of zooplankton and marine snow [e.g., the Underwater Vision Profiler (Picheral et al., 2010) or the Video Plankton Recorder (Davis et al., 1992, 2005)], often targeting the mesozooplankton size range (~0.2–2 mm). For these devices, a visual classification that is based on morphological features is very fruitful, and taxonomic guides can often be used to identify zooplankton to a fairly high taxonomic level (sometimes down to species). For single photodetectors or simple photodetector arrays, classification is much harder as information on particle type is very limited. However, with a combination of different devices (for example different wavelengths of backscatter, or backscatter combined with fluorescence measurements), some level of classification can be achieved, e.g., the chlorophyll fluorescence to red light backscatter ratio can be used to estimate the relative abundances of algal vs. non-algal particles (Iversen et al., 2010; Barbieux et al., 2018; Schallenberg et al., 2019).
Methods Based on Visual Classification
While underwater imaging of marine particles has recently become widely used, image analysis software tools have lagged behind hardware developments (Hu and Davis, 2005). The large amount of visual data emerging from in situ plankton samplers, benchtop systems, and cabled underwater observatories require automatic procedures. Besides saving time, automation avoids bias and errors inherent to the fatiguing process of manually classifying vast amounts of images (Culverhouse et al., 2003). Several automated methods for the analysis and classification of plankton and particle images have been developed, but their limited accuracy (around 80% on a realistic number of classes in recent attempts; Zheng et al., 2017) still requires significant manual verification to obtain accurate counts and identification or to further identify behavioral modes like trophodynamic plankton—particle interactions (Möller et al., 2012).
Image processing typically proceeds along the following steps. (1) When necessary, focus detection is used to restrict the effort to in-focus particles by eliminating out-of-focus frames. (2) Objects (i.e., “regions of interest”) are isolated from full frames using binarization, segmentation, and/or connectivity routines. For underwater image analysis, this step may be challenging due to variable illumination, scales, and orientation of objects and their non-rigid deformation (Py et al., 2016). Also, large organisms may exceed the sampling frame (and therefore be cut) or obstruct other, smaller objects. (3) Once detected, regions of interest are processed individually to compute size and extract morphological features used for automatic classification. These regions of interest are often saved as separate files (“vignettes”) for classification techniques that require an image as input.
The classification process then starts with the manual labeling of a set of images of objects by human operators, to provide examples on which machine learning classifiers can be trained. This training (or learning) set has to be as representative of the full data set as possible. Larger training set sizes usually significantly improve the performance of the classifier, although it saturates at some point (Gorsky et al., 2010; Ellen et al., 2015). Therefore, this step is time-consuming.
A wide variety of morphological features and classifiers have been trained to sort particle images automatically into plankton taxa or particle categories. Their full review is beyond the scope of this paper; Benfield et al. (2007) wrote a good synthesis of the field and a more recent list of papers is in the introduction by Zheng et al. (2017). Briefly, the morphological features can be global descriptors of the object (such as the area, the average gray level, etc.; Grosjean et al., 2004; Sosik and Olson, 2007) or the concatenation of local shape and texture characteristics, such as Fourier descriptors (Tang et al., 1998) or Histograms of Oriented Gradients (Bi et al., 2015). The former is more immediately interpretable with respect to the overall characteristics of the object (big vs. small, dark vs. light, etc.), in particular for ecologists; the latter is more common in the image analysis domain because it often yields better classification results. Once the set of features is chosen, the difference between the various classifiers (Support Vector Machines, Random Forests, Artificial Neural Networks, etc.) is usually small (Grosjean et al., 2004). Rather, accuracy is gained by introducing richer input images (such as color images), by combining different types of features (such as shape and texture; Hu and Davis, 2006), or by combining classifiers into ensemble models (Ellen et al., 2015; Zheng et al., 2017).
Finally, recently developed Convolutional Neural Networks (CNNs) have scored higher than any other technique on major image classification challenges (Krizhevsky et al., 2012). CNNs learn both the extraction of relevant features directly from the images and their classification. Thanks, in part, to an online machine learning competition that prompted the interest of the computer vision community and provided a standardized test dataset (https://www.kaggle.com/c/datasciencebowl, 2015), CNNs are increasingly used to classify marine particle images (e.g., Py et al., 2016; Luo et al., 2018). Training a CNN is computationally intensive and requires a large number of training examples. Fortunately, this constraint can be partly alleviated by kick-starting the process using a model pre-trained on another dataset because the features they extract are often quite generic (transfer learning; Orenstein and Beijbom, 2017; Lumini and Nanni, 2019), by guiding human operators to grow the training set with only problematic images (active learning; Bochinski et al., 2019), or by post-processing the features output by the network to learn new classes after the training step (low-shot learning; Schröder et al., 2019).
While these techniques hold promise, the classification of marine particle images is a challenging task because the image quality is often suboptimal for smaller particles, the range of sizes of particles is huge, and a few classes are much more numerous than others, which makes it difficult to tune the classification performance on the rarer, often interesting, classes. Moreover, novel classes that occur in the sample but do not occur in the learning set will also be misclassified.
Methods Based on Optical Properties
For single photodetectors or simple photodetector arrays that do not capture particle images, or for particle images that are small relative to image resolution, a combination of optical properties and/or a size parameter can provide information useful for very broad particle classification.
For example, the ratio of backscattering to beam attenuation is related to the ratio of organic to inorganic matter (Jamet et al., 2018) and to the refractive index, which can indicate particle composition (Twardowski et al., 2001). The ratio of chlorophyll fluorescence to optical backscattering can inform about the contribution of phytoplankton to particulate matter; and bulk birefringence can be a proxy for suspended CaCO3 concentrations (Guay and Bishop, 2002).
Another example is the combination of the spike signals detected by several single photodetectors, such as backscattering and fluorescence sensors. These sensors can identify individual particles larger than ~150 μm by the brief spikes they induce when passing through the sensor's sampling frame. The ratio of fluorescence spikes to backscattering spikes in a population of particles can distinguish aggregates of phytoplankton from other large particles (Briggs et al., 2011). The ratio of spikes of different wavelengths of backscattering, or beam attenuation spikes to backscattering spikes, likely contains further information, although this information has not yet been investigated.
The combination of size and transparency can inform whether the particle is an aggregate or zooplankton (e.g., Petrik et al., 2013; see also Box 1b). Devices measuring fluorescence can distinguish particles containing chlorophyll (phytoplankton) and/or phycoerythrin (cyanobacteria) from other particles, and cameras measuring birefringence have been used to distinguish particles containing calcium carbonate (Bishop et al., 2016). For particle images, particle brightness (or light attenuation for light field images) and color are optical properties that can help distinguish particle types (Wilson et al., 2008).
Data Deposition and Sharing
As a next step, processed data should be deposited and made freely available for future research. Currently, there is no standardized procedure to deposit data on optical particle measurements, and there is a clear lack of agreed metadata, particle descriptors, data formats, classification criteria, and accessibility. Any community agreement should consider the FAIR Guiding Principles (Wilkinson et al., 2016), a set of standards to improve the findability, accessibility, interoperability, and reusability of data. As discussed above, we need increased transparency and standardization for data sharing, comparison and future data interpretation. Embedding well-structured metadata and data provenance information in data workflows are fundamental to ensuring user trust in data and any data products generated (Buck et al., 2019). As discussed in section Data Processing: Sizing, using common standards such as controlled vocabularies to annotate data help reduce ambiguity and facilitate interoperability. Many journals require datasets to be cited with Digital Object Identifiers to support scientific results. In addition, persistent identifiers (PIDs) are well-established in the academic community to improve transparency, and there are international efforts to use PIDs to identify “real-world” instruments2. Such tools could help a user to relate back to the manufacturer's calibration or configurations of a device to put particle size data into context. Several marine observational programmes use agreed formats that are machine-readable and enriched with common standards to facilitate data sharing, automation and comparison within a community. For “live” planktonic particles a start point is the widely-adopted Darwin Core format used by the Ocean Biogeographic Information System (OBIS) for biogeographic data (Nakamura et al., 2017), but it lacks many descriptors necessary for it to be directly applied to the wide range of particles in the ocean. The Argo Climate Forecast (CF)-NetCDF is more flexible. However, NetCDF requires complex software and some level of expertise to access it. The World Wide Web Consortium's (W3C) “CSV on the Web”3 offers the same benefits as NetCDF but in a simpler format that may be more accessible to the biogeochemical community, requiring standard software such as Microsoft Excel.
Ideally, all raw images (i.e., full frames) should be saved. However, raw images require considerable storage capacity and often contain mostly empty space. Instead, a common practice is to save segmented individual particle images (“vignettes”). Vignettes should be saved to the highest resolution to facilitate re-analysis and avoid deterioration if compressed. Vignettes should be saved on a global databank or distributed database, allowing others to carry out their routines on the raw particle images, particularly machine learning and image processing for classification. While a similar approach has been started (e.g., EcoTaxa, https://ecotaxa.obs-vlfr.fr; Picheral et al., 2017), a current big limitation is the required storage capacity, especially with particle imaging becoming ever more popular. EcoTaxa alone already hosts >80 million vignettes (as of May 2019). In addition, funding bodies that require data deposition often prefer only the particle descriptors of each image in text-format as it is less memory intensive. When describing images in text-format, there is substantial information loss. For example, particles are often described in terms of size and particle type only. Besides the inconsistencies and ambiguities of sizing (see Section Data Processing: Sizing), potentially crucial information (e.g., color, shape, and texture) are lost. We therefore recommend depositing raw images and/or vignettes whenever possible. Finally, a unique identifier or hashtag could also be assigned to each particle image to allow tracking of information regarding this particle. e.g., different scientists might carry out different image processing steps or assign different classifications to the same particle depending on their data analysis procedures or classification scheme. Furthermore, more detailed analysis on single particles, including sinking velocity measurements and carbon content, might be available for some particles. If a unique identifier is assigned, identification and detailed information could be harvested and used for further in-depth analyses and meta-analyses.
Any software and image analysis codes used to process images should be assigned a PID and cited in accompanying documentation to improve transparency to users. Image analysis codes should also be made available using code-hosting facilities (such as GitHub).
From Image to Particle Flux
Optical particle measurements and classification are only the first steps to understanding particle dynamics in the ocean and the biological pump. The next steps involve the conversion of this information into flux estimates. Particle fluxes are typically calculated as
where F is the matter flux (in mg m−2 d−1), c is matter concentration (typically in mg m−3), wavg and wpart are the bulk and individual particle sinking velocity, respectively (in m d−1), and mpart is the matter content of an individual particle (in mg C) with Σ(mpart × wpart) being the sum of all particles within a known sampling volume (Vsample in m−3). These equations can be further expanded to explicitly separate the composition of particles from their masses and numerical abundance to represent the fluxes of specific components, e.g., for the particulate organic carbon (POC) flux:
in which n is the number of particles in that class (n = 1 for individuals), Vpart the particle volume (typically in mm3), and ρi is the density of the constituent i in the particle (e.g., mg C mm−3). The sum is then taken over all particles and normalized to the sampled volume.
Optical devices for particle measurements provide great direct estimates of particle numbers (n) and good estimates for particle volume (Vpart), but they are not able to provide direct information for chemical particle composition (i.e., ρi, with i being POC or any other component of interest such as carbonate or silica minerals). Hence, all optical devices require an estimation of the approximate elemental particle composition in order to estimate POC concentrations and, ultimately, flux.
Particle sinking velocities (w) can be estimated using a range of methods (Section Sinking Velocity); however, most optical devices are not capable of providing sinking velocities and rely on additional data or assumptions. Two bulk optical methods bypass the need for information on sinking velocities by quantifying particle fluxes more directly either by optically measuring particle accumulation on a horizontal surface (“optical sediment trap”; Bishop, 2004; Estapa et al., 2013; Bourne et al., 2019) or by tracking the accumulation of integrated particle concentrations below a depth threshold (Dall'Olmo and Mork, 2014).
Both chemical particle composition and sinking velocity can vary greatly. POC content can be as low as ~1% by weight in particle fluxes dominated by lithogenic and biogenic minerals (e.g., Armstrong et al., 2002; Klaas and Archer, 2002) and as high as 40% in aggregates (e.g., Alldredge, 1998). Sinking velocities typically range from 1 to 1,000 m d−1 (e.g., Kriest and Evans, 1999; Turner, 2002; Laurenceau-Cornec et al., 2015a). Hence, the biggest bottleneck associated with translating optical particle measurements into accurate flux estimates is the uncertainty in these two parameters. We explore some approaches to measure particle composition and sinking velocity in the following sections.
Organic Matter Content and Concentrations
The approaches for estimating bulk matter concentrations (c) and matter content of individual particles (ρi × Vpart) differ in that the former is an estimate based on the entire sample whereas the latter is specific to objects of interest.
