- 1Department of Biology, University of Louisiana at Lafayette, Lafayette, LA, United States
- 2Moss Landing Marine Laboratories, Moss Landing, CA, United States
- 3Buckley Environmental, LLC, Mount Pleasant, SC, United States
- 4Department of Biological Sciences, Bowling Green State University, Bowling Green, OH, United States
- 5Cooperative Institute for Great Lakes Research (CIGLR), University of Michigan, Ann Arbor, MI, United States
- 6Ocean Sciences Department, University of California, Santa Cruz, Santa Cruz, CA, United States
- 7Department of Oceanography, University of Hawai’i at Mānoa, Honolulu, HI, United States
- 8Cherokee Nation Businesses at NOAA Great Lakes Environmental Research Laboratory, Ann Arbor, MI, United States
- 9Chesapeake Biological Laboratory, University of Maryland Center for Environmental Science, Solomons, MD, United States
Recurrent blooms of harmful algae and cyanobacteria (HABs) plague many coastal and inland waters throughout the United States and have significant socioeconomic impacts to the adjacent communities. Notable HAB events in recent years continue to underscore the many remaining gaps in knowledge and increased needs for technological advances leading to early detection. This review summarizes the main research and management priorities that can be addressed through ocean observation-based approaches and technological solutions for harmful algal blooms, provides an update to the state of the technology to detect HAB events based on recent activities of the Alliance for Coastal Technologies (ACT), offers considerations for ensuring data quality, and highlights both ongoing challenges and opportunities for solutions in integrating HAB-focused technologies in research and management. Specifically, technological advances are discussed for remote sensing (both multispectral satellite and hyperspectral); deployable in situ detection of HAB species on fixed or mobile platforms (based on bulk or taxa-specific biomass, images, or molecular approaches); and field-based and/or rapid quantitative detection of HAB toxins (via molecular and analytical chemistry methods). Suggestions for addressing challenges to continued development and adoption of new technologies are summarized, based on a consensus-building workshop hosted by ACT, including dealing with the uncertainties in investment for HAB research, monitoring, and management. Challenges associated with choosing appropriate technologies for a given ecosystem and/or management concern are also addressed, and examples of programs that are leveraging and combining complementary approaches are highlighted.
Introduction
Phytoplankton are important primary producers in aquatic ecosystems and play integral roles in the cycling of carbon and other elements, food web production, and broader ecosystem function. In recent decades, ongoing eutrophication (Anderson et al., 2008; Heisler et al., 2008), increasing temperatures (Paerl and Huisman, 2008), and food web modifications (e.g., over-harvest of shellfish; Gobler et al., 2005), have been associated with an increased prevalence of phytoplankton blooms with toxic or deleterious properties. Some harmful algal blooms (HABs) are predicted to continue to increase with ongoing climate change (Hallegraeff, 2010) due to combined effects on water column structure, temperature, coastal development, and eutrophication (Rabalais et al., 2009; O’Neil et al., 2012; Paerl et al., 2016). There is evidence that increased CO2 (and reduced pH) in marine and freshwater ecosystems can also exacerbate blooms and increase toxicity of some HAB species, especially when combined with changes in nutrient availability (e.g., Fu et al., 2010; Tatters et al., 2012; Visser et al., 2016).
Recurrent blooms of harmful algae and cyanobacteria plague many coastal and inland waters throughout the United States and globally, and have significant socioeconomic impacts to the adjacent communities (Kudela et al., 2015a). However, the definition of a “bloom” varies as certain species may also have significant harmful impacts at concentrations below densities that will form a visible “bloom” (e.g., Dinophysis spp. which can be harmful at <103 cells L–1; Reguera et al., 2014). Notable HAB events continue to underscore the many remaining gaps in knowledge and increased needs for technological advances. Some exemplars include the toxic Microcystis blooms in Lake Erie that have been the subject of research for nearly two decades; yet the factors leading up to the 2014 closure of drinking water facilities in Toledo, Ohio, point to direct and still vulnerable linkages between biology and water supply and the potential for such significant human impacts in the future (Steffen et al., 2017). Furthermore, due to these persistent blooms, the states of Ohio and Michigan have declared their portions of western Lake Erie’s open waters as “impaired” due to cyanobacterial HABs under the Clean Water Act (Davis et al., 2019). In Florida, recent toxic cyanobacterial blooms in Lake Okeechobee (which have been occurring since the mid-1980s) led to a “State of Emergency” declaration for multiple counties in 2016 (Kramer et al., 2018) and 2018. The 2018 red tide events in Florida, caused by Karenia brevis, have made national and international headlines and have helped shape the results of state and federal elections in Florida. The persistent saxitoxin-producing Alexandrium blooms in the Gulf of Maine (McGuire, 2018) and Long Island Sound (Gralla, 2018) have also had economic impacts in those regions. Recently, long-lived HAB events such as the Pseudo-nitzschia bloom along the U.S. West Coast in 2015 also point to a new reality in which the spatial and temporal scales, as well as the potential for widespread human, animal, and ecosystem health impacts are maximized (McCabe et al., 2016; Ryan et al., 2017; Zhu et al., 2017). These challenges are mirrored globally with recent examples, including an expansion of Pseudochattonella cf. verruculosa to the south and Alexandrium catenella to the north in Chile, leading to massive fisheries closures in 2016, and disruption by A. catenella in Tasmania, southeastern Australia, from 2012 to 2017 of the fishing industry in poorly monitored coastal waters (Trainer et al., 2019).
HABs pose an increasingly complex issue for researchers and managers across the freshwater-marine continuum that has been a focal subject for the past two decades. A major challenge in understanding, forecasting, and ultimately mitigating HAB events across aquatic ecosystems is their high degree of heterogeneity (temporal and spatial) in species composition, non-point source factors that contribute to blooms, and imperfect relationships between biomass and toxicity. The relationship among HABs and eutrophication, for example, requires multiple temporal scales of study and interaction with ecologists, climate scientists, modelers, policy-makers, and managers to understand, prepare for, respond to, and mitigate such events (Glibert et al., 2010). A critical component to understanding, predicting, and addressing the significant ecological, economic, and human health issue posed by HABs lies in the research, monitoring, and management communities’ ability to (1) understand the factors and mechanisms contributing to HABs – both generally and specifically, (2) robustly detect changes in biomass, especially with respect to taxa that include HAB species, and (3) provide the earliest possible indications of toxic events to most effectively protect animal and human health and mitigate economic losses. Ultimately, investments in policy and research into HAB ecology, management, and mitigation have been mandated by the U.S. Federal Harmful Algal Bloom and Hypoxia Research and Control Act (HABHRCA), which was authorized by Congress in 1998 and reauthorized in 2004, 2014, and December 20181. These investments have more recently been advanced as part of the Ecological Forecasting Roadmap program within the U.S. National Oceanic and Atmospheric Administration (NOAA2).
Cullen (2008) suggested that a “revolution in coastal oceanography, monitoring, and management [was] inevitable,” a prediction that has seen progress but not entirely come to fruition a decade later. Recent activities of the Alliance for Coastal Technologies (ACT), and other groups, are attempting to move the ocean observing community further toward this goal. ACT is a component of the U.S. Integrated Ocean Observing System (IOOS) with the specific functions to serve as: (1) an independent, third-party testbed for evaluating existing, new, and developing aquatic sensors and sensor platforms, (2) a comprehensive data and information clearinghouse on environmental technologies, and (3) a forum for capacity and consensus building. This review summarizes the main research and management priorities that can be addressed through ocean observation-based approaches and technological solutions for harmful algal blooms, provides an update to the state of the technology to detect HAB events based on recent ACT activities, offers considerations for ensuring data quality, and highlights both ongoing challenges and opportunities for solutions in integrating HAB-focused technologies in research and management.
Priorities in Research and Management
Despite decades of research into the causes and effects of HABs, fundamental questions regarding the biology and ecology of the organisms – both alone and within complex ecosystems – and their toxins (Granéli and Flynn, 2006) still exist. Decades of research have identified many of the harmful species responsible for toxic blooms; however, the physiological drivers of intracellular toxin production have not been fully elucidated. Moreover, the genetic controls on toxin production and their variability within and among species and strains are also still largely unknown. This is compounded by underlying cryptic diversity within genera/species that can affect detection capabilities for monitoring purposes. On a broader scale, factors (biotic and abiotic) controlling bloom initiation, persistence, and decline are not fully known. The variables involved can be very complex and work synergistically, including availability of micro- and macronutrients, physical parameters (e.g., irradiance, salinity, temperature, water column mixing, pH), biological influences (e.g., bacteria, viruses, grazers, fungal parasites), and species/strain diversity within blooms. Further, the impact on and fate of marine and freshwater toxins in local food webs, along with toxin dynamics (timescales for uptake, retention, and depuration) in targeted species (e.g., shellfish, commercially important finfish) are also critical areas of ongoing research.
While researchers work to connect these complex factors with a common goal to better understand bloom events, managers and other stakeholders are charged with incorporating tools for rapid and inexpensive detection of species and their toxins in a variety of ecosystems. To facilitate reaching the objectives of these diverse groups, ACT convened a recent workshop that brought together a broad range of experts from regional, national and international agencies, as well as instrument manufacturers, to identify current needs and challenges associated with HAB technologies and their transition into management applications. The myriad of potential factors outlined above that can impact bloom dynamics were threaded through discussions that resulted in a consensus of priorities (Alliance for Coastal Technologies [ACT], 2017). Several over-arching themes emerged. First, a need was recognized to strike a balance between development of user-friendly robust sensors (deployable/handheld) that are not cost-prohibitive (e.g., instrument, reagents, expertise) and that have broad applicability (e.g., detection of multiple species and/or toxins). Second, establishment of community-derived certified reference standards (for both toxins and organisms) for use during sensor (or kit) development to better understand the sensor/kit’s suitability across broad geographic regions was seen as a needed activity which could be readily accomplished. Third, a need was identified to increase the standardization of using those sensors as an early warning system (in line with regulations), especially where there is a threat to human health. Fourth, the leveraging of multiple platforms was identified as a way to increase the spatial and temporal resolution of observations; however, issues related to storing the vast quantities of data generated from those efforts and how to derive usable information to incorporate into prompt management decisions and public outreach were also discussed. Finally, workshop participants identified the need to establish more long-term sources of funding as a priority in support of greater networked platforms and instrumentation, thereby ensuring better continuity in valuable data sets.
Quantifying Effects on Ecology and Economies
Complex chemical signals from HAB species can lead to difficulty in understanding the significant, yet variable, effects of HABs on ecosystems and organisms. HAB species generally share some toxic, noxious, or allelochemical properties that enable them to escape predation, at least to some effect (Turner and Granéli, 2006). Members of the micro- and mesozooplankton are often unable to consume toxin-producing HAB species (e.g., Turner, 2010), and these deterrent properties may also be highly species- (e.g., Stauffer et al., 2017) and/or condition-specific (e.g., Davis and Gobler, 2010). HAB toxins and allelochemicals may also impact communities of organisms elsewhere in the food web, for example through shifts in microbial food webs, especially under low grazing pressures (Weissbach et al., 2011). Such vast ecological effects of HABs have traditionally been extremely challenging to adequately quantify; however, increased efforts to network platforms and sensors will continue to push through this bottleneck.
Economic costs of HABs are difficult to accurately assess and vary greatly among systems. With a focus on marine HABs, Anderson et al. (2000) reported costs of approximately $50 million USD per year to the U.S. economy (in 2000 dollars), primarily based on losses due to public health effects and commercial fisheries. In freshwater systems in the United States, potential eutrophication-related economic losses of $4.6 billion USD annually (in 2008 dollars) are attributed, at least in part, to cyanobacterial blooms (Dodds et al., 2009). In Florida, annual blooms of K. brevis cause $22 million USD per year in losses due to medical expenses and lost work days and $18 million USD per year in losses to commercial fishing (in 2000 dollars; Florida Department of Health). On the U.S. West Coast, the months-long Pseudo-nitzschia bloom in 2015 resulted in losses of $48 million USD to the Dungeness crab industry, alone (Brown, 2016). Most studies have been focused on HAB events in the United States, a bias in data that has been identified in other reviews of economic costs (Sanseverino et al., 2016). A few exceptions exist (as reviewed in Sanseverino et al., 2016), which estimate costs on human health in Canada ($670,000/year CAD; Todd, 1993), impacts to commercial fisheries in the United Kingdom (up to £118,00/year; Pretty et al., 2003), and monitoring and management costs in European countries (ranging from €30,000 to over €7 billion/year; Anderson et al., 2001; Ahlvik and Hyytiäinen, 2015), Australia (e.g., $1–8.7 million/year AUD; ATECH, 2000; as reviewed by Steffensen, 2008), and New Zealand ($50,000/quarter NZD; Kouzminov et al., 2007). These estimates differ in the HAB effects considered and the methods used to derive them.
The inability to accurately define and quantify HAB impacts is further exemplified by the complexities of ongoing events in the Great Lakes where losses in property values, commercial fishing, recreation, and cultural services intersect. The Lake Erie basin is the most densely populated of the Laurentian Great Lakes (International Joint Commission, 2014), providing ecosystem services (including drinking water) for 11.6 million people (Lake Erie Lakewide Action and Management Plan Work Group [LAMP], 2011) and generating over $7 billion USD in annual revenue through tourism and fishery industries (U. S. Department of Agriculture, 2005). Increasingly dense and toxic HAB events have had significant negative impacts on these facets of local economies. Estimated partial economic losses of $65–71 million USD per year have been reported as a result of western Lake Erie HABs (Bingham et al., 2015). In Ohio, alone, beach recreation losses at Maumee Bay State Park and recreational fishing losses due to HABs were estimated to exceed $3.7 million USD in 2011 (International Joint Commission, 2014), which coincided with the second largest cyanobacteria HAB event ever recorded in Lake Erie (Michalak et al., 2013). These economic figures are likely underestimates and, indeed, do not account for the costs of events like the 2014 Toledo water crisis that left over 400,000 residents without drinkable tap water for just over 48 h.
The need to understand, predict, and mitigate HAB events such as those described above creates a strong impetus for investment into research and management strategies. Bernard et al. (2014) estimated that an approximate global figure for HAB-related economic losses across marine and freshwater systems could be estimated at ±$10 billion USD annually (as of 2014). Based on this and using a typical value of information (VOI) estimate of 1% of the “resource” (in this case HAB-related losses; Macauley, 2006), a comprehensive HAB observing and forecasting information system would represent a value of ±$100 million USD annually, providing a reasonable first estimate for how much could or should be invested in HAB monitoring efforts. A Clearly, such observing and forecasting systems represent investments with the potential for high return.
