- 1Japan Agency for Marine-Earth Science and Technology, Yokohama, Japan
- 2Centre for Maritime Research and Experimentation (CMRE), La Spezia, Italy
- 3Centro Euro-Mediterraneo per i Cambiamenti Climatici, Bologna, Italy
- 4Atmosphere and Ocean Research Institute, The University of Tokyo, Chiba, Japan
- 5Everlasting Nature of Asia (ELNA), Yokohama, Japan
In this work, we added the assimilation of subsurface temperature measurements obtained from sea turtles around the Arafura Sea from June to October 2017 into an operational seasonal prediction system. The impact of these measurements was explored by conducting so-called ocean observing system experiments. It was found that the prediction of regional sea surface temperatures around the Arafura Sea was significantly improved at 3–4 months of lead time. The results also showed that the addition of temperature measurements from sea turtles into the existing Global Ocean Observing System (including satellites, mooring buoys, ships, and profiling floats) may open a new door to improving regional seasonal prediction through better representation of the initial state of the upper ocean.
Introduction
Observations of ocean temperature and salinity are essential for the initialization of seasonal prediction with a dynamical ocean–atmosphere coupled model (Vidard et al., 2007) because a potential source of seasonal predictability arises from the long memory associated with the high heat capacity of the ocean relative to the atmosphere. In particular, the sea surface temperature (SST) in tropical oceans is key, as shown in the pioneering work of Bjerknes (1969). Accurate prediction of tropical climate variability modes, such as the El Niño-Southern Oscillation (ENSO) and Indian Ocean Dipole, is crucial for successful seasonal prediction around the world (Saji et al., 1999; Shukla et al., 2000; Wang et al., 2000; Hoerling and Kumar, 2001; Behera and Yamagata, 2003; Saji and Yamagata, 2003; Kosaka et al., 2013) and, thus, for societal applications in agriculture, human health, and natural disaster prevention (e.g., Hashizume et al., 2009; Iizumi et al., 2013; Takaya et al., 2014; Yuan and Yamagata, 2015; Ikeda et al., 2017; Oettli et al., 2018).
The amount of ocean observations has increased enormously over the recent decade. SST is the most important variable to understand air–sea interactions. As of late 1981, satellite measurements have greatly improved such data coverage with respect to in situ observations alone, and they have provided fine-resolution SST data in real time (Reynolds et al., 2002, 2007). Subsurface temperature observations are primarily provided by mooring buoys, research vessels, profiling floats, and others. The mooring buoys located in the tropical oceans consist of the Tropical Atmosphere–Ocean/Triangle Trans-Ocean Buoy Network (TAO/TRITON) in the tropical Pacific (Mcphaden et al., 1998; Ando et al., 2017; Smith et al., 2019), the Research Moored Array for African-Asian-Australian Monsoon Analysis and Prediction (RAMA) in the tropical Indian Ocean (McPhaden et al., 2008; Hermes et al., 2019), and the Pilot Research Moored Array in the Tropical Atlantic (PIRATA) (Bourles et al., 2008; Foltz et al., 2019). The global Volunteer Observing Ship (VOS) program provides expendable bathythermograph (XBT) measurements along merchant shipping routes. More recently, the international Argo network of drifting profilers has greatly improved the data coverage of subsurface temperature and salinity, revolutionizing subsurface ocean sampling (e.g., Hosoda et al., 2008).
Observational datasets for use in data assimilation are thus created by collecting all measurements from the aforementioned sources and homogenizing the data in terms of format and, in some cases, vertical sampling and accuracy, before eventually bias-correcting the data. For instance, Version 4 of the Met Office Hadley Center series “EN4” gathers data from all types of ocean profiling measurements that provide temperature and (when available) salinity and provides global quality-controlled ocean temperature and salinity profiles in near real time (Good et al., 2013).
As an additional data source, ocean observational data from marine animal-borne instruments via satellites in near real time are emerging (Harcourt et al., 2019). Some marine animal-borne instruments can provide in situ subsurface temperature profile data from parts of the oceans wherein little or no other data are currently available. For example, the Argo float, a very powerful tool for quasi- real-time monitoring of high-quality subsurface temperatures and salinity fields, cannot cover areas of shallow ocean like the coastal regions and the marginal seas because the float is designed for the upper 2,000 m of the ice-free global ocean. If some of the animal-borne instruments were additionally capable of real-time subsurface temperature data beyond the data coverage already achieved by the present Global Ocean Observing System, the information could be proven for all ranges of prediction: weather, subseasonal, seasonal, multiyear, decadal, and longer-scales climate prediction. The first use of marine mammal data in operational oceanography dates back to 2004 (see Boehme et al., 2009). Previous studies also demonstrated the benefit of marine animal-borne instruments in ocean analysis, reanalysis, and nowcast/forecast systems (Roquet et al., 2013; Carse et al., 2015; Miyazawa et al., 2015, 2019; Mallett et al., 2018).
In the present study, we focused on the Arafura Sea, one of the marginal seas of the Maritime Continent. With its complex distribution of several islands and shallow oceans, the region experiences some of the warmest ocean temperatures of the world and is commonly known as the “boiler box” of the global weather and climate (Ramage, 1968; Neale and Slingo, 2003). Moreover, this region is strongly related to the northern Great Barrier Reef, wherein coral bleaching has widely been reported in marine heat waves (Hughes et al., 2017). Subsurface temperature data, however, remains largely unavailable in the area.
From June to October 2017, we successfully obtained temperature profile data from sea turtles in that area. To investigate the impacts of turtle-based temperature-depth profiles on seasonal prediction, we performed observing system experiments (e.g., Oke and Schiller, 2007; Fujii et al., 2015) with a quasi-real-time seasonal prediction system based on a coupled general circulation model (GCM), named SINTEX-F2 (Doi et al., 2017). For the original ensemble reforecast experiments, the EN4 dataset (including all types of ocean measurements except for those from animal-borne instruments) was used for the initialization. For this current study, however, we performed new ensemble reforecast experiments with additional temperature measurements from the sea turtles at the initialization and also explored the differences between the outputs of these runs and those of the original runs. We believe that our research could open a new door in improving regional seasonal prediction skills through better representation of the initial state of the subsurface ocean via integrating marine animal-borne instruments into the present Global Ocean Observing System.
Materials and Methods
Temperature Profiles From Sea Turtles
In May and June 2017, Satellite Relay Data Loggers (SRDL; Sea Mammal Research Unit, St Andrews, United Kingdom) were deployed on five olive ridley turtles (Lepidochelys olivacea) nesting on a beach in Jeen Syrup and the Warmamedi beach in Jeen Womon (0°22′ to 0°25′ S, 132°34′ to 132°48′ E) near the top of Bird’s Head Peninsula, West Papua, Indonesia (Figure 1A). Olive ridley turtles were considered suitable for subsurface temperature observation at the study site because this species more frequently dives into the deeper subsurface ocean and spends more time at the subsurface than other species of hard-shelled sea turtles (Polovina et al., 2003, 2004). After the SRDLs were glued on their carapaces using epoxy resin, the turtles were released from the beaches. The SRDLs measured depth every 4 s and recorded individual dives when the turtles submerged to a depth below 3 m for more than 30 s. They also measured ambient temperatures every 4 s at an accuracy of 0.1°C, and 10 depth-temperature points were selected based on the conventional broken-stick algorithm used for XBTs (Narazaki et al., 2015). Furthermore, the depth-temperature data were randomly transmitted via the Argos system (www.argos-system.org). The SRDL has a temperature accuracy comparable to that of an XBT, though the CTD-SRDL has far better accuracy (Siegelman et al., 2019). The data obtained from sea turtles underlying the study are available at https://fbox.jamstec.go.jp/public/3v08gAbSZIDAm20BqNVtpiKzIEVOabKqvUsV8HldZIPM/m/3~$/times%20$~5AD2lA.
