- 1Stockholm Resilience Centre, Stockholm University, Stockholm, Sweden
- 2Marine Biology Research Division, Scripps Institution of Oceanography, University of California, San Diego, La Jolla, CA, United States
- 3National Center for Ecological Analysis and Synthesis, Santa Barbara, CA, United States
- 4Future Earth, Colorado State University, Fort Collins, CO, United States
- 5MarFishEco Fisheries Consultants, London, United Kingdom
- 6CSIRO Marine and Atmospheric Research, Hobart, TAS, Australia
- 7Nicholas School of the Environment, Duke University, Beaufort, NC, United States
The sustainable governance and management of small-scale fisheries (SSF) is challenging, largely due to their dynamic and complex nature. Agent-based modeling (ABM) is a computational modeling approach that can account for the dynamism and complexity in SSF by modeling entities as individual agents with different characteristics and behavior, and simulate how their interactions can give rise to emergent phenomena, such as over-fishing and social inequalities. The structurally realistic design of agent-based models allow stakeholders, experts, and scientists across disciplines and sectors to reconcile different knowledge bases, assumptions, and goals. ABMs can also be designed using any combination of theory, quantitative data, or qualitative data. In this publication we elaborate on the untapped potential of ABM to tackle governance and management challenges in SSF, discuss the limitations of ABM, and review its application in published SSF models. Our review shows that, although few models exist to date, ABM has been used for diverse purposes, including as a research tool for understanding cooperation and over-harvesting, and as a decision-support tool, or participatory tool, in case-specific fisheries. Even though the development of ABMs is often time- and resource intensive, it is the only dynamic modeling approach that can represent entities of different types, their heterogeneity, actions, and interactions, thus doing justice to the complex and dynamic nature of SSF which, if ignored can lead to unintended policy outcomes and less sustainable SSF.
Introduction
The sustainable governance and management of small-scale fisheries (SSFs) has proven to be a tremendous challenge, exacerbated by their complex nature (Mahon et al., 2008; McClanahan et al., 2009; The World Bank, 2012; FAO, 2014). At the heart of this complexity lies the high diversity of the social, institutional, and ecological entities in SSF, the interactions within and among these entities, and the range of outcomes that arise from these interactions (Degnbol and McCay, 2007; Ostrom, 2007; Smith and Basurto, 2019). The wider context that SSF are embedded in adds to this complexity, e.g., international trade relationships, fishers’ migration, technological change, and increased tourism (Kittinger et al., 2013; Crona et al., 2015; Eriksson et al., 2015). Apart from this complexity, the frequent lack of fine resolution cultural, socio-economic, and ecological data complicates the development, implementation, and evaluation of policies and management strategies that are sensitive to the local context (Jentoft, 2017; Johnson et al., 2017; Agapito et al., 2019). The combination of complexity and lack of information limits our understanding of how a policy will be received, and often lie at the heart of unintended policy outcomes (Degnbol and McCay, 2007; Lewison et al., 2019). Important advances have been made by meta-studies highlighting common factors that can be linked to sustainable outcomes, such as leadership, social cohesion, and co-management (Gutierrez et al., 2011; Ovando et al., 2013; Crona et al., 2015; Cinner et al., 2016). However, less attention has been paid to why and how these factors and processes occur in the first place, how they interact to produce (un)intended results, or how they can be promoted through policies.
Agent-based modeling (ABM) is a computational modeling approach that may help embrace complexity and overcome information paucity-related problems. Different from other modeling approaches, ABM allows for the assessment of why and how interactions between different actors and their environment result in a particular outcome, while accounting for the impacts of different external drivers (Bousquet and Le Page, 2004). In a fisheries context, ABM allows exploration of how agents (e.g., fishers, traders, fleets) change their behavior in response to changes in their environment. This is different from standard fisheries models, which typically aim at predicting future states of a fishery by modeling variables at the macro-level (e.g., fleet behavior, fishing effort, market demand) as characteristic of the whole fleet (Quinn, 2003; van Putten et al., 2012; Nielsen et al., 2018). Even though standard fishery models are powerful, they are often not adequately flexible or sensitive to the micro-level complexities of SSF (Weber et al., 2019), nor able to fully represent the social dimension in fisheries (Fulton et al., 2011; van Putten et al., 2012; Burgess et al., 2018).
There are a number of advantages to using ABMs over traditional fisheries models. They enable qualitative and quantitative data to be combined to understand the underlying processes of empirical phenomena (An et al., 2005; Edmonds, 2017; Magliocca et al., 2018). Similarly, ABMs can be interactive and collaborative and have the potential to bring together different stakeholder groups, thus reconciling different knowledge bases, assumptions, and goals (Poteete et al., 2010; Étienne, 2013; Taylor et al., 2016). Finally, they allow the integration of diverse knowledge to ask questions about how particular behaviors at the individual level could give rise to patterns at larger scales and what interactions and processes may have produced a given outcome or pattern (An et al., 2005; Heckbert et al., 2010; Conte and Paolucci, 2014).
This paper aims to elaborate on the untapped potential of ABM to tackle complex governance and management challenges in SSFs. We first outline what ABM is and how the development process of an agent-based model may take place. We present a short review of SSF publications that use ABMs, detail three challenges for SSF management and governance, and use examples from our review to illustrate how ABMs have been used to help address them. We end with a discussion on the potential usefulness of ABM to address contemporary SSF challenges and discuss what is needed to unlock this potential. The intended audience of this paper are those interested in having more tools available to address SSF management and governance issues and questions that relate to complexity, those curious about using ABM, and those who design, manage, or participate in ABM projects.
Agent-Based Modeling in a Nutshell
What Is Agent-Based Modeling?
Agent-based modeling1 is a dynamic, computational approach that represents the actions and interactions of agents and their environment, and simulates how these result in emergent patterns and relationships (Sterman, 2001). In ABMs it is possible to represent agents of different types, their heterogeneity, and the interactions between agents and their environment over time (Box 1). These interactions can be conditional on agents’ own characteristics, the behavior of other agents, and the state of their social–ecological environment. These micro-level interactions result in macro-level (or system-level) outcomes that, in turn, feedback to affect the interactions occurring at the micro-level (Figure 1). This capacity to study micro–macro dynamics and adaptive behavior of agents makes ABM better able to represent complex adaptive system dynamics than other (aggregate) modeling approaches (Levin et al., 2012).
Box 1. The main elements of agent-based models and their characteristics.
Agents
• Diversity of agent types: Agents can reflect different types of entities. “Social” types such as a fisher, trader, household, company, and market, or “ecological” types such as a species or population of species.
• Heterogeneity within agent types: Within types, agents can have different individual characteristics (e.g., gender, social class, skills, or size classes of fish) and/or different preferences (e.g., fishers being more or less risk averse).
• Agent behavior and intelligence: Agents can be reactive by perceiving their environment and responding to change. Agents can also be proactive by taking decisions to reach a goal. Agent behavior can be modeled using behavioral theories such as rational, bounded rational, habitual, or modeled based on empirical data (Smajgl and Barreteau, 2017). The “intelligence” of agents can range from simple decision rules to the use of complex mental models (Lindkvist and Norberg, 2014; Schlüter et al., 2017).
• Agent interactions: Agent decisions, behaviors, and states can be affected by interaction with other agents (e.g., human agents can learn, share knowledge, sell and buy commodities, biological agents can prey, feed, or compete for territory), or with their environment (e.g., making decisions based on their catches).
Environment
• Environment representation: Agents are situated in an environment that can be spatially and/or socially structured (e.g., situated in a land- or seascape, or in a social network).
• Ontological correspondence: The model world and its elements often have clear links to their real-world counterpart, including agent type, behavior, rules and norms, and space.
Dynamics and Interactions
• Time-dynamic: The model moves the simulated world through time by letting the agents act/interact, often reflected in discrete steps (e.g., daily or annual time steps).
• Emergence: Overall (system-level) behaviors and patterns arising from individual agents’ (micro-level) behavior and interactions is called emergence. These patterns can in turn affect the agents (labeled immergence, 2nd order emergence, or downward causation). An agent-based model is by nature multi-level and represents how different scales co-evolve (e.g., how a fishery system changes over time as fishers and fish stocks adapt to each other over time).
Also see, e.g., Conte and Paolucci (2014); Gilbert and Troitzsch (2005), or Railsback and Grimm (2011).