Monitoring HABs for Early Detection of Health Risks
Ecosystem health effects of HABs are widespread, including direct impacts through toxin production and physical damage, as well as indirect effects such as blooms leading to anoxia and hypoxia and disrupting system function and dynamics. Toxic effects are the most widely recognized and impact aquatic organisms, including marine mammals, via bioaccumulation, and can directly impact human health through consumption of contaminated drinking water or shellfish. HABs and their toxins can also negatively impact aquatic birds (Jessup et al., 2009), fish (Chang et al., 1990; Lefebvre et al., 2005; Liefer et al., 2013; Rountos et al., 2014), shellfish (Botes et al., 2003; Glibert et al., 2007), marine mammals (Scholin et al., 2000; Miller et al., 2010), and others. These impacts can range from the tropics (Friedman et al., 2008; Litaker et al., 2010; Cuellar-Martinez et al., 2018) to polar oceans (Berdalet et al., 2015; Lefebvre et al., 2016). In the past decade, the emerging issue of toxic cyanobacteria in estuarine ecosystems has generated additional concern (O’Neil et al., 2012) and expanded the list of affected marine mammals to those spanning the land-sea interface (Miller et al., 2010; Gibble and Kudela, 2014).
Established and emerging technologies discussed in subsequent sections are currently being utilized to protect humans from the harmful effects of HABs. Since 1991, traditional methods to identify HAB species (by microscopy) and toxins [enzyme-linked immunosorbent assays (ELISA) and high-pressure liquid chromatography (HPLC)] have been used to monitor for Pseudo-nitzschia and other HABs in Puget Sound, Washington State, the largest shellfish-producing state on the U.S. West Coast valued at over $77 million USD each year (Trainer et al., 2007; King et al., 2018). In 2003, these methods were used to prompt the first closure of recreational, commercial, and tribal subsistence shellfish harvesting in the region (Trainer et al., 2007), and this approach has been augmented with citizen science into an integrative monitoring network (Trainer and Hardy, 2015). Citizen scientists and volunteers have been identified, more broadly, as valuable tool in HAB monitoring efforts. The NOAA-funded National Phytoplankton Monitoring Network (PMN3) has utilized these cohorts to discover over 225 blooms throughout coastal United States since 2001 and contribute directly to the state monitoring plans of Texas, Mississippi, Alabama, Georgia, and South Carolina. Using a more technology-enabled approach, the first reported bloom of Dinophysis in United States waters was detected in 2008 in the Mission-Aransas estuary of Texas (Campbell et al., 2010). Early detection of this HAB by an Imaging Flow Cytobot (IFCB, see below) and manual microscopical verification allowed for closing of the oyster fishery (Deeds et al., 2010) and cancelation of a local oyster festival at which 30,000 visitors may have been exposed to the toxic shellfish (Campbell et al., 2010; Deeds et al., 2010).
HABs in freshwater systems that also serve as drinking water sources represent an especially critical need for research and implementation of early warning systems. The need to integrate portable, rapid, cost-effective technologies to detect cyanotoxins in water or in shellfish (or other organism) tissue in near-real time is critical, given the high expense of treatment to eliminate microcystins in drinking water. In a 2009 survey of 15 public water systems in Ohio, 66% reported using additional funds to treat for elimination of microcystins with a total cost of over $400,000 USD (Ohio EPA, 2010). Water treatment facilities that have had source water cyanotoxin detections are required by rule to have approved plans that detail their short and long-term actions to deal with cyanobacteria and cyanotoxins (Ohio EPA, 2019a, b). Following the Toledo water crisis, Ohio Environmental Protection Agency (Ohio EPA) approved a $4.4 million USD plan in 2014 for short-term HAB treatment upgrades to the City of Toledo’s water treatment plant and a $44.5 million USD plan in 2018 for long-term HAB treatment upgrades. These upgrades are part of a larger ten-year, $500 million USD plan to upgrade the Toledo treatment system (as reported by Elms, 2019), and other Ohio water systems have invested over $150M in treatment upgrades to address microcystins contamination (H. Raymond, Ohio EPA, personal communication). Importantly, these costs are just for one state, while cyanobacteria HABs are known to occur throughout the Great Lakes (e.g., Davis et al., 2014) as well as in other key freshwater systems worldwide (e.g., Zhu et al., 2014; Ma et al., 2016; Puddick et al., 2019).
Forecast and Predictions of HAB Events
Significant investments have been made by both the research and management communities to advance ecological forecasting and predictions of bloom events. On the West Florida Shelf, blooms of K. brevis in summer-fall affect water quality, food webs, resident health, and tourism economies. Since the 1990s, researchers and managers have used remote sensing to monitor and track these “red tide events” in near-real time (Stumpf et al., 2003), and those efforts now contribute to the twice-weekly HAB bloom forecasts for coastal Florida.4 These forecasts also make use of cell counts provided by a number of partners.5 Considerations of HAB ecology – more specifically, an understanding of upwelling strength and K. brevis success (versus competing diatoms, for example) – have recently been identified as necessary to improving the Florida HAB models (Weisberg et al., 2016).
In Lake Erie, NOAA currently delivers a 5-day operational forecast of Microcystis aeruginosa blooms (HAB Bulletin6) during the summer months when they occur using core observing and modeling components that combine satellite imagery, weather forecasts, and modeled currents (Stumpf et al., 2012; Bridgeman et al., 2013; Obenour et al., 2014). Further research-to-operations activities are focused on development of an improved Lake Erie HAB Tracker,7 which includes modifications to describe vertical distributions of the cyanobacteria based on their biological characteristics (e.g., Rowe et al., 2016). The incorporation of 3-dimensional physical circulation models to these systems is critical, given that the onset of many HAB events may go undetected because the bloom is initially concentrated in discrete thin subsurface layers in the water column that are easily missed by conventional sampling methods (McManus et al., 2008).
Finally, a pre-operational forecast model is under continued development off the California coast of the United States, where a year-long bloom of Pseudo-nitzschia spp. significantly impacted marine ecosystems and coastal economies (McCabe et al., 2016). The California-Harmful Algae Risk Mapping program (C-HARM8) provides experimental nowcast and forecast products based on satellite imagery, physical circulation models, and statistical predictions of bloom or toxin probability (Anderson et al., 2016). While C-HARM is driven entirely by modeled or remotely sensed dynamics, model fit and verification is accomplished by comparison to measured HAB species abundance in the study region (Anderson et al., 2016).
These regionally specific efforts reflect a national directive. NOAA has adopted Ecological Forecasting as a central tenet of its mission and developed an operational framework – or “roadmap” – to build consistency and maximize efficiency of developing high quality forecasting products across ecosystems and for diverse stakeholders (NOAA, 2015). The roadmap is intended to help establish the priorities and coordination of existing monitoring capabilities, and to develop scientific foundation, operational environmental prediction, and service delivery infrastructure. The roadmap strives to maximize the benefit of the extensive observational assets from NOAA and its partners that collects huge amounts of weather, water, climate, oceanographic, coastal, and biological data, and to combine these data with the modeling, computing, and forecasting capacities within the agency to deliver consistent, timely, and reliable ecological forecasts to the American public. Since 2015, NOAA’s initial priorities have focused on developing ecological forecasts for HABs, hypoxia, pathogens, and habitat, specifically in regions of the country where these are issues of major concern (NOAA, 2015).
Even in well-studied systems, however, challenges remain to tracking and forecasting blooms, including ambiguity in what constitutes a “bloom” (e.g., Smayda, 1997), what data should be used to develop and calibrate models of blooms, and how closely measurement methods and metrics must agree (Ho and Michalak, 2015). Furthermore, adoption of ecological forecasting tools are likely to vary among decision makers and stakeholders based, at least in part, on the complexity of the forecast output relative to the integration of such tools into a stakeholder’s decision-making process (e.g., Gill et al., 2018; Davis et al., 2019). Thus, continued refinement of priorities for research and management; cooperation among researchers, forecasters, and the users of forecasts; and investment in best practices to advance our understanding, prediction, monitoring, and management of HABs is crucial.
State of the Technology
To address a varied set of priorities for both research and management, HAB detection technologies come in a wide array of methodological bases and with a huge diversity of costs, usability, and downstream data products. A combination of these technological approaches, platforms, and products are needed to meet recommendations set forth by national and international (i.e., IOC Intergovernmental Panel on Harmful Algal Blooms [IOC-IPHAB], 2009) entities. Table 1 summarizes many of the existing and near-commercial technologies used for detection of biomass, taxa, or the toxins produced by HAB species. Most of the technologies listed in Table 1 are discussed in greater detail in subsequent sections. Readers are directed toward additional reviews which provide a more detailed treatment of many of the technologies that are not discussed in detail (e.g., Ottesen, 2016; Doucette and Kudela, 2017; Glibert et al., 2018) and/or which focus more on classical methods for cell and/or toxin detection (e.g., Frolov et al., 2013; Trainer and Hardy, 2015; Zhang and Zhang, 2015; Otten and Paerl, 2016; Reguera et al., 2016; Association of Public Health Laboratories [APHL], 2017; Medlin and Orozco, 2017; Doucette et al., 2018; Glibert et al., 2018).
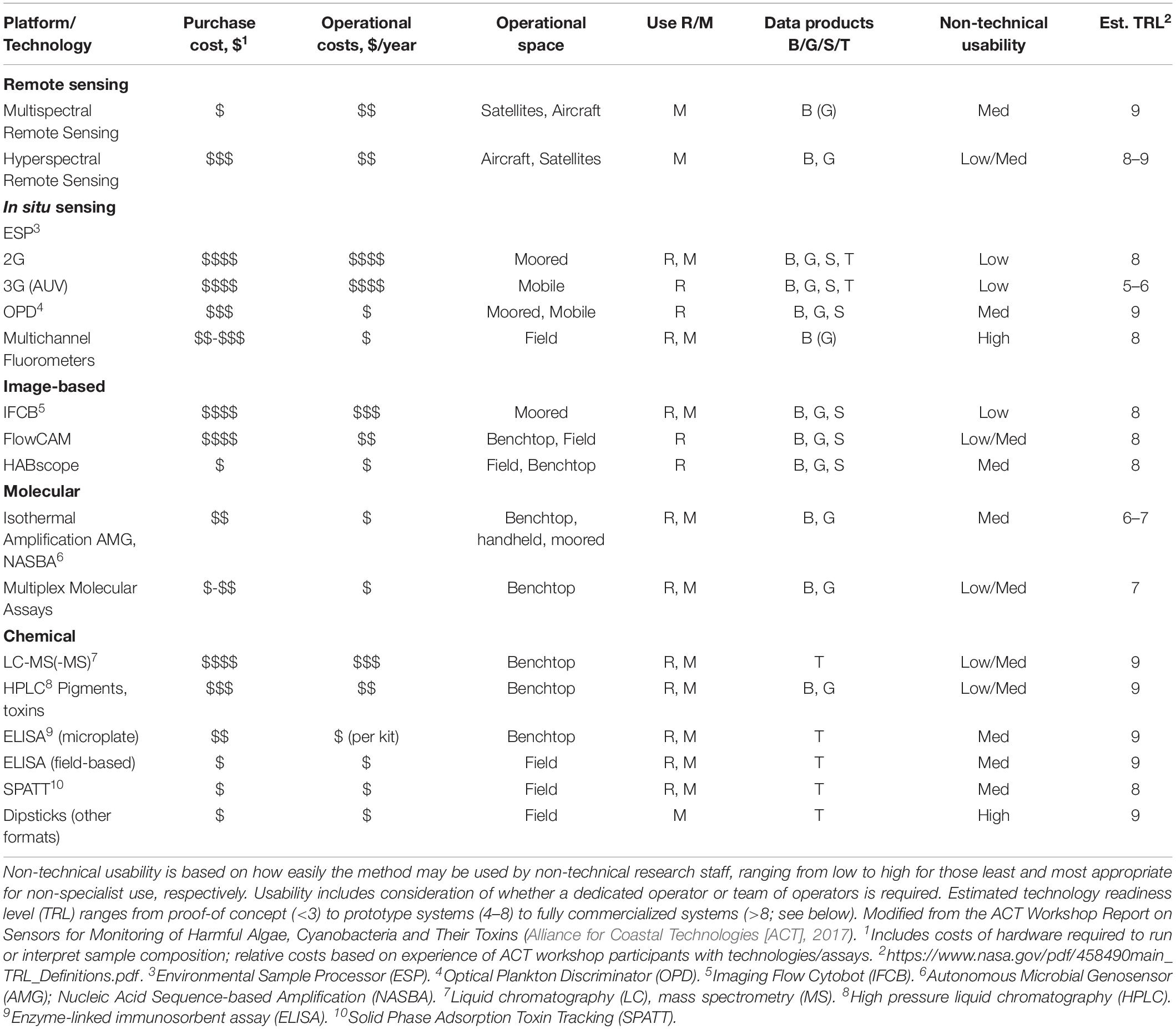
Table 1. Summary of existing technologies currently used to measure HAB biomass, taxa, or toxins, indicating relative purchase and operational costs, operational space (i.e., benchtop versus moored technologies), effectiveness of use for research (R) versus monitoring (M), data products measured (B: biomass, G: genus, S: species, T: toxin).
Remote Sensing Approaches
The mainstay for satellite-based bloom detection has been the use of ocean color to quantify chlorophyll biomass and organic carbon in the upper water column from Sea-viewing Wide Field-of-view Sensor (SeaWiFS), Moderate Resolution Imaging Spectroradiometer (MODIS), Medium Resolution Imaging Spectrometer (MERIS), Visible Infrared Imaging Radiometer Suite (VIIRS), and the ocean and land colour instrument (OLCI) sensor on Sentinel-3. With continuous data since 1997, satellite-based remote sensing offers approximately daily temporal and large spatial resolution (Siegel et al., 2013). Limitations of satellite-based remote sensing, however, include: (1) restriction of data to the surface ocean, typically <15 m in the open ocean but much more shallow in coastal waters, which is an issue if HABs are concentrated in discrete subsurface thin layers (McManus et al., 2008); (2) interferences of cloud cover and land masses, thus limiting usefulness in nearshore waters where HABs may initiate (e.g., Ryan et al., 2008); and (3) restricted resolution of different groups of phytoplankton from complex, changing communities. Efforts to better resolve phytoplankton size- or pigment-based functional groups have been underway for at least the last decade, with variable results. A recent review of algorithms to deconstruct ocean color data into phytoplankton functional groups highlights the advantages and limitations in these methods (Mouw et al., 2017), and at least some methods are capable of differentiating among larger and smaller size-fractions of phytoplankton communities (Mouw et al., 2016). Efforts to correlate functional groups on the basis of pigment suites between in situ hyperspectral remote-sensing reflectance and HPLC pigment concentrations, however, are limited to robust estimates of three chlorophylls (chlorophylls a, b, c1 + c2) and photoprotective carotenoids (Chase et al., 2017). Significant work remains to advance satellite remote sensing approaches to more fully resolve phytoplankton community dynamics or provide early warning for HAB events in complex communities. Such work is continuing through new programs such as NASA’s Plankton, Aerosol, Cloud, ocean Ecosystem (PACE) mission (United States) and the EnMAP mission in Germany (Chase et al., 2017).
