- 1Institute of Marine Research, Bergen, Norway
- 2School of Biological and Marine Science, University of Plymouth, Plymouth, United Kingdom
- 3Gardline Ltd., Great Yarmouth, United Kingdom
Habitat suitability models are being used worldwide to help map and manage marine areas of conservation importance and scientific interest. With groundtruthing, these models may be found to successfully predict patches of occurrence, but whether all patches are part of a larger interbreeding metapopulation is much harder to assert. Here we use a North Atlantic deep-sea case study to demonstrate how dispersal models may help to complete the picture. Pheronema carpenteri is a deep-sea sponge that, in aggregation, forms a vulnerable marine ecosystem in the Atlantic Ocean. Published predictive distribution models from United Kingdom and Irish waters have now gained some support from targeted groundtruthing, but known aggregations are distantly fragmented with little predicted habitat available in-between. Dispersal models were used to provide spatial predictions of the potential connectivity between these patches. As little is known of P. carpenteri’s reproductive methods, twenty-four model set-ups with different dispersal assumptions were simulated to present a large range of potential dispersal patterns. The results suggest that up to 53.1% of the total predicted habitat may be reachable in one generation of dispersal from known populations. Yet, even in the most dispersive scenario, the known populations in the North (Hatton-Rockall Basin) and the South (Porcupine Sea Bight) are predicted to be unconnected, resulting in the relative isolation of these patches across multiple generations. This has implications for Ireland’s future conservation efforts as they may have to conserve patches from more than one metapopulation. This means that conserving one patch may not demographically support the other, requiring additional attentions to ensure that marine protected areas are ecologically coherent and sustainable. This example serves as a demonstration of a combined modeling approach where the comparison between predicted distribution and dispersal maps can highlight areas with higher conservation needs.
Introduction
Habitat suitability models (HSMs) are have growing utility in marine ecology and management (Guisan and Zimmermann, 2000). These models relate species or habitat occurrence data to geographically referenced environmental conditions, providing a means to fill in observational gaps with an occurrence prediction where environmental conditions are known and found to be similar (Elith and Leathwick, 2009). Such maps can be useful in, for example, the planning of marine protected area (MPA) networks and fishery management (Ross and Howell, 2013), targeting sampling efforts (Martin et al., 2014), and understanding marine ecology (Skov et al., 2008).
While HSMs can perform well, one downfall is their failure to account for animal movement. These correlative models are built on wide-coverage abiotic variables, often derived from satellite data, but usually necessarily omit species interactions and dispersal information due to the lack of available data on relevant scales (Elith and Leathwick, 2009; Thuiller et al., 2015; Yates et al., 2018). Without knowledge of species dispersal abilities or the positioning of potential dispersal barriers, it is impossible to know whether fragmented areas of predicted suitable habitat can be reached by animals from known patches. If known patches are far apart then it is possible that they are not part of the same interbreeding metapopulation [sensu Hanski and Gilpin (1991) after Levins (1969)].
Accounting for animal movement is important for sustainable marine management (Cowen and Sponaugle, 2009). The MPA concept relies upon protecting enough of the population to be sustainable, reseeding patches both outside and within the MPAs and allowing the population to persist despite potential destructive activities occurring in surrounding areas (Jones et al., 2007; Ross et al., 2017). If MPAs are positioned across an area thought to represent one metapopulation when in fact there are two, then one or both metapopulations may be insufficiently supported resulting in demographic decline despite best efforts to protect them (Kritzer and Sale, 2004; Sale et al., 2005; Agardy et al., 2011). Consequently, it is wise to reassess any previous distribution predictions, should appropriate animal movement data become available in the future.
Population dynamics attributed to animal movement can be assessed in multiple ways. Classical animal tracking methods may be appropriate for larger motile species, but benthic invertebrates, which are especially suited to HSMs due to having a sessile or limited-mobility adult life stage, are more complex to track due to dispersal predominantly occurring during a larval phase (Levin, 2006; Cowen and Sponaugle, 2009). Population genetics can provide a connectivity assessment showing the relatedness of faunal patches where suitable markers can be identified, but this requires expensive and difficult sampling to collect and process multiple organisms within each patch (Hedgecock et al., 2007). Geochemical tracers are appropriate for animals with calcified structures that persist from their dispersive life stage to adulthood, but again require both physical samples and a means to interpret the geochemical markers that are discovered (Thorrold et al., 2007). Biophysical dispersal models (DMs) integrate known species occurrence and life history data with numerical models of ocean currents to predict the possible pathways of dispersal and infer spatially explicit population connectivity patterns (Werner et al., 2007; Metaxas and Saunders, 2009). As a benefit, these models can be made without physical samples, but ultimately predictions remain unverified, necessitating later groundtruthing using one of the sampling-dependent methods (Cowen and Sponaugle, 2009; Ross et al., 2019).
Cautiously, DMs are gaining popularity in marine ecology. Together with the increase of accessible computational power, the ability to try out multiple scenarios and provide a low-cost best guess, prior to intensive sampling programs, is an attractive proposition (particularly in the deep sea where physical sampling is especially expensive and difficult). For example, simulating dispersal has facilitated the analysis of coral bleaching recovery potential (Bode et al., 2018), the effects of dispersal barriers (Wood et al., 2016), the effects of larval behavior on dispersal potential (North et al., 2008), the discovery of likely larval behaviors (James et al., 2019), and the location of undiscovered populations (Yearsley and Sigwart, 2011).
Given the abilities of both HSMs and DMs, it is now possible to consider habitat distribution and dispersal patterns together. Such studies can highlight the potential for invasive species spread (Inglis et al., 2006; Sullivan et al., 2012), site restoration success (Elsäßer et al., 2013), and identifying spawning sites (Hinrichsen et al., 2016). Although only theoretical until groundtruthing can be completed, this form of combined modeling assessment can also offer some insights into potential metapopulation structure.
This study considers both habitat distribution and dispersal patterns to investigate the potential metapopulation structure of a vulnerable marine ecosystem (VME) found in the deep North Atlantic. VMEs are defined by the international Food and Agriculture Organization (FAO) as communities with attributes which may be considered unique or rare, functionally significant, fragile, having limited recovery potential, or providing structural complexity to the benefit of biodiversity (FAO, 2009). In the Northeast Atlantic, OSPAR (the OSlo PARis commission for the Protection of the Marine Environment of the North-East Atlantic) has defined deep sea sponge aggregations as one such VME (OSPAR, 2010), with this study focusing on aggregations of the hexactinellid sponge Pheronema carpenteri (Wyville Thomson, 1869) in United Kingdom and Irish waters (which are themselves explicitly listed as a type of deep sea sponge aggregation VME (OSPAR, 2010)). Pheronema carpenteri occurs in high densities (up to 1.5 m2) at known patches in the Porcupine Seabight (Rice et al., 1990) and the Hatton Rockall Basin (Howell et al., 2014; Neat et al., 2019), with historical records of additional aggregations (“the Holtenia grounds,” referring to their original name Holtenia carpenteri) in the Northern Rockall Trough (Wyville Thomson, 1874). The sponges themselves seem to provide some elevation and shelter to other megabenthic invertebrates, but their presence notably may promote increased abundance and diversity in macrobenthos within their spicule mats (Bett and Rice, 1992). As hexactinellids, these aggregations may also play an important part as a sink in the marine silicon cycle which is thought to influence primary productivity and the carbon cycle (Maldonado et al., 2005; Hendry et al., 2019). Presently aggregations are found between 950 and 1350 m in the study area, beyond the 800 m legal depth limit for EU deep-sea trawl fisheries but, as they occur on gently sloping soft sediment, they could be impacted if this limit were to change. Currently only one MPA is designated for their protection (the Hatton-Rockall Basin Nature Conservation MPA).
Almost nothing is known about the life history of this VME’s umbrella species, P. carpenteri, but there are some observations which can be used to frame dispersal simulations. Generally, Hexactinellids are currently assumed to be viviparous with lecithotrophic larvae and short planktonic larval durations (PLDs, <24 h), but these assumptions are based on limited observations of only a few species (Leys and Ereskovsky, 2006). The short PLD is only known from one shallow water cave-dwelling species (Oopsacas minuta Topsent, 1927; Boury-Esnault et al., 1999) which is unlikely to be representative of these deep-water taxa: deep-sea species are thought to have longer PLDs than their shallow water counterparts (Hilário et al., 2015). Records of “wandering populations” of P. carpenteri with juveniles found on the edge of patches (Barthel et al., 1996) may suggest very limited dispersal capabilities, but we have also observed solitary P. carpenteri distant from any known patch, suggesting that another more dispersive mode may also be possible. This could be explained by also employing asexual reproduction which is thought to be less common in sponges but is known from some hexactinellids and may even be the dominant form of reproduction for some species (Teixidó et al., 2006). Asexual reproduction takes the form of budding or fragmentation of the adult (Teixidó et al., 2006) which may be less dispersive, but buds have also been collected from the water column (Dayton et al., 2013). Two early texts (Wyville Thomson, 1869; Kent, 1870) on the species (where it is referred to as Holtenia carpenteri or Pheronema greyi) suggest that P. carpenteri may produce gemmules, a method more common in freshwater sponges where a small coated cluster of cells are released (Simpson and Fell, 1974). If this is true, then a more dispersive asexual mode may be possible.
