- 1AZTI, Pasaia, Spain
- 2AZTI, Sukarrieta, Spain
- 3International Seafood Sustainability Foundation (ISSF), Washington, DC, United States
- 4Indian Ocean Tuna Commission, Victoria, Seychelles
- 5Centre for Environmental Policy, Imperial College London, London, United Kingdom
Fisheries are constrained by ecosystem productivity and management effectiveness. Climate change is already producing impacts on marine ecosystems through overall changes in habitats, productivity and increased variability of environmental conditions. The way how these will affect fisheries is under debate and, also there is uncertainty on the best course of action to mitigate climate change impacts on fisheries. Harvest control rules are sets of pre-agreed rules that can be used to determine catch limits periodically and describe how harvest is automatically controlled by management in relation to the state of some indicator of stock status. In 2017, the International Commission for the Conservation of Atlantic Tunas adopted a harvest control rule for North Atlantic albacore. This harvest control rule was evaluated using Management Strategy Evaluation against the main sources of uncertainty inherent to this fishery. Here, we used the same framework to evaluate the robustness of the adopted rule against two types of potential climate change impacts on North Atlantic albacore dynamics. First, we evaluated how the control rule would perform in the event of overall changes in productivity in the North Atlantic and second, against increases in climate driven recruitment variability. Overall, our results suggest that the adopted harvest control rule is robust to these climate driven impacts and also suggests bounds at which the current management framework would be vulnerable to climate change. Throughout the manuscript we also discuss the potential of harvest control rules and harvest strategies to adapt fisheries management to a changing environment. Our main conclusion is that despite the many uncertainties on climate impacts on fisheries, efficient fisheries management and HCRs will be critical to ensure the sustainability of fisheries in the future.
Introduction
Marine fisheries are an important source of food and livelihood worldwide (Garcia and Rosenberg, 2010; Rice and Garcia, 2011). Traditionally, fisheries management has aimed at maintaining fish stocks at levels that can produce their Maximum Sustainable Yield (MSY), i.e., the exploitation rate where the response of stocks to fishing through individual growth and recruitment operates at its maximum capacity (Merino et al., 2014). In a deterministic sense, at this level, average fish biomass remains stable over time and the amount of fish that can be sustainably harvested is maximized (Schaefer, 1954). In reality, marine fisheries are all but stable, they are subject to drivers of change such as environmental variability and shifts, inefficient management and to the many sources of uncertainty inherent to natural systems (Kiureghiana and Ditlevsen, 2009; Fromentin et al., 2014; Strong and Oakley, 2014).
Climate change (CC) has emerged as an important driver of change of marine ecosystems and fisheries. Global warming is modifying oceanic biological response patterns by changing habitats’ physical and chemical properties (Sarmiento et al., 2004), by amplifying environmental fluctuations (Lehodey et al., 2006) and by modifying the overall flux of energy and production through marine ecosystems (Brander, 2007; Blanchard et al., 2012). Climate change influences fishery production through changes in primary production, food web interactions and the life history and distribution of fish (Blanchard et al., 2012). Changes in primary production follow from oceanic physical and chemical environment (Sarmiento et al., 2004), while changes in primary production affect the productivity of the food webs (Brander, 2007; Blanchard et al., 2012). Also, fish are adapted to their environment through life-history strategies or traits, such as their growth and fecundity (Moyle et al., 1986; Vila-Gispert et al., 2005), which are predicted to be affected by climate driven environmental changes (Chavez et al., 2003; Cheung et al., 2012).
The most prominent biological response from fish stocks are changes in distribution (Cheung et al., 2010), phenology (Poloczanska et al., 2013; Asch, 2015; Poloczanska et al., 2016) and productivity (Cheung et al., 2010, 2012; Merino et al., 2012; Free et al., 2019). Overall, global warming is predicted to increase fisheries catch potential in higher latitudes and to decrease in tropical regions due to the poleward shift in the geographical distribution of fish stocks in the Northern Hemisphere (Cheung et al., 2010). Fisheries productivity will also be affected by climate driven changes on key processes such as growth and recruitment. For example, it is expected that lower oxygen and changes in metabolism will produce an overall reduction in fish size (Cheung et al., 2012). Long-term changes in temperature can also affect the early stages’ survival and produce regime shifts in recruitment (Brander, 2007; Shoji et al., 2011). With regards to fish life cycle events, CC is expected to amplify the variability, frequency and intensity of fluctuations, in particular for pelagic stocks (Chavez et al., 2003; Alheit et al., 2009; Barange and Perry, 2009). However, there is still significant uncertainty in projecting marine fisheries under CC (Cheung et al., 2016) and on quantifying the direct impacts of climate on fisheries performance (Brander, 2007).
In this context, there is also considerable uncertainty on the course of action to manage fisheries subject to a changing climate (Arnason, 2006). One way to address uncertainty in fisheries is the application of the principles of the Precautionary Approach, including the adoption of harvest strategies. The Precautionary Approach (PA) aims at improving the management of fish resources by exercising prudent foresight to avoid unacceptable or undesirable situations, taking into account that changes in fisheries systems are not well understood and are only slowly reversible (FAO, 1995). Harvest strategies are the systematic series of human actions undertaken to monitor fish stocks, assess its state, implement scientific advice and make management decisions. A harvest strategy is a pre-agreed set of steps that can specify changes to the total allowable catch (TAC), or any other measure, based on updated monitoring data and methods of analysis. Among others, adopting a harvest strategy requires specifying management objectives, performance indicators, the data and methods of analysis to estimate stock status and a decision rule (harvest control rule, HCR) based on the status of the stock or fishery indicators.
Tunas sustain some of the world’s most valuable fisheries and dominate marine ecosystems worldwide (Juan-Jordá et al., 2011). The International Commission for the Conservation of Atlantic Tunas (ICCAT) is responsible for the sustainable management of Atlantic tunas and has a generic management objective of achieving long-term yields with a high probability of stocks not being overfished (B < BMSY) and no overfishing occurring (F < FMSY) and with a low probability of stocks being outside biological limits. ICCAT’s objective is In line with the United Nations Conference on Straddling Fish Stocks and Highly Migratory Fish Stocks (UN, 1995) that defines the fishing mortality associated to MSY (FMSY) as an upper limit instead of a target, but until 2017, the probability associated to not being overfished and overfishing not occurring was not specified. Note that when a stock is fluctuating around its MSY, the probability of not being overfished is approximately 50%, and the same happens with overfishing occurring. Therefore, a management objective that is in line with international conventions and with the PA should assign a probability higher than 50% for overfishing not occurring and not being overfished. In 2017, ICCAT specified components of a harvest strategy for North Atlantic albacore, including a HCR. This rule was adopted to achieve a management objective of maintaining North Atlantic albacore at biomass (B) levels above, and fishing mortality (F) below, that corresponding to its MSY with a probability higher than 60%, i.e., [p(B > BMSY and F < FMSY) ≥ 60%] (ICCAT, 2017).
In essence, the adopted HCR specifies a maximum fishing mortality that will be used to fix TAC when biomass is estimated to be above BMSY, and a gradual reduction of fishing mortality when biomass is estimated to be below BMSY. The HCR also contemplates a limit reference point that would trigger the automatic closure of the fishery or setting the fishing mortality to a minimum. This HCR has been used to set catch limits for North Atlantic albacore for the period 2018–2020 calculated using the data and methods of analysis used in the latest stock assessment of North Atlantic albacore (ICCAT, 2016c, 2017).
Management Strategy Evaluation (MSE) is considered the most appropriate way to assess the consequences of uncertainty for achieving fisheries management goals (Punt et al., 2014) and it is being used to support the adoption of HCRs and harvest strategies worldwide. The capacity of the HCR adopted for North Atlantic albacore to achieve ICCAT’s management objectives was evaluated against the most important sources of uncertainty inherent to this fishery using MSE (Merino et al., 2017b, c). The characterized uncertainty includes using different data sources, values for key biological parameters such as natural mortality and steepness [which is defined as the fraction of recruitment from an unexploited population obtained when the spawning biomass is at 20% of the unexploited level (Lee et al., 2012)] and fishery dynamics (catchability). When using MSE, uncertainty is generally characterized through the Operating Models (OM). These represent alternatives for the “true” underlying dynamics of the fishery resource and generate data that is used in the Management Procedure (MP) component that describes harvest strategies (the data and methods of analysis to estimate stock status and the HCR) in a numerical simulation framework. The evaluation of North Atlantic albacore HCR includes scores in fisheries performance indicators of sustainability, safety (the probability of the stock remaining above the biomass limit), catch and variability (ICCAT, 2016a; Merino et al., 2017c).
How CC will affect tunas in general, and North Atlantic albacore in particular, is being investigated (Dufour et al., 2010; Dueri et al., 2014; Arrizabalaga et al., 2015; Lehodey et al., 2015; Chust et al., 2019; Errauskin-Extramiana et al., 2019) and given the long life-span of albacore (compared to other tuna stocks) it is crucial to evaluate the combined influences of fishing, environmental variability and CC on this species (Dragon et al., 2015). Here, we build a series of exploratory scenarios to characterize climate impacts on North Atlantic albacore to evaluate if the HCR adopted for this stock would still be an adequate tool for achieving sustainability goals and for maintaining fisheries performance under CC. Future scenarios are alternative images of how the future might unfold and are an appropriate tool for exploring how driving forces may influence the future and assess on the associated uncertainties (IPCC, 2007). Here, we build scenarios from the OM considered for North Atlantic albacore (Merino et al., 2017b) by modifying the albacore growth, recruitment variability and productivity in the MSE simulation framework. The scenarios for these three biological processes include gradual changes from lowest to highest from possible values that will ultimately affect productivity and fisheries performance. The aim of this is to gain confidence in the current management system of North Atlantic albacore and to identify at which levels the sustainability of this stock may be jeopardized.
