- 1GEOMAR Helmholtz Centre for Ocean Research Kiel, Kiel, Germany
- 2IFREMER, Plouzané, France
- 3Jet Propulsion Laboratory, California Institute of Technology, Pasadena, CA, United States
- 4Joint Institute for the Study of the Atmosphere and Ocean, University of Washington, Seattle, WA, United States
- 5Southern Ocean Observing System, University of Tasmania, Hobart, TAS, Australia
- 6NIOZ Royal Netherlands Institute for Sea Research, and Utrecht University, Texel, Netherlands
- 7National Oceanography Centre–British Oceanographic Data Centre, Liverpool, United Kingdom
- 8NOAA Pacific Marine Environmental Laboratory, Seattle, WA, United States
- 9NOAA National Centers for Environmental Information, Silver Spring, MD, United States
- 10Scripps Institution of Oceanography, University of California, San Diego, La Jolla, CA, United States
- 11Istituto Nazionale di Oceanografia e di Geofisica Sperimentale, Sgonico, Italy
- 12British Geological Survey, Nottingham, United Kingdom
- 13Woods Hole Oceanographic Institution, Woods Hole, MA, United States
- 14Instituto de Oceanografia, Universidade Federal do Rio Grande, Rio Grande, Brazil
- 15ETT, Genova, Italy
- 16Bjerknes Centre for Climate Research, University of Bergen, Bergen, Norway
- 17National Snow and Ice Data Center, University of Colorado Boulder, Boulder, CO, United States
- 18Royal Belgian Institute for Natural Sciences, Brussels, Belgium
- 19Earth Science Information Partners, Boulder, CO, United States
- 20MARIS Mariene Informatie Service, Voorburg, Netherlands
- 21Arctic Portal, Akureyri, Iceland
- 22GODAE Ocean Services, Melbourne, VIC, Australia
- 23U.S. Integrated Ocean Observing System, Silver Spring, MD, United States
- 24Fisheries and Oceans, Science Branch, Maritimes Region Ocean Data and Information Section, Dartmouth, NS, Canada
- 25American Geophysical Union, Washington, DC, United States
- 26Norwegian Polar Institute, Tromsø, Norway
- 27National Computational Infrastructure, Australian National University, Canberra, ACT, Australia
- 28Informatics Institute, University of Amsterdam, Amsterdam, Netherlands
Well-founded data management systems are of vital importance for ocean observing systems as they ensure that essential data are not only collected but also retained and made accessible for analysis and application by current and future users. Effective data management requires collaboration across activities including observations, metadata and data assembly, quality assurance and control (QA/QC), and data publication that enables local and interoperable discovery and access and secures archiving that guarantees long-term preservation. To achieve this, data should be findable, accessible, interoperable, and reusable (FAIR). Here, we outline how these principles apply to ocean data and illustrate them with a few examples. In recent decades, ocean data managers, in close collaboration with international organizations, have played an active role in the improvement of environmental data standardization, accessibility, and interoperability through different projects, enhancing access to observation data at all stages of the data life cycle and fostering the development of integrated services targeted to research, regulatory, and operational users. As ocean observing systems evolve and an increasing number of autonomous platforms and sensors are deployed, the volume and variety of data increase dramatically. For instance, there are more than 70 data catalogs that contain metadata records for the polar oceans, a situation that makes comprehensive data discovery beyond the capacity of most researchers. To better serve research, operational, and commercial users, more efficient turnaround of quality data in known formats and made available through Web services is necessary. In particular, automation of data workflows will be critical to reduce friction throughout the data value chain. Adhering to the FAIR principles with free, timely, and unrestricted access to ocean observation data is beneficial for the originators, has obvious benefits for users, and is an essential foundation for the development of new services made possible with big data technologies.
Introduction
Well-functioning and fit-for-purpose data management systems are essential to the sustained ocean observing system. This quote from the Intergovernmental Oceanographic Commission (IOC) of United Nations Educational, Scientific, and Cultural Organization (UNESCO) Oceanographic Data Exchange Policy articulates the high-level mandate for and the essence of the requirements for oceanographic data flow:
“The timely, free and unrestricted international exchange of oceanographic data is essential for the efficient acquisition, integration and use of ocean observations gathered by the countries of the world for a wide variety of purposes including the prediction of weather and climate, the operational forecasting of the marine environment, the preservation of life, the mitigation of human-induced changes in the marine and coastal environment, as well as for the advancement of scientific understanding that makes this possible.”
It is worthwhile to note that the IOC member states, in this policy, agreed to “provide timely, free and unrestricted access to all data, associated metadata and products generated under the auspices of IOC programs,” and encouraged the member states to do the same also for non-IOC programs. Although this is an excellent aspiration, in practice, this principle has been only loosely enforced by the IOC.
Fit-for-purpose data management systems are of vital importance as they ensure that essential data are not only collected but also retained and made accessible for analysis and application for current and future users. Data management systems that facilitate free and open access, use, and interpretation of data and products must be included as essential elements of the ocean observing system. Effective data management is based on collaboration across activities including observing, metadata and data assembly, quality assurance and control (QA/QC), and data publication. It enables local and interoperable discovery and access and secures archiving that guarantees long-term preservation.
As ocean observing systems evolve with an increasing number of autonomous platforms and sensors being deployed, measuring an increased range of essential ocean variables (EOVs), the volume and diversity of data are increasing dramatically. Automation of data workflows1 and effective standards will be critical to reduce data friction throughout the whole data life cycle (e.g., Taylor et al., 2006). This increased efficiency is relevant for all data types, from physical observations to biogeochemical observations and biological and ecosystem observations. With the development of information technologies, researchers expect easy access to a wide range of data and data products. As it becomes easier to aggregate huge amounts of data, the risk of mixing “apples with oranges” increases if the delivery services are not well designed and the data and data products are not clearly described using standardized schemas.
The challenge of enabling optimal use of research data and methods is complex with multiple stakeholders: researchers wanting to share their data and interpretations; professional data publishers offering their services; software and tool builders providing data analysis and processing services; funding agencies (private and public) increasingly concerned with proper data stewardship; and a data science community mining, integrating, and analyzing the output to advance discovery. Computational analysis to discover meaningful patterns in massive, interlinked datasets is rapidly becoming a routine research activity.
The global ocean data system should be designed as an interoperable system of systems that will allow data to be easily findable, accessible, interoperable, and allowing reusability through thematic integrated products and services. The long-term goal is to develop a data system of systems that allows the development of data services at different levels with a guarantee that the best version of the observed data is used at all levels. Data quality, interoperability, and good discoverability can only be assured with a standardized, traceable workflow throughout the lifetime of the datasets. This paper reviews recent developments in technical capacity and requirement setting of a data management system for the Global Ocean Observing System (GOOS). The focus is on EOVs, and the content reflects the increased attention to biogeochemical and biological ecosystem EOVs, building on successes of the physical data system that has evolved the fastest.
The Challenges
Over previous decades, the requirements for ocean information on an ever-increasingly diverse range of issues have increased. In the past, data management systems have largely developed in isolation and with different objectives to serve particular communities or funding routes. Here, we list some of the main challenges in moving ocean data management toward the FAIR principles of being findable, accessible, interoperable, and reusable (see next section for more details).
Wide Diversity
The diversity of oceanographic data makes it difficult for the scientist or application developer to find, understand, and use data to optimal benefit. Significant time is invested in these activities before the actual research or data utilization can begin, while provenance and traceability are required for the sake of reproducibility. While automation can bring improved efficiencies to data management for some data types, there is highly variable uptake of these automation methods, and some disciplines will require considerable progress in standardizing observation methods and data management processes before they can take full advantage of these advances.
Multitude of Disparate Data Management Structures
The existence of a multitude of disparate data management infrastructures currently imposes problems for observing systems. These include delayed and duplicated data receipts, versioning issues, missing data and metadata, and undocumented data processing procedures. The interoperability issues resulting from the existence and use of various data management infrastructures are fundamental and wide ranging. Resources are often not available to resolve these issues by wholesale replacement of existing systems. For instance, there are more than 70 data catalouges for the Polar Ocean, see Box 1.
BOX 1. Polar Oceans.
The Southern Ocean links the world’s major ocean basins and the upper and lower levels of global ocean circulation (Meredith et al., 2013). Research in this region is largely conducted under the auspices of the Antarctic Treaty System (https://www.ats.aq/e/ats.htm). The polar oceans provide a particular example of the need for global cooperation, given the logistical challenges of working in high-latitude environments and the strategic and scientific importance to nations well beyond those that share geographic borders with the polar regions. The distances and logistical challenges in these oceans mean that much oceanographic research is conducted by national polar research programs rather than purely oceanographic programs. In data management terms, the data centers serving these communities must meet data and metadata standards for both terrestrial and national data communities, in addition to those agreed in the international oceanographic community. Serving ‘many masters’ as these polar data centers do highlights the need for oceanographic data managers to use agreed standards. More than 70 metadata catalogs hosting polar data have been identified, many of which are not part of metadata federations or aggregations and, hence, put comprehensive data discovery beyond the capacity of most researchers.
In order to achieve FAIR, the Southern Ocean Observing System (SOOS) explicitly accounts for differences in the workflows and levels of technological integration among its scientific communities and seeks to make data available through a variety of paths to “meet each scientist where they are.” SOOS, along with the EMODnet Physics group, is making standardized and aggregated in situ datasets available for exploration and download through SOOSmap (http://www.soos.aq/news/current-news/362-explore-southern-ocean-soosmap). For the long tail of non-standardized data, SOOS is working with Arctic and Antarctic data management groups to investigate the best ways to achieve federated metadata search and to ensure that the EMODnet/SOOSmap infrastructure can be directly linked to virtual labs. SOOS is encouraging scientists in its community to use existing data discovery tools to identify key datasets that should be standardized and federated to publish through SOOSmap. Along this line, SOOS, the Standing Committee on Antarctic Data Management (SCADM), and the Arctic Data Committee (ADC) have founded the POLDER (http://www.soos.aq/data/federatedsearch) initiative (Polar Data Discovery Enhancement Research) to identify and advocate the needs of the polar data community in the development of federated metadata search for polar oceanographic and terrestrial data. The activities to date, described above, have largely focused on the findability and accessibility of polar ocean data, and it is likely that these will continue to be significant activities for the next decade. As new observing technologies develop, observing systems are encouraging researchers to standardize formats and QC processing, which should considerably improve on the interoperability and reusability of those datasets.
Increased Volume of Data
The past 10 years have seen the development of autonomous platforms able to acquire accurate measurements during years-long deployments (e.g., Argo, glider, moorings, and ships-of-opportunity). These platforms are transmitting as much data in 1 year as has been acquired in the past century (Figure 1). This rapid increase in data volume puts high demands even on well-organized and interoperable data management systems. Not only is the real time (RT) in situ data increasing in volume, but also new variables, particularly for biogeochemistry and biology, are frequently being transmitted in RT. This has also resulted in a concomitant increase in the workload for delayed-mode data processing that corrects for biases that accumulate during time spent at sea.