Our previously published HSM in the region shows a potential separation of major P. carpenteri aggregation habitat patches in the north and south (Ross and Howell, 2013), posing questions of metapopulation structure and population connectivity. This study utilizes the published HSM and combines it with new DMs to explore:
(a) Whether the predicted habitat patches in the north and south are likely to be connected,
(b) The dispersal considerations for designing sponge VME protection efforts in the region.
Materials and Methods
The Study Area and the HSM
In 2013, we published a habitat suitability model for Pheronema carpenteri aggregation habitats in United Kingdom and Irish waters, centered around the Rockall Trough (Figure 1, Ross and Howell, 2013). The HSM was built in Maxent (version 3.3.3, Phillips et al., 2004, 2006) and based on aggregation [not species, see Howell et al. (2011)] presence data from 222 research transects collected between 1977–2000 (photographic and trawl) and 2005–2011 (photographic and video). The model was driven by topographic variables derived from the General Bathymetric Chart of the Oceans (GEBCO, 2008) at a resolution of 30 arc-seconds (roughly 750 m at this latitude). The HSM was internally evaluated using presence/absence data (repeat splits of 75% training, 25% test data) with an Area Under the Curve of 0.99, and sensitivity 0.96, specificity 0.95, and percent correctly classified 0.95 (the latter three being threshold-dependent metrics based on the best performing threshold that maximizes sensitivity and specificity, 0.19). All assessment metric values were considered to be “excellent” (above 0.9). As a consequence, to the best of our knowledge, and prior to extensive validation, this model is adequate for the purposes of this study [but see other studies on issues with HSM performance metrics and the potential for artificially good performance ratings (Liu et al., 2005; Lobo et al., 2008; Gregr et al., 2019)]. For more information see Ross and Howell (2013).
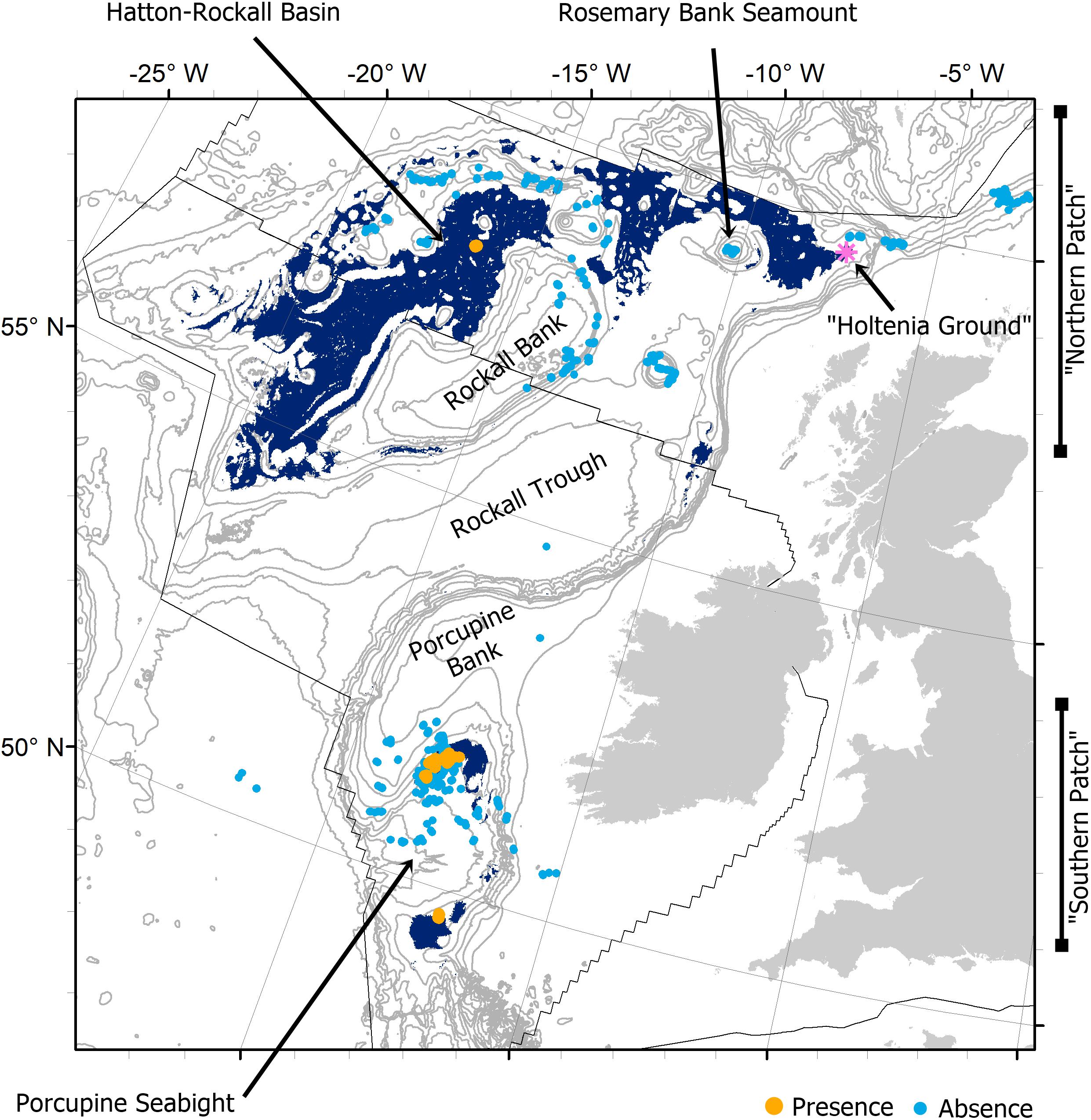
Figure 1. A map of the study area showing the predicted distribution of Pheronema carpenteri aggregations in United Kingdom and Irish waters (after Ross and Howell, 2013). The prediction shows all areas where the likelihood of P. carpenteri aggregation presence is above 0.19 (a threshold selected to maximize the sensitivity and specificity metrics of assessment). Presence and absence data used to train the HSM (after Ross and Howell, 2013) are shown to contextualize known aggregations. Names of locations mentioned in the text are labeled. Background bathymetry contours from GEBCO show every 200–1000 m depth, then every 500 m. Black lines show the current extent of the United Kingdom and Irish continental shelves within the study area.
A subsequent HSM based on high resolution bathymetry (200 m multibeam) tested worse than the GEBCO-based model and predicted a distribution over an area that was 53% larger (Ross et al., 2015). It is possible that the coarser resolution GEBCO model may better reflect the scales of oceanographic drivers that influence these aggregations: Rice et al. (1990) posit that P. carpenteri aggregations may be situated proximate to, but not within, areas of enhanced near-bottom velocities that may resuspend organic matter to the sponges feeding advantage. Until further validation data can be collected it remains unknown which prediction is closest to reality, but for the purposes of this study the precautionary principle advises testing the most spatially restricted prediction.
Pheronema carpenteri aggregations were predominantly predicted to occur in the Hatton Rockall Basin (HRB) and the Northern Rockall Trough (“the northern patch”), and around the upper slopes of the Porcupine Seabight (PSB) (“the southern patch”, Ross and Howell, 2013, Figure 1). The nearest intermediate areas between the southern and northern patches are on the south-eastern slopes of Rockall Bank to the northwest and on the continental slope beside the Hebrides Terrace Seamount to the northeast. The habitat gap is formed by the topographic high of Porcupine Bank, and the topographic lows of the Porcupine Abyssal Plain and the Rockall Trough (Figure 1).
The DMs
Dispersal simulations require three components:
• Biological data to parameterize simulations [e.g., release locations, planktonic larval duration, larval growth, behavior in the water column, etc, see Metaxas and Saunders (2009)],
• A hydrodynamic model that supplies current velocity instructions,
• A dispersal simulator that follows the hydrodynamic instructions whilst integrating time and biological parameters.