To sum up, the objective of this work is to evaluate the robustness of the HCR adopted for North Atlantic albacore against a range of exploratory scenarios of climate change impacts on this stock. These include potential changes in fish growth, recruitment productivity and variability.
Materials and Methods
The MSE for North Atlantic Albacore
Management strategy evaluation involves using simulation to compare the relative effectiveness of different combinations of (i) data collection schemes, (ii) methods of analysis, and (iii) subsequent process leading management actions (Punt et al., 2014), i.e., different MPs, for achieving fisheries management objectives. In this document, MSE is used to evaluate if the HCR adopted for North Atlantic albacore is robust to the uncertainties derived from a changing climate. For this, a MSE framework has been developed following a series of guidelines and best practices, including six basic steps (Rademayer et al., 2007; Punt et al., 2014):
Identification of Management Objectives and Performance Statistics
The foundational objective of ICCAT is to maintain populations at levels that can permit the MSY (or above). This is converted into operational objectives relative to stock status, safety, catch and stability through ICCAT’s recommendation 16–06 (ICCAT, 2016b) for North Atlantic albacore: The management objective is to maintain the stock in the green quadrant of the Kobe plot (B > BMSY and F < FMSY) with at least 60% probability and a low probability of being outside biological limits (low probability not specified), while maximizing long-term catch (how much catch is maximum is not specified), and minimizing the inter-annual fluctuations in Total Allowable Catch (TAC) (variability not specified). The use of biological limits is generic in ICCAT recommendations but it refers to values of biomass where the recruitment for future years would be jeopardized. To evaluate how the HCR can help achieving those objectives, we use four performance indicators: Probability of being in the green quadrant, probability of biomass falling below the limit reference point established for this stock (Blim = 0.4 x BMSY), long term catch, and interannual variability. We evaluate these performance metrics for the period 2040–2060. This period was chosen because it is in the temporal range of climate change impact predictions (IPCC, 2007; Cheung et al., 2012; Merino et al., 2012; Barange et al., 2014). Here, we evaluate the capacity of the HCR to achieve management objectives by scoring its performance on four indicators.
Selection of Hypotheses of System Dynamics
MSE requires characterizing the main sources of uncertainty inherent to fisheries. These generally include gaps in biological processes and fishery dynamics. The first are often dealt with hypotheses on input biological parameters to population dynamics’ models; and the second with hypotheses over the available datasets or parts of them. The uncertainties explored in the original North Atlantic albacore MSE (Merino et al., 2017b, c) include hypotheses on the available data series, together with natural mortality, stock recruitment relationship and fishery dynamics scenarios (see Table 1, Past dynamics).
Here, we add three additional hypotheses in relation to the potential responses of the stock to CC: (a) Climate change will affect recruitment variability: An increase in future recruitment variability [from current values (20%) to extreme variability (+200%)], (b) Climate change will affect fish body size: variations in the average body weight [from severe reductions (-30%) to a slight increase (+10%)] in response to sea warming and lower oxygen and, (c) Climate change will produce long term changes in recruitment: a recruitment regime shift [from moderately negative (-20%) to moderately positive (+ 20%)], reflected in the expected recruitment at unfished biomass. These changes are introduced in the simulation by modifying the average weight-per-age, the productivity parameter (generally formulated as α) in the Beverton-Holt stock-recruitment model, and on a recruitment variability coefficient.
Constructing OMs
OMs are representations of the “true” dynamics of the system and may include a set of the most plausible hypotheses or unlikely but not impossible situations (ISSF, 2013). In MSE frameworks the OMs represent the system that will be managed through MPs, i.e., the “true” system that is observed, analyzed and managed through data collection systems, stock assessment and HCRs. The OM built for the North Atlantic albacore are conditioned from the hypotheses and assumptions made in the 2013 assessment using the model Multifan-CL (Kleiber et al., 2012; Kell et al., 2013; Merino et al., 2017b). The different model set-ups were prepared choosing alternative model and data options (see Table 1 and Merino et al., 2017b for a more detailed description of the OMs). The OMs were conditioned using R (Project for Statistical Computing1) functions and libraries from the FLR-project2. The conditioned OMs are objects composed by a single fishery and include parameters (selectivity, growth, natural mortality, stock-recruitment and maturity), time series of catch and biomass (in total and by age) and harvest time series, among other information. Finally, the OMs were projected forward to 2015 with total catch information from 2012–2014, and to 2019 with catch and TAC from 2015–2018.
Overall, there were 132 initial OMs. For this work, we modified the initial set of OMs to represent CC impacts by changing stock recruitment relationships (4 options), variability in the recruitment dynamics (4 options) and changing the age vs body weight curve (4 options), resulting in 1,584 OMs (132 × 12).
Defining MPs
MPs represent how the true dynamics underlying fisheries exploitation are represented through stock assessment and driven by fisheries management. A population-model-based framework within which the data obtained from the fishery are analyzed and the current status and productivity of the fishery are estimated through a stock assessment model (Rademayer et al., 2007). The outputs of this are plugged into a HCR that, provides recommendation for management action. In this study, one observational error model (OEM) generates simulated abundance indices for fitting the biomass dynamic model used to evaluate North Atlantic albacore in 2016, to estimate stock status and productivity. These are used in combination with the HCR adopted in 2017 to determine TAC every 3 years (as happened when the HCR was adopted in ICCAT). The following sections provide a detailed description of the components of the MP:
Observation Error Model
In MSE, the OM is used to simulate resource dynamics in order to evaluate the performance of a MP. Where the MP is the combination of pre-defined data, together with an algorithm to which such data are input to provide a value for a management control measure. To link the OM and the MP it is necessary to develop an OEM to generate fishery-dependent or fishery-independent resource monitoring data. The OEM reflects the uncertainties, between the actual dynamics of the resource and perceptions arising from observations and assumptions by modeling the differences between the measured value of a resource index and the actual value in the OM (Kell and Mosqueira, 2016). A procedure to simulate catch per unit of effort data (CPUE) from the OM and compare the properties of the simulated to those used in the latest assessment of North Atlantic albacore was used (Merino et al., 2017a). This method (eq. 1) is used to generate four abundance indices (Spanish baitboat, China Taipei longline, Japanese longline, and a combined index to simulate Venezuelan and United States longline, which were the indices used in the 2016 assessment of this stock) from the OMs:
Stock Assessment
The indices generated with equation 1 are used to fit the biomass dynamic model mpb, which was used in the 2016 stock assessment of North Atlantic albacore (ICCAT, 2016c; Kell, 2016). The fits are made using the same specifications and modeling choices as in 2016, i.e., CPUE series starting as in the stock assessment (Table 2) and the same starting values used in 2016 with the Fox model (Fox, 1970; Table 3).
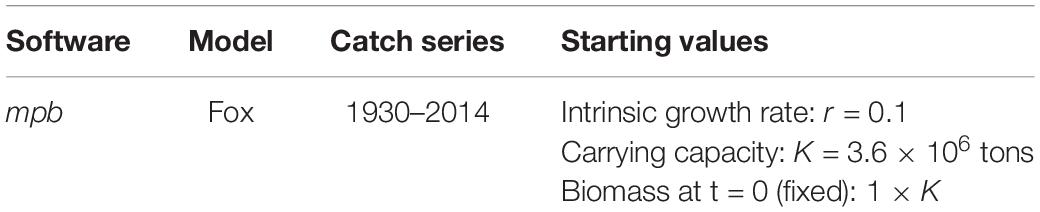
Table 3. Specifications of the biomass dynamic used for the 2016 stock assessment and also used in this MSE.
Harvest Control Rules
Harvest control rules describe how harvest is automatically controlled by management in relation to the state of some indicator of stock status (ISSF, 2013). In the rule adopted for North Atlantic albacore (Figure 1), when the stock level is above the precautionary threshold (Bthresh = BMSY), the fishing mortality applied to the stock will be the target fishing mortality (Ftar = 0.8xFMSY). When the stock falls below Bthresh but above the limit reference point Blim (0.4xBMSY), the fishing mortality will be gradually reduced from Ftar. When the stock falls below Blim, the remedial management action will be determined by Fmin (0.1 x FMSY). As part of HCRs, threshold and limit reference points are intended to restrict harvesting to avoid highly undesirable states of the stock, such as the impairment of the recruitment, from which recovery could be irreversible or slowly reversible.
