- 1Applied Physics Laboratory, University of Washington, Seattle, WA, United States
- 2Cooperative Institute for Research in Environmental Sciences, University of Colorado, Boulder, Boulder, CO, United States
- 3Earth Systems Research Laboratory, National Oceanographic and Atmospheric Administration, Boulder, CO, United States
- 4International Arctic Research Center, University of Alaska Fairbanks, Fairbanks, AK, United States
- 5Department of Geology and Geophysics, Yale University, New Haven, CT, United States
- 6British Antarctic Survey, Cambridge, United Kingdom
- 7Nansen Environmental and Remote Sensing Center, Bergen, Norway
- 8Center for Ocean-Atmospheric Prediction Studies, Florida State University, Tallahassee, FL, United States
- 9Norwegian Polar Institute, Fram Centre, Tromsø, Norway
- 10Center for Environmental Science, University of Maryland, Solomons, MD, United States
- 11Korean Polar Research Institute, Incheon, South Korea
- 12Alaska Ocean Observing System, Anchorage, AK, United States
- 13Oden Institute, The University of Texas at Austin, Austin, TX, United States
- 14Alfred-Wegener-Institut Helmholtz-Zentrum für Polar- und Meeresforschung, Bremerhaven, Germany
- 15Partnership for Observation of the Global Oceans, Plymouth Marine Laboratory, Plymouth, United Kingdom
- 16Physical Oceanography Division, Atlantic Oceanographic and Meteorological Laboratory, National Oceanic and Atmospheric Administration, Miami, FL, United States
- 17Cooperative Institute for Marine and Atmospheric Studies, University of Miami, Miami, FL, United States
- 18Institute of Oceanology, Polish Academy of Sciences, Sopot, Poland
- 19Department of Meteorology and Bolin Centre for Climate Research, Stockholm University, Stockholm, Sweden
- 20Institute of Geophysics and Planetary Physics, Scripps Institution of Oceanography, La Jolla, CA, United States
- 21Marine Biogeochemistry and Oceanography, Norwegian Institute for Water Research, Oslo, Norway
- 22Bjerknes Centre for Climate Research, University of Bergen, Bergen, Norway
- 23Graduate School of Oceanography, The University of Rhode Island, Narragansett, RI, United States
- 24Oceanography and Climate, Institute of Marine Research, Bergen, Norway
Rapid Arctic warming drives profound change in the marine environment that have significant socio-economic impacts within the Arctic and beyond, including climate and weather hazards, food security, transportation, infrastructure planning and resource extraction. These concerns drive efforts to understand and predict Arctic environmental change and motivate development of an Arctic Region Component of the Global Ocean Observing System (ARCGOOS) capable of collecting the broad, sustained observations needed to support these endeavors. This paper provides a roadmap for establishing the ARCGOOS. ARCGOOS development must be underpinned by a broadly endorsed framework grounded in high-level policy drivers and the scientific and operational objectives that stem from them. This should be guided by a transparent, internationally accepted governance structure with recognized authority and organizational relationships with the national agencies that ultimately execute network plans. A governance model for ARCGOOS must guide selection of objectives, assess performance and fitness-to-purpose, and advocate for resources. A requirements-based framework for an ARCGOOS begins with the Societal Benefit Areas (SBAs) that underpin the system. SBAs motivate investments and define the system’s science and operational objectives. Objectives can then be used to identify key observables and their scope. The domains of planning/policy, strategy, and tactics define scope ranging from decades and basins to focused observing with near real time data delivery. Patterns emerge when this analysis is integrated across an appropriate set of SBAs and science/operational objectives, identifying impactful variables and the scope of the measurements. When weighted for technological readiness and logistical feasibility, this can be used to select Essential ARCGOOS Variables, analogous to Essential Ocean Variables of the Global Ocean Observing System. The Arctic presents distinct needs and challenges, demanding novel observing strategies. Cost, traceability and ability to integrate region-specific knowledge have to be balanced, in an approach that builds on existing and new observing infrastructure. ARCGOOS should benefit from established data infrastructures following the Findable, Accessible, Interoperable, Reuseable Principles to ensure preservation and sharing of data and derived products. Linking to the Sustaining Arctic Observing Networks (SAON) process and involving Arctic stakeholders, for example through liaison with the International Arctic Science Committee (IASC), can help ensure success.
Introduction
Motivation
Nowhere on Earth are the effects of climate change more apparent than in the Arctic. Long-term temperature records clearly show that the region is warming at more than double the global average (Richter-Menge et al., 2018; Box et al., 2019), an effect known as Arctic amplification. The rapid warming, identified in early climate models (e.g., Manabe and Stouffer, 1980), impacts all areas of the Arctic, from the tundra to the highest mountains, from to the deepest part of the Arctic Ocean through to the upper levels of the atmosphere (AMAP, 2017). The speed of these changes is particularly worrying, as most have occurred within a generation, thus making adaptation for the people, animals and plants that live in the region particularly challenging (Crépin et al., 2017).
It is fair to say that the Arctic marine environment has borne the brunt of these changes. For example, a key indicator of climate change, summertime sea ice extent, has declined substantially. Satellite measurements have revealed that sea ice extent has declined by roughly a half, due to melting and export, since the late 1970s; with the 12 lowest minimum sea ice extents all occurring in the last 12 years (Stroeve and Notz, 2018). The remaining ice is thinner (Lindsay and Schweiger, 2015) and more mobile (e.g., Rampal et al., 2011), and the area of thick multiyear ice has significantly reduced (Kwok, 2018). The Arctic is rapidly moving toward a seasonal ice cover.
Regionally, a warmer Arctic will accelerate sea ice loss, increase coastal erosion, alter ecosystem dynamics, increase glacial runoff and enhance permafrost decay, thus impacting the people (and infrastructure) that rely on the Arctic marine environment for their livelihood (AMAP, 2017). These changes are setting off complex chain reactions that have far-reaching environmental, social and economic impacts extending far beyond the region. A recent socio-economic analysis, performed within the EU funded ICE-ARC (Ice, Climate, Economics- Arctic Research on Change) program, calculated that compared with previous estimates, acceleration of climate change driven by thawing Arctic land permafrost and melting sea ice and land snow could cause up to USD $70 trillion worth of extra economic losses globally over the next three centuries under the levels of mitigation ambition consistent with the current national pledges, known as Nationally Determined Contributions (NDCs) (total climate change cost of this scenario: $1400 trillion). Importantly, their analysis revealed that if global warming is limited to 1.5°C in 2100 in line with the Paris Climate Agreement, the additional cost will be reduced to $25 trillion (total climate change cost of this scenario: $600 trillion) (Yumashev et al., 2019).
However, because our projected greenhouse gas emissions path is not clear, the extent and impact of future changes in the Arctic marine environment remain uncertain. At present the most likely scenario is projected to cause a global average warming of 3.0°C above pre-industrial temperatures by 2100, and very likely an ice-free summer Arctic before the mid-century (Sigmond et al., 2018). If global average temperatures stabilize at 1.5°C above the pre-industrial levels, as stipulated in the Paris Agreement, the chances for an ice-free summer are predicted to be quite low, about 2.5% (Jahn, 2018). It is important to realize that even under a 1.5°C warmer world the Arctic temperatures will rise up to 5°C (Schlosser et al., 2016).
Understanding the full extent of changes underway, predicting their impacts, and positioning to exploit new opportunities requires a sustained effort to collect and interpret a great volume of highly diverse information. This paper aims to provide a roadmap for the complex and daunting task of establishing the ARCGOOS. Efforts to define and develop an inclusive ARCGOOS have been ongoing. In 2006, a report from the US National Academy of Sciences’ Polar Research Board entitled, ‘Toward an Integrated Arctic Observing Network,’ (National Research Council [NRC], 2006) recommended that an Arctic observing network should be initiated, for which a sustained observing system for the Arctic Ocean would fulfill the critical marine component of the envisioned linked system of observing systems. Since then the concept has been refined through the bi-yearly Arctic Observing Summit (AOS) workshops and reports (Murray et al., 2018). The AOS is an important planning activity that complements ongoing efforts of the SAON process, a multi-governmental body that has a joint mandate from the Arctic Council and the IASC. The need for an internationally coordinated observing system in the Arctic Ocean was stressed at the highest level in 2016 and 2018 at the first and second “Arctic Science Ministerials” respectively (German Arctic Office., 2019). The output from these high level discussions focused in part on the grand challenge of coordinating and advancing Arctic observing, with a recognition of the role SAON might play as a regional facilitator.
In summary, a successful ARCGOOS needs the cooperation of a broad range of experts including different scientific disciplines, economic sectors and society, and indigenous peoples. An ARCGOOS that is co-designed with multiple partners and user needs in mind will have the greatest likelihood of long-term sustainability, usability, and relevance. A recent collaborative effort, the IAOAF (Science, and Technology Policy Institute [STPI], and Sustaining Arctic Observing Networks [SAON], 2017) provides an effective means to comprehensively assess the impact of an ARCGOOS across users from an Arctic-specific set of SBAs. Areas include regionally relevant considerations like “Accessible, Available, and Sustainable Food,” “Disaster Mitigation,” and “Maintain areas of cultural significance in the Arctic.” This multi-faceted cooperation is essential to ensure ARCGOOS plays a crucial role in the sustainable development of the Arctic in accordance with the United Nations 17 Sustainable Development Goals (SDGs), and importantly to inform scientific, economic, environmental and societal policies. An ARCGOOS must be flexible to respond to the changing needs and conditions of the region and provide the needed information for Arctic peoples and wider society, science, the private sector and decision makers. Whilst the development of an ARCGOOS is moving forward, a financial commitment remains elusive.
Objectives
This paper advocates a requirements-based approach for developing an ARCGOOS. Successful development and sustained operation of an ARCGOOS requires a broadly supported framework within which essential observational targets and approaches for observing them will be identified, the appropriate system metrics are monitored and integrated data delivery systems developed. Through observational targets, like the EOVs of the FOO (Lindstrom et al., 2012), relevant observing actions from contributing entities can be aligned, leveraged and made accessible. Observing targets and approaches are both intimately tied to the intended benefits of such an observing system. Motivated by the need to understand ecosystem response to sea ice retreat and other Arctic environmental change, and the potential implications for food security, the PAG (see section “Examples”) coordinates the DBO (Moore and Grebmeier, 2018) and Pacific Arctic Climate Ecosystem Observatory. These two systems provide contemporary examples of sustained international observing in which a diverse, lightly coordinated consortium aligns around the consistent collection of a suite of essential measurements at mutually agreed-upon locations. The governance and operating structures employed by the consortium enables broad international collaboration capable of sustaining measurements over extended, decadal timescales and can be readily scaled to tackle new regions and measurements.
Design of an ARCGOOS can be optimized through application of quantitative approaches – defined as mathematically rigorous assessments of impact, redundancy, representativeness, and efficiency of existing, planned or hypothetical observational networks using numerical or statistical models. These quantitative approaches will help in determining observational sites and strategies (where, when, and for how long to conduct measurements, what variables are the most critical/influential/sensitive to observe, etc.). In environments undergoing rapid change, or for more complicated predictand variables, for example parameters integrating biophysical measures of food security, quantitative design approaches likely have to be refined through expert input.
The design and implementation tradeoffs of the ARCGOOS must be systematically evaluated in a transparent and broadly acceptable manner. It is thus critical to have an accepted benefit structure and quantitative framework for evaluating ARCGOOS impacts that incorporates a broad range of expertise, including science, decision and policy makers. The IAOAF (Science, and Technology Policy Institute [STPI], and Sustaining Arctic Observing Networks [SAON], 2017), which has identified 12 Arctic-specific SBAs supported (Figure 1) by 160+ Key Objectives, provides a valuable starting point for an ARCGOOS framework and assessment system, and it will elucidate variables of highest common dependence. The range of interests so identified will impact how those variables are observed and data are shared.
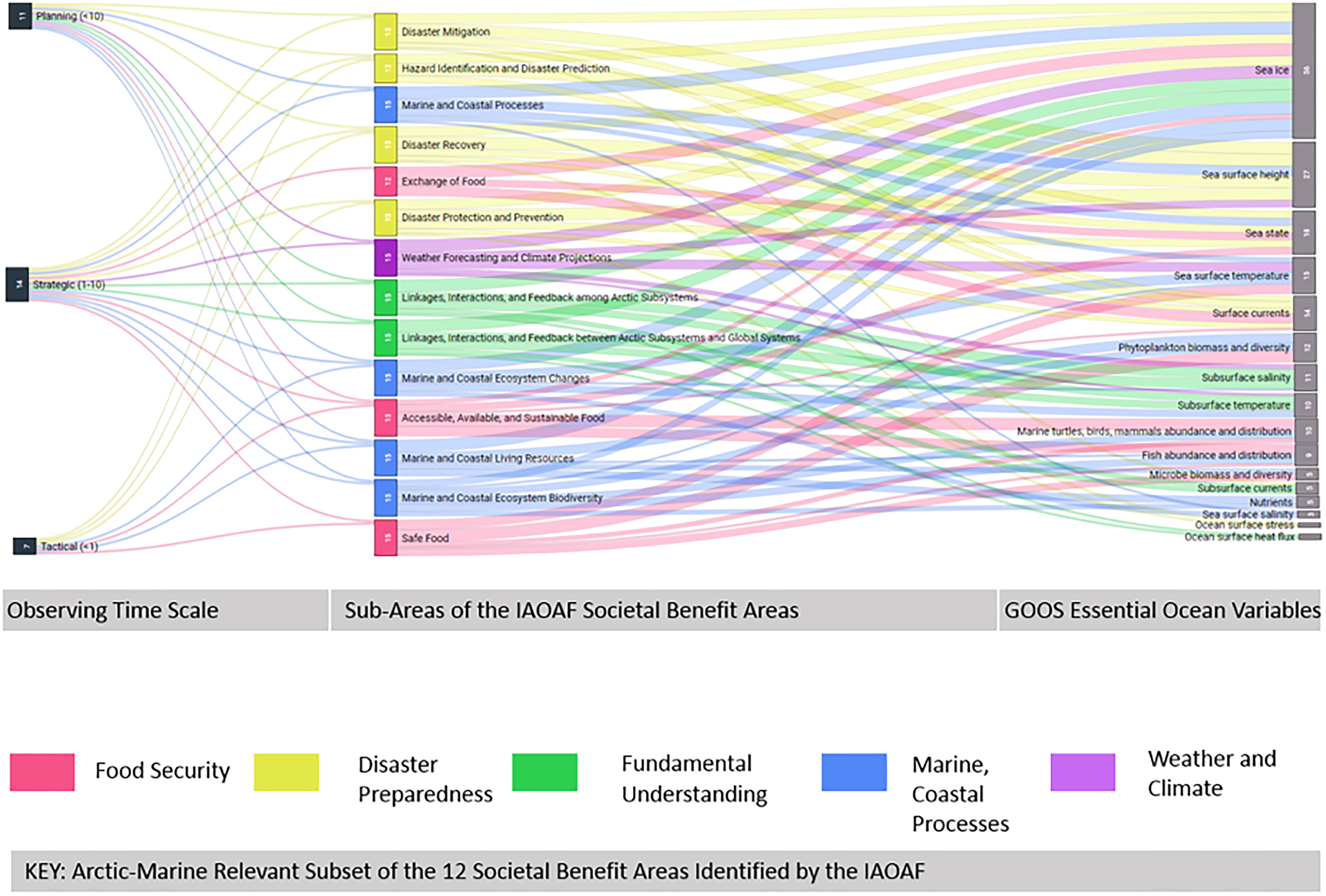
Figure 1. In this example, temporal scales for observing information (left) are related to IAOAF Societal Benefit Sub-Areas of 5 ocean-relevant SBA’s (center) and in turn linked to GOOS EOV’s of highest impact to that topic. Through such relational mapping, the IAOAF can support the design of ARCGOOS through: (1) clarifying the user base for the observing system; (2) demonstrating variables of highest common dependence; (3) identifying the range of interests that will impact how those variables are observed and data is shared.