Biological Parameters
Due to the lack of life history data available for P. carpenteri, a suite of biological parameters were used to bracket simulations into four different potential scenarios (S1–S4) that could be relevant to this species and the habitat it creates:
• S1 – The most retentive simulation (with respect to achievable dispersal distance): Assumed a terrain-hugging dispersal of either an asexual bud or fragment, or a passive cloud of larvae traveling together as released from observed locations. This may be the case if buds, fragments, or larvae are trapped in the bottom boundary layer, are negatively buoyant, or have a positive geotaxis. This may represent the mechanism for the observed wandering populations.
• S2 – Moderate retentive: Assumed a passive non-terrain hugging individual larvae or bud with limited random diffusion away from their cohort, as released from observed locations. The diffusive component is a proxy for either limited random swimming behavior or oceanographic mixing.
• S3 – Moderate dispersive: Echoed the second but parameterized with strong random diffusion away from the cohort. This would represent larvae that swim actively, have a phototaxis, have positive buoyancy, or encounter turbulent oceanographic mixing.
• S4 – The most dispersive: Echoed the high diffusion scenario (S3), but simulated release is from across all predicted suitable habitat (not just releasing from observed locations).
It is possible that there may be very specific active swimming behavior in P. carpenteri larvae, e.g., specific times spent at specific depths, but this is impossible to represent without a priori information. The random diffusive kicks of different severity in S2 and S3/4 can capture some of this potential and may allow at least the possibility of traversing major topographic barriers to dispersal which is potentially the main dispersive advantage of a vertical migration strategy (Ross et al., 2017). Alternatively, active swimming may have a retentive effect (Cowen et al., 2006), in which case the dispersive predicted dispersal patterns from this study may be more dispersive than is realistic. Either way the bracketed scenarios should capture a realistic range of dispersal patterns given the knowledge that is currently available.
For the purposes of this study two different dispersal simulators were used in order to capture different biological assumptions. The Marine Geospatial Ecology Tools simulator (MGET) tracks the expansion of a footprint of dispersal so is suited for passive dispersal or consideration of an area of influence (used in S1). The Connectivity Modeling Tools simulator (CMS) is closer to an individual based model tracking each particle independently and allowing the integration of behaviors and random walk patterns (used in S2–S4). The dispersal simulators are explained in more detail in section “The Dispersal Simulators.”
Dispersal model release locations were based on both observational data and the HSM. The observations of P. carpenteri aggregations that were used to build the HSM were used in S1–S3 as release locations optimized to reflect the horizontal sensitivity of the dispersal simulator (3 km separation). For S4, a regular grid of release locations, spaced at the resolution of the hydrodynamic model (1/12°, see section “The Hydrodynamic Model”), was created to span all predicted areas of suitable habitat according to the HSM. For all release locations see Supplementary Data.
It is unknown what temporal pattern may be found in P. carpenteri reproduction: whether spawning is possible year-round or follows a seasonal pattern. Both have been observed in hexactinellids (Leys and Ereskovsky, 2006). It therefore seemed prudent to adopt a year-round release frequency, allowing the full range of seasonal variability in dispersal patterns to be captured in predictions. To reduce computational load this was represented as a monthly release of larvae.
A range of PLDs was used to time the simulations in each of the four scenarios. The observed 24 h PLD of the shallow water hexactinellid Oopsacas minuta (Boury-Esnault et al., 1999) was considered to be the minimum potential PLD for P. carpenteri which is a deep-water species and is therefore likely to spend longer in the water column (Hilário et al., 2015). Simulations within each of the four scenarios were therefore run for 1, 5, 10, 20, 30, and 50 days to capture a range of potential PLDs (totaling 24 model set-ups). Hilário et al. (2015) calculated that the average known PLD of a deep sea organism is 35 days so the range used in this study should span the majority of likely scenarios.
Due to the different natures of the dispersal simulators used the number of larvae released is different for S1 and S2–S4. While in reality the number of larvae released could range anywhere from tens to millions, model simulations are better treated as statistical representations with numbers chosen to capture the range of predicted dispersal directions available in the model. In S1 an initial density of 10 000 particles (theoretical larvae) per km2 was used as recommended by Treml et al. (2008). In S2–S4, as a balance of computational power and ease of interpretation, 100 particles were released and tracked individually to provide a proportional representation of likely dispersal pathways.
Table 1 shows a summary of the parameterization of each of the four scenarios.
The Hydrodynamic Model
For this study the hydrodynamic model was the freely available Hybrid Coordinate Ocean Model (HYCOM, Chassignet et al., 2007). This is a global model gridded at 1/12° (approx. 8 km), with daily outputs available online spanning 40 depth layers down to 5000 m. Simulations were run using outputs from three selected years (2003, 2007, 2010) to capture extremes of the North Atlantic Oscillation (neutral, positive, and negative, respectively) which may differently affect dispersal predictions (Fox et al., 2016; Ross et al., 2016) and allowed a reduced computational demand.
HYCOM has been tested locally for DM applications with sensitivity testing (Ross et al., 2016) and a model comparison (Ross et al., 2019). Tests showed a broad agreement with dominant current directions and speeds, but a tendency to give over-dispersive predictions due to the absence of tides (typical in large scale models) and the type of algorithm it uses to handle horizontal pressure gradient errors (Ross et al., 2016, 2019). The results are therefore interpreted assuming dispersal is likely to be more restrictive in reality.
The Dispersal Simulators
Two different dispersal simulators were used together with the HYCOM outputs. Each simulator utilizes different assumptions and algorithms. The life history traits of the simulated species should be used to decide what type of simulator is likely to be relevant, but in this case a lack of life history data necessitates testing different scenarios to capture a range of possible predicted dispersal abilities.
The Coral Reef Larval Connectivity Model available in the Marine Geospatial Ecology Tools (MGET, Roberts et al., 2010; which is based on Treml et al., 2008) package (version 0.8a52), attached to ArcGIS version 9.3 was used to simulate dispersal within the benthic boundary layer, with particles moving as a spreading cloud or as aggregated chunks (the retentive scenario, S1). Only the HYCOM depth rasters (obtained through MGET) over which the adults have been found in the study area (900–1400 m) were selected. These were then converted into a single terrain-following (sigma) raster using a python batch script which instructed the ArcGIS spatial analyst extension to mosaic the HYCOM rasters ordered from the deepest to shallowest (retaining only the deepest velocity values). Consequently, depths shallower than 900 m were treated as ‘land’ by the model. Simulations were run in a polar stereographic projection with data gaps filled using ArcGIS’s inverse distance weighting interpolation. The MGET simulator has been successfully applied to dispersal predictions of reef fish (Mora et al., 2011), sargassum (Mattio et al., 2013) and an assessment of MPA connectivity (Crochelet et al., 2016) among other studies.
The connectivity modeling system (CMS, Paris et al., 2013) is a standalone Linux-based simulator which was used to statistically represent individual larvae dispersing above the benthic boundary layer (in the three moderate/dispersive scenarios). These simulations, hosted by the University of Plymouth High Performance Computing cluster, were driven by HYCOM z-level outputs. Although HYCOM outputs are not supplied with vertical velocities as standard, these simulations incorporated vertical velocities computed using the continuity equation, allowing some vertical movement in the water column, supplemented by random diffusivity kicks every hour. Among other studies, the CMS simulator has recently been used to: estimate the connectivity of shrimp between management units (Le Corre et al., 2019), track oil spills (Ainsworth et al., 2018) and water masses (Ypma et al., 2019), and assess the deep refugia hypothesis in mesophotic coral ecosystems (Sponaugle and Cowen, 2019).
Analysis
The results of all simulations were visualized in ArcGIS 10.3 for qualitative and semi-quantitative evaluation. S1 daily output rasters were summed to show the predicted dispersal footprint for each of the six PLDs. Matlab was used to convert S2–S4 netcdf outputs into rasters of larval density comparable to the outputs from S1. Raster footprints of dispersal per PLD per scenario were compared with the footprint of suitable habitat from the Ross and Howell (2013) HSM. All rasters were compared at a resolution of 4 km (∼1/2 HYCOM resolution) and projected in Albers Equal Area Conic with modified standard parallels (parallel 1 = 50.2°, parallel 2 = 58.5°).
The percentage overlap between footprints of dispersal and the HSM predicted suitable habitat was calculated as a measure of how much habitat was within reach of one individual (or one generation if considering gene flow from the release location).
The most dispersive scenario, S4, was then evaluated further with the footprints of dispersal from northern and southern populations visualized separately and considered together with predicted larval densities which operates as a proxy for likelihood of connectivity potential.