Bulk estimates are based on the entire particle field and how they affect the optical properties of the water volume they are in. In simple terms, the more matter there is, the more light will be scattered and absorbed by the particles (i.e., less light will be transmitted through the water). Devices that measure scatter and transmission in one way or another can therefore be used to infer bulk matter concentrations. Empirical data have shown that both particulate backscattering (bbp) and attenuation (cp) are good proxies for the concentration of POC in pelagic environments (Gardner et al., 1993; Bishop, 2009; Bishop and Wood, 2009), though the relationships are dependent on the local particle populations and potentially on methodological differences such as sensor calibrations (Cetinić et al., 2012). To use such bulk proxies, it is therefore preferable to take POC samples along with the optical property measurements to derive a site-specific relationship between the two parameters. In addition, natural variability in lithogenic matter concentrations (e.g., sediment load) can strongly affect the relationship between POC and bbpor cp (e.g., Reynolds et al., 2016), so these optical proxies cannot be used where sediments dominate the optical signal.
For individual particles, determination of the organic matter content becomes more complex. The typical work process is as follows. The optical device images a volume of water, the individual particles are sized (section Data Processing: Sizing), and the size estimate is converted into POC content using empirical relationships. As imaged particles are rarely also captured and brought to the surface for elemental analysis, most studies rely on published size-to-POC conversion equations. The most commonly applied equation is the one by Alldredge (1998). Alldredge (1998) photographed particles (>0.5 mm in diameter) in situ from the surface ocean in the Santa Barbara Basin, pooled these according to size and type (three size classes and four types), and analyzed them for POC, particulate organic nitrogen and dry mass. Organic matter content of particles clearly increased with increasing size (p < 0.001, R2 = 0.86, n = 25), though larger aggregates contained less matter per unit volume than smaller aggregates (Alldredge, 1998). Later laboratory experiments have shown that the size-to-POC conversion for aggregates is strongly dependent on the phytoplankton community composition (Ploug et al., 2008a; Iversen and Ploug, 2010) and can vary substantially from the size-to-POC conversion for zooplankton fecal pellets (Gonzalez and Smetacek, 1994). These observations suggest that there is substantial variability in the size-to-POC conversions depending on plankton community structure, particle type, season, location and likely depth (Kriest and Evans, 1999; Kriest, 2002; Iversen et al., 2010).
On the most basic level, the uncertainties associated with converting a single particle image into POC concentrations are not different from the problems encountered for bulk analysis. The main difference is that the sample size of large particles imaged using holographic or photographic devices is relatively small compared to the sample size imaged by e.g., backscatter sensors. Backscatter sensors integrate over a much larger particle population size, which can therefore be related to bulk POC measurements with more certainty. A similar bulk approach could be applied to large particles to reduce uncertainties in the conversion. Waite (unpublished) explored this possibility by filtering large volumes (50–100 L) of seawater onto a mesh of known mesh size (in this case 20 μm), rinsing the material off and measuring its POC content. The POC content of these particles related reasonably well to the particle biovolume measured using an Underwater Vision Profiler, providing a site-specific size-to-POC conversion (Figure 4). While gravitational filtration or pumping (e.g., as in situ stand-alone pumping systems) could cause particles to break up and change characteristics, they are relatively easy to make, not very time demanding and not very prone to subjective bias. This “bulk approach” for larger particles might thus be more feasible at sea than studies on individual particles, and could be adopted for quasi-global measurements.
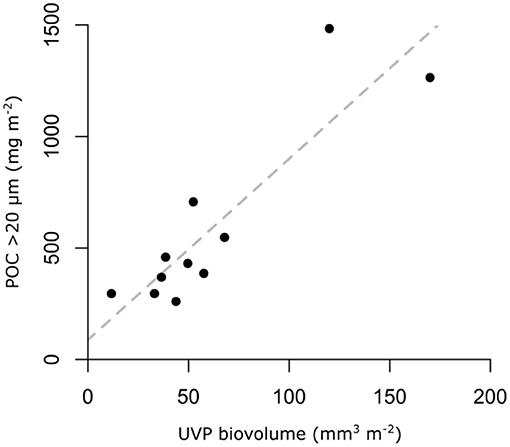
Figure 4. Relationship between particle biovolume measured using an Underwater Vision Profiler (UVP) and particulate organic carbon (POC) content of particles retained on a 20 μm mesh. Dashed line shows linear regression POC [mg m−2] = 87.8 + 8.1 biovolume [mm3 m−2]; p = 0.0001, R2 = 0.7 (Waite, unpublished).
There is clearly a need for more data on size-to-POC relationships and how they vary in space and time. We therefore highly recommend a concerted effort to collect information on size-to-POC relationships. These measurements are relatively tedious to collect and often only yield a few data points, which is frequently deemed insufficient for data interpretation purposes. However, a joined effort and data portal that collates all these individual measurements would soon produce a respectable database that could form the basis for more accurate and appropriate size-to-POC conversion equations.
Sinking Velocity
The range of recorded sinking velocities of particles in the oceans spans from negative (i.e., particles float toward the surface; Azetsu-Scott and Passow, 2004; Acuña et al., 2010) to several km per day (e.g., salp fecal pellets; Iversen et al., 2017). When considering the sinking velocity of an individual particle, a first approximation can be obtained from Stoke's law (originally derived for spherical, non-porous particles and laminar flow) combined with White's approximation (White, 1991), which suggest that sinking velocity increases linearly with excess density (the difference from the water density) and the square of particle diameter (i.e., linearly with the particle area). Building on these expectations, many studies have tried to relate sinking velocity primarily to size, which has been shown to be a useful predictor for particles generated in controlled environments (e.g., roller tanks; Gärdes et al., 2011; Iversen and Ploug, 2013; Iversen and Robert, 2015). However, strong relationships were only observed when all particles were generated using the same water/plankton community (Iversen et al., 2010). When particles were made by different plankton communities, size alone was a bad predictor (e.g., Diercks and Asper, 1997) strongly supporting notions that particle densities and shapes vary widely depending on the source material (Iversen et al., 2010).
Packaging and porosity contribute appreciably to determining sinking velocities. On the one hand, adding ballasting materials, such as diatom frustules, to aggregates may lead to an increase in sinking velocities owing to the increase in excess density. On the other hand, the addition of ballasting mineral particles to marine particle populations frequently leads to smaller more densely packed aggregates that sink slower because of their smaller size (Hamm, 2002; Passow et al., 2014). Mucous-rich particles have been shown to float despite relatively large sizes (Azetsu-Scott and Passow, 2004; Bochdansky et al., 2016), whereas oil- or plastic-containing aggregates have been shown to sink rapidly despite the presence of substances with an excess density smaller than seawater (Long et al., 2015; Passow et al., 2019). In natural environments, particles are formed through different mechanisms, by different organisms, and under varying environmental conditions that affect aggregation (e.g., salinity, pH, minerals), ballasting (e.g., dust deposition, sediment load; Iversen et al., 2010; Iversen and Robert, 2015; van der Jagt et al., 2018) and sinking behavior (e.g., viscosity; Taucher et al., 2014). A universal conversion of size-to-sinking velocity is hence impracticable (Jouandet et al., 2011).
Nonetheless, estimates of size-to-sinking-velocity relationships are powerful when determined site-specifically for either distinct types of particles or large particle populations, which negates the effect of individual outliers. To do so, a sufficient number of particles needs to be imaged and their sinking velocities measured for each location and—if possible—for each particle class. Unfortunately, measuring the sinking velocity of individual particles directly remains challenging, and many studies rely on indirectly approximated sinking velocities from bulk measurements. The following sections explore the most commonly used methods for determining sinking velocities for individual particles and from bulk measurements.
Individual Particles
The majority of data on individual particle sinking velocities has been generated ex-situ. A big advantage of ex situ sinking velocity measurements is that the particles can be retrieved after the measurement and analyzed for elemental composition. It is thus possible to generate data on size, sinking velocity and carbon content for individual particles, allowing the calculation of carbon flux (by that particle) with relatively high certainty. Box 3 lists some of the methods used to measure the sinking velocity of individual particles ex situ.
Box 3. Ex situ measurements of particle sinking velocities.
(a) Sedimentation column
A method that is cheap and simple to set up is to measure the time it takes for a particle to pass two marker points in a large measuring cylinder full of seawater (O'Brien et al., 2006; Riley et al., 2012; Cavan et al., 2015). To replicate in situ sinking behavior, the experimental setup should be set to the same temperature and salinity as the particle origin. While this method sounds straight forward, there are several caveats. (1) Subtle differences in temperature between the inside and outside of the experimental container introduce convection currents that strongly affect slow-sinking particles. (2) Salinity gradients can develop relatively quickly, sometimes leading to a complete halt of the settling particle. (3) Particles are often introduced into the settling column with peripheral water (e.g., when using a pipette), which may have a different density (temperature and/or salinity) to the water in the settling column. The measured sinking velocity will therefore reflect the behavior of the introduced water as much as that of the particle itself. (4) The downward motion of the particle and, potentially, water around it causes an upward motion of water elsewhere in the container. Depending on the size of the container, the resulting internal turbulence may affect particle sinking behavior. (5) When measurements are taken at sea, the motion of the ship may influence the measurement. Uncertainties in the final sinking velocity measurement are therefore potentially large.
To mitigate some of these problems, cold lights should be used as even short exposures of light instantly create turbulence flow in the sinking cylinder. The measuring cylinder should also be as large as possible in diameter to reduce wall effects. Microgradients of salinity can be used to shut down all convection in experimental cylinders (O'Brien et al., 2006). For all experiments, flow conditions in the cylinder are best tested with some dye or neutrally buoyant particles to ensure that the water in the cylinder is quiescent (Ploug et al., 2008a).
(b) Ex-situ time-lapse
A more sophisticated version of the sediment column is the combination of a sinking column or roller tank with cameras. There are several examples, and we here explain the FlowCAM method (Bach et al., 2012) and the Orbit method (Ploug et al., 2010). More elaborate setups may involve two video cameras, which can be aligned to give a 3D view of sinking (Ploug et al., 2008a).
The FlowCAM is a laboratory piece of equipment that is used to measure particle concentration, type and size. Conventionally particles are pushed through a cuvette past a camera that takes photographs, and particles are automatically counted. Depending on the magnification, particles down to 20 μm can be measured. The FlowCAM can be adapted to measure sinking velocities. For this, instead of being pushed past the camera, particles are left to settle in a tube leading to the cuvette and camera so that, by the time they reach the field of view (FOV), particles are settling at their natural velocity (Bach et al., 2012). Multiple images are taken (up to 12 per second) and, knowing the number of images taken per particle and the distance traveled through the FOV, sinking velocities can be calculated. As for the sedimentation column, the temperature and salinity should be the same as at the particle's origin. Some of the benefits of this method are that it is very quick and semi-automatic, allows a relatively large particle size range to be measured, and gives individual particle characteristics (e.g., ESD, type, color) that can be associated with individual sinking velocities. Some drawbacks include wall effects as particles sink toward the cuvette, magnification limiting the maximum size of particle that can be measured, aggregation within the settling tube prior to entrance into the cuvette, and convection currents if the temperature is not constant.
The “Orbit method” measures sinking velocities of particles directly in roller tanks (Ploug et al., 2010). Particles are incubated in a rolling tank where solid body rotation has been established. Video capture of several orbits allows for “repeat” calculations of sinking velocities and thus present solid values. This method is non-destructive, and particles may be used for later analysis.
(c) Flow Chamber
The Flow Chamber allows measurements of all three particle dimensions (height, length, and width), sinking velocity and micro-sensor measurements while the particle is kept suspended by an upward-directed flow matching the settling velocity of the particle (Ploug and Jørgensen, 1999; Wekerle et al., 2018). The Flow Chamber system is filled with filtered seawater with in situ temperature and salinity. The upward flow is adjusted with a needle valve until the particle remains suspended at a distance of one particle diameter above the net, whereby the particle sinking velocity is equal the flow velocity. The sinking velocity of each particle is then calculated from the flow rate divided by the cross-sectional area of the flow chamber. Unfortunately, this method is time-intensive, allowing investigation of only a few (typically <10) particles per hour.
Briefly, particles for ex situ measurements are either generated in roller tanks or collected and resuspended. The first approach is problematic as these particles may—as described above—not reflect natural particles and particle behaviors (Ploug, 2001; Prairie et al., 2015). Its advantages are that it allows the testing of targeted interactions and effects on sinking velocities. The second approach can be tricky as collection and handling procedures may alter the particles (i.e., fragmentation, aggregation, change of shape, porosity, etc.). To measure sinking velocities, individual particles are introduced into an experimental container, such as a tank or settling column, and their sinking velocity is measured either traditionally with a stop-watch (Box 3a) or using time-lapse photography (i.e., video analysis; Box 3b). Another method is the use of a flow chamber, where the particle is kept in suspension by an upward flow of water (Box 3c; Ploug and Jørgensen, 1999; Peterson et al., 2005; Iversen and Ploug, 2010); The flow speed thus reflects the sinking velocity of the particle. This method further allows simultaneous imaging of the particle as well as additional measurements such as oxygen gradients within the particle (Ploug and Grossart, 1999; Belcher et al., 2016a,b).