A successful framework for ARCGOOS needs to be led by an authoritative, international body or consortium of bodies with the mandate to coordinate observations at the intersection of Arctic and oceanic systems. Several bodies partially address such a mandate. For oceanic observing, the GOOS and the POGO each have meaningful roles to play. GOOS is a program executed by the IOC of the United Nations Educational, Scientific and Cultural Organization (UNESCO) with the mandate to sustain a coordinated set of ocean observations globally (Lindstrom et al., 2012). The European component of GOOS, EuroGOOS, founded in 1994, is an international association of 44 insitutitions from 18 countries coordinating ocean observations in five regional seas, including the European sector of the Arctic. POGO was founded in 1999 by directors of oceanographic institutions around the world as a forum to promote and advance the observation of the global ocean. POGO’s membership includes most of the world’s leading ocean science and technology institutions, whose expertise, experience and infrastructure provide the unique and long term capability to design, build, operate and innovate the GOOS. For Arctic observing, SAON has the joint mandate of Arctic Council and the IASC. SAON has been invited by the GRAs Council to participate as the Arctic representative and has been recognized as a Community Activity “Arctic GEOSS” under the GEO. As with all ocean/ice observing, transnational partnership and transnational access are critical. The 2017 Arctic Council “Agreement on Enhancing International Arctic Scientific Cooperation” should prove useful.
Scope
The SBAs dictate diverse, overlapping needs for a sustained Arctic observing system, including documenting Arctic environmental change, understanding the Arctic Ocean’s role in climate, supporting planning and decision making, and providing near real-time support for operators in the Arctic (Figure 1, middle column). These needs strongly constrain system design by defining spatial and temporal scope and resolution, and by dictating the speed at which data and products must be delivered.
A first step toward organizing these requirements comes in recognizing that there are three overlapping domains of information needs: 1) long-range planning and policy; 2) strategy; and 3) tactics (Figure 1, left column). Long-range planning concerns the use of science to inform governance and management of the natural and built environment and human communities on decadal timescales, and thus focuses on long-term sustainability through planning for infrastructure, activity, regulation and environmental protection. Observations for policy must characterize environmental change and support model-based prediction. These needs prioritize measurements with large geographic scope (national to pan-Arctic) sustained over the long, decadal timescales needed to resolve climate variability. Scientific rigor, accuracy and precision are important considerations due to the subtle nature of some climate signals. Successful collection of sustained, climate-quality measurements requires long-term planning (National Academies of Sciences Engineering Medicine [NASEM], 2017). The distributed nature of these observations will place many sites far from areas of human activity, presenting logistical challenges that will limit the range of variables that can be observed. Policy frameworks respond slowly to new information, making data updates at annual or longer timeframes sufficient. Long records hold great value in the policy domain, prioritizing collection of decadal time series of consistent, high-quality measurements with broad geographic distribution.
Strategy involves the use of environmental data to support seasonal to decadal planning by governments, industry, science and communities. Here, an Arctic observing system might support activities such as decision-making surrounding trans-Arctic shipping, resource extraction or the design of new vessels. Observations focus on regions of planned activity, and are thus limited in geographic coverage, but might still require data at regional or pan-Arctic scales to support numerical prediction. Data from these systems would also contribute to numerical prediction efforts. An understanding of synoptic-scale events can be important for robust decision making. For example, knowing both the statistics of internal waves and eddy variability and the physics that govern their generation and propagation can be important for planning resource extraction and designing the necessary offshore structures. The need for robust statistics makes long, sustained records valuable, while also driving the requirement for regional measurements that resolve synoptic scales of a few days and kilometers. Strategic decisions can unfold more rapidly than those in the policy domain, and thus require more rapid access to data, with updates at daily to annual intervals.
Tactics encompasses environmental/situational awareness and data collection in support of operational forecasting, informing decisions surrounding day-to-day activity. How far out onto the ice can I venture? What’s the most cost or time efficient route for my vessel? Data needs for any given application will be narrow in geographic and temporal coverage, focused on the time and place where activity unfolds. Rapid and robust data delivery and analysis is critical, as tactical information loses value quickly with the passage of time. All data and analysis products should be provided with ample metadata, including estimates of uncertainty, and documentation of processing and quality control.
This paper focuses on frameworks for systems aimed at “Planning/Policy” and “Strategy,” thus emphasizing the challenges associated with sustained observations over the larger temporal and spatial scales (years to decades, regional to pan-Arctic). The paper considers how such systems might be optimized to provide tactical information when feasible, and how frameworks might be engineered to more readily integrate these needs into the design process. It further recognizes that a focus on longer-term understanding will ultimately support the most effective use of tactical information.
A Path to an Arctic Ocean Observing System
The following sections describe a process (Figure 1) that begins with selecting a subset of SBAs (Figure 1, denoted by color) that require understanding and information to support long-term planning, policy making and strategy (section “Framework and Governance”). These requirements drive the selection of research questions and operational objectives (Figure 1, middle column), from which flow identification of EAVs (Figure 1, right column), along with a broad sense of the scope (Figure 1, left column) of the necessary observing effort (section “System Design”). Subsequent design efforts (section “System Optimization”) focus on understanding and optimizing the scope and scales of the observations needed to deliver the required information within specified uncertainties and representativeness. Implementation (see section “System Implementation”) considers the technologies and approaches that could be employed to deliver the measurements specified in the design phase.
The implementation of an ARCGOOS that covers the breadth of these three application domains amounts to more than mere incremental technological and methodological innovation, cross-linkage of system components, and response to new scientific discoveries. Rather, it represents a challenge that requires transformative approaches, integrating physical and biological marine sciences, engineering, computational, social and behavioral sciences, and other knowledge systems (traditional). Convergence of these methods (McNutt, 2017) has not been achieved yet at this scale, but could be possible in the Arctic, where connections between different disciplines, sectors and knowledge systems are possibly tighter than elsewhere on the globe (Young, 2009). However, such transformational, convergent approaches require highly collaborative efforts from the regional to the international level, sustained by resourcing mechanisms that transcend challenges at the national scale. These approaches have to inform scope, design and implementation of the ARCGOOS – kernels of which are apparent in some of the examples listed in section “Examples.”
Framework and Governance
Introduction
A sustainable ARCGOOS capable of advancing system-wide, collaborative sampling of the Arctic Ocean must be underpinned by a broadly endorsed framework grounded in: (i) high-level policy drivers (articulated as SBAs), and (ii) scientific and operational objectives that stem from these drivers. A framework provides a systems-level view of observing requirements, best practices and data delivery plans toward applications for which the observing system is intended. Requirements and best practices for system design, including platforms and instrumentation, data processing, quality control and long term preservation, as well as for dissemination strategies, derive from the scientific and operational objectives. This will provide a well-defined pathway toward implementation. Given the complexity of what a framework should accomplish, considerations for developing a successful observing framework for ARCGOOS will be discussed in the following.
First, the ARCGOOS requires an effective, internationally accepted governance structure with recognized authority building on the work of national agencies and marine institutions that ultimately execute observing network plans. Second, the ARCGOOS requires a clear framework for defining the scientific and operational objectives that support the selected SBAs. This requires a systems-level perspective to identify the necessary measurements (variables, scale and scope), and a methodology for prioritizing those measurements as a function of impact across the suite of objectives and SBAs. Given the vast number of potential scientific objectives and areas of interest in the Arctic marine system, the system-level view of the ARCGOOS framework should include a mechanism for weighing trade-offs based on importance, urgency and technical maturity. The framework should include a strategy to allow for the collaborative development of the most urgent set of requirements, while outlining a development pathway for less urgent requirements. It should include an evaluation process which allows the system to mature and change over time. Most urgently, the need for both governance and a framework need to be recognized and initiated by those in the best position to do so. This section explores each of these general aspects in greater detail, with specific recommendations for the ARCGOOS.
Governance and the SBA-Driven ARCGOOS
Governance guides the broad objectives of the ARCGOOS by identifying the most relevant policy mandates, as embodied by the SBAs, conducts ongoing evaluations of performance and fitness-to-purpose, and advocates for resources to support design and implementation. Given the fundamental importance of selecting SBAs, the ARCGOOS governance structure will need to identify and engage the bodies that have the most relevant policy mandate to lead efforts topically and regionally. It should further consider how these bodies are connected to the partners and resources needed to succeed with transnational implementation. Governance arrangements have implications for the observing framework that a network will use (e.g., extend an existing framework or create its own); the policy drivers that will guide observing priorities (e.g., climate-oriented versus operational); and the resource sharing schemes, including transnational access, data and cyberinfrastructure. Lastly, the governance structure must define clear lines of responsibility and processes for decision-making.
ARCGOOS sits at the confluence of many relevant governance schemes, including those of global actors (e.g., GOOS, POGO, GEO, WMO), thematic observing efforts (e.g., SCOR, Global Cryosphere Watch (GCW), GEO-CRI, Climate and Cryosphere (CliC), IASC), and plural regional, indigenous and national organizations. Achieving alignment between national, regional, thematic and existing global governance schemes is critical for all, and will influence the selection of policy drivers for the observing system. An example is whether the ARCGOOS should follow the SBAs outlined by GEO or refer to something more regionally specific like the IAOAF SBAs (Dobricic et al., 2018).
As the global coordinating body for ocean observing GOOS provides the mandate and framework under which ARCGOOS should operate. GOOS fosters the development of regional GOOS systems through GRAs. EuroGOOS, the European component of GOOS, contributes observations in the European Arctic with its Arctic Regional Ocean Observing System. POGO is an international non-governmental organization supporting the development of GOOS through innovation, advocacy and capacity development. POGO has recently been focusing on the challenges facing sustained Arctic observations, but needs to liaise with organizations and programs already active in the region to ensure that its activities are complementary.
The Global Climate Observing System (GCOS) is a United Nations-ratified program which regularly assesses the status of global climate observations and produces guidance for its improvement. GCOS is co-sponsored by the WMO, the Intergovernmental Oceanographic Commission of the United Nations Educational, Scientific and Cultural Organization (IOC-UNESCO), the United Nations Environment Programme (UN Environment), and the International Science Council (ISC). ARCGOOS will contribute to GCOS through its climate related objectives.
GEO is an intergovernmental organization that works to actively improve and coordinate global earth observing systems, and their connection to users, promoting broad, open data sharing to the benefit of society. ARCGOOS can connect to GEO through its initiatives such as the Cold Regions Initiative (GEO-CRI), Biodiversity Observations Network (GEOBON) and Blue Planet Initiative. ARCGOOS would clearly benefit from reviewing the overlapping policy drivers of these organizations, such as the UNFCCC and its Paris Agreement, the Sendai Framework for Disaster Reduction and the United Nations SDGs. It would also benefit from working with some or all to take advantage of their strong agreements for transnational cooperation and data sharing.
A number of existing regional and topical observing systems serve specific SBAs, providing examples of governance structures that should be accounted for in the development of the ARCGOOS. Examples of such systems include the International Arctic Buoy Program (IABP, perhaps the oldest international Arctic observing program), the FRAM, the Svalbard Integrated arctic Earth Observation System (SIOS), the PAG, the CBMP and the IOOS-AOOS. The Arctic Regional Ocean Observing System (Arctic ROOS1), under EuroGOOS, provides an example of formal collaboration between European marine institutions. These systems are well established and will provide building blocks for ARCGOOS.
Since the IPY, SAON has emerged with a governance mandate to coordinate Arctic observing. It was jointly initiated and later formalized by the Arctic Council and IASC through the 2011 Nuuk Declaration (Arctic Council, 2011). This mandate has been renewed and reinforced through the Joint Statement of the 2016 and the 2018 Arctic Science Ministerials2 yet SAON’s still-maturing strategy and lack of resources have limited its ability to develop clear direction for regional networks. SAON’s policy mandate comes from the Arctic Council, which has working groups focussing on climate and contaminants (AMAP), ecosystems and biodiversity (CAFF), marine activity (PAME), sustainability (SDWG) and more.
At the intersection of GOOS, GEO, POGO and SAON lies the strengths of long-standing intergovernmental agreements to collaboratively observe the global ocean and related systems, a consortium of ocean observing expertise, and strong regional policy drivers and engagement with indigenous communities with sovereign rights in the Arctic. In the same manner as the SOOS was developed jointly through the work of SCOR, the SCAR and POGO, ARCGOOS development should proceed under a consortium governance approach.
Framework Design
A framework for the ARCGOOS must support a system-level view of policy drivers, the resulting scientific challenges, essential variables and the interrelationships that bind them. SBAs are central to the ARCGOOS framework, motivating investment in an ARCGOOS and associated scientific efforts. The results of the “Impact assessment study on societal benefits of Arctic observing systems” (IMOBAR) project has demonstrated the economic value that accrues from Arctic observations in support of the IAOAF SBAs (Dobricic et al., 2018). Science objectives derive from the information needs of the selected SBAs. An effective ARCGOOS must be firmly grounded in science, with system design tailored to the identified science questions. EAVs can be identified as those that offer maximum impact, as measured by the number or urgency of science objectives and SBAs served, and feasibility, as measured by technological maturity and logistical constraints. System scope and temporal and spatial resolution requirements flow from design studies aimed at achieving efficient delivery of the required data. Data delivery systems should support the range of objectives that the framework identifies. The ARCGOOS framework should also provide processes for continued system evaluation, and evolution in response to changing science needs and introduction of new technologies.
A system-level view overcomes the difficulty of looking at pieces of the Arctic marine system in isolation, while not getting overwhelmed by the complexity of their relationships. This view should also be a starting point for identifying which aspects of the observing system have the greatest impact on the policy drivers. The SBA frameworks provide a mechanism to systematically assess the impact of different observing targets and develop a hierarchy of key observables – or essential variables – around which to organize.
Even as the ARCGOOS seeks alignment of relevant governance schemes, it should also strive to align with relevant global observing frameworks. From a practical standpoint, global frameworks have made tremendous progress and many national funding programs have developed to support them. The ARCGOOS could leverage this progress, along with existing international agreements for collaborative sampling and data dissemination. For example, the GOOS FOO is organized around EOVs. EOVs support the scientific objectives of the FOO while providing a systematic means to align and coordinate observing strategies. EOVs allow discrete aspects of the observing system to develop independently of one another, as they reach sufficient maturity. Other global essential variable systems like the GCOS ECVs, the GEOBON Essential Biodiversity Variables, or the WMO’s GCW observing specifications should be taken into consideration, leveraged and extended where possible.