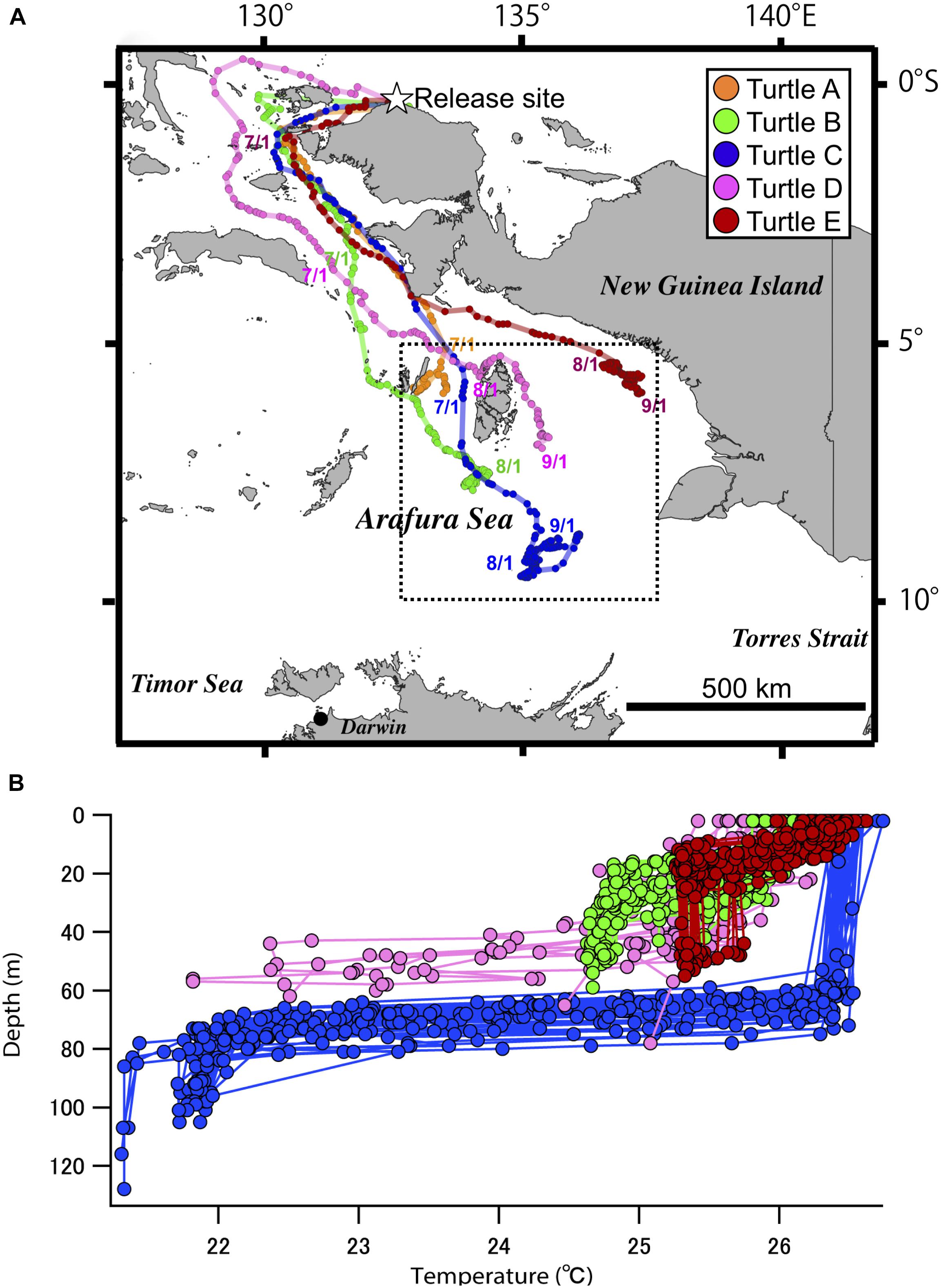
Figure 1. (A) Migration routes of the five olive ridley turtles from June to October 2017. Each color indicates each individual, and color circles indicate the position where depth-temperature profiles were transmitted. (B) Depth–temperature profiles obtained from turtles in the region of 133°–138°E, 10°–5°S (shown by black dotted box in panel A) during the period from July 27 to August 6, 2017. The colors are the same as (A).
All five turtles migrated to the Arafura Sea located between northern Australia and New Guinea Island. They also stayed there for about 3 months until transmission ceased (Figure 1A). We successfully obtained more than 1,000 depth-temperature profile measurements from June to October 2017 during the sea turtles’ dives. In particular, temperature profiles from the surface to a depth of about 120 m were obtained around August 1, 2017 in the region of 133°–138°E, 10°–5°S (Figure 1B). We could see the differences among the temperature profiles of the four turtles. It is not surprising because the migration routes of the turtles were also different, even inside the region of 133°–138°E, 10°–5°S; the four turtles were at the different locations at this date (Figure 1A).
Dynamical Seasonal Prediction System
The dynamical seasonal prediction system used here was based on an ocean–atmosphere–land–sea ice coupled climate model, which is called the SINTEX-F2 coupled model (Masson et al., 2012; Sasaki et al., 2013). The atmospheric component (ECHAM5) had a horizontal resolution of T106 with 31 vertical levels. Meanwhile, the oceanic component (OPA9) had a horizontal resolution of a 0.5° × 0.5° tripolar grid, known as the ORCA05 configuration, with 31 vertical levels. The vertical grid points of the ocean model in the upper 150 m-depth were 5, 15, 25, 35, 45, 55, 65, 75, 85, 95, 106, 11, 129, and 142 m. The atmospheric and oceanic fluxes were exchanged every 2 h with no flux correction.
The initialization scheme blended the continuous SST-nudging scheme with monthly three-dimensional variational ocean data assimilation (3DVAR) of subsurface data (Doi et al., 2017). The high-resolution daily NOAA OISST analysis with 0.25 degree latitude × 0.25 degree longitude resolution (Reynolds et al., 2007) was used for the SST-nudging scheme from 1982 to present (Doi et al., 2016). The 3DVAR was conducted every first day of each month using the EN4 in situ profile data of temperature and salinity (Good et al., 2013) in the temporal range of ±5 days before and after the assimilation time. The details of the 3DVAR scheme used here are discussed in Storto et al. (2011, 2014) and Doi et al. (2017).
This dynamical seasonal prediction system has six original ensemble members, which are used for the quasi-real-time operational seasonal predictions made by the Application Laboratory, Japan Agency for Marine-Earth Science Technology (APL/JAMSTEC). The six-ensemble members are generated by two steps: (1) three large negative feedback values (−2,400, −1,200, and −800 W m–2 K–1) for the SST-nudging scheme in the initialization phase (Luo et al., 2005; Doi et al., 2016) and (2) two different modeling methods for the ocean vertical mixing that is induced by small vertical scale structures within and above the equatorial thermocline (Sasaki et al., 2012). Therefore, the six-ensemble prediction system can, to some extent, take into account uncertainties of both initial conditions and model physics. We conducted reforecasts with a 12-month lead time, starting from the first day of each month from January 1983 to the present. The prediction anomalies were determined by removing a posteriori the model mean climate at each lead time using the reforecast outputs during the period from 1983 to 2015. This seasonal prediction system shows the high prediction skill of ENSO, the Indian Ocean Dipole, and their associated abnormal climate events, particularly in the tropics. A more detailed overview of this seasonal prediction system and its prediction skill can be found in Doi et al. (2017). This prediction system has also been used in multiyear prediction (Morioka et al., 2018a, b; Ogata et al., 2019).
Ocean Observing System Experiments
Based on the original reforecast experiments with the SINTEX-F2 system, we additionally assimilated the temperature profile data from sea turtles during the assimilation cycles corresponding to the starting dates on the first days of July and August 2017. Here, we used the data gathered ±5 days before and after the assimilation time and then configured the original reforecast experiments. We also performed six-ensemble reforecast experiments from the new initial state of August 1, 2017; this was after assimilating the sea turtles’ measurements (experiment “wTurtle_forecast”) and when sea turtle data were abundant around the Arafura Sea (Figure 1A). Figure 2 shows the horizontal map of the subsurface ocean observational points at 50–150 m depths that were assimilated in the SINTEX-F2 seasonal prediction system during the period from June to August 2017. The temperature measurements (red dots) from the sea turtles covered the subsurface ocean around the Arafura Sea, where no other data were previously available, at least from the EN4 dataset (black dots). Then, we assimilated the temperature data obtained by the sea turtles after passing quality control procedures. The quality control procedures were embedded in the variational data assimilation scheme and relied on background quality checks, wherein observations with a square misfit larger than thrice the sum of the observation and background error variances were rejected [see Storto (2016) for further details on the background quality check].
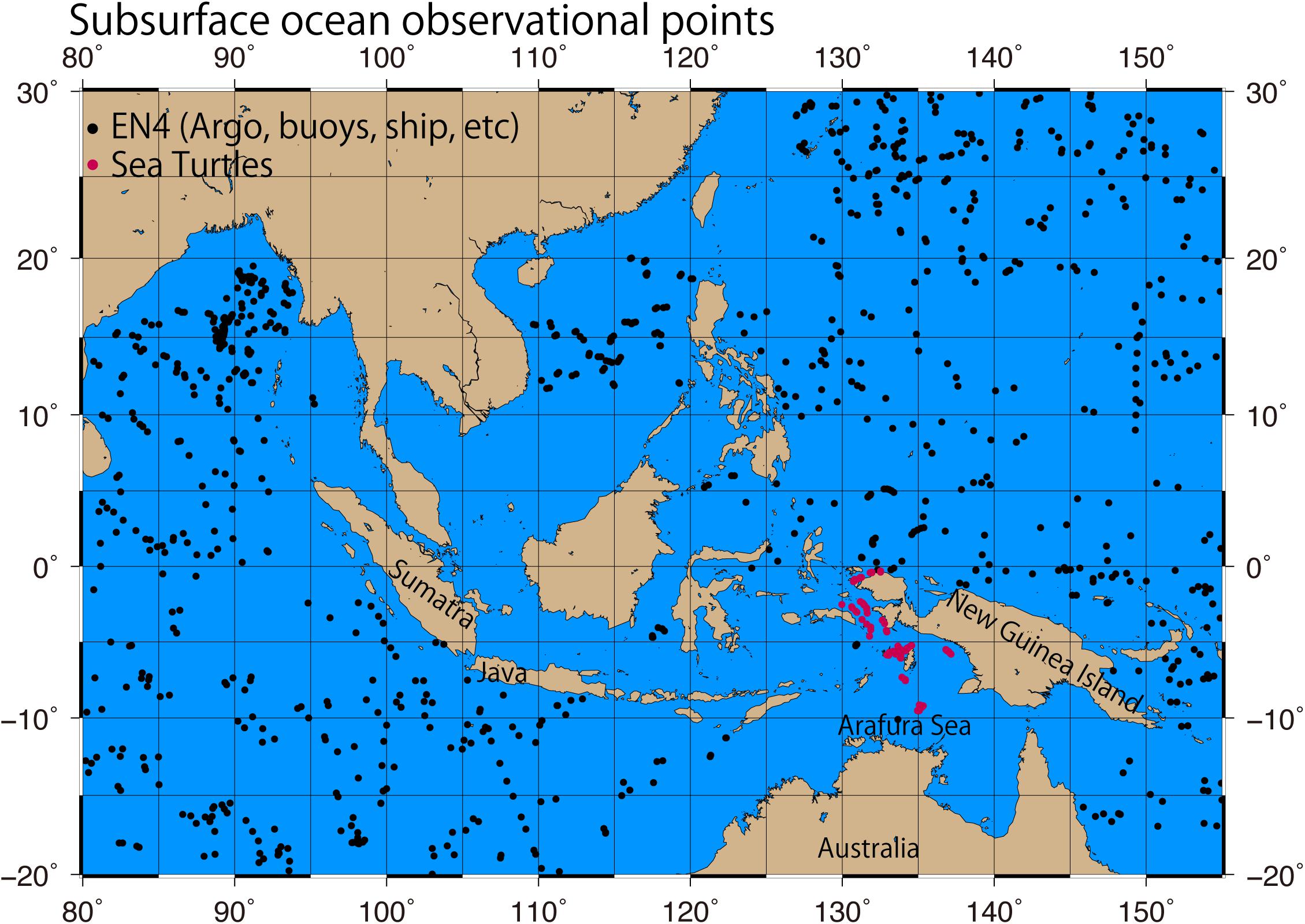
Figure 2. Horizontal map of the subsurface ocean observational points in 50–150 m-depth that are assimilated to the SINTEX-F2 seasonal prediction system during June–August 2017 (black dots, EN4 datasets; red dots, measurements from sea turtles).