Compared to space-borne sensors, lower altitude hyperspectral imaging (HSI) sensors have the ability to fly beneath clouds where passive satellite sensors are unable to detect and offer hundreds of narrow bands (10–20 nm) compared to the limited bands from satellite sensors. With benefits derived from lower altitude sensors, HSI sensors provides an information-rich diversity of spectral signatures that can be used to sense a wide range of surfaces, habitats, taxonomic groups, and changes over time in all of these signatures. HSI of aquatic targets has the potential to expand our knowledge of dynamic coastal ecosystems; however, more investigation is needed to fully realize the extent of its utility (McManus et al., 2018). Specific questions hyperspectral techniques can address include: “what is the biodiversity of aquatic systems, and more specifically what different phytoplankton communities are present, including toxic species?” The increased spectral detail strengthens the ability to discriminate between different types of phytoplankton (Palacios et al., 2015) and the timing of HAB events based on the shift in the phytoplankton community structure between seasons. This provides data validation for ecosystem modelers, and individual hyperspectral images act as an early warning HAB detection system for drinking water intake managers (e.g., western basin of Lake Erie; Vander Woude et al., 2019) and managers of recreational water bodies impacted by HABs (e.g., Kudela et al., 2015b). Examples of HSI sensors that are used for HAB detection include the Resonon Pika II and Pika L, operated by NOAA’s Great Lakes Environmental Laboratory with weekly flights to detect HABs beneath clouds and nearshore (Vander Woude et al., 2019) and bio-optical algorithms to discriminate phytoplankton functional types that include diatoms, cyanobacteria, chrysophytes, and chlorophytes from the imagery. An HSI sensor’s spectral detail is also typically matched by a finer spatial resolution to delineate pixel features and, in the case of drinking water intakes, can provide the level of detail needed for municipalities in combination with satellite remote sensing products. However, the costs associated with flying these HSI-equipped aircraft can be significant, which means that HSI may not be viable as a first line of monitoring without a sustained funding source. A current alternative at NOAA GLERL is educating drinking water managers how to operate a simple unmanned aircraft system (UAS, Wu et al., 2019).
Remote sensing in all capacities can present a broad view of HAB conditions (e.g., Clark et al., 2017), act as a rapid response tool in cases where imagery is used in HAB forecasting models (i.e., HAB Tracker, Rowe et al., 2016) and for products including the NOAA HAB Bulletins (i.e., Lake Erie and the Gulf of Mexico), and advance understanding of the extent of HABs. However, it is worth noting that the capability to sub-divide whole phytoplankton biomass into functional groups based on a combination of backscatter properties to estimate size and/or absorption peaks of pigments (and via satellite-based or HSI systems) will still lack the resolution required to detect HAB taxa or differentiate toxic from non-toxic members of the same genera or species (e.g., Stumpf et al., 2016).
In situ Sensing Approaches
Methods for measuring phytoplankton biomass in situ in the environment range from single- to multi-spectral fluorometers, absorption/backscatter sensors, and particle size analyzers to quantify particles based on in vivo pigments or size characteristics. Since these technologies provide only bulk or size-based signals that are not specific to HAB taxa, they are not included in the current discussion of HAB technologies. The reader is directed to other recent reviews of technologies for quantifying planktonic ecosystems for more information (Lombard et al., 2019). Moving beyond bulk biomass, the diagnostic pigments whose unique absorption spectra are used to discriminate among phytoplankton classes using benchtop HPLC (Mackey et al., 1996; Garrido and Roy, 2015) are also targets for more recently developed multi-channel fluorometers that measure in vivo pigments (Gregor and Marsalek, 2004; Proctor and Roesler, 2010).
A key utility of these new multi-excitation fluorometers is to be able to track fine-scale variations in not just phytoplankton density, but in community composition as well. Recent verification testing of multi-channel fluorometers by ACT highlights their advantages and limitations in resolving potential HAB taxa from complex community matrices under a variety of applications including laboratory testing, underway surface mapping, and moored deployments. Within the recent ACT technology evaluation, a 28-day moored deployment of the PhycoProbeTM (BBE Moldaenke) was conducted in Chesapeake Bay at Solomons, MD (Figure 1). The PhycoProbeTM was able to characterize changes in the phytoplankton community structure across daily to monthly scales in relationship to variations in drivers such as river discharge, salinity, and tidal mixing. The PhycoProbeTM leverages multichannel excitation and fluorescence to enable discrimination among multiple high-level algal classes based on pigment signatures (green algae, blue-green algae, diatoms, and cryptophytes). The utility of these instruments to be incorporated into underway surveys is further highlighted in Figure 2, which shows the contoured results from over 2,000 observations from the PhycoProbeTM over a 50 km survey in western Lake Erie. While the results shown are for chlorophyll-a biomass as an integrated measure, the high spatial and temporal observations are able to resolve detailed patterns identifying hot spots within the lake and patterns of dispersal of the Maumee Bay source water into the open lake (Figure 1). Complete summaries of the ACT technology evaluation of multi-excitation fluorometers can be obtained in product-specific technical reports9. Continued innovations in development of sensors to quantify biomass (Zeng and Li, 2015; Gull et al., 2016) and, specifically, to discriminate among phytoplankton and potential HAB taxa include scaling down such technologies in size, power requirements, and cost (Zieger et al., 2018).
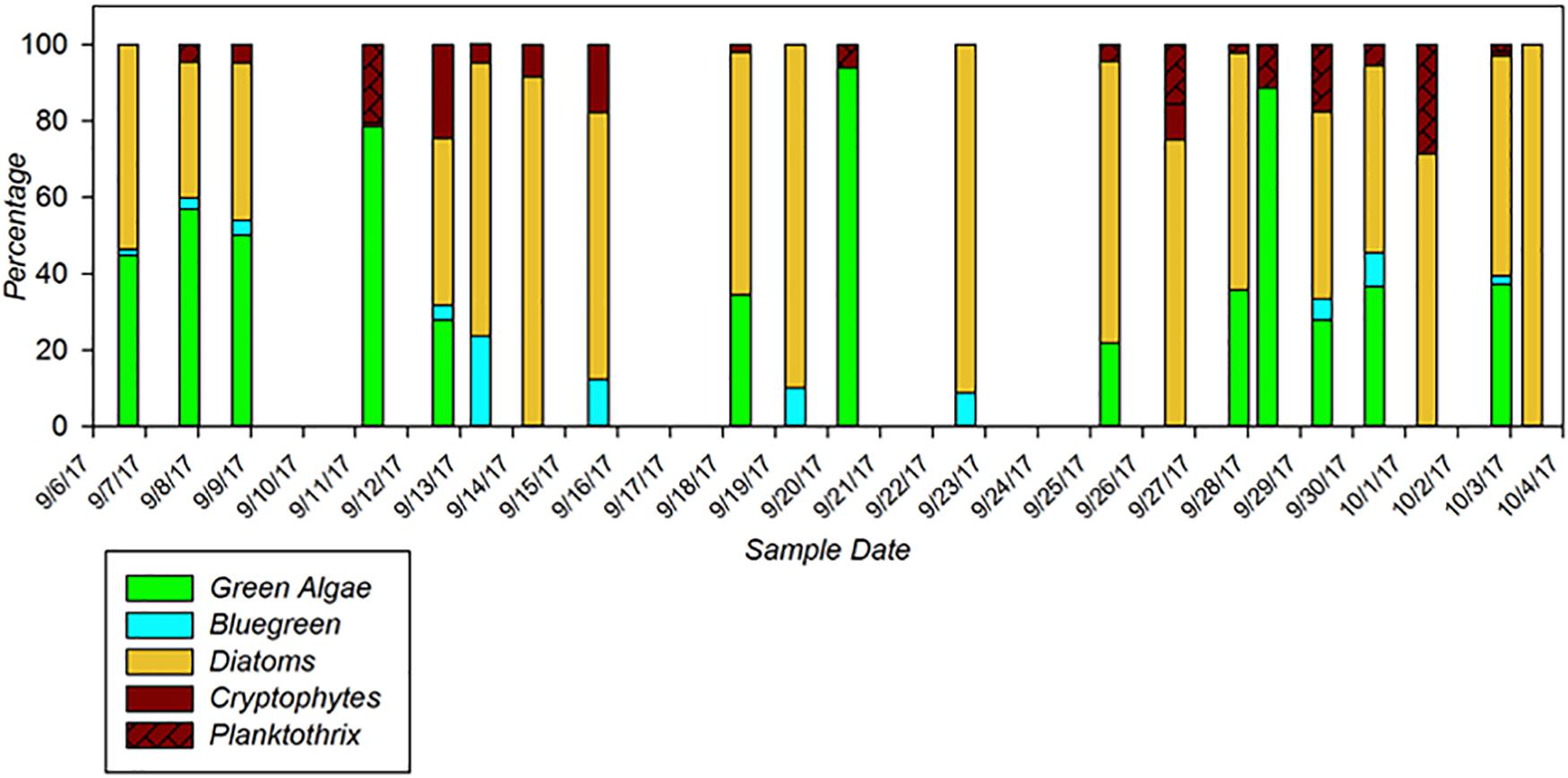
Figure 1. Phytoplankton group classifications generated by the Moldaenke Gmbh PhycoProbeTM during a 2-week moored deployment in the Chesapeake Bay during the ACT performance verification of in situ fluorometers for detecting HABs. Colors indicate major phytoplankton groups, including green algae (green), cyanobacteria/bluegreen algae (blue), diatoms (orange), cryptophytes (brown), and the cyanobacteria Planktothrix (brown, hatched). Modified from ACT Verification Report (www.act-us.info/evaluations.php).
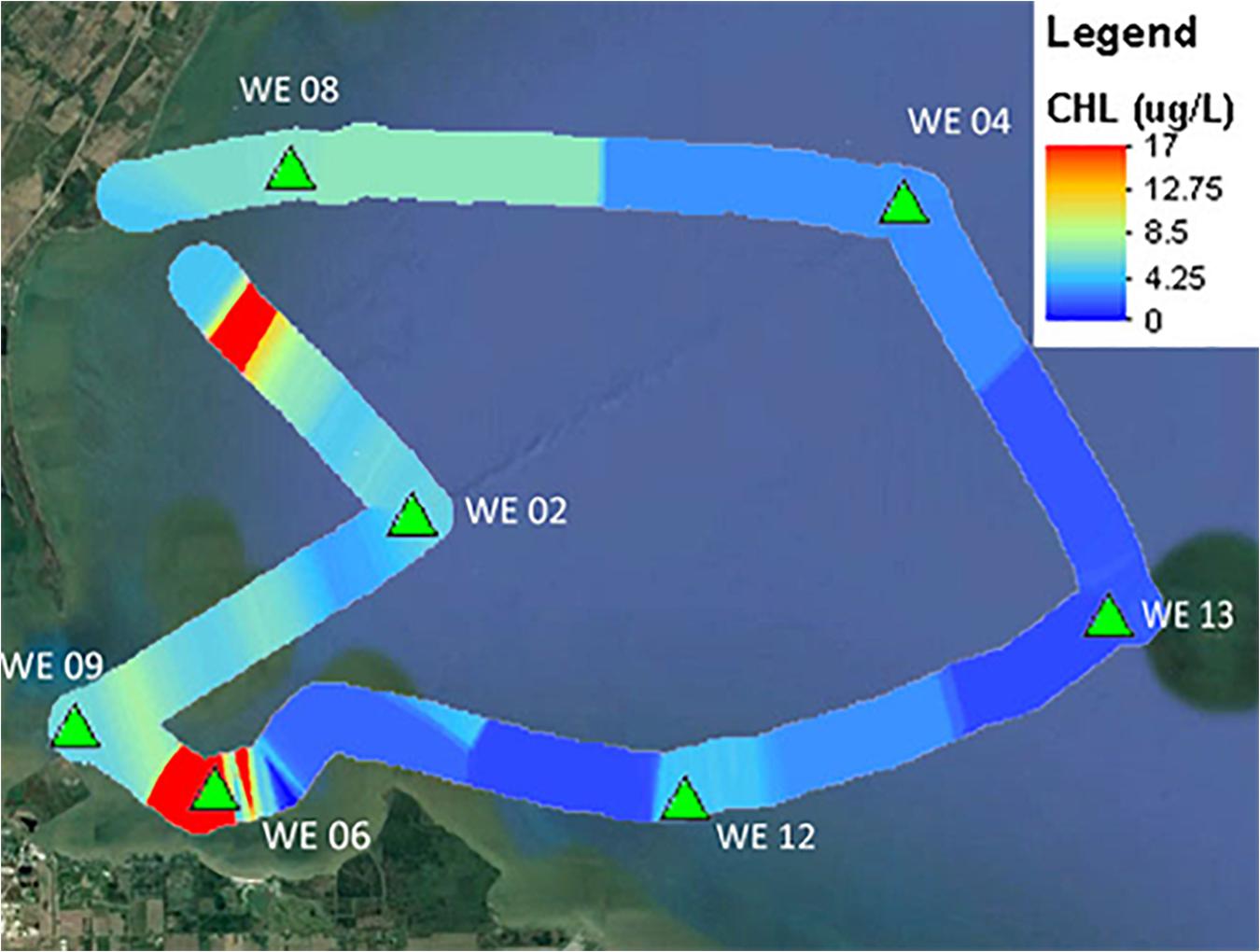
Figure 2. Chlorophyll-a contour plot generated by the PhycoProbeTM during an underway mapping survey in western Lake Erie during the ACT performance verification of in situ fluorometers for detecting HABs. Color bar indicates chlorophyll-a concentration ranging from high (red) to low (blue). Modified from ACT Verification Report (www.act-us.info/evaluations.php).
Other in situ sensing approaches bring optical, chemical, and/or molecular methods to moored and mobile platforms. The Optical Plankton Discriminator (OPD), also known as the Brevebuster, was developed in the late 1990s by Mote Marine Laboratory to detect optical signatures of the toxic dinoflagellate, K. brevis, based on its unique pigment signature in mixed phytoplankton communities (Kirkpatrick et al., 2000; Shapiro et al., 2015). Since its initial development for K. brevis detection, the OPD approach has been used to discriminate other taxa (e.g., Zapata, 2005), as well as CDOM (Kirkpatrick et al., 2011), an important tracer of land-based runoff into coastal oceans.
Similarly, the Environmental Sample Processor (ESP), originally developed as a “lab in a can” for moored deployments (Scholin, 1998; Scholin et al., 2001), uses immuno- or molecular probe-based assays to detect and quantify HAB species (Greenfield et al., 2008; Bowers et al., 2017) and toxin (Doucette et al., 2009). The ESP is also capable of collecting and storing fixed samples for later analyses by standard or benchtop methods (e.g., Bowers et al., 2016). Recent deployments on the US West coast have been used to determine the feasibility of simultaneous detection of fecal indicators and HAB species toward same-day alert systems for managers (Yamahara et al., 2015); to detect HAB populations in relation to oceanic processes and bloom initiation (Seegers et al., 2015); and to understand fluctuations in a key HAB species and its toxin in Monterey Bay throughout an un-precedented coast-wide bloom event (Ryan et al., 2017; Bowers et al., 2018). In the Great Lakes, ESP technology was first applied in 2016 to provide for near-real time detection of microcystin concentrations to support timely source water monitoring for the Toledo Drinking Water Plant managers. The application of this technology significantly advanced the frequency and timeliness of toxin monitoring. Prior ship-based monitoring only produced a weekly measurement, whereas the ESP enabled measurements at a twice daily frequency. Efforts are underway to incorporate these local in situ toxin observations into lake-scale operational hydrodynamic-based forecasting models which could then provide 3 to 5-day forecasts of potential delivery of high toxin concentrations to specific locations of intakes or public beaches. ESP deployments in the Gulf of Maine also contribute to the forecasts and monitoring of Alexandrium blooms used to manage shellfisheries in that area (Canfield, 2013).