If ARCGOOS is to follow the pathway of merging and extending existing essential variable frameworks, it would need to attend to two important considerations. First, global EOVs were identified and prioritized under globally relevant policy mandates. ARCGOOS should review and refine these policy mandates for Arctic relevance. As a result of the first Arctic Science Ministerial, SAON, in partnership with the STPI, jointly developed a key collaboration tool through the IAOAF. The IAOAF was developed through SAON partners, national, indigenous and non-governmental organizations, and articulated 12 Arctic-specific SBAs, supported by more than 160 key objectives for regional observing. While broad, it provides a critical starting point for bridging between global and regionally significant observing targets.
Second, Arctic regional climate and ecosystems themselves are unique and require specific observing strategies. Ice cover impedes satellite services for geopositioning and communication of underwater platforms, and threatens instruments at or near the ice-ocean interface. The Argo program (Riser et al., 2016), which revolutionized climate-scale observing in the ice-free oceans with an array of roughly 4000 profiling floats, has not yet gained traction in the Arctic because of its reliance on satellite services for data transfer and geolocation. Polar night limits the seasonal range of visible satellite imagery. Both sea and land ice play important roles in dynamic coastal ecosystems. The demanding environment, remoteness and operational challenges make logistics a stronger constraint than they might be in lower latitude oceans. This has produced a culture of shared logistics that can encourage interdisciplinary research and, in more recent years, has motivated accelerated efforts to employ autonomous platforms.
Strategy for Initiating a Framework
At the second Arctic Science Ministerial in Berlin (German Arctic Office., 2019), ministers from 24 countries emphasized the urgency of implementing sustained Arctic observing and pointed to SAON to demonstrate the necessary leadership. SAON’s Committee on Observing Networks (CON) is initiating a new task force to advance the application of IAOAF SBAs toward framework design. Recently, SAON was invited to participate in the GOOS GRAs, and POGO has become more engaged with SAON. As a follow up we recommend that SAON invites GOOS GRAs, GEOSS, WMO, and POGO to form an exploratory task team to make recommendations for Arctic Ocean Observing System. ARCGOOS could serve as an excellent focal point to follow up the implementation of the task team recommendations.
Requirements-Driven Identification of Essential ARCGOOS Variables
Applied within the context of the broad Arctic observing domains described above, and governed by the processes discussed in section “Framework and Governance,” the GOOS EOV approach provides a framework for guiding the selection of observables and scales to be addressed by the various components of an ARCGOOS. EAVs are selected for relevance to climate, operational services and ocean health, modulated by feasibility and cost effectiveness. Unique aspects of the Arctic Ocean and its role in global climate imprint on the shape of the ARCGOOS, while practical considerations, including logistics, resource availability, and sustainability place strong constraints on scope. While the ultimate selection and definition of EAVs, and the scales at which they must be measured, should flow from the identification of SBAs and the science questions they engender (section “Framework and Governance”), the GOOS EOVs provide a starting point for considering some of the ARCGOOS baseline variables and how they might be shaped by challenges and concerns particular to the Arctic.
To illustrate the flow that might lead from SBAs to the identification of EAVs, consider the example of Arctic heat and freshwater budgets. Several SBAs, including Fundamental Understanding of Arctic Systems, Environmental Quality, Food Security, Weather and Climate (all linked to the sustainable development of the region), motivate the need to understand and document the fate of Arctic Ocean heat and freshwater. The Arctic freshwater cycle plays an important role in modulating the Arctic energy balance and in global climate (e.g., Prowse et al., 2015; Carmack et al., 2016). Upper ocean freshwater modulates sea ice formation and melt by insulating ice from warmer waters below (e.g., Aagaard and Coachman, 1975) and, through this, impacts coupling between the upper ocean and local atmospheric forcing. Sea ice growth and melt are also fundamental components of the Arctic heat balance, which plays an important role in constraining meridional atmospheric energy transport (Nakamura and Oort, 1988). Seasonal changes in sea ice timing and extent directly influence marine ecosystems both through loss of habitat for ice-obligate species such as polar cod and ice seals, but also via changes in the timing and amount of primary productivity (e.g., Arrigo et al., 2008; MacIntyre et al., 2015). Arctic residents in turn have a subsistence reliance on local food sources (Eicken et al., 2009; Nilsson et al., 2013), the shifts in which impact both their food security and culture. Globally, the fisheries of the Bering and Barents Seas are highly productive and vulnerable to system-wide shifts (Hunt and Megrey, 2005; Wassmann, 2015). Measurements of ocean temperature, salinity and mass are fundamental to understanding in these areas, and thus have the broad impact that suggest them as EAVs.
Science and operational needs, derived from the SBAs, identify EAVs (Figure 1) and, in a broad sense, the scope and scale at which they must be measured. Continuing the example, documenting the fate of freshwater and heat will require maps of temperature and salinity suitable for quantifying storage and fluxes. Surrounded by land, the Arctic Ocean communicates with the Pacific and Atlantic Oceans through a handful of gateways, with additional inputs from net precipitation and riverine inflow. Estimates of heat and freshwater storage will require measurements suitable for mapping the interior of the ocean under the drifting sea ice, along with observations over the shallow shelves that account for over a third of the Arctic Ocean surface area and are the focus of most human activity. Technological and political challenges have limited access to some Arctic shelf systems, creating a critical gap in the observing system. Flux estimates will require sustained observations of temperature, salinity and velocity at the main ocean gateways (Bering, Fram and Davis Straits and the Barents Sea Opening), and in-situ recording of discharge rates and temperatures for the major rivers (Mackenzie, Yukon, Ob, Yenisey and Lena rivers), as well as quantification of sea ice-associated export fluxes in the North American and Eurasian Arctic and broad estimates of net precipitation. For this example, the SBAs point to science for long-term planning, policy and strategy, and thus longer timescales and sustained measurements over years to decades.
Applying a similar analysis systematically across multiple SBAs identifies a suite of EAVs along with usage patterns that can be interpreted as a measure of impact (Figure 1, right column). For this example, SBAs yield science and operational objectives, each of which points to a maximum of five dependent variables, specified in priority order. Impact can be gauged by the number of objectives served weighted by priority within each objective, with high-impact variables being candidates for EAVs. While illustrative of the process, this simplified evaluation does not capture some important factors that must be accounted for in a more thorough EAV analysis. Interdependencies can elevate the impact of some variables. For example, although sea ice ranks among the most impactful EAVs, understanding and predicting sea ice evolution requires a host of other measurements. These are termed ‘supporting variables’ in the GOOS EOV framework, and include subsurface temperature and salinity, radiative surface fluxes, surface air temperature, precipitation and wind velocity. EAV definitions would build on those created for GOOS EOVs, incorporating refinements specific to the needs of Arctic observing. Scope (Figure 1, left column) is not reflected in this analysis. The challenges associated with Arctic observing make weighting EAV candidates for technological readiness and logistical feasibility particularly important.
Taking into account the caveats articulated above, the simplified example plotted in Figure 1 identifies potential high-impact EAVs that include sea ice, sea surface height, sea state, phytoplankton, surface and subsurface temperature, salinity and ocean velocity. Although this subset is clearly incomplete, the example serves to illustrate how a requirements-driven framework might be used to trace and understand the impact of observations across multiple scientific and operational endeavors. This knowledge then informs planning and investment toward developing the ARCGOOS.
Overview of Arctic Marine System Observables
Ocean
The energy imbalance in Earth’s climate system is caused by rising concentrations of heat-trapping gases. About 93% of the energy imbalance accumulates in the ocean as increased ocean heat content (Cheng et al., 2019). Heat introduced by summertime solar warming, and through Pacific and Atlantic inflows, plays an increasingly important role in the extent and timing of sea ice growth and retreat (e.g., Carmack et al., 2015), while freshwater (salinity) forms a cold halocline that modulates contact between sea ice and the warmer, subsurface waters below (Aagaard and Coachman, 1975). To document changes in upper ocean heat content, stratification and the resulting impact on sea ice, water-column measurements must include in situ temperature and salinity profiles from the ocean surface through the warm core of the Atlantic Water Layer (at least to 500 m depth) with sufficient vertical resolution to quantify mixed-layer depth and the strength of the strong halocline stratification at the mixed layer base. Waters deeper than this change on timescales longer than interannual and deep measurements every several years will suffice.
Other high-priority water column observations might include dissolved oxygen, velocity, nutrients, bio-optical measurements (sensor-based proxies for phytoplankton) and seawater inorganic carbon chemistry (at least two of the four variables: dissolved inorganic carbon, total alkalinity, pCO2, and pH). Geochemical parameters (trace elements and isotopes – TEI) including chlorofluorocarbons (CFCs), sulfur hexafluoride (SF6), tritium, helium isotopes, stable isotopes of water and 14C integrate over large spatial and temporal scales, and can thus be useful for understanding water mass formation and movement in the Arctic Ocean. These TEI have been successfully used with the US GEOTRACES study and other studies to investigate water mass structure, pathways, and residence times. Recently, ‘ocean sound’ was approved as an EOV by GOOS. This is a highly relevant EOV to monitor for the Arctic region due to the increased human influence on the ocean acoustic environment.
High-priority sea surface measurements include sea state and sea level. Sea state observations might include remotely sensed and in situ measurement of surface waves and in situ observations of upper ocean velocity and turbulence. Sea level monitoring relies on a combination of remote sensing (satellite altimetry and gravimetry measurements; e.g., Armitage et al., 2016), bottom pressure and coastal tide gauges (Proshutinsky et al., 2004; Volkov and Pujol, 2012; Calafat et al., 2013; Peralta-Ferriz and Morison, 2014). Measurements of sea level provide information on ocean dynamics and thermohaline properties at weekly to interannual timescales as well as longer-term land ice (and snow) melt.
Sea Ice
Sea ice plays a central role in Arctic marine environments. Its presence constrains atmosphere-ocean interaction (e.g., Rösel et al., 2018), structures marine ecosystems (e.g., Nicolaus et al., 2012; Wassmann, 2015), and greatly affects human activities (Eicken et al., 2009; Andrews et al., 2017). Sea ice loss is both an indicator of broader, system-wide transformations (Polyakov et al., 2017; Wilkinson and Julienne, 2018) as well as a driver of change in many of the components of the Arctic system. In this context, concentration, thickness, roughness and mechanical characteristics, in addition to snow cover, optical and biogeochemical properties of the Arctic ice pack have been identified as high-priority variables. Reductions in sea ice extent (the 12 lowest minimum sea ice extents all occurred in the last 12 years, Stroeve and Notz, 2018) and thickness (Lindsay and Schweiger, 2015; Kwok, 2018) and the underlying changes in the heat and momentum budget call for detailed observations of ice thickness and snow depth at the basin-scale, but with a resolution sufficient to capture key processes, e.g., in the context of ice deformation or its evolution as a critical habitat (Post et al., 2013).
Recent advances in remote sensing, in particular in terms of radar and laser altimetry, and synthetic aperture radar (e.g., Markus et al., 2017; Kwok, 2018) have the potential to greatly improve our understanding of the transformation of the Arctic Ocean from a perennially to a seasonally ice-covered body of water. However, remote sensing, modeling, and prediction system development all require in situ observations for constraint and validation. This problem is exacerbated by the fact that our research understanding and model development are built on an Arctic formerly dominated by multi-year ice. Near-term correction of this paradigm is needed if we are to provide more accurate predictions on sea ice behavior and evolution on planning/policy to strategic to tactical timescales. For improving understanding and to be able to predict changes, the new conditions need more in situ and remote sensing observations, especially for the winter season from which relatively little data exist (Webster et al., 2018; Gerland et al., 2019). Satellite remote sensing provides good spatial and temporal coverage of the Arctic Ocean through long-term programs such as the Defense Meteorological Satellite Program (DMSP), the RADARSAT Constellation Mission and the Copernicus Sentinel program. Copernicus is the most ambitious satellite program today, with more than 10 satellites providing near real time data for environmental and climate monitoring, in particular ocean and sea ice observations. However, there are substantial gaps in in situ observations of Arctic sea ice variables such as sea ice thickness and snow cover, both in terms of coverage and longevity (Sandven et al., 2018).
The increase in human activity and potential for maritime disasters in the presence of ice (e.g., Barber et al., 2018), as well as the need to better understand potential restructuring of food webs as a result of sea ice changes, call for a new suite of sustained observations targeting biogeochemical variables and the coastal zone where much of human activity is concentrated and risks are most pronounced (Eicken and Mahoney, 2015). Much of the demand for these types of observations can be met through a combination of newly developed autonomous sensing systems (Whitlock, 2018) and community-based observations (Johnson et al., 2015).
Atmosphere
The atmosphere links all components of the Arctic climate system (ocean, ice, land, biology), driving changes within each of them, responding to these changes, and extending their influence across spatial areas as extensive as hemispheric. Understanding the complex, interconnected processes and exchanges of energy between the atmosphere and the underlying ocean, sea ice, and land surfaces plays a central role in the modulation of weather, the seasonal growth and decay of sea ice, and climate. Within the atmospheric column, transport of heat, humidity, aerosols and momentum are all important contributors in the generation of clouds with potential impacts on global circulation patterns governing regional climate.
Essential atmospheric parameters that need to be monitored to quantify the surface energy balance are atmosphere-ocean (-ice and -land) radiative and turbulent fluxes; these govern ocean and sea-ice dynamics and thermodynamics. At the same time, changes in ocean heat content and sea ice conditions alter the heat fluxes to the atmosphere. Atmosphere-sea ice-ocean fluxes of momentum and freshwater must also be quantified. For example, winds play a central role in driving oceanic mixing from above through momentum exchange. The role of sea ice in this exchange remains an open question, with changes to ice concentration and roughness likely to alter the efficiency of momentum transfer from atmosphere to ocean and the extent to which wind stress can impact the mixed layer through waves and other mixing processes. Additionally, wind speed, in conjunction with the temperature contrast between the ocean and atmosphere dictate the magnitude of turbulent heat exchange between these bodies, elevating the importance of wind orientation and localized flow and the need to understand lower tropospheric dynamics. Salinity controls upper ocean stratification in the Arctic. Freshwater inputs thus modulate mixing and the distribution of momentum and heat within the upper ocean.
Although the Arctic represents one of the most challenging environments, year-round measurements are needed to capture the annual cycle of energy exchanges over sea ice, open ocean, and land, and to constrain global atmospheric reanalyses, which can be problematic in data-sparse regions such as the Arctic (e.g., Lindsay et al., 2014). These measurements would include long and shortwave radiation, wind, air temperature and relative humidity profiles (within the atmospheric boundary layer), and turbulent fluxes of heat and momentum. The next level of priorities might include aerosols, clouds, precipitation, atmospheric chemistry and ocean-atmosphere CO2 fluxes. Seasonal and interannual changes of atmospheric CO2 are poorly resolved over the Arctic and the role of the Arctic Ocean as a CO2 sink is yet to be determined. Remote sensing can provide measurements of near-surface winds over open water, but other measurements must be made using in situ.