In situ sinking velocities of individual particles have been measured by divers (Alldredge and Gotschalk, 1988) or using time-lapse camera systems taking rapid sequential photos of particles as they settle through the water column. To negate lateral advection and internal waves, some camera systems image particles as they settle through a column (Diercks and Asper, 1997; Cartwright et al., 2013), other systems are installed on neutrally-buoyant drifting platforms in a Lagrangian fashion (Pilskaln et al., 1998, Lampitt and Iversen, unpublished) or as moored platforms making seasonal measurements of settling velocities on individual aggregates in the deep ocean (Diercks et al., 2018; Iversen, unpublished). Images are often taken in bursts every few hours to conservatively use battery power and data storage, and the capture rate during these bursts needs to be sufficiently fast to accurately track fast-sinking particles (Diercks et al., 2018). The advantage of these methods is that they provide the most accurate sinking velocity estimates of natural particles to date as they minimize any manipulation and preserve in situ conditions. Moreover, they can collect data on a large number of particles spanning a relatively large particle size range (depending on the camera specifications), and their data can thus be used to construct both particle size spectra as well as sinking velocity spectra and investigate the relationship between size, shape, and sinking velocity. On the flip side, construction and deployment of time-lapse camera systems are relatively complicated and expensive. A noteworthy methodology to calculate sinking velocity from such in situ image time series is particle image velocimetry (PIV; e.g., Steinbuck et al., 2010; Smith and Friedrichs, 2015), which has also been used to observe filtration rates of larvaceans in situ (Katija et al., 2017).
Bulk Measurements
Approaches to estimate the average sinking velocity for the entire particle population or a particle size class are plentiful with a great variety of methodologies (Box 4). These methods are mostly based on in situ observations. in situ approaches often use an observed change in characteristic (e.g., Chlorophyll a) over time and depth (Box 4a) or modified sediment traps that allow the collection of differential settling particles (Box 4b). When combined with conventional sediment traps or gel traps, in situ observations of particle size spectra can be used to infer sinking velocities of individual particle size classes (Box 4c) (Guidi et al., 2008; McDonnell and Buesseler, 2010) and to estimate mass fluxes (Guidi et al., 2008; Iversen et al., 2010; McDonnell and Buesseler, 2012; Nowald et al., 2015). Recently, the use of naturally occurring radiotracer pairs (210Po/210Pb and 234Th/238U) has been added as a tool to estimate average sinking velocities (Box 4d) (Villa-Alfageme et al., 2014, 2016). The “SETCOL method” is a relatively easy and fast ex situ method that can provide sinking velocity estimates for selected particle groups (e.g., specific species, types or size classes) (Box 4e; Bienfang, 1981).
Box 4. Bulk measurements of sinking velocities.
(a) Depth and time correlations
High temporal and vertical resolution sampling of particle concentration allows the calculation of “bulk” estimates of particle settling velocities using correlations between variations in concentration at different depths. This method works best when the sinking velocity of an identifiable group of particles is homogeneous, and when the concentration of the tracked particles is pulsed or highly variable in time, exceeding variability due to advection across spatial gradients. This method has been applied to optical backscattering, fluorescence, and beam attenuation data collected by ships and autonomous gliders (Briggs et al., 2011) and to LOPC data collected by an autonomous profiling float (Jackson et al., 2015).
(b) Indented rotating sphere trap
The indented rotating sphere (IRS) trap allows particles to be separated in situ according to their sinking velocities. Particles land on a sphere and, after an amount of time (typically 12–24 h), the sphere rotates releasing the particles to be collected in a sample carousel that also rotates at specific time intervals. This setup results in sinking velocity bins between 0.68 and >980 m d−1. IRS traps can be moored at any depth and usually deployed on a monthly time frame. This method is useful for determining the relative importance of sinking fluxes. For instance, the IRS was used to show that slow and fast sinking particle fluxes were larger than intermediate sinking fluxes in the Mediterranean (Alonso-González et al., 2010). In the northwest Pacific, it revealed how a power-law attenuation of flux with depth (Martin et al., 1987) can be explained by a proportionally higher loss of slow-sinking particles with depth (Trull et al., 2008).
An advantage of this method is that collecting particles in situ negates any imposed temperature or density changes when trying to replicate settings in the laboratory. Some limitations are that particles may partially collapse on the ball in the IRS, reducing porosity and potentially increasing sinking velocities (Peterson et al., 2005). The sphere rotation time could be reduced giving less time for particles to be altered whilst sitting on the sphere, but this would increase the lowest settling velocity, e.g., 3 h rotation = minimal sinking velocity of 5.4 m d−1 (Peterson et al., 2009).
(c) Size distribution
As there is an existing theoretical link between particle size and sinking velocities (derived from Stoke's Law or other more refined expressions of the balance between drag and buoyancy forces; e.g., Laurenceau-Cornec et al., 2015b), estimations of particle sinking velocity can be directly back-calculated from joint observations of particle size distribution. The step from sinking velocities to fluxes (as measured by sediment traps) requires the conversion from particle size to volume to carbon content (for details see methods by Guidi et al. (2008) Equations 4, 6, and 9). However, this method assumes that particle excess density remains constant from one particle size to another, which is unlikely as a result of both fractal assemblage of aggregate particles (e.g., Jackson and Lochmann, 1992; Kriest and Evans, 1999) and differences in ballasting of phytoplankton via their frustules (e.g., Klaas and Archer, 2002).
In order to resolve this issue, McDonnell and Buesseler (2012) developed a method that allows the back-calculation of particle sinking velocities from simultaneous measurements of particle size distribution from camera systems and from gel traps. While this method is very promising, its global use is limited because it is very time-consuming and costly. However, it can provide useful insights into time and space variabilities of the relationship between particle size and sinking velocity.
(d) Radioactive tracers
The radioactive pairs Thorium-234/Uranium-238 and Polonium-210/Lead-219 allow the estimation of particle fluxes. A relatively novel method, a one-box inverse model, further uses 210Po/210Pb or 234Th/238U profiles to calculate in situ sinking velocities in the upper mesopelagic, allowing observations of changes in sinking rates with depth (Villa-Alfageme et al., 2014, 2016). Samples are collected using Niskin bottles or in situ pumps and average sinking velocities are calculated for each sample. The simple one-box model assumes a steady-state system, which is not always appropriate in changing conditions, such as blooms, as 210Po or 234Th have relatively long half-lives (Villa-Alfageme et al., 2014). It is important to note that calculated sinking velocities tend to be <100 m d−1 and represent an average sinking velocity for the entire particle population at each depth. Estimates also depend on how samples are collected, e.g., whether particles are filtered (e.g., using Stand Alone Pumping Systems), and whether they include the suspended non-sinking fraction or not.
(e) Settling tube
The “SETCOL” method (Bienfang, 1981) is an ex situ bulk method that can be used to estimate sinking rates of selected particle groups. This method is primarily used to measure phytoplankton cell sinking velocities, and the same principle is applied for the Marine Snow Catcher (Riley et al., 2012; Giering et al., 2016; Cavan et al., 2017). A homogenous water sample is placed or collected inside a settling column and an initial sample is taken. After a pre-determined time interval, the concentration of particles in different parts of the settling column (e.g., the bottom, middle, and top) is measured. The average sinking velocity can now be calculated by the difference in biomass in the different parts of the settling column between the start and end of the settling period. This method is relatively easy and fast, though the final estimate strongly depends on the settling time and dimensions of the settling column. It has useful applications in laboratory culture experiments, yielding precise sinking velocity estimates for culture species when comprehensively replicated (Waite et al., 1992). In addition, for field samples, SETCOL can be combined with size-fractionation or type-specific particle counts to provide sinking velocity estimates for selected groups (Waite and Nodder, 2001).
Optical settling tubes work on the same principle but monitor the change in concentration throughout the entire settling period using an optical device, such as a transmissometer, fitted near the bottom of the settling tube. This set-up allows the calculation of particle size distribution and settling velocity distribution (Zaneveld et al., 1982) and has been used in situ (e.g., Kineke et al., 1989; Spinrad et al., 1989; Murray et al., 1996), though these studies focussed on coastal or near-benthic particles.
Models as a Tool
Optical devices for particle measurements provide a wealth of information, which can be used far beyond simple particle classification and carbon flux estimates. For example, particle size distributions can reveal trophic positions (Zhou, 2006; Basedow et al., 2016) and, when combined with models, particle interactions such as aggregation and disaggregation [(O'Brien et al., 2004; Stemmann et al., 2004b; Karakas et al., 2009).
Models can play two essential scientific roles. (1) They synthesize our best understanding of a process. Our understanding can then be tested and improved by comparing the quantitative predictions by models with observations and experiments. (2) Models can be used to estimate hard-to-measure parameters. For example, despite successful measurements of aggregation/disaggregation parameters in the laboratory (Waite et al., 1997b; O'Brien et al., 2004; Iversen and Ploug, 2013), to date, we have no means of observing in situ particle aggregation and disaggregation rates. A comparison of modeled and observed particle size distributions, however, has been successfully used to infer these rates between particle size classes (Stemmann et al., 2004a,b; Karakas et al., 2009). To use models with any degree of accuracy or precision requires a careful assembly of additional data beyond just the observed size distributions, such as phytoplankton community composition and size distributions in sediment traps (Jackson et al., 2005).
Models of marine particle size distributions commonly describe particle interactions in terms of coagulation theory (Burd and Jackson, 2009), which provides the basic framework for calculating the rates of collisions between particles. These models require estimates for a suite of biophysical parameters that will vary between sites and models (Table 2). For example, a simple model that assumes a single source of particle requires relatively easy-to-get auxiliary data (e.g., primary production; Jackson, 1990), whereas a complex model, e.g., simulating coagulation between different types of particles such as diatoms and fecal pellets, will require complex auxiliary data (e.g., production rates for each type of particle; Jackson, 2001; Jokulsdottir and Archer, 2016). Moreover, as coagulation depends on physical processes and particle geometry, estimates of turbulent dissipation rates and particle fractal dimensions may also be needed. Some of these parameters (such as turbulent dissipation rates) can be estimated from detailed measurements of physical water-column, properties whereas particle-based parameters such as fractal dimensions can be obtained from particle image analysis (e.g., Kilps et al., 1994). These complex auxiliary data are largely contextual data that provide the model with the correct biophysical description to estimate the particle size distribution and make meaningful comparisons between model results and observations. Models can be made without such contextual data with the missing parameters considered as fitting parameters, a situation that becomes more and more unsatisfactory as the number of missing parameters increases.
Although both modeled and observed particle size distributions can be calculated using different widths of the size bins, it is useful to know in advance of the model calculations what these size bins are. This is particularly critical because of the different rationales for choosing bin sizes in models and data; bin sizes in models are often chosen to improve computational speed, whereas bin sizes in data are often chosen to optimize signal over noise. In addition, models and observations should use the same definition of particle size (i.e., the modeler should be aware of how the size was calculated from the images; Jackson et al., 1997).
Estimates of the uncertainties of the observed particle size distribution can help when using the model as a tool to help interpret the observations. This is because model results tend to be smooth functions of particle size, often approximated by power laws over specific size ranges (Burd and Jackson, 2002), whereas observed distributions show varying degrees of noise (Jackson et al., 1997). Significant differences between the modeled and observed distributions may suggest the presence of certain processes, such as size-specific grazing rates, and departures from a smooth power-law distribution may indicate the occurrence of significant disaggregation (Burd and Jackson, 2002). Contextual data such as turbulent dissipation rates and zooplankton abundances may help to identify the possible causes of differences between modeled and observed distributions.
A good example of how models and particle image data can be used together to infer both rates and processes is provided by the work of Stemmann et al. (2004a,b). This study used data from the UVP and compared observed particle size spectra to modeled ones, finding that below the euphotic zone physical aggregation processes were less important than disaggregation processes.
Particle aggregation codes have been publicly available for some time and can be used to help interpret data. For example, a simple coagulation model coded using Matlab and based on the original code of Jackson and Lochmann (1992) is available at https://github.com/BurdLab, and a more complex Fortran model with multiple particle sources (Jokulsdottir and Archer, 2016) is available at https://github.com/tinnsi/SLAMS. As with all tools, using these models is not always a trivial endeavor. To effectively use these models, we suggest that observational programmes consider the following recommendations:
• Provide estimates of contextual biophysical parameters such as primary production, turbulence dissipation rates, particle composition.
• State the definition of the particle size metric used to calculate particle sizes and the bin widths used to determine the size distribution.
The two largest issues that face accurate modeling of particle size distributions are our limited predictive understanding of particle stickiness (“aggregation potential”) and particle strength (“disaggregation potential”). Particle stickiness between inorganic particles has long been understood in terms of electric potentials, but the presence of organic substances such as transparent exopolymer particles (TEP) makes understanding and measuring stickiness much harder (Waite et al., 1997b). Although disaggregation measurements have been made (O'Brien et al., 2004) and models of particle disaggregation exist (e.g., Hill, 1996), models still require significant assumptions about the strength of aggregates (which may relate to the particle stickiness) and the size distribution of daughter particles. To move beyond the current models will require a better understanding of what controls these parameters and how they affect the aggregation/disaggregation process. Additionally, we are only just beginning to be able to model biological processes and distributions of solutes, such as oxygen, carbon dioxide and dissolved organic matter, within sinking permeable aggregates with different internal structure and porosities, i.e. marine snow (Moradi et al., 2018).