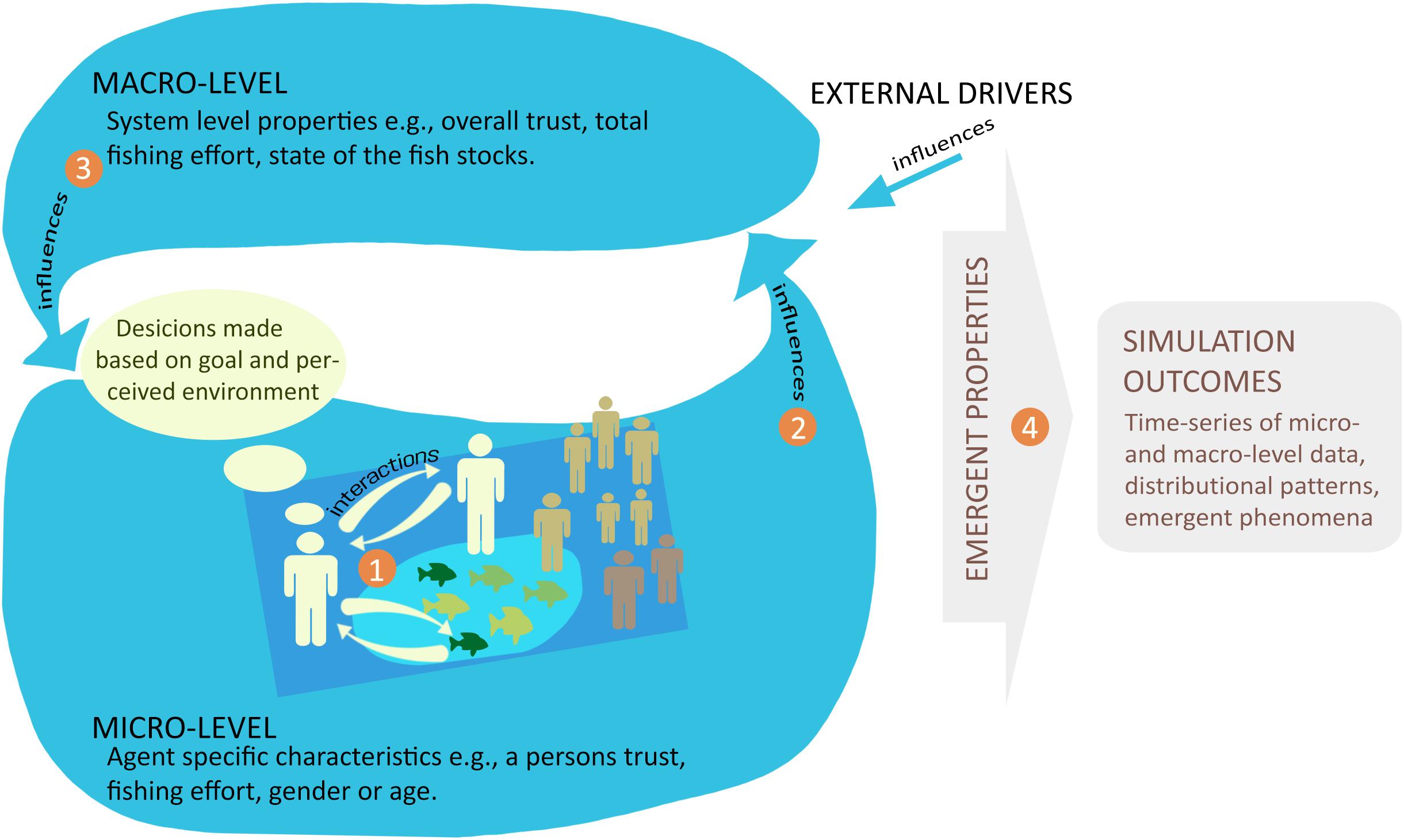
Figure 1. Conceptual description of an ABM. In a co-evolving process, actions at the micro-level change the aggregated variables at the macro-level, which in turn influences actions and interactions at the micro-level. This co-evolutionary process continues until the end of the simulation. (1) Agents perceive their environment, including other agents, and act according to their goal. (2) The micro-level dynamics influences the macro-level, possibly influenced by exogenous drivers. (3) The state of the macro-level variables in turn influences the behavior of the agents at the micro-level. (4) During and after the simulation distributional patterns within micro-level variables may be observed and measured, and emergent properties at the macro-level.
Developing an Agent-Based Model
There are several phases in the development of ABMs: design, implement/build, test, experiment and analyze, and communicate. The exact shape these different development phases take is linked to the purpose and the context of which the model is developed within. The purpose of an ABM can range from basic theoretical understanding of a phenomenon or problem to assessing a case specific policy or management intervention (Edmonds, 2017). Models can be developed as part of individual research projects or multi-stakeholder collaboration processes, which will also influence the purpose and characteristics of the models.
In the design phase the agents and processes relevant to the problem are detailed, and a conceptual model of the ABM is developed. This includes the context in which agents are embedded, assumptions about agent behavior and interactions. The level of complexity of, and focus on, the social and ecological components are also decided upon. The design phase builds on any combination of literature, expert knowledge, and data (e.g., from interviews, surveys, network analysis, laboratory experiments, or participatory processes), and is often a collaborative process between modelers and/or other stakeholders (e.g., researchers, fishery actors, decision makers).
In the implementation phase a programmer translates the conceptual model into code. It often entails even further specification of model details. The type of data and level of detail depend on the focus and purpose of the project. A decision management tool developed for a specific case requires a strong base of case-specific data, whereas an exploration of general phenomena can be based on representative approximations of theoretically derived dynamics. In the testing phase the built model is verified and validated according to the model’s purpose, for instance verifying the model behavior and outputs according to model specifications and validating them according to empirical observations (Balci, 2010; David et al., 2017).
The experiment and analysis phase involves using the model as a virtual laboratory to ask the model questions by developing virtual experiments. Some settings are changed in the model and outcomes are observed. As in the model design phase, the experimentation and analysis is often performed in collaboration with project members and/or other stakeholders. Stakeholders and project members (indirectly or directly) use the model to understand various issues, such as the implications of different assumptions or policies, trade-offs, distributional patterns, and emergent phenomena. The last phase, model communication, should, besides regular science communication, be done through publishing the model in an open source library accompanied standardized model documentation, such as the ODD + D (Overview, Design Concepts, and Details + Decision; Müller et al., 2013) and/or TRACE (TRAnsparent and Comprehensive Ecological modeling documentation; Grimm et al., 2014). For further readings on model development see Gilbert and Troitzsch (2005) or Railsback and Grimm (2011).
How Has Agent-Based Modeling Addressed Small-Scale Fisheries Challenges Thus Far?
Review of Agent-Based Models in Small-Scale Fisheries
We reviewed the literature on the application of ABMs in SSF. A keyword search of Web of Science [(“small-scale fisher∗”) OR (“artisanal fisher∗”) OR (“subsistence fisher∗) OR (“coastal fisher∗”)] AND (Agent-based OR multi-agent OR ABM) resulted in six relevant publications based on the criteria that the models should include both social and ecological components, and be linked to governance, management, or policy issues. Through an extended search for papers on fish trade and references within the first set of papers, six additional publications were found. For further details on the review procedure, see the Supplementary Material.
The published models were used to understand a number of different phenomena, such as increased harvests emerging from cooperative forms of management (Gutierrez et al., 2017); factors facilitating the emergence of self-governance (Wilson et al., 2007); increased harvests in relation to the placement of marine protected areas (Rudd et al., 2003); the emergence of either fishing cooperatives or patron–client relationships as the dominant form of self-governance (Lindkvist et al., 2017); the emergence of balanced harvesting (Plank et al., 2017); long-term effects of tourism and urbanization on coral reef health and fisheries (Perez et al., 2009; Melbourne-Thomas et al., 2011); and causes of overfishing and environmental (reef) degradation (Bousquet et al., 1993, 1994a,b; Worrapimphong et al., 2010; Forrester et al., 2014). In all papers, a single fishery or fishing community was modeled, with a notable exception of Perez et al. (2009) and Melbourne-Thomas et al. (2011) who move beyond a single community to study four geographical areas of economic development that depended on one shared coral reef and several shared fisheries.
We identified three different purposes of the ABMs uncovered in our review: (1) to understand how some SSF phenomena emerge, and to explain the mechanisms (i.e., factors and processes) that were effective (Wilson et al., 2007; Lindkvist et al., 2017; Plank et al., 2017); (2) as policy assessment tools to identify and explain the mechanisms behind why some policy, or way of organizing the fishery, may be better than another (Rudd et al., 2003; Melbourne-Thomas et al., 2011; Gutierrez et al., 2017); and (3) as a participatory tool to co-produce knowledge about a fishery with stakeholders, as pioneered by the Companion Modeling team2 (Bousquet et al., 1993, 1994a,b; Worrapimphong et al., 2010). Perez et al. (2009) and Forrester et al. (2014) also developed their ABM through participatory approaches but did not use role playing games.
The flexibility of ABM creates a diversity of possible ABM applications on how to solve some of the most pressing challenges of studying and managing SSF. Figure 2 draws out some of the key diversities along four axes and situates the reviewed publications. We find that half of the studies aimed to understand a phenomenon or problem and half to evaluating a policy or management plan (Figure 2A). Models more often had an ecological, or social–ecological focus than a purely social focus (Figure 2B). The models tended to build on detailed empirical data rather than on theories (Figure 2C). Finally, models more commonly had a participatory focus than a purely academic focus (Figure 2D). Specific features of the models’ agents, agent behaviors and interactions, linkages to globalized drivers, and how the models deal with data input and output, are further described in the Supplementary Material. Next, we draw on the strengths of ABM and its applicability to SSF challenges.