New Sensors Creating New Formats
A major challenge is the management of novel data types produced from new sensors that require mapping to existing standards and conventions or the creation of new ones.
Widely Used Formats Not Universally Applicable
Implementation of widely used standards and formats can be beyond the capabilities of many scientific communities, even when the benefits of using those formats are clear. While some progress has been made through tools like the National Oceanic and Atmospheric Administration (NOAA) National Centers for Environmental Information’s Network Common Data Form (NetCDF) templates2, which help data producers across disciplines create Climate and Forecast (CF) and Attribute Conventions Dataset Discovery (ACDD)-compliant NetCDF, truly comprehensive adoption has not yet been achieved. IT activities are typically funded by science activities, so the requirements of particular science communities may be addressed effectively. However, this can be at the expense of universal interoperability.
Gap Between Data-Producing Scientists and Downstream Users of the Data
It is incumbent upon our community to develop or leverage existing tools that can bridge the gap between data-producing scientists and downstream users of the data, i.e., to remove barriers from “owning data” to “sharing data” for maximal community benefit would require cultural changes. Efficient (FAIR) sharing of data is a social responsibility of scientists, mostly funded by the society, to support the blue economy and ocean information building.
Development of Common Protocols Takes Time
As identified by de La Beaujardière et al. (2010) and Hankin et al. (2010), the development and adoption of common standards for data/metadata (Keeley et al., 2010) and sharing protocols (Pouliquen et al., 2010) take time, coordination, and careful testing.
Best Practices Poorly Defined
At present, best-practice data management (Pearlman et al., 2019) is often largely undefined and is generally left as a decision for the data curator and/or data publisher, although community standards for metadata, data formats, communication protocols, and data server software infrastructure are the foundation for interoperability. Data that are poorly documented can be considered lost and will have little or no value without access to the team that collected the data. Even the research team that collected the data will be challenged to remember details, or find notes, on how the data were collected if it is not properly curated at the time of collection and review.
The Fair Principles
Open and free data policies are widely encouraged and increasingly required by many organizations, including the IOC and International Oceanographic Data and Information Exchange (IODE), the European Commission, and the Antarctic Treaty System, as well as many funding and operational agencies. Interoperability among data services has become a priority with the development of the FAIR principles3, a set of guiding principles to make data:
• Findable
Each dataset should be identified by a unique persistent identifier and described by rich, standardized metadata that clearly include the persistent identifier. The metadata record should be indexed in a catalog and carried with the data.
• Accessible
The dataset and its metadata record should be retrievable by using the persistent identifier and a standardized communications protocol. In turn, that protocol should allow for authentication and authorization, where necessary. All metadata records should remain accessible even when the datasets they describe are not easily accessible.
• Interoperable
Both metadata and datasets use formal, accessible, shared, and broadly applicable vocabularies and/or ontologies to describe themselves. They should also use vocabularies that follow FAIR principles and provide qualified references to other relevant metadata and data. Importantly, the data and metadata should be machine accessible and parsable.
• Reusable
To meet this principle, data must already be findable, accessible, and interoperable. Additionally, the data and metadata should be sufficiently richly described that it can be readily integrated with other data sources. Published data objects should contain enough information on their provenance to enable them to be properly cited and should meet domain-relevant community standards.
The FAIR data principles are gaining increasing traction across all scientific domains, triggered by an important publication that radically influenced data management and data sharing developments (Wilkinson et al., 2016). The FAIR principles specifically focus on machine use of data and metadata because this is more difficult than achieving FAIR data for human users, whose intuitive sense of semantics and ability to infer meaning from contextual cues assists them in navigating non-standardized datasets and their metadata descriptions. While full implementation of the FAIR principles may rarely be achieved, these principles are designed to encourage data managers to take steps along a continuum from unstructured, undocumented data to fully FAIR data (Wilkinson et al., 2016).
As ocean observing systems develop, they will need to account for the patchy legacy of data management approaches. Data management infrastructure—both technical and human—will need to be flexible to assist those disciplines and nations without access to sophisticated data management systems to make their data FAIR, while also encouraging continued development toward the FAIR principles from those disciplines and nations with a strong history of data management.
Oceanographic data systems generally possess a high level of FAIRness compared to many other disciplines. The major, but certainly not only, hurdle today is machine-to-machine aspects of interoperability. At the same time, we acknowledge that in some disciplines, it is important to the scientists who collect a dataset that they be given the opportunity to publish their findings before the data are made public. There is nothing in the FAIR principles that precludes data originators from embargoing their data for a limited period, even though the sustained ocean observing system heavily relies on timely availability of data. We believe that giving scientific data originators the option of embargo can be important to maintain confidence in the data management system.
The Past Decade
The process of developing a multidisciplinary, integrated ocean observing system for operational uses, including sustained scientific research, follows the guidelines of the framework for ocean observing (Lindstrom et al., 2012). This framework was a major outcome of the OceanObs’09 conference and was developed through sponsorship of IOC, and the implementation is coordinated by the GOOS. Important aspects of the Framework of Ocean Observing (FOO) are the focus on EOVs and the expansion of GOOS to cover the biogeochemical and biological/ecological domains as well as physical variables. During the last few years, the ocean community has been working toward agreeing on a set of EOVs for physics, biogeochemistry, and biology/ecosystems. As a result, ocean data management systems have put emphasis on servicing the need to observe and report on EOVs for sustained ocean observing systems.
Both the means to acquire these data and the way they are used have evolved greatly in the past 10 years. In the past decade, new Global Data Assembly Centers (GDACs) were set up for some in situ networks, applying the OceanObs’09 recommendations (Pouliquen et al., 2010). In their contribution to OceanObs’09, (Hankin et al., 2010) recommended key areas in which oceanographic data managers should focus their attention during the decade that is now coming to a close. Their recommendations focused on what they considered pragmatic and realistic actions to improve the accessibility and interoperability of ocean-focused data. These included (1) working toward adopting common standards for data and metadata building on progress made in the past decade (Keeley et al., 2010); (2) establishment of a single entry point (GDAC) concept to network data or for aggregated products (Pouliquen et al., 2010); (3) the use of common standards that allow systems to interoperate; (4) leveraging the efforts of commercial search engines through the adoption of Web services with tools (Blower et al., 2010); (5) developing and adopting data models based on sampling geometry; (6) developing semantic Web tools to allow straightforward translations between metadata standards; and (7) specific recommendations for meteorological data, data archiving processes, biological data, satellite data, and software toolkits for systems developments. In particular, they advocated for all ocean observations to be made universally accessible through CF-compliant NetCDF files using common vocabularies served through Open Geospatial Consortium (OGC) Web services or commonly used tools such as Open-source Project for a Network Data Access Protocol (OPeNDAP) servers.
At the time of writing, these formats are already widely used for model outputs and satellite products. The last decade has seen the introduction of the discrete sampling geometries (DSG) into the CF standard as of version 1.6, released in December 2011. These geometries are designed to provide representations for in situ features such as time series, vertical profiles, and surface trajectories. More mature networks are currently implementing these features in their NetCDF data representations. Recently, these standards have also been embraced by the biogeochemical community, e.g., the Surface Ocean CO2 Atlas (SOCAT) project uses NetCDF, CF-compliant DSG as the backbone of its data ingest and QC system. The European SeaDataNet community, working on standards for validated, archived data, has adopted Ocean Data View (ODV) ASCII format as well as SeaDataNet NetCDF CF for the observation datasets (profiles, time series, and trajectories), and NetCDF CF for its data products such as climatologies. However, the biological communities have not embraced the CF conventions to describe their data but have converged on different standards; e.g., biological data standards are curated by the Biodiversity Information Standards (TDWG). The most popular standard for sharing biodiversity information is Darwin Core, which enables integration between the two largest communities, the Global Biodiversity Information Facility (GBIF) and Ocean Biogeographic Information System (OBIS). The difference between physical and biological data standards has likely to do with the requirement (RT vs. delayed-mode data delivery), the amount of data to be handled (physical data tend to have significant higher volume), and the methods of data collection.
In addition to developments within the oceanographic community, key interdisciplinary communities have emerged to address data informatics topics common to multiple communities. These include the American Geophysical Union (AGU) and European Geophysical Union (EGU) Earth Space and Science Informatics groups and the Research Data Alliance (RDA). Examples of efforts that have had links to these communities are the FAIR principles and developments in the use of Digital Object Identifiers (DOI).
Progress in information technologies over the past decade with an increase in data services available via the internet has led to the emergence of new computing paradigms and technologies such as high-performance and high-throughput computing; cloud, edge, and fog computing; big data analytics; machine learning; and virtual research environments. This opens up significant opportunities but requires FAIR data management practices to be implemented. One example demonstrating how embracing new technologies has improved data access for uses occurred when NOAA’s Big Data Project (BDP) partnered with Amazon Web Services (AWS) to provide access to the complete historical archive of the Level-II Next Generation Weather Radar (NEXRAD). NEXRAD data are used for a variety of purposes, including weather forecasting, water management, etc. With these data available in the cloud, the data were accessed 2.3 times as often as the historical monthly access rates4, indicating it was much easier to find and use for data consumers. Similarly, within the European H2020 ENVRIplus project, a subscription system was developed, allowing faster subsampling on the full Argo dataset, and semantic search based on FAIR Argo metadata system is under development using Elasticsearch and big data technologies.
Another example is SeaDataNet, which connects in excess of 110 data centers in Europe and gives harmonized discovery and access to a large volume of marine and ocean datasets. For this purpose, SeaDataNet dynamically maintains so-called data buffers for specific parameters. SeaDataNet is also performing data discovery and access as well as data buffer services for several European Marine Observation and Data Network (EMODnet) projects. Several data products are delivered with DOI, OGC Web services, NetCDF (CF), and other formats, depending on their user communities. In addition, all products carry SeaDataNet PIDs and related metadata for the used basis datasets for acknowledging data originators and following FAIRness principles. The experience is that these harmonized and validated data products are popular with users, encouraging more data centers to join the marine data infrastructures for standardized exchange. SeaDataNet is making good progress with developing a collaborative and high-performing cloud and virtual research environment (VRE), configured with tools and services for processing essential marine data. Using OGC, ISO, and W3C standards and incorporating scientific expertise, dynamic workflows are configured for analyzing, processing, and combining subsets of data. The VRE and workflows will allow data product teams to work more efficiently for processing large amounts of input datasets and generating data products collaboratively, while also adopting innovations like machine learning for QA/QC of large data collections. This way, the production cycle for data products can be reduced in duration and higher-quality products can be achieved.