To evaluate the impact of adding temperature measurements from sea turtles into the SINTEX-F2 seasonal prediction system, the signal against the noise from the chaotic nature of the climate system was defined as the statistically significant difference in the ensemble means between the Ctl_forecast and the wTurtle_forecast beyond the 90% confidence levels on a paired t-test. To evaluate the SST predictions, the NOAA OISSTv2 (Reynolds et al., 2002) was used as the reference for the monthly SST. The monthly climatologies were calculated by averaging the monthly data over the period from 1983 to 2015, and anomalies were defined as deviations from them. To evaluate the teleconnection from the Arafura Sea, we also used the NCEP/NCAR reanalysis data (Kalnay et al., 1996) for the 2 m-air temperature as well as the GPCP data (Adler et al., 2003) for precipitation.
Results
We started compiling differences in the ocean mixed layer depth in August 2017, at 0–1 months lead time after initialization. The mixed layer depth of the wTurtle_forecast was shallower than that of the Ctl_forecast in the Arafura Sea, as shown in Figure 3A. The maximum difference was about 10 m in the region of 133°–138°E, 10°–5°S, which is consistent with the location of the temperature measurements from the sea turtles (Figures 1, 2). Figure 1B shows the depth–temperature profiles in this region on the August 1st 2017, i.e., the initialized date of the ocean observing system experiments. The total number of observation points from the sea turtles from the surface to around a depth of 120 m (976 points) was assimilated into the seasonal prediction system in this region on the August 1, 2017 (Figure 4). The wTurtle_forecast showed the shallower mixed layer depth and a lower temperature by about 1°C around 60–80 m depths relative to the Ctl_forecast. Thus, it is reasonable to assume that the assimilation of those observations strengthened the ocean stratification in shallow areas in which no data were available because models at a relatively coarse resolution tend to smooth out the vertical structure and stratification. As the mean mixed layer depth in the climatology was about 35 m there, the difference was beyond 20% of the mean. Thus, we can expect that this difference in the subsurface ocean may influence the seasonal climate at a few months lead time. Note that there were some differences observed outside of the Arafura Sea, e.g., around Java-Sumatra. Although the differences were statistically significant beyond the 90% confidence levels, the values were only on the order of 3-m. Therefore, we expect that these differences outside of the Arafura Sea are just noise, and do not strongly influence the seasonal predictions at a few months lead time.
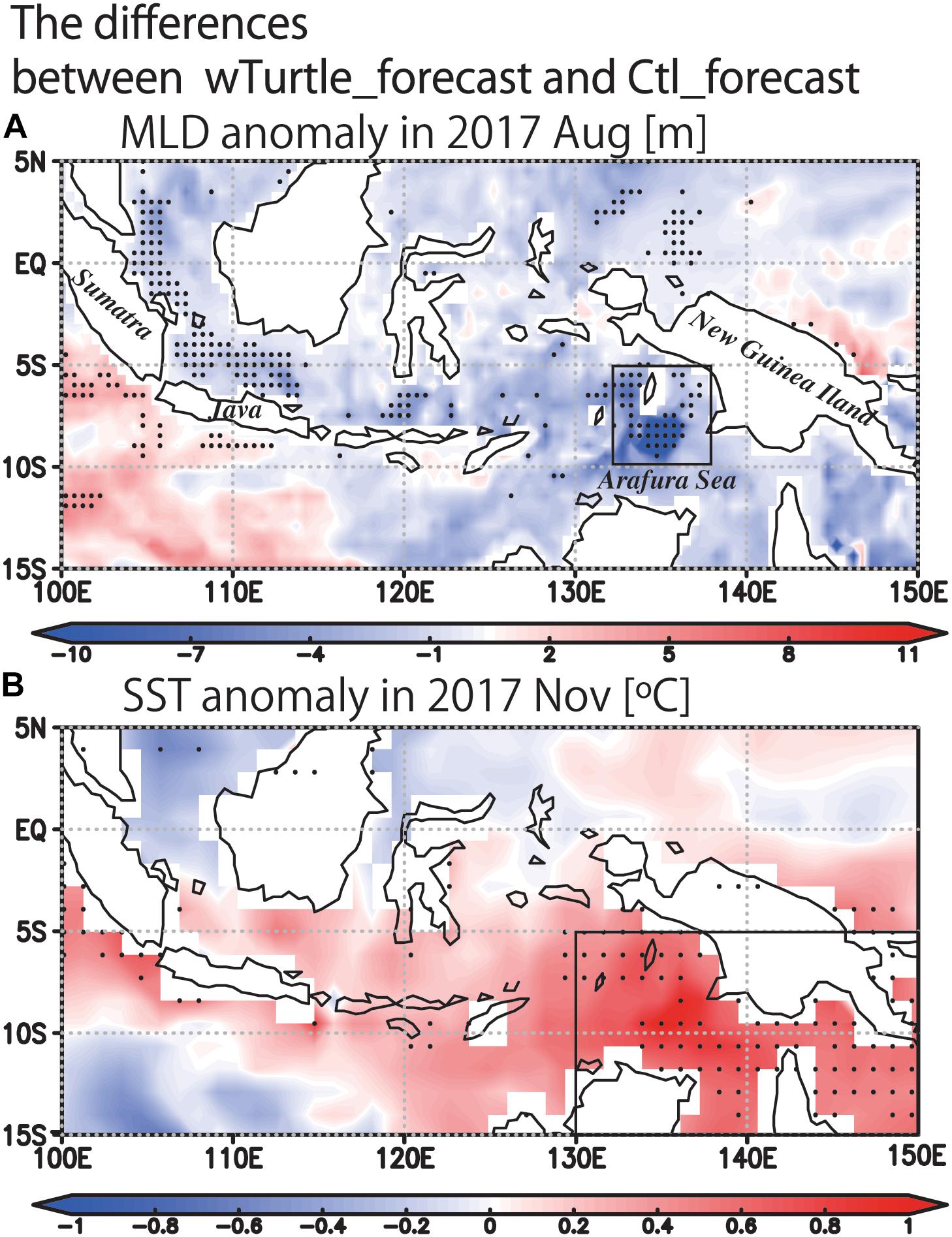
Figure 3. (A) Difference in the ocean mixed layer depth (MLD) in August 2017 between the ensemble mean of wTurtle_forecast and that of Ctl_forecast (m). Black dots indicate the statistically significant difference in the ensemble means between them beyond the 90% confidence levels on paired t-test are shown by black dots. The region for Figure 4 is shown by a black box. (B) The same as (A) but for the sea surface temperature (SST) in November 2017 (°C). The region for Figure 6 is shown by a black box.