Conclusion
Optical devices for particle measurements have the potential to provide high spatial and temporal resolution of particle fluxes, though we are still far away from accurately estimating fluxes based on optical devices alone. The biggest bottleneck is the uncertainty associated with translating an optical signal, such as an image, into an accurate flux estimate, which requires additional information such as the sinking velocity and density of the particle. Moreover, the parallel development of various devices and analysis routines currently hinders meaningful comparison between techniques. Such comparisons are urgently needed as well as the standardization across methods of data collection, analysis, and data archiving.
In situ optical methods provide us with the ability to identify particles in the water column with minimal disturbance, and the fast imaging rates offer high-resolution information on their spatial distributions and interactions. For zooplankton, images give much higher vertical and horizontal resolution than nets and provide much better taxonomic identification than acoustic methods. For aggregates, in situ optical methods inform on the structure of aggregates without fragmenting them or changing their shape as pump systems do or integrating them spatially and temporally as sediment traps do. While no single method captures the entire size range of particles in the ocean at once, a combination of devices can produce a particle spectrum from a few micrometers to several centimeters in size.
Qualitative observations can inform us about biological mechanisms driving particle structure and spatiotemporal variability. Potential insights include both qualitative information about which processes are important (e.g., direct observations of zooplankton–aggregate interactions) as well as quantitative model validation (e.g., using aggregate size distributions to constrain aggregation and disaggregation models). These insights are critical for improving our mechanistic, predictive understanding of processes controlling POC in the ocean.
Continuing developments in this field will move us closer toward the goal of estimating particle flux by constraining the carbon in each particle and its sinking velocity. Yet, this goal requires more ex situ and in situ experimentation, validation, and regional calibration to overcome the fundamental problem of translating optical information on particles (a standing stock) into useful estimates of carbon flux (a process). With a collective effort, optically based flux estimates may soon be feasible on large scales when we draw on the entire wealth of information contained in images from the interior of the ocean.
Author Contributions
SG coordinated the writing of the manuscript. All authors provided valuable input on the full manuscript.
Funding
This work was supported by Scientific Committee on Oceanic Research (SCOR). SG's time was funded by UKRI through National Capability funding.
Conflict of Interest
The authors declare that the research was conducted in the absence of any commercial or financial relationships that could be construed as a potential conflict of interest.
The handling editor is currently co-organizing a Research Topic with several of the authors SG and EC, and confirms the absence of any other collaboration.
Acknowledgments
We thank the Scientific Committee on Oceanic Research (SCOR) and all members of the Working Group 150 (TOMCAT: Translation of Optical Measurements into particle Content, Aggregation & Transfer) for stimulating this work. Our thanks extend to the two reviewers.
Footnotes
1. ^https://www.bodc.ac.uk/resources/vocabularies/vocabulary_search/L22/
2. ^https://www.rd-alliance.org/group/persistent-identification-instruments/case-statement/persistent-identification-instruments
References
Acuña, J., López-Alvarez, M., Nogueira, E., and González-Taboada, F. (2010). Diatom flotation at the onset of the spring phytoplankton bloom: an in situ experiment. Mar. Ecol. Prog. Ser. 400, 115–125. doi: 10.3354/meps08405
Alldredge, A. (1998). The carbon, nitrogen and mass content of marine snow as a function of aggregate size. Deep Sea Res. 45, 529–541. doi: 10.1016/S0967-0637(97)00048-4
Alldredge, A. L., and Gotschalk, C. (1988). In situ settling behavior of marine snow. Limnol. Oceanogr. 33, 339–351. doi: 10.4319/lo.1988.33.3.0339
Alldredge, A. L., and Silver, M. W. (1988). Characteristics, dynamics, and significance of marine snow. Progr. Oceanogr. 20, 41–82. doi: 10.1016/0079-6611(88)90053-5
Alonso-González, I. J., Arístegui, J., Lee, C., Sanchez-Vidal, A., Calafat, A., Fabrés, J., et al. (2010). Role of slowly settling particles in the ocean carbon cycle. Geophys. Res. Lett. 37:L13608. doi: 10.1029/2010GL043827
Armstrong, R. A., Lee, C., Hedges, J. I., Honjo, S., and Wakeham, S. G. (2002). A new mechanistic model for organic carbon fluxes in the ocean based on the quantitative association of POC with ballast minerals. Deep Sea Res. 49, 219–236. doi: 10.1016/S0967-0645(01)00101-1
Azetsu-Scott, K., and Passow, U. (2004). Ascending marine particles: significance of transparent expolymer particles (TEP) in the upper ocean. Limnol. Oceanogr. 49, 741–748. doi: 10.4319/lo.2004.49.3.0741
Bach, L. T., Riebesell, U., Sett, S., Febiri, S., Rzepka, P., and Schulz, K. G. (2012). An approach for particle sinking velocity measurements in the 3–400 μm size range and considerations on the effect of temperature on sinking rates. Mar. Biol. 159, 1853–1864. doi: 10.1007/s00227-012-1945-2
Barbieux, M., Uitz, J., Bricaud, A., Organelli, E., Poteau, A., Schmechtig, C., et al. (2018). Assessing the variability in the relationship between the particulate backscattering coefficient and the chlorophyll a concentration from a global biogeochemical-argo database. J. Geophys. Res. 123, 1229–1250. doi: 10.1002/2017JC013030
Basedow, S. L., de Silva, N. A. L., Bode, A., and van Beusekorn, J. (2016). Trophic positions of mesozooplankton across the North Atlantic: estimates derived from biovolume spectrum theories and stable isotope analyses. J. Plankton Res. 38, 1364–1378. doi: 10.1093/plankt/fbw070
Basedow, S. L., Tande, K. S., Norrbin, M. F., and Kristiansen, S. A. (2013). Capturing quantitative zooplankton information in the sea: performance test of laser optical plankton counter and video plankton recorder in a Calanus finmarchicus dominated summer situation. Prog. Oceanogr. 108, 72–80. doi: 10.1016/j.pocean.2012.10.005
Basedow, S. L., Zhou, M., and Tande, K. S. (2014). Secondary production at the Polar Front, Barents Sea, August 2007. J. Mar. Syst. 130, 147–159. doi: 10.1016/j.jmarsys.2013.07.015
Belcher, A., Iversen, M., Giering, S., Riou, V., Henson, S. A., Berline, L., et al. (2016a). Depth-resolved particle-associated microbial respiration in the northeast Atlantic. Biogeosciences 13, 4927–4943. doi: 10.5194/bg-13-4927-2016
Belcher, A., Iversen, M., Manno, C., Henson, S. A., Tarling, G. A., and Sanders, R. (2016b). The role of particle associated microbes in remineralization of fecal pellets in the upper mesopelagic of the Scotia Sea, Antarctica. Limnol. Oceanogr. 61, 1049–1064. doi: 10.1002/lno.10269
Benfield, M., Grosjean, P., Culverhouse, P., Irigolen, X., Sieracki, M., Lopez-Urrutia, A., et al. (2007). RAPID: research on automated plankton identification. Oceanography 20, 172–187. doi: 10.5670/oceanog.2007.63
Benson, T., and French, J. R. (2007). InSiPID: a new low-cost instrument for in situ particle size measurements in estuarine and coastal waters. J. Sea Res. 58, 167–188. doi: 10.1016/j.seares.2007.04.003
Bi, H., Guo, Z., Benfield, M. C., Fan, C., Ford, M., Shahrestani, S., et al. (2015). A semi-automated image analysis procedure for in situ plankton imaging systems. PLoS ONE. 10:e0127121. doi: 10.1371/journal.pone.0127121
Bienfang, P. K. (1981). SETCOL—a technologically simple and reliable method for measuring phytoplankton sinking rates. Can. J. Fish. Aquat. Sci. 38, 1289–1294. doi: 10.1139/f81-173
Bishop, J. (2009). Autonomous observations of the ocean biological carbon pump. Oceanography 22, 182–193. doi: 10.5670/oceanog.2009.48
Bishop, J. K., Davis, R. E., and Sherman, J. T. (2002). Robotic observations of dust storm enhancement of carbon biomass in the North Pacific. Science 298, 817–821. doi: 10.1126/science.1074961
Bishop, J. K. B. (1986). The correction and suspended particulate matter calibration of Sea Tech transmissometer data. Deep Sea Res. 33, 121–134. doi: 10.1016/0198-0149(86)90111-1
Bishop, J. K. B. (1999). Transmissometer measurement of POC. Deep Sea Res. 46, 353–369. doi: 10.1016/S0967-0637(98)00069-7
Bishop, J. K. B. (2004). Robotic observations of enhanced carbon biomass and export at 55 S during SOFeX. Science 304, 417–420. doi: 10.1126/science.1087717
Bishop, J. K. B., Fong, M. B., and Wood, T. J. (2016). Robotic observations of high wintertime carbon export in California coastal waters. Biogeosciences 13, 3109–3129. doi: 10.5194/bg-13-3109-2016
Bishop, J. K. B., and Wood, T. J. (2009). Year-round observations of carbon biomass and flux variability in the Southern Ocean. Global Biogeochem. Cycles. 23:GB2019. doi: 10.1029/2008GB003206
Blanchard, J. L., Heneghan, R. F., Everett, J. D., Trebilco, R., and Richardson, A. J. (2017). From bacteria to whales: using functional size spectra to model marine ecosystems. Trends Ecol. Evol. 32, 174–186. doi: 10.1016/j.tree.2016.12.003
Bochdansky, A. B., Clouse, M. A., and Herndl, G. J. (2016). Dragon kings of the deep sea: marine particles deviate markedly from the common number-size spectrum. Sci. Rep. 6:22633. doi: 10.1038/srep22633
Bochdansky, A. B., Jericho, M. H., and Herndl, G. J. (2013). Development and deployment of a point-source digital inline holographic microscope for the study of plankton and particles to a depth of 6,000 m. Limnol. Oceanogr. 11, 28–40. doi: 10.4319/lom.2013.11.28
Bochinski, E., Bacha, G., Eiselein, V., Walles, T. J. W., Nejstgaard, J. C., and Sikora, T. (2019). Deep Active Learning for In Situ Plankton Classification. Cham: Springer, 5–15. doi: 10.1007/978-3-030-05792-3_1
Boss, E., Twardowski, M. S., and Herring, S. (2001). Shape of the particulate beam attenuation spectrum and its inversion to obtain the shape of the particulate size distribution. Appl. Opt. 40:4885. doi: 10.1364/AO.40.004885
Bourne, H. L., Bishop, J. K. B., Wood, T. J., Loew, T. J., and Liu, Y. (2019). Carbon flux explorer optical assessment of C, N, and P fluxes. Biogeosciences 16, 1249–1264. doi: 10.5194/bg-16-1249-2019
Boyd, P. W., and Trull, T. W. (2007). Understanding the export of biogenic particles in oceanic waters: is there consensus? Progr. Oceanogr. 72, 276–312. doi: 10.1016/j.pocean.2006.10.007
Briggs, N., Perry, M. J., Cetinić, I., Lee, C., D'Asaro, E., Gray, A. M., et al. (2011). High-resolution observations of aggregate flux during a sub-polar North Atlantic spring bloom. Deep Sea Res. 58, 1031–1039. doi: 10.1016/j.dsr.2011.07.007
Briggs, N. T., Slade, W. H., Boss, E., and Perry, M. J. (2013). Method for estimating mean particle size from high-frequency fluctuations in beam attenuation or scattering measurements. Appl. Opt. 52:6710. doi: 10.1364/AO.52.006710
Buck, J. J. H., Bainbridge, S. J., Burger, E. F., Kraberg, A. C., Casari, M., Casey, K. S., et al. (2019). Ocean data product integration through innovation-the next level of data interoperability. Front. Mar. Sci. 6:32. doi: 10.3389/fmars.2019.00032
Buesseler, K. O. (1998). The decoupling of production and particle export in the surface ocean. Global Biogeochem. Cycles 12, 297–310. doi: 10.1029/97GB03366
Buesseler, K. O. (2001). Ocean biogeochemistry and the global carbon cycle: an introduction to the U.S. Joint Global Ocean Flux Study. Oceanography 14, 1–5. doi: 10.5670/oceanog.2001.01
Buesseler, K. O., Antia, A. N., Chen, M., Fowler, S. W., Gardner, W. D., Gustafsson, O., et al. (2007). An assessment of the use of sediment traps for estimating upper ocean particle fluxes. J. Mar. Res. 65, 345–416. doi: 10.1357/002224007781567621
Buesseler, K. O., Benitez-Nelson, C. R., Moran, S. B., Burd, A., Charette, M., Cochran, J. K., et al. (2006). An assessment of particulate organic carbon to thorium-234 ratios in the ocean and their impact on the application of 234Th as a POC flux proxy. Mar. Chem. 100, 213–233. doi: 10.1016/j.marchem.2005.10.013
Burd, A. B., and Jackson, G. A. (2002). Modeling steady-state particle size spectra. Environ. Sci. Technol. 36, 323–327. doi: 10.1021/es010982n
Burd, A. B., and Jackson, G. A. (2009). Particle aggregation. Ann. Rev. Mar. Sci. 1, 65–90. doi: 10.1146/annurev.marine.010908.163904
Cartwright, G. M., Friedrichs, C. T., and Smith, S. J. (2013). A test of the ADV-based Reynolds flux method for in situ estimation of sediment settling velocity in a muddy estuary. Geo-Mar Lett. 33, 477–484. doi: 10.1007/s00367-013-0340-4
Cavan, E. L., Henson, S. A., Belcher, A., and Sanders, R. (2017). Role of zooplankton in determining the efficiency of the biological carbon pump. Biogeosciences 14, 177–186. doi: 10.5194/bg-14-177-2017
Cavan, E. L., Le Moigne, F. A. C. C., Poulton, A. J., Tarling, G. A., Ward, P., Daniels, C. J., et al. (2015). Attenuation of particulate organic carbon flux in the Scotia Sea, Southern Ocean, is controlled by zooplankton fecal pellets. Geophys. Res. Lett. 42, 821–830. doi: 10.1002/2014GL062744
Ceballos-Romero, E., De Soto, F., Le Moigne, F. A. C., García-Tenorio, R., and Villa-Alfageme, M. (2018). 234Th-derived particle fluxes and seasonal variability: when is the SS assumption reliable? Insights from a novel approach for carbon flux simulation. Geophys. Res. Lett. 45, 13,414–13,426. doi: 10.1029/2018GL079968
Cetinić, I., Perry, M. J., Briggs, N. T., Kallin, E., D'Asaro, E. A., and Lee, C. M. (2012). Particulate organiccarbon and inherent optical properties during 2008 North Atlantic Bloom Experiment. J. Geophys. Res. 117:C06028. doi: 10.1029/2011JC007771.