Hankin et al. (2010) provided a series of predictions and recommendations for how oceanographic data management systems would evolve over the past decade. Their prediction that data management would likely improve incrementally rather than in ‘heroic leaps’ has held true, though some of their other predictions have proved overly optimistic. Despite considerable progress and effort toward the goals outlined by Hankin et al. (2010), oceanographic data are generally not yet managed through independent and interoperable data management systems, forming a system of systems. Semantic interoperability tools are only patchily translating terminology, codes, conceptual models, and relationships across data and metadata standards. Progress has been made on all of these fronts, but true international interoperability seems only to have been achieved for a small fraction of the kinds of data being collected in the world’s oceans. Excellent progress has been made when it comes to many physical and meteorological variables needed by the operational ocean community and on validated archives of marine data via metadata standards and semantics like the British Oceanographic Data Centre’s (BODC) Natural Environment Research Council (NERC) Vocabulary Server. However, the biogeochemical (BGC) and biological data communities are still striving for improvement and need increased and sustained funding to meet observing systems’ needs. There are multiple reasons for the slower progress of BGC and biological communities when it comes to data interoperability. These communities are largely operating more in “research mode,” with low requirement for fast and interoperable data exchange and with a large and complex set of variables being measured. The definition of EOVs and wider acceptance of best practices will likely help remedy the situation.
The Current Situation
A great abundance of regularly acquired environmental data exist for a wide range of disciplines derived from both in situ and remote sensing observing platforms, available in real-time, near real-time, and delayed modes. These data are acquired within routine monitoring activities and scientific surveys by a few thousand institutes and agencies around the world. A number of projects have been working of improving data management practices for sustained ocean observing, for instance the AtlantOS project (see Box 2).
BOX 2. AltantOS.
AltantOS is an EU-funded project with the aim of enhancing and optimizing the integrated Atlantic Ocean Observing System. The targeted European data system within the AtlantOS project enhances and integrates existing data systems to ingest and deliver more in situ data. The existing data systems are diverse, and integrators are charged with integrating the data streams. These integrated system are mature systems with long-term experience and established procedures for data collection and management, often agreed at an international level; trying to implement a sovereign and rigid set of rules would be highly challenging and not in the best interest of the observing system. By relying on sustained infrastructure, AtlantOS has furthered the implementation of the FAIR principles for Atlantic observations, achieved through a system of systems where ocean observations are made available to users on a free and unrestricted basis, ensuring timely, full, and open exchange of data, metadata, and products. This includes improving interfaces with observing networks, European monitoring centers, European nations’ oceanographic data centers, and the way exiting integrators exchange data and products using FAIR principles. AtlantOS recommended to integrate existing standards and protocols, rather than reinvent the wheel, by first implementing a minimum set of mandatory information for metadata. Using agreed vocabularies in the data processing and distribution chain allows for traceability of the observations. AtlantOS encourages open and free data policy and focuses on data quality by implementing a set of common near real-time QC procedures for seven EOVs acquired in near real time. AtlantOS enhances access to network data by setting up a unique entry point to discover and download existing data, either by integrating the data in existing Global Data Centers or by setting up new ones, recognizing the importance of existing integrators. The enhancement of monitoring facilities offered by JCOMM in situ Observations Programme Support Centre (JCOMMOPS) associated with the documentation of existing services through a unique catalog is an important element for the development of integrator services. They also allow efficient connection to the Global Earth Observation System of Systems (GEOSS).
Increasingly, scientists directly consider societal needs and benefits, policy dimensions, environmental health, business needs (“blue economy”), and the operational utility of their research. The societal (both from the public and private sector actors) need of ocean information is increasing as society is relying more and more on the ocean for food, energy, natural materials, transport, etc. This results in integrative research that includes the social sciences and humanities as part of a much-needed holistic perspective on environmental change. It also introduces the need to ensure that open data policies consider ethical dimensions of such policies. While the default should be fully open data, in some cases (e.g., personally identifiable information, health data, sensitive species information, some indigenous knowledge, etc.), specific management and dissemination methods must be employed to avoid harm (i.e., aggregation, anonymization, etc.).
Data management practices across oceanographic sciences are highly variable in terms of their sophistication and the levels of support they offer to data providers to make their data available in a timely, free, and unrestricted manner. A good practice example is in the Argo program where observations from floats are uploaded by satellite link to national Data Assembly Centres (DACs) where homogeneous automated QC processes are applied, and the data made available rapidly through two Global Data Assembly Centres (GDACs) that synchronize their data holdings many times a day, see Box 3. All data from this program are open and free, in a highly standardized format, which allows data users to aggregate it, subset it, and manipulate it with comparative ease. Figure 2 illustrates typical interfaces between observing networks and data systems, and Figure 3 illustrates the need for data management systems to cover a variety of scales.
BOX 3. Argo.
Since its design in 2001, Argo data have become the dominant source of in situ data in the physical oceanographic community. The national DACs receiving data via satellite transmission decode and QC the data according to a set of RT automatic tests. Erroneous data are corrected or flagged and then sent to two GDACs and the GTS. The GDACs collect data from the 11 DACs, synchronize their databases daily, and serve the resulting data products on FTP sites (http://www.argodatamgt.org/Access-to-data/Access-via-FTP-on-GDAC). The Argo Information Centre (AIC http://argo.jcommops.org/) monitors the status of the Argo program, including data distribution and metadata on float location, model, transmission system, owner, and other variables. The Argo Regional Centers (ARCs) perform a variety of tasks including coordinating float deployments, consistency checks on delayed-mode QC, finding additional reference data for delayed-mode work, adopting floats for delayed-mode QC, and producing Argo data products.
Argo supports FAIR principles with an open and free data policy both in real time and in delayed mode. The FAIR principles are also supported by the Argo team to enhance the interoperability of the Argo data system. Argo was the first network to apply a unique ID for each float in the program (unique WMO numbers) and has worked with the RDA to develop a strategy for managing DOIs for continuously increasing datasets (DOI with a monthly tag for the GDAC monthly snapshots) (http://www.argodatamgt.org/Access-to-data/Argo-DOI-Digital-Object-Identifier). The metadata attached to a float, containing information such as serial number of the sensors and other technical information, support analysis from the Argo GDAC, a strategy that has shown its efficiency when anomalies were detected. QC of Argo data involves a complex sequence of both automatic and manual tests to produce delayed-mode data of high quality. With 400 profiles daily, the burden on human resources dedicated to QC is large and Argo is investigating machine learning solutions to improve the process.
While the complete data chain has been developed for the Argo core mission (P/T/S, 0-2000 dbar), the extension to Deep Argo and Biogeochemical (BGC) Argo is under development with a similar philosophy for the data system, realizing the increased need for resources to accommodate new data streams (Roemmich, 2019).
Since Argo is often used in conjunction with data from other platforms, an important next step is enhancing the interoperability of Argo data with other data systems by implementing the FAIR principles. Improving the FAIRness of the Argo data system may require updates in data and metadata formats as standards and user requirements evolve. Serving high-quality data is a top priority for Argo, and comparison with other observing systems will help each system improve data quality and services.
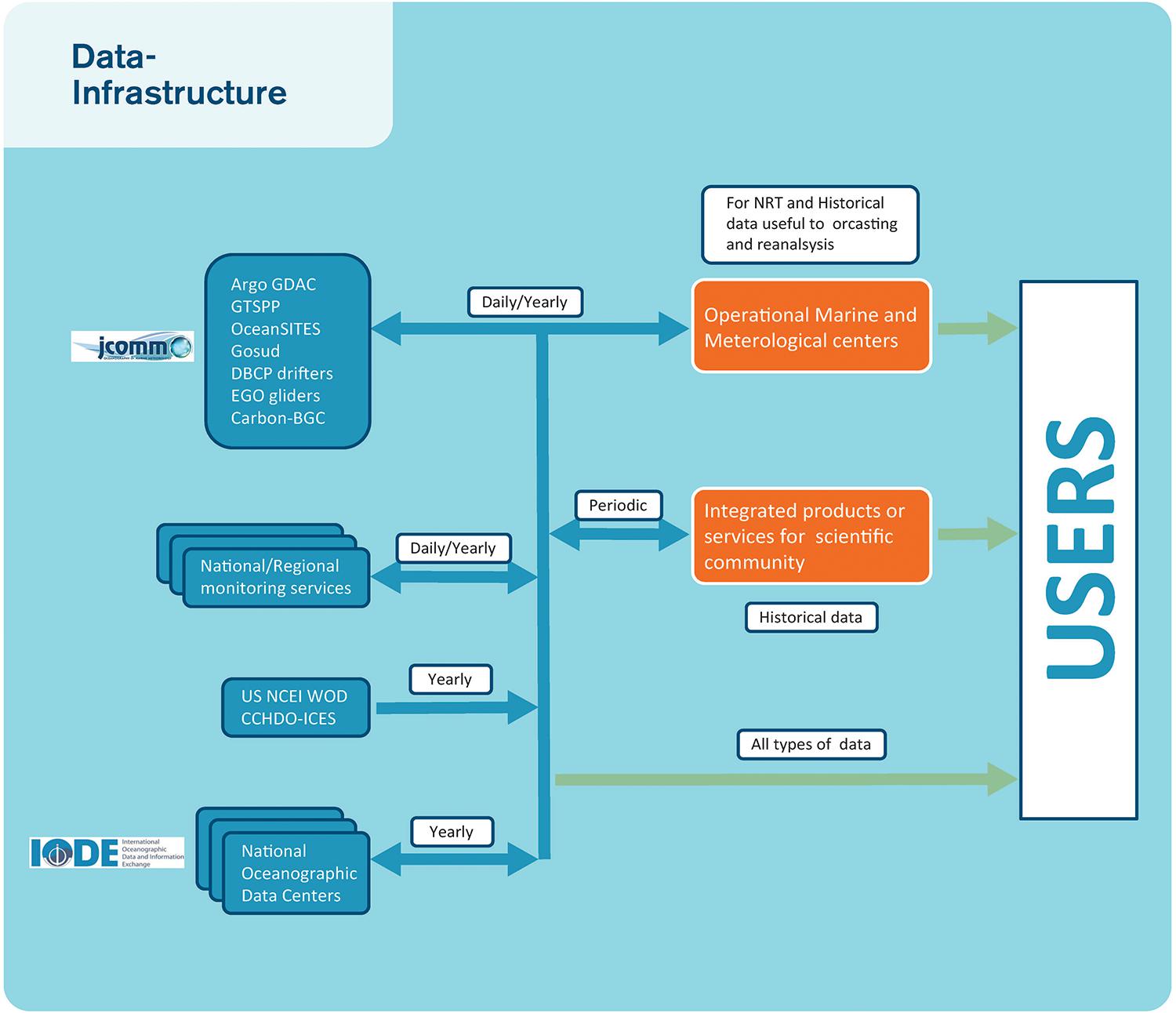
Figure 2. An example of interfaces with observing networks, monitoring centers, and Nations Oceanographic data centers needed to build user products and how the integrators can collaborate together exchanging data and products using FAIR principles.