Such in situ sensing approaches are being increasingly incorporated into mobile, autonomous platforms, including autonomous underwater vehicles (AUVs) and autonomous surface vehicles (ASVs), which expand the spatiotemporal range of these instruments (Robbins et al., 2006; Schofield et al., 2008). Both the OPD and ESP have been successfully deployed on moored (Seegers et al., 2015; Yamahara et al., 2015; Ryan et al., 2017; Bowers et al., 2018), ship-based (Schofield et al., 2006), and autonomous mobile platforms (Hails et al., 2009; Beckler et al., 2018), with the third generation ESP model (ESP-3G) specifically designed for integration into a long range AUV (LRAUV) platform (Pargett et al., 2015). The capabilities of these mobile deployments may help to offset the high purchase costs (Table 1) and limited deployment duration of such instruments (i.e., due to power consumption) by providing temporally and spatially rich data at lower per sample cost (versus ship-based sampling); however, more detailed economic analyses of such sampling approaches and returns on investment are not readily available.
Image-Based Approaches
Several lab and field-portable image-based technologies have been developed over the last decade, and many of them are routinely used as part of HAB monitoring programs. Perhaps the best known are the FlowCAM (Fluid Imaging Technologies Inc.) and IFCB (McLane Research Laboratories Inc.). The FlowCAM uses fluidic principles based on flow cytometry, coupled with light microscope-like optics to detect and monitor for the presence of HAB species. Although primarily a benchtop instrument, its portability outside of the laboratory has been demonstrated by dockside and shipboard applications. The autonomous IFCB is a submersible, imaging flow cytometer. The IFCB combines flow cytometry and video technology to generate high-resolution images of particles ranging from <10 to 150 μm in size. Both instruments provide cytometric and image data, and both are configured with software that can be used to identify phytoplankton based on a combination of cytometric and visual features. The ability of the IFCB to monitor the entire phytoplankton assemblage coupled to an automated image classifier provides the ability to identify HAB taxa in near real-time. The efficacy of this approach has been demonstrated along the coast of Texas, United States, where resource managers were alerted to the presence of a toxic bloom of Dinophysis, averting potentially serious human exposure through consumption of shellfish (Harred and Campbell, 2014). However, it must be noted that effective and accurate identification of phytoplankton species using automated algorithms remains a bottleneck to use of these technologies and often still requires human assistance (Culverhouse et al., 2006; Benfield et al., 2007), although advancements are being made (Luo et al., 2018).
Other imaging and/or cytometric systems are also commercially available, including the CytoSense (Cytobuoy b.v.) system which can be integrated into a CytoBuoy for field operations. The CytoSense/CytoBuoy uses dual lasers to scan a phytoplankton cell’s surface, providing information on cell size and pigment distribution within individual cells. It can image particles ranging in size from 0.5 to 900 μm in diameter and several mm in length and can discriminate the number of cells in chain-forming species. Automated classification is supported through image capture, similar to the IFCB.
Rapid Detection of Toxins
A suite of robust, lab-based analytical methods have been developed and are currently accepted for detection and quantification of HAB toxins. These include liquid chromatography coupled with tandem mass spectrometry (LC-MS-MS), ELISA, and the mouse bioassay, among others. This paper is focused on newer, field-deployable technologies or rapid quantification methods better suited for integration into ocean observing and monitoring systems. As such, readers are directed toward the many published reviews, and methodological descriptions of these standard analytical approaches (Harada et al., 1999; Pierce and Kirkpatrick, 2001; Kudela, 2011; Kaushik and Balasubramanian, 2013; Weller, 2013; Sanseverino et al., 2017; Glibert et al., 2018).
Molecular Approaches
Molecular methods to detect HAB cells have been developed and refined over the past few decades; however the elucidation of specific genes associated with toxin production has been a long and arduous process (see Cembella and John, 2006, for a review on the complex nature of HAB toxin gene discovery). Some pathways are well-established (cyanotoxins: Nishizawa et al., 1999; Tillett et al., 2000), while others have come online more recently (domoic acid: Brunson et al., 2018) or have yet to be described. Given this lag, there is a paucity of molecular assays presently available that target toxin genes, and only recently have these assays moved into commercialization (e.g., phytoxigeneTM)10. At present, Ohio EPA has been an early adopter of the CyanoDTec kit (phytoxigeneTM) in routine monitoring of toxins associated with cyanobacteria (Ohio EPA, 2016). Routine monitoring of Microcystis in 2016 using this approach showed 100% correspondence of microcystin toxin detection above a 1.6 μg L–1 threshold with microcystin genes (mcyE; Ohio EPA, 2017).
Chemical Approaches
Commercially available strip or “dip-stick”-style toxin detection technologies have been available for some time; however, there are significant limitations to their application. While they can provide a rapid method for assessing if certain toxins of interest (e.g., microcystins, cylindrospermopsin, and anatoxin-a) are present in a sample (either water or tissue), the results are only semi-quantitative, appropriate only for initial screening, and thus of limited benefit to water managers (U.S. EPA, 2017). Newly developed technologies that are currently in beta-testing stages allow for low-cost, easy to use, rapid, single sample multiplex analysis of several HAB Toxins. One such system is the MBio Toxin System,11 which has been demonstrated to detect a wide range of freshwater and marine HAB toxins (Devlin et al., 2013; Meneely et al., 2013; McNamee et al., 2014; Reverté et al., 2017) and has the capability for expanded multiplexing, as required for detecting additional toxins. The MBio Toxin System delivers quantitative results in a small footprint and robust format that can be used in the field (Bickman et al., 2018) and can thus improve HAB monitoring, forecasting, and management by allowing for real-time, cost-effective, quantitative on-the-spot testing. By reducing the cost and time associated with making high-quality HAB toxin measurements, more tests can be performed and results can be used for real-time decision-making. Furthermore, in its current configuration, this system can detect two different HAB toxins simultaneously. For example, the MBio MC/CYN Toxin System can simultaneously detect microcystins and cylindrospermopsin using the same cartridge (Bickman et al., 2018). The first-generation MBio Toxin System has been available for commercial sale since 2018 and has been tested with 11 different MC congeners and demonstrated cross-reactivities ranging from 26 to 100% (Bickman et al., 2018).
Example results from the first generation MBio system applied in the Great Lakes as part of the 2018 ACT Technology Evaluation are shown in Figure 3. These results show concentrations of both total and dissolved microcystin, data which were all produced in the field within a few hours of sample collection. The range and limit of detection for this technology compare well to the standard bench-top ELISA procedures used in most routine freshwater monitoring applications. Moreover, the ability to generate monitoring results locally and at hourly time-scales suggests a major advancement in monitoring efficiency and application.
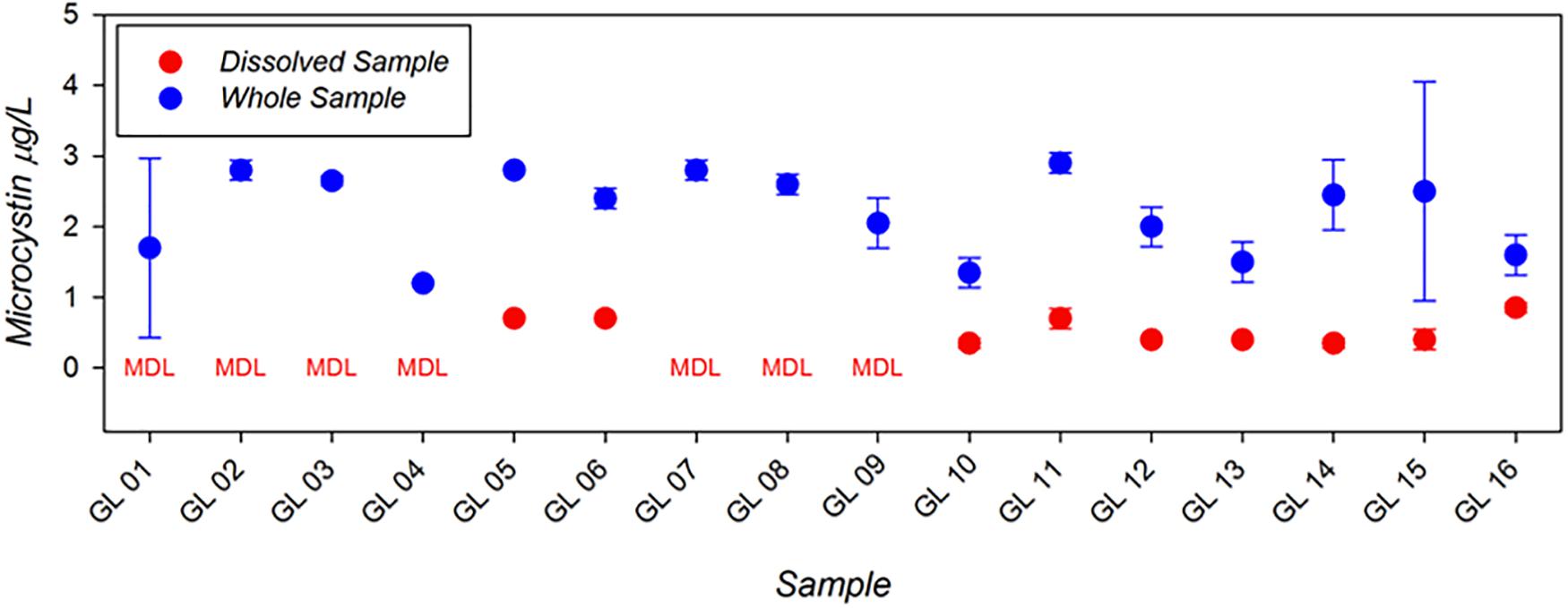
Figure 3. Microcystin analysis conducted in the Great Lakes as part of the ACT HAB Toxin detection technology evaluation using the rapid, field-portable MBio toxin detection system. Data shown represent dissolved (red) and whole water (dissolved + particulate; blue) concentrations of Microcystin (μg/L). Data below the minimum detection limit are indicated (“MDL”). Modified from ACT Demonstration Reports (www.act-us.info/evaluations.php).
In addition to the MBio instrument and kit, a second developing technology from Beacon Analytical Systems, Inc.12 was tested in the ACT Technology Evaluation. The Beacon Field Tube Kits quantify dissolved and particulate-associated phycotoxin concentrations via immunologic assay in marine or fresh water samples. These tests are designed to enable rapid, quantitative assessment of samples in the field (i.e., dock-side) without need for electricity and with minimal need for specialized equipment. ACT evaluated kits designed to measure MC and domoic acid, both of which are based on competitive ELISA techniques. Particulate-associated toxin is measured from a filtered sample which is manually crushed, while dissolved toxin can be measured in the filtered volume directly if it is present at sufficient concentration. The presence of filter particulate has no impact on assay performance. Color development is quantified using a hand-held portable spectrometer. Analyses are accompanied by known negative and positive controls, and a calibration curve can be created on-site with known certified standards or applied from previously generated curves to translate color absorbance into units of toxin concentration. Initial optimization of assay and reagent design for these kits enables the quantification of toxin concentrations over a nearly 100-fold range. The entire process of sample preparation and analysis can be accomplished in 1.5 h, enabling a timely response to the dynamic conditions of HABs.
Ongoing Challenges and Opportunities for Solutions
Many of the priorities outlined in this paper are also closely aligned to challenges associated with transferring observing technologies of any kind into the hands of a diverse group of end-users. The biological unknowns (e.g., toxin congeners and pathways, cryptic species) can hinder the development of broadly applicable test kits/sensors for species and toxins, and the construction of standards sets desired for ground-truthing measurements. Specifically, what defines a “bloom” varies widely between HAB species, and some toxin-producers may remain at low abundances. What defines a toxic “event” is largely determined by regulatory agencies concerned with food and human safety but may not be a useful metric for researchers and managers attempting to understand or mitigate HABs before they become acutely dangerous. These differences in definition and focus contribute to the intricacies of quality control (QC) and inter-calibration efforts for existing and new methodologies, thus necessitating the need for communicating known factors that influence variability in both analytical techniques and target organisms.
Weighing the various factors in choosing a sensor, instrument, or test kit can be overwhelming, as what is “good enough” for the application of the results must be decided. Such a threshold is likely to be different when, for example, making decisions to manage and minimize human exposure to toxins (for which regulatory limits exist and in which case data accuracy and precision around those limits is paramount) than when monitoring for long-term changes in an ecosystem (in which case long-term consistency, usability, and minimal cost might be priorities). Affordable instruments now on the market have expanded the use of observing systems; however long-term costs associated with maintenance and procuring proprietary reagents (in turn, subject to manufacturing delays) can still be a hurdle that is largely unshared among users (see more on this, below). In many cases (e.g., handheld qPCR technology, ESP, IFCB) a considerable amount of expertise is still required for operational purposes and data analysis (“Non-Technical Usability” column in Table 1), especially when data outputs must be rapidly transformed into digestible information useful for a wide stakeholder audience. Consensus recommendations from a community workshop assessing the state of HAB monitoring technologies (Alliance for Coastal Technologies [ACT], 2017) identified several barriers and highlighted potential opportunities for solutions to adoption of technology-based monitoring systems. These are discussed in more detail in the subsequent sections.
Stimulating, Diversifying, and Maintaining Investment
Purchase costs, the infrastructure needed for power requirements and the storage and transmission of data, and technical expertise needed to work with new technological approaches can be an enormous financial hurdle for agencies and researchers to overcome. While there is an obvious need for a standardized and integrated national network of regional HAB observing systems incorporating multiple observational technologies, realizing this need is dependent, in part, on continued investment in both autonomous, in situ HAB detection technology along with end user-accessible, highly portable formats to spatially expand HAB detection beyond autonomous regional sentinel monitoring sites. Unfortunately, it can often take a serious HAB event to finally secure buy-in from local legislators and the public for long-term funding and support, even though a pro-active versus reactive management approach would likely save money and resources. One important component missing from the HAB issue at-large is a thorough economic assessment of localized events that threaten communities (often chronically) and their natural resources. Suggestions are being made for expanding the investment base away from the classic funders of HAB research and technology development (i.e., federal agencies). These suggestions include strategically identifying and tapping into alternate sources of support/partnerships to advance sensor technologies including obvious HAB-impacted industries (e.g., aquaculture), larger market capitalization industries which rely on clean/toxin-free water (e.g., milling, brewing), and industries (e.g., cosmetics, medical) which may have more lucrative applications for technologies currently being developed for HABs.