Results
Maps of predicted larval dispersal under the four scenarios, each with six PLDs, are visualized in Figure 2 (24 dispersal footprints in total). As expected, scenarios S1–S4 show progressively greater potential distances of dispersal. After 50 days of dispersal the maximum straight-line distance traveled was 140 km (S1, terrain-hugging/passive), 470 km (S2, escape BBL/low diffusion), 695 km (S3, escape BBL/high diffusion), and >700 km (S4, escape BBL/high diffusive/HSM releases; particles exit the model domain).
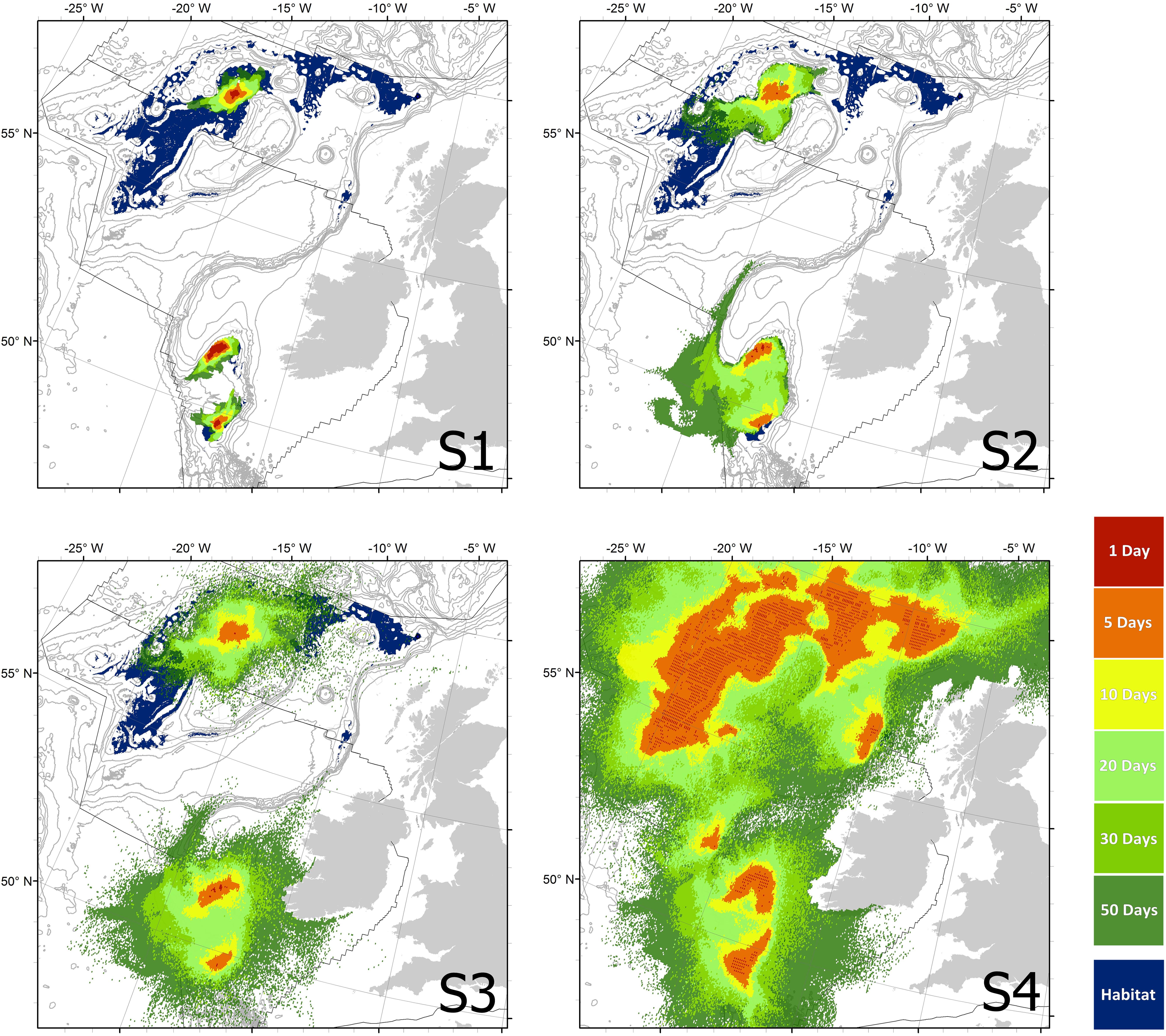
Figure 2. Maps of the four scenarios showing the habitat suitability model (Ross and Howell, 2013) predicted habitat overlaid with the footprints of each of the six Planktonic Larval Durations (PLDs). Background bathymetry contours from GEBCO show every 200–1000 m depth, then every 500 m. Black lines show the current extent of the United Kingdom and Irish continental shelves within the study area.
Table 2 shows the proportions of habitat that could be reached by each dispersal footprint launched from observed populations (S1–S3). While S1 generally predicted a reduced dispersal potential, this terrain hugging strategy may allow a greater proportion of habitat to be reached in 1 day (1.6% as compared to 0.3%) than a dispersal strategy that escapes the benthic boundary layer. After 5 days S2–S3 are consistently more dispersive.
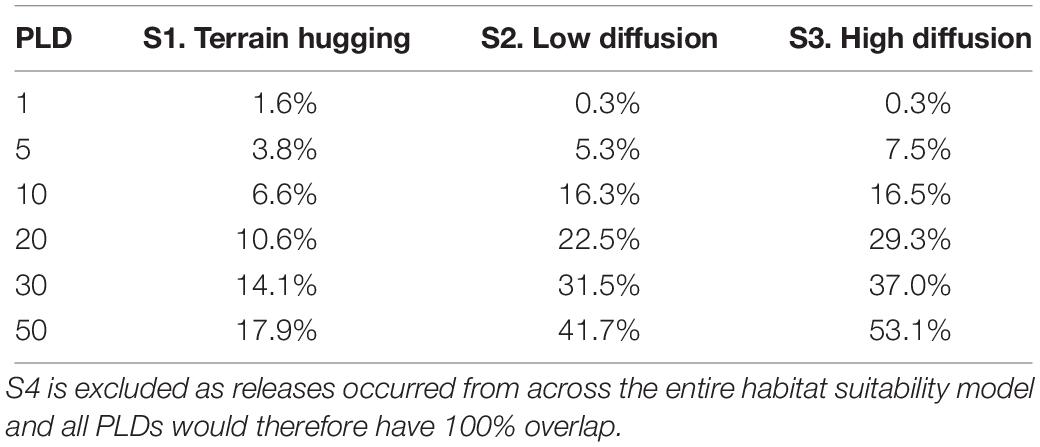
Table 2. The percentage of predicted suitable habitat (after Ross and Howell, 2013) that is overlapped by the dispersal footprint (from Figure 2) of each scenario and planktonic larval duration simulated when released from known aggregations.
All PLDs in S1 resulted in isolated patches to the north and south of the Rockall Trough, but also within the Porcupine Seabight. If this scenario is realistic then none of the known habitat patches are likely to be connected, potentially representing at least three separate metapopulations.
S2 allowed a more diffuse dispersal, but under this scenario larvae cannot vertically migrate far enough to overcome dispersal barriers such as Rockall Bank and Porcupine Bank. This scenario suggests that all patches in the Porcupine Seabight could be part of the same metapopulation if P. carpenteri has a PLD of 20 days or more.
S3 with the added diffusion, permits dispersal over Rockall Bank and Porcupine Bank, suggesting that propagules may possibly be found in shallower shelf and coastal waters at approximately 200m depth (see S1 and S2, Figure 2). Under S3 a maximum of 53.1% of habitat can be reached by larvae from known extant populations (Table 2), but there is no potential connection either between the north and south patches, nor between the observed Hatton Rockall Basin aggregations and the historic ‘Holtenia grounds’ in the north-eastern Rockall Trough.
S4 looks positive from the visualization of the dispersal footprints shown in Figure 2: where there are clearly larvae with the potential to cross the Rockall Trough and maybe even connect the north and south patches. However, S4 can be re-visualized as shown in Figure 3: separating the releases from across predicted suitable habitat in the northern and southern patches and showing densities of larvae that highlight the areas with highest likelihood of connection. From this perspective there is still a strong separation of northern and southern patches. From the southern releases there may be a very low chance of some larvae reaching the southernmost predicted suitable habitat in the northern patch, but the fact that these appear as isolated pixels of low larval density suggests a high likelihood of error in this prediction. Furthermore, these sparse connections are to ungroundtruthed predicted habitat which may yet be proven unsuitable.