Tools, such as Environmental Research Division’s Data Access Program (ERDDAP), that allow scientists to work in their format of choice, but make the data available through interoperable formats, such as NetCDF and Web services, without an added burden on the scientist, are crucial to increasing interoperability. Additionally, tools such as ODV, Thematic Real-time Environmental Distributed Data Services (THREDDS), and ERDDAP can reduce the technical barrier that NetCDF presents. For real-time data, the need for interoperable data streaming (for instance by piping different processes) has been partially borne out of the technological context (i.e., digital sensors) and of the impracticality of data transformations after the operational time of the sensor. Although real-time data exchange for many EOVs has been operational for decades, facilitated by World Meteorological Organization (WMO) standards, it is still difficult for non-operational users to get access to GTS data (see Box 4). In the European context, the EMODnet (Miguez et al., 2019) physics is trying to bridge the gap between real-time and delayed-mode data streams by linking existing data management systems developed in both communities, see Box 5. In addition, the Joint Technical Committee for Oceanography and Marine Meteorology (JCOMM) Observations Coordination Group (OCG) has led a successful pilot project to improve both distribution and access of real-time data from the Global Telecommunication System (GTS) Pinardi (2019). Leveraging and ensuring a sustainable and consistent implementation of such tools are also crucial in an era where funding is tight and where data management is often an afterthought. However, increasingly, management planning is obligatory, and funding and allocation of costs for data management and adopting FAIR principles are now encouraged. One example would be Argo (see text Box 3).
BOX 4. Evolution of the WMO Information System (WIS).
The GTS, implemented by the WMO, has been successful in meeting its primary objective—the cost-effective dissemination of meteorological information in near RT. However, the GTS was developed in a period when teletype communication was the norm and, as such, the capabilities of this system no longer meet the needs of the modern era. While the GTS is well managed, reliable, and effective, it is also limited in capability, expensive, complex, and with restricted access. To address the shortcomings of the GTS and expanding the data services offered by the WMO, the WIS offers three services areas: (1) routine collection and dissemination service for time-critical and operation-critical data and products; (2) data discovery, access, and retrieval service; and (3) timely delivery service for data and products. WIS plans to expand the GTS offerings through the utilization of public networks, including the global Internet. This service area holds great promise to provide greater access to WMO data services5. The WIS does present a unique opportunity to increase the dissemination of marine observations and modeling data for use in operational forecasting. One such opportunity is for the WIS to adapt data transmission message standards to accommodate new platforms and current data conventions used in the oceanographic community. Through this evolution, the hope is that the WIS will provide not only greater access to near RT data but also streamlined procedures for data publishing without the procedural overhead now imposed by the GTS.
BOX 5. European marine data initiatives.
At the European scale, enhancing in situ data observation and product FAIRness has been identified as a priority, and collaboration between the different actors has been fostered through the EuroGOOS “DATA Management, Exchange and Quality” (DATAMEQ) working group. Within DATAMEQ, close collaboration is fostered between the observing network operators, the regional monitoring systems within EUROGOOS, and the main infrastructures dealing with in situ observations Copernicus Marine Service (CMEMS), SeaDataNet, and EMODnet. In particular, important are agreements on vocabularies, agreements on common QC automatic procedures for a selection of EOVs, improvements on interfaces between the different components to facilitate integrated product elaboration like those provided by CMEMS, EMODnet, or SeaDataNet. These three infrastructures have signed a memorandum of understanding (MoU) to strengthen and sustain their collaboration and move toward common products delivered by more than one infrastructure. The success came from a step-by-step approach, focusing first on physics and extending gradually to biogeochemistry, with biology being a new target. Another factor of success was a win-win relation established with in situ observing system operators that can use the European services for extending the use of their observations. Improving FAIRness of the European infrastructures is an important objective, and major steps should be achieved in the ENVRI-FAIR H2020 project in the next 4 years with experience that will be shared within EuroGOOS through the DATAMEQ working group within the EuroSea H2020 project.
It is important to recognize that part of the failure to build fully interoperable data systems is that the use of a self-describing and highly effective data format such as NetCDF is beyond the technical reach of many science groups, even when they see the benefits of using the format.
Data Management for Biology and Ecosystems
In the biological and ecological disciplines, there is often a 5-year lag in data publication so that the majority of effort is still allocated to data archeology rather than uploading more recent observations (Muller-Karger et al., 2018). Much of this delay is inevitable as the processing and identification of samples may take some time due to processing techniques and the consultation of taxonomic experts. Where the oceanographic community started working together in the 1980s on data archeology, standardization, and routine surveys, such cooperation has a much shorter history in many biological disciplines. In areas where this has happened, such as fisheries, the data are often not publicly available due to political and/or economic sensitivities. Development of systems that allow automated collection and/or analysis of samples is in its infancy. An exception may be in the field of aquatic telemetry, where a significant effort has been made to automate data acquisition and publication (Hussey et al., 2015; Treasure et al., 2017; Hoenner et al., 2018).
Continuous biological data acquisition is at an early stage, leading to little standardization and movement toward automated processes. A notable exception is the progress made by the Integrated Marine Observing System (IMOS) in Australia on timely distribution of non-physical data5. The vast majority of biodiversity research or monitoring happens by discrete sampling or human observation from a distance, each with their heterogeneous sampling protocols and statistical consequences. Within marine biology data management, the OBIS has contributed toward vocabulary and metadata-level harmonization. For instance, the release of the Darwin Core Event Core in 2015 enables structured information on the sampling protocol and links a number of observations to a sampling event. This critical addition enables users to model population monitoring, simultaneous counting, and capture–recapture schemes—knowledge of which is essential to use such datasets for Essential Biodiversity Variable (EBV) products. For ingestion of data into OBIS, extensive semiautomated QC checks are employed, including completion of mandatory fields, correct typing and formatting of fields, and basic geographical checks, as well as taxonomical backbone mapping. The Darwin Core data standard means that there is little interest among biodiversity researchers in adopting formats and conventions from other disciplines, such as NetCDF and CF to primary occurrence data (e.g., presence/absence, abundance, and density measurements). To fully achieve interdisciplinary interoperability, current data catalog solutions will need additional interoperability layers, possibly based on those already mature in other domains, rather than building new services.
While some nations have considerable budgets for ocean observing and have significant resources to devote to data management, researchers in other nations have been left without professional data management support to aid them in publishing and curating their data (Parsons et al., 2011). Capacity development in data management and use is critical for a global reach and impact of ocean information. For instance, IOC/UNESCO’s IODE are building capacities around the world via training and online learning materials6. In most countries, most of the time, data management is poorly funded compared to data acquisition, and therefore, the data are often not processed at a level suitable for true interoperability that would allow the full data life cycle to be documented. Internationally comparable numbers to assess this quantitatively could not be found, but personal experience of the authors demonstrates that all oceanographic disciplines and nations lack the sustained resources needed to fully underpin global and regional ocean observing systems.
The Fair Principles in Practice
It has become clear that no single data access portal and application will ever fully satisfy the data access and requirements of all users. Rather, individual communities have very specific needs when it comes to how they access and use data, although cross-community data access is becoming more and more important. Rather than try to funnel users to an unfamiliar data portal, it is more valuable to focus on making data available through interoperable platforms. This can include direct access using protocols like the Data Access Protocol (DAP) Buck et al. (2019) or small, agile data portals that can potentially be easily and quickly built using the services provided by the data platform or be the result of thorough and consistent work over the years. A fundamental issue with data portals is their long-term maintenance, especially when they replicate the underlying data. Data portals need to link to or regularly synchronize with the underlying data to avoid decoupled copies of datasets becoming increasingly different over time.
Professional data management is an essential element of the FAIRness of an observing system and should be designed and properly funded as part of the cost of collecting the observations. New data types, especially from autonomous observing platforms, will need to have professional data management streams developed for them. At the same time, the new focus on EOVs means that many older observing platforms will need to have new data management workflows developed and applied to the legacy data. Data management needs to be structured to work across EOVs and from local to global scales (Figure 3). As the volume and diversity of data increase, so does the need for professional data managers. The broader oceanographic community need to follow the path of physical oceanographers in terms of establishing DACs and GDACs to curate observations, along with the necessary standardized data management processes (Figure 4). This cannot be done without providing adequate and sustainable funding for the technical data management, as well as for the necessary coordination needed to define and agree on the best processes to be used.
Since one of the key tasks of data managers is to preserve data for the long term, it is imperative that new data management repositories or data assembly centers have sustainable, long-term funding, with the possible exception of targeted data rescue efforts. A common rule of thumb for scientific data management funding is that at least 5 to 10% of the funding for a science project should be committed to managing the resulting data. While it is difficult to accurately price the global cost of either oceanographic programs or data management efforts, the total cost of a single research voyage, for instance, is considerably higher than the cost of hiring a data manager for a year. For instance, a study by Shepherd (2018) indicate annual gains in the order of a billion euro within the EU of ocean/marine information being accessible. As an example of a comparatively well-funded data management activity, Australia’s IMOS apportions 10% of its budget to building and maintaining the Australian Ocean Data Network (AODN), that is in addition to primary data management performed before the data reach the AODN (Lara-Lopez et al., 2016). Similarly, the TPOS 2020 2nd report recommend that 10% of observing effect should go toward data and information management7.
Figure 5 illustrates the principles of our suggestion, where data are delivered to the system from various networks or individual data providers through a data exchange backbone facilitated by appropriate tools and services to serve a wide data flow path from acquisition to user services.
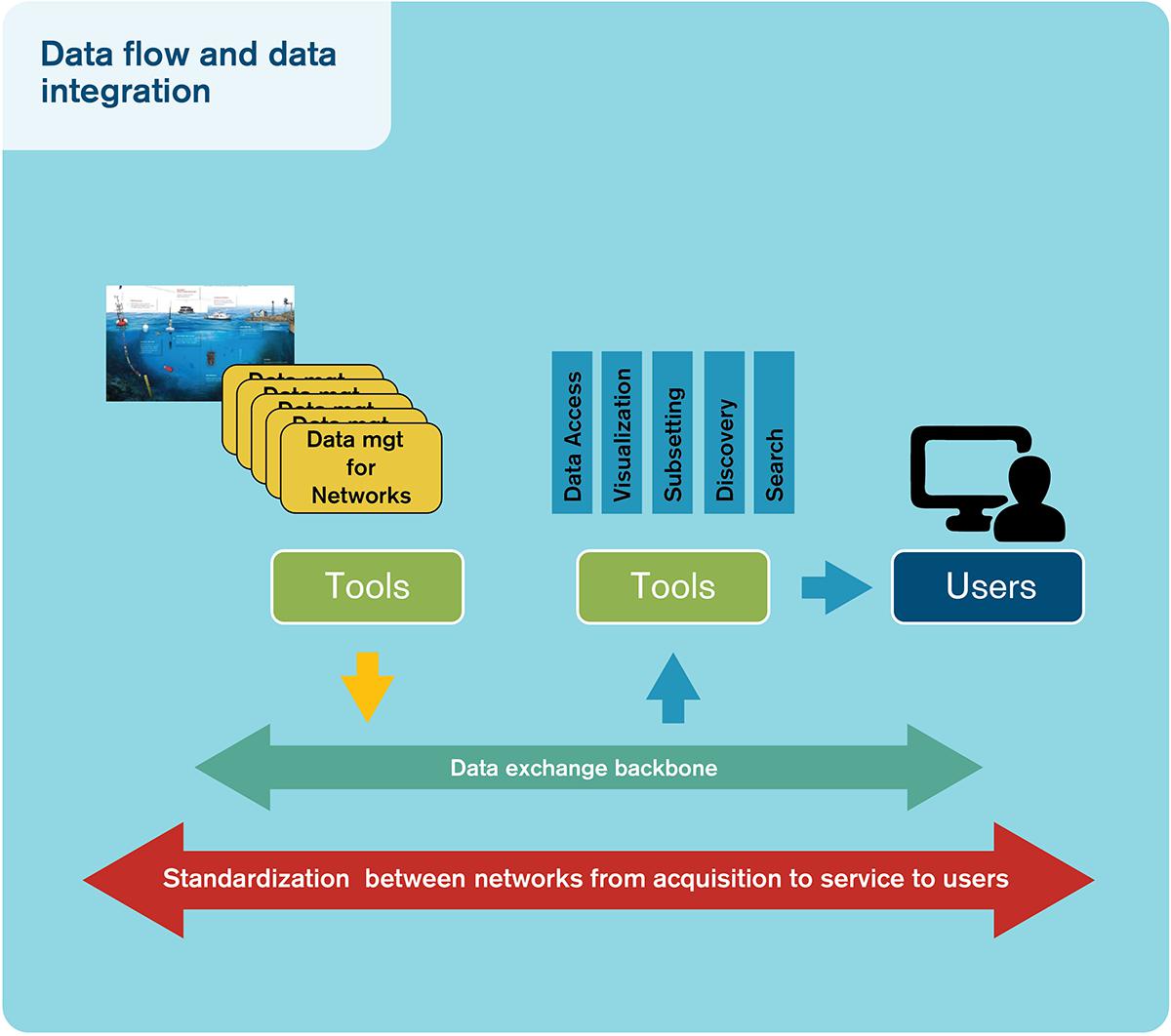
Figure 5. Schematic representation of data flow from observing units through a data exchange backbone to, ultimately, users.