Checkley, D. M., Davis, R. E., Herman, A. W., Jackson, G. A., Beanlands, B., and Regier, L. A. (2008). Assessing plankton and other particles in situ with the SOLOPC. Limnol. Oceanogr. 53, 2123–2136. doi: 10.4319/lo.2008.53.5_part_2.2123
Christiansen, S., Hoving, H.-J., Schütte, F., Hauss, H., Karstensen, J., Körtzinger, A., et al. (2018). Particulate matter flux interception in oceanic mesoscale eddies by the polychaete Poeobius sp. Limnol. Oceanogr. 63, 2093–2109. doi: 10.1002/lno.10926
Conte, M., and Weber, J. (2014). Particle flux in the deep Sargasso sea: the 35-year oceanic flux program time series. Oceanography 27, 142–147. doi: 10.5670/oceanog.2014.17
Cowen, R. K., and Guigand, C. M. (2008). In situ ichthyoplankton imaging system (I SIIS): system design and preliminary results. Limnol. Oceanogr. 6, 126–132. doi: 10.4319/lom.2008.6.126
Culverhouse, P., Williams, R., Reguera, B., Herry, V., and González-Gil, S. (2003). Do experts make mistakes? A comparison of human and machine identification of dinoflagellates. Mar. Ecol. Prog. Ser. 247, 17–25. doi: 10.3354/meps247017
Dall'Olmo, G., and Mork, K. A. (2014). Carbon export by small particles in the Norwegian Sea. Geophys. Res. Lett. 41, 2921–2927. doi: 10.1002/2014GL059244
Davies, E. J., Buscombe, D., Graham, G. W., and Nimmo-Smith, W. A. M. (2015). Evaluating unsupervised methods to size and classify suspended particles using digital in-line holography. J. Atmos. Ocean. Technol. 32, 1241–1256. doi: 10.1175/JTECH-D-14-00157.1
Davis, C. S., Gallager, S. M., and Solow, A. R. (1992). Microaggregations of oceanic plankton observed by towed video microscopy. Science 257, 230–232. doi: 10.1126/science.257.5067.230
Davis, C. S., Thwaites, F. T., Gallager, S. M., and Hu, Q. (2005). A three-axis fast-tow digital Video Plankton Recorder for rapid surveys of plankton taxa and hydrography. Limnol. Oceanogr. Methods 3, 59–74. doi: 10.4319/lom.2005.3.59
DeVries, T., Primeau, F., and Deutsch, C. (2012). The sequestration efficiency of the biological pump. Geophys. Res. Lett. 39:L13601 doi: 10.1029/2012GL051963
Diercks, A.-R., and Asper, V. L. (1997). In situ settling speeds of marine snow aggregates below the mixed layer: black sea and Gulf of Mexico. Deep Sea Res. 44, 385–398. doi: 10.1016/S0967-0637(96)00104-5
Diercks, A.-R., Dike, C., Asper, V. L., DiMarco, S. F., Chanton, J. P., and Passow, U. (2018). Scales of seafloor sediment resuspension in the northern Gulf of Mexico. Elem. Sci. Anth. 6:32. doi: 10.1525/elementa.285
Ebersbach, F., and Trull, T. W. (2008). Sinking particle properties from polyacrylamide gels during the KErguelen Ocean and Plateau compared Study (KEOPS): zooplankton control of carbon export in an area of persistent natural iron inputs in the Southern Ocean. Limnol. Oceanogr. 53, 212–224. doi: 10.4319/lo.2008.53.1.0212
Ebersbach, F., Trull, T. W., Davies, D. M., and Bray, S. G. (2011). Controls on mesopelagic particle fluxes in the Sub-Antarctic and Polar Frontal Zones in the Southern Ocean south of Australia in summer-Perspectives from free-drifting sediment traps. Deep Sea Res. 58, 2260–2276. doi: 10.1016/j.dsr2.2011.05.025
Ellen, J., Li, H., and Ohman, M. D. (2015). “Quantifying California current plankton samples with efficient machine learning techniques,” in OCEANS 2015—MTS/IEEE Washington (Washington, DC: IEEE), 1–9. doi: 10.23919/OCEANS.2015.7404607
Estapa, M. L., Buesseler, K., Boss, E., and Gerbi, G. (2013). Autonomous, high-resolution observations of particle flux in the oligotrophic ocean. Biogeosciences 10, 5517–5531. doi: 10.5194/bg-10-5517-2013
Finkel, Z. V., Beardall, J., Flynn, K. J., Quigg, A., Rees, T. a. V., and Raven, J. A. (2010). Phytoplankton in a changing world: cell size and elemental stoichiometry. J. Plankton. Res. 32,119–137. doi: 10.1093/plankt/fbp098
Fischer, G., Romero, O., Merkel, U., Donner, B., Iversen, M. H., Nowald, N., et al. (2016). Deep ocean mass fluxes in the coastal upwelling off Mauritania from 1998 to 2012: variability on seasonal to decadal timescales. Biogeosciences 13, 3071–3090. doi: 10.5194/bg-13-3071-2016
Flintrop, C. M., Rogge, A., Miksch, S., Thiele, S., Waite, A. M., and Iversen, M. H. (2018). Embedding and slicing of intact in situ collected marine snow. Limnol. Oceanogr. 16, 339–355. doi: 10.1002/lom3.10251
Francois, R., Honjo, S., Krishfield, R., and Manganini, S. (2002). Factors controlling the flux of organic carbon to the bathypelagic zone of the ocean. Global Biogeochem. Cycles. 16:1087. doi: 10.1029/2001GB001722
Gaardsted, F., Tande, K. S., and Basedow, S. L. (2010). Measuring copepod abundance in deep-water winter habitats in the NE Norwegian Sea: intercomparison of results from laser optical plankton counter and multinet. Fish. Oceanogr. 19, 480–492. doi: 10.1111/j.1365-2419.2010.00558.x
Gärdes, A., Iversen, M. H., Grossart, H.-P., Passow, U., and Ullrich, M. S. (2011). Diatom-associated bacteria are required for aggregation of Thalassiosira weissflogii. ISME J. 5, 436–445. doi: 10.1038/ismej.2010.145
Gardner, W. D., Blakey, J. C., Walsh, I. D., Richardson, M. J., Pegau, S., Zaneveld, J. R. V., et al. (2001). Optics, particles, stratification, and storms on the New England continental shelf. J. Geophys. Res. 106, 9473–9497. doi: 10.1029/2000JC900161
Gardner, W. D., Mishonov, A. V., and Richardson, M. J. (2006). Global POC concentrations from in situ and satellite data. Deep Sea Res. 53, 718–740. doi: 10.1016/j.dsr2.2006.01.029
Gardner, W. D., Walsh, I. D., and Richardson, M. J. (1993). Biophysical forcing of particle production and distribution during a spring bloom in the North Atlantic. Deep Sea Res. 40, 171–195. doi: 10.1016/0967-0645(93)90012-C
Gartner, J. W., Cheng, R. T., Wang, P.-F., and Richter, K. (2001). Laboratory and field evaluations of the LISST-100 instrument for suspended particle size determinations. Marine Geol. 175, 199–219. doi: 10.1016/S0025-3227(01)00137-2
Gehlen, M., Bopp, L., Emprin, N., Aumont, O., Heinze, C., and Ragueneau, O. (2006). Reconciling surface ocean productivity, export fluxes and sediment composition in a global biogeochemical model. Biogeosciences 3, 521–537. doi: 10.5194/bg-3-521-2006
Giering, S. L. C, and Hosking, B. (in review). The interpretation of particle size, shape and carbon flux of marine particle images is strongly affected by the choice of particle detection algorithm. Front. Mar. Sci.
Giering, S. L. C., and Humphreys, M. P. (2018). “Biological Pump,” in Encyclopedia of Geochemistry, Encyclopedia of Earth Sciences Series, ed W. White. (Cham: Springer), 1–6. doi: 10.1007/978-3-319-39193-9_154-1
Giering, S. L. C., Sanders, R., Lampitt, R. S., Anderson, T. R., Tamburini, C., Boutrif, M., et al. (2014). Reconciliation of the carbon budget in the ocean's twilight zone. Nature 507, 480–483. doi: 10.1038/nature13123
Giering, S. L. C., Sanders, R., Martin, A. P., Lindemann, C., Möller, K. O., Daniels, C. J., et al. (2016). High export via small particles before the onset of the North Atlantic spring bloom. J. Geophys. Res. Ocean. 121, 6929–6945. doi: 10.1002/2016JC012048
Gonzalez, H., and Smetacek, V. (1994). The possible role of the cyclopoid copepod Oithona in retarding vertical flux of zooplankton faecal material. Mar. Ecol. Prog. Ser. 113, 233–246. doi: 10.3354/meps113233
Gorsky, G., Ohman, M. D., Picheral, M., Gasparini, S., Stemmann, L., Romagnan, J.-B., et al. (2010). Digital zooplankton image analysis using the ZooScan integrated system. J. Plankton Res. 32, 285–303. doi: 10.1093/plankt/fbp124
Graham, G. W., and Nimmo Smith, W. A. M. (2010). The application of holography to the analysis of size and settling velocity of suspended cohesive sediments. Limnol. Oceanogr. Methods 8, 1–15. doi: 10.4319/lom.2010.8.1
Grosjean, P., Picheral, M., Warembourg, C., and Gorsky, G. (2004). Enumeration, measurement, and identification of net zooplankton samples using the ZOOSCAN digital imaging system. ICES J. Mar. Sci. 61, 518–525. doi: 10.1016/j.icesjms.2004.03.012
Guay, C. K., and Bishop, J. K. (2002). A rapid birefringence method for measuring suspended CaCO3 concentrations in seawater. Deep Sea Res. 49, 197–210. doi: 10.1016/S0967-0637(01)00049-8
Guidi, L., Jackson, G. A., Stemmann, L., Miquel, J. C., Picheral, M., and Gorsky, G. (2008). Relationship between particle size distribution and flux in the mesopelagic zone. Deep. Res. 55, 1364–1374. doi: 10.1016/j.dsr.2008.05.014
Guidi, L., Legendre, L., Reygondeau, G., Uitz, J., Stemmann, L., and Henson, S. A. (2015). A new look at ocean carbon remineralization for estimating deepwater sequestration. Global Biogeochem. Cycles 29, 1044–1059. doi: 10.1002/2014GB005063
Hamm, C. E. (2002). Interactive aggregation and sedimentation of diatoms and clay-sized lithogenic material. Limnol. Oceanogr. 47, 1790–1795. doi: 10.4319/lo.2002.47.6.1790
Heinze, C., Meyer, S., Goris, N., Anderson, L., Steinfeldt, R., Chang, N., et al. (2015). The ocean carbon sink—impacts, vulnerabilities and challenges. Earth Syst. Dyn. 6, 327–358. doi: 10.5194/esd-6-327-2015
Henson, S. A., Sanders, R., Madsen, E., Morris, P. J., Le Moigne, F., and Quartly, G. D. (2011). A reduced estimate of the strength of the ocean's biological carbon pump. Geophys. Res. Lett. 38:L04606. doi: 10.1029/2011GL046735
Herman, A. W. (2004). The next generation of Optical Plankton Counter: the Laser-OPC. J. Plankton Res. 26, 1135–1145. doi: 10.1093/plankt/fbh095
Hill, P. S. (1996). Sectional and discrete representations of floc breakage in agitated suspensions. Deep Sea Res. 43, 679–702. doi: 10.1016/0967-0637(96)00030-1
Holland, H. D. (2006). The oxygenation of the atmosphere and oceans. Philos. Trans. R. Soc. B Biol. Sci. 361, 903–915. doi: 10.1098/rstb.2006.1838
Honjo, S. (1996). “Fluxes of particles to the interior of the open oceans,” in Particle Flux in the Ocean, eds V. Ittekkot, P. Schafer, S. Honjo, and P. J. Depetris (New York, NY: John Wiley & Sons), 91–154.