Choosing the Right Technologies
With technological advancements, the options can become overwhelming for a researcher or manager trying to build a HAB-focused program. There is no one “right” answer for effectively monitoring and managing HABs, and a primary goal should be to deliver a system that is fit for purpose (Anderson et al., 2019). Research applications are different from regulatory applications, and technologies related to food safety, clinical test procedures, or drinking water analysis require much more rigorous quality assurance and control (QA/QC), validations and even multi-laboratory validation or comparison of “approved or official methods.” The concept of “operational” technology or models also requires further definition, as it typically connotes the ultimate of the routine, vetted, and immediately available products of the National Weather Service (NWS). In reality, however, “operational” readiness in a research or management application may mean that a technology has been verified, is being used according to a set of accepted methods, and is producing data with quantifiable quality metrics (see following section), even if it is neither as comprehensive in spatiotemporal scope nor as immediately available as the NWS connotation would suggest. Evaluation of the efficacy of new technologies require comparison to suitable reference (“Gold”) standards; however, every technology user is not able to equally access such standards, if they exist, at any given time. Employing robust, transparent, and publicly accessible technology performance verification models – such as those provided by the former U.S. EPA Environmental Technology Verification (ETV) and the current NOAA-funded ACT programs – can help overcome this barrier.
There is often a need and benefit for measurement of multiple parameter measurements (i.e., species abundance, toxin concentrations, and supporting environmental data such as chlorophyll, temperature, salinity, dissolved oxygen) that can dovetail with early warning at the species level. Continuous observations are important to integrate over large time scales and go hand-in-hand to inform the treatment and mitigation processes. Networked platforms are highly favorable, leveraging broader spatial and temporal data acquisition and Data Availability. For example, toxin-only detection systems lack the ability of an agency to track cells in the water column as they lead up to a HAB event, thus limiting efforts to predict toxic events before they happen. To achieve the goal of networking current technologies, better inter-calibration between devices and applications is needed.
Choosing the right technologies may also require coordinated use of complementary approaches, which is becoming increasingly useful in adaptive management strategies to mitigate HABs via monitoring. Increased mobility, which provides greater spatial coverage and an ability to adaptively monitor changing blooms, can overcome some of the limitations of moored platforms, which often require being manually moved to another location (including to depth). The U.S. Great Lakes Restoration Initiative (GLRI) is an example of a program using a multi-agency approach to strategically target the biggest threats to the Great Lakes ecosystem and accelerate progress toward achieving goals that include safe drinking and recreational waters and elimination of HABs13. The GLRI funds multiple projects that use complementary technologies; one such project is the Synthesis, Observation and Response (SOAR) project at NOAA Great Lakes Environmental Research Laboratory (GLERL). SOAR provides baseline real-time environmental information on Lakes Michigan, Huron, and Erie. The goals of this project are to assess water quality status and trends and ecosystem health through a variety of sampling methods, including manned aircraft systems with an HSI sensor, instrumented buoys, and shipboard sampling for environmental and bio-optical parameters. Future endeavors of the SOAR project include coordinated use of a manned aircraft system equipped with an HSI sensor flying over the flight track of an AUV equipped with an ESP-3G. These types of coordinated campaigns allow HABs to be imaged both from above and below the surface, thus providing the three-dimensional perspective of HAB events and robust evidence of their extent both spatially and with depth. Coordinated efforts extend beyond the use of autonomous systems to also include moored or deployed instrumentation as important components of networks designed to alert and forecast bloom events. Examples of such multi-asset campaigns can be found around the United States (Rowe et al., 2016; Ryan et al., 2017) as well as globally (Pinardi et al., 2008; Stumpf et al., 2010; Kaitala, 2019) and represent the intersection of research and monitoring.
To ensure that continued development of new technologies and application pipelines are “fit for purpose,” developers must also engage stakeholder input at an early stage and throughout their process (Alliance for Coastal Technologies [ACT], 2017). To further promote adoption of technological advances in monitoring, several strategies have been recommended, such as continuing efforts toward inclusion and education of stakeholders in the latest in HAB detection technologies. Reaching out to such stakeholder communities (e.g., by attending shellfish meetings), requesting HAB-focused sections or sessions at meetings, inviting representatives of industries to regional or national HAB-related meetings, and offering to accompany stakeholders in the field with new technologies are ways in which scientists and technology developers can continue to engage end users and potential investors in HAB research.
Quality Assurance and Quality Control Considerations
Quality assurance (QA) and quality control (QC) considerations for assessing performance of sensors or assays for HAB species or toxin detection do not vary substantially from general considerations for performing water quality analyses. Laboratories that contribute to monitoring biological systems should have a Quality Management System that is appropriate for their purposes (e.g., McManus et al., 2003) and/or accredited or compliant with internationally accepted quality standards, i.e., ISO/IEC 17025:2017 (International Organization for Standardization [IOS], 2017) or NELAC 2009 TNI Standards (National Environmental Laboratory Accreditation Conference Institute [NELAC], 2014). Sample collection and analysis should maintain strict adherence to a rigorous QA plan and implement QC practices that incorporate blanks (e.g., field, equipment, matrix, and sampling trip) to determine whether sample collection and handling procedures have introduced contaminants to the samples. Replicate samples (e.g., split, concurrent, and sequential) are another important element of a comprehensive sampling plan that measure variability introduced during sample collection and during short-term environmental fluctuation. QC measures for quantitative phytoplankton analysis should also include both intra-laboratory (same chamber and sample) and inter-laboratory (if need analyses are distributed across labs) calibration procedures. U.S. EPA-approved methods exist for traditional toxin analyses, including LC/MS/MS (Shoemaker et al., 2015), LC/ESI-MS/MS (U.S. EPA, 2015), and ELISA (U.S. EPA, 2016) methods. Ideally, these methods require an initial demonstration of capability and ongoing calibration checks of the laboratory instrumentation. Taken together, development of a Quality Management System, taking into consideration recommended QA/QC practices, and consistent documentation should allow for users to both document and account for any issues in their analyses, while also allowing for more informative reporting of both the quantity values of the measured analyte (i.e., HAB species or toxin concentration) in addition to a measure of uncertainty of this value.
Coordination to Overcome Bottlenecks
Given both the biological and regional diversity of HAB species and toxin profiles, it should be acknowledged that most detection technologies will likely require regional tuning rather than a “one size fits all” strategy. Image analysis of complex, non-uniform, three-dimensional organisms such as plankton can be a significant bottleneck for translating raw images collected by image-based tools (e.g., Bi et al., 2015). Combined with regional differences in dominant taxa, this bottleneck can become even more significant of a barrier. One way to address this limitation is to build and promote regional “communities of practice” that can share the burden of, for example, developing the regionally specific classification systems required to detect HAB species on the image-based technologies (e.g., Sosik and Olson, 2007). Cloud-supported computing options can help enable sharing and cross-region collaborations for building shared image classification libraries (Alliance for Coastal Technologies [ACT], 2017).
Additionally, standardizing species identifications (e.g., Moestrup et al., 2009; Rosen and St. Amand, 2015) and extraction protocols for the target toxins in regionally coherent study regions (e.g., Loftin et al., 2008; Gulf of Mexico Alliance, 2014) can help ensure that research and monitoring efforts done by different groups are consistent and comparable. Such consistency can enhance the data available for modelers and managers to use in their efforts, without the significant efforts associated with method cross-comparison.
Concluding Remarks
There are more technologies and methods available today to detect HAB events, species, and toxins in the context of ocean observing than ever before, suggesting that the “revolution in coastal oceanography, monitoring, and management” (Cullen, 2008) is underway. New and emerging technologies are building our abilities to assess HABs remotely and rapidly in the field, and to simultaneously detect species and toxins. These technologies open new doors for researching and monitoring HABs and, ultimately, build the potential for prediction and mitigation of these ecologically and economically damaging events. With new technologies, however, come challenges to maintaining investments in HAB monitoring and management, choosing the right tool or suite of tools for the purpose, ensuring data quality and translatability, and overcoming the bottlenecks that come with more spatially, temporally, and taxonomically rich data. Through diversification, integration, coordination, and collaboration, these challenges can be met, and the potential of technology advancement for HAB research and monitoring can be more fully met.
Author Contributions
BS led preparation of the work, including devising the structure of the manuscript, and coordinating the writing team. TJ and HP adapted results from the ACT Verification Reports. All authors contributed to the intellectual and material preparation of the manuscript.
Funding
This work was funded by the National Oceanic and Atmospheric Administration and the U.S. Environmental Protection Agency, through the U.S. Integrated Ocean Observing System (Award #NA16NOS012OO). Funding was awarded to Cooperative Institute for Great Lakes Research (CIGLR) through the NOAA Cooperative Agreement with the University of Michigan (Award #NA17OAR4320152). This is CIGLR contribution number 1143.
Conflict of Interest Statement
The authors declare that the research was conducted in the absence of any commercial or financial relationships that could be construed as a potential conflict of interest.
Acknowledgments
We thank the entire ACT Testing Team, our Technical Advisory Committee members, and participating technology developers/manufacturers. We also thank H. Raymond for assistance in documenting Ohio EPA rule and policy updates.
Footnotes
- ^ https://coastalscience.noaa.gov/research/stressor-impacts-mitigation/habhrca/
- ^ https://oceanservice.noaa.gov/ecoforecasting/
- ^ https://coastalscience.noaa.gov/project/national-phytoplankton-monitoring-network/
- ^ https://tidesandcurrents.noaa.gov/hab/gomx.html
- ^ https://tidesandcurrents.noaa.gov/hab/contributors
- ^ https://www.glerl.noaa.gov/res/HABs_and_Hypoxia/bulletin.html
- ^ www.glerl.noaa.gov/res/HABs_and_Hypoxia/habTracker.html
- ^ www.cencoos.org/data/models/habs
- ^ http://www.act-us.info/evaluations.php
- ^ www.phytoxigene.com/
- ^ http://mbiodx.com/
- ^ www.beaconkits.com
- ^ https://www.glri.us/
References
Ahlvik, L., and Hyytiäinen, K. (2015). Value of adaptation in water protection — Economic impacts of uncertain climate change in the Baltic Sea. Ecol. Econ. 116, 231–240. doi: 10.1016/j.ecolecon.2015.04.027
Alliance for Coastal Technologies [ACT] (2017). “Sensors for monitoring of harmful algae, cyanobacteria and their toxins. technical report series: ref. no. [UMCES]CBL 17–026,” in Proceedings of the Alliance for Coastal Technologies Workshop, (Solomons, MD).
Anderson, C. R., Berdalet, E., Kudela, R. M., Cusack, C. K., Silke, J., O’rourke, E., et al. (2019). Scaling up from regional case studies to a global harmful algal bloom observing system. Front. Mar. Sci. 6:250. doi: 10.3389/fmars.2019.00250
Anderson, C. R., Kudela, R. M., Kahru, M., Chao, Y., Rosenfeld, L. K., Bahr, F. L., et al. (2016). Initial skill assessment of the California harmful algae risk mapping (C-HARM) system. Harmful Algae 59, 1–18. doi: 10.1016/j.hal.2016.08.006
Anderson, D., Hoagland, P., Kaoru, Y., and White, A. W. (2000). Estimated Annual Economic Impacts From Harmful Algal Blooms (HABs) in the United States. Woods Hole Oceanographic Institution Technical Report: WHOI-2000–2011. Woods Hole, MA: Woods Hole Oceanogr Inst.
Anderson, D. M., Andersen, P., Bricelj, V. M., Cullen, J. J., and Rensel, J. E. (2001). “Monitoring and management strategies for harmful algal blooms in coastal waters, APEC #201-MR-01.1,” in Proceedings of the Asia Pacific Economic Program, Singapore, and Intergovernmental Oceanographic Commission Technical Series, (Paris).
Anderson, D. M., Burkholder, J. M., Cochlan, W. P., Glibert, P. M., Gobler, C. J., Heil, C. A., et al. (2008). Harmful algal blooms and eutrophication: examining linkages from selected coastal regions of the United States. Harmful Algae 8, 39–53. doi: 10.1016/j.hal.2008.08.017
Association of Public Health Laboratories [APHL] (2017). A Freshwater Algal Toxin Guidance Document for Public Health Laboratories. Available at: https://www.aphl.org/aboutAPHL/publications/Documents/EH-2017May-HAB-Toolkit.pdf
ATECH (2000). “Cost of algal blooms,” in Report to Land and Water Resources Research and Development Corporation (LWRRDC), (Canberra, ACT: Atech). Available at: http://lwa.gov.au/products/pr990308
Beckler, J., Arutunian, E., Currier, R., Milbrandt, E., and Duncan, S. (2018). Harmful algae bloom monitoring via a sustainable, sail-powered mobile platform for in-land and coastal monitoring. bioRxiv 473827.
Benfield, M. C., Grosjean, P., Culverhouse, P. F., Irigoien, X., Sieracki, M. E., Lopez-Urrutia, A., et al. (2007). RAPID: research on automated plankton identification. Oceanography 20, 172–187. doi: 10.1016/j.chroma.2018.06.027
Berdalet, E., Fleming, L. E., Gowen, R., Davidson, K., Hess, P., Backer, L. C., et al. (2015). Marine harmful algal blooms, human health and wellbeing: challenges and opportunities in the 21st century. J. Mar. Biol. Assoc. U. K. 2015:10.1017/S0025315415001733. doi: 10.1017/S0025315415001733
Bernard, S., Kudela, R., and Velo-Suárez, L. (2014). “Developing global capabilities for the observation and prediction of harmful algal blooms,” in Oceans and Society: Blue Planet, eds S. Djavidnia, V. Cheung, M. Ott, and S. Seeyave (Cambridge: Cambridge Scholars Publishing), 46–52.
Bi, H., Guo, Z., Benfield, M. C., Fan, C., Ford, M., Shahrestani, S., et al. (2015). A semi-automated image analysis procedure for in situ plankton imaging systems. PLoS One 10:e0127121. doi: 10.1371/journal.pone.0127121
Bickman, S. R., Campbell, K., Elliott, C., Murphy, C., O’Kennedy, R., Papst, P., et al. (2018). An innovative portable biosensor system for the rapid detection of freshwater cyanobacterial algal bloom toxins. Environ. Sci. Technol. 52, 11691–11698. doi: 10.1021/acs.est.8b02769
Bingham, M., Sinha, S. K., and Lupi, F. (2015). Economic Benefits of Reducing Harmful Algal Blooms in Lake Erie. Gainesville, FL: Environmental Consulting & Technology, Inc.