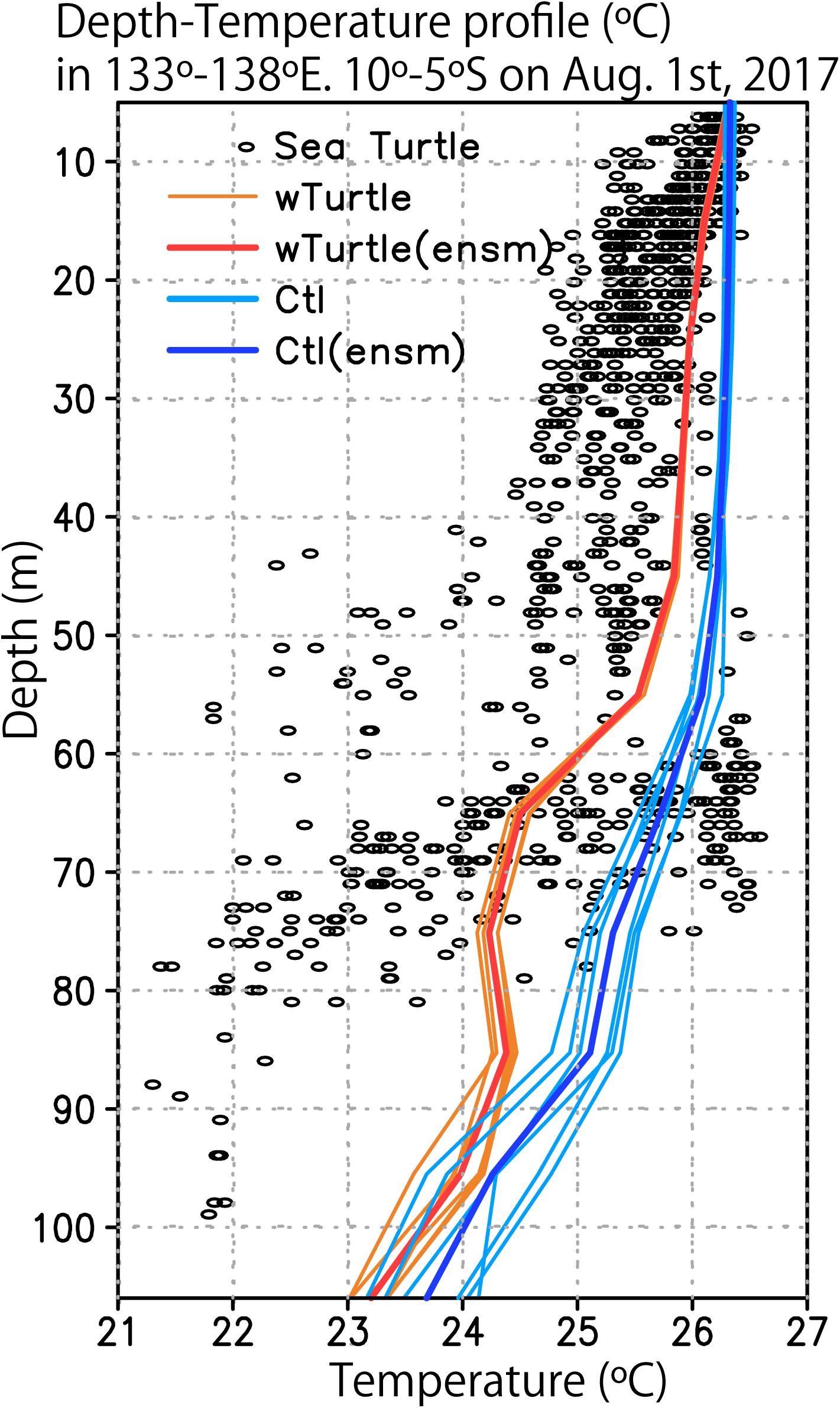
Figure 4. The depth–temperature profiles in 133°–138°E and 10°–5°S on the August 1, 2017, initialization. Circle mark shows the observation from sea turtles assimilated to the seasonal prediction system in this region (976 points). The regional averages of the depth-temperature profiles in 133°–138°E, and 10°–5°S on the August 1, 2017, initialization with the six-ensemble members by Ctl_forecast (thin right blue, each ensemble member; thick blue, ensemble mean) and the six-ensemble members by wTurtle_forecast (thin orange, each ensemble member; thick red, ensemble mean) are shown.
Figure 3B shows the SST difference in November 2017, 3–4 months lead time after the initialization. The SST predicted by the wTurtle_forecast was higher in the Arafura Sea than that predicted by the Ctl_forecast (Figure 3B). Figure 5 shows the SST anomalies found from the observations, the Ctl_forecast, and the wTurtle_forecast. It can be seen that the wTurtle_forecast was indeed more realistic over the Arafura Sea. We calculated the spatial root mean square error (RMSE) of the difference in the SST between the observation and the predictions for the region of 130°–150°E, 15°S–5°S. While the RMSE in the Ctl_forecast is 0.58, that in the wTurtle_forecast is 0.28, only about 50% of the RMSE in the Ctl_forecast. Meanwhile, Figure 6 shows the time series of the SST anomaly prediction averaged in 130°–150°E, 15°–5°S. For August 2017, the observations show that the SST was higher than normal in this region; following this, the observations show that the positive SST anomaly persisted through the austral summer. However, the ensemble mean of the Ctl_forecast predicted that the positive SST anomaly would quickly decay and that this region would return to a normal state after October. As shown in the wTurtle_forecast, the sea turtle data assimilation was able to precondition the shallow mixed layer depth in the austral winter and sustain the positive SST anomaly in the following austral spring/summer, which, otherwise, would have soon vanished without proper constraint of the mixed layer depth, as in the prediction of the Ctl_forecast.
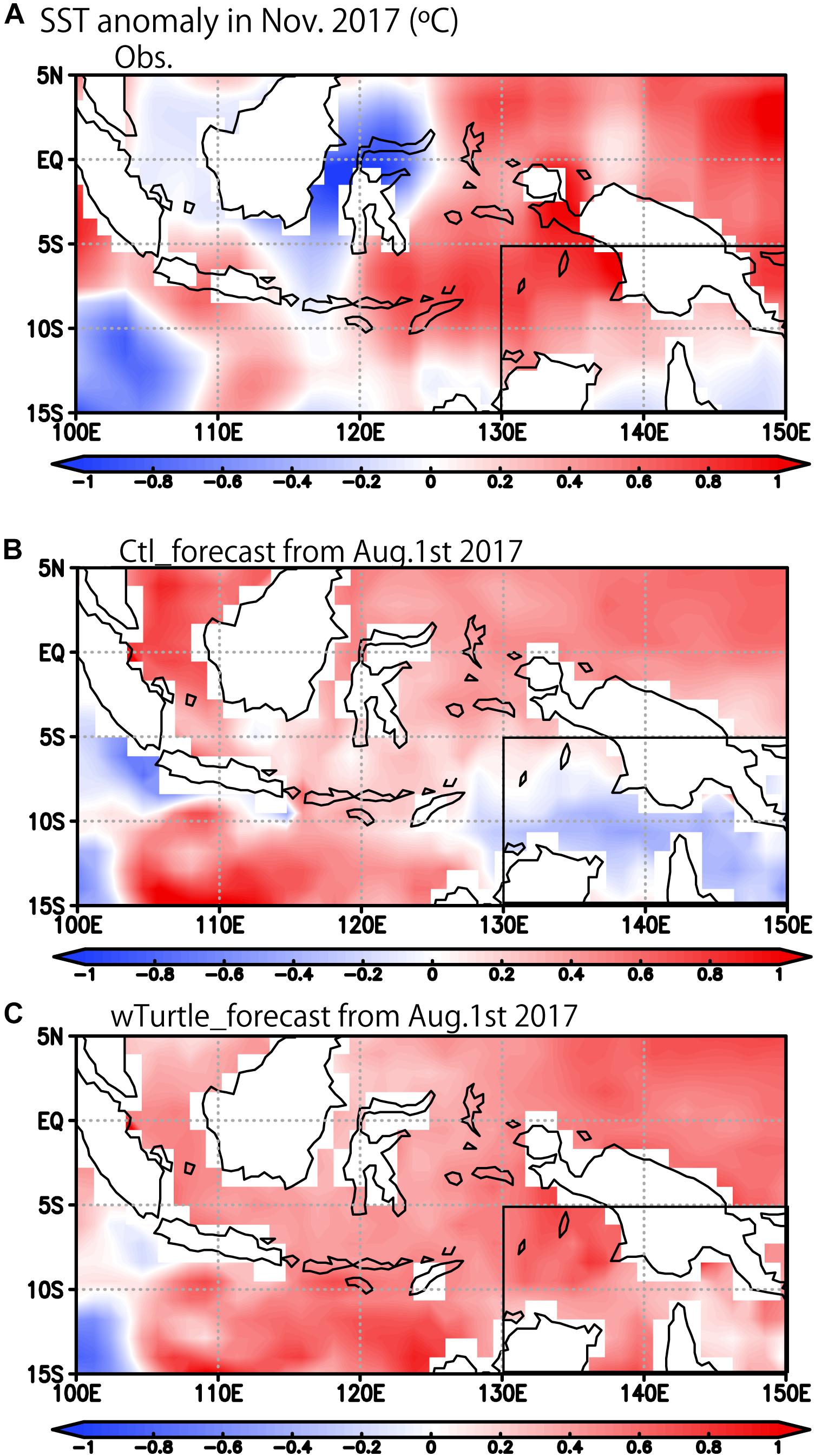
Figure 5. SST anomaly in November 2017 from (A) the observations, (B) the Ctl_forecast, and (C) the wTurtle_forecast issued on August 1, 2017 (°C). The region for Figure 6 is shown by a black box.