System Design
Key guidance and constraints on the design of an ARCGOOS derive from the consensus that the system has to cover a range of purposes and applications National Research Council [NRC] (2006), Lee et al. (2015), and Science, and Technology Policy Institute [STPI], and Sustaining Arctic Observing Networks [SAON] (2017). Consequently, it is helpful to consider the expected benefits, requirements, and demands on system components by different user communities in the context of planning and policy, strategy, and tactics – as laid out in section “Introduction.” From these three aspects of system characteristics, a range of observing system design criteria and approaches can be derived, augmented by the constraints placed upon the system at the implementation stage (Table 1, see also section “System Implementation” and Lee et al., 2015 for further background). A distinguishing characteristic of the Arctic region compared to other marine environments is the tight coupling between the different components of the extant social-environmental systems, corresponding to the need for similar fit between different disciplines, sectors and knowledge systems (e.g., Young, 2009). These circumstances place major demands on the process of observing system design. Below, we consider how such transformational challenges can be addressed through a portfolio of different design approaches and briefly discuss their advantages and drawbacks, drawing on specific examples from the Arctic where possible.
Past assessments and surveys conducted by SAON, the AOSs (Murray et al., 2018; Sandven et al., 2018), and related efforts e.g., INTAROS (Ludvigsen et al., 2018) demonstrate that an integrated Arctic observing system will not be designed in its entirety from the ground up. Rather, with a range of observing activities, from numerous research projects (such as those highlighted in section “Examples”), agency-led programs at the national and international level (e.g., the International Arctic Buoy Program3), and community-based monitoring efforts (Johnson et al., 2015; Williams et al., 2018). ARCGOOS design and implementation needs to leverage from a range of existing observing efforts operating at different scales with different objectives and missions. In fact, the sparsity of observations and resources in Arctic marine settings have resulted in operators and planners having to disproportionately rely on data from research observing networks (Lee et al., 2015; Eicken et al., 2016, Ludvigsen et al., 2018). These challenges need to be addressed through development of a framework that allows for the design of new components and optimization, modification, and integration of existing pieces to go hand in hand (AOS Executive Organizing Committee, 2018). Global observing frameworks have grappled with this issue, though maybe not to the extent as required in the Arctic.
While a number of observing frameworks exist, over the past decade or so approaches to derive specific requirements from mission and SBAs have been converging. The GOOS FOO (Lindstrom et al., 2012; Figure 2) is particularly well suited in the Arctic since it aligns with other relevant efforts under GEO and GCOS. Further guidance on the combination of observing system design and optimization can be drawn from the Ten GCOS Climate Monitoring Principles4, four of which explicitly address key aspects of integrating new observations into nascent, loosely assembled networks:
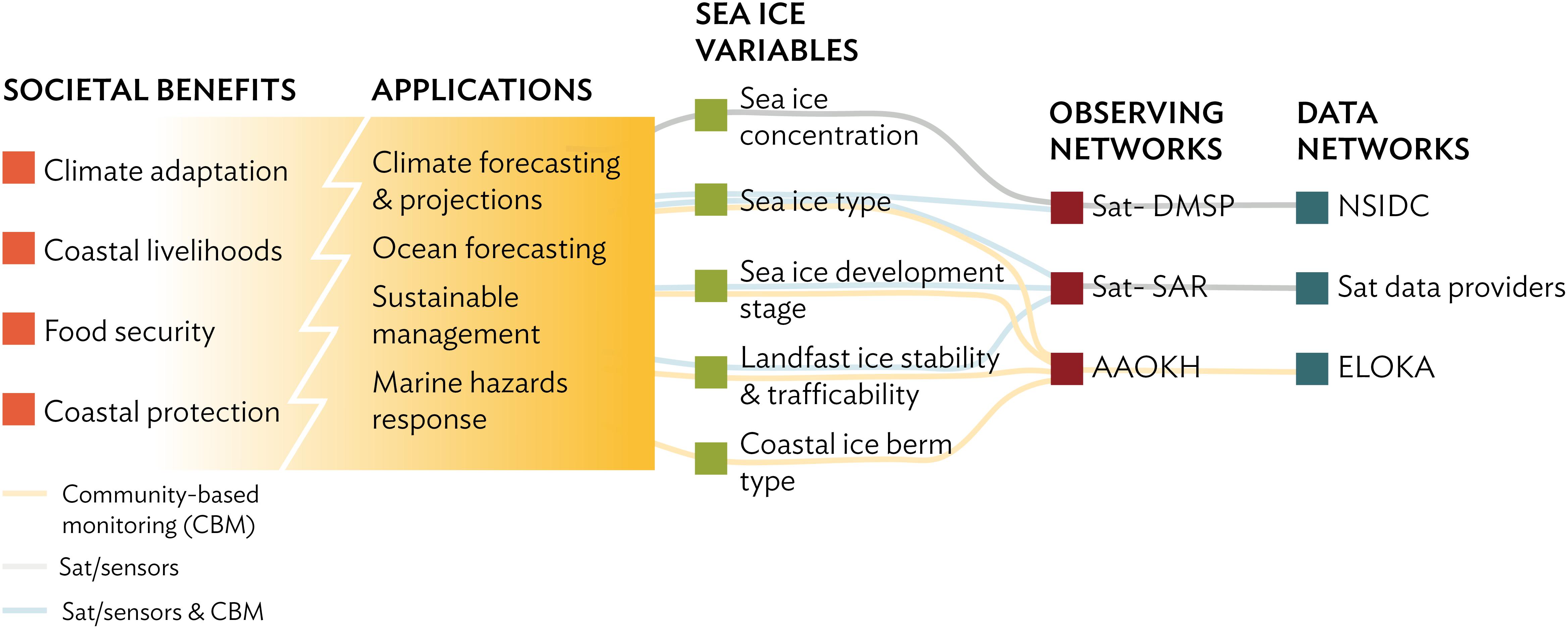
Figure 2. (Adopted from Lindstrom et al., 2012) Example of observing network design and implementation as seen through the lens of a top-down approach based on Starkweather et al. (2018). Shown is the link between societal benefit areas, EOVs, and observing and data management systems for the GOOS FOO (Lindstrom et al., 2012) as applied to Arctic sea-ice observations. Abbreviations: DMSP, Defense Meteorological Satellite Program; SAR, Synthetic Aperture Radar; NSIDC, National Snow and Ice Data Center.
(1) The impact of new systems or changes to existing systems should be assessed prior to implementation.
(2) Consideration of the needs for environmental and climate-monitoring products and assessments, such as IPCC assessments, should be integrated into national, regional and global observing priorities.
(3) High priority for additional observations should be focused on regions sensitive to change, data-poor regions, poorly observed parameters, and key measurements with inadequate temporal resolution.
(4) The conversion of research observing systems to long-term operations in a carefully planned manner should be promoted.
The three different user-base contexts and associated information needs (augmented by information guiding system implementation) can be linked to different observing system design and optimization methods appropriate for the particular application (Table 1; Lee et al., 2015). Progressively finer detail needs to be resolved in moving from the policy to the tactics and implementation scale. At the policy and planning level, information needs and observing program design focus on broad societal benefits and applications. To be sure, such demands on the system still require identification of EOVs, but as demonstrated in the value tree analysis (VTA) carried out by the STPI and SAON in 2017, siting of observations and temporal resolution are not as much of a concern in demonstrating value to policy makers and funding organizations. For example, the European Union’s Horizon 2020 Program supported a high level study that focused mainly on demonstrating economic value of a portfolio of observations in broadly defined sectors, without delving into the detail that would be part of tactics or even strategy-focused observations (Dobricic et al., 2018).
A key challenge at the policy and planning scale is the identification of suitable target variables. Here, the use of participatory scenarios has proven valuable in having observing system users or stakeholders identify indicator variables (Flynn et al., 2018; Preston and Lovecraft, 2018) that can then be translated into or linked to EOVs. Even at this level of data use, it is in principle possible to derive more specific guidance on the siting of specific observations. For example, Vargas-Moreno et al. (2016) were able to use geospatially explicit scenarios to identify broad target regions for monitoring linked to energy and resource development in coastal and offshore Alaska. Along the same lines, institutional analysis, with a focus on formal and informal regulations governing specific ecosystem services or societal benefits, can provide some guidance on the locus of observations (Lovecraft et al., 2013). While studies such as Science, and Technology Policy Institute [STPI], and Sustaining Arctic Observing Networks [SAON] (2017) or Dobricic et al. (2018) can help address the due diligence required by policymakers and funding agencies in terms of guiding sustained observations, there is comparatively little reliance on the approaches outlined in Table 1 to date.
At the Strategy and even more so at the tactics and system implementation level, more detail is required in terms of the specifications as well as the observing assets and networks and data management aspects of the monitoring (Table 1 and Figure 2). The necessary detail can be obtained through a combination of different methods, with heuristic input from experts being the most common. In fact, all of the examples listed in section “Examples” draw most heavily on input by experts, typically researchers or agency personnel who are conducting the actual measurements. While this group often has extensive knowledge of both the underlying science and the technology details that are relevant for system design, such input is typically poorly traceable and may not meet requirements, e.g., by regulatory agencies where such decisions may have to hold up in court.
System Optimization
Design of ARCGOOS should be dictated by cost-efficiency and representativeness of sampled EOVs. Quantitative methods, including OSEs, OSSEs, or adjoint-based optimal experimental design can assist the design of ARCGOOS. Below we briefly describe and discuss each of these methods.
Observing System Experiments involve analyses designed to withhold or aggregate certain subsets of data to quantitatively assess the impact of different observational platforms within already implemented observing systems (Oke et al., 2015). Impacts are assessed versus observations withheld (or aggregated) from assimilation or from state-of-the-art analysis products derived from observations. One disadvantage of OSEs is that the reference experiment that includes all data might not represent the true state, thus the assessed impacts of the withheld data relative to this reference experiment might not fully capture the true impacts. An additional disadvantage is that OSEs can only assess impacts of existing but not of future observing systems.
Observing System Simulation Experiments follow the same procedures as OSEs, but assimilate synthetic observations instead of existing real-world observations. OSSE systems (Atlas, 1997; Hoffman and Atlas, 2015) facilitate quantitative impact assessment on analyses and strategies of planned observing systems, and of different deployment strategies for existing systems. An OSSE system consists of three components: (a) NR, which is a free-running model, that represents the groundtruth, i.e., assumed to be representative of the true ocean; b) data-assimilative ocean forecast system that couples different ocean models (the Forecast Model, or FM) to an ocean DA scheme; and c) simulations of ocean observations within the NR containing realistic or expected observational errors for each observing platform being assessed for assimilation into the forecast system. Ocean profiles (e.g., temperature, salinity) and other observations are generally re-mapped prior to assimilation (Halliwell et al., 2014). Impacts can then be assessed by comparing experimental analyses to high-resolution three-dimensional representations of the “groundtruth” ocean provided by the NR. Therefore, OSSE systems can not only assess new observing systems and strategies, but also assess impacts of existing observing systems more thoroughly than it is possible with OSEs. OSSEs also have an advantage over linearized procedures to assess impact, such as adjoint methods, because the influence of non-linear processes on observing system impact can be documented. The disadvantage of OSSEs is that the NR might not fully capture the real-world ocean dynamics (Hoffman and Atlas, 2015). In addition, the impact assessment can be dependent on the model-system being used.
In contrast to both OSEs and OSSEs, where statistical approach is an important component of the DA step, adjoint-based methods utilize the dynamical information in the tangent linear and adjoint models of the underlying general circulation model (GCM). Through the equations which capture conservation and constitutive laws, propagation of information up- and down-stream of any quantity of interest (QoI) is used to (a) assess impactful regions where new observations can be potentially deployed (Marotzke et al., 1999; Zanna et al., 2010; Heimbach et al., 2011; Nguyen et al., 2017; Stammer et al., 2018); (b) assess the redundancy of existing observing networks (Köhl and Stammer, 2004; Moore et al., 2017b); (c) quantify the impacts of selected existing/new observational networks on reducing posterior uncertainties of the GCM control parameters and/or potential unobserved remote QoI (Moore et al., 2011, 2017a; Bui-Thanh et al., 2012; Kalmikov and Heimbach, 2014, 2018; Kaminski et al., 2015, 2018); (d) find an optimal observing network through Hessian-based OED that minimizes the posterior uncertainties as a function of the control parameters and/or targeted QoI (Alexanderian et al., 2016; Loose, 2019). The advantage of the adjoint-based methods is not only the quantification of uncertainty reduction of the GCM control parameters and/or any specific QoI to the observing network but also the identification of dynamical connection and causal relationship between them. The disadvantage of these methods is the prohibitive computational cost of calculating the full Hessian and its inverse. Recent advances in the field of computational sciences (e.g., Flath et al., 2011; Bui-Thanh et al., 2012; Loose, 2019) have helped reduce the computational cost and thus can make adjoint-based OEDs tractable for oceanographic applications.
To date, OSE, OSSE, and adjoint-based OED have not been fully utilized for optimization of observational work due to the disadvantages associated with each of the methods as discussed above. As a result, there are only a few examples of OSEs employed to provide quantitative, traceable guidance on key aspects of observing system design (e.g., Panteleev et al., 2009; Jung et al., 2016; Kaminski et al., 2018, Stammer et al., 2018). An argument put forward against over-reliance on OSEs and OSSEs for system design is that measurements in a rapidly changing Arctic are typically meant to provide information that can help anticipate or prepare for major changes and transformations. Hence, the siting and overall sampling strategy may not account for the impacts of rapidly changing conditions not captured or predicted by the model. This is particularly true if statistical models are relied upon. For example, in the Arctic Ocean the loss of perennial sea ice has impacts on many other components of the marine system that are still poorly captured by models and in particular statistics of past behavior (e.g., Lindsay and Zhang, 2006).
However, it should be noted that comprehensive observations covering both the spatial and temporal scales required to fully measure and understand the rapidly changing Arctic are also not available. In addition, ad hoc estimates of spatial/temporal correlation scales are often utilized to interpolate data within the existing observational systems without full knowledge of error covariance (Wunsch, 2006). Thus, a careful synthesis of different bodies of knowledge is needed to guide system design. In other words, an approach where the imperfect tools available within OSE, OSSE, and adjoint-based OED should be employed in iterative steps with deployments of observations to push forward development and improvements for ocean applications, similar to the steady progress achieved in DA and models used for NWP applications (e.g., Bauer et al., 2015).
Advances in forward modeling and 4D-var DA have facilitated model guidance that is more tightly linked to, e.g., sensor and observing technology constraints such that OS(S)Es and OEDs can potentially help support the design of the sensor systems themselves (Kaminski et al., 2018). It should be expected that, due to the potentially limited and serious deficiencies, an optimal network based on these quantitative tools might not capture the dynamics when compared to field observations and other political/economic constraints. One example is the case at the Bering Strait where, based on inverse modeling and drawing on a large dataset for the region, Panteleev et al. (2009) determined that optimal observations of Bering Strait throughflow should include two moorings located within the Strait and two at some distance further south. In practice, due to economical and political constraints, and in combination with knowledge gained from the existing observations at the strait, the array has been reduced to three moorings, two in the eastern channel and next to the Alaskan Coast, and one located north of the strait (Woodgate, 2018). Such discrepancies should be anticipated in the iterative process, and should be used to facilitate further communication and system co-design by modelers and observationalists. Important QoI should be identified, e.g., monitoring ocean dynamics, detecting temporal variability on a range of scales, capturing air-sea interaction processes, or detecting sea level changes. Proposed observing systems based on different OS(S)Es and OEDs should be weighed against each other and used judiciously, as with any decision-making.