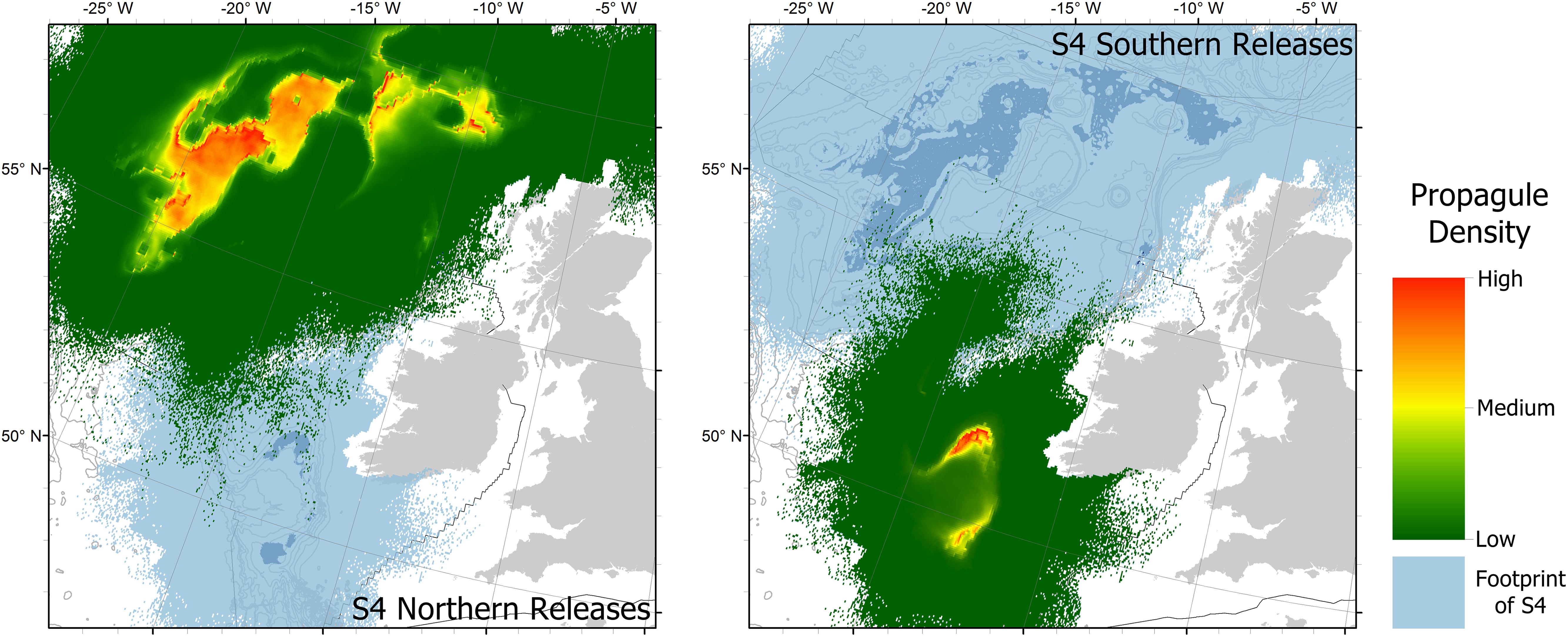
Figure 3. The scenario 4, 50 day Planktonic Larval Duration (PLD) simulations re-visualized showing the full footprint of scenario 4 broken down into those released from the north, and those released from the south. Colors now show densities of larvae rather than PLDs. Background bathymetry contours from GEBCO show every 200–1000 m depth, then every 500 m. Black lines show the current extent of the United Kingdom and Irish continental shelves within the study area.
Discussion
Are Predicted Habitat Patches in the North and South Likely to Be Connected?
Dispersal predictions from DMs can help to refine and interpret predictions of suitable habitat. This study shows that the observed aggregations of Pheronema carpenteri in the Hatton Rockall Basin and the Porcupine Seabight may be members of separate metapopulations that are unable to support each other with new recruits, even if all predicted habitat is found to be accurate.
While models are by definition imperfect simplifications of reality, the combined issues of these models (DM and HSM) suggest that these predictions are more likely to be overestimates, predicting both more expansive dispersal than is likely, due to lack of tides and diffusive numerical handling of pressure gradient errors within HYCOM (Müller et al., 2010; Ross et al., 2017, 2019), and more suitable habitat than is actually available (Ross and Howell, 2013; Ross et al., 2015).
More recent observations can begin the process of groundtruthing the HSM used in this study. Presence has been confirmed at another location in the northern Hatton Rockall Basin (Neat et al., 2019), in line with model predictions. However, a recent survey (RV Celtic Explorer CE15011, 2015, Authors pers. comm) undertook three video transects (of <1 km) in the southern Hatton Rockall Basin finding no P. carpenteri where it was predicted, although some associated fauna were present. This limited evidence is insufficient to properly groundtruth the HSM, but notably the bottom temperatures recorded in this southern region (∼4.1°C) were lower than at the known P. carpenteri aggregations (5.07–6.41°C; Howell et al., 2014, Authors pers. comm). Therefore, it may be that a minimum temperature around 5°C is necessary to present optimal conditions for aggregation formation in this region. If so, then the sparse predicted connections from southern releases into the northern patch (see Figure 3) are even less probable as they would attempt to settle within this too-cold region (see Supplementary Figure S1 with 5°C isobath approximation). The presence of P. carpenteri aggregations has also recently been confirmed on Rosemary Bank Seamount, albeit together with other sponge species (McIntyre et al., 2016); an area not captured by the prediction of the Ross and Howell (2013) HSM. However, the historic Holtenia ground (Wyville Thomson, 1874), which is the type locality for this species (formerly named Holtenia greyi), is predicted by the HSM as an area of suitable habitat (but has not been confirmed as an extant presence locality, to our knowledge). A higher resolution model based on multibeam bathymetry and oceanographic layers including temperature is currently in development with the hope of improving upon the existing published HSMs (Ross and Howell, 2013; Ross et al., 2015; Howell et al., 2016).
The DMs will also require groundtruthing in the future. Population genetic sampling of P. carpenteri is ongoing in the region and hopefully will eventually be able to confirm whether the predicted isolation of the southern metapopulation is indeed the case. The results of population genetics when compared to these DMs may also help discern more about the likely reproductive modes and timings of P. carpenteri and the role of dispersal in any genetic structuring. In the absence of more traditional observation methods, such a comparison can provide information that will better refine future DMs for this VME in other areas.
Notably the HSM and the bathymetry it is based upon are hugely important to the process of targeting new sampling areas, increasing the number of observations of this VME, and informing management decisions. Offshore and deep-water studies, such as this one, remain limited by the expense and difficulty of obtaining samples and observing the seafloor at great depths. Without seafloor mapping, this study, and much of this field of research, would not be possible.
What Are the Implications for Conservation Management in the Region?
The predicted separation of metapopulations in the north (predominantly United Kingdom) and south (predominantly Ireland) could greatly affect conservation efforts for this species. To ensure that MPA networks are sustainable and ecologically coherent, ideally MPAs should protect a healthy portion of each metapopulation, such that, should the unprotected areas be destroyed, the species will persist and be able to reseed the area. This is made more complicated by national jurisdictions, with the study area spanning the extended exclusive economic zones of both the United Kingdom and Ireland.
In United Kingdom waters there is clear intention to make efforts to conserve this VME in line with OSPAR advice. Currently only one Scottish MPA is designated specifically for the protection of P. carpenteri aggregations in the study region [the Hatton Rockall Basin Nature Conservation MPA (NCMPA)], with Rosemary Bank Seamount NCMPA also acknowledging the presence of similar aggregations among other VMEs (McIntyre et al., 2016).
Ireland is yet to protect this VME but has also committed to doing so, as a signatory to OSPAR, and is currently undertaking a major baseline survey effort to inform conservation planning and management (“SeaRover”, funded by the European Maritime and Fisheries Fund). When future MPAs are planned for the protection of this VME, Ireland will be faced with the possibility of encountering two separate metapopulations in Irish waters. Should aggregations be found in the Irish Hatton Rockall Basin, their protection cannot be assumed to be supportive of conservation efforts in the southern patch spanning the Porcupine Seabight.
It is possible that the southern metapopulation extends southwards into the Bay of Biscay. The next nearest currently designated MPA containing P. carpenteri aggregations is currently Le Danois Bank in the Spanish El Cachucho MPA (Le Danois, 1948; García-Alegre et al., 2014), with the geographic population extending as far south as Morocco if not further. Nearer patches in French waters have been recorded (Guillaumont et al., 2011, 2012) but are also yet to be protected, leaving the Porcupine Seabight population particularly sensitive to future impact without a protected larval supply.