Botes, L., Smit, A. J., and Cook, P. A. (2003). The potential threat of algal blooms to the abalone (Haliotis midae) mariculture industry situated around the South African coast. Harmful Algae 2, 247–259. doi: 10.1016/s1568-9883(03)00044-1
Bowers, H. A., Marin, R. III, Birch, J. M., and Scholin, C. A. (2017). Sandwich hybridization probes for the detection of Pseudo-nitzschia (Bacillariophyceae) species: an update to existing probes and a description of new probes. Harmful Algae 70, 37–51. doi: 10.1016/j.hal.2017.10.005
Bowers, H. A., Marin Iii, R., Birch, J. M., Scholin, C. A., and Doucette, G. J. (2016). Recovery and identification of Pseudo-nitzschia (Bacillariophyceae) frustules from natural samples acquired using the environmental sample processor. J. Phycol. 52, 135–140. doi: 10.1111/jpy.12369
Bowers, H. A., Ryan, J. P., Hayashi, K., Woods, A. L., Marin, R., Smith, G. J., et al. (2018). Diversity and toxicity of Pseudo-nitzschia species in Monterey Bay: perspectives from targeted and adaptive sampling. Harmful Algae 78, 129–141. doi: 10.1016/j.hal.2018.08.006
Bridgeman, T. B., Chaffin, J. D., and Filbrun, J. E. (2013). A novel method for tracking western Lake Erie microcystis blooms, 2002–2011. J. Great Lakes Res. 39, 83–89. doi: 10.1016/j.jglr.2012.11.004
Brown, E. G. (2016). Office of the Governor to the US Department of Commerce. Available at: https://nrm.dfg.ca.gov/FileHandler.ashx?DocumentID=116284 (accessed March 14, 2019).
Brunson, J. K., Mckinnie, S. M. K., Chekan, J. R., Mccrow, J. P., Miles, Z. D., Bertrand, E. M., et al. (2018). Biosynthesis of the neurotoxin domoic acid in a bloom-forming diatom. Science 361, 1356–1358. doi: 10.1126/science.aau0382
Campbell, L., Olson, R. J., Sosik, H. M., Abraham, A., Henrichs, D. W., Hyatt, C. J., et al. (2010). First harmful Dinophysis (Dinphyceae, Dinophysiales) bloom in the U.S. revealed by automated imagine flow cytometry. J. Phycol. 46, 66–75. doi: 10.1111/j.1529-8817.2009.00791.x
Canfield, C. (2013). ‘Laboratory in a can’ monitors Maine red tide. South Portland, ME: Portland Press Herald.
Cembella, A., and John, U. (2006). “Molecular physiology of toxin production and growth regulation in harmful algae,” in Ecology of Harmful Algae, eds E. Granéli and J. T. Turner (Berlin: Springer), 215–227. doi: 10.1007/978-3-540-32210-8_17
Chang, F. H., Anderson, C., and Boustead, N. C. (1990). First record of a Heterosigma (Raphidophyceae) bloom with associated mortality of cage-reared salmon in Big Glory Bay, New Zealand. N. Z. J. Mar. Freshw. Res. 24, 461–469. doi: 10.1080/00288330.1990.9516437
Chase, A. P., Boss, E., Cetiniæ, I., and Slade, W. (2017). Estimation of phytoplankton accessory pigments from hyperspectral reflectance spectra: toward a global algorithm. J. Geophys. Res. Oceans 122, 9725–9743. doi: 10.1002/2017jc012859
Clark, J. M., Schaeffer, B. A., Darling, J. A., Urquhart, E. A., Johnston, J. M., Ignatius, A., et al. (2017). Satellite monitoring of cyanobacterial harmful algal bloom frequency in recreational waters and drinking source waters. Ecol. Indic. 80, 84–95. doi: 10.1016/j.ecolind.2017.04.046
Cuellar-Martinez, T., Ruiz-Fernández, A. C., Alonso-Hernández, C., Amaya-Monterrosa, O., Quintanilla, R., Carrillo-Ovalle, H. L., et al. (2018). Addressing the problem of harmful algal blooms in Latin America and the Caribbean- a regional network for early warning and response. Front. Mar. Sci. 5:409. doi: 10.3389/fmars.2018.00409
Cullen, J. (2008). “Observation and prediction of harmful algal blooms,” in Real-Time Coastal Observing Systems for Marine Ecosystem Dynamics and Harmful Algal Blooms: Theory, Instrumentation and Modelling, eds M. Babin, et al. (Paris: UNESCO), 1–41.
Culverhouse, P. F., Williams, R., Benfield, M., Flood, P. R., Sell, A. F., Mazzocchi, M. G., et al. (2006). Automatic image analysis of plankton: future perspectives. Mar. Ecol. Prog. Ser. 312, 297–309. doi: 10.3354/meps312297
Davis, T., and Gobler, C. (2010). Grazing by mesozoplankton and microzooplankton on toxic and non-toxic strains of Microcystis in the Transquaking River, a tributary of Chesapeake Bay. J. Plankt. Res. 33Google Scholar
Davis, T. W., Stumpf, R., Bullerjahn, G. S., Mckay, R. M. L., Chaffin, J. D., Bridgeman, T. B., et al. (2019). Science meets policy: a framework for determining impairment designation criteria for large waterbodies affected by cyanobacterial harmful algal blooms. Harmful Algae 81, 59–64. doi: 10.1016/j.hal.2018.11.016
Davis, T. W., Watson, S. B., Rozmarynowycz, M. J., Ciborowski, J. J. H., Mckay, R. M., Bullerjahn, G. S., et al. (2014). Phylogenies of microcystin-producing cyanobacteria in the lower Laurentian Great Lakes suggest extensive genetic connectivity. PLoS One 9:e106093. doi: 10.1371/journal.pone.0106093
Deeds, J. R., Wiles, K., Heideman, G. B. T., White, K. D., and Abraham, A. (2010). First U.S. report of shellfish harvesting closures due to confirmed okadaic acid in Texas Gulf coast oysters. Toxicon 55, 1138–1146. doi: 10.1016/j.toxicon.2010.01.003
Devlin, S., Meneely, J. P., Greer, B., Greef, C., Lochhead, M. J., and Elliott, C. T. (2013). Next generation planar waveguide detection of microcystins in freshwater and cyanobacterial extracts, utilising a novel lysis method for portable sample preparation and analysis. Anal. Chim. Acta 769, 108–113. doi: 10.1016/j.aca.2013.01.033
Dodds, W. K., Bouska, W. W., Eitzmann, J. L., Pilger, T. J., Pitts, K. L., Riley, A. J., et al. (2009). Eutrophication of U.S. Freshwaters: analysis of potential economic damages. Environ. Sci. Technol. 43, 12–19. doi: 10.1021/es801217q
Doucette, G. J., and Kudela, R. M. (2017). “Chapter Twelve In situ and real-time identification of toxins and toxin-producing microorganisms in the environment,” in Recent Advances in the Analysis of Marine Toxins, eds J. Diogène and M. Campàs (Amsterdam: Elsevier), 411–443. doi: 10.1016/bs.coac.2017.06.006
Doucette, G. J., Medlin, L. K., Mccarron, P., and Hess, P. (2018). “Detection and surveillance of harmful algal bloom species and toxins,” in Harmful Algal Blooms: A Compendium Desk Reference, eds S. E. Shumway, J. M. Burkholder, and S. L. Morton (Hoboken, NJ: John Wiley & Sons, Ltd.).
Doucette, G. J., Mikulski, C. M., Jones, K. L., King, K. L., Greenfield, D. I., Marin, R., et al. (2009). Remote, subsurface detection of the algal toxin domoic acid onboard the environmental sample processor: assay development and field trials. Harmful Algae 8, 880–888. doi: 10.1016/j.hal.2009.04.006
Elms, S. (2019). Big Renovations Continue at Toledo’s Water Treatment Plant. Available at: https://www.toledoblade.com/local/city/2019/05/08/big-renovations-continue-at-toledo-collins-park-water-treatment-plant/stories/20190508014 (accessed October 9, 2019)
Friedman, M. A., Fleming, L. E., Fernandez, M., Bienfang, P., Schrank, K., Dickey, R., et al. (2008). Ciguatera fish poisoning: treatment, prevention and management. Mar. Drugs 6, 456–479. doi: 10.3390/md20080022
Frolov, S., Kudela, R. M., and Bellingham, J. G. (2013). Monitoring of harmful algal blooms in the era of diminishing resources: a case study of the U.S. West Coast. Harmful Algae 2, 1–12. doi: 10.1016/j.hal.2012.11.001
Fu, F.-X., Place, A. R., Garcia, N. S., and Hutchins, D. A. (2010). CO2 and phosphate availability control the toxicity of the harmful bloom dinoflagellate Karlodinium veneficum. Aquat. Microb. Ecol. 59, 55–65. doi: 10.3354/ame01396
Garrido, J. L., and Roy, S. (2015). The use of HPLC for the characterization of phytoplankton pigments. Methods Mol. Biol. 1308, 241–252. doi: 10.1007/978-1-4939-2684-8_15
Gibble, C. M., and Kudela, R. M. (2014). Detection of persistent microcystin toxins at the land–sea interface in Monterey Bay, California. Harmful Algae 39, 146–153. doi: 10.1016/j.hal.2014.07.004
Gill, D., Rowe, M., and Joshi, S. J. (2018). Fishing in greener waters: understanding the impact of harmful algal blooms on Lake Erie anglers and the potential for adoption of a forecast model. J. Environ. Manag. 227, 248–255. doi: 10.1016/j.jenvman.2018.08.074
Glibert, P. M., Alexander, J., Meritt, D. W., North, E. W., and Stoecker, D. K. (2007). Harmful algae pose additional challenges for oyster restoration: impacts of the harmful algae Karlodinium veneficum and Prorocentrum minimum on early life stages of the oysters Crassostrea virginica and Crassostrea ariakensis. J. Shellfish Res. 26, 919–925. doi: 10.2983/0730-8000(2007)26
Glibert, P. M., Allen, J. I., Bouwman, A. F., Brown, C. W., Flynn, K. J., Lewitus, A. J., et al. (2010). Modeling of HABs and eutrophication: status, advances, challenges. J. Mar. Syst. 83, 262–275. doi: 10.1016/j.jmarsys.2010.05.004
Glibert, P. M., Pitcher, G. C., Bernard, S., and Li, M. (2018). “Advancements and continuing challenges of emerging technologies and tools for detecting harmful algal blooms, their antecedent conditions and toxins, and applications in predictive models,” in Global Ecology and Oceanography of Harmful Algal Blooms. Ecological Studies (Analysis and Synthesis), eds P. Glibert, E. Berdalet, M. Burford, G. Pitcher, and M. Zhou (Cham: Springer).
Gobler, C. J., Lonsdale, D. J., and Boyer, G. L. (2005). A review of the causes, effects, and potential management of harmful brown tide blooms caused by Aureococcus anophagefferens (Hargraves et Sieburth). Estuaries 28, 726–749. doi: 10.1007/bf02732911
Granéli, E., and Flynn, K. (2006). “Chemical and physical factors influencing toxin content,” in Ecology of Harmful Algae. Ecological Studies (Analysis and Synthesis), eds E. Granéli and J. T. Turner (Berlin: Springer).
Greenfield, D. I., Marin Iii, R., Doucette, G. J., Mikulski, C., Jones, K., Jensen, S., et al. (2008). Field applications of the second-generation environmental sample processor (ESP) for remote detection of harmful algae: 2006-2007. Limnol. Oceanogr. 6, 667–679. doi: 10.4319/lom.2008.6.667
Gregor, J., and Marsalek, B. (2004). Freshwater phytoplankton quantification by chlorophyll a: a comparative study of in vitro, in vivo and in situ methods. Water Res. 38, 517–522. doi: 10.1016/j.watres.2003.10.033
Gulf of Mexico Alliance (2014). Resource Guide for Harmful Algal Bloom Toxin Sampling and Analysis. Ocean Springs, MS: Gulf of Mexico Alliance.
Gull, C., Minkov, M. T., Pereira, E., and Miranda Nacif, J. A. (2016). “A low-cost chlorophyll fluorescence sensor system,” in Proceedings of the VI Brazilian Symposium on Computing Systems Engineering (SBESC), (Joao Pessoa), 186–191.
Hails, A., Boyes, C., Boyes, A., Currier, R. D., Henderson, K., Kotlewski, A., et al. (2009). “The optical phytoplankton discriminator,” in Proceedings of the OCEANS 2009, (Biloxi, MS), 1–4.
Hallegraeff, G. M. (2010). Ocean climate change, phytoplankton community responses, and harmful algal blooms: a formidable predictive challenge. J. Phycol. 46, 220–235. doi: 10.1111/j.1529-8817.2010.00815.x
Harada, K.-I., Kondo, F., and Lawton, L. (1999). “Chapter 13. Laboratory analysis of cyanotoxins,” in Toxic Cyanobacteria in Water: A guide to their public health consequences, monitoring and management, eds I. Chorus and J. Bartram (Boca Raton, FL: CRC Press).
Harred, L. B., and Campbell, L. (2014). Predicting harmful algal blooms: a case study with Dinophysis ovum in the Gulf of Mexico. J. Plankt. Res. 36, 1434–1445. doi: 10.1093/plankt/fbu070
Heisler, J., Glibert, P. M., Burkholder, J. M., Anderson, D. M., Cochlan, W., Dennison, W. C., et al. (2008). Eutrophication and harmful algal blooms: a scientific consensus. Harmful Algae 8, 3–13. doi: 10.1016/j.hal.2008.08.006
Ho, J. C., and Michalak, A. M. (2015). Challenges in tracking harmful algal blooms: a synthesis of evidence from Lake Erie. J. Great Lakes Res. 41, 317–325. doi: 10.1016/j.jglr.2015.01.001
International Joint Commission (2014). A Balanced Diet for Lake Erie: Reducing Phosphorus Loadings and Harmful Algal Blooms. Washington, DC: International Joint Commission.
International Organization for Standardization [IOS] (2017). General Requirements for the Competence of Testing and Calibration Laboratories (Standard ISO/IEC 17025: 2017). Geneva: IOS.
IOC Intergovernmental Panel on Harmful Algal Blooms [IOC-IPHAB] (2009). IOC-IPHAB Recommended Procedures for Automated and Semi-Automated HAB-Monitoring and Forecasting within the Global Ocean Observing System. Paris: Intergovernmental Commission of UNESCO.
Jessup, D. A., Miller, M. A., Ryan, J. P., Nevins, H. M., Kerkering, H. A., Mekebri, A., et al. (2009). Mass stranding of marine birds caused by a surfactant-producing red tide. PLoS One 4:e4550. doi: 10.1371/journal.pone.0004550
Kaitala, S. (2019). Development of the Operational Observation System of Harmful Algal Blooms as a BOOS Project. Copenhagen: ICES.
Kaushik, R., and Balasubramanian, R. (2013). Methods and approaches used for detection of cyanotoxins in environmental samples: a review. Crit. Rev. Environ. Sci. Technol. 43, 1349–1383. doi: 10.1021/acssensors.9b00376
King, T., Claassen, L., Borchert, J., and Trainer, V. (2018). “Soundtoxins: a puget sound harmful algae monitoring partnership,” in Proceedings of the Salish Sea Ecosystem Conference, (Seattle, WA).
Kirkpatrick, G. J., Millie, D. F., Moline, M. A., Lohrenz, S. E., and Schofield, O. M. (2011). “Automated, in-water determination of colored dissolved organic material and phytoplankton community structure using the optical phytoplankton discriminator,” in Proceedings of the SPIE 8030, Ocean Sensing and Monitoring III, 80300E, (Orlando, FL).