System Implementation
Arctic Region Component of the Global Ocean Observing System implementation will involve a complex network of national and international partners, addressing a myriad of scientific and operational objectives, under significant and varied logistical challenges. In practice, implementation of a sustained ARCGOOS will mean adapting and integrating existing observing activities alongside the development of new systems deliberately designed to meet identified ARCGOOS needs. Although the choice of specific operational approaches and technologies clearly depends on the requirements and constraints of the specific system, some considerations that apply more broadly are discussed here.
An ARCGOOS capable of spanning diverse demands will need to rely on a heterogeneous mix of reference frameworks and approaches. The needs of SBAs that require data in the domains of planning/policy and strategy skew toward measurements at the basin and pan-Arctic scales. At present, such observations are often collected by stochastic sampling using large arrays of drifting instruments such as Argo (e.g., Argo Steering Team, 1998; Riser et al., 2016), the Global Drifter Program (e.g., Lumpkin and Pazos, 2007; Centurioni et al., 2017), IABP5 and the Ice-Tethered Profiler program (Krishfield et al., 2008). Instruments are deployed in sufficient numbers to achieve a target density (e.g., one float per 300 km for Argo), with the system relying on drift to maintain distribution over the target region. Although collected by drifting instruments, measurements are interpreted in an Eulerian (geographically fixed) frame. Though powerful, this approach has limitations. The same currents that distribute platforms also create areas of convergence and divergence, which concentrate or exclude instruments from those regions. Instruments rapidly flush from areas of strong flow, limiting measurements in many regions that are of particular importance. Eulerian measurements e.g., moorings, sea floor installations, tied to key locations, such as across straits and boundary currents, address these weaknesses. Fixed installations complement the broad stochastic array by ensuring consistent data return at specific temporal and spatial resolution. Lastly, measurements collected in the Lagrangian frame, drifting with a water parcel or ice floe, can be employed to isolate time evolution driven by internal processes from advective effects. For instance, estimate the difference between observing the time-evolution of a single ice floe and recording variability in sea ice due to different ice floes passing through the domain.
Approaches that incorporate small, long-endurance autonomous platforms, including ice-based instruments, surface drifters, profiling floats, underwater gliders and autonomous surface vessels, offer capabilities that address many of the challenges confronting ARCGOOS implementation. Operational constraints have historically restricted measurements to the times and locations accessible by ships and aircraft, thus biasing observations toward summertime regions lacking thick, multiyear sea ice. Some autonomous platforms, such as ice-based observatories, profiling floats and gliders, offer endurance that exceeds a year, thus providing persistent sampling through the full annual cycle. Flexible deployment logistics afforded by small size, coupled with endurance and mobility (self-propelled, or drifting in water or sea ice) allows access to remote regions. Relatively low unit cost provides the potential to scale to large numbers, both for achieving large scope and for creating robust systems that are resilient to instrument losses. Systems of autonomous platforms (e.g., Lee et al., 2017) can provide a flexible observing capability that can be readily reconfigured in response to changing needs and objectives. Lastly, relatively light logistical requirements and low cost help make sustained operation over many-year timescales more tractable.
Autonomous approaches also face challenges. Sensor technologies currently limit autonomous platforms to a modest suite of variables, with clear needs for advances in biological, biogeochemical and atmospheric sensors. Sustained collection of critical in situ observations, for measurements that cannot be made autonomously and for referencing autonomous sensors, will require periodic ship-based sampling, such as that planned by the Synoptic Arctic Survey (Anderson et al., 2018). When operating beneath ice, autonomous platforms cannot access satellite services (GPS and Iridium), and must instead rely on acoustic networks (Lee and Gobat, 2006; ANCHOR Working Group, 2008; Mikhalevsky et al., 2015) for underwater geopositioning (UW-GPS) and telemetry. Acoustic infrastructure might thus be required to maximize the utility of autonomous approaches. Acoustic networks augmented with hydrophones and oceanographic instruments could provide a scalable multipurpose ocean observing system including UW-GPS, acoustic thermometry along fixed sections, passive acoustics, and oceanographic point measurements (e.g., Mikhalevsky et al., 2015).
A critical and growing component of the ARCGOOS is satellite remote sensing. Satellite mounted sensors provide a pan-Arctic observing capability. They are a powerful tool to put land/marine/ice based in-situ measurements in a large-scale context. There are numerous satellite mounted sensors, from different space agencies, that have significant relevance for the Arctic region. With respect to sea ice, passive microwave satellite measurements (which can see through clouds and the polar night) can be used to obtain sea ice characteristics such as sea ice concentration and extent (Martin and Cavalieri, 1989; Bareiss and Goergen, 2005). Passive microwave measurements have been made from US satellites since the late 1970s, thus providing a continuous record of sea ice conditions for over 40 years. Pan-Arctic sea ice thickness can be calculated from radar (ESA) and, with the 2018 launch of ICESat-2, laser (NASA) altimetry by accurately measuring the height of the ice (and snow) above the sea surface (its freeboard) and converting this information into an ice thickness (e.g., Kwok and Cunningham, 2008; Laxon et al., 2013). Scatterometer-derived information can be used for identification of the sea ice edge (Long and Hicks, 2005) as well as to provide high-resolution information on ice type (Kwok, 2004). The most widely used satellite sensor for operational human activities in ice covered seas is Synthetic Aperture Radar (SAR). SAR images, which also see through clouds and the polar night, are used routinely for ship navigation of ice-covered waters through to inferring sea ice characteristics (Willmes et al., 2010), sea ice drift (e.g., Spreen et al., 2011) and deformation (e.g., Bouillon and Rampal, 2015).
Many recent advances in physical oceanography have become possible due to the advent of altimetry and gravimetry satellites that observe the total and ocean mass components of sea level change. Radar altimetry cannot measure sea level over sea ice, but significant progress has been made in data retrieval over leads and polynyas (Laxon, 1994; Peacock and Laxon, 2004; Farrell et al., 2012; Kwok and Morison, 2016; Anderson et al., 2018). Satellite altimetry measurements have proven to be efficient for monitoring the large-scale variability of ocean circulation in the Arctic (e.g., Armitage et al., 2017).
While the spatial coverage of satellite altimetry data is limited to leads and open ocean, the Gravity Recovery and Climate Experiment (GRACE) and its follow-on (GRACE-FO) missions provide basin-wide observations of monthly ocean mass variations that also reveal changes in Arctic Ocean circulation patterns (Volkov and Landerer, 2013; Peralta-Ferriz and Morison, 2014). Subtracting GRACE records from altimetric sea level yields estimates of the steric sea level variability (due to density changes), which are indicative of changes in the Arctic Ocean freshwater content (Häkkinen and Proshutinsky, 2004; Giles et al., 2012; Morison et al., 2012). The combined use of satellite derived geostrophic surface currents and subsurface velocities derived from hydrography data makes it possible to routinely monitor the volume transport of the warm and saline Atlantic Water in the Nordic Seas (Chafik et al., 2015; Raj et al., 2018). In the near-future, progress can be made with wide-swath Surface Water and Ocean Topography (SWOT) interferometric altimetry mission, scheduled for launch in 2021. With a spatial resolution of about 10 km this mission will further enhance our knowledge about mesoscale and coastal zone dynamics (e.g., Fu and Ferrari, 2008). High-resolution surface velocity data from Synthetic Aperture Radar data (SAR) is another potential source of information about ocean circulation (Chapron et al., 2005; Johannessen et al., 2008; Hansen et al., 2012). Other satellite sensors provide information on, for example, phytoplankton blooms, sea surface temperature and salinity, albedo, and surface wind speeds.
In addition to identification of SBAs and the resulting ARCGOOS objectives, the engagement of local communities is also critical to implementation. Many communities already maintain a persistent presence in key areas for the observing system, and could thus inform and support the sustained collection of high-quality in situ measurements. Furthermore, the efficient operation of autonomous platforms depends highly on local logistics, such that a sustained measurement program would benefit greatly from partnerships with communities near the target region. By nature, community-based monitoring programs tend to focus on those issues of greatest concern to local stakeholders, thus having considerable potential to influence on-the-ground management activities e.g., acoustic pollution from seismic surveys. It is found that CBM programs inform decisions at local, regional and national levels and often provide insight into processes and changes not captured in agency or research-driven monitoring programs. An evaluation of the capabilities, good practice and challenges of CBM programs is provided by Danielsen et al. (2018). Engagement surrounding implementation might also establish relationships that guide analyses and enhance uptake of the resulting products.
Although not a direct component of the sustained observing system, focused, limited-duration process studies will continue to be crucial for advancing understanding of the marine system and informing the evolution of ARCGOOS. Examples include recent international programs focused on the Marginal Ice Zone during melt (Lee et al., 2017), Arctic Sea state and boundary layer physics during freeze up (Thomson et al., 2018) and the large Multidisciplinary drifting Observatory for the Study of Arctic Climate (MOSAiC), which will conduct intensive measurements of that atmosphere-ice-ocean system using the German icebreaker Polarstern as a drifting platform for a year.
The changing Arctic presents new challenges and opportunities to ARCGOOS implementation. Steady decline in sea ice extent, accompanied by changes in thickness distribution and character, make landing aircraft on the ice, and thus deploying instruments, increasingly difficult. The growing dominance of first year ice makes it more challenging to deploy ice-based platforms onto floes that are likely to survive beyond a year, motivating new platform designs that can withstand the rigors of melt-out, open water drift and freeze-in. In contrast, growing open water extent in summer eases the operation of autonomous platforms sampling within the water column, as they have increasing access to satellite services. Important opportunities for the ARCGOOS include exploiting the current generation of autonomous platforms and accelerated development of sensors for water column biology and biogeochemistry and for fully autonomous, untended atmospheric measurements including radiative fluxes, winds, aerosols and clouds.
Examples
There are many broad and important efforts underway regionally and topically. These include, for example, observing systems characterizing Arctic gateways (Fram Strait, e.g., Soltwedel et al., 2013; Hansen et al., 2013; Rabe et al., 2013; Sagen et al., 2016; de Steur et al., 2018; Bering Strait, e.g., Woodgate,. 2018; Davis Strait, e.g., Curry et al., 2014), a network of distributed, drifting Ice Tethered Profilers (e.g., Toole et al., 2011) and observing systems under the PAG, such as the DBO (see Moore and Grebmeier, 2018). Basin-wide Arctic Ocean observations are particularly challenging, but efforts such as the NABOS (e.g., Polyakov et al., 2017) and the INTAROS are leading the way. These examples demonstrate that international collaboration with common scientific and logistical work components is crucial for meeting the challenges of the complexity of natural systems, demanding specific tailored observing systems suitable for Arctic conditions. They offer successful models for operating and sustaining systems driven by science choices, motivated by immediate SBAs.
Fisheries potential and sound management in the Pacific sector of the Arctic Ocean requires understanding how the marine ecosystem will respond to seasonal shifts in the timing of spring sea ice retreat and/or delays in fall sea ice formation. To more systematically track the broad biological response to sea ice retreat and associated environmental change, an international consortium of scientists implemented a coordinated DBO that includes selected biological measurements at multiple trophic levels (Moore and Grebmeier, 2018). These measurements are being made simultaneously with hydrographic surveys and satellite observations. The DBO focuses on biological “hotspot” locations in the Bering and Chukchi Seas. From European side intensive environmental and ecosystem monitoring programs are carried out in the Barents Sea used for resource management in this important region for fisheries in Norway and Russia.
The internationally coordinated NABOS has focused on the detection of Arctic Ocean change in the Eurasian Basin. The program’s primary monitoring tool is a series of moorings and quasi-synoptic oceanographic surveys to quantify circulation, water mass transformations, and key mechanisms of variability in the Arctic Ocean, including links to lower-latitude processes. The observing array captured several warming events in the Atlantic Water layer, and a general weakening of the stratification in this sector of the Arctic (Carmack et al., 2015). As successful as these programs are, they are still reliant on the good will of independent Arctic nations to allow for international monitoring efforts within their territorial seas or exclusive economic zones.
Integrated Arctic Observation System involves 48 partners from 20 different countries to contribute to the development of an INTAROS addressing ocean, atmosphere and land. With a focus on coordination, observing system assessments and data integration for user applications. INTAROS aims to produce a roadmap for a sustained Arctic observing that will encompass coordination, data collection, data handling, and services benefiting specific stakeholders.
These regional and topical efforts are not presently framed within a unified strategy for synthesis (e.g., Behrendt et al., 2018) or network development. Beyond advancing more coordination of existing efforts (capabilities-based approaches), we recommend requirements-driven approaches, which require a shared assessment system to identify and weigh priorities.
Vision
The different assessments, planning processes, and systems referenced above provide a foundation for a multi-function ARCGOOS. The functions associated with an ARCGOOS (Figure 1) for a subset of all SBAs, serve broad and diverse end user and scientific communities. The considerations in this review paper strongly support the establishment of ARCGOOS with the following benefits:
• The scientific community has at its disposal decadal scale observations of a broad array of variables that describe the state and dynamics of core Arctic social-environmental systems in order to track and anticipate major changes, inform model development, and support validation of remote sensing data and model output, and advance our understanding of an environment undergoing transformative, globally relevant change;
• Arctic residents and communities can rely on a combination of community-based monitoring and sustained pan-Arctic sensor-based observations to help them understand and respond to a rapidly changing Arctic, preparing for rapid- and slow-onset hazards and supporting sustainable development;
• National, local, and Indigenous governments are receiving focused, meaningful information about long term change and variability from an observing system that supports planning and decision-making for community health and sustainability;
• The global community of Arctic and non-Arctic countries is furnished with data from Arctic observing networks that guides long-term planning to minimize the impacts of environmental hazards associated with a rapidly changing Arctic;
• Resource management agencies access reliable long-term records available to inform prudent management action and policy;
• Emergency response organizations draw on a suite of near real-time environmental observations that guide emergency response and hazard mitigation efforts;
• The global community is able to trace the impacts and determine the efficacy of international treaty systems and mitigative measures meant to address the negative impacts of climate change by drawing on an observing system with the requisite sensitivity and resolution.
With this review paper a foundation has been laid for the design and optimization of ARCGOOS leveraging existing system components at the international level. SAON in particular has emerged as the entity that is both tasked and capable – with additional resources emerging following the second Arctic Science Ministerial in October 2018 – to address this challenge. Collaboration and coordination with national, regional, and local efforts, and global observing programs such as GEOSS, GCOS, GOOS, and others is essential. A further critical component to observing system success is to ensure relevance of the system to the people of the Arctic. The latter can only be achieved through co-design and co-management of such a system and the data it produces. Recent assessments by Johnson et al. (2015) and Williams et al. (2018) as well as a survey of best practices by Fidel et al. (2017) and Danielsen et al., 2018 point the way on how to achieve these outcomes through integration of community-based observations.
We conclude with a few specific recommendations drawn from this review article and some of the documents and initiativesthis work draws on.