A new EU regulation has banned bottom trawling below 800 m (Regulation (EU) 2016/2336) potentially resulting in the incidental protection of this VME, but the regulation is still being approved at the national level, could be subject to change in the future, and is difficult to enforce over such a large area. Bottom trawling arguably poses the biggest threat to P. carpenteri aggregations, which favor gently sloping soft sediment bottoms at continental slope depths (900–1300 m) which can be found proximate to land. The porcupine seabight aggregations already show evidence of bottom trawling through some patches in the past (Vieira, 2017) and have continued proximity to bottom fishing activities (Gerritsen and Lordan, 2011, 2014). It is therefore paramount that bottom trawling remains restricted in areas where this VME is found, with the Porcupine Seabight metapopulation being the most vulnerable to destruction.
Beyond the Case Study
While this study was designed to investigate the implications of habitat fragmentation for one VME in the North Atlantic, it also serves to highlight the need to investigate HSM predictions more closely in general. In datapoor situations HSMs can provide a best guess at species or habitat distribution and extent, but without investigating dispersal or connectivity it can be impossible to know how many interbreeding populations are present across a fragmented habitat. This case study demonstrates that, on occasion, an assumed larger metapopulation can turn out to be more than one, with critical implications for conservation decisions in the future.
Data Availability
All datasets generated for this study are included in the manuscript and/or the Supplementary Files.
AUTHOR CONTRIBUTIONS
All authors conceived and developed the idea, and wrote the manuscript. RR and EW carried out the modeling. KH provided the supervision and obtained the funding.
Funding
The majority of the work was funded by the University of Plymouth. KH and RR had additional support from the NERC funded DeepLinks project and the EU Seventh Framework Programme EUROFLEETS2. Gardline Ltd. supported EW during writing. The Norwegian Institute of Marine Research supported the writing of this article, and the IMR library covered open access publication fees.
Conflict of Interest Statement
EW was employed by the company Gardline Ltd. at the time of writing, but Gardline Ltd. did not have any influence on the undertaking of this study.
The remaining authors declare that the research was conducted in the absence of any commercial or financial relationships that could be construed as a potential conflict of interest.
Acknowledgments
The authors would like to thank their current institutes (RR, Institute of Marine Research; EW, Gardline Ltd.; and KH, University of Plymouth) for supporting the writing of this manuscript, and the University of Plymouth for the rest of the work. The authors would also like to thank Peter Mills at the University of Plymouth High Performance Computing Centre, and the other members of the Deep Sea Conservation Research Unit (CRU) for valuable discussion and support.
Supplementary Material
The Supplementary Material for this article can be found online at: https://www.frontiersin.org/articles/10.3389/fmars.2019.00574/full#supplementary-material
References
Agardy, T., Notarbartolo di Sciara, G., and Christie, P. (2011). Mind the gap: addressing the shortcomings of marine protected areas through large scale marine spatial planning. Mar. Policy 35, 226–232. doi: 10.1016/j.marpol.2010.10.006
Ainsworth, C. H., Paris, C. B., Perlin, N., Dornberger, L. N., Patterson, W. F. III, Chancellor, E., et al. (2018). Impacts of the deepwater horizon oil spill evaluated using an end-to-end ecosystem model. PLoS One 13:e0190840. doi: 10.1371/journal.pone.0190840
Barthel, D., Tendal, O. S., and Thiel, H. (1996). A wandering population of the hexactineliid sponge pheronema carpenteri on the continental slope off morocco, Northwest Africa. Mar. Ecol. 17, 603–616. doi: 10.1111/j.1439-0485.1996.tb00420.x
Bett, B. J., and Rice, A. L. (1992). The influence of hexactinellid sponge (Pheronema carpenteri) spicules on the patchy distribution of macrobenthos in the porcupine seabight (bathyal NE Atlantic). Ophelia 36, 217–226. doi: 10.1080/00785326.1992.10430372
Bode, M., Bode, L., Choukroun, S., James, M. K., and Mason, L. B. (2018). Resilient reefs may exist, but can larval dispersal models find them? PLoS Biol. 16:e2005964. doi: 10.1371/journal.pbio.2005964
Boury-Esnault, N., Efremova, S., Bézac, C., and Vacelet, J. (1999). Reproduction of a hexactinellid sponge: first description of gastrulation by cellular delamination in the Porifera. Invertebr. Reprod. Devl. 35, 187–201. doi: 10.1080/07924259.1999.9652385
Chassignet, E. P., Hurlburt, H. E., Martin Smedstad, O., Halliwell, G. R., Patrick, J. H., Wallcraft, A. J., et al. (2007). The HYCOM (HYbrid coordinate ocean model) data assimilative system. J. Mar. Syst. 65, 60–83. doi: 10.1016/j.jmarsys.2005.09.016
Cowen, R. K., Paris, C. B., and Srinivasan, A. (2006). Scaling of connectivity in marine populations. Science 311, 522–527. doi: 10.1126/science.1122039
Cowen, R. K., and Sponaugle, S. (2009). Larval dispersal and marine population connectivity. Annu. Rev. Mar. Sci. 1, 443–466. doi: 10.1146/annurev.marine.010908.163757
Crochelet, E., Roberts, J., Lagabrielle, E., Obura, D., Petit, M., and Chabanet, P. (2016). A model-based assessment of reef larvae dispersal in the Western Indian Ocean reveals regional connectivity patterns — potential implications for conservation policies. Reg. Stud. Mar. Sci. 7, 159–167. doi: 10.1016/j.rsma.2016.06.007
Dayton, P. K., Kim, S., Jarrell, S. C., Oliver, J. S., Hammerstrom, K., Fisher, J. L., et al. (2013). Recruitment, growth and mortality of an antarctic hexactinellid sponge, anoxycalyx joubini. PLoS One 8:e56939. doi: 10.1371/journal.pone.0056939
Elith, J., and Leathwick, J. R. (2009). Species distribution models: ecological explanation and prediction across space and time. Ann. Rev. Ecol. Evol. Syst. 40, 677–697. doi: 10.1146/annurev.ecolsys.110308.120159
Elsäßer, B., Fariñas-Franco, J. M., Wilson, C. D., Kregting, L., and Roberts, D. (2013). Identifying optimal sites for natural recovery and restoration of impacted biogenic habitats in a special area of conservation using hydrodynamic and habitat suitability modelling. J. Sea Res. 77, 11–21. doi: 10.1016/j.seares.2012.12.006
FAO (2009). ). Paragraph 42. International Guidelines for the Management of Deep-sea Fisheries in the High Seas. Rome: FAO, 9–10.
Fox, A. D., Henry, L. A., Corne, D. W., and Roberts, J. M. (2016). Sensitivity of marine protected area network connectivity to atmospheric variability. R. Soc. Open Sci. 3:160494. doi: 10.1098/rsos.160494
García-Alegre, A., Sánchez, F., Gómez-Ballesteros, M., Hinz, H., Serrano, A., and Parra, S. (2014). Modelling and mapping the local distribution of representative species on the Le Danois Bank, El cachucho marine protected area (Cantabrian Sea). Dee -Sea Res. 106, 151–164. doi: 10.1016/j.dsr2.2013.12.012
GEBCO (2008). General Bathymetric Chart of the Oceans (GEBCO): The GEBCO_08 Grid. Available at: https://www.gebco.net/data_and_products/gridded_bathymetry_data/documents/gebco_08.pdf (accessed September, 2019).
Gerritsen, H., and Lordan, C. (2011). Integrating vessel monitoring systems (VMS) data with daily catch data from logbooks to explore the spatial distribution of catch and effort at high resolution. ICES J. Mar. Sci. 68, 245–252. doi: 10.1093/icesjms/fsq137
Gerritsen, H. D., and Lordan, C. (2014). Atlas of Commercial Fisheries Around Ireland, 2 Edn, Ireland: Marine Institute, 59.
Gregr, E. J., Palacios, D. M., Thompson, A., and Chan, K. M. A. (2019). Why less complexity produces better forecasts: an independent data evaluation of kelp habitat models. Ecography 42, 428–443. doi: 10.1111/ecog.03470
Guillaumont, B., Van Den Beld, I., Davies, J., and Bayle, C. (2012). Habitats particuliers des biocénoses du bathyal et de l’abyssal. Sous-région marine Golfe de Gascogne. Evaluation Initiale DCSMM. MEDDE, AAMP, Ifremer, Ref. DCSMM/EI/EE/GDG/29/2012. 10. Available at: https://www.academia.edu/1864493/Habitats_particuliers_des_bioc%C3%A9noses_du_bathyal_et_de_labyssal_SRM_GDG (accessed September, 2019).