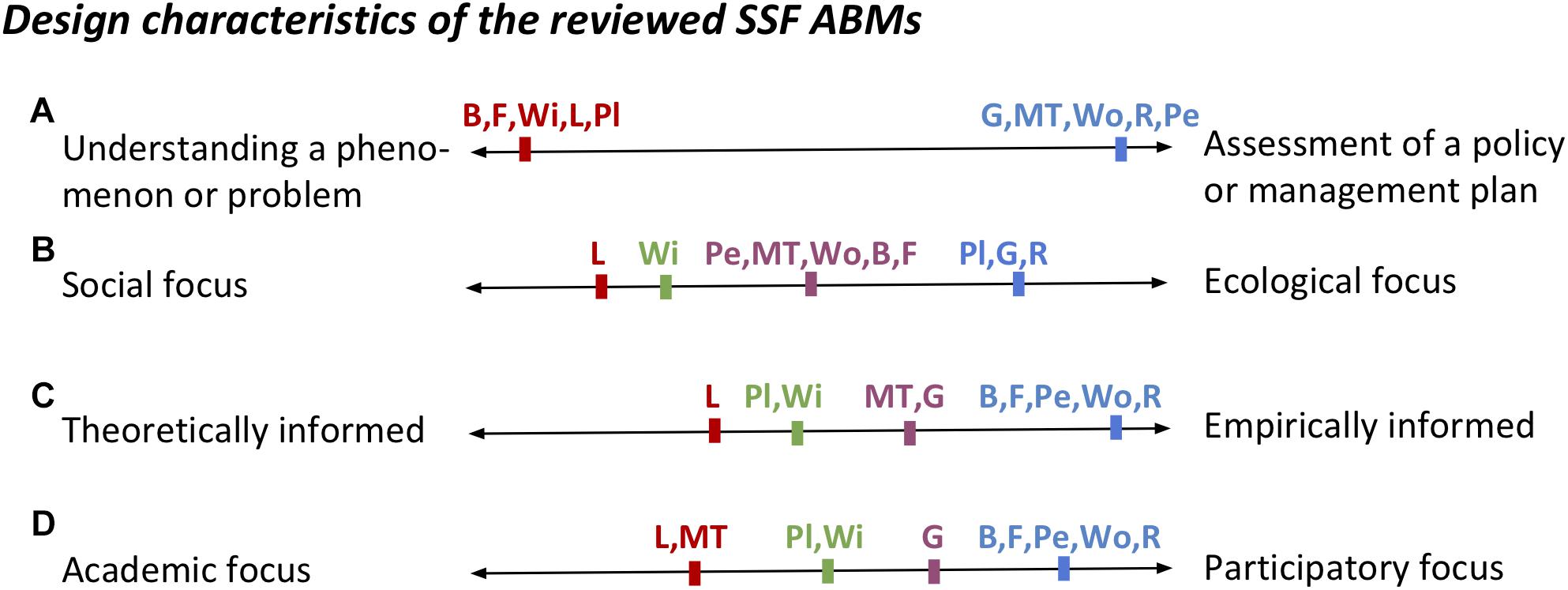
Figure 2. Key differences in ABM purpose and development issues for different SSF models. The colored labels represent the SSF models, which are subjectively placed along four gradients based on the results from the review. The gradients represent: (A) a stronger model purpose of understanding (participatory or not) versus assessment; (B) model design has a more social focus versus ecological focus (midpoint indicates equal social and ecological focus); (C) the model is more strongly based on theories versus data; and (D) the development process of the model involves mostly researchers versus a strong participatory focus. B, Bousquet et al. (1993; 1994a; 1994b); F, Forrester et al. (2014); G, Gutierrez et al. (2017); L, Lindkvist et al. (2017); MT, Melbourne-Thomas et al. (2011); Pl, Plank et al. (2017); Pe, Perez et al. (2009); R, Rudd et al. (2003); Wi, Wilson et al. (2007); and Wo, Worrapimphong et al. (2010).
Agent-Based Modeling for Addressing Small-Scale Fisheries Challenges
Small-scale fisheries management is exposed to many challenges that are rooted in their complexity and data scarcity characteristics, as evidenced by the models from our review. We outline three challenges for SSF management that ABM could help to address: (1) improve the way collective action and heterogeneity in human behavior is incorporated in research and management; (2) develop policies that are sensitive to local contexts while also accounting for regional and global contexts; (3) tackle data paucity and uncertainty. We reflect on how ABM could contribute to solving these three challenges and illustrate this with the SSF models from our review.
Challenge 1: Collective Action and Human Behavior
A key challenge for the sustainable governance and management of SSF revolves around collective action, cooperation, and human behavior (Townsend et al., 2008; Gutierrez et al., 2011; Basurto et al., 2013; Ovando et al., 2013). Locally this can occur through self-governance and co-management initiatives, but at broader scales by linking to other organizations at regional, national, and global levels, as well as by connecting to neighboring fishing communities to gain support for locally managed resources (Marín et al., 2012; Finkbeiner and Basurto, 2015; Oliver et al., 2015). Such initiatives require social cohesion and trust between actors (Ostrom, 1990; Gutierrez et al., 2011; Basurto et al., 2013; Ovando et al., 2013; Kosamu, 2015; Oliver et al., 2015).
Agent-based modeling can represent collective action by: (1) simulating how self-organization can emerge; (2) simulating how trust or loyalty increases or decreases within a group; (3) simulating how fishers harvest a resource based on their self-organization and the levels of trust; (4) accounting for the spatial distribution of resources and fishers movements; and (5) modeling interactions such as information exchange between fishing organizations.
The model by Lindkvist et al. (2017) was developed to investigate under which conditions cooperative and non-cooperative forms of self-governance may establish and persist in a hypothetical fishing community in Northwest Mexico. Their ABM was based on in-depth fieldwork, surveys with fishers, interviews with fish buyers, and a fish buyers’ logbook, to capture key hypotheses of fishers’ day-to-day fishing and trading. Model results indicated that high diversity in fishers’ reliability, and low initial trust between members of the cooperative, makes the establishment of cooperatives difficult. Their results also showed that once cooperatives establish, they cope better with seasonal variability in fish resources and provide long-term security for fishers compared to non-cooperative forms of self-governance.
Wilson et al. (2007) designed an ABM to understand the emergence of self-governance in the lobster gangs of Maine, United States. Results show that in the Maine lobster fishery biological and technological circumstances combined with individual self-interest created conditions favorable for collective action. The model describes the way collective action emerges from the adaptive behavior of competing fishers. The model simulates the dynamic adaptation (learning) of fishers interacting in a complex, changing (social) environment, and provides an example of analyzing micro-level processes that emerged into social–ecological fishery patterns at the macro-level.
Gutierrez et al. (2017) developed a spatially explicit ABM for the sea urchin fishery off San Diego, CA, United States. They assessed the benefits of cooperative harvesting by incorporating spatial and temporal variation in fishery yields. They found that the marketability of sea urchin roe depended on gonad (reproductive organs) yield and quality, which in turn depended on the spatial and temporal conditions of associated kelp beds. However, competition among divers within a non-cooperative system created a “race for shellfish” preventing higher gonad yield per unit of effort. Model results showed that, for the most cooperative scenario where information sharing among divers was greatest and harvest was coordinated, sea urchin catches were at least 10% higher and gonad yield 35% higher than in the non-cooperative scenario. As such, information sharing and organized harvesting typical of well-functioning cooperatives led to the more sustainable use of local resources.
Plank et al. (2017) investigated the consequences of allowing a fixed number of fishers in a SSF to choose the size of the fish they aim to catch. They examine this from a game-theoretic perspective and test their predictions using an agent-based model for fishers’ decisions coupled with a size-spectrum model for the dynamics of a single fish species. They show that small-scale gillnet fishers, operating without size-based regulations, end up catching small and large fish in proportion to their productivity, in other words balanced harvesting. This is significant because it shows that, far from being unachievable, balanced harvesting can emerge without external intervention under some circumstances. Controls are needed to prevent overfishing, but minimum size regulations alone are not sufficient to achieve this. Instead, size regulations can reduce sustainable yields by confining fishing to a relatively unproductive part of the size-spectrum.
The reviewed papers thus have been used to study the emergence of collective action and the establishment and persistence of cooperative groups by Wilson et al. (2007) and Lindkvist et al. (2017), respectively; Gutierrez et al. (2017) demonstrated the benefits of cooperative harvesting strategies while Plank et al. (2017) model self-interested non-cooperative agents that obtain the goal of balanced harvesting. All models from these publications were based on individual fishers and their decision-making. However, none of the models studied the different levels and scales that SSF are a part of, such as the implications of connecting horizontally across communities or connecting vertically up to other organizations at regional, national, or international level.
Challenge 2: Developing Policies That Are Sensitive to Local Contexts While Accounting for Regional and Global Dynamics
Fisheries policies come in different forms, each with different benefits and constraints, and it is unlikely there is a one-size fits-all solution (Degnbol and McCay, 2007; Ostrom, 2007; Berkes, 2012; Chaigneau and Brown, 2016). On the one hand, an effective policy has to account for the local context of a fishing community and for the heterogeneity in fisher characteristics, perceptions, and behavior (Mwaipopo et al., 2010; Bennett and Dearden, 2014; Gelcich and O’Keeffe, 2016; Singh et al., 2018; Wosu, 2019). At the same time, SSF are embedded within, and interact with, larger scales such as regional and national institutions, and global markets (Crona et al., 2015; Pace and Gephart, 2017; Bennett and Basurto, 2018). An effective policy should address a specific governance problem while considering the complexity and interactions within the broader fishery system. For instance, to obtain sustainable social–ecological outcomes across geographical scales for one fishery, a policy needs to account for any interdependencies with other fisheries, both in terms of potential food web interactions, and in terms of displacements of fishing effort when fishers enter or exit a fishery area (Gaines et al., 2010; Berkes, 2012).
Agent-based models can be used to represent and thus study the role of context and scales over time because they can reflect heterogeneous agents; history, culture, and individual differences between fishers (such as fishers’ perceptions and typical behaviors). ABMs are, however, also able to represent interactions beyond a local SSF with other distal interactions such as global market dynamics or climate change. This may be helpful in the process of integrating knowledge from different stakeholders, experts, and researchers (Weber et al., 2019).