Honjo, S., Manganini, S. J., Krishfield, R. A., and Francois, R. (2008). Particulate organic carbon fluxes to the ocean interior and factors controlling the biological pump: a synthesis of global sediment trap programs since 1983. Prog. Oceanogr. 76, 217–285. doi: 10.1016/j.pocean.2007.11.003
Hu, Q., and Davis, C. (2005). Automatic plankton image recognition with co-occurrence matrices and Support Vector Machine. Mar. Ecol. Prog. Ser. 295, 21–31. doi: 10.3354/meps295021
Hu, Q., and Davis, C. (2006). Accurate automatic quantification of taxa-specific plankton abundance using dual classification with correction. Mar. Ecol. Prog. Ser. 306, 51–61. doi: 10.3354/meps306051
Iversen, M., and Poulsen, L. (2007). Coprorhexy, coprophagy, and coprochaly in the copepods Calanus helgolandicus, Pseudocalanus elongatus, and Oithona similis. Mar. Ecol. Progr. Ser. 350, 79–89. doi: 10.3354/meps07095
Iversen, M. H., Nowald, N., Ploug, H., Jackson, G. A., and Fischer, G. (2010). High resolution profiles of vertical particulate organic matter export off Cape Blanc, Mauritania: degradation processes and ballasting effects. Deep Sea Res. 57, 771–784. doi: 10.1016/j.dsr.2010.03.007
Iversen, M. H., Pakhomov, E. A., Hunt, B. P. V., van der Jagt, H., Wolf-Gladrow, D., and Klaas, C. (2017). Sinkers or floaters? Contribution from salp pellets to the export flux during a large bloom event in the Southern Ocean. Deep Sea Res. 138, 116–125. doi: 10.1016/j.dsr2.2016.12.004
Iversen, M. H., and Ploug, H. (2010). Ballast minerals and the sinking carbon flux in the ocean: carbon-specific respiration rates and sinking velocity of marine snow aggregates. Biogeosciences 7, 2613–2624. doi: 10.5194/bg-7-2613-2010
Iversen, M. H., and Ploug, H. (2013). Temperature effects on carbon-specific respiration rate and sinking velocity of diatom aggregates—potential implications for deep ocean export processes. Biogeosciences 10, 4073–4085. doi: 10.5194/bg-10-4073-2013
Iversen, M. H., and Robert, M. L. (2015). Ballasting effects of smectite on aggregate formation and export from a natural plankton community. Mar. Chem. 175, 18–27. doi: 10.1016/j.marchem.2015.04.009
Jackson, G. A. (1990). A model of the formation of marine algal flocs by physical coagulation processes. Deep Sea Res. 37, 1197–1211. doi: 10.1016/0198-0149(90)90038-W
Jackson, G. A. (2001). Effect of coagulation on a model planktonic food web. Deep Sea Res. 48, 95–123. doi: 10.1016/S0967-0637(00)00040-6
Jackson, G. A., and Checkley, D. M. (2011). Particle size distributions in the upper 100 m water column and their implications for animal feeding in the plankton. Deep Sea Res. 58, 283–297. doi: 10.1016/j.dsr.2010.12.008
Jackson, G. A., Checkley, D. M., and Dagg, M. (2015). Settling of particles in the upper 100 m of the ocean detected with autonomous profiling floats off California. Deep Sea Res. 99, 75–86. doi: 10.1016/j.dsr.2015.02.001
Jackson, G. A., and Lochmann, S. E. (1992). Effect of coagulation on nutrient and light limitation of an algal bloom. Limnol. Oceanogr. 37, 77–89. doi: 10.4319/lo.1992.37.1.0077
Jackson, G. A., Maffione, R., Costello, D. K., Alldredge, A. L., Logan, B. E., and Dam, H. G. (1997). Particle size spectra between 1 μm and 1 cm at Monterey Bay determined using multiple instruments. Deep Sea Res. 44, 1739–1767. doi: 10.1016/S0967-0637(97)00029-0
Jackson, G. A., Waite, A. M., and Boyd, P. W. (2005). Role of algal aggregation in vertical carbon export during SOIREE and in other low biomass environments. Geophys. Res. Lett. 32:L13607. doi: 10.1029/2005GL023180
Jamet, C., Twardowski, M. S., and Loisel, H. (2018). “Analytical model to derive suspended particulate matter concentration in natural waters by inversion of optical attenuation and backscattering,” in Ocean Sensing and Monitoring X, eds W. Hou, and R. A. Arnone (Santa Rosa, CA: SPIE), 18. doi: 10.1117/12.2309995
Jannasch, H., Zafiriou, O. C., and Farrington, J. W. (1980). A sequencing sediment trap for time-series studies of fragile particles. Limnol. Oceanogr. 25, 939–943. doi: 10.4319/lo.1980.25.5.0939
Jokulsdottir, T., and Archer, D. (2016). A stochastic, Lagrangian model of sinking biogenic aggregates in the ocean (SLAMS 1.0): model formulation, validation and sensitivity. Geosci. Model Dev. 9, 1455–1476. doi: 10.5194/gmd-9-1455-2016
Jónasdóttir, S. H., Visser, A. W., Richardson, K., and Heath, M. R. (2015). Seasonal copepod lipid pump promotes carbon sequestration in the deep North Atlantic. Proc. Natl. Acad. Sci. U.S.A. 112, 12122–12126. doi: 10.1073/pnas.1512110112
Jouandet, M.-P., Trull, T. W., Guidi, L., Picheral, M., Ebersbach, F., Stemmann, L., et al. (2011). Optical imaging of mesopelagic particles indicates deep carbon flux beneath a natural iron-fertilized bloom in the Southern Ocean. Limnol. Oceanogr. 56, 1130–1140. doi: 10.4319/lo.2011.56.3.1130
Karakas, G., Nowald, N., Schäfer-Neth, C., Iversen, M. H., Barkmann, W., Fischer, G., et al. (2009). Impact of particle aggregation on vertical fluxes of organic matter. Prog. Oceanogr. 83, 331–341. doi: 10.1016/j.pocean.2009.07.047
Katija, K., Sherlock, R. E., Sherman, A. D., and Robison, B. H. (2017). New technology reveals the role of giant larvaceans in oceanic carbon cycling. Sci. Adv. 3:e1602374. doi: 10.1126/sciadv.1602374
Kiko, R., Biastoch, A., Brandt, P., Cravatte, S., Hauss, H., Hummels, R., et al. (2017). Biological and physical influences on marine snowfall at the equator. Nat. Geosci. 10, 852–858. doi: 10.1038/ngeo3042
Kilps, J. R., Logan, B. E., and Alldredge, A. L. (1994). Fractal dimensions of marine snow determined from image analysis of in situ photographs. Deep Sea Res. 41, 1159–1169. doi: 10.1016/0967-0637(94)90038-8
Kineke, G. C., Sternberg, R. W., and Johnson, R. (1989). A new instrument for measuring settling velocities in situ. Mar. Geol. 90, 149–158. doi: 10.1016/0025-3227(89)90038-8
Kiørboe, T., Saiz, E., and Visser, A. (1999). Hydrodynamic signal perception in the copepod Acartia tonsa. Mar. Ecol. Prog. Ser. 179, 97–111. doi: 10.3354/meps179097
Klaas, C., and Archer, D. E. (2002). Association of sinking organic matter with various types of mineral ballast in the deep sea: implications for the rain ratio. Global Biochem. Cycles. 16:1116. doi: 10.1029/2001GB001765
Kriest, I. (2002). Different parameterizations of marine snow in a 1D-model and their influence on representation of marine snow, nitrogen budget and sedimentation. Deep Sea Res. 49, 2133–2162. doi: 10.1016/S0967-0637(02)00127-9
Kriest, I., and Evans, G. T. (1999). Representing phytoplankton aggregates in biogeochemical models. Deep Sea Res. 46, 1841–1859. doi: 10.1016/S0967-0637(99)00032-1
Krizhevsky, A., Sutskever, I., and Hinton, G. E. (2012). ImageNet Classification with Deep Convolutional Neural Networks. 1097–1105. Available online at: https://papers.nips.cc/paper/4824-imagenet-classification-with-deep-convolutional-neural-networks (accessed May 9, 2019).
Kwon, E. Y., Primeau, F., and Sarmiento, J. L. (2009). The impact of remineralization depth on the air–sea carbon balance. Nat. Geosci. 2, 630–635. doi: 10.1038/ngeo612
Laurenceau-Cornec, E. C., Trull, T. W., Davies, D. M., Bray, S. G., Doran, J., Planchon, F., et al. (2015b). The relative importance of phytoplankton aggregates and zooplankton fecal pellets to carbon export: insights from free-drifting sediment trap deployments in naturally iron-fertilised waters near the Kerguelen Plateau. Biogeosciences 12, 1007–1027. doi: 10.5194/bg-12-1007-2015
Laurenceau-Cornec, E. C., Trull, T. W., Davies, D. M., De La Rocha, C. L., and Blain, S. (2015a). Phytoplankton morphology controls on marine snow sinking velocity. Mar. Ecol. Progr. Ser. 520, 35–56. doi: 10.3354/meps11116
Le Moigne, F. A. C., Villa-Alfageme, M., Sanders, R. J., Marsay, C., Henson, S., and García-Tenorio, R. (2013). Export of organic carbon and biominerals derived from 234Th and 210Po at the Porcupine Abyssal Plain. Deep Sea Res. 72, 88–101. doi: 10.1016/j.dsr.2012.10.010
Le Quéré, C., Rödenbeck, C., Buitenhuis, E. T., Conway, T. J., Langenfelds, R., Gomez, A., et al. (2007). Saturation of the southern ocean CO2 sink due to recent climate change. Science 316, 1735–1738. doi: 10.1126/science.1136188
Lindsay, D. J., Yamaguchi, A., Nishikawa, J., Sabates, A., Fuentes, V., Hall, M. R., et al. (2014). Vertical profiles of marine particulates: a step towards global scale comparisons using an Autonomous Visual Plankton Recorder. Bull. Plankt. Soc. Jpn. 61, 72–81. doi: 10.24763/bpsj.61.1_72
Lombard, F., Boss, E., Waite, A. M., Vogt, M., Uitz, J., Stemmann, L., et al. (2019). Globally consistent quantitative observations of planktonic ecosystems. Front. Mar. Sci. 6:196. doi: 10.3389/fmars.2019.00196
Long, M., Moriceau, B., Gallinari, M., Lambert, C., Huvet, A., Raffray, J., et al. (2015). Interactions between microplastics and phytoplankton aggregates: impact on their respective fates. Mar. Chem. 175, 39–46. doi: 10.1016/j.marchem.2015.04.003
Lumini, A., and Nanni, L. (2019). Deep learning and transfer learning features for plankton classification. Ecol. Inform. 51, 33–43. doi: 10.1016/j.ecoinf.2019.02.007
Luo, J. Y., Irisson, J. O., Graham, B., Guigand, C., Sarafraz, A., Mader, C., et al. (2018). Automated plankton image analysis using convolutional neural networks. Limnol. Oceanogr. Methods 16, 814–827. doi: 10.1002/lom3.10285
Marcolin, C. R., Lopes, R. M., and Jackson, G. A. (2015). Estimating zooplankton vertical distribution from combined LOPC and ZooScan observations on the Brazilian Coast. Mar. Biol. 162, 2171–2186. doi: 10.1007/s00227-015-2753-2
Martin, J. H., Knauer, G. A., Karl, D. M., and Broenkow, W. W. (1987). VERTEX: carbon cycling in the Northeast Pacific. Deep Sea Res. 34, 267–285. doi: 10.1016/0198-0149(87)90086-0
Martin, P., Lampitt, R. S., Jane Perry, M., Sanders, R., Lee, C., and D'Asaro, E. (2011). Export and mesopelagic particle flux during a North Atlantic spring diatom bloom. Deep Sea Res. 58, 338–349. doi: 10.1016/j.dsr.2011.01.006
Matear, R. J., and Hirst, A. C. (1999). Climate change feedback on the future oceanic CO2 uptake. Tellus B Chem. Phys. Meteorol. 51,722–733. doi: 10.3402/tellusb.v51i3.16472.