The FAIR Principles and the FOO
In building any data system, a choice must be made between focusing on variables or on platforms/sampling events. It does not, in principle, matter from which platform observations of a variable originates, as long as the appropriate metadata are preserved, including estimation of the observation accuracy. This is in line with the focus on EOVs in the FOO. For RT data, it is essential to work with network operators to ensure complete data transmission and processing, appropriate QC steps, and labeling for practical/operational reasons. The integration of EOV data from different sensor, platforms, and networks require adequate characterization of accuracy and precision so a user can decide which data to use for a specific purpose. For delayed-mode data, a first level of delayed-mode processing, correcting for offset or drift, must be performed at the platform level, as it requires solid technical knowledge on the measurement procedure. A second step must focus again on the data in an EOV perspective, allowing for evaluation of cross-platform coherency. This assures the most complete coverage by combining EOV data from multiple platforms and assures the highest possible quality. An interoperable data system should facilitate comparing the data on one EOV coming from different platforms with other products, for instance, surface ocean temperature from satellites or from Argo floats.
The FAIR principles now enjoy broad recognition through the data community, and increasingly in the ocean observing community (e.g., the GOOS 2030 Strategy8 and the FOO). The FOO (Lindstrom et al., 2012) draw data and information systems, at the conceptual stage, in the discussion of maturity levels and in the discussion of processes of the Framework. However, it treats requirements, observations, data, and information as sequential, distinct parts of the framework and uniquely associates data and information with the outputs. In reality, those requirements will include data and information characteristics sitting alongside the EOVs. For example, an operational ocean prediction center that requires rapid access to physical data will have requirements in terms of quality, ease of access, and metadata. A climate assessment activity, on the other hand, will usually have a narrower requirement in terms of variables, but will emphasize quality, metadata, continuity, and comprehensive spatiotemporal coverage. The methods of data assembly and QC will be very important.
It is also clear that the requirements from stakeholders (providers and users) occur at both the input side and the output side according to the FOO framework. The observing systems themselves also have requirements; the effectiveness of data and information systems determines the impact of observing elements.
Several oceanographic organizations/projects are already embracing the FAIR principles alongside the consideration of EOVs and requirements, e.g., the Atlantic Blueprint vision document De Young et al. (2019); the latter conclude that “Following the FAIR principles must be a guiding principle for building and maintaining the Atlantic Ocean data management system.” This should apply to GOOS and the FOO more generally so that data and information are recognized as essential alongside EOVs, with the variables replaced by a set of static attributes. Requirement setting for data and information systems should be performed alongside requirement setting for EOVs. The requirements will emanate from three levels: (a) from the data users who are specifying needed accuracy and sampling on variables, (b) from operators of observing elements, and (c) from the value chain of ocean observing where delivering fit-for-purpose data and products is paramount.
The essential elements of data and information might include requirements to follow FAIR principles. RT data submission should be as close to real time as possible but delayed enough to assure quality is fit for purpose, and the communication system should be open and accessible. Furthermore, QA/QC should be integrated with the instrument/platform and automated as far as possible. The FOO should also assess access and assembly of digital object identifiers and the use of long-term archives in the requirement setting.
The FAIR principles should not need to evolve with technological change, but the essential requirements for data and information almost certainly will. Just as new observing technologies, like gliders or the proposed Surface Water Ocean Topography (SWOT) satellite mission, open up new possibilities for data and products at different resolutions and quality, changing IT capabilities and emerging general standards will provide opportunities for improved solutions for data and information systems, encouraging stricter requirements.
Testing FAIRness
Some oceanographic data types already meet the FAIR principles. These are mostly those data for which international agreements already exist on the observation methods, dataset structures, and an infrastructure to coordinate data aggregation and QC processes. For most in situ oceanographic data types, however, the FAIR principles have only been partially implemented. Datasets that have been published through general purpose or national data centers are typically somewhat findable and accessible, though their findability is limited if the data center’s metadata holdings are not connected to metadata aggregators or federations. Over the next decade, we anticipate general improvement in the implementation of the FAIR principles, leading to increased accessibility of data.
Many data systems are in the process of becoming FAIR; some even claim to be FAIR. However, it is important to utilize tools to measure FAIRness from a machine perspective. The GO FAIR office, active in the Netherlands, Germany, and France, has taken the initiative to develop metrics for testing FAIR readiness9. The metrics will assess the degree to which a data product meets the FAIR principles as accurately as possible. The aim is to give data holders the means to check where improvement is required (Wilkinson et al., 2018). For instance, SeaDataNet is a candidate implementation network, and the first FAIRness assessment has been made using the new metrics, providing a practical test10. SeaDataNet has made considerable progress in the last decade on metadata and data standardization on both syntax and semantics. Focus has been given to the development of data access services and Web services to find and access the data.
Integrated Data Services
Integrated data services can be important tools in the FAIR process, facilitating uptake by users. DACs and Thematic Acquisitions Centers (TACs) assemble data from different providers to provide integrated products and services. While a DAC is linked to a network and the DAC process data from acquisition to data delivery, a TAC is a thematic center that aggregates data from other data centers to provide a service for a specific type of users.
The role of TACs is to collect, process, and QC upstream satellite and in situ data. The satellite TAC’s main functions are to work on homogenization and intercalibration of data from multiple missions (so called L2P processing) and the development of higher-level data products. In situ TACs deal with the collection of data from a wide range of networks by the development of homogenized QC and validation procedures as well as high-quality data products. Such an approach can be used at global- and regional-level generating products in near real time as well as reprocessed data.
Such services have been developed in different continents, and collaboration exists between these initiatives through the Global Ocean Data Assimilation Experiment (GODAE) Ocean View for Operational services, RDA, and IODE. Past decades have seen the development of TACs [e.g., CMEMS, SeaDataNet, International Council for the Exploration of the Sea (ICES), or EMODnet] that developed cross-platform services targeted to a wider range of users. Other thematic centers have developed specific data products and services for specific EOVs [e.g., SOCAT for surface ocean pCO2 data, Global Ocean Data Analysis Project for Carbon (GLODAP) for interior ocean biogeochemistry (see Box 6), GEOTRACES for trace element chemistry, or OBIS for biodiversity and biogeography]. Setting up GDACs to provide an interoperable data platform for data access will allow for services on an EOV basis or a network basis. In turn, this will facilitate a rigorous and effective quality assessment service.
BOX 6. SOCAT and GLODAP.
The SOCAT and GLODAP are two complementary carbon-related data products. SOCAT is a QCed global ocean surface carbon dioxide (CO2) dataset (Pfeil et al., 2013), and GLODAP is a QCed, internally consistent, global interior ocean data product on carbon-relevant variables.
SOCAT supports the FAIR data principles by leveraging current data standards, archiving the data and providing DOIs as well as providing interoperable Web services to access the data. In addition, SOCAT has implemented a semiautomated data ingestion dashboard that allows the SOCAT data providers to submit data into the SOCAT QC system. Functionally, this means that scientists can work in their native data formats, and the ingestion system will manage the more technical tasks of creating standards-compliant files, associating the proper metadata with the files, providing submission to national data centers, and, finally, making the data available through high-level Web services. This automation of services has allowed SOCAT to evolve from a release every 4 years to annual releases. These annual releases serve to inform global products such as the Global Carbon Project’s Annual Carbon Budget (http://www.globalcarbonproject.org/carbonbudget/). The automated system used by SOCAT demonstrates a method to efficiently manage the higher volumes of data expected with the future of new ocean observing efforts.
The GLODAP (https://www.glodap.info/) product (Olsen et al., 2016) has evolved since its first release in 2004 (Key et al., 2004), with improved routines to retrieve data, for primary and secondary QC, and for archiving and viewing results from these processes. However, a large fraction of the work to produce the product is still manual, which places heavy demands on the community. The GLODAP community has begun to look at building SOCAT-like processes to handle future GLODAP releases in a more effective and efficient way.
Data and Information Products
Data products are also useful ways of avoiding duplicate versions of the same dataset ingested in the analysis. Satellite data managers regularly produce higher-level data products that include quality flags and suggested editing features to fully applied QC and regular geolocated gridded data. NASA, NOAA, EUMETSAT, ESA, and other agencies use self-describing file formats [e.g., NetCDF, Hierarchical Data Format (HDF)] and CF metadata conventions. This makes indexing the data easier for the data centers’ databases and makes sure that the quality flags are easily paired with their science variable for mid-level products so users can QC the data for their specific research needs. It lends itself to producing higher-level products, usually gridded, for data assimilation into models.
Web services are APIs, typically hosted by the data center. The Physical Ocean Distributed Active Archive Center (PO.DAAC) provides Web services to search on datasets and granule/file metadata and data. From there, the user can discover what files/granules fit their need, either by parameter, region, or time range. The user can then retrieve the entire dataset or subset it. This allows well-informed users to easily access data with their code as well as having smaller specialized data centers supplementing their community with satellite data without the need to archive themselves. As the data follow widely adopted conventions, specialized data readers are not required for the user to analyze the data.
Derived and model data outputs rely on knowing the quality of the data before assimilation by proper metadata. This provides traceability of the data assimilation and easier ingestion because the linkages between data and any quality flags are provided.