Several ABMs we reviewed were aimed at assisting in the design process of policy development and a governance plan while accounting for the cross-scale and embedded nature of the socio-ecological system (Bousquet et al., 1993, 1994a,b; Perez et al., 2009; Worrapimphong et al., 2010; Melbourne-Thomas et al., 2011; Forrester et al., 2014). The model developed by Perez et al. (2009) is an exemplary ABM that combines knowledge from different stakeholders and researchers. The focus of their study was on the interactions between tourism, urbanization, fishing, and coral reef health in four fishing communities that shared the same reefs and fisheries in the Quintana Roo region, Mexico. Model results revealed an interesting causality of increasing tourism and urbanization leading to degraded reefs, concomitantly causing a decrease in tourism, which in turn forced tourism workers to turn to (illegal) fishing, causing a sequential collapse of three fisheries. The model was able to re-produce time series and projected future scenarios. However, the authors claimed that the strength of the model lay in its capacity to integrate social, economic, and ecological components into a coherent framework that can inform multi-level governance issues and public policy. Melbourne-Thomas et al. (2011) extended Perez et al.’s (2009) model by coupling it with a biophysical model of the Quintana Roo region. A preliminary evaluation of the coupled model system gave reasonable predictions for fisheries and ecological variables and indicated that the model could be used to examine scenarios for future social–ecological change in Quintana Roo.
The study in the upper gulf of Thailand by Worrapimphong et al. (2010) addressed the relationships between increasing tourism in the coastal wetlands and the increasing pressure on locally important razor clam populations. The purpose of this modeling study was to develop a management plan and to engage the different stakeholders in collective discussions. This was achieved through combining an ABM with role-playing games. The games promoted alternative clam management plans, such as establishment and rotation of zones closed for harvesting, and quota systems. These plans were more deeply investigated with the ABM to simulate different scenarios (reserve, quota, and combinations of reserve and quota), which enabled better-informed discussions between different stakeholders. As a result of the work, stakeholders were able to present a sustainable management plan to regional policy-makers.
Forrester et al. (2014) depart from the assumption that complex social–ecological systems are not amenable to simple mathematical modeling and posit that solving sustainability challenges calls for an integrated approach. Based on empirical knowledge of local stakeholders and experts, they mapped conceptions of beach management units in Kenya. Two models were developed: one local-level ABM; and one overarching multiple-entity conceptual model of the system which provided a general landscape within which the structurally realistic ABM could exist and be understood. The two representations together contributed to understanding the links between the local system and other levels of decision-making. The authors stated that this model combination helped develop easily accessible tools for stakeholders, by representing the system both in a bottom-up the (the ABM) and top-down (the conceptual model) manner and by capturing and conceptualizing ecosystem dynamics and processes, and the broader system it is embedded within.
These models represent the interdisciplinary, multi-sector, and multi-scale approach called for by many SSF researchers (McClanahan et al., 2009; Kittinger et al., 2013; Bennett and Dearden, 2014; Crona et al., 2015; Purcell and Pomeroy, 2015; Chaigneau and Brown, 2016; Gelcich and O’Keeffe, 2016; Basurto et al., 2017). Whether these modeling projects have been successful in improving governance and management in SSFs is yet to be determined.
Challenge 3: Dealing With Data Paucity and Uncertainty
In most SSFs data on catches, stock status and environmental, economic, and social attributes are often unavailable (Kalikoski and Franz, 2014; Jentoft and Chuenpagdee, 2015; Johnson et al., 2017; Giron-Nava et al., 2018; Agapito et al., 2019). Furthermore, the data that are available are often misreported or patchy (The World Bank, 2012; Cisneros-Montemayor et al., 2013). This generates a gap in the possibilities to understand how specific policies and changes in fisher behavior might affect a fishery from an ecological, social, or economic perspective (Fulton et al., 2011). In light of this information paucity it is difficult to know what empirical data are most useful to inform management (FAO, 2017; Jentoft, 2017). This challenge becomes even larger given the need to tailor policies to the local context and to account for external drivers. As such, information on other system variables such as how fishers respond to particular policies or incentives, how fishers are organized, levels of trust in a fishery and market dynamics may be more important for the design and implementation of policies (Basurto et al., 2013; Crona et al., 2015; Finkbeiner et al., 2017; Battista et al., 2018).
The challenge of data paucity in design and analysis concerns every modeling approach including ABM. Data enable models to be grounded in reality, but post hoc data also provide a means for testing the link between model results and the real world. A lack or absence of data can in some cases prevent model development in the first place. However, even in data-poor situations ABM can make use of qualitative data from experts or anthropological studies (Ghorbani et al., 2015), thus contributing toward understanding complex issues by testing and comparing different theories, explanations, and important variables in a “virtual” model-based laboratory. ABM can also inform priorities for future data collection (Worrapimphong et al., 2010; Cooper and Jarre, 2017; Lindkvist et al., 2017; Burgess et al., 2018). For example, testing how sustainable resource use, profits, and satisfaction are affected by different assumptions for fishers’ behavior can give insight into priorities for data collection (Burgess et al., 2018). In the case of the aforementioned razor clam fishery in the gulf of Thailand (Worrapimphong et al., 2010) there was a lack of data for the population parameters (natural mortality rate, carrying capacity, and number of recruits per female), which led to uncertainties in the outcomes of different management options. This knowledge gap indicated which parameters needed to be further studied, but also that some management options, such as quota schemes, could better account for this uncertainty and still result in sustainable harvests. Similarly, in the absence of location choice data, simulating fisher movements as a response to different drivers such as price, increased catches, or crowding could help to understand the dynamics of fisheries exploitation (e.g., Carpenter and Brock, 2004; Soulié and Thébaud, 2006; Barbier and Watson, 2016).
The complex adaptive systems’ nature of SSFs means that incorporating additional data cannot always reduce uncertainty. While more data will certainly enhance understanding, key structural uncertainties about processes and interactions in SSF and fisher responses to different policies are likely to remain. Policy-making thus needs to manage this uncertainty through the design of adaptive and reflexive processes (Dunn et al., 2016). ABM can help assess the possible range of expected outcomes, given uncertainty about human behavior (Fulton et al., 2011), and to test how robust management strategies are based on key uncertainties (van Putten et al., 2012).
Opportunities and Limitations
Agent-based modeling is a tool that can deal with the complexity of SSFs by assessing consequences of interactions and assumptions on how these interactions occur. Ironically this also creates complex challenges for the model development process by requiring decisions on what parameters and processes to include in the model and which to leave out. In the analysis phase, questions may arise around what exact variables are driving the results, and more general questions such as: “will the model output change if we increase the number of agents?,” or “if we simulate a longer time period will results change?.” Consequently, time, resources, and active collaboration between those involved in designing, developing, and interpreting an ABM are key to successful outcomes of the modeling project.
The complexity, and the extent to which SSF are embedded into a larger-scale contexts, mean that a thorough investigation of policy solutions to a place-based problem will not be simple. The cultural, socio-economic, and ecological characteristics of a local SSF fishery or fishing community, and their wider context must be considered. While an agent-based model can be developed to incorporate these dimensions, the type of nuanced understanding that an ABM can provide may not be the policy-maker’s priority because they may need concrete results with minimal uncertainty, and solutions that fit within the current policy schemes (Taylor et al., 2016; Allison et al., 2018). In these situations, models can instead be used to test interdependencies, investigate trade-offs among policies, while simultaneously including important heterogeneity among fisheries actors.
Like any modeling approach, ABM suffers from misconceptions and critique, such as being costly or opaque. However, in most cases there are two sides of the argument. ABM can be costly in terms of time and expertise, the cost may be higher if the purpose is predict outcomes of a policy intervention in a case-specific situation, but can be lower if the purpose is to understand a general phenomenon. Cost can also be higher if prior models do not exist, or if models need to be built from scratch for different scales or situations. This cost could be reduced if based on existing models, however, as our review showed, few models exist thus it may be difficult to find a model to build from. Although The Network for Computational Modeling in Social and Ecological Sciences3 provides a library of ABMs of social–ecological systems that are open source and reusable, few fisheries models have been published there. At the code hosting platform GitHub4 the agent-based model POSEIDON is freely available for fisheries research (Bailey et al., 2019), other examples include the DISPLACE model for spatial fishing planning and effort displacement (Bastardie et al., 2013), and more case-specific models (e.g., Yu et al., 2009; Cenek and Franklin, 2017). However, using such extant models still requires coding and there is no standard software or module library for fisheries ABMs to date. In the future, the availability of comprehensive libraries of (re)useable models could help to make ABM available to a broader user community in SSF.
Rigorous conventions for documenting ABMs (e.g., ODD by Grimm et al., 2010; and ODD + D by Müller et al., 2013) have been adopted by large parts of the ABM community which helps increase the accessibility and transparency of ABMs thus facilitating sharing and adaptation of parts of existing models. Ultimately, further experimentation with ABM in SSF, if supported by rigorous documentation and open-source sharing according to standardized protocols, may coalesce into an accessible resource that would facilitate adaptation and adoption of existing code, or even a common ABM framework for addressing typical, regularly encountered SSF questions. Inspiration could be taken from other modeling frameworks such as Ecopath with Ecosim (Villasante et al., 2016), where a common software, model, data sharing framework, and active community of practice and training networks have facilitated applications in diverse fisheries contexts around the world. ABM could also be integrated with such fisheries modeling approaches to move toward integrating more social dynamics and agent-based features into ecologically based models, which could also assist in reducing the cost and broadening the use of ABMs.