Recommendation 1: While progress has been made in several disciplines or sectors, what is lacking is a framework that facilitates and supports coordinated implementation and integration of observing system components that fill critical thematic, regional, or trans-sectoral gaps. We recognize that an Arctic Ocean observing system will require such a framework that allows for the optimization, modification, and integration of existing observing programs and networks to go hand in hand with design and implementation of new observing components. Quantitative, simulation-based frameworks for optimal observing network design (including, OSE, OSSE, OED) should be advanced, matured and be made available as a tool for supporting ARCGOOS efforts. Such an approach should build on capacity and leadership by SAON, partnering with global observing programs and systems (in particular those called out above) where appropriate. Private-public partnerships and the approach taken by the Copernicus, a European Program for the establishment of capacity for earth observation, may serve as models and incentives for increased attention needed at the level of potential public and private sector funders of such activities.
Recommendation 2: An emerging governance structure under SAON will help minimize duplication, channel resources, and support shared benefits. What is urgently needed as a first step is international collaboration on developing a roadmap for an integrated observing system that meets user requirements as well as putting in place components that draw on existing networks and help maximize shared benefits in the near- and mid-term. The AOS and venues such as OceanObs need to be drawn upon as platforms to advance exchange and define specific action. AOS6 in particular provides a forum for different user groups, engineers and sensor network designers, and the broader research community to come together and make progress with some of the thornier issues, that require intense, focused input and reconciliation of different approaches. The Arctic-themed Community White Papers submitted to OceanObs’19 illustrate both the breadth of contributions and the potential for developing closer links and optimization along regional or sectoral interfaces. Beyond these surveys, a commitment toward parsing and consolidation of planning efforts is needed.
Recommendation 3: An efficient ARCGOOS that provides information across the range of scales demanded by the SBAs and missions laid out above, needs to focus attention on the integration of remote sensing and in-situ observations. Robustness and reliable data delivery play a major role in the design of observing systems, as well as multipurpose use of autonomous observing platforms and infrastructure. Low-cost, long-endurance autonomous platforms offer promising new approaches for large-scale, sustained observing. Development of small, low-power sensors suitable for deployment on these platforms requires further support and attention by both the engineering and research community. Development and implementation of systems for geopositioning and telemetry is needed to make underwater autonomous observing systems fully operational.
Recommendation 4: To maximize the uptake of observations and derived products, all data must be secured in long-term storage in an established data repository adhering to best practices for scientific data management. This includes standardized metadata describing the content, processing and quality control procedures applied, and assignment of unique persistent identifiers (such as DOIs). Datasets must be searchable and accessible through standard protocols, enabling a wide range of software clients to utilize them, and must be accompanied by a data license. The license will define acceptable use of the data, and include a citation statement linking back to the data providers giving them credit for their datasets. There is a need for a governance structure to coordinate the many ongoing initiatives in Spatial Data Infrastructures (SDIs) for Arctic data to ensure all future ARCGOOS data follow the FAIR Principles. SAON, through its Arctic Data Committee, should take the lead in establishing a pan-Arctic SDI in collaboration with the major players in Arctic data management.
Recommendation 5: To ensure societal relevance, address critical capacity issues, and acknowledge relevant expertise and authority, Indigenous peoples of the Arctic and Arctic residents need to be involved in the definition, design, and implementation of an Arctic Ocean observing system; co-design of the system, co-management of resulting data products, and integration of community-based observations into the observing system fabric best serve this goal.
Author Contributions
CL, SSt, HE, M-LT, JW, and SSa contributed to the overall concept, writing of the initial draft, and revision of all sections. DD, SG, JG, JI, S-HK, MM, IP, BR, SSe, DV, AN, and TH contributed writing to individual sections. AB-M, MD, GG, AK, AO, TR, HS, ØS, HS, LC, RR, and KS contributed ideas and material for individual sections. All authors have read and approved the submitted version.
Funding
CL was supported by the US Office of Naval Research under the grant N00014-16-1-2377. SSt was supported by the US National Oceanic and Atmospheric Administration – Arctic Research Program. DD was supported by HYCOM NOPP (Award No. N00014-15-1-2594). SSa, TH, HS, AK, KS, and AB-M were supported by INTAROS project. This project has received funding from the European Union’s Horizon 2020 Research and Innovation Programme under the grant agreement number 727890. BR strongly benefited from the work within the German Helmholtz Association strategic investment Frontiers in Arctic Marine Monitoring (FRAM). DV was supported by the Atlantic Oceanographic and Meteorological Laboratory under the auspices of the Cooperative Institute for Marine and Atmospheric Studies of the University of Miami and NOAA (cooperative agreement NA10OAR4320143). JG was supported by the US National Science Foundation Arctic Observing Network grant #1702456 and National Oceanic and Atmospheric Administration grant #CINAR 22309.02. SG was supported in part by the Norwegian Polar Institute’s project “The Fram Strait Arctic Outflow Observatory.” LC was supported by the Swedish National Space Agency (Dnr:133/17).
Conflict of Interest Statement
The authors declare that the research was conducted in the absence of any commercial or financial relationships that could be construed as a potential conflict of interest.
The reviewer PH declared a shared affiliation and a past co-authorship, with one of the authors, AN, to the handling Editor at the time of review. The peer review was handled under the close supervision of the Chief Editors to ensure an objective process.
Abbreviations
AMAP, Arctic Monitoring and Assessment Programme; ARCGOOS, Arctic Region Component of the Global Ocean Observing System; CAFF, Conservation of Flora and Fauna; CBMP, Circumpolar Biodiversity Monitoring Program; DA, Data Assimilation; DBO, Distributed Biological Observatory; EAV, Essential ARCGOOS Variable; ECV, Essential Climate Variable; EOV, Essential Ocean Variable; FOO, Framework for Ocean Observing; FRAM, Frontiers in Arctic Marine Monitoring; GOOS, Global Ocean Observing System; GEO, Group on Earth Observations; GEOBON, GEO Biodiversity Observation Network; GEOCRI, GEO Cold Regions Initiative; GEOSS, Global Earth Observation System of Systems; GRA, GOOS Regional Alliance; IAOAF, International Arctic Observing Assessment Framework; IASC, International Arctic Science Committee; IMOBAR, Impact Assessment on a Long-Term Investment on Arctic Observations; INTAROS, Integrated Arctic Observation System; IOC, Intergovernmental Oceanographic Commission; IOOS-AOOS, US Integrated Ocean Observing System – Alaska Ocean Observing System; IPY, International Polar Year; NABOS, Nansen and Amundsen Basins Observational System; NR, Nature Run; OED, Optimal Experimental Design; OSE, Observing System Experiment; OSSE, Observing System Simulation Experiment; PAG, Pacific Arctic Group; PAME, Protection of the Arctic Marine Environment; POGO, Partnership for Observation of the Global Ocean; SAON, Sustaining Arctic Observing Networks; SBA, Societal Benefit Area; SCAR, Scientific Committee on Antarctic Research; SCOR, Scientific Committee on Oceanic Research; SDM, Structured Decision-Making; SDWG, Sustainable Development Working Group; SOOS, Southern Ocean Observing System; STPI, Science and Technology Policy Institute; UNFCCC, United Nations Framework Convention on Climate Change; WMO, World Meteorological Organization.
Footnotes
- ^ https://arctic-roos.org/
- ^ https://www.arcticscienceministerial.org/en/conclusions-1740.html
- ^ http://iabp.apl.washington.edu/
- ^ https://gcos.wmo.int/en/essential-climate-variables/gcos-monitoring-principles (retrieved January 24, 2018).
- ^ http://iabp.apl.washington.edu
- ^ http://www.arcticobservingsummit.org/
References
Aagaard, K., and Coachman, L. (1975). Toward an ice-free Arctic ocean. Eos Trans. AGU 56, 484–486. doi: 10.1029/EO056i007p00484
Alexanderian, A., Petra, N., Stadler, G., and Ghattas, O. (2016). A fast and scalable method for a-optimal design of experiments for infinite-dimensional bayesian non-linear inverse problems. SIAM J. Sci. Comput. 38, A243–A272.
AMAP (2017). Snow, Water, Ice and Permafrost in the Arctic (SWIPA). Oslo: Arctic Monitoring and Assessment Programme (AMAP), 269.
Anchor Working Group. (2008). Acoustic Navigation and Communication for High-Latitude Ocean Research. Seattle, WA: University of Washington, 54.
Anderson, L. G., Ashjian, C., Azetsu-Scott, K., Carmack, E., Chierici, M., Cho, K.-H., et al. (2018). Synoptic Arctic Survey – a Pan-Arctic Research Program. Science and Implementation Plan. 40. Available at: http://www.synopticarcticsurvey.info/ (accessed January 25, 2019).
Andrews, J., Babb, D., and Barber, D. G. (2017). Climate change and sea ice: shipping accessibility on the marine transportation corridor through Hudson Bay and Hudson Strait (1980–2014). Elem. Sci. Anth. 5:15. doi: 10.1525/elementa.130
AOS Executive Organizing Committee [AOS EOC] (2018). Report of the 4th Arctic Observing Summit: AOS 2018, Davos Switzerland, 24-26 June 2018. Calgary, AB: International Study of Arctic Change (ISAC) Program Office.
Arctic Council (2011). Nuuk Declaration. Canada, Denmark, Faroe Islands, Finland, Greenland, Iceland, Norway, Russian Federation, Sweden, United States of America. Available at: https://oaarchive.arctic-council.org/handle/11374/92 (accessed May 12, 2011).
Argo Steering Team (1998). On the Design and Implementation of Argo: An Initial Plan for a Global Array of Profiling Floats. International CLIVAR Project Office Report 21, GODAE Report 5. Melbourne, VIC: GODAE International Project Office, 32.
Armitage, T. W. K., Bacon, S., Ridout, A. L., Petty, A. A., Wolbach, S., and Tsamados, M. (2017). Arctic Ocean surface geostrophic circulation 2003-2014. Cryosphere 11, 1767–1780. doi: 10.5194/tc-11-1767-2017
Armitage, T. W. K., Bacon, S., Ridout, A. L., Thomas, S. F., Aksenov, Y., and Wingham, D. J. (2016). Arctic sea surface height variability and change from satellite radar altimetry and GRACE, 2003-2014. J. Geophys. Res. Oceans 121, 4303–4322. doi: 10.1002/2015JC011579
Arrigo, K. R., van Dijken, G., and Pabi, S. (2008). Impact of a shrinking Arctic ice cover on marine primary production. Geophys. Res. Lett. 35:L19603. doi: 10.1029/2008GL035028
Atlas, R. (1997). Atmospheric observations and experiments to assess their usefulness in data assimilation. J. Meteor. Soc. Japan 75, 111–130. doi: 10.2151/jmsj1965.75.1b_111
Barber, D. G., Babb, D. G., Ehn, J. K., Chan, W., Matthes, L., Dalman, L. A., et al. (2018). Increasing mobility of high Arctic Sea ice increases marine hazards off the east coast of Newfoundland. Geophys. Res. Lett. 45, 2370–2379. doi: 10.1002/2017gl076587
Bareiss, J., and Goergen, K. (2005). Spatial and temporal variability of sea ice in the Laptev sea: analysis and review of satellite passive-microwave data and model results, 1997 to 2002. Glob. Plan. Change 48, 28–54. doi: 10.1016/j.gloplacha.2004.12.004
Bauer, P., Thorpe, A., and Brunet, G. (2015). The quiet revolution of numerical weather prediction. Nature 525, 47–55. doi: 10.1038/nature14956
Behrendt, A., Sumata, H., Rabe, B., and Schauer, U. (2018). UDASH – unified database for Arctic and Subarctic hydrography. Earth Syst. Sci. Data 10, 1119–1138. doi: 10.5194/essd-10-1119-2018
Bouillon, S., and Rampal, P. (2015). On producing sea ice deformation data sets from SAR-derived sea ice motion. Cryosphere 9, 663–673. doi: 10.5194/tc-9-663-2015
Box, J. E., Colgon, W. T., Christensen, T. R., Schmidt, N. M., Lund, M., and Parmentier, F. W. (2019). Key indicators of Arctic climate change: 1971-2017. Environ. Res. Lett. 14:045010.
Bui-Thanh, T., Burstedde, C., Ghattas, O., Martin, J., Stadler, G., and Wilcox, L. C. (2012). “Extreme-scale UQ for Bayesian Inverse Problems Governed by PDEs,” in Proceedings of the International Conference on High Performance Computing, Networking, Storage and Analysis, SC ’12, (Los Alamitos, CA: IEEE Computer Society Press), 3:1–3:11.
Calafat, F. M., Chambers, D. P., and Tsimplis, M. N. (2013). Interannual to decadal sea level variability in the coastal zones of the Norwegian and Siberian seas: the role of atmospheric forcing. J. Geophys. Res. 118, 1287–1301. doi: 10.1002/JGRC.20106
Carmack, E., Polyakov, I., Padman, L., Fer, I., Hunke, E., Hutchings, J., et al. (2015). Toward quantifying the increasing role of oceanic heat in sea ice loss in the New Arctic. Bull. Amer. Meteor. Soc. 96, 2079–2105. doi: 10.1175/BAMS-D-13-00177.1
Carmack, E. C., Yamamoto-Kawai, M., Haine, T. W. N., Bacon, S., Bluhm, B. A., Lique, C., et al. (2016). Freshwater and its role in the Arctic marine system: sources, disposition, storage, export, and physical and biogeochemical consequences in the Arctic and global oceans. J. Geophys. Res. Biogeosci. 121, 675–717. doi: 10.1002/2015JG003140
Centurioni, L., Horányi, A., Cardinali, C., Charpentier, E., and Lumpkin, R. (2017). A global ocean observing system for measuring sea level atmospheric pressure: effects and impacts on numerical weather prediction. Bull. Am. Meteorol. Soc. 98, 231–238. doi: 10.1175/bams-d-15-00080.1
Chafik, L., Nilsson, J., and Skagseth, Ø, and Lundberg, P. (2015). On the flow of Atlantic water and temperature anomalies in the Nordic seas toward the Arctic ocean. J. Geophys. Res. Oceans 120, 7897–7918. doi: 10.1002/2015JC011012
Chapron, B., Collard, F., and Ardhuin, F. (2005). Direct measurements of ocean surface velocity from space: interpretation and validation. J. Geophys. Res. Oceans 110:7008.