Data Discovery for Non-EOVs
Full data interoperability is the appropriate goal for data types that already have agreed standards and processes for collecting, documenting, and QCing the data. However, new variables will be observed and new observing methods are continuously being developed. It will always take time for new observation types to be validated and replicated. There are also historical datasets that gain new perceived value, making them “worth” aggregating in a standardized format (Griffin, 2015). It is important to provide data discovery tools, allowing researchers and data managers to find those datasets not yet entrained in sophisticated data management systems. For those datasets, it may not be possible to achieve all of the FAIR principles, but at least three of them—findability, accessibility, and reusability—can be applied to almost all ocean data.
To date, these non-standardized datasets are principally housed in nation- and discipline-specific data centers, which publish discovery metadata through in-house catalogs and through metadata aggregators. In the past, a common approach has been to ensure that a single master copy of data is preserved, but the accompanying metadata records have been translated among differing standards and republished through aggregating metadata catalogs to increase the visibility of the dataset. Combined with a lack of persistent identifiers on records, this has resulted in a legacy of partially duplicated metadata records across multiple catalogs, with limited capacity to keep those duplicates updated. These partially duplicated records provide a considerable challenge to data discovery, and we are not aware of any mature deduplication algorithms being applied in federated metadata discovery tools.
Increasingly, oceanographic metadata managers are investigating options to develop federated metadata search tools. Such tools will allow simultaneous searching of multiple metadata catalogs, thus considerably improving the findability and accessibility of non-standardized datasets while reducing opportunities for semi-duplicated metadata records to proliferate.
Federation of metadata search requires a brokering mechanism, which, in turn, requires well-understood crosswalks between the common metadata standards, vocabularies, and profiles in use by a community. This is done by having data that are in self-describing file formats (e.g., NetCDF or HDF) or well and regularly, easy-to-read file formats (e.g., ASCII). Following metadata standards is also a requirement as the brokering mechanisms can recognize the metadata and properly handle the data. The newly launched Google Dataset Search11 (currently as early release while gathering requirements for future iterations) relies on well-formatted metadata and tags following the schema.org definitions, where they see discipline-specific requirements on search and discovery as the responsibility of the discipline to implement. Smaller, more tightly focused federations will pose fewer challenges in terms of brokering these differences and will, hence, likely result in stronger search algorithms. In contrast, global federations will trade off searching power for larger holdings and greater economies of scale in terms of tool development. It is likely that the next decade will see a proliferation of metadata federations as communities balance the relative costs and benefits of small and large federations.
Standards and Best Practices
To achieve optimal use of research data and methods, we recognize the need to follow common community standards and best practices for data systems outlined by recognized international bodies such as IODE and GOOS. Implementing these practices requires that well-defined workflows are followed and that a sustained infrastructure is in place where the chain from data delivery by observing systems, initial QC/QA and feedback from the data center to the primary data producers, integration in data products, and arching in recognized data repositories are supported (Pearlman et al., 2019). Specialist support from data centers is a relatively small cost with potentially large positive gains in terms of timely data submission and quality. Careful metadata and quality flags are important attributes for ocean data, including information on the level of QC.
Best practices and standards for data management are increasingly being implemented by many observing networks and projects on national or continental scales. Successful cooperation among different operators is important for developing and promoting standards and best practices that facilitate the interoperability of systems.
Credit Through Publication: Data Citation, Persistent Identifiers, Etc.
Credit for developing new datasets, through attribution and citation, has several challenges as the supporting infrastructure needed for this is not fully developed. Journals increasingly require data that support an article to be in a FAIR-aligned repository that is open, uses persistent identifiers, and supports data citation (see the COPDESS Web site5).
We recognize that a significant fraction of the ocean data is collected “in research mode,” i.e., from project-based science with limited-term funding agreements; in science, output is largely measured in citable publications rather than in published datasets (Mons et al., 2011). It is imperative that scientists are incentivized to make their data publicly available. Options include using data citation tools, such as DOIs, that make it easier to credit the originating scientist and publishing data reports as peer-reviewed publications [e.g., Earth System Science Data (ESSD)]. Alternatively, a metadata record can contain fields identifying the data provider, giving recognition. To remedy this issue, the Coalition for Publishing Data in Earth and Space Sciences (COPDESS)12 is working with the scientific data facilities and scholarly publishers “to help translate the aspirations of open, available, and useful data from policy into practice.”
Other efforts helping to automate data citations include a new task force by ORCID to work with repositories in capturing data creator ORCIDs and the necessary linking, and the MakeDataCount project13 defining consistent ways to count data usage and providing tools to repositories to consistently show these metrics.
Attribution and credit for data starts with the data repository and the registration of a persistent identifier associated with that data. Included in this metadata are the names and ORCIDs of the data creators. Through the registration process of the persistent identifier and services available through ORCID, a new dataset is made known in the research data infrastructure and linked with the repository and publication. For instance, publishers need to implement the full capability of the CrossRef Citation schema14 to include the portion about relationships. This identifies, in a machine-readable way, which citations are data and their association with research papers, which is particularly important for persistent identifiers that are not a DOI. Data facilities further need to capture the name of the data creator(s) along with their ORCID and provide that information when registering the persistent identifier for the dataset. This should also be provided to ORCID through the provided API that is created for repositories that have not implemented a globally unique persistent identifier. Similarly, researchers need to identify the best possible repository for their data, preferably one that is familiar with that type of data, providing curation services and is FAIR aligned.
Persistent Identifiers for Data and/or products (PID) is another important piece of metadata for traceability of processing (proper identification and versioning) on one side and traceability of use by end users and organizing feedback to providers. Such PIDs can be attached to the platform such as a WMO number for Argo, drifters, meteorological moorings, ICES code for vessels, etc. DOIs can also be attached to a version of a dataset, e.g., DOI on data from a research cruise, on one glider mission, on versions of aggregated products like for SOCAT, GLODAP, or the Coriolis Ocean database for ReAnalysis (CORA), or on a periodic snapshot of a GDAC. Different strategies have been developed in the past 10 years, and the PID technology has evolved to be able to manage network data that evolve continuously.
As datasets are aggregated into data products, it is not yet obvious how to give appropriate credit to all of the originating scientists who generated the data, with potentially thousands of scientists contributing to a single research article. Work is needed to develop ways to efficiently and effectively give credit to all those who have contributed. Similarly, data centers that curated datasets integrated into data products need to be efficiently credited as contributors, perhaps by ensuring that the full lineage of a metadata record is maintained through all levels of aggregation and federation, maybe by exploring the blockchain concept in marine data lineage. Certification of repositories (e.g., CoreTrustSeal15) plays a key role; there is strong alignment between the tenets of FAIR and the elements of certifications to help researchers and publishers make an informed decision on determining which repositories meet criteria and are FAIR aligned. Recognizing that data to support research is valuable as stand-alone products enables the community to work with institutions to enhance promotion and tenure criteria to publish data and data products. The more we cite data from repositories that support data citation, the more we can link that data back to cruises and other research objects from the same research effort and show a more complete view and demonstrate value.
Additionally, unambiguous citation of the underlying data used in the climate assessment is becoming a requirement for transparency. An example of such a policy emerged in the United States under Barack Obama’s presidency with the open government initiative16 and open data policies on European Commission projects17.
Implementing FAIR
The Enabling FAIR Data project, which promotes mandatory exchanges across all journals to provide data creators the attribution and credit for the effort, building upon COPDESS through the commitment statement18 and author guidelines19, addresses this issue:
“Publication of scholarly articles in the Earth, Space, and Environmental science community is conditional upon the concurrent availability of the data underpinning the research finding, with only a few, standard, widely adopted exceptions, such as around privacy for human subjects or to protect heritage field samples. These data should, to the greatest extent possible, be shared, open, and stored in community-approved FAIR-aligned repositories. Leading repositories provide additional quality checks around domain data and data services and facilitate discovery and reuse of data and other research outputs.”
The recently started ENVRI-FAIR project aims to implement FAIR across the European research infrastructures, which include Argo, the European Multidisciplinary Seafloor and water column Observatory (EMSO), and the Integrated Carbon Observation System (ICOS). SeaDataNet is facilitating horizontal synergy between these research infrastructures (RIs) in the marine subdomain, but also toward RIs in the atmospheric, biological, and solid earth subdomains. The time of writing the project was at the stage of assessing FAIRness of the research infrastructures with a total project duration of 4 years.
Data Services of the Future
Generating data that follow FAIR principles can be expensive upfront but generally pays big dividends in the long term. It is easier for the data producer to make data products according to their own needs, but that makes reuse much harder for other users. Those data products may not be formatted according to established standards, making it harder for users to read and access them. The quality of the data is not as obvious if it lacks quality flags or provenance information. This creates more work for the user as they try to understand the best way to read and use the data for their needs. When the data producer creates data that follow metadata standards and are well formatted, they facilitate access and use of the data. This implies some overhead as making the data products comply with standards is not a trivial task. It should, however, be noted that even if the workload for the data product producer is not trivial, we believe that it is a worthwhile endeavor when considering the amount of time that is saved for the users. Well-formatted data can almost act as their own service as there are many tools, software, and protocols that recognize NetCDF and HDF data that follow CF conventions, so less effort is needed by the user for finding, reading, and interpreting the data. Data that follow the FAIR principles also make it easier to attribute credit to the creators.
Information and Communication Technology (ICT) and VRE
One of the grand challenges of eScience is providing machine-readable data as the main substrate for knowledge discovery and to assure that these processes run smoothly and are sustainably.
At all levels of the value-adding chain (observation providers, products developer, and downstream services), we are facing an exponential increase in the volume of data acquired by different means of earth observing (in situ or satellites) or products made available to the community (in situ, satellite, and model outputs). Progress in the development of Internet and fast telecommunications services; big data capabilities, including machine learning and artificial intelligence; and standardized Web-services have and will continue to revolutionize the services available to users. VREs allowing users to remotely conduct processing not possible on desktop systems and to retrieve only the outputs (data/products) that are needed for their applications are being facilitated through cloud systems. These are providing important storage space and computing facilities through high-performance computers and are under development in most regions.
The addition of cloud and VRE as mainstream tools introduces opportunities and challenges. As a community, we must now consider “cloud-to-cloud” interoperability. This will build on standards and methods already in place and evolving for service-to-service interoperability. We must now consider how to ensure that data and application code (e.g., model code) developed on different platforms can be effectively integrated (e.g., Zacharewicz et al., 2017;Chang and Reinsch, 2018). Interoperability between the different elements of the Earth data management system is becoming a necessity driven by research and operational user requirements, and this will continue to evolve as cloud and VREs become more prominent and numerous. While big data and cloud tools will change the way services to users evolve in coming years, they will require sustainability in their development and in the funding scheme by member states.
Web Services and Smart Sensors
The acceleration of data production, diversity, and RT availability will increasingly demand machine-based processing of data flows. Data processing will require that machines (algorithms) be provided with properly structured metadata and data to discover and have access to services for catalogs, observations, and alerts on the Web. These Web services should be provided via uniform and compatible encodings, using community-adopted standards. Availability of open standards, supported by open-source software developers, and the advent of smart sensors, e.g., equipped with standard metadata on-board their communication interface and capable of data preprocessing, will support the FAIR principles for real-time data. Examples of such standards are the OGC sensor Web enablement (SWE)20 with the community-specific marine SWE profile21 or the use of services such as data streaming22. While still on the path to maturity and with limited uptake in the marine community, these technologies should soon enable the transition to real-time machine-based processing and production of data products.