In social–ecological systems research ABMs are becoming more common and are generally regarded as a useful scientific tool, but they still suffer from critique for inconsistent documentation and testing, as well as lack of transparency in communicating results (Schulze et al., 2017). Skepticism toward ABM may partly be driven by academic expertise and to a certain extent territorialism, but there is rightful criticism that ABM requires better documentation, testing, and communication to support understanding (Waldherr and Wijermans, 2013). ABMs will only be useful for improving SSF governance and management if they are developed following good modeling practice. This entails a better understanding among fisheries scientists and managers about why, when, and how to use ABM in SSF (see Box 2 for a summary).
Box 2. Summary points.
ABMs can improve research, management, and governance of SSF in a globalized context while taking account of the complex nature of individual entities and data paucity because:
• One can model interactions, processes, and mechanisms at the micro-level to understand and explain emergent outcomes at the macro-level.
• They do not always rely on quantitative data. Depending on the purpose of the model it may be desirable to substantiate model design and validate model outcomes with expert knowledge and qualitative assessments and/or theories.
• It is possible to integrate different types of data, knowledge, and theories in a realistic setting which makes it easier to integrate knowledge from stakeholders, experts, and scientists across sectors and disciplines.
• The development phase can reveal which agents and interactions are important to understand the dynamics of a system. They can also point to which variables and processes require further investigation, especially with regards to individual decision-making, and give rise to new questions that can be explored empirically or through modeling.
• They allow the exploration of how changes can affect actors or the environment. For example, changes in micro-level distributional patterns such as income or catch, or in macro-level variables such as the biomass of fish, or biophysical conditions.
What is ABM less useful for?
• Problems that are simple enough that an ABM becomes redundant.
• Phenomena where the agents, their decisions, and situations do not differ, i.e., heterogeneity is not key in emergent behavior, in which case computationally cheaper models should be considered.
What are points of caution?
• Depending on the purpose of the study, the model development process may be too time consuming and costly.
• Balancing the complexity of building the model with the interpretability of the outcomes is difficult.
• Extensive documentation of agents, interactions and mechanisms through relevant protocols need to be utilized in order to avoid making the model opaque to others.
NB. Some of the summary points raised here may also apply to other modeling approaches.
Frontiers for Agent-Based Modeling in Small-Scale Fisheries
Models are models and can never replace empirical studies, because the questions that models can answer are often different from those that empirical studies address (Levin et al., 2012; Schlüter et al., 2012). However, often multi-method approaches that combine modeling and empirical work can reveal insights and understanding that cannot be gained by a single method. With this complementarity in mind, we elaborate on the empirically defined research questions and areas of research where the features of ABMs could be pertinent in the context of SSF.
All standard fisheries models recognize the importance of aggregate fishing effort, but ABMs offer the potential to understand the micro-level processes that generate this effort. An understanding of total fishing effort as an emergent outcome from individual fishers’ characteristics and decisions offers more realistic policy levers to influence effort; not by unenforceable attempts to command and control fishing effort, but by influencing the context and outcome of individual interactions that ultimately determine the total effort. An ABM focuses on decision-making processes of fisheries actors and their embeddedness in social and ecological contexts. Therefore, new empirical questions related to the behavior of fishers can emerge in the modeling development phase (Cooper and Jarre, 2017; Lindkvist et al., 2017; Schlüter et al., 2019). In a similar way, ABM is also well suited to explore how individuals may react differently to new interventions (Epstein, 2008). An illustrative example of how this can be done is suggested by Battista et al. (2018), who present a step-wise approach of how new interventions at the individual level can be introduced in a fishery, but do not recognize the utility of ABM in their approach. In this case, ABM offers an additional step of virtual experimentation, before the proposed step of experimentally testing interventions in laboratory or field experiments. ABM thus can help assess, understand, and explain SSF outcomes in a way that accounts for heterogeneity in behavior, the characteristics of fisheries actors, and the diversity of the ecosystem, to do justice to the complex nature of SSF. This is something that no other modeling approach can do.
Small-scale fisheries are inherently multi-level with important interactions across geographic or organizational scales such as trade or climate change impacts. ABMs are a suitable method for the study of cross-scale interactions, which are difficult to represent using simpler mathematical models. However, none of the models we reviewed addressed interactions beyond the local or regional level such as global market dynamics. The potential for ABM to explore interactions with regional or global processes thus remains an important frontier for future exploration. In fact, none of the ABMs include trade dynamics at any scale yet it is one of the most challenging issues faced by SSF (Béné et al., 2010; Crona et al., 2015; Pace and Gephart, 2017). Classical ABMs on trade by Epstein and Axtell (1996), Kirman and Vriend (2001), and Tesfatsion (1997) provide insights into heterogeneous trading actors and factors that mediate trade relationships such as loyalty, that influence trade patterns and price dynamics. A possible frontier is to couple or reuse parts of these trade models in fisheries contexts to help understand how trade at one or multiple scales affects SSF.
Another topical domain where ABM has a large potential is the mobility and migration of fishers, which is a well recognized adaptation strategy in SSF around the world (FAO, 2017). Movements of fishers can be connected to international markets through teleconnections, which is a known driver of sequential overexploitation in some fisheries (Berkes et al., 2006; Eriksson et al., 2015). However, the conditions that trigger or constrain fishers’ movements has to date been neglected (Swartz et al., 2010; Anderson et al., 2011). ABMs are a viable choice to analyze exploitation patterns and the diverse mechanisms driving these from the bottom-up, even in situations in which there is a lack of quantitative data on the movement patterns of fishers. Instead, expert knowledge or data about what drives fishers to move can be modeled to understand the emergent movement patterns of individual fishers. Here, the ontological realism of ABMs is an advantage because it is easier to relate characteristics and behaviors of agents to real world experiences than determining a mathematical functional form of a variable relationship. Carpenter and Brock (2004) have studied fisher mobility between lakes, while Soulié and Thébaud (2006) modeled mobility in a theoretical, highly regulated fishery; however, our review has not identified any studies on mobility or migration in coastal SSF.
Finally, the challenge of dealing with data scarcity and uncertainty opens up a novel pathway for using ABMs in SSF. As Cooper and Jarre (2017) highlight, ABMs may provide novel grounds for identifying and understanding what social and economic data are useful to quantitatively collect over time or to further investigate qualitatively.
We conclude that the ability of ABM’s to address SSF as complex adaptive systems, taking account of diversity and heterogeneity of actors and interactions within and across scales, can improve our understanding of SSF dynamics, and inform policy and management actions. ABMs can serve as boundary objects and virtual laboratories to integrate different knowledge sources or problem perceptions and test assumptions and possible explanations of phenomena in a collaborative or participatory process, with the aim of exploring their consequences for system outcomes or unraveling complex underlying causes. The need to better understand complex causation and causal mechanisms in the governance of social–ecological systems has recently been highlighted (Biesbroek et al., 2017; Ferraro et al., 2019; Schlüter et al., 2019). While policy assessment in virtual ABM laboratories can help assess the uncertainty associated with a policy or management intervention, using ABM to scrutinize possible explanations of SSF phenomena can help build understanding and theory for enhanced governance (Schlüter et al., 2019). In summary, ABM is a promising approach to develop complexity-based analyses and understand the governance of the dynamics of SSF, particularly when applied in larger processes involving multiple stakeholders. While such processes can be time intensive, they can do justice to the complex and dynamic nature of SSF, which, if ignored can lead to unintended policy outcomes.
Author Contributions
AJ, EL, and BG-M provided the original idea. EL, AJ, BG-M, XB, TD, NW, MS, AG-N, and IP designed the manuscript outline and content, and reviewed the manuscript. EL performed the review and wrote the original manuscript. EL, NW, TD, and BG-M prepared the conceptual design of the visualizations. EL, NW, BG-M, IP, TD, MS, and AG-N edited parts of the manuscript.
Funding
EL, AJ, MS, and XB received funding from the National Science Foundation Grant DEB-1632648. EL received funding from the Swedish Research Council (VR, Forte, Formas) and Sida (The Swedish International Development Cooperation Agency) Dnr 2018-05862. XB received funding from the David and Lucile Packard Foundation Grant 2014-39664 and the Walton Family Foundation Grant 2013-1295. AG-N received funding from the CONTACYT CVU 579944 and Future Earth. BG-M and MS received funding from the European Research Council (ERC) under the European Union’s Horizon 2020 Research and Innovation Programme (Grant Agreement No. 682472 — MUSES). NW and TD were supported by funding from the Sida through the GRAID Program at the Stockholm Resilience Centre, Stockholm University. We are grateful for Stockholm University for funding the publication fees of this manuscript. EL, MS, BG-M, TD, and NW gratefully acknowledge the financial support by a core grant to the Stockholm Resilience Centre by Mistra and Sida.
Conflict of Interest
AJ was employed by company MarFishEco.
The remaining authors declare that the research was conducted in the absence of any commercial or financial relationships that could be construed as a potential conflict of interest.
Acknowledgments
We would like to acknowledge the inspiring, thoughtful, and constructive comments of the two reviewers, which significantly improved the paper.