Kirkpatrick, G. J., Millie, D. F., Moline, M. A., and Schofield, O. (2000). Optical discrimination of a phytoplankton species in natural mixed populations. Limnol. Oceanogr. 45, 467–471. doi: 10.4319/lo.2000.45.2.0467
Kouzminov, A., Ruck, J., and Wood, S. A. (2007). New Zealand risk management approach for toxic cyanobacteria in drinking water. Aust. N. Z. J. Public Health 31, 275–281. doi: 10.1111/j.1467-842x.2007.00061.x
Kramer, B. J., Davis, T. W., Meyer, K. A., Rosen, B. H., Goleski, J. A., Dick, G. J., et al. (2018). Nitrogen limitation, toxin synthesis potential, and toxicity of cyanobacterial populations in Lake Okeechobee and the St. Lucie River Estuary, Florida, during the 2016 state of emergency event. PLoS One 13:e0196278. doi: 10.1371/journal.pone.0196278
Kudela, R. M. (2011). Characterization and deployment of solid phase adsorption toxin tracking (SPATT) resin for monitoring of microcystins in fresh and saltwater. Harmful Algae 11, 117–125. doi: 10.1016/j.hal.2011.08.006
Kudela, R. M., Berdalet, E., Bernard, S., Burford, M., Fernand, L., Lu, S., et al. (2015a). Harmful Algal Blooms. A Scientific Summary for Policy Makers. Paris: IOC/UNESCO.
Kudela, R. M., Palacios, S. L., Austerberry, D. C., Accorsi, E. K., Guild, L. S., Torres-Perez, J., et al. (2015b). Application of hyperspectral remote sensing to cyanobacterial blooms in inland waters. Remote Sens. Environ. 167, 196–205. doi: 10.1016/j.rse.2015.01.025
Lake Erie Lakewide Action, and Management Plan Work Group [LAMP]. (2011). Lake Erie Binational Nutrient Management Strategy: Protecting Lake Erie by Managing Phosphorus. Washington, DC: Lake Erie LAMP Management Committee.
Lefebvre, K. A., Elder, N. E., Hershberger, P. K., Trainer, V. L., Stehr, C. M., and Scholz, N. L. (2005). Dissolved saxitoxin causes transient inhibition of sensorimotor function in larval Pacific herring (Clupea harengus pallasi). Mar. Biol. 147, 1393–1402. doi: 10.1007/s00227-005-0048-8
Lefebvre, K. A., Quakenbush, L., Frame, E., Huntington, K. B., Sheffield, G., Stimmelmayr, R., et al. (2016). Prevalence of algal toxins in Alaskan marine mammals foraging in a changing arctic and subarctic environment. Harmful Algae 55, 13–24. doi: 10.1016/j.hal.2016.01.007
Liefer, J. D., Robertson, A., Macintyre, H. L., Smith, W. L., and Dorsey, C. P. (2013). Characterization of a toxic Pseudo-nitzschia spp. bloom in the Northern Gulf of Mexico associated with domoic acid accumulation in fish. Harmful Algae 26, 20–32. doi: 10.1016/j.hal.2013.03.002
Litaker, R. W., Vandersea, M. W., Faust, M. A., Kibler, S. R., Nau, A. W., Holland, W. C., et al. (2010). Global distribution of ciguatera causing dinoflagellates in the genus Gambierdiscus. Toxicon 56, 711–730. doi: 10.1016/j.toxicon.2010.05.017
Loftin, K. A., Meyer, M. T., Rubio, F., Kamp, L., Humphries, E., and Whereat, E. (2008). Comparison of Two Cell Lysis Procedures for Recovery of Microcystins in Water Samples from Silver Lake in Dover, Delaware, with Microcystin Producing Cyanobacterial Accumulations. Reston, VA: U.S. Geological Survey.
Lombard, F., Boss, E., Waite, A. M., Vogt, M., Uitz, J., Stemmann, L., et al. (2019). Globally consistent quantitative observations of planktonic ecosystems. Front. Mar. Sci. 6:196. doi: 10.3389/fmars.2019.00196
Luo, J. Y., Irisson, J.-O., Graham, B., Guigand, C., Sarafraz, A., Mader, C., et al. (2018). Automated plankton image analysis using convolutional neural networks. Limnol. Oceanogr. Methods 16, 814–827. doi: 10.1002/lom3.10285
Ma, J., Qin, B., Paerl, H. W., Brookes, J. D., Hall, N. S., Shi, K., et al. (2016). The persistence of cyanobacterial (Microcystis spp.) blooms throughout winter in Lake Taihu, China. Limnol. Oceanogr. 61, 711–722. doi: 10.1002/lno.10246
Macauley, M. K. (2006). The value of information: measuring the contribution of space-derived earth science data to resource management. Space Policy 22, 274–282. doi: 10.1016/j.spacepol.2006.08.003
Mackey, M. D., Mackey, D. J., Higgins, H. W., and Wright, S. W. (1996). CHEMTAX - A program for estimating class abundances from chemical markers: application to HPLC measurements of phytoplankton. Mar. Ecol. Prog. Ser. 144, 265–283. doi: 10.3354/meps144265
McCabe, R. M., Hickey, B. M., Kudela, R. M., Lefebvre, K. A., Adams, N. G., Bill, B. D., et al. (2016). An unprecedented coastwide toxic algal bloom linked to anomalous ocean conditions. Geophys. Res. Lett. 43, 366–310.
McGuire, P. (2018). In New Cautionary Approach, Maine Shellfish Areas will be Closed at First Sign of Toxins. South Portland, ME: Portland Press Herald.
McManus, M., Largier, J., Palomino, E., Wilkinson, L., and Washburn, L. (2003). Data Management Techniques for NEOCO. The Network for Environmental Observations of the Coastal Ocean. Sea Technology. Paris: UNESCO.
McManus, M. A., Hochberg, E. J., Joyce, K., Palacios, P. L., Vander Woude, A., and Schar, D. (2018). Alliance for Coastal Technologies Workshop Proceedings: Hyperspectral Imaging of Coastal Waters. Honolulu, HI: University of Hawaii at Manoa.
McManus, M. A., Kudela, R. M., Silver, M. W., Steward, G. F., Donaghay, P. L., and Sullivan, J. M. (2008). Cryptic blooms: are thin layers the missing connection? Estuar. Coasts 31, 396–401. doi: 10.1007/s12237-007-9025-4
McNamee, S. E., Elliott, C. T., Greer, B., Lochhead, M., and Campbell, K. (2014). Development of a planar waveguide microarray for the monitoring and early detection of five harmful algal toxins in water and cultures. Environ. Sci. Technol. 48, 13340–13349. doi: 10.1021/es504172j
Medlin, L. K., and Orozco, J. (2017). Molecular techniques for the detection of organisms in aquatic environments, with emphasis on harmful algal bloom species. Sensors 17:1184. doi: 10.3390/s17051184
Meneely, J. P., Campbell, K., Greef, C., Lochhead, M. J., and Elliott, C. T. (2013). Development and validation of an ultrasensitive fluorescence planar waveguide biosensor for the detection of paralytic shellfish toxins in marine algae. Biosens. Bioelectron. 41, 691–697. doi: 10.1016/j.bios.2012.09.043
Michalak, A. M., Anderson, E. J., Beletsky, D., Boland, S., Bosch, N. S., Bridgeman, T. B., et al. (2013). Record-setting algal bloom in Lake Erie caused by agricultural and meteorological trends consistent with expected future conditions. Proc. Natl. Acad. Sci. U.S.A. 110, 6448–6452. doi: 10.1073/pnas.1216006110
Miller, M. A., Kudela, R. M., Mekebri, A., Crane, D., Oates, S. C., Tinker, M. T., et al. (2010). Evidence for a novel marine harmful algal bloom: Cyanotoxin (Microcystin) transfer from Land to Sea Otters. PLoS One 5:e12576. doi: 10.1371/journal.pone.0012576
Moestrup, Ø., Akselmann, R., Fraga, S., Hoppenrath, M., Iwataki, M., Komárek, J., et al. (2009). IOC-UNESCO Taxonomic Reference List of Harmful Micro Algae. Available at http://www.marinespecies.org/hab (accessed May 18, 2018)
Mouw, C. B., Barnett, A., Mckinley, G. A., Gloege, L., and Pilcher, D. (2016). Phytoplankton size impact on export flux in the global ocean. Glob. Biogeochem. Cycles 30, 1542–1562. doi: 10.1002/2015gb005355
Mouw, C. B., Hardman-Mountford, N. J., Alvain, S., Bracher, A., Brewin, R. J. W., Bricaud, A., et al. (2017). A consumer’s guide to satellite remote sensing of multiple phytoplankton groups in the Global Ocean. Front. Mar. Sci. 4:41. doi: 10.3389/fmars.2017.00041
National Environmental Laboratory Accreditation Conference Institute [NELAC] (2014). General Requirements for Field Sampling and Measurement Organizations (TNI NEFAP Standard FSMO-V1). Weatherford, TX: NELAC.
Nishizawa, T., Asayama, M., Fujii, K., Harada, K., and Shirai, M. (1999). Genetic analysis of the peptide synthetase genes for a cyclic heptapeptide microcystin in Microcystis spp. J. Biochem. 126, 520–529. doi: 10.1093/oxfordjournals.jbchem.a022481
NOAA (2015). A Strategic Vision for NOAA’s Ecological Forecasting Roadmap, 2015-2019. Silver Spring, MD: National Oceanic and Atmospheric Administration.
Obenour, D. R., Gronewold, A. D., Stow, C. A., and Scavia, D. (2014). Using a Bayesian hierarchical model to improve Lake Erie cyanobacteria bloom forecasts. Water Resourc. Res. 50, 7847–7860. doi: 10.1002/2014wr015616
Ohio EPA (2016). Quantitative Polymerase Chain Reaction (qPCR) for Determination of Cyanobacterial and Cyanotoxin-Producing Genes. Columbus, OH: Ohio EPA.
Ohio EPA (2019a). Developing a Harmful Algal Bloom (HAB) Treatment Optimization Protocol – Guidance for Public Water Systems. DRAFT, Version 2.1. Columbus, OH: Ohio EPA.
Ohio EPA (2019b). Guidance For Public Water Systems – Developing a Harmful Algal Bloom (HAB) General Plan. Version 2.0. Columbus, OH: Ohio EPA.
O’Neil, J. M., Davis, T. W., Burford, M. A., and Gobler, C. J. (2012). The rise of harmful cyanobacteria blooms: the potential roles of eutrophication and climate change. Harmful Algae 14, 313–334. doi: 10.1016/j.hal.2011.10.027
Otten, T. G., and Paerl, H. W. (2016). “Best practices for cyanobacterial harmful algal bloom monitoring,” in Manual of Environmental Microbiology, 4th Edn, (Washington, WA: ASM Press).
Ottesen, E. A. (2016). Probing the living ocean with ecogenomic sensors. Curr. Opin. Microbiol. 31, 132–139. doi: 10.1016/j.mib.2016.03.012
Paerl, H. W., Gardner, W. S., Havens, K. E., Joyner, A. R., Mccarthy, M. J., Newell, S. E., et al. (2016). Mitigating cyanobacterial harmful algal blooms in aquatic ecosystems impacted by climate change and anthropogenic nutrients. Harmful Algae 54, 213–222. doi: 10.1016/j.hal.2015.09.009
Paerl, H. W., and Huisman, J. (2008). Blooms like it hot. Science 320, 57–58. doi: 10.1126/science.1155398
Palacios, S. L., Kudela, R. M., Guild, L. S., Negrey, K. H., Torres-Perez, J., Broughton, J., et al. (2015). Remote sensing of phytoplankton functional types in the coastal ocean from the HyspIRI Preparatory Flight Campaign. Remote Sens. Environ. 167, 269–280. doi: 10.1016/j.rse.2015.05.014
Pargett, D. M., Birch, J. M., Preston, C. M., Ryan, J. P., Zhang, Y., and Scholin, C. A. (2015). “Development of a mobile ecogenomic sensor,” in Proceedings of the OCEANS 2015 - MTS/IEEE Washington, (Washington, DC).
Pierce, R. H., and Kirkpatrick, G. J. (2001). Innovative techniques for harmful algal toxin analysis. Environ. Toxicol. Chem. 20, 107–114. doi: 10.1002/etc.5620200110
Pinardi, N., Fratianni, C., and Adani, M. (2008). “Use of real-time observations in an operational ocean data assimilation system: the Mediterranean case,” in Real-time Coastal Observing Systems for Marine Ecosystem Dynamics and Harmful Algal Blooms: Theory, Instrumentation and Modelling, eds M. Babin, C. S. Roesler, and J. J. Cullen (Paris: UNESCO).
Pretty, J. N., Mason, C. F., Nedwell, D. B., Hine, R. E., Leaf, S., Dils, R., et al. (2003). Environmental costs of freshwater eutrophication in England and Wales. Environ. Sci. Technol. 37, 201–208. doi: 10.1021/es020793k
Proctor, C. W., and Roesler, C. S. (2010). New insights on obtaining phytoplankton concentration and composition from in situ multispectral chlorophyll fluorescence. Limnol. Oceanogr. Methods 8, 695–708. doi: 10.4319/lom.2010.8.0695
Puddick, J., Thomson-Laing, G., and Wood, S. A. (2019). Microcystins in New Zealand: a review of occurrence, congener diversity and cell quotas. N. Z. J. Bot. 59, 93–111. doi: 10.1080/0028825x.2019.1573742
Rabalais, N. N., Turner, R. E., Diaz, R. J., and Justic, D. (2009). Global change and eutrophication of coastal waters. ICES J. Mar. Sci. 66, 1528–1537. doi: 10.1093/icesjms/fsp047
Reguera, B., Alonso, R., Moreira, A., Méndez, S., and Dechraoui-Bottein, M.-Y. (2016). Guide for Designing and Implementing a Plan to Monitor Toxin-Producing Micro-Algae. Paris: Unesco & IAEA.
Reguera, B., Riobó, P., Rodríguez, F., Díaz, P. A., Pizarro, G., Paz, B., et al. (2014). Dinophysis toxins: causative organisms, distribution and fate in shellfish. Mar. Drugs 12, 394–461. doi: 10.3390/md12010394
Reverté, L., Campàs, M., Yakes, B. J., Deeds, J. R., Katikou, P., Kawatsu, K., et al. (2017). Tetrodotoxin detection in puffer fish by a sensitive planar waveguide immunosensor. Sensors a Actuat. B Chem. 253, 967–976. doi: 10.1016/j.snb.2017.06.181
Robbins, I. C., Kirkpatrick, G. J., Blackwell, S. M., Hillier, J., Knight, C. A., Moline, M. A., et al. (2006). Improved monitoring of HABs using autonomous underwater vehicles (AUV). Harmful Algae 5, 749–761. doi: 10.1016/j.hal.2006.03.005
Rosen, B. H., and St. Amand, A. (2015). Field and Laboratory Guide to Freshwater Cyanobacteria Harmful Algal Blooms for Native American and Alaska Native communities. Reston, VA: U.S. Geological Survey.