Summary and Recommendations
We have pointed out some challenges to support sustained and efficient ocean data management and pointed to the FAIR principles to guide future directions, and have shown some examples where the FAIR principles have started to demonstrate added value. Here, we try so summarize some of the main recommendations moving forward for the next decade, without going into technical details.
To progress toward increasing FAIRness of ocean data, there is a need to introduce the why and how of FAIR dataic to early career scientists in marine science so that adoption of these principles and this approach to data management becomes second nature and a natural part of doing ocean observations. We realize that future graduate students might not even go to sea but receive the data they need from autonomous platforms delivered through various integrated data services (Vance et al., 2019).
Hodson (2018) outline several recommendations to foster the FAIR principles in a recent EU report. For instance, they state that research facilities should be incentivized to follow FAIR principles by reviewing the FAIRness of data management processes. They also suggest that FAIR data practices should be included in the assessment of research contributions and career progression and that infrastructure and services that enable FAIR data must also be recognized and rewarded accordingly. A FAIR data policy is essential to enhance the accessibility of data, foster reuse of existing data, and turn observation data into information needed by end users. The FAIR principles should be embraced in assessing the technical readiness level for data and information. They should be an integral part of assessing requirements in the framework for ocean observing, and the principles should be backed up by appropriate investment in data and information management. Both pilot and mature systems should comply with the FAIR principles. Although there is great heterogeneity among oceanographic disciplines in terms of their progress toward the FAIR principles, considerable progress over the next decade can, and should, be made.
When data are not FAIR, access is limited to researchers in tight-knit networks. When data are FAIR, access is opened to people who are working outside their main discipline and outside the academy. The corollary of this is that there is an overhead in making data FAIR, which may be beyond the capacity of data creators working outside academia. It is therefore crucial that those organizations with the resources to develop data sharing tools do so in ways that are accessible to those outside their institutions.
Among the data lifetime models available, there are certain commonalities in the sense that they describe a series of steps in standardizing the handling of data from discovery to publication. These steps include (a) data acquisition, processing, and QA; (b) data description and representation; (c) data cataloging and dissemination; and d) repository services and preservation (Crowston and Qin, 2011). Modern data management infrastructures are needed to support the ocean observing system so that all these activities along the data flow pipeline are more automated and fault tolerant. Progressively, the systems should advance toward interoperability; this serves both the routine data exchanges within and between the observation networks, as well as user-friendly tools for data/products discovery viewing and access.
It is worth considering that the large majority of oceanographic ocean observations are funded by public funds; there are almost always requirements from the finding agency to make the data public, realizing that the exact requirements and the level to which they are imposed vary greatly from country to country. Submitting ocean data to a repository do not automatically mean that the data follow the FAIR principle. An important aspect is the latency of the process and the level of FAIRness of the data portal to which the data have been submitted. A FAIR data policy should be a priority for all marine datasets and should be supported by nations and stakeholders.
In chapter 2, we listed some challenges; here, we suggest actions to mitigate those.
Large Diversity
A high level of interoperability is required to harmonize these dispersed data systems to allow for easy access by users. Improving metadata services will be key at many steps in the data life cycle. For non-standardized datasets, better metadata search tools will be crucial. Leveraging the research infrastructures data systems to provide infrastructure for data of all EOVs at the same level of accessibility should be a priority. Data centers and scientific funding agencies should make international coordination an explicit part of data management staff’s job description in order to achieve these outcomes.
Multitude of Disparate Data Management Structures
These existing systems need to be used as the ‘building blocks’ for an interoperable framework of data management systems. As an example on how this can work is the EMODnet effort in Europe, improving free, timely, and unrestricted access to interoperable European marine data, building on existing database, and open sharing infrastructures such as SeaDataNet, CMEMS, European Ocean Biogeographic Information System (EurOBIS), ICES, and EGDI (Shepherd, 2018). Similarly, open source data platform tools, such as ERDDAP, can reduce the burden of providing interoperable data services for users and improve data uptake by consumers.
Increased Volume of Data
As a consequence of increased data volume, it has become clear that it is beneficial to spread the data processing workload across many institutes and that harmonization of data processing and distribution is a priority. The data management systems must also be able to grow with the increasing volume of data expected in the future, leveraging on the IODE network of National Oceanographic Data Centres (NODCs), Associate Data Units (ADUs), online information sources, repositories, and learning objects. Based on robust FAIR data systems to deliver observation data and products, service development will drastically change in nature with the development of Big Data infrastructure and machine learning techniques that should foster reusability of existing observation and products exponentially.
New Sensors Creating New Formats
For all sensors, more complete metadata that are captured early in the data life cycle will improve the reusability of observations for many purposes. In communities that struggle to meet FAIR principles, leveraging existing tools can help those communities significantly increase their level of data interoperability with a minimum of resources. In addition, working more closely with sensor manufacturers to provide metadata directly from the sensors in community-accepted standards and conventions will make it much easier to properly document data further downstream in the data life cycle.
Widely Used Formats Not Universally Applicable
IT solutions need to meet the needs of particular science communities while at the same time facilitating the universal interoperability we desire. Both open source tools and services with community governance and commercial solutions are potentially possible.
Gap Between Data-Producing Scientists and Downstream Users of the Data
Modern data management architecture and infrastructures are needed so that all activities along the data pipeline are understood and efficient and progressively advanced toward interoperability. This serves the routine data exchanges within and between the observation networks, as well as user-centered tools for finding, accessing, analyzing, and (re)using data/products. Community standards for metadata, data formats, communication protocols, and data server software infrastructure are the foundation for interoperability. A key component is close monitoring and QC of data streams with communication between the observing system operators and data managers. Data centers need to give high priority to the use of modern information and communication technologies.
Development of Common Protocols Takes Time
These efforts, along with best-practice elaboration, should be organized and properly funded to develop new standards and enhance existing ones to meet the needs of the community in a reasonable timeframe.
Best Practices Poorly Defined
There is a growing need to identify the most efficient and systematic strategy for processing data all the way from the initial planning of data collection to the availability of the data products and their dissemination, i.e., development of a data management plan that evolves throughout the life cycle of the data. Data management for large, dispersed datasets benefits significantly from a well-established and standardized approach starting even before the data are collected. The FAIRness of the data system will rely on continuously updated standards and best practices for metadata and data harmonization as well as QA/QC common procedures; such activity should be properly funded at national, continent, and international level. This also includes support, training, and outreach to the teams that will develop data systems for networks or thematic services. Data can be more easily found and utilized if they are properly managed, follow best practices, are described with exhaustive and structured meta information, and are assigned persistent identifiers. These goals can be achieved by following internationally agreed standards and protocols for file formats, “content” (vocabularies/conventions), and “packaging” (metadata standards) and ensuring the data are preserved and curated in a sustained repository. Investing in the development and maintenance of freely available software utilities will pay dividends by assisting data producers in publishing data that meet community standards. Support for the implementation of standards and best practices by the research infrastructures is key to enhance interoperability and reusability of existing data and to avoid duplication of efforts.
Taking these steps will not only allow the scientist or developer to download the data and apply traditional data analysis techniques but will also enable the use of modern tools to transform, manipulate, visualize, and utilize the data in novel ways. These tools and platforms help ensure that true data interoperability is achieved, enabling interdisciplinary studies with a range of data from different domains. In addition, they promote reusability by making sure data can be understood by those who did not produce the data. These capacities are vital for international, interdisciplinary ocean observing systems.
Author Contributions
TT, SP, JH, KO’B, and PB helped to conceive the study, coordinated the author contributions, wrote and edited the manuscript, and contributed to tables and figures. TdB, JB, EB, TC, KC, SD, AG, HG, VH, DK, JM, AN, BP, PP, AVdP, ER, DeS, AS, NS, DiS, TS, SS, MT, PT, ST, TV, MW, LW, and ZZ contributed to the manuscript ideas and text.
Funding
We thank the funding agencies and the data management projects that have made this work possible through dedicated funding for the data management activities and improvements. TT and JB acknowledge support from the EU Horizon 2020 project AtlantOS (grant agreement 633211). JM acknowledges support from the Integrated Oceanography and Multiple Uses of the Continental Shelf and the Adjacent Ocean Integrated Center of Oceanography (INCT-Mar COI, CNPq, Proc. 565062/2010-7). DS acknowledges support from the H2020 project SeaDataCloud (grant agreement 730960). SP acknowledges support from the EU Horizon 2020 project ENVRIplus (grant agreement 654182). AN acknowledges support from the EMODnet Physics (grant number EASME/EMFF/2016/1.3.1.2-Lot3/SI2.749411). HG acknowledges funding from the EU H2020 Ocean Data Interoperability Platform (ODIP) project (Grant No: 654310). JH acknowledges that funding came from the National Aeronautics and Space Agency as managed by the California Institute of Technology under task number 80NM0018F0848. AVdP acknowledges support from Belspo in the framework the EU Lifewatch ERIC (grant agreement FR/36/AN3). KO’B acknowledges that his publication is partially funded by the Joint Institute for the Study of the Atmosphere and Ocean (JISAO) under NOAA Cooperative Agreement NA15OAR4320063, Contribution No. 2018-0175.
Conflict of Interest Statement
AN was employed by the company ETT.
The remaining authors declare that the research was conducted in the absence of any commercial or financial relationships that could be construed as a potential conflict of interest.
The reviewer J-BC declares an ongoing collaboration on a project with the authors AN, AG, and DS as a contribution to the OceanObs collaboration, a decadal conference series on ocean observation. The peer review was handled under the close supervision of the Chief Editors to ensure an objective process.
Footnotes
- ^ See, for instance, https://www.wfmc.org/
- ^ https://www.nodc.noaa.gov/data/formats/netcdf
- ^ https://www.force11.org/group/fairgroup/fairprinciples
- ^ https://www.ncei.noaa.gov/news/noaa-expands-big-data-access
- ^ https://portal.aodn.org.au/
- ^ For examble, Ocean Teacher, https://classroom.oceanteacher.org/
- ^ http://tpos2020.org/project-reports/second-report/
- ^ http://www.goosocean.org/index.php?option=com_oe&task=viewDocumentRecord&docID=24590
- ^ https://www.go-fair.org/technology/go-fair-metrics/
- ^ https://www.seadatanet.org/Data-Access
- ^ https://toolbox.google.com/datasetsearch
- ^ COPDESS.org
- ^ https://makedatacount.org
- ^ https://support.crossref.org/hc/en-us/sections/202832803-Crossref-schema
- ^ https://www.coretrustseal.org/
- ^ https://obamawhitehouse.archives.gov/open
- ^ http://ec.europa.eu/research/participants/docs/h2020-funding-guide/cross-cutting-issues/open-access-dissemination_en.htm
- ^ http://www.copdess.org/enabling-fair-data-project/commitment-to-enabling-fair-data-in-the-earth-space-and-environmental-sciences/
- ^ https://zenodo.org/record/1447108#.XUAR9EFS-Uk
- ^ http://www.opengeospatial.org/ogc/markets-technologies/swe
- ^ https://odip.github.io/MarineProfilesForSWE/
- ^ https://aws.amazon.com/streaming-data/
References
Blower, J., Hankin, S. C., Keeley, R., Pouliquen, S., Beaujardire, J. D. L., Berghe, E. V., et al. (2010). “Ocean data dissemination: new challenges for data integration”,” in Proceedings of OceanObs’09: Sustained Ocean Observations and Information for Society, eds J. Hall, D. E. Harrison, and D. Stammer (Paris: ESA Publication).