Cheng, L., Abraham, J., Hausfather, Z., and Trenberth, K. E. (2019). How fast are the oceans warming? Science 363, 128–129. doi: 10.1126/science.aav7619
Crépin, A. S., Karcher, M., and Gascard, J. C. (2017). Arctic climate change, economy and society (ACCESS): integrated perspectives. Ambio 46, (Suppl. 3), 341–354. doi: 10.1007/s13280-017-0953-3
Curry, B., Lee, C. M., Petrie, B., Moritz, R., and Kwok, R. (2014). Multi-year volume, liquid 1978 freshwater, and sea ice transports through Davis Strait, 2004-2010. J. Phys. Oceanogr. 44, 1244–1266. doi: 10.1175/JPO-D-13-0177.1
Danielsen, F., Fidel, M., Johnson, N., Poulsen, M. K., Eicken, H., Alba, A., et al. (2018). INTAROS D4.1. Community based Monitoring Programmes in the Arctic: Capabilities, Good Practice and Challenges. Research and Innovation Action under EC Horizon 2020 Grant Agreement no. 727890. Brussels: European Commission.
de Steur, L., Peralta-Ferriz, C., and Pavlova, O. (2018). Freshwater export in the East Greenland current freshens the North Atlantic. Geophys. Res. Lett. 45, 359–313. doi: 10.1029/2018GL080207
Dobricic, S., Monforti, F., Pozzoli, L., Wilson, J., Gambardella, A., and Tilche, A. (2018). Impact Assessment Study on Societal Benefits of Arctic Observing Systems IMOBAR. Luxembourg: European Union, doi: 10.2760/713084
Eicken, H., Lee, O. A., and Lovecraft, A. L. (2016). Evolving roles of Observing Systems and data Co-management in Arctic Ocean Governance, OCEANS 2016 MTS/IEEE Monterey. Monterey, CA: IEEE, 1–8. doi: 10.1109/OCEANS.2016.7761298
Eicken, H., Lovecraft, A. L., and Druckenmiller, M. L. (2009). Sea-ice system services: a framework to help identify and meet information needs relevant for Arctic observing networks. Arctic 62, 119–136.
Eicken, H., and Mahoney, A. R. (2015). “Sea ice: Hazards, risks, and implications for disasters,” in Coastal and Marine Hazards, Risks, and Disasters, eds J. Ellis and D. J. Sherman (Amsterdam: Elsevier), 381–401. doi: 10.1016/b978-0-12-396483-0.00013-3
Farrell, S. L., McAdoo, D. C., Laxon, S. W., Zwally, H. J., Li, D., Ridout, A., et al. (2012). Mean dynamic topography of the arctic ocean,Geophys. Res. Lett. 39:L01601. doi: 10.1029/2011GL050052
Fidel, M., Johnson, N., Danielsen, F., Eicken, H., Iversen, L., Lee, O., et al. (2017). INTAROS Community-based Monitoring Experience Exchange Workshop Report. Alaska, AK: Yukon River Inter-Tribal Watershed Council (YRITWC).
Flath, H., Wilcox, L., Akçelik, V., Hill, J., van Bloemen Waanders, B., and Ghattas, O. (2011). Fast algorithms for bayesian uncertainty quantification in large-scale linear inverse problems based on low-rank partial hessian approximations. SIAM J. Sci. Comput. 33, 407–432. doi: 10.1137/090780717
Flynn, M., Ford, J. D., Pearce, T., and Harper, S. Ihacc Research Team. (2018). Participatory scenario planning and climate change impacts, adaptation and vulnerability research in the Arctic. Environ. Sci. Policy 79, 45–53. doi: 10.1016/j.envsci.2017.10.012
Fu, L.-L., and Ferrari, R. (2008). Observing oceanic submesoscale processes from space. Eos Trans. Am. Geophys. Union 89:488. doi: 10.1029/2008eo480003
Gerland, S., Barber, D., Meier, W., Mundy, C. J., Holland, M., Kern, S., et al. (2019). Essential gaps and uncertainties in the understanding of the roles and functions of Arctic sea ice. Environ. Res. Lett. 14:043002. doi: 10.1088/1748-9326/ab09b3
German Arctic Office. (2019). Co-Operation in Arctic Science — Challenges and Joint Actions. Report of the 2nd Arctic Science Ministerial. Bonn: Federal Ministry of Education and Research, 150.
Giles, K. A., Laxon, S. W., Ridout, A. L., Wingham, D. J., and Bacon, S. (2012). Western Arctic ocean freshwater storage increased by wind-driven spin-up of the Beaufort Gyre. Nat. Geosci. 5, 194–197. doi: 10.1038/NGEO1379
Häkkinen, S., and Proshutinsky, A. (2004). Freshwater content variability in the Arctic ocean. J. Geophys. Res. 109:C03051. doi: 10.1029/2003JC001940
Halliwell, G. R., Srinivasan, A., Kourafalou, V., Yang, H., Willey, D., Le Hénaff, M., et al. (2014). Rigorous evaluation of a fraternal twin ocean OSSE system in the open Gulf of Mexico. J. Atmos. Ocean. Technol. 31, 105–130. doi: 10.1175/JTECH-D-13-00011.1
Hansen, E., Gerland, S., Granskog, M. A., Pavlova, O., Renner, A. H. H., Haapala, J., et al. (2013). Thinning of Arctic sea ice observed in Fram Strait: 1990–2011. J. Geophys. Res. 18, 5202–5221. doi: 10.1002/jgrc.20393
Hansen, M. W., Johannessen, J. A., and Raj, R. P. (2012). “Mapping the Nordic Seas surface velocity using Envisat ASAR,” in Proceedings of the ESA SeaSAR 2012, Tromsø.
Heimbach, P., Wunsch, C., Ponte, R. M., Forget, G., Hill, C., and Utke, J. (2011). Timescales and regions of the sensitivity of Atlantic meridional volume and heat trans- port: toward observing system design. Deep Sea Res. Part 2 Topic. Stud. Oceanogr. 58, 1858–1879. doi: 10.1016/j.dsr2.2010.10.065
Hoffman, R. N., and Atlas, R. (2015). Future observing system simulation experiments. Bull. Am. Meteorol. Soc. 97, 1601–1616. doi: 10.1175/bams-d-15-00200.1
Hunt, G. L. Jr., and Megrey, B. A. (2005). Comparison of the biophysical and trophic characteristics of the Bering and Barents Seas. ICES J. Mar. Sci. 62, 1245–1255. doi: 10.1016/j.icesjms.2005.04.008
Jahn, A. (2018). Reduced probability of ice-free summers for 1.5°C compared to 2°C warming. Nat. Clim. Change 8, 409–413. doi: 10.1038/s41558-018-0127-8
Johannessen, J. A., Chapron, B., Collard, F., Kudryavtsev, V., Mouche, A., Akimov, D., et al. (2008). Direct ocean surface velocity measurements from Space: improved quantitative interpretation of Envisat ASAR observations. Geophys. Res. Lett. 35:L22608.
Johnson, N., Alessa, L., Behe, C., Danielsen, F., Gearheard, S., Gofman-Wallingford, V., et al. (2015). The contributions of community-based monitoring and traditional knowledge to Arctic observing networks: reflections on the state of the field. Arctic 68, 28–40.
Jung, T., Gordon, N. D., Bauer, P., Bromwich, D. H., Chevallier, M., Day, J. J., et al. (2016). Advancing polar prediction capabilities on daily to seasonal time scales. Bull. Am. Meteorol. Soc. 97, 1631–1647. doi: 10.1175/bams-d-14-00246.1
Kalmikov, A., and Heimbach, P. (2014). A hessian-based method for uncertainty quantification in global ocean state estimation. SIAM J. Sci. Comput. 36:S267–S295.
Kalmikov, A. G., and Heimbach, P. (2018). On barotropic mechanisms of uncertainty propagation in estimation of drake passage transport. arXiv
Kaminski, T., Kauker, F., Eicken, H., and Karcher, M. (2015). Exploring the utility of quantitative network design in evaluating Arctic sea ice thickness sampling strategies. Cryosphere 9, 1721–1733. doi: 10.5194/tc-9-1721-2015
Kaminski, T., Kauker, F., Toudal Pedersen, L., Voßbeck, M., Haak, H., Niederdrenk, L., et al. (2018). Arctic mission benefit analysis: impact of sea ice thickness, freeboard, and snow depth products on sea ice forecast performance. Cryosphere 12, 2569–2594. doi: 10.5194/tc-12-2569-2018
Köhl, A., and Stammer, D. (2004). Optimal observations for variational data assimilation. J. Phys. Oceanogr. 34, 529–542. doi: 10.1175/2513.1
Krishfield, R., Toole, J., Proshutinsky, A., and Timmermans, M.-L. (2008). Automated ice-tethered profilers for seawater observations under pack ice in all seasons. J. Atmos. Oceanic Technol. 25, 2091–2105. doi: 10.1175/2008jtecho587.1
Kwok, R. (2004). Annual cycles of multiyear sea ice coverage of the Arctic Ocean: 1999–2003. J. Geophys. Res. 109:C11004. doi: 10.1029/2003JC002238
Kwok, R. (2018). Arctic sea ice thickness, volume, and multiyear ice coverage: losses and coupled variability (1958–2018). Environ. Res. Lett. 13:105005. doi: 10.1088/1748-9326/aae3ec
Kwok, R., and Cunningham, G. F. (2008). ICESat over Arctic sea ice: estimation of snow depth and ice thickness. J. Geophys. Res. 113:C08010. doi: 10.1029/2008JC004753
Kwok, R., and Morison, J. (2016). Sea surface height and dynamic topography of the ice-covered oceans from CryoSat-2: 2011–2014. J. Geophys. Res. Oceans 121, 674–692. doi: 10.1002/2015JC011357
Laxon, S. (1994). Sea ice altimeter processing scheme at the EODC. Int. J. Remote Sens. 15, 915–924. doi: 10.1080/01431169408954124
Laxon, S., Giles, K. A., Ridout, A., Wingham, D. J., Willatt, R. C., Cullen, R., et al. (2013). CryoSat-2 estimates of Arctic sea ice thickness and volume. Geophys. Res. Lett 40, 732–737. doi: 10.1098/rsta.2014.0157
Lee, C. M., and Gobat, J. I. (2006). Acoustic navigation and communication for high-latitude ocean research workshop. EOSTrans. AGU 87:268. doi: 10.1029/2006eo270004
Lee, C. M., and Thomson, J. The Marginal Ice Zone and Arctic Sea State Teams (2017). An autonomous approach to observing the seasonal ice zone in the western Arctic. Oceanography 30, 56–68. doi: 10.5670/oceanog.2017.222
Lee, O., Eicken, H., Kling, G., and Lee, C. (2015). A framework for prioritization, design and coordination of arctic long-term observing networks: a perspective from the US SEARCH program. Arctic 68, 76–88.
Lindsay, R., and Schweiger, A. (2015). Arctic sea ice thickness loss determined using subsurface, aircraft, and satellite observations. Cryosphere 9, 269–283. doi: 10.5194/tc-9-269-2015
Lindsay, R., Wensnahan, M., Schweiger, A., and Zhang, J. (2014). Evaluation of seven different atmospheric reanalysis products in the Arctic. J. Clim. 27, 2588–2606. doi: 10.1175/jcli-d-13-00014.1
Lindsay, R. W., and Zhang, J. (2006). Arctic ocean ice thickness: modes of variability and the best locations from which to monitor them. J. Phys. Oceanogr. 36, 496–506. doi: 10.1175/jpo2861.1
Lindstrom, E., Gunn, J., Fischer, A., McCurdy, A., and Glover, L. (2012). A Framework for Ocean Observing. By the Task Team for an Integrated Framework for Sustained Ocean Observing. Paris: UNESCO, doi: 10.5270/OceanObs09-FOO
Long, D. G., and Hicks, B. R. (2005). Standard BYU QuikSCAT/SeaWinds Land/Ice Image Products, Report. Provo, UT: Brigham Young University.
Loose, N. (2019). Adjoint Modeling and Observing System Design in the Subpolar North Atlantic. Ph.D. dissertation, University of Bergen, Bergen.
Lovecraft, A. L., Meek, C. L., and Eicken, H. (2013). Connecting scientific observations to stakeholder needs in sea ice social-environmental systems: the institutional geography of northern Alaska. Polar Geogr. 36, 105–125. doi: 10.1080/1088937x.2012.733893
Ludvigsen, C. A., Pirazzini, R., Sagen, H., Hamre, T., Sandven, S., Stette, M., et al. (2018). INTAROS Deliverable 2.1. Report on Present Observing Capacities and Gaps: Ocean and Sea Ice Observing System. Research and Innovation Action under EC Horizon 2020 Grant Agreement no. 727890. Brussels: European Commission.
Lumpkin, R., and Pazos, M. (2007). Measuring surface currents with Surface Velocity Program drifters: the instrument, its data, and some recent results. Chapter two of Lagrangian Analysis and Prediction of Coastal and Ocean Dynamics (LAPCOD), eds A. Griffa, A. D. Kirwan, A. J. Mariano, T. Ozgokmen, and T. Rossby (New York, NY: Cambridge University Press).
MacIntyre, K. Q., Stafford, K. M., Conn, P. B., Laidre, K. L., and Boveng, P. L. (2015). The relationship between sea ice concentration and the spatio-temporal distribution of vocalizing bearded seals Erignathus barbatus in the Bering, Chukchi, and Beaufort Seas from 2008 to 2011. Prog. Ocean. 136, 241–249. doi: 10.1016/j.pocean.2015.05.008
Manabe, S., and Stouffer, R. J. (1980). Sensitivity of the global climate model to an increase of CO2 in the atmosphere. J. Geophys. Res. 85, 5529–5554.
Markus, T., Neumann, T., Martino, A., Abdalati, W., Brunt, K., Csatho, B., et al. (2017). The ice, cloud, and land elevation satellite-2 (ICESat-2): science requirements, concept, and implementation. Remote Sens. Environ. 190, 260–273. doi: 10.1016/j.rse.2016.12.029
Marotzke, J., Giering, R., Zhang, K. Q., Stammer, D., Hill, C., and Lee, T. (1999). Construction of the adjoint MIT ocean general circulation model and application to Atlantic heat transport sensitivity. J. Geophys. Res. Oceans 104, 29529–29547. doi: 10.1029/1999jc900236
Martin, S., and Cavalieri, D. J. (1989). Contributions of the Siberian shelf polynyas to the Arctic ocean intermediate and deep water. J. Geophys. Res 94, 12725–12738. doi: 10.1029/JC094iC09p12725
Mikhalevsky, P. N., Sagen, H., Worcester, P. F., Baggeroer, A. B., Orcutt, J., Moore, S. E., et al. (2015). Multipurpose acoustic networks in the integrated Arctic ocean observing system. Arctic 68, 1–17.
Moore, A. M., Arango, H. G., Broquet, G., Edwards, C., Veneziani, M., Powell, B., et al. (2011). The regional ocean modeling system (ROMS) 4-dimensional variational data assimilation systems: part ii - perfor- mance and application to the California current system. Progr. Oceanogr. 91, 50–73. doi: 10.1016/j.pocean.2011.05.003
Moore, A. M., Arango, H. G., and Edwards, C. A. (2017a). Reduced-rank array modes of the California current observing system. J. Geophys. Res. Oceans 123, 452–465. doi: 10.1002/2017jc013172
Moore, A. M., Jacox, M. G., Crawford, W. J., Laughlin, B., Edwards, C. A., and Fiechter, J. (2017b). The impact of the ocean observing system on estimates of the California current circulation spanning three decades. Progr. Oceanogr. 156, 41–60. doi: 10.1016/j.pocean.2017.05.009
Moore, S. E., and Grebmeier, J. M. (2018). The distributed biological observatory: linking physics to biology in the Pacific Arctic region. Arctic 71, (Suppl. 1), 1–7. doi: 10.14430/arctic4606
Morison, J., Kwok, R., Peralta-Ferriz, C., Alkire, M., Rigor, I., Andersen, R., et al. (2012). Changing Arctic Ocean freshwater pathways. Nature 481, 66–70. doi: 10.1038/nature10705
Murray, M. S., Sankar, R. D., and Ibarguchi, G. (2018). The Arctic Observing Summit, Background and Synthesis of Outcomes 2013-2016. Calgary, AB: International Study of Arctic Change (ISAC) Program Office.