McDonnell, A. M. P., and Buesseler, K. O. (2010). Variability in the average sinking velocity of marine particles. Limnol. Oceanogr. 55, 2085–2096. doi: 10.4319/lo.2010.55.5.2085
McDonnell, A. M. P., and Buesseler, K. O. (2012). A new method for the estimation of sinking particle fluxes from measurements of the particle size distribution, average sinking velocity, and carbon content. Limnol. Oceanogr. Methods 10, 329–346. doi: 10.4319/lom.2012.10.329
Miklasz, K. A., and Denny, M. W. (2010). Diatom sinkings speeds: improved predictions and insight from a modified Stokes' law. Limnol. Oceanogr. 55, 2513–2525. doi: 10.4319/lo.2010.55.6.2513
Möller, K., St. John, M., Temming, A., Floeter, J., Sell, A., Herrmann, J., et al. (2012). Marine snow, zooplankton and thin layers: indications of a trophic link from small-scale sampling with the Video Plankton Recorder. Mar. Ecol. Prog. Ser. 468, 57–69. doi: 10.3354/meps09984
Moore, J. K., and Villareal, T. A. (1996). Size-ascent relationships in positively buoyant marine diatoms. Limnol. OCeanogr. 41, 1514–1520. doi: 10.4319/lo.1996.41.7.1514
Moradi, N., Liu, B., Iversen, M., Kuypers, M. M., Ploug, H., and Khalili, A. (2018). A new mathematical model to explore microbial processes and their constraints in phytoplankton colonies and sinking marine aggregates. Sci. Adv. 4:eaat1991. doi: 10.1126/sciadv.aat1991
Mori, M., and Lindsay, D. J. (2008). Body pigmentation changes in the planktonic crustacean Vibilia stebbingi (Amphipoda: Hyperiidea) under different light regimes, with notes on implications for the development of automated plankton identification systems. JAMSTEC R. Res. Develop. 8, 37–45. doi: 10.5918/jamstecr.8.37
Murray, P. B., McCave, I. N., Owen, T. R. E., Mason, M., and Green, M. O. (1996). A robust in situ settling velocity box for coastal seas. J. Sea Res. 36, 101–107. doi: 10.1016/S1385-1101(96)90778-9
Nakamura, Y., Somiya, R., Suzuki, N., Hidaka-Umetsu, M., Yamaguchi, A., and Lindsay, D. J. (2017). Optics-based surveys of large unicellular zooplankton: a case study on radiolarians and phaeodarians. Plank. Benthos Res. 12, 96–103. doi: 10.3800/pbr.12.95
Nayak, A. R., McFarland, M. N., Sullivan, J. M., and Twardowski, M. S. (2018). Evidence for ubiquitous preferential particle orientation in representative oceanic shear flows. Limnol. Oceanogr. 63, 122–143. doi: 10.1002/lno.10618
Nowald, N., Iversen, M. H., Fischer, G., Ratmeyer, V., and Wefer, G. (2015). Time series of in situ particle properties and sediment trap fluxes in the coastal upwelling filament off Cape Blanc, Mauritania. Prog. Oceanogr. 137, 1–11. doi: 10.1016/j.pocean.2014.12.015
O'Brien, K. R., Meyer, D. L., Waite, A. M., Ivey, G. N., and Hamilton, D. P. (2004). Disaggregation of Microcystis aeruginosa colonies under turbulent mixing: laboratory experiments in a grid-stirred tank. Hydrobiologia 519, 143–152 doi: 10.1023/B:HYDR.0000026501.02125.cf
O'Brien, K. R., Waite, A. M., Alexander, B. L., Perry, K. A., and Neumann, L. E. (2006). Particle tracking in a salinity gradient: a method for measuring sinking rate of individual phytoplankton in the laboratory. Limnol. Oceanogr. Methods 4, 329–335 doi: 10.4319/lom.2006.4.329
Ohman, M. D., Davis, R. E., Sherman, J. T., Grindley, K. R., Whitmore, B. M., Nickels, C. F., et al. (2019). Zooglider: an autonomous vehicle for optical and acoustic sensing of zooplankton. Limnol. Oceanogr. 17, 69–86. doi: 10.1002/lom3.10301
Ohman, M. D., Powell, J. R., Picheral, M., and Jensen, D. W. (2012). Mesozooplankton and particulate matter responses to a deep-water frontal system in the southern California Current System. J. Plankton Res. 34, 815–827. doi: 10.1093/plankt/fbs028
Orenstein, E. C., and Beijbom, O. (2017). “Transfer learning and deep feature extraction for planktonic image data sets,” in 2017 IEEE Winter Conference on Applications of Computer Vision (WACV) (Santa Rosa, CA: IEEE), 1082–1088. doi: 10.1109/WACV.2017.125
Passow, U., and Carlson, C. (2012). The biological pump in a high CO2 world. Mar. Ecol. Prog. Ser. 470, 249–271. doi: 10.3354/meps09985
Passow, U., De La Rocha, C. L., Fairfield, C., and Schmidt, K. (2014). Aggregation as a function of and mineral particles. Limnol. Oceanogr. 59, 532–547. doi: 10.4319/lo.2014.59.2.0532
Passow, U., Sweet, J., Francis, S., Xu, C., Dissanayake, A., Lin, Y., et al. (2019). Incorporation of oil into diatom aggregates. Mar. Ecol. Prog. Ser. 612, 65–86. doi: 10.3354/meps12881
Peterson, M. L., Fabres, J., Wakeham, S. G., Lee, C., Alonso, I. J., and Miquel, J. C. (2009). Sampling the vertical particle flux in the upper water column using a large diameter free-drifting NetTrap adapted to an Indented Rotating Sphere sediment trap. Deep Sea Res. 56, 1547–1557. doi: 10.1016/j.dsr2.2008.12.020
Peterson, M. L., Wakeham, S. G., Lee, C., Askea, M. A., and Miquel, J. C. (2005). Novel techniques for collection of sinking particles in the ocean and determining their settling rates. Limnol. Oceanogr. Methods 3, 520–532. doi: 10.4319/lom.2005.3.520
Petrik, C. M., Jackson, G. A., and Checkley, D. M. (2013). Aggregates and their distributions determined from LOPC observations made using an autonomous profiling float. Deep Sea Res. 74, 64–81. doi: 10.1016/j.dsr.2012.12.009
Picheral, M., Colin, S., and Irisson, J.-O. (2017). EcoTaxa, a Tool for the Taxonomic Classification of Images. Available online at: http://ecotaxa.obs-vlfr.fr (accessed January 14, 2020).
Picheral, M., Guidi, L., Stemmann, L., Karl, D. M., Iddaoud, G., and Gorsky, G. (2010). The Underwater Vision Profiler 5: an advanced instrument for high spatial resolution studies of particle size spectra and zooplankton. Limnol. Oceanogr. Methods 8, 462–473. doi: 10.4319/lom.2010.8.462
Pilskaln, C. H., Lehmann, C., Paduan, J. B., and Silver, M. W. (1998). Spatial and temporal dynamics in marine aggregate abundance, sinking rate, and flux: Monterey Bay, central California. Deep Sea Res. 45, 1803–1837. doi: 10.1016/S0967-0645(98)80018-0
Ploug, H. (2001). Small-scale oxygen fluxes and remineralization in sinking aggregates. Limnol. Oceanogr. 46, 1624–1631. doi: 10.4319/lo.2001.46.7.1624
Ploug, H., and Grossart, H.-P. (1999). Bacterial production and respiration in suspended aggregates - a matter of the incubation method. Aquat. Microb. Ecol. 20, 21–29. doi: 10.3354/ame020021
Ploug, H., Iversen, M. H., and Fischer, G. (2008b). Ballast, sinking velocity, and apparent diffusivity within marine snow and zooplankton fecal pellets: implications for substrate turnover by attached bacteria. Limnol. Oceanogr. 53, 1878–1886. doi: 10.4319/lo.2008.53.5.1878
Ploug, H., Iversen, M. H., Koski, M., and Buitenhuis, E. T. (2008a). Production, oxygen respiration rates, and sinking velocity of copepod fecal pellets: direct measurements of ballasting by opal and calcite. Limnol. Oceanogr. 53, 469–476. doi: 10.4319/lo.2008.53.2.0469
Ploug, H., and Jørgensen, B. (1999). A net-jet flow system for mass transfer and microsensor studies of sinking aggregates. Mar. Ecol. Prog. Ser. 176, 279–290. doi: 10.3354/meps176279
Ploug, H., Terbrüggen, A., Kaufmann, A., Wolf-Gladrow, D., and Passow, U. (2010). A novel method to measure particle sinking velocity in vitro, and its comparison to three other in vitro methods. Limnol. Oceanogr. Methods 8, 386–393. doi: 10.4319/lom.2010.8.386
Poulsen, L., and Iversen, M. (2008). Degradation of copepod fecal pellets: key role of protozooplankton. Mar. Ecol. Progr. Ser. 367, 1–13. doi: 10.3354/meps07611
Prairie, J. C., Ziervogel, K., Camassa, R., McLaughlin, R. M., White, B. L., Dewald, C., et al. (2015). Delayed settling of marine snow: effects of density gradient and particle properties and implications for carbon cycling. Mar. Chem. 175, 28–38. doi: 10.1016/j.marchem.2015.04.006
Py, O., Hong, H., and Zhongzhi, S. (2016). “Plankton classification with deep convolutional neural networks,” in 2016 IEEE Information Technology, Networking, Electronic and Automation Control Conference (Chongqing: IEEE), 132−136. doi: 10.1109/ITNEC.2016.7560334
Ramondenc, S., Madeleine, G., Lombard, F., Santinelli, C., Stemmann, L., Gorsky, G., et al. (2016). An initial carbon export assessment in the Mediterranean Sea based on drifting sediment traps and the Underwater Vision Profiler data sets. Deep Sea Res. 117, 107–119. doi: 10.1016/j.dsr.2016.08.015
Rembauville, M., Briggs, N., Ardyna, M., Uitz, J., Catala, P., Penkerc'h, C., et al. (2017). Plankton assemblage estimated with BGC-Argo floats in the Southern Ocean: implications for seasonal successions and particle export. J. Geophys. Res. Ocean. 122, 8278–8292. doi: 10.1002/2017JC013067
Reygondeau, G., Guidi, L., Beaugrand, G., Henson, S. A., Koubbi, P., MacKenzie, B. R., et al. (2018). Global biogeochemical provinces of the mesopelagic zone. J. Biogeogr. 45, 500–514. doi: 10.1111/jbi.13149
Reynolds, R. A., Stramski, D., and Neukermans, G. (2016). Optical backscattering by particles in Arctic seawater and relationships to particle mass concentration, size distribution, and bulk composition. Limnol. Oceanogr. 61, 1869–1890. doi: 10.1002/lno.10341
Riley, J. S., Sanders, R., Marsay, C., Le Moigne, F. A. C., Achterberg, E. P., and Poulton, A. J. (2012). The relative contribution of fast and slow sinking particles to ocean carbon export. Global Biogeochem. Cycles 26:GB1026. doi: 10.1029/2011GB004085
Roca-Martí, M., Puigcorbé, V., Iversen, M. H., van der loeff, M. R., Klaas, C., Cheah, W., et al. (2017). High particulate organic carbon export during the decline of a vast diatom bloom in the Atlantic sector of the Southern Ocean. Deep Sea Res. 138, 102–115, doi: 10.1016/j.dsr2.2015.12.007
Saminsky, M. W., and Gallager, S. M (2018). “Plankton image classification, storage, visualization, and analytics leveraging cloud computing,” in Poster Presentation at Ocean Sciences Meeting 2018 (Portland).
Samson, S., Langebrake, L., Patten, J., and Lembke, C. (2004). Shadowed Image Particle Profiling and Evaluation Recorder. 15. Available online at: https://patentimages.storage.googleapis.com/25/63/1a/dae1f3cba0f871/US20040109586A1.pdf (accessed January 14, 2020).
Schallenberg, C., Harley, J. W., Jansen, P., Davies, D. M., and Trull, T. W. (2019). Multi-year observations of fluorescence and backscatter at the Southern Ocean Time Series (SOTS) shed light on two distinct seasonal bio-optical regimes. Front. Mar. Sci. 6. doi: 10.3389/fmars.2019.00595
Schlitzer, R. (2002). Carbon export fluxes in the Southern Ocean: results from inverse modeling and comparison with satellite-based estimates. Deep Sea Res. 49, 1623–1644. doi: 10.1016/S0967-0645(02)00004-8
Schmid, M. S., Aubry, C., Grigor, J., and Fortier, L. (2016). The LOKI underwater imaging system and an automatic identification model for the detection of zooplankton taxa in the Arctic Ocean. Methods Oceanogr. 15–16, 129–160. doi: 10.1016/j.mio.2016.03.003
Schröder, S. M., Kiko, R., Irisson, J. O., and Koch, R. (2019). “Low-shot learning of plankton categories,” in Pattern Recognition. GCPR 2018. Lecture Notes in Computer Science, Vol. 11269, eds T. Brox, A. Bruhn, and M. Fritz (Cham: Springer).