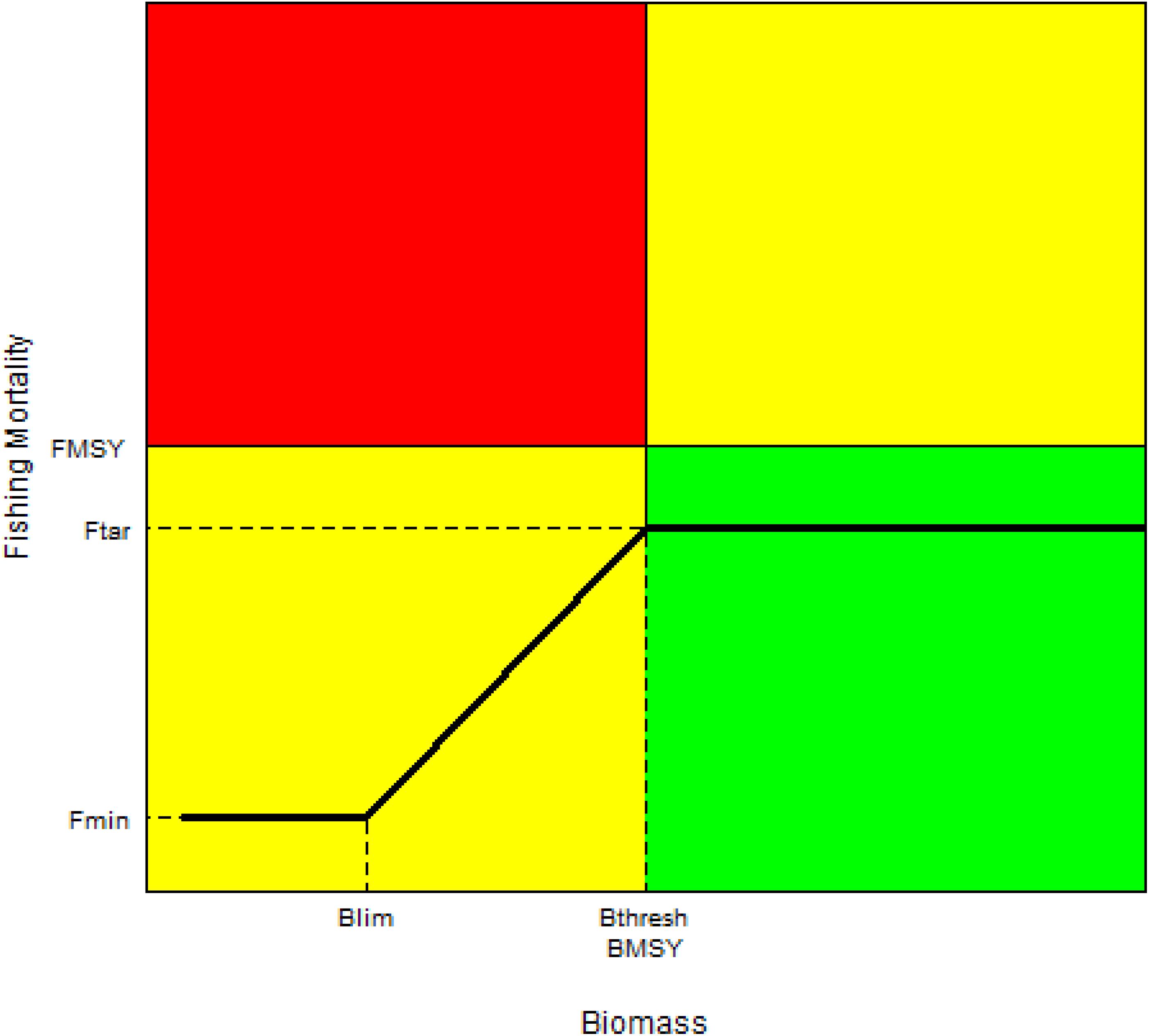
Figure 1. The HCR adopted for North Atlantic albacore displayed in a Kobe plot. The coordinates of the HCR are: Bthresh (= BMSY), Blim (Limit RP), Fmin (F that would be applied if the stock is assessed to be below Blim and, Ftar = target fishing mortality, which will be applied when the stock is assessed to be above Bthresh = BMSY.
In addition, in the spirit of avoiding the adverse effects of potentially inaccurate stock assessments and to increase stability, two control parameters are included in the adopted HCR: First, TAC will not exceed a maximum of 50,000 tons and, second, a maximum 20% of change in TAC will be allowed when the stock is estimated to be above BMSY.
Simulation With Feedback
The OMs and the MPs were linked through specifically tailored R functions and libraries from the FLR project in the MSE framework (Figure 2). The OMs produce series of biomass, catch and fishing mortality, which are measured every 3 years to generate series of catch and abundance indices through the OEM. These are then used to fit the surplus production stock assessment model, mpb. The outputs of this model include estimates of biomass and fishing mortality, reference points (BMSY, FMSY, MSY) and model parameters. These are plugged into the HCR to set catch limits, which are then used to project forward the OMs for three more years. This process is simulated every 3 years for the duration of the simulation, until 2065.
Summary and Interpretation of Performance Statistics
The evaluation of HCRs is completed with the summaries and interpretation of the performance of the OMs. Four groups of indicators relative to stock status, safety, catch and stability are used and compared to the values estimated for the HCR without the CC included.
Results
The CC scenarios formulated here predict changes on North albacore stock’s dynamics, changes including productivity and variability. In the modeling framework used here, stocks’ productivity is specified from stock recruitment relationships, growth equations and mortality parameters. With these, we can calculate stock’s equilibria between spawning stock biomass (SSB), recruitment, fishing mortality and catch (Figure 3). These figures illustrate how CC would modulate stocks’ productivity and abundance and how we have accounted for these changes in our modeling framework. For this, we have generated scenarios of body shrinkage (-30, -20, and-10%) and increase (+ 10%), and recruitment baseline variations from moderate (± 10%) to high (± 20%). We show how CC affected one OM (the Base Case considered in the 2013 stock assessment), but the 132 OMs are modified likewise for the simulations. The productivity of the stock (equilibrium catch) would be more affected by recruitment shifts than by changes in fish body weight (Figure 3).
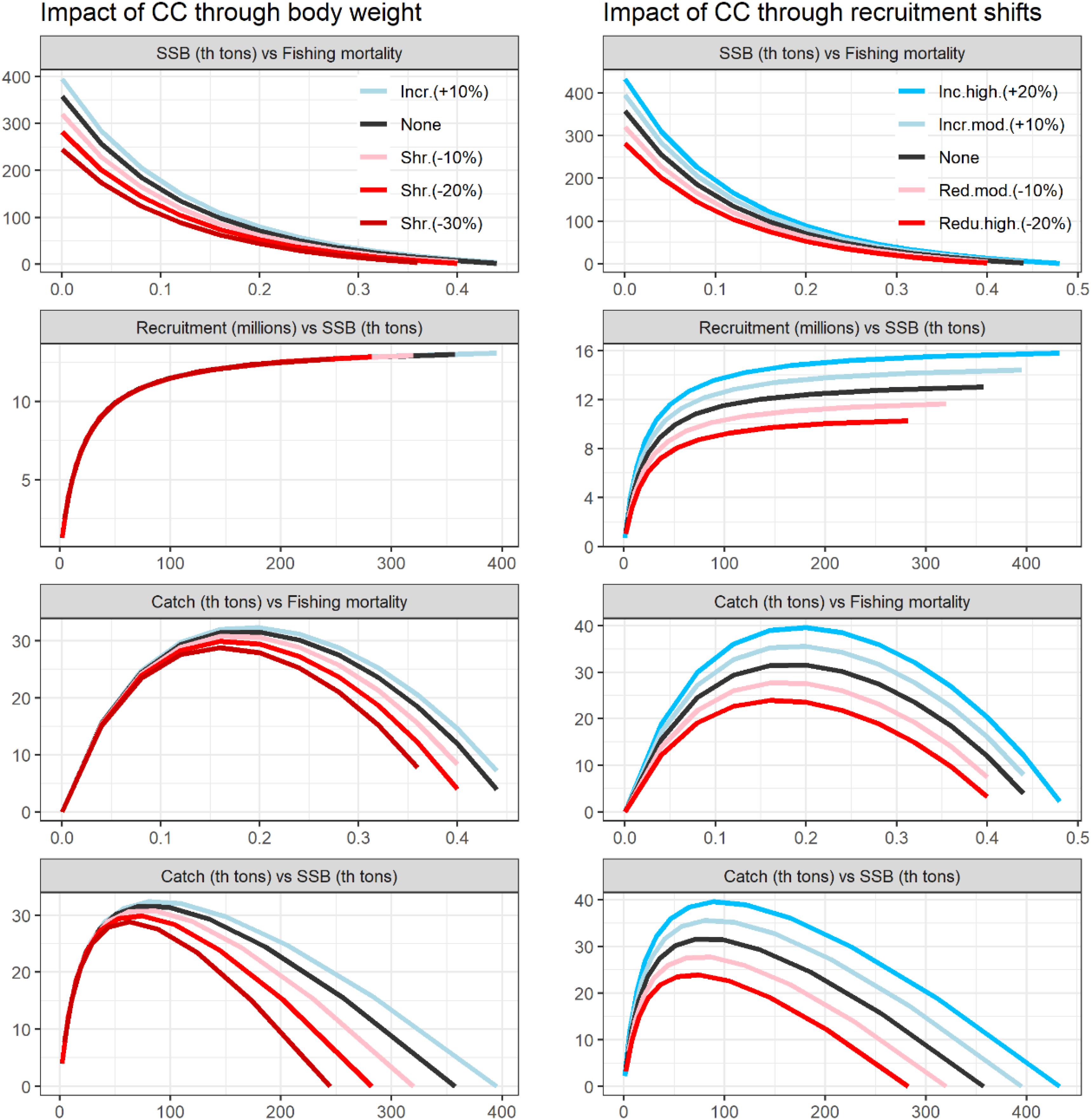
Figure 3. Climate change driven changes in the North Atlantic albacore equilibria based on hypothetical but realistic changes in body weight and recruitment. Colors reflect from large negative effects (red) to positive effects (blue) and the intensity of the color reflects the expected change from large (dark colors) to small changes (light colors).
Figure 4 shows how CC would affect albacore’s productivity and abundance (MSY and unfished biomass, K), in all the OM used in this study. The characterized response of fish stocks to CC were predicted to be larger through changes in recruitment than through changes in body growth (Figure 4). A differential impact of ±10% in body weight would produce a 0.99 thousand tons change on stocks’ productivity (MSY) while the same differential impact on recruitment shift would produce a variation of 4.19 thousand tons. The stock’s response to climate will also modify the niche they could occupy. This is reflected on stocks’ carrying capacity (K) or unfished biomass, the SSB level that would be achieved if fishing mortality was reduced to zero. In this case, the differential impact is very similar when recruitment or body growth are modified: A change of ± 10% produces a change of ± 70 thousandtons in K in both cases. Figure 4 also shows the differences in productivity across OMs for the same differential impact. For example, for the assumption of no climate impact, North Atlantic albacore MSY ranges between 60 to 25 thousand tons, with most OM estimated MSY between 25 and 40 thousand tons.