Supplementary Material
The Supplementary Material for this article can be found online at: https://www.frontiersin.org/articles/10.3389/fmars.2019.00733/full#supplementary-material
Footnotes
- ^ Also sometimes referred to as individual-based models (IBMs). Although they are methodologically the same as ABMs, their label is predominantly used in ecology where agents are often ecological entities, e.g., deer, fish, or trees (Grimm, 1999).
- ^ https://www.commod.org
- ^ https://www.comses.net/
- ^ https://github.com/
References
Agapito, M., Chuenpagdee, R., Devillers, R., Gee, J., Johnson, A. F., Pierce, G. J., et al. (2019). “Beyond the basics: improving information about small-scale fisheries,” in Transdisciplinarity for Small-Scale Fisheries Governance: Analysis and Practice, eds R. Chuenpagdee, and S. Jentoft, (Cham: Springer International Publishing), 377–395. doi: 10.1007/978-3-319-94938-3_20
Allison, A. E., Dickson, M. E., Fisher, K. T., and Thrush, S. F. (2018). Dilemmas of modelling and decision-making in environmental research. Environ. Model. Softw. 99, 147–155. doi: 10.1016/j.envsoft.2017.09.015
An, L., Linderman, M., Qi, J., Shortridge, A., and Liu, J. (2005). Exploring complexity in a human–environment system: an agent-based spatial model for multidisciplinary and multiscale integration. Ann. Assoc. Am. Geogr. 95, 54–79. doi: 10.1111/j.1467-8306.2005.00450.x
Anderson, S. C., Flemming, J. M., Watson, R., and Lotze, H. K. (2011). Serial exploitation of global sea cucumber fisheries. Fish Fish. 12, 317–339. doi: 10.1111/j.1467-2979.2010.00397.x
Bailey, R. M., Carrella, E., Axtell, R., Burgess, M. G., Cabral, R. B., Drexler, M., et al. (2019). A computational approach to managing coupled human–environmental systems: the POSEIDON model of ocean fisheries. Sustain. Sci. 14, 259–275. doi: 10.1007/s11625-018-0579-9
Balci, O. (2010). Golden rules of verification, validation, testing, and certification of modeling and simulation applications. SCS MS Mag. 4, 1–7.
Barbier, M., and Watson, J. R. (2016). The spatial dynamics of predators and the benefits and costs of sharing information. PLoS Comput. Biol. 12:e1005147. doi: 10.1371/journal.pcbi.1005147
Bastardie, F., Nielsen, J. R., and Miethe, T. (2013). DISPLACE: a dynamic, individual-based model for spatial fishing planning and effort displacement—integrating underlying fish population models. Can. J. Fish. Aquat. Sci. 71, 366–386. doi: 10.1139/cjfas-2013-0126
Basurto, X., Bennett, A., Weaver, A. H., Rodriguez-Van Dyck, S., and Aceves-Bueno, J.-S. (2013). Cooperative and noncooperative strategies for small-scale fisheries’ self-governance in the globalization era: implications for conservation. Ecol. Soc. 18:38. doi: 10.5751/ES-05673-180438
Basurto, X., Virdin, J., Smith, H., and Juskus, R. (2017). Strengthening Governance of Small-Scale Fisheries: an Initial Assessment of Theory and Practice. Geneva: Oak Foundation.
Battista, W., Romero-Canyas, R., Smith, S. L., Fraire, J., Effron, M., Larson-Konar, D., et al. (2018). Behavior change interventions to reduce illegal fishing. Front. Mar. Sci. 5:403. doi: 10.3389/fmars.2018.00403
Béné, C., Lawton, R., and Allison, E. H. (2010). “Trade matters in the fight against poverty”: narratives, perceptions, and (lack of) evidence in the case of fish trade in Africa. World Dev. 38, 933–954. doi: 10.1016/j.worlddev.2009.12.010
Bennett, A., and Basurto, X. (2018). Local institutional responses to global market pressures: the sea cucumber trade in Yucatán, Mexico. World Dev. 102, 57–70. doi: 10.1016/j.worlddev.2017.09.006
Bennett, N. J., and Dearden, P. (2014). Why local people do not support conservation: community perceptions of marine protected area livelihood impacts, governance and management in Thailand. Mar. Policy 44, 107–116. doi: 10.1016/j.marpol.2013.08.017
Berkes, F. (2012). Implementing ecosystem-based management: evolution or revolution? Fish Fish. 13, 465–476. doi: 10.1111/j.1467-2979.2011.00452.x
Berkes, F., Hughes, T. P., Steneck, R. S., Wilson, J. A., Bellwood, D. R., Crona, B., et al. (2006). Globalization, roving bandits, and marine resources. Science 311, 1557–1558. doi: 10.1126/science.1122804
Biesbroek, R., Dupuis, J., and Wellstead, A. (2017). Explaining through causal mechanisms: resilience and governance of social–ecological systems. Curr. Opin. Environ. Sustain. 28, 64–70. doi: 10.1016/j.cosust.2017.08.007
Bousquet, F., Cambier, C., and Morand, P. (1994a). Distributed artificial intelligence and object-oriented modelling of a fishery. Math. Comput. Model. 20, 97–107. doi: 10.1016/0895-7177(94)90234-8
Bousquet, F., Cambier, C., Mullon, C., Morand, P., and Quensiere, J. (1994b). “Simulating fishermen’s society,” in Simulating Societices: the Computer Simulation of Social Phenomena, eds N. Gilbert, and J. Doran, (London: UCL Press), 143–162.
Bousquet, F., Cambier, C., Mullon, C., Morand, P., Quensière, J., and Pavé, A. (1993). Simulating the interaction between a society and a renewable resource. J. Biol. Syst. 1, 199–214. doi: 10.1142/s0218339093000148
Bousquet, F., and Le Page, C. (2004). Multi-agent simulations and ecosystem management: a review. Ecol. Model. 176, 313–332. doi: 10.1016/j.ecolmodel.2004.01.011
Burgess, M. G., Carrella, E., Drexler, M., Axtell, R. L., Bailey, R. M., Watson, J. R., et al. (2018). Opportunities for agent-based modeling in fisheries social science. SocArXiv [Preprint] doi: 10.31235/osf.io/gzhm5
Carpenter, S. R., and Brock, W. A. (2004). Spatial complexity, resilience, and policy diversity: fishing on lake-rich landscapes. Ecol. Soc. 9:8.
Cenek, M., and Franklin, M. (2017). An adaptable agent-based model for guiding multi-species Pacific salmon fisheries management within a SES framework. Ecol. Model. 360, 132–149. doi: 10.1016/j.ecolmodel.2017.06.024
Chaigneau, T., and Brown, K. (2016). Challenging the win-win discourse on conservation and development: analyzing support for marine protected areas. Ecol. Soc. 21:36. doi: 10.5751/ES-08204-210136
Cinner, J. E., Huchery, C., MacNeil, M. A., Graham, N. A., McClanahan, T. R., Maina, J., et al. (2016). Bright spots among the world’s coral reefs. Nature 535:416.
Cisneros-Montemayor, A. M., Cisneros-Mata, M. A., Harper, S., and Pauly, D. (2013). Extent and implications of IUU catch in Mexico’s marine fisheries. Mar. Policy 39, 283–288. doi: 10.1016/j.marpol.2012.12.003
Conte, R., and Paolucci, M. (2014). On agent-based modeling and computational social science. Front. Psychol. 5:668. doi: 10.3389/fpsyg.2014.00668
Cooper, R., and Jarre, A. (2017). An agent-based model of the south african offshore hake trawl industry: part I model description and validation. Ecol. Econ. 142, 268–281. doi: 10.1016/j.ecolecon.2017.06.026
Crona, B. I., Van Holt, T., Petersson, M., Daw, T. M., and Buchary, E. (2015). Using social–ecological syndromes to understand impacts of international seafood trade on small-scale fisheries. Glob. Environ. Change 35, 162–175. doi: 10.1016/j.gloenvcha.2015.07.006
David, N., Fachada, N., and Rosa, A. C. (2017). “Verifying and Validating Simulations,” in Simulating Social Complexity: A Handbook, eds B. Edmonds, and R. Meyer, (Cham: Springer International Publishing), 173–204. doi: 10.1007/978-3-319-66948-9_9
Degnbol, P., and McCay, B. J. (2007). Unintended and perverse consequences of ignoring linkages in fisheries systems. ICES J. Mar. Sci. 64, 793–797. doi: 10.1093/icesjms/fsm040
Dunn, D. C., Maxwell, S. M., Boustany, A. M., and Halpin, P. N. (2016). Dynamic ocean management increases the efficiency and efficacy of fisheries management. Proc. Natl. Acad. Sci. U.S.A. 113, 668–673. doi: 10.1073/pnas.1513626113
Edmonds, B. (2017). “Different modelling purposes,” in Simulating Social Complexity: A Handbook, eds B. Edmonds, and R. Meyer, (Cham: Springer International Publishing), 39–58.
Epstein, J. M., and Axtell, R. (1996). Growing Artificial Societies: Social Science From the Bottom Up. Washington, DC: Brookings Institution Press.
Eriksson, H., Österblom, H., Crona, B., Troell, M., Andrew, N., Wilen, J., et al. (2015). Contagious exploitation of marine resources. Front. Ecol. Environ. 13, 435–440. doi: 10.1890/140312
Étienne, M. (ed.) (2013). Companion Modelling: A Participatory Approach to Support Sustainable Development. Dordrecht: Springer Science & Business Media.