Guillaumont, B., Van den Beld, I. M. J., Davies, J. S., Bayle, C., Bourillet, J.-F., and De Mol, L. (2011). Vulnerable Marine Ecosystems of the Bay of Biscay (NE Atlantic). The Geohab 2011 conference, Marine Geological and Biological Habitat Mapping, 3-6 May. Special Issue 1 Bulletin of the Geological Society of Finland. Espoo: Geological Survey of Finland. 46. Available at: http://www.geologinenseura.fi/bulletin/Special_Issue_1_2011/Bulletin_Abstrakt2011_netti.pdf (accessed September, 2019).
Guisan, A., and Zimmermann, N. E. (2000). Predictive habitat distribution models in ecology. Ecol. Model. 135, 147–186. doi: 10.1016/S0304-3800(00)00354-9
Hanski, I., and Gilpin, M. (1991). Metapopulation dynamics: brief history and conceptual domain. Biol. J. Linnean Soc. 42, 3–16. doi: 10.1111/j.1095-8312.1991.tb00548.x
Hedgecock, D., Barber, P. H., and Edmands, S. (2007). Genetic approaches to measuring connectivity. Oceanography 20, 70–79. doi: 10.5670/oceanog.2007.30
Hendry, K. R., Cassarino, L., Bates, S. L., Culwick, T., Frost, M., Goodwin, C., et al. (2019). Silicon isotopic systematics of deep-sea sponge grounds in the North Atlantic. Q. Sci. Rev. 210, 1–14. doi: 10.1016/j.quascirev.2019.02.017
Hilário, A., Metaxas, A., Gaudron, S. M., Howell, K. L., Mercier, A., Mestre, N. C., et al. (2015). Estimating dispersal distance in the deep sea: challenges and applications to marine reserves. Front. Mar. Sci. 2:6. doi: 10.3389/fmars.2015.00006
Hinrichsen, H.-H., Lehmann, A., Petereit, C., Nissling, A., Ustups, D., Bergström, U., et al. (2016). Spawning areas of eastern baltic cod revisited: using hydrodynamic modelling to reveal spawning habitat suitability, egg survival probability, and connectivity patterns. Prog. Oceanog. 143, 13–25. doi: 10.1016/j.pocean.2016.02.004
Howell, K. L., Holt, R., Pulido Endrino, I., and Stewart, H. (2011). When the species is also a habitat: comparing the predictively modelled distributions of Lophelia pertusa and the reef habitat it forms. Biol. Conserv. 144, 2656–2665. doi: 10.1016/j.biocon.2011.07.025
Howell, K. L., Huvenne, V., Piechaud, N., Robert, K., and Ross, R. E. (2014). Analysis of Biological Data From the JC060 Survey of Areas of Conservation Interest in Deep Waters off North and West Scotland. JNCC Report No. 528. Peterborough: JNCC.
Howell, K. L., Piechaud, N., Downie, A. L., and Kenny, A. (2016). The distribution of deep-sea sponge aggregations in the North Atlantic and implications for their effective spatial management. Deep Sea Res. Part I Oceanogr. Res. Pap. 115, 309–320. doi: 10.1016/j.dsr.2016.07.005
Inglis, G. J., Hurren, H., Oldman, J., and Haskew, R. (2006). Using habitat suitability index and particle dispersion models for early detection of marine invaders. Ecol. Appl. 16, 1377–1390. doi: 10.1890/1051-0761(2006)016%5B1377:uhsiap%5D2.0.co;2
James, M., Polton, J., Brereton, A., Howell, K., Nimmo-Smith, A., and Knights, A. (2019). Reverse-engineering field-derived vertical distribution profiles to infer larval swimming behaviours. Proc. Natl. Acad. Sci. U.S.A. 116, 11818–11823. doi: 10.1073/pnas.1900238116
Jones, G. P., Srinivasan, M., and Almany, G. R. (2007). Population connectivity and conservation of marine biodiversity. Oceanography 20, 100–111. doi: 10.5670/oceanog.2007.33
Kent, W. S. (1870). On the “HEXACTINELLIDÆ”, or hexradiate spiculed siliceous sponges taken in the ‘Norna’ expedition off the coast of spain and portugal. with description of new species, and revision of the order. Mon. Micros. J. 4, 241–252. doi: 10.1111/j.1365-2818.1870.tb01123.x
Kritzer, J. P., and Sale, P. F. (2004). Metapopulation ecology in the sea: from Levins’ model to marine ecology and fisheries science. Fish Fish. 5, 131–140. doi: 10.1111/j.1467-2979.2004.00131.x
Le Corre, N., Pepin, P., Han, G., Ma, Z., and Snelgrove, P. V. R. (2019). Assessing connectivity patterns among management units of the newfoundland and labrador shrimp population. FIrsheries Oceanogr. 28, 183–202. doi: 10.1111/fog.12401
Levin, L. A. (2006). Recent progress in understanding larval dispersal: new directions and digressions. Integr. Comp. Biol. 46, 282–297. doi: 10.1093/icb/icj024
Levins, R. (1969). Some demographic and genetic consequences of environmental heterogeneity for biological control. Bull. Entomol. Soc. Am. 15, 237–240. doi: 10.1093/besa/15.3.237
Leys, S. P., and Ereskovsky, A. V. (2006). Embryogenesis and larval differentiation in sponges. Can. J. Zool. 84, 262–287. doi: 10.1139/Z05-170
Liu, C., Berry, P. M., Dawson, T. P., and Pearson, R. G. (2005). Selecting thresholds of occurrence in the prediction of species distributions. Ecography 28, 385–393. doi: 10.1111/j.0906-7590.2005.03957.x
Lobo, J. M., Jiménez-Valverde, A., and Real, R. (2008). AUC: a misleading measure of the performance of predictive distribution models. Global Ecol. Biogeogr. 17, 145–151. doi: 10.1111/j.1466-8238.2007.00358.x
Maldonado, M., Carmona, M. C., Velásquez, Z., Puig, A., Cruzado, A., López, A., et al. (2005). Siliceous sponges as a silicon sink: an overlooked aspect of benthopelagic coupling in the marine silicon cycle. Limnol. Oceanogr. 50, 799–809. doi: 10.4319/lo.2005.50.3.0799
Martin, C. S., Giannoulaki, M., De Leo, F., Scardi, M., Salomidi, M., Knittweis, L., et al. (2014). Coralligenous and maërl habitats: predictive modelling to identify their spatial distributions across the Mediterranean Sea. Sci. Rep. 4:5073. doi: 10.1038/srep05073
Mattio, L., Zubia, M., Loveday, B., Crochelet, E., Duong, N., Payri, C. E., et al. (2013). Sargassum (Fucales, Phaeophyceae) in mauritius and réunion, western Indian Ocean: taxonomic revision and biogeography using hydrodynamic dispersal models. Phycologia 52, 578–594. doi: 10.2216/13-150.1
McIntyre, F. D., Drewery, J., Eerkes-Medrano, D., and Neat, F. C. (2016). Distribution and diversity of deep-sea sponge grounds on the Rosemary Bank Seamount, NE Atlantic. Mar. Biol. 163:143. doi: 10.1007/s00227-016-2913-z
Metaxas, A., and Saunders, M. (2009). Quantifying the “bio-” components in biophysical models of larval transport in marine benthic invertebrates: advances and pitfalls. Biol. Bull. 216, 257–272. doi: 10.1086/BBLv216n3p257
Mora, C., Treml, E. A., Roberts, J., Crosby, K., Roy, D., and Tittensor, D. P. (2011). High connectivity among habitats precludes the relationship between dispersal and range size in tropical reef fishes. Ecography 35, 89–96. doi: 10.1111/j.1600-0587.2011.06874.x
Müller, M., Haak, H., Jungclaus, J. H., Sündermann, J., and Thomas, M. (2010). The effect of ocean tides on a climate model simulation. Ocean Model. 35, 304–313. doi: 10.1016/j.ocemod.2010.09.001
Neat, F. C., Jamieson, A. J., Stewart, H. A., Narayanaswamy, B. E., Collie, N., Stewart, M., et al. (2019). Visual evidence of reduced seafloor conditions and indications of a cold-seep ecosystem from the Hatton–Rockall basin (NE Atlantic). J. Mar. Biol. Assoc. U.K. 99, 271–277. doi: 10.1017/S0025315418000115
North, E. W., Schlag, Z., Hood, R. R., Li, M., Zhong, L., Gross, T., et al. (2008). Vertical swimming behavior influences the dispersal of simulated oyster larvae in a coupled particle-tracking and hydrodynamic model of Chesapeake Bay. Mar. Ecol. Progress Series 359, 99–115. doi: 10.3354/meps07317
OSPAR, (2010). Background Document for Deep-Sea Sponge Aggregations. OSPAR Biodiversity and Ecosystems Series 47. Available at: https://www.ospar.org/documents?d=7234 (accessed August 2019).