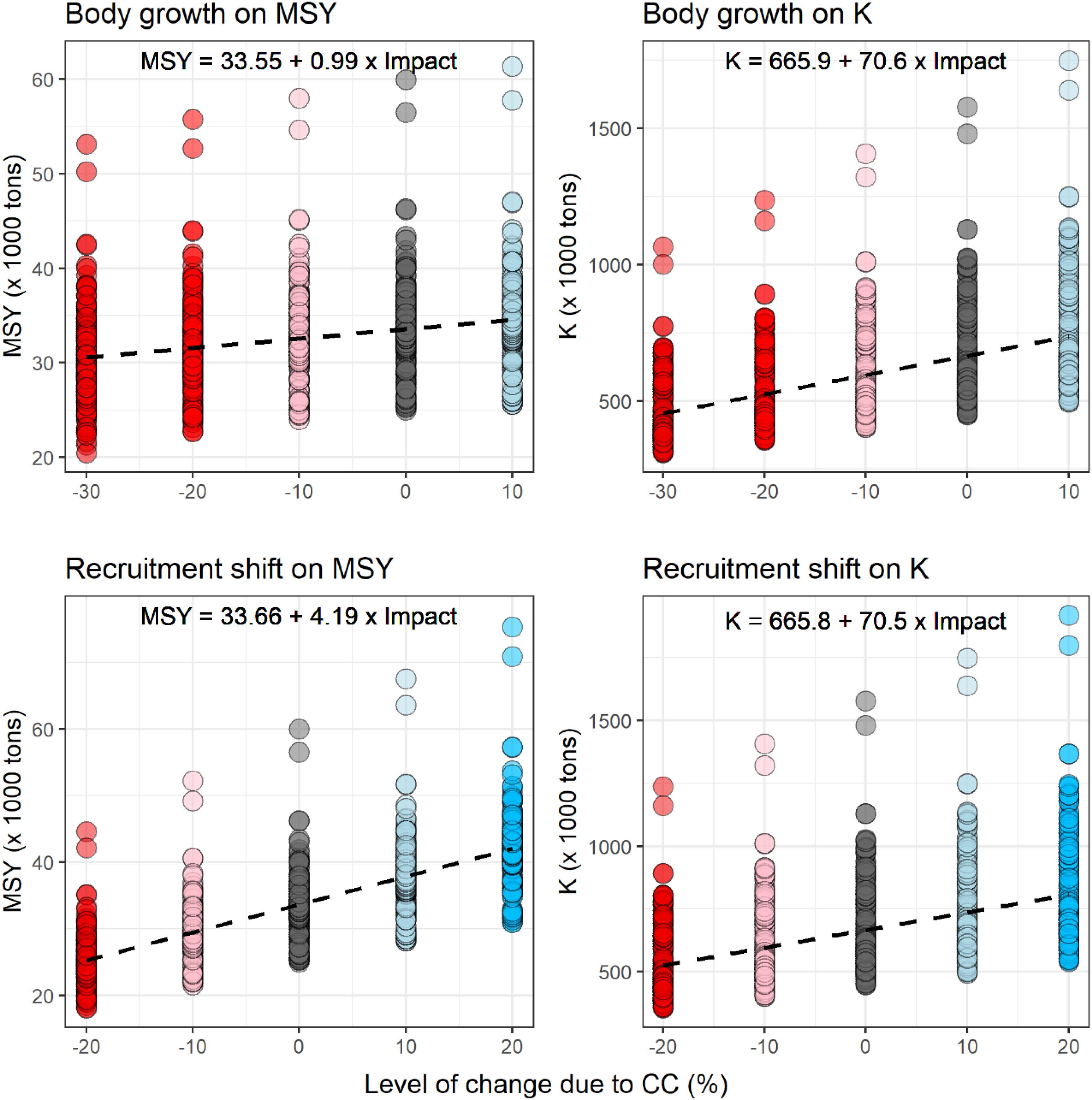
Figure 4. Impacts of CC on stocks’ productivity (MSY) and unfished biomass (K) explored in this study. Each point represents the reference point of one OM for different levels of impact of CC through body growth (top) and recruitment shift (bottom). Colors reflect from large negative effects (red) to positive effects (blue) and the intensity of the color reflects the expected change from large (dark colors) to small changes (light colors).
Figure 5 shows one OM projected forward through the simulation period with no CC impacts. This figure shows how for a single projection the numerical framework produces a range of estimates for each year and that the uncertainty expands through the simulation. In the simulations, variability is caused by the stochastic recruitment model in the OM and to the uncertainty on the CPUE indices used in the MP, generated by the OEM. Thus, the results of each simulation can be expressed as probability distributions similar to what happened when results were aggregated for all OMs for each climate impact.
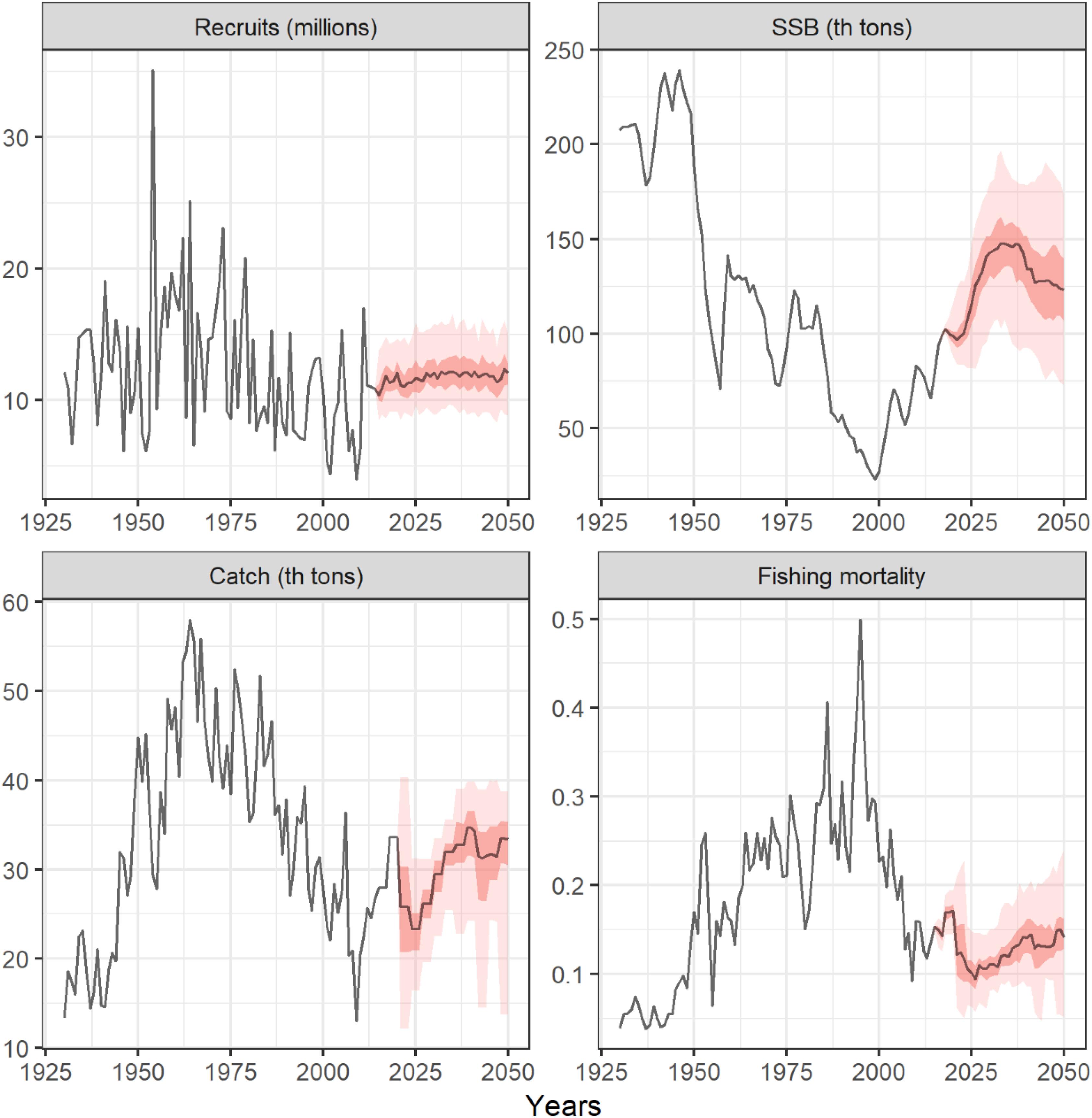
Figure 5. Example of one OM projected forward with the HCR adopted for North Atlantic albacore under no climate change effects. Solid lines are median trajectories and pink and light-pink areas represent the 25–75% and the 10–90% quantiles, respectively, for recruits, spawning stock biomass, catch and fishing mortality.
For all the climate impacts characterized in this study, the HCR adopted for North Atlantic albacore in 2017 would achieve ICCAT’s management objective of maintaining the stock in the green quadrant of the Kobe plot with a probability higher than 60% (Figure 6). With regards to keeping the stock above biological limits, North Atlantic albacore would be above the limit reference point adopted for this stock at least with a probability of 95.7% (Recruitment shift of -10%). With regards to long term-catch, this would range between 20.6 thousand tons and 35.5 thousand tons (similar to recent catches). The stability of catches would be very similar across scenarios with Mean Absolute Proportional (MAP) change in catch ranging from 7.4 to 10.3%.