FAO (2014). The State of the World Fisheries and Aquaculture 2014: Opportunities and Challenges. Rome: Food and Agriculture Organization of the United Nations.
FAO (2017). Voluntary Guidelines for Securing Sustainable Small-Scale Fisheries in the Context of Food Security and Poverty Eradication. Rome: Food & Agriculture Organization of the United Nations.
Ferraro, P. J., Sanchirico, J. N., and Smith, M. D. (2019). Causal inference in coupled human and natural systems. Proc. Natl. Acad. Sci. U.S.A. 116, 5311–5318. doi: 10.1073/pnas.1805563115
Finkbeiner, E. M., and Basurto, X. (2015). Re-defining co-management to facilitate small-scale fisheries reform: an illustration from northwest Mexico. Mar. Policy 51, 433–441. doi: 10.1016/j.marpol.2014.10.010
Finkbeiner, E. M., Bennett, N. J., Frawley, T. H., Mason, J. G., Briscoe, D. K., Brooks, C. M., et al. (2017). Reconstructing overfishing: moving beyond malthus for effective and equitable solutions. Fish Fish. 18, 1180–1191. doi: 10.1111/faf.12245
Forrester, J., Greaves, R., Noble, H., and Taylor, R. (2014). Modeling social-ecological problems in coastal ecosystems: a case study. Complexity 19, 73–82. doi: 10.1002/cplx.21524
Fulton, E. A., Smith, A. D., Smith, D. C., and van Putten, I. E. (2011). Human behaviour: the key source of uncertainty in fisheries management. Fish Fish. 12, 2–17. doi: 10.1111/j.1467-2979.2010.00371.x
Gaines, S. D., White, C., Carr, M. H., and Palumbi, S. R. (2010). Designing marine reserve networks for both conservation and fisheries management. Proc. Natl. Acad. Sci. U.S.A. 107, 18286–18293. doi: 10.1073/pnas.0906473107
Gelcich, S., and O’Keeffe, J. (2016). Emerging frontiers in perceptions research for aquatic conservation. Aquat. Conserv. Mar. Freshw. Ecosyst. 26, 986–994. doi: 10.1002/aqc.2714
Ghorbani, A., Dijkema, G., and Schrauwen, N. (2015). Structuring qualitative data for agent-based modelling. J. Artif. Soc. Soc. Simul. 18:2. doi: 10.18564/jasss.2573
Gilbert, N., and Troitzsch, K. (2005). Simulation for the Social Scientist. Maidenhead: McGraw-Hill Education.
Giron-Nava, A., Johnson, A. F., Cisneros-Montemayor, A. M., and Aburto-Oropeza, O. (2018). Managing at maximum sustainable yield does not ensure economic well-being for artisanal fishers. Fish Fish. 20, 214–223. doi: 10.1111/faf.12332
Grimm, V. (1999). Ten years of individual-based modelling in ecology: what have we learned and what could we learn in the future? Ecol. Model. 115, 129–148. doi: 10.1016/s0304-3800(98)00188-4
Grimm, V., Augusiak, J., Focks, A., Frank, B. M., Gabsi, F., Johnston, A. S., et al. (2014). Towards better modelling and decision support: documenting model development, testing, and analysis using TRACE. Ecol. Model. 280, 129–139. doi: 10.1016/j.ecolmodel.2014.01.018
Grimm, V., Berger, U., DeAngelis, D. L., Polhill, J. G., Giske, J., and Railsback, S. F. (2010). The ODD protocol: a review and first update. Ecol. Model. 221, 2760–2768. doi: 10.1016/j.ecolmodel.2010.08.019
Gutierrez, N. L., Halmay, P., Hilborn, R., Punt, A. E., and Schroeter, S. (2017). Exploring benefits of spatial cooperative harvesting in a sea urchin fishery: an agent-based approach. Ecosphere 8:e01829. doi: 10.1002/ecs2.1829
Gutierrez, N. L., Hilborn, R., and Defeo, O. (2011). Leadership, social capital and incentives promote successful fisheries. Nature 470, 386–389. doi: 10.1038/nature09689
Heckbert, S., Baynes, T., and Reeson, A. (2010). Agent-based modeling in ecological economics. Ann. N. Y. Acad. Sci. 1185, 39–53. doi: 10.1111/j.1749-6632.2009.05286.x
Jentoft, S. (2017). Small-scale fisheries within maritime spatial planning: knowledge integration and power. J. Environ. Policy Plan. 19, 266–278. doi: 10.1080/1523908x.2017.1304210
Jentoft, S., and Chuenpagdee, R. (eds) (2015). Interactive Governance for Small-Scale Fisheries: Global Reflections. Cham: Springer International Publishing.
Johnson, A. F., Moreno-Báez, M., Giron-Nava, A., Corominas, J., Erisman, B., Ezcurra, E., et al. (2017). A spatial method to calculate small-scale fisheries effort in data poor scenarios. PLoS One 12:e0174064. doi: 10.1371/journal.pone.0174064
Kalikoski, D., and Franz, N. (eds) (2014). “Strengthening organizations and collective action in fisheries,” in A way Forward in Implementing the International Guidelines for Securing Sustainable Small-Scale Fisheries Fisheries Aquaculture Proceedings, (Rome: Food and Agriculture Organization of the United Nations):168.
Kirman, A. P., and Vriend, N. J. (2001). Evolving market structure: an ACE model of price dispersion and loyalty. J. Econ. Dyn. Control 25, 459–502. doi: 10.1016/s0165-1889(00)00033-6
Kittinger, J. N., Finkbeiner, E. M., Ban, N. C., Broad, K., Carr, M. H., Cinner, J. E., et al. (2013). Emerging frontiers in social-ecological systems research for sustainability of small-scale fisheries. Curr. Opin. Environ. Sustain. 5, 352–357. doi: 10.1016/j.cosust.2013.06.008
Kosamu, I. B. (2015). Conditions for sustainability of small-scale fisheries in developing countries. Fish. Res. 161, 365–373. doi: 10.1016/j.fishres.2014.09.002
Levin, S., Xepapadeas, T., Crépin, A.-S., Norberg, J., de Zeeuw, A., Folke, C., et al. (2012). Social-ecological systems as complex adaptive systems: modeling and policy implications. Environ. Dev. Econ. 18, 1–22. doi: 10.1017/S1355770X12000460
Lewison, R. L., Johnson, A. F., Gan, J., Pelc, R., Westfall, K., and Helvey, M. (2019). Accounting for unintended consequences of resource policy: connecting research that addresses displacement of environmental impacts. Conserv. Lett. 12:e12628. doi: 10.1111/conl.12628
Lindkvist, E., Basurto, X., and Schlüter, M. (2017). Micro-level explanations for emergent patterns of self-governance arrangements in small-scale fisheries—A modeling approach. PLoS One 12:e0175532. doi: 10.1371/journal.pone.0175532
Lindkvist, E., and Norberg, J. (2014). Modeling experiential learning: the challenges posed by threshold dynamics for sustainable renewable resource management. Ecol. Econ. 104, 107–118. doi: 10.1016/j.ecolecon.2014.04.018
Magliocca, N. R., Ellis, E. C., Allington, G. R., de Bremond, A., Dell’Angelo, J., Mertz, O., et al. (2018). Closing global knowledge gaps: producing generalized knowledge from case studies of social-ecological systems. Glob. Environ. Change 50, 1–14. doi: 10.1016/j.gloenvcha.2018.03.003
Mahon, R., McConney, P., and Rathindra, R. (2008). Governing fisheries as complex adaptive systems. Mar. Policy 32, 104–112. doi: 10.1016/j.marpol.2007.04.011
Marín, A., Gelcich, S., Castilla, J. C., and Berkes, F. (2012). Exploring social capital in chile’s coastal benthic comanagement system using a network approach. Ecol. Soc. 17:13. doi: 10.5751/ES-04562-170113
McClanahan, T. R., Castilla, J. C., White, A. T., and Defeo, O. (2009). Healing small-scale fisheries by facilitating complex socio-ecological systems. Rev. Fish Biol. Fish. 19, 33–47. doi: 10.1007/s11160-008-9088-8
Melbourne-Thomas, J., Johnson, C. R., Perez, P., Eustache, J., Fulton, E. A., and Cleland, D. (2011). Coupling biophysical and socioeconomic models for coral reef systems in quintana roo, Mexican Caribbean. Ecol. Soc. 16:23. doi: 10.5751/ES-04208-160323
Müller, B., Bohn, F., Dreßler, G., Groeneveld, J., Klassert, C., Martin, R., et al. (2013). Describing human decisions in agent-based models – ODD + D, an extension of the ODD protocol. Environ. Model. Softw. 48, 37–48. doi: 10.1016/j.envsoft.2013.06.003
Mwaipopo, R., Lange, G.-M., and Breton, Y. (2010). Understanding the human dimensions in the management of coastal and marine resources in the WIO region. Ocean Coast. Manag. 53, 147–149. doi: 10.1016/j.ocecoaman.2010.01.005
Nielsen, J. R., Thunberg, E., Holland, D. S., Schmidt, J. O., Fulton, E. A., Bastardie, F., et al. (2018). Integrated ecological–economic fisheries models—Evaluation, review and challenges for implementation. Fish Fish. 19, 1–29.