Rountos, K. J., Tang, Y.-Z., Cerrato, R. M., Gobler, C. J., and Pikitch, E. K. (2014). Toxicity of the harmful dinoflagellate Cochlodinium polykrikoides to early life stages of three estuarine forage fish. Mar. Ecol. Prog. Ser. 505, 81–94. doi: 10.3354/meps10793
Rowe, M. D., Anderson, E. J., Wynne, T. T., Stumpf, R. P., Fanslow, D. L., Kijanka, K., et al. (2016). Vertical distribution of buoyant Microcystis blooms in a Lagrangian particle tracking model for short-term forecasts in Lake Erie. J. Geophys. Res. Oceans 121, 5296–5314. doi: 10.1002/2016jc011720
Ryan, J. P., Gower, J. F. R., King, S. A., Bissett, W. P., Fischer, A. M., Kudela, R. M., et al. (2008). A coastal ocean extreme bloom incubator. Geophys. Res. Lett. 35, L12602.
Ryan, J. P., Kudela, R. M., Birch, J. M., Blum, M., Bowers, H. A., Chavez, F. P., et al. (2017). Causality of an extreme harmful algal bloom in Monterey Bay, California, during the 2014–2016 northeast Pacific warm anomaly. Geophys. Res. Lett. 44, 5571–5579. doi: 10.1002/2017gl072637
Sanseverino, I., António, D. C., Loos, R., and Lettieri, T. (2017). “Cyanotoxins: Methods and Approaches for their Analysis and Detection”. Technical Report: EUR 28624. Luxembourg: European Union.
Sanseverino, I., Conduto, D., Pozzoli, L., Dobricic, S., and Lettieri, T. (2016). “Algal Bloom and its Economic Impact”, in: EUR 27905 EN. Luxembourg: European Union.
Schofield, O., Bosch, J., Glenn, S., Kirkpatrick, G., Kerfoot, J., Lohrenz, S., et al. (2008). “Bio-optics in integrated ocean observing networks: potential for studying harmful algal blooms,” in Real Time Coastal Observing Systems for Ecosystem Dynamics and Harmful Algal Blooms, eds M. Babin, C. S. Roesler, and J. J. Cullen (Paris: UNESCO).
Schofield, O., Kerfoot, J., Mahoney, K., Moline, M., Oliver, M., Lohrenz, S., et al. (2006). Vertical migration of the toxic dinoflagellate Karenia brevis and the impact on ocean optical properties. J. Geophys. Res. Oceans 111:C06009.
Scholin, C. (1998). “The development and application of molecular probes and novel instrumentation for detection of harmful algae,” in Proceedings of the Ocean Community Conference ’98, (Washington, DC).
Scholin, C., Massion, E. I., Wright, D. K., Cline, D. E., Mellinger, E., and Brown, M. (2001). Aquatic autosampler device. US Patent No. 6187530. Washington, DC: U.S. Patent and Trademark Office.
Scholin, C. A., Gulland, F., Doucette, G. J., Benson, S., Busman, M., Chavez, F. P., et al. (2000). Mortality of sea lions along the central California coast linked to a toxic diatom bloom. Nature 403, 80–84. doi: 10.1038/47481
Seegers, B. N., Birch, J. M., Marin, R., Scholin, C. A., Caron, D. A., Seubert, E. L., et al. (2015). Subsurface seeding of surface harmful algal blooms observed through the integration of autonomous gliders, moored environmental sample processors, and satellite remote sensing in southern California. Limnol. Oceanogr. 60, 754–764. doi: 10.1002/lno.10082
Shapiro, J., Dixon, L. K., Schofield, O. M., Kirkpatrick, B., and Kirkpatrick, G. J. (2015). “ New sensors for ocean observing: the optical phytoplankton discriminator,” in Coastal Ocean Observing Systems, eds Y. Liu, H. Kerkering, and R. H. Weisberg (Boston: Academic Press), 326–350. doi: 10.1016/b978-0-12-802022-7.00018-3
Shoemaker, J., Tettenhorst, D., and Delacruz, A. (2015). Method 544. Determination of Microcystins and Nodularin in Drinking Water by Solid Phase Extraction and Liquid Chromatography/Tandem Mass Spectrometry (lc/MS/MS). Washington, DC: U.S. EPA.
Siegel, D. A., Behrenfeld, M. J., Maritorena, S., McClain, C. R., Antoine, D., Bailey, S. W., et al. (2013). Regional to global assessments of phytoplankton dynamics from the SeaWiFS mission. Remote Sens. Environ. 135, 77–91. doi: 10.1016/j.rse.2013.03.025
Smayda, T. J. (1997). What is a bloom? A commentary. Limnol. Oceanogr. 42, 1132–1136. doi: 10.4319/lo.1997.42.5_part_2.1132
Sosik, H. M., and Olson, R. J. (2007). Automated taxonomic classification of phytoplankton sampled with imaging-in-flow cytometry. Limnol. Oceanogr. Methods 5, 204–216. doi: 10.4319/lom.2007.5.204
Stauffer, B. A., Gellene, A. G., Rico, D., Sur, C., and Caron, D. A. (2017). Grazing of the heterotrophic dinoflagellate Noctiluca scintillans on dinoflagellate and raphidophyte prey. Aquat. Microb. Ecol. 80, 193–207. doi: 10.3354/ame01849
Steffen, M. M., Davis, T. W., Mckay, R. M. L., Bullerjahn, G. S., Krausfeldt, L. E., Stough, J. M. A., et al. (2017). Ecophysiological Examination of the Lake Erie Microcystis bloom in 2014: linkages between biology and the water supply shutdown of Toledo, OH. Environ. Sci. Technol. 51, 6745–6755. doi: 10.1021/acs.est.7b00856
Steffensen, D. A. (2008). Economic cost of cyanobacterial blooms. Adv. Exp. Med. Biol. 619, 855–865. doi: 10.1007/978-0-387-75865-7_37
Stumpf, R., Fleming, V., and Granéli, E. (2010). “Integration of data for nowcasting of harmful algal blooms,” in Proceedings of the OceanObs’09: Sustained Ocean Observations and Information for Society, (Venice), 1–11.
Stumpf, R. P., Culver, M. E., Tester, P. A., Tomlinson, M., Kirkpatrick, G. J., Pederson, B. A., et al. (2003). Monitoring Karenia brevis blooms in the Gulf of Mexico using satellite ocean color imagery and other data. Harmful Algae 2, 147–160. doi: 10.1016/s1568-9883(02)00083-5
Stumpf, R. P., Davis, T. W., Wynne, T. T., Graham, J. L., Loftin, K. A., Johengen, T. H., et al. (2016). Challenges for mapping cyanotoxin patterns from remote sensing of cyanobacteria. Harmful Algae 54, 160–173. doi: 10.1016/j.hal.2016.01.005
Stumpf, R. P., Wynne, T. T., Baker, D. B., and Fahnenstiel, G. L. (2012). Interannual Variability of cyanobacterial blooms in Lake Erie. PLoS One 7:e42444. doi: 10.1371/journal.pone.0042444
Tatters, A. O., Fu, F. X., and Hutchins, D. A. (2012). High CO2 and silicate limitation synergistically increase the toxicity of Pseudo-nitzshia fraudulenta. PLoS One 7:e32116. doi: 10.1371/journal.pone.0032116
Tillett, D., Dittmann, E., Erhard, M., Von Dohren, H., Borner, T., and Neilan, B. A. (2000). Structural organization of microcystin biosynthesis in Microcystis aeruginosa PCC7806: an integrated peptide-polyketide synthetase system. Chem. Biol. 7, 753–764. doi: 10.1016/s1074-5521(00)00021-1
Todd, E. C. D. (1993). “Estimated costs of paralytic shellfish, diarrhetic shellfish and ciguatera poisoning in Canada (English),” in Proceedings of the 6th International Conference on Toxic Marine Phytoplankton, Harmful Marine Algal Blooms, (Paris: Intercept Ltd.), 831–834.
Trainer, V. L., Cochlan, W. P., Erickson, A., Bill, B. D., Cox, F. H., Borchert, J. A., et al. (2007). Recent domoic acid closures of shellfish harvest areas in Washington State inland waterways. Harmful Algae 6, 449–459. doi: 10.1016/j.hal.2006.12.001
Trainer, V. L., and Hardy, F. J. (2015). Integrative monitoring of marine and freshwater harmful algae in Washington State for public health protection. Toxins 7, 1206–1234. doi: 10.3390/toxins7041206
Trainer, V. L., Moore, S. K., Hallegraeff, G., Kudela, R. M., Clement, A., Mardones, J. I., et al. (2019). Pelagic harmful algal blooms and climate change: lessons from nature’s experiments with extremes. Harmful Algae. (in press). doi: 10.1016/j.hal.2019.03.009
Turner, J. T. (2010). Zooplankton community grazing impact on a bloom of Alexandrium fundyense in the Gulf of Maine. Harmful Algae 9, 578–589. doi: 10.1016/j.hal.2010.04.008
Turner, J. T., and Granéli, E. (2006). ““Top-down” predation control on marine harmful algae,” in Ecology of Harmful Algae, eds E. Granéli and J. T. Turner (Berlin: Springer-Verlag), 355–366. doi: 10.1007/978-3-540-32210-8_27
U. S. Department of Agriculture (2005). Western Lake Erie Basin Water Resources Protection Plan. Washington DC: USDA.
U.S. EPA (2015). Method 545: Determination of Cylindrospermopsin and Anatoxin-a in Drinking Water by Liquid Chromatography Electrospray Ionization Tandem Mass Spectrometry (LC/ESI-MS/MS). Washington, DC: U.S. EPA.
U.S. EPA (2016). Method 546: Determination of Total Microcystins and Nodularins in Drinking Water and Ambient Water by Adda Enzyme-Linked Immunosorbent Assay. Washington, DC: U.S. EPA.
U.S. EPA (2017). Recommendations for Cyanobacteria and Cyanotoxin Monitoring in Recreational Waters. Washington, DC: U.S. EPA Office of Water.
Vander Woude, A., Ruberg, S., Johengen, T., Miller, R., and Stuart, D. (2019). Spatial and temporal scales of variability of cyanobacteria harmful algal blooms from NOAA GLERL airborne hyperspectral imagery. J. Great Lakes Res. 45, 536–546. doi: 10.1016/j.jglr.2019.02.006
Visser, P. M., Verspagen, J. M. H., Sandrini, G., Stal, L. J., Matthijs, H. C. P., Davis, T. W., et al. (2016). How rising CO2 and global warming may stimulate harmful cyanobacterial blooms. Harmful Algae 54, 145–159. doi: 10.1016/j.hal.2015.12.006
Weisberg, R. H., Zheng, L., Liu, Y., Corcoran, A. A., Lembke, C., Hu, C., et al. (2016). Karenia brevis blooms on the West Florida Shelf: a comparative study of the robust 2012 bloom and the nearly null 2013 event. Cont. Shelf Res. 120, 106–121. doi: 10.1016/j.csr.2016.03.011
Weissbach, A., Rudstrom, M., Olofsson, M., Bechemin, C., Icely, J., Newton, A., et al. (2011). Phytoplankton allelochemical interactions change microbial food web dynamics. Limnol. Oceanogr. 56, 899–909. doi: 10.4319/lo.2011.56.3.0899
Weller, M. G. (2013). Immunoassays and biosensors for the detection of cyanobacterial toxins in water. Sensors 13, 15085–15112. doi: 10.3390/s131115085
Wu, D., Li, R., Zhang, F., and Liu, J. (2019). A review on drone-based harmful algae blooms monitoring. Environ. Monitor. Assess. 191:211. doi: 10.1007/s10661-019-7365-8
Yamahara, K. M., Demir-Hilton, E., Preston, C. M., Marin, R. III, Pargett, D., Roman, B., et al. (2015). Simultaneous monitoring of faecal indicators and harmful algae using an in-situ autonomous sensor. Lett. Appl. Microbiol. 61, 130–138. doi: 10.1111/lam.12432
Zapata, M. (2005). Recent advances in pigment analysis as applied to picophytoplankton. Vie et Milieu 55, 233–248.
Zeng, L., and Li, D. (2015). Development of in situ sensors for chlorophyll concentration measurement. J. Sensors 2015:16.
Zhang, C., and Zhang, J. (2015). Current techniques for detecting and monitoring algal toxins and causative harmful algal blooms. J. Environ. Anal. Chem. 2:123. doi: 10.4172/2380-2391.1000123
Zhu, L., Wu, Y., Song, L., and Gan, N. (2014). Ecological dynamics of toxic microcystis spp. and microcystin-degrading bacteria in Dianchi Lake, China. Appl. Environ. Microbiol. 80, 1874–1881. doi: 10.1128/AEM.02972-13
Zhu, Z., Qu, P., Fu, F., Tennenbaum, N., Tatters, A. O., Hutchins, D. A., et al. (2017). Understanding the blob bloom: warming increases toxicity and abundance of the harmful bloom diatom Pseudo-nitzschia in California coastal waters. Harmful Algae 67, 36–43. doi: 10.1016/j.hal.2017.06.004
Zieger, S. E., Mistlberger, G., Troi, L., Lang, A., Confalonieri, F., Klimant, I., et al. (2018). Compact and low-cost fluorescence based flow-through analyzer for early-stage classification of potentially toxic algae and in situ semiquantification. Environ. Sci. Technol. 52, 7399–7408. doi: 10.1021/acs.est.8b00578
Keywords: harmful algal blooms, toxins, ocean observing, environmental technology, rapid detection, ecological forecasting
Citation: Stauffer BA, Bowers HA, Buckley E, Davis TW, Johengen TH, Kudela R, McManus MA, Purcell H, Smith GJ, Vander Woude A and Tamburri MN (2019) Considerations in Harmful Algal Bloom Research and Monitoring: Perspectives From a Consensus-Building Workshop and Technology Testing. Front. Mar. Sci. 6:399. doi: 10.3389/fmars.2019.00399
Received: 15 March 2019; Accepted: 27 June 2019;
Published: 16 July 2019.
Edited by:
Jay S. Pearlman, Institute of Electrical and Electronics Engineers, FranceReviewed by:
Joe Silke, Marine Institute, IrelandEmmanuel Devred, Department of Fisheries and Oceans, Canada
Copyright © 2019 Stauffer, Bowers, Buckley, Davis, Johengen, Kudela, McManus, Purcell, Smith, Vander Woude and Tamburri. This is an open-access article distributed under the terms of the Creative Commons Attribution License (CC BY). The use, distribution or reproduction in other forums is permitted, provided the original author(s) and the copyright owner(s) are credited and that the original publication in this journal is cited, in accordance with accepted academic practice. No use, distribution or reproduction is permitted which does not comply with these terms.
*Correspondence: Beth A. Stauffer, c3RhdWZmZXJAbG91aXNpYW5hLmVkdQ==