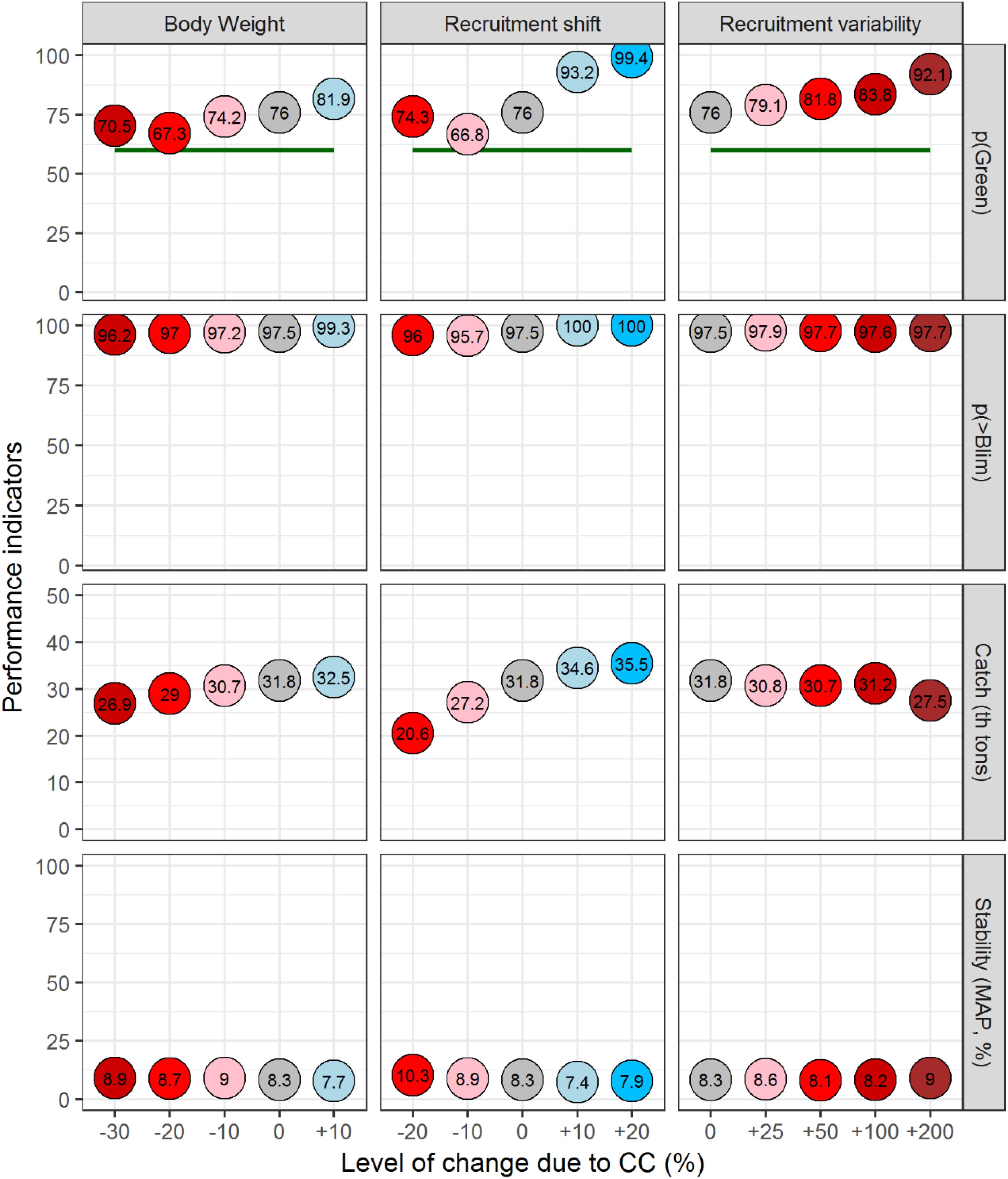
Figure 6. Performance of the North Atlantic albacore fishery represented with median values of indicators of sustainability (pGreen = probability of being in the green quadrant of the Kobe plot), safety (probability of biomass staying above Blim), catch and stability (Mean Annual Proportional change of TAC). A single probabilistic estimate (median) is used to illustrate fisheries performance of sustainability, safety, catch and variability across OMs. The values for each indicator are printed inside the circles. Colors reflect from large negative effects (red) to positive effects (blue) and the intensity of the color reflects the expected change from large (dark colors) to small changes (light colors).
Figure 7 illustrates the heterogeneity of the “true dynamics” considered in the North Atlantic albacore MSE. As the impact goes more negative (i.e., lower body weight, lower recruitment and higher variability), the amount of OMs that deviate from the median value increases. Thus, the uncertainty considered in the initial set of OMs is amplified when stocks are subject to CC impacts and many of the potential “true” trajectories diverge. For example, when the OMs are projected without any climate impact, more than 75% of the OMs reach the management objective of 60% probability of being in the green quadrant of the Kobe plot (gray box above 60%, Figure 7). However, with the negative impacts on productivity due to body weight and recruitment reduction modeled here, nearly 40% of the OMs would not reach the management objective (values of -30, -20, and -10%). In contrast, the increased variability leads to lower average catch and an increased probability of being in the green quadrant of the Kobe plot. This appeared to be because with larger values of variability, the MP stock status estimator is underestimating biomass and therefore, setting TACs at lower levels. When looking at catch and stability indicators a wide range of performances are observed across OMs for the same climate impact, with variability also increasing for the more negative impacts.
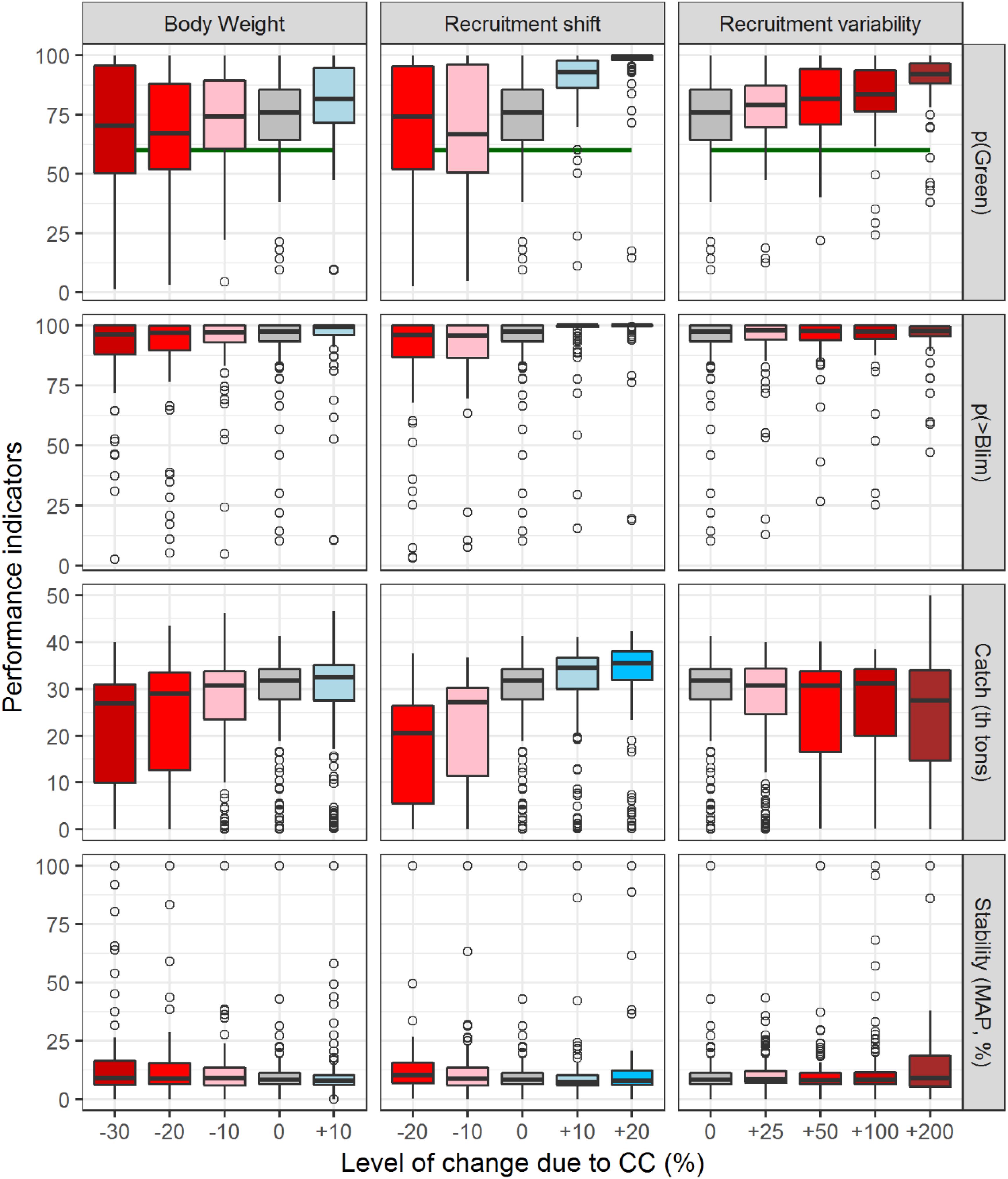
Figure 7. Performance of the North Atlantic albacore fishery represented with box and whisker plots that represents the variety of results achieved across OMs. Boxes represent the 25–75% of OMs and whisks represent 5–95%. Points are outlier OMs. This figure shows indicators of sustainability (pGreen = probability of being in the green quadrant of the Kobe plot), safety (probability of biomass staying above Blim), catch and stability (Mean Annual Proportional change of TAC). Colors reflect from large negative effects (red) to positive effects (blue) and the intensity of the color reflects the expected change from large (dark colors) to small changes (light colors).