Oliver, T. A., Oleson, K. L. L., Ratsimbazafy, H., Raberinary, D., Benbow, S., and Harris, A. (2015). Positive catch & economic benefits of periodic octopus fishery closures: do effective, narrowly targeted actions ‘catalyze’ broader management? PLoS One 10:e0129075. doi: 10.1371/journal.pone.0129075
Ostrom, E. (2007). A diagnostic approach for going beyond panaceas. Proc. Natl. Acad. Sci. U.S.A. 104, 15181–15187. doi: 10.1073/pnas.0702288104
Ovando, D. A., Deacon, R. T., Lester, S. E., Costello, C., Van Leuvan, T., McIlwain, K., et al. (2013). Conservation incentives and collective choices in cooperative fisheries. Mar. Policy 37, 132–140. doi: 10.1016/j.marpol.2012.03.012
Pace, M. L., and Gephart, J. A. (2017). Trade: a driver of present and future ecosystems. Ecosystems 20, 44–53. doi: 10.1007/s10021-016-0021-z
Perez, P., Dray, A., Cheland, D., and Ariaz-Gonzalez, J. E. (2009). “An agent-based model to address coastal management issues in the Yucatan Peninsula, Mexico,” in 18th World IMACS / MODSIM Congress, Cairns, eds R. S. Andressen, and L. T. H. Braddock Newham, (Canberra: IMACS/MODSIM):7.
Plank, M. J., Kolding, J., Law, R., Gerritsen, H. D., and Reid, D. (2017). Balanced harvesting can emerge from fishing decisions by individual fishers in a small-scale fishery. Fish Fish. 18, 212–225. doi: 10.1111/faf.12172
Poteete, A. R., Janssen, M. A., and Ostrom, E. (2010). Working Together: Collective Action, the Commons, and Multiple Methods in Practice. Princeton, NJ: Princeton University Press.
Purcell, S. W., and Pomeroy, R. S. (2015). Driving small-scale fisheries in developing countries. Front. Mar. Sci. 2:44. doi: 10.3389/fmars.2015.00044
Quinn, T. J. (2003). Ruminations on the development and future of population dynamics models in fisheries. Nat. Resour. Model. 16, 341–392. doi: 10.1111/j.1939-7445.2003.tb00119.x
Railsback, S. F., and Grimm, V. (2011). Agent-Based and Individual-Based Modeling: A Practical Introduction. Princeton, NJ: Princeton University Press.
Rudd, M. A., Railsback, S., Danylchuk, A., and Clerveaux, W. (2003). “Developing a spatially explicit agent-based model of queen conch distribution in a Marine Protected Area in the Turks and Caicos Islands,” in Proceedings of the Gulf Caribbean Fisheries Institute (GCFI), (Marathon, GD: GCFI), 259–271.
Schlüter, M., Baeza, A., Dressler, G., Frank, K., Groeneveld, J., Jager, W., et al. (2017). A framework for mapping and comparing behavioural theories in models of social-ecological systems. Ecol. Econ. 131, 21–35. doi: 10.1016/j.ecolecon.2016.08.008
Schlüter, M., Mcallister, R. R. J., Arlinghaus, R., Bunnefeld, N., Eisenack, K., Holker, F., et al. (2012). New horizons for managing the environment: a review of coupled social-ecological systems modeling. Nat. Resour. Model. 25, 219–272. doi: 10.1111/j.1939-7445.2011.00108.x
Schlüter, M., Orach, K., Lindkvist, E., Martin, R., Wijermans, N., Bodin, Ö, et al. (2019). Toward a methodology for explaining and theorizing about social-ecological phenomena. Curr. Opin. Environ. Sustain. 39, 44–53. doi: 10.1016/j.cosust.2019.06.011
Schulze, J., Müller, B., Groeneveld, J., and Grimm, V. (2017). Agent-based modelling of social-ecological systems: achievements, challenges, and a way forward. J. Artif. Soc. Soc. Simul. 20:8.
Singh, G. G., Cisneros-Montemayor, A. M., Swartz, W., Cheung, W., Guy, J. A., Kenny, T.-A., et al. (2018). A rapid assessment of co-benefits and trade-offs among sustainable development goals. Mar. Policy 93, 223–231. doi: 10.1016/j.marpol.2017.05.030
Smajgl, A., and Barreteau, O. (2017). Framing options for characterising and parameterising human agents in empirical ABM. Environ. Model. Softw. 93, 29–41. doi: 10.1016/j.envsoft.2017.02.011
Smith, H., and Basurto, X. (2019). Defining small-scale fisheries and examining the role of science in shaping perceptions of who and what counts: a systematic review. Front. Mar. Sci. 6:236. doi: 10.3389/fmars.2019.00236
Soulié, J.-C., and Thébaud, O. (2006). Modeling fleet response in regulated fisheries: an agent-based approach. Math. Comput. Model. 44, 553–564. doi: 10.1016/j.mcm.2005.02.011
Sterman, J. D. (2001). System dynamics modeling: tools for learning in a complex world. Calif. Manage. Rev. 43, 8–25. doi: 10.1109/TVCG.2018.2825424
Swartz, W., Sala, E., Tracey, S., Watson, R., and Pauly, D. (2010). The spatial expansion and ecological footprint of fisheries (1950 to present). PLoS One 5:e15143. doi: 10.1371/journal.pone.0015143
Taylor, R., Besa, M. C., and Forrester, J. (2016). Agent-Based Modelling: A Tool for Addressing the Complexity of Environment and Development Policy Issues.Oxford: Stockholm Environment Institute.
Tesfatsion, L. (1997). “A Trade Network Game with Endogenous Partner Selection,” in Computational Approaches to Economic Problems, eds H. Amman, B. Rustem, and A. Whinston, (Boston, MA: Springer US), 249–269. doi: 10.1007/978-1-4757-2644-2_17
The World Bank (2012). Hidden Harvest?: the Global Contribution of Capture Fisheries. Available at: http://documents.worldbank.org/curated/en/515701468152718292/Hidden-harvest-the-global-contribution-of-capture-fisheries (Accessed April 9, 2018)
Townsend, R., Shotton, R., and Uchida, H. (2008). Case Studies in Fisheries Self-Governance. Rome: Food and Agriculture Organization of the United Nations.
van Putten, I. E., Kulmala, S., Thébaud, O., Dowling, N., Hamon, K. G., Hutton, T., et al. (2012). Theories and behavioural drivers underlying fleet dynamics models. Fish Fish. 13, 216–235. doi: 10.1111/j.1467-2979.2011.00430.x
Villasante, S., Arreguín-Sánchez, F., Heymans, J. J., Libralato, S., Piroddi, C., Christensen, V., et al. (2016). Modelling marine ecosystems using the Ecopath with Ecosim food web approach: new insights to address complex dynamics after 30 years of developments. Ecol. Model. 331, 1–4. doi: 10.1016/j.ecolmodel.2016.04.017
Waldherr, A., and Wijermans, N. (2013). Communicating social simulation models to sceptical minds. J. Artif. Soc. Soc. Simul. 16:13.
Weber, C. T., Borit, M., and Aschan, M. (2019). An interdisciplinary insight into the human dimension in fisheries models. A systematic literature review in a european union context. Front. Mar. Sci. 6:369. doi: 10.3389/fmars.2019.00369
Wilson, J., Yan, L., and Wilson, C. (2007). The precursors of governance in the Maine lobster fishery. Proc. Natl. Acad. Sci. U.S.A. 104, 15212–15217. doi: 10.1073/pnas.0702241104
Worrapimphong, K., Gajaseni, N., Le Page, C., and Bousquet, F. (2010). A companion modeling approach applied to fishery management. Environ. Model. Softw. 25, 1334–1344. doi: 10.1016/j.envsoft.2010.03.012
Wosu, A. (2019). Access and institutions in a small-scale octopus fishery: a gendered perspective. Mar. Policy 108:103649. doi: 10.1016/j.marpol.2019.103649
Yu, R., Pan, M., Railsback, S. F., and Leung, P. (2009). “A prototype agent based fishery management model of hawaii’s longline fishery,” in 18th World IMACS Congress and MODSIM09 International Congress on Modelling and Simulation, eds R. S. Anderssen, R. D. Braddock, and L. T. H. Newham, (Canberra: IMACS/MODSIM).
Keywords: interdisciplinary methods, interactions, data paucity, integrated systems, complex adaptive systems, social–ecological systems
Citation: Lindkvist E, Wijermans N, Daw TM, Gonzalez-Mon B, Giron-Nava A, Johnson AF, van Putten I, Basurto X and Schlüter M (2020) Navigating Complexities: Agent-Based Modeling to Support Research, Governance, and Management in Small-Scale Fisheries. Front. Mar. Sci. 6:733. doi: 10.3389/fmars.2019.00733
Received: 14 December 2018; Accepted: 11 November 2019;
Published: 17 January 2020.
Edited by:
Steven W. Purcell, Southern Cross University, AustraliaReviewed by:
Oscar Sosa-Nishizaki, Ensenada Center for Scientific Research and Higher Education (CICESE), MexicoErnesto Carrella, University of Oxford, United Kingdom
Copyright © 2020 Lindkvist, Wijermans, Daw, Gonzalez-Mon, Giron-Nava, Johnson, van Putten, Basurto and Schlüter. This is an open-access article distributed under the terms of the Creative Commons Attribution License (CC BY). The use, distribution or reproduction in other forums is permitted, provided the original author(s) and the copyright owner(s) are credited and that the original publication in this journal is cited, in accordance with accepted academic practice. No use, distribution or reproduction is permitted which does not comply with these terms.
*Correspondence: Emilie Lindkvist, emilie.lindkvist@su.se