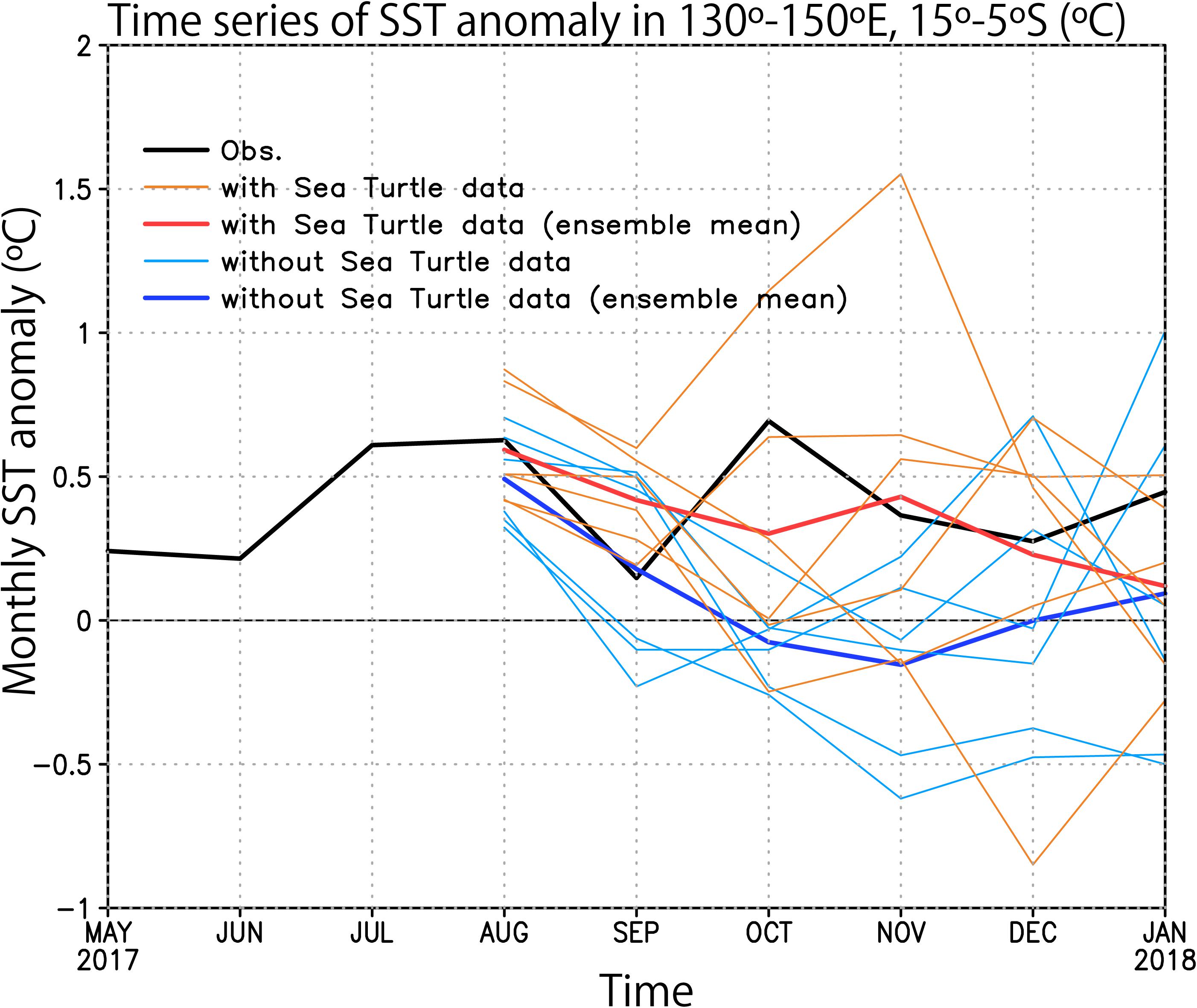
Figure 6. Monthly SST anomaly averaged in 130°–150°E, 15°S–5°S during the latter half of 2017 (°C) from the observational data of NOAA OISSTv2 (black) and the prediction from the August 1, 2017, initialization with the six-ensemble by the Ctl_forecast (thin right blue, each ensemble member; thick blue, ensemble mean) and the six-ensemble by the wTurtle_forecast (thin orange, each ensemble member; thick red, ensemble mean).
Discussion
We have shown that additional assimilation of temperature measurements from sea turtles can potentially improve regional seasonal climate prediction around the Arafura Sea, as in the 2017 warm event case. The present study was similarly a pilot study, and the results may have been influenced by the model, assimilation, and manner of generating the ensemble members. The results were based on the model initialization within July–August 2017 as a mere case study; the animal-borne measurements by sea turtles in the study area are still unique and the available data is very limited. However, the present study can support the merits of integrating marine animal-borne instruments into the present Global Ocean Observing System from the perspective of better representing the initial state of numerical seasonal prediction. We hope that our study encourages efforts for sea turtle-borne observations development in terms of deployment, collection, and processing so that we may clearly demonstrate the robustness and importance of their impact more clearly. Note that some data from marine mammals in high-latitude regions are already included in EN4. Very recently, Patel et al. (2018) suggested that the use of sea turtle-borne telemetry devices has the potential to improve the resolution of in situ temperatures through depth data and, in turn, improve oceanographic model outputs. However, as of yet, sea turtle data have not been used for predictions. Moreover, to the best of these authors’ knowledge, this is the first time that marine animal data have been used for seasonal prediction in the tropical regions. Sometimes, the impact may be regional, as shown in this study, but highly accurate predictions of regional ocean and climate may open a new door to better societal applications for the surrounding rim countries. The turtle measurements of in situ temperatures were originally motivated by a research interest in marine biology; however, they may also contribute to operational seasonal prediction, and thus climate services, and complement the coastal ocean observation network, which is not accessible by autonomous profilers. Indeed, sea turtle data are now being collected in a delayed time mode but, in the future, may also be disseminated in near real time. Such near real-time collection and dissemination services are now in development.
Figure 7 shows the horizontal distributions of the differences in SST and mixed layer depth anomalies between the wTurtle_forecast (ensemble mean) and the Ctl_forecast (ensemble mean) from August to November 2017. The anomalously shallow mixed layer depth seems to lead the positive SST anomaly in the following month around the Arafura Sea. The signal of the SST prediction extended eastward from the Arafura Sea and then appeared in a broader region relative to the signal of the mixed layer depth at 0–1 months lead time. This feature was clearly enhanced in the warmest ensemble member of the wTurtle_forecast. How could the shallow mixed layer depth around the Arafura Sea in the austral winter persist/generate the positive SST anomaly in the following austral spring/summer? After the austral winter, this region warms up, primarily due to the surface heat flux, as a normal seasonal evolution (e.g., Halkides et al., 2011). The anomalously shallow mixed layer depth could have enhanced the sensitivity of the mixed layer temperature to seasonally modulate atmospheric warming through the shallow mixed layer depth, leading to the positive SST anomaly in the following season. That is, heat fluxes are basically the same, but with a shallower mixed layer depth they can imply a stronger warming. This mechanism could potentially work in other regions on a seasonal time scale, as shown in previous works (Doi et al., 2010; Morioka et al., 2011; Kataoka et al., 2017; Tozuka et al., 2018). Although the heat budget analysis through posterior diagnostics was performed, it was still too difficult to capture the signal relative to the noise in order to reasonably trace that mechanism. As shown in Figure 6, the ensemble spread with the wTurtle_forecast was larger than that with the Ctl_forecast. This may have been partly due to the fact that the mixed layer depth in the wTurtle_forecast was shallower than that in the Ctl_forecast and could have enhanced the response of the mixed layer temperature, i.e., proxy of SST, to the noisy atmospheric forcing in this region. We also checked the differences in the rainfall between the Ctl_forecast and the wTurtle_forecast. However, we could not find a significant impact because the rainfall prediction was very noisy. These difficulties may have been partly due to the small ensemble size of the ocean observing system experiments and the availability of the sea turtle data only in limited periods and regions. To address these points, we plan to perform large ensemble reforecast experiments for more cases, although their costs are high and beyond operational capability (Doi et al., 2019).