Should the impact of CC on North Atlantic albacore productivity be positive [i.e., greater body growth (+ 10%) or increased recruitment (+10%, +20%)], the median catch would likely increase. The HCR adopted for this stock contemplates a maximum TAC of 50 thousand tons and for some OMs, this value is well below their MSY. Therefore, for a number of model runs the increased productivity would not result in a proportional increase in catch and this would lead to higher values of probability of being within the green quadrant, which means that this may be a very precautionary management outcome.
Discussion
Our results demonstrate that the HCR adopted in 2017 for North Atlantic albacore is adequate to achieve ICCAT’s sustainability objectives under CC. Albacore’s biological response to global warming will modulate the maximum productivity and predicted long term catches of North Atlantic fisheries but will also increase their variability. Importantly, CC is predicted to amplify the uncertainties identified for this fishery. These conclusions rely upon the results of an MSE framework specifically tailored to characterize the uncertainties inherent to this fishery and to the predictions of stocks response to global warming. The MSE was built from the most recent stock assessments of North Atlantic albacore and the decision rule adopted ICCAT’s Recommendation 17-04.
This study aims at reducing the uncertainties on the course of action necessary to mitigate the impacts of global environmental change on fisheries (Arnason, 2006) under the principles of the Precautionary Approach (FAO, 1995). This principle recommends to determine the status of fish stocks relative to target and limit reference points, to predict the outcomes of management alternatives for reaching the targets and avoiding the limits and to characterize uncertainty in all cases (Garcia, 1996). A target is a management objective based on a level of biomass that should be achieved and maintained with high probability and a limit indicates the stock size below which the stock is in serious danger of collapse. In the case of North Atlantic albacore, ICCAT adopted the management objective of maintaining the stock in the green quadrant of the Kobe plot with at least 60% probability while maximizing long-term catch and recommended testing alternative target, threshold and limit reference points (ICCAT, 2015). To achieve the management objective, ICCAT adopted reference points and a HCR (ICCAT, 2017) that wwere evaluated against the most important sources of uncertainty identified for this stock (Merino et al., 2017a, b,c). Previous studies by Merino et al. (2017a, b, c) evaluated a range of alternative HCRs against the performance indicators used here to support ICCAT adopting the HCR evaluated here (ICCAT, 2017). This study adds to the previous works by characterizing the uncertainty on CC impacts on North Atlantic fish stocks and by evaluating the robustness of the HCR to keep the stock at sustainable levels under these impacts. Also, this study predicts the performance of North Atlantic albacore fisheries in the long term under CC including its catch and variability in annual catches.
Predicted changes in ocean productivity have been used to estimate proportional changes of fish catch under CC (Lehodey et al., 2006; Cheung et al., 2010, 2012; Blanchard et al., 2012; Arrizabalaga et al., 2015; Errauskin-Extramiana et al., 2019; Plagányi, 2019). However, decreases or increases from current catch levels may depend on management decisions now and in the future, rather than on the fish response to CC alone (Barange, 2019). Therefore, it is of great importance to incorporate fishery management when projecting future fisheries scenarios. Few studies have evaluated the synergic impact of CC and management in marine fisheries (Chavez et al., 2003; Merino et al., 2012; Barange et al., 2014). For example, Barange et al. (2014) conclude that despite the projected human population increase and predicted CC impacts, global demands for fish will be met if strategies for sustainable fisheries are implemented. Merino et al. (2012) suggest that efficient management and technological development in aquaculture, and not climate change, will be the most significant factor in securing sustainable fish production in the future. Here, we first evaluate the direct impact of CC on North Atlantic albacore’s maximum productivity alone using scenarios built from a range of predicted fish responses through body weight and recruitment. Then, we evaluate the ability of the current stock assessment and decision rule for North Atlantic albacore for each fish response scenario by estimating the probability of the stock being in the Green quadrant of the Kobe plot, the risk of falling below safe limits, the long-term catch and its variability. Our results suggest that the capacity of the management scheme adopted by ICCAT is likely to achieve sustainability objectives under CC, representing yet another benefit of well-designed HCRs.
MSE is the appropriate tool to characterize the impacts of uncertainty in fisheries and to evaluate the robustness of management plans toward achieving sustainability (Punt et al., 2014). The MSE used here was peer reviewed and overall, it was found that the MSE framework used in 2017 “appears to be high quality and robust to uncertainty” (Scully, 2018). However, some suggestions for improvement were made and therefore, the code used here is not exactly the same used in 2017. For example, the MP model did not have a mechanism to set TACs if a stock assessment failed to converge in a year of the MSE simulation. We have improved that by setting a rule for maintaining the previous TAC in these cases. In the previous version, the model estimated very low biomass and catch was decreased to a minimum. Also, we don’t only show point estimates with medians across OMs (Figure 6) but we also report on the uncertainty around the medians by using boxplots with outliers (Figure 7). Finally, the OMs used in this study don’t include one group of OM used in the MSE evaluation of North Atlantic albacore, the Multifan-CL scenarios starting in 1950s. This is because when we tried to run the CC scenarios the OMs of this group showed problems in the projections, which will need to be understood. These problems consist on the model crashing during the simulation but the underlying reasons for this will need to be further investigated.
In this study we have characterized CC impacts by modifying the biological properties of the simulated stock of North Atlantic albacore. Starting from a suite of OM developed to characterize current uncertainties (Merino et al., 2017b), we have built a set of new OM by modifying the initial OM with changes in body-growth and recruitment equations. The new set of OM represent a number of potential “true dynamics” for North Atlantic albacore under CC. From the climate impacts characterized in the OM, variations in recruitment levels are the major driver of change on fish stocks’ maximum sustainable catch. The range of impacts characterized here is not arbitrary: Cheung et al. (2012) estimate changes in individual-level maximum body size of fishes for the Atlantic within a range of -30 to 10% with median at ∼-10%. With regards to the impact of environmental regime shifts, Chavez et al. (2003) identify warm and cool periods in the Eastern Pacific that are directly linked with the abundance of anchovy and sardine, which end-up modulating the recruitment of yellowfin tuna (Maunder and Watters, 2001). Using coupled ocean-biogeochemical-population dynamics models, Lehodey et al. (2003), identify climate related changes in Pacific skipjack, yellowfin and South Pacific albacore within a range comparable with the values used here. For North Atlantic albacore in particular, stock-recruitment-environment models have been fitted to identify changes in recruitment (Arregui et al., 2006). The Beverton–Holt model used here was tested with the added factor of the NAO index and yielded estimates of the productivity parameter (α) ranging from ∼0.7 to ∼1.1 × 107, approximately in a range of -20 to +20% of its value without the environmental impact (Arregui et al., 2006). With regards to the recruitment variability scenarios explored here, current state is described with a coefficient of variability (CV) of 20% on recruitment which is similar to other studies (Maunder and Watters, 2001). From this value, we increase variability up to +200%, representing a CV of 0.8. This variability is linked to large-scale atmospheric forcing (Chavez et al., 2003) and could potentially decrease resilience to fishing (Kuparinen et al., 2014). The values used in this work range between the medium to large values considered in a recent study for a variety of Atlantic stocks (Kuparinen et al., 2014). This study is carried out with a model that does not have a spatial component and therefore, the impact of climate change on habitat was directly estimated.
Another salient conclusion of our study is that for increased impacts of CC, the variability of behaviors among OMs increases. For example, fishery’s performance is relatively well centered in the median and boxes for the simulations without climate impacts (Figure 7, gray box). In contrast, the increased negative impacts seem to produce a wider range of results within the set of OMs. This effect is somehow masked by the relatively stable median performances (Figure 6) but corroborates that CC is predicted to increase the uncertainty on the dynamics of fish stocks and natural systems (Cheung et al., 2016) and therefore, further investigations will be necessary to reduce this uncertainty and to improve the understanding the environmental impacts on this stock and in fisheries in general. We also recommend further investigations to explore the combined impacts of biological responses to CC evaluated in this study. It is expected that changes in growth will happen simultaneously to changes in recruitment variability and productivity and this is not evaluated in this study.
Overall, our estimates of CC impacts on fisheries performance were expected: The higher the increase in overall productivity, the higher the catch. However, this study produced results that were initially unexpected: Those scenarios simulating the most negative impacts of CC (-30% of body growth and -20% in recruitment productivity) and increased variability lead to higher values of probability of being in the green quadrant of the Kobe plot (pGreen). This is explained by the capacity of the biomass dynamic model used in the MP. With the set of new OMs and for the more negative impacts of CC the MP has difficulties in fitting the generated data with the range of parameters used and, this lack of convergence leads to lower catches even if the stock is at safe levels. In these cases, the stock biomass continues to increase. With regards to the positive impacts (increased body growth and recruitment), the pGreen achieved may be undesirable and larger sustainable catches would be possible. In the current HCR, there is a limit to the increase of TAC and also a maximum limit of 50 thousand tons, which in the case of potential productivity increases could hamper the performance of fisheries unnecessarily. Therefore, the potential future adoption of a model based MP that includes not only the HCR but also the model and starting values should be flexible to modifying these values to reach a better convergence and a more accurate estimate of stock status. A possible way to do so would be to adopt the data, model and the HCR but leaving some modeling choices open to the working groups, like the bounds for the parameters or their initial values. Also, should there be evidence of environmentally driven changes on the productivity of the stock, there should be flexibility on the inclusion (or not) of parts of long data series. This was somehow done in the last assessment of North Atlantic albacore, when CPUE data prior to 1981 was discarded as potential proxy of stock abundance due to a number of reasons, including the potential change in recruitment regimes throughout the history of the fishery (ICCAT, 2016c; Kell et al., 2016). Finally, the stability clauses of the current HCR should also be eventually adapted if there is evidence of a large-scale environmentally driven change in productivity.