Buck, J. J. H., Bainbridge, S. J., Burger, E., Kraberg, A., Casari, M., Casey, K. S., et al. (2019). Ocean data product integration through innovation—the next level of data interoperability. Front. Mar. Sci. 6:32. doi: 10.3389/fmars.2019.00032
Chang, W. L., and Reinsch, R. (2018). DRAFT NIST Big Data Interoperability FrameworkAdoption and modernization.version 3. Gaithersburg, MD: National Institute of Standards and Technology
Crowston, K., and Qin, J. (2011). “A capability maturity model for scientific data management: evidence from the literature,” in American Society for Information Science and Technology Annual Meeting, (Hoboken: Wiley).
de La Beaujardière, J., Beegle-Krause, C., Bermudez, L., Hankin, S., Hazard, L., Howlett, E., et al. (2010). “Ocean and coastal data management,” in Proceedings of OceanObs’09: Sustained Ocean Observations and Information for Society, eds J. Hall, D. E. Harrison, and D. Stammer (Paris: ESA Publication).
De Young, B., Visbeck, M., De Araujo Filho, M. C., Baringer, M. O. N., Black, C.A., Buch, E., et al. (2019). An integrated all-atlantic ocean observing system in 2030. Front. Mar. Sci. 6:428. doi: 10.3389/fmars.2019.00428
Hankin, S., Bermudez, L., Bowler, J. D., Blumenthal, B., Casey, K. S., Fornwall, M., et al. (2010). “Data management for the ocean sciences—perspectives for the next decade,”,” in Procedings of OceanObs’09: Sustained Ocean Observations and Information for Society, Vol. 1, eds J. Hall, D. E. Harrison, and D. Stammer (Venice: ESA Publication).
Hodson, J. (2018). FAIR Data Action Plan. Interim recommendations and actions from the European Commission Expert Group on FAIR data. Zenodo doi: 10.5281/zenodo.1285290
Hoenner, X., Huveneers, C., Steckenreuter, A., Simpfendorfer, C., Tattersall, K., Jaine, F., et al. (2018). Australia’s continental-scale acoustic tracking database and its automated quality control process. Sci. Data 5:170206. doi: 10.1038/sdata.2017.206
Hussey, N. E., Kessel, S. T., Aarestrup, K., Cooke, S. J., Cowley, P. D., Fisk, A. T., et al. (2015). Aquatic animal telemetry: a panoramic window into the underwater world. Science 348, 1255642. doi: 10.1126/science.1255642
Keeley, R., Woodruff, S., Pouliquen, S., Conkright-Gregg, M., and Reed, G. (2010). “The development of the data system and growth in data sharing,” in Proceedings of OceanObs’09: Sustained Ocean Observations and Information for Society, eds J. Hall, D. E. Harrison, and D. Stammer (Paris: ESA Publication).
Key, R. M., Kozyr, A., Sabine, C. L., Lee, K., Wanninkhof, R., Bullister, J. L., et al. (2004). ). A global ocean carbon climatology: results from global data analysis project (GLODAP). Global Biogeochem. Cycles 18:GB4031.
Lara-Lopez, A., Moltmann, T., and Proctor, R. (2016). Australia’s Integrated marine observing system (imos): data impacts and lessons learned. Mar. Technol. Soc. J. 50, 22–33. doi: 10.4031/MTSJ.50.3.1
Lindstrom, E., Gunn, J., Fischer, A., Mccurdy, A., Glover, L., Alverson, K., et al. (2012). A Framework for Ocean observing. by the Task Team for an Integrated Framework for Sustained Ocean Observing. Paris: UNESCO.
Meredith, M. P., Schofield, O., Newman, L., Urban, E., and Sparrow, M. (2013). The vision for a southern ocean observing system. Curr. Opin. Environ. Sustain. 5, 306–313. doi: 10.1016/j.cosust.2013.03.002
Miguez, B. M., Novellino, A., Vinci, M., Claus, S., Calewaert, J., Vallius, H., et al. (2019). The european marine observation and data network (emodnet): visions and roles of the gateway to marine data in europe. Front. Mar. Sci. 6:313.
Mons, B., Van Haagen, H., Chichester, C., Hoen, P.-B. T., Den Dunnen, J. T., Van Ommen, G., et al. (2011). The value of data. Nat. Genet. 43:281. doi: 10.1038/ng0411-281
Muller-Karger, F. E., Miloslavich, P., Bax, N. J., Simmons, S., Costello, M. J., Sousa Pinto, I., et al. (2018). Advancing marine biological observations and data requirements of the complementary essential ocean variables (EOVs) and essential biodiversity variables (EBVs) frameworks. Front. Mar. Sci. 5:211. doi: 10.3389/fmars.2018.00211
Olsen, A., Key, R. M., Van Heuven, S., Lauvset, S. K., Velo, A., Lin, X., et al. (2016). The Global ocean data analysis project version 2 (GLODAPv2)—an internally consistent data product for the world ocean. Earth Syst. Sci. Data 8, 297–323. doi: 10.5194/essd-8-297-2016
Parsons, M. A., de Bruin, T., Tomlinson, S., Campbell, H., Godøy, Ø, Leclert, J., et al. (2011). The state of polar data—the IPY experience. Edmonton: C. Press.
Pearlman, J., Bushnell, M., Coppola, L., Karstensen, J., Buttigieg, P. L., Pearlman, F., et al. (2019). Evolving and sustaining ocean best practices and standards for the next decade. Front. Mar. Sci. 6:277. doi: 10.3389/fmars.2019.00277
Pfeil, B., Olsen, A., Bakker, D. C. E., Hankin, S., Koyuk, H., Kozyr, A., et al. (2013). A uniform, quality controlled Surface Ocean CO2 Atlas (SOCAT). Earth Syst. Sci. Data 5, 125–143. doi: 10.5194/essd-5-125-2013
Pinardi, N. (2019). Marine monitoring to services: the IOC of UNESCO and WMO experience. Front. Mar. Sci. doi: 10.3389/fmars.2019.00410
Pouliquen, S., Hankin, S., Keeley, R., Blower, J., Donlon, C., Kozyr, A., et al. (2010). “).“The development of the data system and growth in data sharing”,” in OceanObs’09: Sustained Ocean Observations and Information for Society, eds J. Hall, D. E. Harrison, and D. Stammer (Paris: ESA Publication).
Roemmich, D. (2019). On the future of Argo: a global, full-depth, multi-disciplinary array. Front. Mar. Sci. doi: 10.3389/fmars.2019.00439
Shepherd, I. (2018). European efforts to make marine data more accessible. Ethics Sci. Environ. Polit. 18, 75–81. doi: 10.3354/esep00181
Taylor, I. J., Deelman, E., Gannon, D. B., and Shields, M. (2006). Workflows for e-Science: Scientific Workflows for Grids. Berlin: Springer-Verlag.
Treasure, A. M., Roquet, F., Ansorge, I. J., Bester, M. N., Boehme, L., Bornemann, H., et al. (2017). Marine mammals exploring the oceans pole to pole: a review of the MEOP consortium. Oceanography 30, 132–138. doi: 10.5670/oceanog.2017.234
Vance, T. C., Wengren, M., Burger, E., Hernandez, D., Kearns, T., Medina-Lopez, E., et al. (2019). From the oceans to the cloud: opportunities and challenges for data, models, computation and workflows. Front. Mar. Sci. 6:211. doi: 10.3389/fmars.2019.00211
Wilkinson, M. D., Dumontier, M., Aalbersberg, I. J., Appleton, G., Axton, M., Baak, A., et al. (2016). The FAIR guiding principles for scientific data management and stewardship. Sci. Data 3:160018. doi: 10.1038/sdata.2016.18
Wilkinson, M. D., Sansone, S.-A., Schultes, E., Doorn, P., Bonino da Silva Santos, L. O., and Dumontier, M. (2018). A design framework and exemplar metrics for FAIRness. Sci. Data 5:180118. doi: 10.1038/sdata.2018.118
Zacharewicz, G., Diallo, S., Ducq, Y., Agostinho, C., Jardim-Goncalves, R., Bazoun, H., et al. (2017). Model-based approaches for interoperability of next generation enterprise information systems: state of the art and future challenges. Inf. Syst. E-Bus. Manag. 15, 229–256. doi: 10.1007/s10257-016-0317-8
Keywords: FAIR, ocean, data management, data services, ocean observing, standardization, interoperability
Citation: Tanhua T, Pouliquen S, Hausman J, O’Brien K, Bricher P, de Bruin T, Buck JJH, Burger EF, Carval T, Casey KS, Diggs S, Giorgetti A, Glaves H, Harscoat V, Kinkade D, Muelbert JH, Novellino A, Pfeil B, Pulsifer PL, Van de Putte A, Robinson E, Schaap D, Smirnov A, Smith N, Snowden D, Spears T, Stall S, Tacoma M, Thijsse P, Tronstad S, Vandenberghe T, Wengren M, Wyborn L and Zhao Z (2019) Ocean FAIR Data Services. Front. Mar. Sci. 6:440. doi: 10.3389/fmars.2019.00440
Received: 15 November 2018; Accepted: 05 July 2019;
Published: 07 August 2019.
Edited by:
Amos Tiereyangn Kabo-bah, University of Energy and Natural Resources, GhanaReviewed by:
Jan-Bart Calewaert, European Marine Observation and Data Network (EMODnet), BelgiumGreg Zacharewicz, Institut Mines-Télécom Mines Alès, France
Copyright © 2019 Tanhua, Pouliquen, Hausman, O’Brien, Bricher, de Bruin, Buck, Burger, Carval, Casey, Diggs, Giorgetti, Glaves, Harscoat, Kinkade, Muelbert, Novellino, Pfeil, Pulsifer, Van de Putte, Robinson, Schaap, Smirnov, Smith, Snowden, Spears, Stall, Tacoma, Thijsse, Tronstad, Vandenberghe, Wengren, Wyborn and Zhao. This is an open-access article distributed under the terms of the Creative Commons Attribution License (CC BY). The use, distribution or reproduction in other forums is permitted, provided the original author(s) and the copyright owner(s) are credited and that the original publication in this journal is cited, in accordance with accepted academic practice. No use, distribution or reproduction is permitted which does not comply with these terms.
*Correspondence: Toste Tanhua, dHRhbmh1YUBnZW9tYXIuZGU=