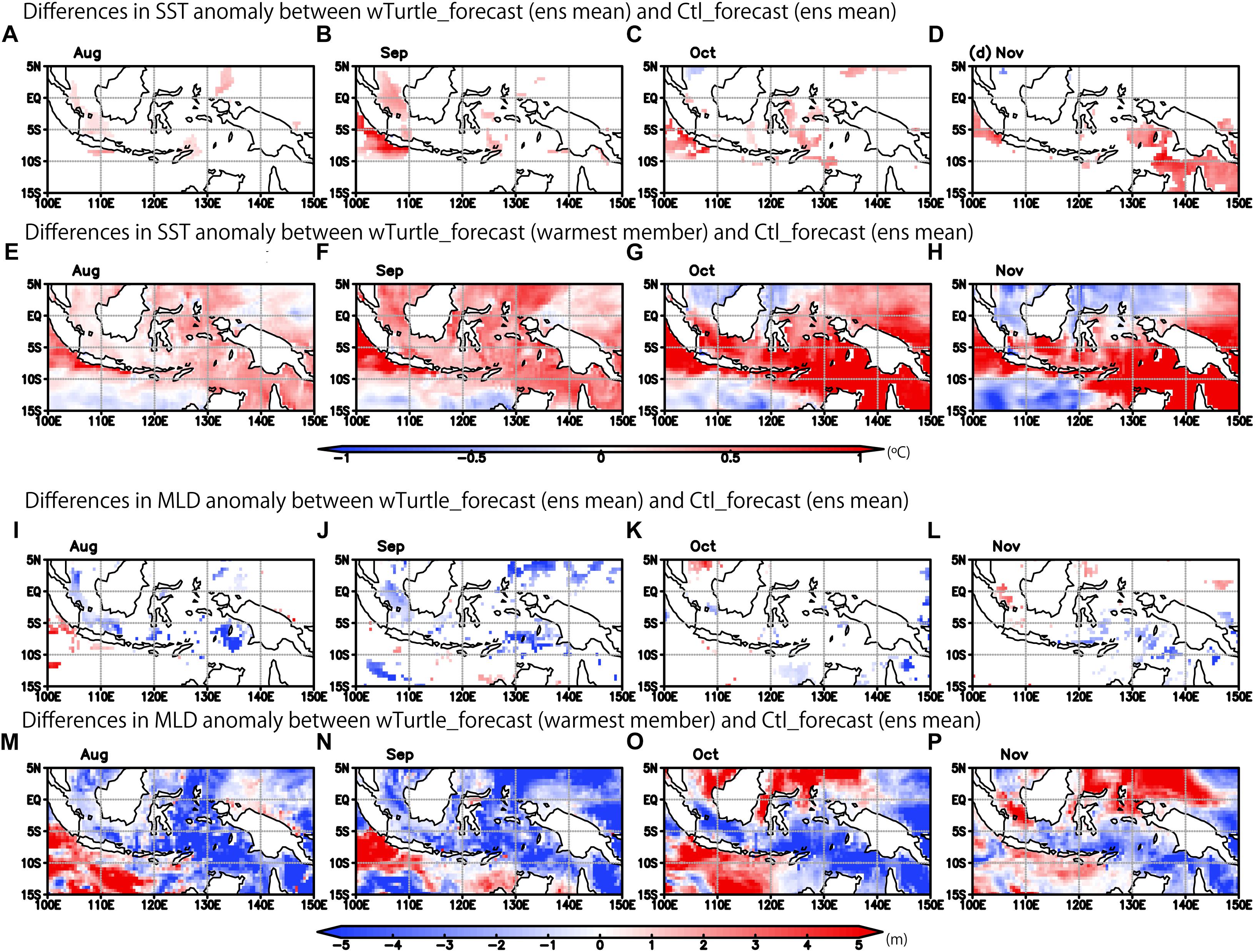
Figure 7. Differences in SST anomalies between the wTurtle_forecast (ensemble mean) and the Ctl_forecast (ensemble mean) issued on August 1, 2017, for (A) August, (B) September, (C) October, and (D) November 2017 (°C). The statistically significant difference beyond the 90% confidence levels on paired t-test are shown by shaded parts. Differences in SST anomalies between the warmest ensemble members of the wTurtle_forecast (shown in Figure 6) and the Ctl_forecast (ensemble mean) issued on August 1, 2017, for (E) August, (F) September, (G) October, and (H) November 2017 (°C). (I–L) The same as (A–D) but for the mixed layer depth (m). (M–P) The same as (E–H) but for the mixed layer depth (m).
What is the importance of the Arafura Sea with regard to the large-scale climate? We calculated the horizontal distribution of the correlation of the SST anomaly in the Arafura Sea and the 2 m air temperature anomaly in the global regions for the October–December averages during 1983–2015 using the observational data and the reanalysis data (figures not shown). It can be seen that the warm SST anomaly in the Arafura Sea was linked with the warm 2 m air temperature anomaly in some parts of the Philippine Sea and Coral Sea. As shown in the partial correlation analysis, this relationship could be robust, even if the influences from large-scale tropical climate variations such as ENSO and the Indian Ocean Dipole were linearly removed (figures not shown). With regard to precipitation, it can be seen that the warm SST anomaly in the Arafura Sea was linked with the wetter-than-normal condition over the Maritime Continent and northern Australia (figures not shown). Although a high correlation does not always show causality, these results may suggest the potential importance of seasonal prediction of the SST anomaly in the Arafura Sea for some parts of the Philippine Sea, Coral Sea, Maritime Continent, and northern Australia. In addition, some previous works already suggested a relationship between the SST anomaly in the Arafura Sea and the larger-scale circulations. Kawamura et al. (2002) showed that the local climate over the Arafura Sea is important for the onset mechanism of the Australian summer monsoon. Indonesian rainfall variability is influenced not only by ENSO but also by local air–sea interactions, including those in the Arafura Sea region (Hendon, 2003; Kubota et al., 2011). Additionally, Zhang et al. (2016) suggested that seasonal prediction of an extratropical climate (e.g., the East Asian climate) is partly dependent upon the prediction skill for rainfall over the Maritime Continent, demonstrating the roles of remote and local forcing in the variation and prediction of the regional rainfall there. Since the complicated relationships between the local climate over the Arafura Sea and the larger-scale circulations are not yet well understood and are still difficult for climate models to capture (e.g., Schiemann et al., 2014), higher resolution and better representation of the air–sea coupling process in climate models may be needed to more clearly display the potential importance of the local climate over the Arafura Sea in the large-scale climate.
The quality of the temperature profile data captured by sea turtles was discussed and compared to the other in situ data of the Global Temperature and Salinity Profile Program (GTSPP) by Miyazawa et al. (2019). Although it was difficult to match up the sampling positions and times of the two data sources exactly, the aforementioned study checked the dependency of their mutual consistency as a function of the horizontal distance between them. A higher correlation and smaller root mean square difference between them suggested a sufficient reliability of the turtle measurements compared to the usual GTSPP data. McMahon et al. (2005) also compared the thermistor of SRDLs with that of the Argo floats. The Argo floats have much better temperature sensors than SRDLs; the accuracy of SRDL temperature data is 0.1°C, which is at least one order of magnitude lower than that of the Argo floats. Note that other manufacturers are also building loggers with various degrees of accuracy. However, some marine animal-borne instruments with SRDLs could potentially be beneficial in areas wherein little or no other subsurface ocean data are currently available. Further studies on quality assessment may be required before compiling the temperature measurements from sea turtles into the existing Global Ocean Observing System in order to assimilate these data into operational short-range prediction systems as well.
Data Availability Statement
The datasets generated for this study are available on request to the corresponding author.
Ethics Statement
The fieldwork was conducted with permission from Dinas Perikanan, Pemerinta Kabupaten Tambrauw (19630701 199403 1 009). All procedures performed in this study involving animals were approved by the Animal Experimental Committee of the University of Tokyo and conducted in accordance with the Guidelines for the Care of Experimental Animals (A17-13).
Author Contributions
TD performed the seasonal prediction system on a basis of the ocean-atmosphere coupled model, and analyzed the observation data and model prediction outputs. AS mainly contributed to the assimilation of temperature measurement from sea turtle into the seasonal prediction system. TF, HS, and KS mainly contributed to the temperature measurements from sea turtles. All authors contributed to designing the research, interpreting results, and writing the manuscript.
Funding
This work was supported by a research project entitled with “Cyber Ocean: Next generation navigation system on the sea” funded by the CREST program (JPMJCR1685) of Japan Science and Technology Agency and JSPS KAKENHI Grant Number 16K17810.
Conflict of Interest
The authors declare that the research was conducted in the absence of any commercial or financial relationships that could be construed as a potential conflict of interest.
Acknowledgments
We would like to thank Satomi Kondo, Gunawan Hadiko, and Jamal for assisting with the fieldwork. Dr. Takuji Noda of Bio-Logging Solutions helped us greatly with the data format of the temperature measurements taken by the sea turtles. The SINTEX-F seasonal climate prediction system was run by the Earth Simulator at JAMSTEC (see http://www.jamstec.go.jp/es/en/index.html for the system overview). We are grateful to Drs. Wataru Sasaki, Jing-Jia Luo, Sebastian Masson, Antonio Navarra, Swadhin Behera, Toshio Yamagata, and our European colleagues of INGV/CMCC, L’OCEAN, and MPI for their valuable contributions in developing the prototypes of the systems. We would also like to thank Drs. Yasumasa Miyazawa and Akira Kuwano-Yosida for their helpful comments and suggestions. The GMT and GrADS software were used to create the figures and maps.