Paris, C. B., Helgers, J., van Sebille, E., and Srinivasan, A. (2013). Connectivity modeling system: a probabilistic modeling tool for the multi-scale tracking of biotic and abiotic variability in the ocean. Environ. Mod. Softw. 42, 47–54. doi: 10.1016/j.envsoft.2012.12.006
Phillips, S. J., Anderson, R. P., and Shapire, R. E. (2006). Maximum entropy modeling of species geographic distributions. Ecol. Mod. 190, 231–259. doi: 10.1016/j.ecolmodel.2005.03.026
Phillips, S. J., Dudík, M., and Schapire, R. E. (2004). “A Maximum Entropy Approach to Species Distribution Modeling,” in Proceedings of the Twenty-First International Conference on Machine Learning, (New York, NY: ACM), 655–662.
Rice, A. L., Thurston, M. H., and New, A. L. (1990). Dense aggregations of a hexactinellid sponge, Pheronema carpenteri, in the Porcupine Seabight (northeast Atlantic Ocean), and possible causes. Prog. Oceanog. 24, 179–196. doi: 10.1016/0079-6611(90)90029-2
Roberts, J. J., Best, B. D., Dunn, D. C., Treml, E. A., and Halpin, P. N. (2010). Marine geospatial ecology tools: an integrated framework for ecological geoprocessing with ArcGIS, Python, R, MATLAB, and C++. Environ. Mod. and Softw. 25, 1197–1207. doi: 10.1016/j.envsoft.2010.03.029
Ross, R. E., and Howell, K. L. (2013). Use of predictive habitat modelling to assess the distribution and extent of the current protection of ‘listed’ deep-sea habitats. Divers. Distrib. 19, 433–445. doi: 10.1111/ddi.12010
Ross, R. E., Nimmo-Smith, W. A. M., and Howell, K. L. (2016). Increasing the depth of current understanding: sensitivity testing of deep-sea larval dispersal models for ecologists. PLoS One 11:e0161220. doi: 10.1371/journal.pone.0161220
Ross, R. E., Nimmo-Smith, W. A. M., and Howell, K. L. (2017). Towards ‘ecological coherence’: assessing larval dispersal within a network of existing marine protected areas. Deep Sea Res. Part I 126, 128–138. doi: 10.1016/j.dsr.2017.06.004
Ross, R. E., Nimmo-Smith, W. A. M., Torres, R., and Howell, K. L. (2019). Modelling marine larval dispersal: a cautionary deep-sea tale for ecology and conservation. Boirxiv
Ross, L. K., Ross, R. E., Stewart, H. A., and Howell, K. L. (2015). The influence of data resolution on predicted distribution and estimates of extent of current protection of three ‘listed’ deep-sea habitats. PLoS One 10:e0140061. doi: 10.1371/journal.pone.0140061
Sale, P. F., Cowen, R. K., Danilowicz, B. S., Jones, G. P., Kritzer, J. P., Lindeman, K. C., et al. (2005). Critical science gaps impede use of no-take fishery reserves. Trends Ecol. Evol. 20, 74–80. doi: 10.1016/j.tree.2004.11.007
Simpson, T. L., and Fell, P. E. (1974). Dormancy among the porifera: gemmule formation and germination in fresh-water and marine sponges. Trans. Am. Microsc. Soc. 93, 544–577. doi: 10.2307/3225157
Skov, H., Humphreys, E., Garthe, S., Geitner, K., Grémillet, D., Hamer, K. C., et al. (2008). Application of habitat suitability modelling to trackingdata of marine animals as a means of analysing their feeding habitats. Ecol. Mod. 212, 504–512. doi: 10.1016/j.ecolmodel.2007.11.006
Sponaugle, S., and Cowen, R. K. (2019). “Coral Ecosystem Connectivity Between Pulley Ridge and the Florida Keys,” in Mesophotic Coral Ecosystems. Coral Reefs of the World, Vol. 12, eds Y. Loya, K. Puglise, and T. Bridge, (Switzerland: Springer), 897–907. doi: 10.1007/978-3-319-92735-0_46
Sullivan, M. J. P., Davies, R. G., Reino, L., and Franco, A. M. A. (2012). Using dispersal information to model the species–environment relationship of spreading non-native species. Methods Ecol. Evol. 3, 870–879. doi: 10.1111/j.2041-210X.2012.00219.x
Teixidó, N., Gili, J.-M., Uriz, M.-J., Gutt, J., and Arntz, W. E. (2006). Observations of asexual reproductive strategies in Antarctic hexactinellid sponges from ROV video records. Deep Sea Res. II 53, 972–984. doi: 10.1016/j.dsr2.2006.02.008
Thorrold, S. R., Zacherl, D. C., and Levin, L. A. (2007). Population connectivity and larval dispersal using geochemical signatures in calcified structures. Oceanography 20, 80–89. doi: 10.5670/oceanog.2007.31
Thuiller, W., Pollock, L. J., Gueguen, M., and Münkemüller, T. (2015). From species distributions to meta-communities. Ecol. Lett. 18, 1321–1328. doi: 10.1111/ele.12526
Treml, E. A., Halpin, P. N., Urban, D. L., and Pratson, L. F. (2008). Modeling population connectivity by ocean currents, a graph-theoretic approach for marine conservation. Landsc. Ecol. 23, 19–36. doi: 10.1007/s10980-007-9138-y
Vieira, R. P. (2017). Functioning and vulnerability of continental slope ecosystems: combining stable isotope and visual survey approaches. Ph.D. Thesis, University of Southampton: Southampton, 158.
Werner, F. E., Cowen, R. K., and Paris, C. B. (2007). Coupled biological and physical models: present capabilities and necessary developments for future studies of population connectivity. Oceanography 20, 54–69. doi: 10.5670/oceanog.2007.29
Wood, S., Baums, I. B., Paris, C. B., Ridgwell, A., Kessler, W. S., and Hendy, E. J. (2016). El Niño and coral larval dispersal across the eastern pacific marine barrier. Nat. Commun. 7:12571. doi: 10.1038/ncomms12571
Wyville Thomson, C. (1869). On holtenia, a genus of vitreous sponges. Proc. R. Soc. Lond. 18, 114–122. doi: 10.1098/rspl.1869.0010
Yates, K. L., Bouchet, P. J., Caley, M. J., Mengersen, K., Randing, C. F., Parnell, S., et al. (2018). Outstanding challenges in the transferability of ecological models. Tends Ecol. Evol. 33, 790–802. doi: 10.1016/j.tree.2018.08.001
Yearsley, J. M., and Sigwart, J. D. (2011). Larval transport modeling of deep-sea invertebrates can aid the search for undiscovered populations. PLoS One 6:e23063. doi: 10.1371/journal.pone.0023063
Ypma, S. L., Brüggemann, N., Georgiou, S., Spence, P., Dijkstra, H. A., Pietrzak, J. D., et al. (2019). Pathways and watermass transformation of Atlantic water entering the nordic seas through denmark strait in two high resolution ocean models. Deep Sea Res. Part I 145, 59–72. doi: 10.1016/j.dsr.2019.02.002
Keywords: Pheronema carpenteri, deep sea sponge aggregations, dispersal model, habitat suitability model, vulnerable marine ecosystem, connectivity, metapopulation
Citation: Ross RE, Wort EJG and Howell KL (2019) Combining Distribution and Dispersal Models to Identify a Particularly Vulnerable Marine Ecosystem. Front. Mar. Sci. 6:574. doi: 10.3389/fmars.2019.00574
Received: 14 June 2019; Accepted: 29 August 2019;
Published: 18 September 2019.
Edited by:
Vincent Lecours, University of Florida, United StatesReviewed by:
Lisa Ann Levin, University of California, San Diego, United StatesErik Simon-Lledó, University of Southampton, United Kingdom
Copyright © 2019 Ross, Wort and Howell. This is an open-access article distributed under the terms of the Creative Commons Attribution License (CC BY). The use, distribution or reproduction in other forums is permitted, provided the original author(s) and the copyright owner(s) are credited and that the original publication in this journal is cited, in accordance with accepted academic practice. No use, distribution or reproduction is permitted which does not comply with these terms.
*Correspondence: Rebecca E. Ross, Rebecca.Ross@hi.no