We have only characterized phenological changes through variability in recruitment but fish are subject to many other sources of climate-driven variability (Barange and Perry, 2009). For example, North Atlantic albacore has shown phenological and migratory trends that affect fisheries, specially a northward trend in habitat (Chust et al., 2019). The reasons for these changes are still uncertain and understanding their underlying causes would improve management significantly and contribute to mitigating their impacts. In particular, distribution changes will affect albacore fisheries performance in different latitudes. The nations that have historically targeted albacore (Spain, France) are prone to be negatively affected by geographical changes while nations at higher latitudes could be benefited.
The best option to address the CC challenge is still undefined (Maury et al., 2017), but the inefficient management can only amplify its effects and is considered responsible for the inefficient stewardship of global fisheries (Bank, 2009). A lagged response to natural productivity changes to reduce fishing pressure can amplify fish population collapses (Essington et al., 2015). Globally, effective management of fisheries is still an exception (Mora et al., 2009) and our study adds to previous research (Brander, 2007; Merino et al., 2012; Barange et al., 2014; Barange, 2019) in emphasizing the importance of ensuring efficient management of fisheries in a changing climate and that fisheries management appears to be more important than direct CC impacts predicted in fisheries.
Data Availability Statement
The datasets generated for this study are available on request to the corresponding author.
Author Contributions
GM designed the study, ran the simulations, collected the results, produced the figures, and wrote the manuscript. LK, PD, and GM developed the numerical framework. All other authors contributed to the writing of the manuscript.
Funding
This study was contribution no. 943 from AZTI (Marine Research Division). This work was carried out with the financial support from the Viceconsejería de Agricultura, Pesca y Política Alimentaria of the Basque Government (Spain).
Conflict of Interest
The authors declare that the research was conducted in the absence of any commercial or financial relationships that could be construed as a potential conflict of interest.
Footnotes
References
Alheit, J., Roy, C., and Kifani, S. (2009). “Decada-scale variability in populations,” in Climate Change and Small Pelagic Fish Stocks, eds D. Checkley, J. Alheit, Y. Oozeki, and C. Roy, (Cambridge: Cambridge University Press), 382.
Arnason, R. (2006). “Global warming, small pelagic fisheries and risk,” in Climate Change and the Economics of the World’s Fisheries, eds R. Hanneson, M. Barange, and S. J. Herrick, (Cheltenham: Edwar Elgar Publishing Limited), 1–32.
Arregui, I., Arrizabalaga, H., Kirby, D. S., and Martin-Gonzalez, J. M. (2006). Stock–environment–recruitment models for North Atlantic albacore (Thunnus alalunga). Fish. Oceanogr. 15, 402–412. doi: 10.1111/j.1365-2419.2005.00399.x
Arrizabalaga, H., Dufour, F., Kell, L. T., Merino, G., Ibaibarriaga, L., Chust, G., et al. (2015). Worldwide habitat preferences of tuna species. Deep Sea Res. Part II 113, 102–112. doi: 10.1111/cobi.12268
Asch, R. G. (2015). Climate change and decadal shifts in the phenology of larval fishes in the California current ecosystem. Proc. Natl. Acad. Sci. U.S.A. 112, 4065–4074. doi: 10.1073/pnas.1421946112
Bank, W. (2009). The Sunken Billions: The Economic Justification for Fisheries Reform. Washington, DC: FAO.
Barange, M. (2019). Avoiding misinterpretation of climate change projections of fish catches. ICES J. Mar. Sci. fsz061. doi: 10.1093/icesjms/fsz061
Barange, M., Merino, G., Blanchard, J. L., Scholtens, J., Harle, J., Allison, E. H., et al. (2014). Impacts of climate change on marine ecosystem production in societies dependent on fisheries. Nat. Clim. Change 4, 211–216. doi: 10.1111/gcb.14512
Barange, M., and Perry, R. I. (2009). Physical and Ecological Impacts of Climate Change Relevant to Marine and Inland Capture Fisheries and Aquaculture. Rome: FAO.
Blanchard, J., Jennings, S., Holmes, R., Harle, J., Merino, G., Allen, I., et al. (2012). Potential consequences of climate change on primary production and fish production in 28 large marine ecosystems. Philos. Trans. R. Soc. Lond. Biol. Sci. 367, 2979–2989. doi: 10.1098/rstb.2012.0231
Brander, K. (2007). Global fish production and climate change. Proc. Natl. Acad. Sci. U.S.A. 104, 19709–19714.
Chavez, F. P., Ryan, J., Lluch-Cota, S. E., and Niquen, M. (2003). From anchovies to sardines and back: multidecadal change in the Pacific ocean. Science 299, 217–221. doi: 10.1126/science.1075880
Cheung, W., Lam, V., Sarmiento, J. L., Kearney, K., Watson, R., Zeller, D., et al. (2010). Large-scale redistribution of maximum fisheries catch potential in the global ocean under climate change. Glob. Change Biol. 16, 24–35. doi: 10.1111/j.1365-2486.2009.01995.x
Cheung, W. L., Sarmiento, J. L., Dunne, J. L., Frölicher, T. L., Lam, V. W. Y., Deng Palomares, M. L., et al. (2012). Shrinking of fishes exacerbates impacts of global ocean changes on marine ecosystems. Nat. Clim. Change 3, 254–258. doi: 10.1038/nclimate1691
Cheung, W. W. L., Jones, M., Reygondeau, G., Stock, C. A., Lam, V., and Frölicher, T. L. (2016). Structural uncertainty in projecting global fisheries catches under climate change. Ecol. Model. 325, 57–66. doi: 10.1016/j.ecolmodel.2015.12.018
Chust, G., Goikoetxea, N., Ibaibarriaga, L., Sagarminaga, Y., Arregui, I., Fontán, A., et al. (2019). Earlier migration and distribution changes of albacore in the Northeast Atlantic. Fish. Oceanogr. 28, 505–516. doi: 10.1111/fog.12427
Dragon, A.-C., Senina, I., Titaud, O., Calmettes, B., Conchon, A., Arrizabalaga, H., et al. (2015). An ecosystem-driven model for spatial dynamics and stock assessment of North Atlantic albacore. Can. J. Fish. Aquat. Sci. 72, 1–15.
Dueri, S., Bopp, L., and Maury, O. (2014). Projecting the impacts of climate change on skipjack tuna abundance and spatial distribution. Glob. Change Biol. 20, 742–753. doi: 10.1111/gcb.12460
Dufour, F., Arrizabalaga, H., Irigoien, X., and Santiago, J. (2010). Climate impacts on albacore and bluefin tunas migrations phenology and spatial distribution. Prog. Oceanogr. 86, 283–290. doi: 10.1016/j.pocean.2010.04.007
Errauskin-Extramiana, M., Arrizabalaga, H., Hobday, A., Cabré, A., Ibaibarriaga, L., Arregui, I., et al. (2019). Large-scale distribution of tuna species in a warming ocean. Glob. Change Biol. 25, 2043–2060. doi: 10.1111/gcb.14630
Essington, T. E., Moriarty, P. E., Froehlich, H. E., Hodgson, E. E., Koehn, L. E., Oken, K. L., et al. (2015). Fishing amplifies forage fish population collapses. Proc. Natl. Acad. Sci. 112, 6648–6652. doi: 10.1073/pnas.1422020112
Fox, W. W. (1970). An exponential surplus-yield model for optimizing exploited fish populations. Trans. Am. Fish. Soc. 99, 80–88. doi: 10.1577/1548-8659(1970)99<80:aesmfo>2.0.co;2
Free, C. M., Thorson, J. T., Pinsky, M. L., Oken, K. L., Wiedenmann, J., and Jensen, O. P. (2019). Impacts of historical warming on marine fisheries production. Science 363, 978–983.
Fromentin, J. M., Bonhommeau, S., Arrizabalaga, H., and Kell, L. T. (2014). The spectre of uncertainty in management of exploited fish stocks: the illustrative case of Atlantic bluefin tuna. Mar. Policy 47, 8–14. doi: 10.1016/j.marpol.2014.01.018
Garcia, S. (1996). The Precautionary Approach to Fisheries and its Implications for Fishery Research, Technology and Management: an Updated Review in Precautionary Approach to Fisheries. Part 2: Scientific Papers. Rome: FAO, 76.
Garcia, S. M., and Rosenberg, A. A. (2010). Food security and marine capture fisheries: characteristics, trends, drivers and future perspectives. Philos. Trans. R. Soc. B 365, 2869–2880. doi: 10.1098/rstb.2010.0171
ICCAT (2015). Recommendation by ICCAT to Establish Harvest Control Rules for North Atlantic Albacore Stock (Rec 15–14). Madrid: ICCAT.
ICCAT (2016a). ICCAT. Report of the Intersessional Meeting of Panel 2. Sapporo-Japan, July 2016. Madrid: ICCAT.
ICCAT (2016b). Recommendation by ICCAT on a Multi-Annual Conservation and Management Programme for North Atlantic Albacore (Rec 16–16). Madrid: ICCAT.
ICCAT (2016c). Report of the 2016 ICCAT North and South Atlantic Albacore Stock Assessment Meeting. Madrid: ICCAT, 68.
ICCAT (2017). Recommendation by ICCAT on a Harvest Control Rule for North Atlantic Albacore Supplementing the Multiannual Conservation and Management Programme (Rec 16–16). Madrid: ICCAT.
IPCC (2007). “Climate change 2007: synthesis report,” in Contribution of Working Groups I, II and III to the Fourth Assessment Report of the Intergovernmental Panel on Climate Change, eds R. K. Pachauri, and A. Reisinger, (Geneva: IPCC).
ISSF (2013). Report of the 2013 ISSF Stock Assessment Workshop: Harvest Control Rules and Reference Points for Tuna RFMOs. ISSF Technical Report 2013-03. Washington, DC: International Seafood Sustainability Foundation.