References
Adler, R. F., Huffman, G. J., Chang, A., Ferraro, R., Xie, P., Janowiak, J., et al. (2003). The Version 2 Global Precipitation Climatology Project (GPCP) monthly precipitation analysis (1979-Present). J. Hydrometeor. 4, 1147–1167. doi: 10.1175/1525-7541(2003)004<1147:tvgpcp>2.0.co;2
Ando, K., Kuroda, Y., Fujii, Y., Fukuda, T., Hasegawa, T., and Horii, T. (2017). Fifteen years progress of the TRITON array in the Western Pacific and Eastern Indian Oceans. J. Oceanogr. 73, 403–426. doi: 10.1007/s10872-017-0414-4
Behera, S., and Yamagata, T. (2003). Influence of the Indian Ocean dipole on the Southern oscillation. J. Meteorol. Soc. Japan Ser. II 81, 169–177. doi: 10.2151/jmsj.81.169
Bjerknes, J. (1969). Atmospheric teleconnections from the equatorial Pacific. Mon. Weather Rev. 97, 163–172. doi: 10.1175/1520-0493(1969)097<0163:atftep>2.3.co;2
Boehme, L., Lovell, P., Biuw, M., Roquet, F., Nicholson, J., Thorpe, S. E., et al. (2009). Technical note: animal-borne CTD-Satellite relay data loggers for real-time oceanographic data collection. Ocean Sci. 5, 685–695. doi: 10.5194/os-5-685-2009
Bourles, B., Lumpkin, R., Mcphaden, M. J., Fabrice, H., Paulo, N., Edmo, C., et al. (2008). THE PIRATA PROGRAM history, accomplishments, and future directions. Bull. Am. Meteorol. Soc. 89, 1111–1125. doi: 10.1175/2008BAMS2462.1
Carse, F., Martin, M. J., Sellar, A., and Blockley, E. W. (2015). Impact of assimilating temperature and salinity measurements by animal-borne sensors on FOAM ocean model fields. Q. J. R. Meteorol. Soc. 141, 2934–2943. doi: 10.1002/qj.2613
Doi, T., Behera, S. K., and Yamagata, T. (2016). Improved seasonal prediction using the SINTEX-F2 coupled model. J. Adv. Model. Earth Syst. 8, 1847–1867. doi: 10.1002/2016MS000744
Doi, T., Behera, S. K., and Yamagata, T. (2019). Merits of a 108-member ensemble system in ENSO and IOD predictions. J. Clim. 32, 957–972. doi: 10.1175/jcli-d-18-0193.1
Doi, T., Storto, A., Behera, S. K., Navarra, A., and Yamagata, T. (2017). Improved prediction of the Indian Ocean Dipole Mode by use of subsurface ocean observations. J. Clim. 30, 7953–7970. doi: 10.1175/JCLI-D-16-0915.1
Doi, T., Tozuka, T., and Yamagata, T. (2010). The Atlantic meridional mode and its coupled variability with the Guinea dome. J. Clim. 23, 455–475. doi: 10.1175/2009JCLI3198.1
Foltz, G. R., Brandt, P., Richter, I., Rodríguez-Fonseca, B., Hernandez, F., Dengler, M., et al. (2019). The tropical atlantic observing system. Front. Mar. Sci. 6:206. doi: 10.3389/fmars.2019.00206
Fujii, Y., Cummings, J., Xue, Y., Schiller, A., Lee, T., Balmaseda, M. A., et al. (2015). Evaluation of the Tropical Pacific Observing System from the ocean data assimilation perspective. Q. J. R. Meteorol. Soc. 141, 2481–2496. doi: 10.1002/qj.2579
Good, S. A., Martin, M. J., and Rayner, N. A. (2013). EN4: quality controlled ocean temperature and salinity profiles and monthly objective analyses with uncertainty estimates. J. Geophys. Res. Ocean 118, 6704–6716. doi: 10.1002/2013JC009067
Halkides, D., Lee, T., and Kida, S. (2011). Mechanisms controlling the seasonal mixed-layer temperature and salinity of the Indonesian seas. Ocean Dyn. 61, 481–495. doi: 10.1007/s10236-010-0374-3
Harcourt, R., Sequeira, A. M. M., Zhang, X., Roquet, F., Komatsu, K., Heupel, M., et al. (2019). Animal-borne telemetry: an integral component of the ocean observing toolkit. Front. Mar. Sci. 6:326. doi: 10.3389/fmars.2019.00326
Hashizume, M., Terao, T., and Minakawa, N. (2009). The Indian Ocean Dipole and malaria risk in the highlands of western Kenya. Proc. Natl. Acad. Sci. U.S.A. 106, 1857–1862. doi: 10.1073/pnas.0806544106
Hendon, H. H. (2003). Indonesian rainfall variability: Impacts of ENSO and local air-sea interaction. J. Clim. 16, 1775–1790. doi: 10.1175/1520-0442(2003)016<1775:irvioe>2.0.co;2
Hermes, J. C., Masumoto, Y., Beal, L. M., Roxy, M. K., Vialard, J., Andres, M., et al. (2019). A sustained ocean observing system in the indian ocean for climate related scientific knowledge and societal needs. Front. Mar. Sci. 6:355. doi: 10.3389/fmars.2019.00355
Hoerling, M. P., and Kumar, A. (2001). Atmospheric response patterns associated with tropical forcing. J. Clim. 15, 2184–2203. doi: 10.1175/1520-0442(2002)015<2184:arpawt>2.0.co;2
Hosoda, S., Ohira, T., and Nakamura, T. (2008). A monthly mean dataset of global oceanic temperature and salinity derived from Argo float observations. JAMSTEC Rep. Res. Dev 8, 47–59. doi: 10.5918/jamstecr.8.47
Hughes, T. P., Kerry, J. T., Álvarez-noriega, M., Álvarez-romero, J. G., Anderson, K. D., Baird, A. H., et al. (2017). Global warming and recurrent mass bleaching of corals. Nature 543:21707. doi: 10.1038/nature21707
Iizumi, T., Sakuma, H., Yokozawa, M., Luo, J. J., Challinor, A. J., Brown, M. E., et al. (2013). Prediction of seasonal climate-induced variations in global food production. Nat. Clim. Chang. 3, 904–908. doi: 10.1038/nclimate1945
Ikeda, T., Behera, S., Morioka, Y., Minakawa, N., Hashizume, M., Tsuzuki, A., et al. (2017). Seasonally lagged effects of climatic factors on malaria incidence in South Africa. Sci. Rep. 7:2458. doi: 10.1038/s41598-017-02680-6
Kalnay, E., Kanamitsu, M., Kistler, R., Collins, W., Deaven, D., Gandin, L., et al. (1996). The NCEP/NCAR 40-year reanalysis project. Bull. Am. Meteorol. Soc. 77, 437–471.
Kataoka, T., Tozuka, T., and Yamagata, T. (2017). Generation and decay mechanisms of Ningaloo Niño / Niña. J. Geophical Res. 122, 8913–8932. doi: 10.1002/2017JC012966
Kawamura, R., Fukuta, Y., Ueda, H., Matsuura, T., and Iizuka, S. (2002). A mechanism of the onset of the Australian summer monsoon. J. Geophys. Res. Atmos. 107, 1–15.
Kosaka, Y., Xie, S.-P., Lau, N.-C., and Vecchi, G. A. (2013). Origin of seasonal predictability for summer climate over the Northwestern Pacific. Proc. Natl. Acad. Sci. U.S.A. 110, 7574–7579. doi: 10.1073/pnas.1215582110
Kubota, H., Shirooka, R., Jun-Ichi, H., and Syamsudin, F. (2011). Interannual rainfall variability over the eastern maritime continent. J. Meteorol. Soc. Japan 89, 111–112. doi: 10.2151/jmsj.2011-A07
Luo, J. J., Masson, S., Behera, S., Shingu, S., and Yamagata, T. (2005). Seasonal climate predictability in a coupled OAGCM using a different approach for ensemble forecasts. J. Clim. 18, 4474–4497. doi: 10.1175/JCLI3526.1
Mallett, H. K. W., Boehme, L., Fedak, M., Heywood, K. J., Stevens, D. P., and Roquet, F. (2018). Variation in the distribution and properties of Circumpolar deep water in the Eastern Amundsen Sea, on seasonal timescales, using seal-borne tags. Geophys. Res. Lett. 45, 4982–4490. doi: 10.1029/2018GL077430
Masson, S., Terray, P., Madec, G., Luo, J. J., Yamagata, T., and Takahashi, K. (2012). Impact of intra-daily SST variability on ENSO characteristics in a coupled model. Clim. Dyn. 39, 681–707. doi: 10.1007/s00382-011-1247-2
McMahon, C. R., Autret, E., Houghton, J. D., Lovell, P., Myers, A. E., and Hays, G. C. (2005). Animal-borne sensors successfully capture the real-time thermal properties of ocean basins. Limnol Ocean. Meth. 3, 392–398. doi: 10.4319/lom.2005.3.392
Mcphaden, M. J., Busalacchi, J., Cheney, R., Gage, S., Halpern, D., Ji, M., et al. (1998). The Tropical Ocean-Global Atmosphere observing system: a decade of progress. J. Geophys. Res. Ocean. 103, 14169–14240. doi: 10.1029/97jc02906
McPhaden, M. J., Meyers, G., Ando, K., Masumoto, Y., Murty, V. S. N., Ravichandran, M., et al. (2008). The Research Moored Array for African- Asian-Australian Monsoon Analysis and Prediction. Bull. Am. Meteorol. Soc. 90, 459–480. doi: 10.1175/2008BAMS2608.1
Miyazawa, Y., Guo, X., Varlamov, S. M., Miyama, T., and Yoda, K. (2015). Assimilation of the seabird and ship drift data in the north-eastern sea of Japan into an operational ocean nowcast / forecast system. Sci. Rep. 5:17672. doi: 10.1038/srep17672
Miyazawa, Y., Kuwano-Yoshida, A., Doi, T., Nishikawa, H., Narazaki, T., Fukuoka, T., et al. (2019). Temperature profiling measurements by sea turtles improve ocean state estimation in the Kuroshio-Oyashio Confluence region. Ocean Dyn. 69, 267–282. doi: 10.1007/s10236-018-1238-5
Morioka, Y., Doi, T., and Behera, S. K. (2018a). Decadal climate predictability in the southern Indian Ocean captured by SINTEX-F using a simple SST-nudging scheme. Sci. Rep. 8, 1–10. doi: 10.1038/s41598-018-19349-3
Morioka, Y., Doi, T., Storto, A., Masina, S., and Behera, S. K. (2018b). Role of subsurface ocean in decadal climate predictability over the South Atlantic. Sci. Rep. 8, 1–12. doi: 10.1038/s41598-018-26899-z
Morioka, Y., Tozuka, T., and Yamagata, T. (2011). On the Growth and Decay of the Subtropical Dipole Mode in the South Atlantic. J. Clim. 24, 5538–5554. doi: 10.1175/2011JCLI4010.1
Narazaki, T., Sato, K., and Miyazaki, N. (2015). Summer migration to temperate foraging habitats and active winter diving of juvenile loggerhead turtles Caretta caretta in the western North Pacific. Mar. Biol. 162, 1251–1263. doi: 10.1007/s00227-015-2666-0
Neale, R., and Slingo, J. (2003). The maritime continent and its role in the Global Climate: a GCM study. J. Clim. 16, 834–848. doi: 10.1175/1520-0442(2003)016<0834:tmcair>2.0.co;2
Oettli, P., Behera, S. K., and Yamagata, T. (2018). Climate based predictability of oil palm tree yield in Malaysia. Sci. Rep. 8:2271. doi: 10.1038/s41598-018-20298-0
Ogata, T., Doi, T., Morioka, Y., and Behera, S. (2019). Mid-latitude source of the ENSO-spread in SINTEX-F ensemble predictions. Clim. Dyn. 52, 2613–2630. doi: 10.1007/s00382-018-4280-6
Oke, P. R., and Schiller, A. (2007). Impact of Argo, SST, and altimeter data on an eddy-resolving ocean reanalysis. Geophys. Res. Lett. 34, 1–7. doi: 10.1029/2007GL031549
Patel, S. H., Barco, S. G., Crowe, L. M., Manning, J. P., Matzen, E., Smolowitz, R. J., et al. (2018). Estuarine, Coastal and Shelf Science Loggerhead turtles are good ocean-observers in strati fi ed mid-latitude regions. Estuar. Coast. Shelf Sci. 213, 128–136. doi: 10.1016/j.ecss.2018.08.019
Polovina, J. J., Balazs, G. H., Howell, E. A., Parker, D. M., Seki, M. P., and Dutton, P. H. (2004). Forage and migration habitat of loggerhead (Caretta caretta) and olive ridley (Lepidochelys olivacea) sea turtles in the central North Pacific Ocean. Fish. Oceanogr. 13, 36–51. doi: 10.1046/j.1365-2419.2003.00270.x
Polovina, J. J., Howell, E., Parker, D. M., and Balazs, G. H. (2003). Dive-depth distribution of loggerhead (Carretta carretta) and olive ridley (Lepidochelys olivacea) sea turtles in the central North Pacific: might deep longline sets catch fewer turtles? Fish. Bull. 101, 189–193.