Sheldon, R. W., Prakash, A., and Sutcliffe, W. H. (1972). The size distribution of particles in the ocean. Limnol. Oceanogr. 17, 327–340. doi: 10.4319/lo.1972.17.3.0327
Simon, M., Grossart, H. P., Schweitzer, B., and Ploug, H. (2002). Microbial ecology of organic aggregates in aquatic ecosystems. Aquat. Microb. Ecol. 28, 175–211. doi: 10.3354/ame028175
Slade, W. H., and Boss, E. (2015). Spectral attenuation and backscattering as indicators of average particle size. Appl. Opt. 54:7264. doi: 10.1364/AO.54.007264
Smith, S. J., and Friedrichs, C. T. (2015). Image processing methods for in situ estimation of cohesive sediment floc size, settling velocity, and density. Limnol. Oceanogr. 13, 250–264. doi: 10.1002/lom3.10022
Sosik, H. M., and Olson, R. J. (2007). Automated taxonomic classification of phytoplankton sampled with imaging-in-flow cytometry. Limnol. Oceanogr. 5, 204–216. doi: 10.4319/lom.2007.5.204
Spinrad, R. W., Bartz, R., and Kitchen, J. C. (1989). In situ measurements of marine particle settling velocity and size distributions using the remote optical settling tube. J. Geophys. Res. 94:931. doi: 10.1029/JC094iC01p00931
Steinberg, D. K., Carlson, C. A., Bates, N. R., Goldthwait, S. A., Madin, L. P., et al. (2000). Zooplankton vertical migration and the active transport of dissolved organic and inorganic carbon in the Sargasso Sea. Deep Sea Res. 47, 137–158. doi: 10.1016/S0967-0637(99)00052-7
Steinbuck, J. V., Roberts, P. L. D., Troy, C. D., Horner-Devine, A. R., Simonet, F., Uhlman, A. H., et al. (2010). An autonomous open-ocean stereoscopic PIV profiler. J. Atmos. Oceanic Technol. 27, 1362–1380. doi: 10.1175/2010JTECHO694.1
Stemmann, L., Jackson, G. A., and Gorsky, G. (2004a). A vertical model of particle size distributions and fluxes in the midwater column that includes biological and physical processes—Part II: application to a three year survey in the NW Mediterranean Sea. Deep Sea Res. 51, 885–908. doi: 10.1016/j.dsr.2004.03.002
Stemmann, L., Jackson, G. A., and Ianson, D. (2004b). A vertical model of particle size distributions and fluxes in the midwater column that includes biological and physical processes—Part I: model formulation. Deep Sea Res. 51, 865–884. doi: 10.1016/j.dsr.2004.03.001
Svensen, C., Morata, N., and Reigstad, M. (2014). Increased degradation of copepod faecal pellets by co-acting dinoflagellates and Centropages hamatus. Mar. Ecol. Prog. Ser. 516, 61–70. doi: 10.3354/meps10976
Talapatra, S., Hong, J., McFarland, M., Nayak, A., Zhang, C., Katz, J., et al. (2013). Characterization of biophysical interactions in the water column using in situ digital holography. Mar. Ecol. Progr. Ser. 473, 29–51. doi: 10.3354/meps10049
Tang, X., Stewart, W. K., Huang, H., Gallager, S. M., Davis, C. S., Vincent, L., et al. (1998). Automatic plankton image recognition. Artif. Intell. Rev. 12, 177–199. doi: 10.1023/A:1006517211724
Taucher, J., Bach, L. T., Riebesell, U., and Oschlies, A. (2014). The viscosity effect on marine particle flux: a climate relevant feedback mechanism. Global Biogeochem. Cycles 28, 415–422. doi: 10.1002/2013GB004728
Thiele, S., Fuchs, B. M., Amann, R., and Iversen, M. H. (2015). Colonization in the photic zone and subsequent changes during sinking determine bacterial community composition in marine snow. Appl. Environ. Microbiol. 81, 1463–1471. doi: 10.1128/AEM.02570-14
Trudnowska, E., Basedow, S. L., and Blachowiak-Samolyk, K. (2014). Mid-summer mesozooplankton biomass, its size distribution, and estimated production within a glacial Arctic fjord (Hornsund, Svalbard). J. Mar. Syst. 137, 55–66. doi: 10.1016/j.jmarsys.2014.04.010
Trull, T., Bray, S. G., Buesseler, K. O., Lamborg, C. H., Manganini, S., Moy, C., et al. (2008). In situ measurement of mesopelagic particle sinking rates and the control of carbon transfer to the ocean interior during the Vertical Flux in the Global Ocean (VERTIGO) voyages in the North Pacific. Deep Sea Res. 55, 1684–1695. doi: 10.1016/j.dsr2.2008.04.021
Turner, J. T. (2002). Zooplankton fecal pellets, marine snow and sinking phytoplankton blooms. Aquat. Microb. Ecol. 27, 57–102. doi: 10.3354/ame027057
Turner, J. T. (2015). Zooplankton fecal pellets, marine snow, phytodetritus and the ocean's biological pump. Prog. Oceanogr. 130, 205–248. doi: 10.1016/j.pocean.2014.08.005
Twardowski, M., Zhang, X., Vagle, S., Sullivan, J., Freeman, S., Czerski, H., et al. (2012). The optical volume scattering function in a surf zone inverted to derive sediment and bubble particle subpopulations. J. Geophys. Res. 117. doi: 10.1029/2011JC007347
Twardowski, M. S., Boss, E., Macdonald, J. B., Pegau, W. S., Barnard, A. H., and Zaneveld, J. R. V. (2001). A model for estimating bulk refractive index from the optical backscattering ratio and the implications for understanding particle composition in case I and case II waters. J. Geophys. Res. 106, 14129–14142. doi: 10.1029/2000JC000404
van der Jagt, H., Friese, C., Stuut, J.-B. W., Fischer, G., and Iversen, M. H. (2018). The ballasting effect of Saharan dust deposition on aggregate dynamics and carbon export: aggregation, settling, and scavenging potential of marine snow. Limnol. Oceanogr. 63, 1386–1394. doi: 10.1002/lno.10779
Villa-Alfageme, M., de Soto, F., Le Moigne, F. A. C., Giering, S. L. C., Sanders, R., and García-Tenorio, R. (2014). Observations and modeling of slow-sinking particles in the twilight zone. Global Biogeochem. Cycles 28, 1327–1342. doi: 10.1002/2014GB004981
Villa-Alfageme, M., de Soto, F. C., Ceballos, E., Giering, S. L. C., Le Moigne, F. A. C., Henson, S., et al. (2016). Geographical, seasonal, and depth variation in sinking particle speeds in the North Atlantic. Geophys. Res. Lett. 43, 8609–8616. doi: 10.1002/2016GL069233
Visser, A. W. (2001). Hydromechanical signals in the plankton. Mar. Ecol. Prog. Ser. 222, 1–24. doi: 10.3354/meps222001
Visser, A. W., and Jackson, G. A. (2004). Characteristics of the chemical plume behind a sinking particle in a turbulent water column. Mar. Ecol. Prog. Ser. 283, 55–71. doi: 10.3354/meps283055
Volk, T., and Hoffert, I. (1985). “Ocean carbon pumps: analysis of relative strengths and efficiencies in ocean driven atmospheric CO2 changes,” in The Carbon Cycle and Atmospheric CO2: Natural Variations Archean to Present. Chapman Conference Paper, eds E. Sundquist and W. Broecker (Washington, DC: American Geophysical Union, Geophysical Monograph), 99–110.
Waite, A. M., Fisher, A., Thompson, P., and Harrison, P. (1997a). Sinking rate vs. cell volume relationships illuminate sinking rate control mechanisms in marine diatoms. Mar. Ecol. Prog. Ser. 157, 97–108. doi: 10.3354/meps157097
Waite, A. M., Gallager, S., and Dam, H. G. (1997b). New measurements of phytoplankton aggregation in a flocculator using videography and image analysis. Mar. Ecol. Prog. Ser. 155, 77–88 doi: 10.3354/meps155077
Waite, A. M., and Hill, P. (2006). Flocculation and phytoplankton cell size can alter 234Th-based estimates of the vertical flux of particulate organic carbon in the sea. Mar. Chem. 100, 366–375. doi: 10.1016/j.marchem.2005.10.021
Waite, A. M., and Nodder, S. D. (2001). The effect of in situ iron addition on the sinking rates and export flux of Southern Ocean diatoms. Deep Sea Res. 48, 2635–2654. doi: 10.1016/S0967-0645(01)00012-1
Waite, A. M., Safi, K. A., Hall, J. A., and Nodder, S. D. (2000). Mass sedimentation of picoplankton embedded in organic aggregates. Limnol. Oceanogr. 45, 87–97 doi: 10.4319/lo.2000.45.1.0087
Waite, A. M., Thompson, P. A., and Harrison, P. J. (1992). Does energy control the sinking rates of marine diatoms? Limnol. Oceanogr. 37, 468–477. doi: 10.4319/lo.1992.37.3.0468
Walsh, I., and Gardner, W. (1992). “Large aggregate distribution during the Jgofs North Atlantic bloom experiment,” in Primary Productivity and Biogeochemical Cycles in the Sea, Environmental Science Research, Vol. 43, eds P. G. Falkowski, A. D. Woodhead, K. Vivirito (Boston, MA: Springer), 530. doi: 10.1007/978-1-4899-0762-2_70
Weber, T., Cram, J. A., Leung, S. W., DeVries, T., and Deutsch, C. (2016). Deep ocean nutrients imply large latitudinal variation in particle transfer efficiency. Proc. Natl. Acad. Sci. U.S.A. 113, 8606–8611. doi: 10.1073/pnas.1604414113
Wekerle, C., Krumpen, T., Dinter, T., von Appen, W.-J., Iversen, M. H., and Salter, I. (2018). Properties of sediment trap catchment areas in fram strait: results from lagrangian modeling and remote sensing. Front. Mar. Sci. 5:407. doi: 10.3389/fmars.2018.00407
Wilkinson, M. D., Dumontier, M., Aalbersberg, I. j., Appleton, G., Axton, M., et al. (2016). The FAIR Guiding Principles for scientific data management and stewardship. Sci. Data 3:160018. doi: 10.1038/sdata.2016.18
Wilson, S. E., Steinberg, D. K., and Buesseler, K. O. (2008). Changes in fecal pellet characteristics with depth as indicators of zooplankton repackaging of particles in the mesopelagic zone of the subtropical and subarctic North Pacific Ocean. Deep Sea Res. 55, 1636–1647. doi: 10.1016/j.dsr2.2008.04.019
Yu, E.-F., Francois, R., Bacon, M. P., Honjo, S., Fleer, A. P., Manganini, S. J., et al. (2001). Trapping efficiency of bottom-tethered sediment traps estimated from the intercepted fluxes of 230Th and 231Pa. Deep-Sea Res. 48, 865–889. doi: 10.1016/S0967-0637(00)00067-4
Zaneveld, J. R. V., Spinrad, R. W., and Bartz, R. (1982). An optical settling tube for the determination of particle-size distributions. Mar. Geol. 49, 357–375. doi: 10.1016/0025-3227(82)90049-4
Zheng, H., Wang, R., Yu, Z., Wang, N., Gu, Z., and Zheng, B. (2017). Automatic plankton image classification combining multiple view features via multiple kernel learning. BMC Bioinform. 18:570. doi: 10.1186/s12859-017-1954-8
Keywords: sinking particle fluxes, sinking velocities, carbon content, size, image processing, automated classification, in situ optical particle measurements, biological carbon pump
Citation: Giering SLC, Cavan EL, Basedow SL, Briggs N, Burd AB, Darroch LJ, Guidi L, Irisson J-O, Iversen MH, Kiko R, Lindsay D, Marcolin CR, McDonnell AMP, Möller KO, Passow U, Thomalla S, Trull TW and Waite AM (2020) Sinking Organic Particles in the Ocean—Flux Estimates From in situ Optical Devices. Front. Mar. Sci. 6:834. doi: 10.3389/fmars.2019.00834
Received: 01 June 2019; Accepted: 27 December 2019;
Published: 18 February 2020.
Edited by:
Carol Robinson, University of East Anglia, United KingdomReviewed by:
Michael Twardowski, Florida Atlantic University, United StatesAlexander B. Bochdansky, Old Dominion University, United States
Copyright © 2020 Giering, Cavan, Basedow, Briggs, Burd, Darroch, Guidi, Irisson, Iversen, Kiko, Lindsay, Marcolin, McDonnell, Möller, Passow, Thomalla, Trull and Waite. This is an open-access article distributed under the terms of the Creative Commons Attribution License (CC BY). The use, distribution or reproduction in other forums is permitted, provided the original author(s) and the copyright owner(s) are credited and that the original publication in this journal is cited, in accordance with accepted academic practice. No use, distribution or reproduction is permitted which does not comply with these terms.
*Correspondence: Sarah Lou Carolin Giering, cy5naWVyaW5nQG5vYy5hYy51aw==