Juan-Jordá, M. J., Mosqueira, I., Cooper, A. B., Freire, J., and Dulvy, N. K. (2011). Global population trajectories of tunas and their relatives. Proc. Natl. Acad. Sci. U.S.A. 108, 20650–20655. doi: 10.1073/pnas.1107743108
Kell, L. (2016). mpb 1.0.0. A package for implementing management procedures, that can be simulation testing using Management Strategy Evaluation. Available at: https://github.com/laurieKell/mpb
Kell, L., Arrizabalaga, H., Merino, G., and De Bruyn, P. (2016). Conditioning an operating model for North Atlantic albacore (SCRS/2016/023). Collect. Vol. Sci. Pap. ICCAT 73, 1296–1327.
Kell, L., Merino, G., de Bruyn, P., Ortiz de Urbina, J., Arrizabalaga, H., Santiago, J., et al. (2013). An Example of Conditioning an Operating Model Using Multifan-CL. ICCAT, SCRS/2013/34. Madrid: ICCAT.
Kell, L., and Mosqueira, I. (2016). Conditioning Operating Models on Data and Knowledge and Rejecting and Weighting of Hypotheses. SCRS/2016/014. Madrid: ICCAT.
Kiureghiana, A. D., and Ditlevsen, O. (2009). Aleatory or epistemic? Does it matter? Struct. Saf. 31, 105–112. doi: 10.1016/j.strusafe.2008.06.020
Kleiber, P., Hampton, J., Davies, N., Hoyle, S., and Fournier, D. A. (2012). MULTIFAN-CL User’s Guide. 152.
Kuparinen, A., Keith, D. M., and Hutchings, J. A. (2014). Increased environmentally driven recruitment variability decreases resilience to fishing and increases uncertainty of recovery. ICES J. Mar. Sci. 71, 1507–1514. doi: 10.1093/icesjms/fsu021
Lee, H., Piner, R. K., Maunder, M. N., and Methot, R. D. (2012). Can steepness of the stock–recruitment relationship be estimated in fishery stock assessment models? Fish. Res. 12, 254–261. doi: 10.1016/j.fishres.2012.03.001
Lehodey, P., Alheit, J., Barange, M., Baumgartner, T., Beaugrand, G., Drinkwater, K., et al. (2006). Climate variability, fish, and fisheries. J. Clim. 19, 5009–5030.
Lehodey, P., Chai, F., and Hampton, J. (2003). Modelling climate-related variability of tuna populations from a coupled Ocean-biogeochemical-populations dynamics model. Fish Oceanogr. 12, 483–494. doi: 10.1046/j.1365-2419.2003.00244.x
Lehodey, P., Senina, I., Nicol, S., and Hampton, J. (2015). Modelling the impact of climate change on South Pacific albacore tuna. Deep Sea Res. Part II. 113, 246–259. doi: 10.1016/j.dsr2.2014.10.028
Maunder, M. N., and Watters, G. M. (2001). Status of Yellowfin Tuna in the Eastern Pacific Ocean. InterAm. Trop. Tuna Comm. 1, 5–86. doi: 10.1007/s12011-011-9136-4
Maury, O., Campling, L., Arrizabalaga, H., Aumont, O., Bopp, L., Merino, G., et al. (2017). From shared socio-economic pathways (SSPs) to oceanic system pathways (OSPs): building policy-relevant scenarios for global oceanic ecosystems and fisheries. Glob. Environ. Change 45, 203–216. doi: 10.1016/j.gloenvcha.2017.06.007
Merino, G., Arrizabalaga, H., Santiago, J., Sharma, R., Ortiz, de Zarate, V., et al. (2017a). Options for an observation error model for North Atlantic albacore MSE. SCRS/2017/091. Collect. Vol. Sci. Pap. ICCAT 74, 410–431.
Merino, G., Arrizabalaga, H., Santiago, J., Sharma, R., Ortiz de Zarate, V., De Bruyn, P., et al. (2017b). Uncertainty grid for North Atlantic albacore management strategy evaluation: conditioning operating models. ICCAT Collect. Vol. Sci. Pap. 74, 432–456.
Merino, G., Arrizabalaga, H., Santiago, J., Sharma, R., Ortiz de Zarate, V., De Bruyn, P., et al. (2017c). Updated evaluation of harvest control rules for North Atlantic albacore through management strategy evaluation. ICCAT Collect. Vol. Sci. Pap. 74, 457–478.
Merino, G., Barange, M., Blanchard, J. L., Harle, J., Holmes, R., Allen, I., et al. (2012). Can marine fisheries and aquaculture meet fish demand from a growing human population in a changing climate? Glob. Environ. Change 22, 795–806. doi: 10.1016/j.gloenvcha.2012.03.003
Merino, G., Barange, M., Fernandes, J. A., Mullon, C., Cheung, W., Trenkel, V., et al. (2014). Estimating the economic loss of recent North Atlantic fisheries management. Prog. Oceanogr. 129, 314–323. doi: 10.1016/j.pocean.2014.04.022
Mora, C., Myers, R. A., Coll, M., Libralato, S., Pitcher, T. J., Sumaila, U. R., et al. (2009). Management effectiveness of the World’s marine fisheries. PLoS Biol. 7:e1000131. doi: 10.1371/journal.pbio.1000131
Moyle, P. B., Smith, J. J., Daniels, R. A., and Baltz, D. M. (1986). Distribution and ecology of the fishes of Sacramento-San Joaquín drainage system, California: a review. Univ. Calif. Publ. Zool. 115, 225–256.
Poloczanska, E. S., Brown, C. J., Sydeman, W., Kiessling, W., Schoeman, D. S., Moore, P. J., et al. (2013). Global imprint of climate change on marine life. Nat. Clim. Change 3, 919–925. doi: 10.1038/nclimate1958
Poloczanska, E. S., Burrows, M. T., Brown, C. J., Garcia Molinos, J., Halpern, B. S., Hoegh-Guldberg, O., et al. (2016). Responses of marine organisms to climate change across oceans. Front. Mar. Sci. 3:62. doi: 10.3389/fmars.2016.00062
Punt, A., Butterworth, D. D. S., de Moor, C. L., De Olveira, A. A., and Haddon, M. (2014). Management strategy evaluation: best practices. Fish Fish. 17, 303–334. doi: 10.1111/faf.12104
Rademayer, R. A., Plaganyi, E. E., and Butterworth, D. S. (2007). Tips and tricks in designing management procedures. ICES J. Mar. Sci. 64, 618–625. doi: 10.1155/2018/6865156
Rice, J. C., and Garcia, S. M. (2011). Fisheries, food security, climate change and biodiversity: characteristics of the sector and perspectives of emerging issues. ICES J. Mar. Sci. 68, 1343–1353. doi: 10.1093/icesjms/fsr041
Sarmiento, J. L., Slater, R., Barber, R., Bopp, L., Dooney, S. C., Hirst, A. C., et al. (2004). Response of ocean ecosystems to climate warming. Glob. Biogeochem. Cycles 18:GB3003.
Schaefer, M. (1954). Some aspects of the dynamics of populations important to the management of commercial marine fisheries. Bull. Inter Am. Trop. Tuna Comm. 1, 27–56. doi: 10.1016/S0065-2881(09)56003-8
Scully, M. L. (2018). Peer Review of the Code and Algorithms Used With the Management Strategy Evaluation Framework for the North Atlantic Albacore Stock (SCRS/2018/142). Madrid: ICCAT.
Shoji, J., Toshito, S., Mizuno, K., Kamimura, Y., Hori, M., and Hirakawa, K. (2011). Possible effects of global warming on fish recruitment: shifts in spawning season and latitudinal distribution can alter growth of fish early life stages through changes in daylength. ICES J. Mar. Sci. 68, 1165–1169. doi: 10.1093/icesjms/fsr059
Strong, M., and Oakley, J. E. (2014). When is a model good enough? Deriving the expected value of model improvement via specifying internal model discrepancies. SIAM/ASA J. Uncertain. Quantif. 2, 106–125. doi: 10.1137/120889563
UN (1995). “The United Nations agreement for the implementation of the provisions of the united nations convention on the law of the sea of 10 december 1982 relating to the conservation and management of straddling fish stocks and highly migratory fish stocks,” in Proceedings of the United Nations Conference on Straddling Fish Stocks and Highly Migratory Fish Stocks, (New York, NY).
Keywords: North Atlantic albacore, harvest control rule, management strategy evaluation, climate change, adaptability
Citation: Merino G, Arrizabalaga H, Arregui I, Santiago J, Murua H, Urtizberea A, Andonegi E, De Bruyn P and Kell LT (2019) Adaptation of North Atlantic Albacore Fishery to Climate Change: Yet Another Potential Benefit of Harvest Control Rules. Front. Mar. Sci. 6:620. doi: 10.3389/fmars.2019.00620
Received: 17 June 2019; Accepted: 20 September 2019;
Published: 10 October 2019.
Edited by:
Ruben Hernan Roa-Ureta, King Fahd University of Petroleum and Minerals, Saudi ArabiaReviewed by:
Hidetada Kiyofuji, National Research Institute of Far Seas Fisheries, JapanBrett W. Molony, Commonwealth Scientific and Industrial Research Organisation (CSIRO), Australia
Copyright © 2019 Merino, Arrizabalaga, Arregui, Santiago, Murua, Urtizberea, Andonegi, De Bruyn and Kell. This is an open-access article distributed under the terms of the Creative Commons Attribution License (CC BY). The use, distribution or reproduction in other forums is permitted, provided the original author(s) and the copyright owner(s) are credited and that the original publication in this journal is cited, in accordance with accepted academic practice. No use, distribution or reproduction is permitted which does not comply with these terms.
*Correspondence: Gorka Merino, gmerino@azti.es