Ramage, C. S. (1968). ROLE OF A TROPICAL “MARITIME CONTINENT” IN THE ATMOSPHERIC CIRCULATION. Mon. Wea. Rev. 96, 365–370. doi: 10.1016/j.envres.2015.07.017
Reynolds, R. W., Rayner, N. A., Smith, T. M., Stokes, D. C., and Wang, W. (2002). An improved in situ and satellite SST analysis for climate. J. Clim. 15, 1609–1625. doi: 10.1175/1520-0442(2002)015<1609:aiisas>2.0.co;2
Reynolds, R. W., Smith, T. M., Liu, C., Chelton, D. B., Casey, K. S., and Schlax, M. G. (2007). Daily high-resolution-blended analyses for sea surface temperature. J. Clim. 20, 5473–5496. doi: 10.1175/2007JCLI1824.1
Roquet, F., Wunsch, C., Forget, G., Heimbach, P., Guinet, C., Reverdin, G., et al. (2013). Estimates of the Southern Ocean general circulation improved by animal-borne instruments. Geophys. Res. Lett. 40, 6176–6180. doi: 10.1002/2013GL058304
Saji, N. H., Goswami, B. N., Vinayachandran, P. N., and Yamagata, T. (1999). A dipole mode in the tropical Indian Ocean. Nature 401, 360–363. doi: 10.1038/43854
Saji, N. H., and Yamagata, T. (2003). Possible impacts of Indian Ocean Dipole mode events on global climate. Clim. Res. 25, 151–169. doi: 10.3354/cr025151
Sasaki, W., Richards, K. J., and Luo, J. J. (2012). Role of vertical mixing originating from small vertical scale structures above and within the equatorial thermocline in an OGCM. Ocean Model. 5, 29–42. doi: 10.1016/j.ocemod.2012.09.002
Sasaki, W., Richards, K. J., and Luo, J. J. (2013). Impact of vertical mixing induced by small vertical scale structures above and within the equatorial thermocline on the tropical Pacific in a CGCM. Clim. Dyn. 41, 443–453. doi: 10.1007/s00382-012-1593-8
Schiemann, R., Demory, M. E., Mizielinski, M. S., Roberts, M. J., Shaffrey, L. C., Strachan, J., et al. (2014). The sensitivity of the tropical circulation and Maritime Continent precipitation to climate model resolution. Clim. Dyn. 42, 2455–2468. doi: 10.1007/s00382-013-1997-0
Shukla, J., Anderson, J., Baumhefner, D., Brankovic, C., Chang, Y., Kalnay, E., et al. (2000). Dynamical Seasonal Prediction. Bull. Am. Meteorol. Soc. 81, 2593–2606.
Siegelman, L., Roquet, F., Mensah, V., Rivière, P., Pauthenet, E., Picard, B., et al. (2019). Correction and accuracy of high- and low-resolution CTD data from animal-borne instruments. J. Atmos. Ocean. Technol. 36, 745–760. doi: 10.1175/JTECH-D-18-0170.1
Smith, N., Kessler, W. S., Cravatte, S., Sprintall, J., Wijffels, S., Cronin, M. F., et al. (2019). Tropical pacific observing system. Front. Mar. Sci. 6:31. doi: 10.3389/fmars.2019.00031
Storto, A. (2016). Variational quality control of hydrographic profile data with non-Gaussian errors for global ocean variational data assimilation systems. Ocean Model. 104, 226–241. doi: 10.1016/j.ocemod.2016.06.011
Storto, A., Dobricic, S., Masina, S., Di Pietro, P., Storto, A., Dobricic, S., et al. (2011). Assimilating Along-Track Altimetric Observations through Local Hydrostatic Adjustment in a Global Ocean Variational Assimilation System. Mon. Weather Rev. 139, 738–754. doi: 10.1175/2010MWR3350.1
Storto, A., Masina, S., and Dobricic, S. (2014). Estimation and impact of nonuniform horizontal correlation length scales for global ocean physical analyses. J. Atmos. Ocean. Technol. 31, 2330–2349. doi: 10.1175/JTECH-D-14-00042.1
Takaya, A., Morioka, Y., and Behera, S. K. (2014). Role of climate variability in the heatstroke death rates of Kanto region in Japan. Sci. Rep. 4:5655. doi: 10.1038/srep05655
Tozuka, T., Ohishi, S., and Cronin, M. F. (2018). A metric for surface heat flux effect on horizontal sea surface temperature gradients. Clim. Dyn. 51, 547–561. doi: 10.1007/s00382-017-3940-2
Vidard, A., Anderson, D., and Balmaseda, M. (2007). Impact of Ocean Observation Systems on Ocean Analysis and Seasonal Forecasts. Mon. Weather Rev. 135, 409–429. doi: 10.1175/MWR3310.1
Wang, B., Wu, R., and Fu, J. X. (2000). Pacific – East Asian teleconnection: how does ENSO affect east Asian climate? J. Clim. 13, 1517–1536. doi: 10.1175/1520-0442(2000)013<1517:peathd>2.0.co;2
Yuan, C., and Yamagata, T. (2015). Impacts of IOD, ENSO and ENSO Modoki on the Australian Winter Wheat Yields in Recent Decades. Sci. Rep. 5:17252. doi: 10.1038/srep17252
Keywords: seasonal prediction, marine animal-borne instruments, sea turtles, ocean observing system experiment, biologging
Citation: Doi T, Storto A, Fukuoka T, Suganuma H and Sato K (2019) Impacts of Temperature Measurements From Sea Turtles on Seasonal Prediction Around the Arafura Sea. Front. Mar. Sci. 6:719. doi: 10.3389/fmars.2019.00719
Received: 23 July 2019; Accepted: 06 November 2019;
Published: 13 December 2019.
Edited by:
Gilles Reverdin, Centre National de la Recherche Scientifique (CNRS), FranceReviewed by:
Sophie E. Cravatte, Institut de Recherche pour le Développement (IRD), FranceFabien Roquet, University of Gothenburg, Sweden
Copyright © 2019 Doi, Storto, Fukuoka, Suganuma and Sato. This is an open-access article distributed under the terms of the Creative Commons Attribution License (CC BY). The use, distribution or reproduction in other forums is permitted, provided the original author(s) and the copyright owner(s) are credited and that the original publication in this journal is cited, in accordance with accepted academic practice. No use, distribution or reproduction is permitted which does not comply with these terms.
*Correspondence: Takeshi Doi, dGFrZXNoaS5kb2lAamFtc3RlYy5nby5qcA==
†ORCID: Takeshi Doi, orcid.org/0000-0002-7342-9145