Nakamura, N., and Oort, A. H. (1988). Atmospheric heat budgets of the polar regions. J. Geophys. Res. 93, 9510–9524. doi: 10.1029/JD093iD08p09510
National Academies of Sciences, Engineering, and Medicine (2017). Sustaining Ocean Observations to Understand Future Changes in Earth’s Climate. Washington, DC: The National Academies Press, doi: 10.17226/24919
National Research Council (2006). Toward an Integrated Arctic Observing Network. Washington, DC: The National Academies Press, doi: 10.17226/11607
National Research Council (NRC) Committee on Designing an Arctic Observing Network (2006). Toward an integrated Arctic Observing Network. Washington, DC: National Academies Press, 1–182.
Neckles, H. A., Lyons, J. E., Guntenspergen, G. R., Shriver, W. G., and Adamowicz, S. C. (2015). Use of structured decision making to identify monitoring variables and management priorities for salt marsh ecosystems. Estuaries Coasts 38, 1215–1232. doi: 10.1007/s12237-014-9822-5
Nguyen, A., Ocaña, V., Garg, V., Heimbach, P., Toole, J., Krishfield, R., et al. (2017). On the benefit of current and future ALPS data for improving Arctic coupled ocean-sea ice state estimation. Oceanography 30, 69–73. doi: 10.5670/oceanog.2017.223
Nicolaus, M., Katlein, C., Maslanik, J., and Hendricks, S. (2012). Changes in Arctic sea ice result in increasing light transmittance and absorption. Geophys. Res. Lett. 39:L24501. doi: 10.1029/2012GL053738
Nilsson, L. M., Destouni, G., Berner, J., Dudarev, A. A., Mulvad, G., and Odland, J. Ø, et al. (2013). A call for urgent monitoring of food and water security based on relevant indicators for the Arctic. Ambio 42, 816–822. doi: 10.1007/s13280-013-0427-1
Oke, P. R., Larnicol, G., Fujii, Y., Smith, G. C., Lea, D. J., Guinehut, S., et al. (2015). Assessing the impact of observations on ocean forecasts and reanalyses: Part 1, Global studies. J. Oper. Oceanogr. 8, s49–s62. doi: 10.1080/1755876X.2015.1022067
Panteleev, G., Yaremchuk, M., and Nechaev, D. (2009). Optimization of mooring observations in Northern Bering Sea. Dynam. Atmos. Oceans 48, 143–154. doi: 10.1016/j.dynatmoce.2008.11.004
Peacock, N. R., and Laxon, S. W. (2004). Sea surface height determination in the Arctic Ocean from ERS altimetry. J. Geophys. Res. 109:C07001. doi: 10.1029/2001JC001026
Peralta-Ferriz, C., and Morison, J. H. (2014). Arctic ocean circulation patterns revealed by GRACE. J. Clim. 27, 1445–1468. doi: 10.1175/JCLI-D-13-00013.1
Polyakov, I. V., Pnyushkov, A. V., Alkire, M. B., Ashik, I. M., Baumann, T. M., Carmack, E. C., et al. (2017). Greater role for Atlantic inflows on sea-ice loss in the Eurasian Basin of the Arctic Ocean. Science 356, 285–291. doi: 10.1126/science.aai8204
Post, E., Bhatt, U. S., Bitz, C. M., Brodie, J. F., Fulton, T. L., Hebblewhite, M., et al. (2013). Ecological consequences of sea-ice decline. Science 341, 519–524. doi: 10.1126/science.1235225
Preston, B., and Lovecraft, A. L. (2018). “Chapter 8 Scenarios thinking for the Bering-Chukchi-Beaufort Region,” in Adaptation Actions for a Changing Arctic - Perspectives from the Bering/Chukchi/Beaufort Region, ed. AMAP (Oslo: Arctic Monitoring and Assessment Programme (AMAP)), 209–230.
Proshutinsky, A., Ashik, I. M., Dvorkin, E. N., Hakkinen, S., Krishfield, R. A., and Peltier, W. R. (2004). Secular sea level change in the Russian sector of the Arctic Ocean. J. Geophys. Res. 109:C03042. doi: 10.1029/2003JC002007
Prowse, T., Bring, A., Mård, J., and Carmack, E. (2015). Arctic freshwater synthesis: introduction. J. Geophys. Res. Biogeosci. 120, 2121–2131. doi: 10.1002/2015JG003127
Rabe, B., Dodd, P. A., Hansen, E., Falck, E., Schauer, U., Mackensen, A., et al. (2013). Liquid export of Arctic freshwater components through the Fram Strait 1998–2011. Ocean Sci. 9, 91–109. doi: 10.5194/osd-9-91-2013
Raj, R. P., Nilsen, J. E. Ø, Johannessen, J. A., Furevik, T., Andersen, O. B., and Bertino, L. (2018). Quantifying Atlantic water transport to the Nordic seas by remote sensing. Remote Sens. Environ. 216, 758–769. doi: 10.1016/j.rse.2018.04.055
Rampal, P., Weiss, J., Dubois, C., and Campin, J. M. (2011). IPCC climate models do not capture Arctic sea ice drift acceleration: consequences in terms of projected sea ice thinning and decline. J. Geophys. Research 116:C00D07. doi: 10.1029/2011JC007110
Richter-Menge, J., Jeffries, M. O., and Osborne, E. (eds). (2018). The Arctic in “State of the Climate in 2017”. Bull. Amer. Meteor. Soc. 99, S143–S173. doi: 10.1175/2018BAMSStateoftheClimate.I
Rintoul, S. R., Sparrow, M., Meredith, M. P., Wadley, V., Speer, K., Hofmann, E., et al. (2012). The Southern Ocean Observing System: Initial Science and Implementation Strategy. Cambridge: Scientific Committee on Antarctic Research.
Riser, S. C., Freeland, H. J., Roemmich, D., Wijffels, S., Troisi, A., Belbéoch, M., et al. (2016). Fifteen years of ocean observations with the global Argo array. Nat. Clim. Change 5, 145–153. doi: 10.1038/nclimate2872
Rösel, A., Itkin, P., King, J., Divine, D., Wang, C., Granskog, M. A., et al. (2018). Thin sea ice, thick snow, and widespread negative freeboard observed during N-ICE2015 North of Svalbard. J. Geophys. Res. Oceans 123, 1156–1176. doi: 10.1002/2017JC012865
Sagen, H., Dushaw, B. D., Skarsoulis, E. K., Dumont, D., Dzieciuch, M. A., and Beszczynska-Möller, A. (2016). Time series of temperature in Fram Strait determined from the 2008–2009 DAMOCLES acoustic tomography measurements and an ocean model. J. Geophys. Res. 121, 4601–4617. doi: 10.1002/2015JC011591
Sandven, S., Sagen, H., Buch, E., Pirazzini, R., Gustavson, D., Beszczynska-Möller, A., et al. (2018). The in situ component of Arctic observing systems – opportunities and challenges in implementation of platforms and sensors. in Proceedings of the AOS 2018 Statement paper, (Calgary, AB: Arctic Observing Summit).
Schlosser, P., Pfirman, S. L., Pomerance, R., Williams, M., Ack, B., Duffy, P., et al. (2016). A 5°C Arctic in a 2°C World: Challenges and Recommendations for Immediate Action. New York, NY: Columbia University, 10.
Science, and Technology Policy Institute [STPI] Sustaining Arctic Observing Networks [SAON] (2017). International Arctic Observations Assessment Framework. Washington, DC: IDA Science and Technology Policy Institute, 73.
Sigmond, M., Fyfe, J. C., and Swart, N. C. (2018). Ice-free Arctic projections under the Paris agreement. Nat. Clim. Change 8, 404–408. doi: 10.1038/s41558-018-0124-y
Soltwedel, T., Schauer, U., Boebel, O., Nöthig, E.-M., Bracher, A., Metfies, K., et al. (2013). “FRAM - FRontiers in Arctic marine monitoring: Permanent observations in a gateway to the Arctic ocean,” in Proceedings of the OCEANS - Bergen, 2013 MTS/IEEE, (Bergen: IEEE).
Spreen, G., Kwok, R., and Menemenlis, D. (2011). Trends in Arctic sea ice drift and role of wind forcing: 1992–2009. Geophys. Res. Lett. 38:L19501. doi: 10.1029/2011GL048970
Stammer, D., Lyu, G., Pirazzini, R., Naakka, T., Nygaård, T., Vihma, T., et al. (2018). INTAROS D2.12: Observational Gaps Revealed by Model Sensitivity to Observations. Research and Innovation Action under EC Horizon 2020 Grant Agreement no. 727890. Brussels: European Commission.
Starkweather, S., Intrieri, J., Solomon, A., Farrell, S., and Woods, J. (2018). Lessons from the Arctic Observing Framework for Sea Ice Forecasting-A Task of the US Arctic Observing Network (US AON). Washington, DC: AGU Fall Meeting Abstracts.
Stroeve, J., and Notz, D. (2018). Changing state of Arctic sea ice across all seasons. Environ. Res. Lett. 13:103001. doi: 10.1088/1748-9326/aade56
Thomson, J., Ackley, S., Girard-Ardhuin, F., Ardhuin, F., Babanin, A., Boutin, G., et al. (2018). Overview of the Arctic Sea state and boundary layer physics program. J. Geophys. Res.: Oceans 123, 8674–8687. doi: 10.1002/2018JC013766
Toole, J. M., Krishfield, R. A., Timmermans, M.-L., and Proshutinsky, A. (2011). The ice-tethered profiler: argo of the Arctic. Oceanography 24, 126–135. doi: 10.5670/oceanog.2011.64
Vargas-Moreno, J. C., Fradkin, B., Emperador, S., and Lee, O. (2016). Prioritizing Science needs Through Participatory Scenarios for Energy and Resource Development on the North Slope and Adjacent Seas. Boston, MA: GeoAdaptive, LLC.
Volkov, D. L., and Landerer, F. W. (2013). Nonseasonal fluctuations of the Arctic Ocean mass observed by the GRACE satellites. J. Geophys. Res. Oceans 118, 6451–6460. doi: 10.1002/2013JC009341
Volkov, D. L., and Pujol, M.-I. (2012). Quality assessment of a satellite altimetry data product in the Nordic, Barents, and Kara seas. J. Geophys. Res. 117:C03025. doi: 10.1029/2011JC007557
Wassmann, P. (2015). Overarching perspectives of contemporary and future ecosystems in the Arctic Ocean. Progr. Oceanogr. 139, 1–12. doi: 10.1016/j.pocean.2015.08.004
Webster, M., Gerland, S., Holland, M., Hunke, E., Kwok, R., Lecomte, O., et al. (2018). Snow in the changing sea-ice systems. Nat. Clim. Change 8, 946–953. doi: 10.1038/s41558-018-0286-7
Whitlock, J. D. (2018). Autonomous Sea Ice Measurements for the Changing Arctic, Ph.D. dissertation, Dartmouth College, Hanover, NH.
Wilkinson, J., and Julienne, S. (2018). “Polar sea ice as a barometer and driver of change,” in The Routledge Handbook of the Polar Regions London and New York, eds M. Nuttall, T. R. Christensen, and M. J. Siegert (New York, NY: Routledge), 176–184.
Williams, P., Alessa, L., Abatzoglou, J. T., Kliskey, A., Witmer, F., Lee, O., et al. (2018). Community-based observing networks and systems in the Arctic: human perceptions of environmental change and instrument-derived data. Reg. Environ. Change 18, 547–559. doi: 10.1007/s10113-017-1220-7
Willmes, S., Krumpen, T., Adams, S., Rabenstein, L., Haas, C., Hoelemann, J., et al. (2010). Cross-validation of polynya monitoring methods from multisensor satellite and airborne data: a case study from the Laptev Sea. Can. J. Remote Sens. 36, S196–S210.
Woodgate, R. A. (2018). Increases in the Pacific inflow to the Arctic from 1990 to 2015, and insights into seasonal trends and driving mechanisms from year-round Bering Strait mooring data. Progr. Oceanogr. 160, 124–154. doi: 10.1016/j.pocean.2017.12.007
Wunsch, C. (2006). Discrete Inverse and State Estimation Problems: With Geophysical Fluid Applications. Cambridge: Cambridge University Press, doi: 10.1017/CBO9780511535949
Young, O. R. (2009). The Arctic in play: Governance in a time of rapid change. Int. J. Mar. Coastal Law 24, 423–442. doi: 10.1163/157180809x421833
Yumashev, D., Hope, C., Schaefer, K., Riemann-Campe, K., Iglesias-Suarez, F., Jafarov, E., et al. (2019). Climate policy implications of nonlinear decline of Arctic land permafrost, snow and sea ice. Geophys. Res. Abstracts 21:EGU2019-15815.
Keywords: Arctic, observing system, Essential Ocean Variable, autonomous platforms, observing system design, societal benefit areas, Sustaining Arctic Observing Networks, Global Ocean Observing System
Citation: Lee CM, Starkweather S, Eicken H, Timmermans M-L, Wilkinson J, Sandven S, Dukhovskoy D, Gerland S, Grebmeier J, Intrieri JM, Kang S-H, McCammon M, Nguyen AT, Polyakov I, Rabe B, Sagen H, Seeyave S, Volkov D, Beszczynska-Möller A, Chafik L, Dzieciuch M, Goni G, Hamre T, King AL, Olsen A, Raj RP, Rossby T, Skagseth Ø, Søiland H and Sørensen K (2019) A Framework for the Development, Design and Implementation of a Sustained Arctic Ocean Observing System. Front. Mar. Sci. 6:451. doi: 10.3389/fmars.2019.00451
Received: 12 February 2019; Accepted: 05 July 2019;
Published: 08 August 2019.
Edited by:
Tong Lee, NASA Jet Propulsion Laboratory (JPL), United StatesReviewed by:
Patrick Heimbach, The University of Texas at Austin, United StatesAnanda Pascual, Instituto Mediterráneo de Estudios Avanzados (IMEDEA), Spain
Copyright © 2019 Lee, Starkweather, Eicken, Timmermans, Wilkinson, Sandven, Dukhovskoy, Gerland, Grebmeier, Intrieri, Kang, McCammon, Nguyen, Polyakov, Rabe, Sagen, Seeyave, Volkov, Beszczynska-Möller, Chafik, Dzieciuch, Goni, Hamre, King, Olsen, Raj, Rossby, Skagseth, Søiland and Sørensen. This is an open-access article distributed under the terms of the Creative Commons Attribution License (CC BY). The use, distribution or reproduction in other forums is permitted, provided the original author(s) and the copyright owner(s) are credited and that the original publication in this journal is cited, in accordance with accepted academic practice. No use, distribution or reproduction is permitted which does not comply with these terms.
*Correspondence: Craig M. Lee, Y3JhaWdsZWVAdXcuZWR1