- 1Institute for Biological Resources and Marine Biotechnology (IRBIM), Italian National Research Council (CNR), Ancona, Italy
- 2Italian National Institute for Environmental Protection and Research (ISPRA), Rome, Italy
Despite the fact that Mediterranean loggerhead turtles are listed as “Least Concern” by the International Union for the Conservation of Nature (IUCN), they are among the most threatened marine megafauna worldwide, because of fishery-related incidental captures. The northern central Adriatic Sea is one of the most overfished basins of the Mediterranean Sea and it supports a very valuable marine biodiversity, including sea turtles. This study assesses the spatial and seasonal impact of the northern central Adriatic midwater pair trawl fishery on loggerhead turtle (Caretta caretta) by examining incidental catches recorded between 2006 and 2018 and environmental variables. The model predicted seasonal variation of loggerhead distribution. According to previous studies, data analysis indicates that most bycatch events of loggerhead turtle occurred in the northern Adriatic Sea. The present data confirm that the northern Adriatic may be an important foraging area for loggerhead turtle. They also highlight the urgent need for a better understanding of the interactions between sea turtles and fisheries to develop and apply suitable, ad hoc management measures in critical habitats.
Introduction
The incidental capture of non-target species occurring during fishing operations (e.g., Davies et al., 2009; Ortuño Crespo and Dunn, 2017) is one of the major global threat to marine megafauna of conservation concern (e.g., Worm et al., 2006; Lewison et al., 2014). Among those species, sea turtles are particularly vulnerable to the effects of bycatch given their biological and ecological characteristics (e.g., long life cycle, seasonal distribution patterns, long-distant foraging migrations, etc.) and the intensity of fishing effort across a various range of gears (e.g., Veiga et al., 2016; Lewison et al., 2013; Gray and Kennelly, 2018 and references there in).
The Mediterranean Sea is the world’s most overfished sea (Colloca et al., 2017), with the highest bycatch rates of marine turtles (Casale, 2008; Wallace et al., 2013; Casale et al., 2018). The loggerhead turtle (Caretta caretta) is the most abundant species regularly found in this area (Tudela, 2004; Lauriano et al., 2011) listed as “Least Concern” by the International Union for the Conservation of Nature (IUCN) Red List (Casale, 2015). Lucchetti and Sala (2010) and Casale (2011) indicated that yearly more than 132,000 sea turtles are unintentionally caught during fishing operations. Hooking, entanglement and capturing by different fishing gears usually intended to catch valuable commercial species are among the major threats to sea turtles from fisheries activities. A number of studies have already investigated the impact of fishing gears on long-lived marine species of conservation concern, like sea turtles (for example, see reviews by Lucchetti and Sala, 2010; Lewison et al., 2013; Wallace et al., 2013). However, more investigations are needed to evaluate how mortality due to interactions with fisheries varies by species and gear type.
The northern central Adriatic Sea is the most heavily impacted basin in the Mediterranean Sea due to a variety of sources of anthropogenic pressure, mainly intense fishing activities, large urbanized and industrialized areas, and environmental pollution (Lotze et al., 2011; Giani et al., 2012; Romano and Zullo, 2014; UNEP-MAP-RAC/SPA, 2014). This basin supports a rich and valuable marine biodiversity including marine megafauna like loggerhead turtle. Interactions between this species and fisheries are therefore unavoidable. Indeed, a moderate-to high bycatch risk of loggerheads has recently been suggested in the northern Adriatic for bottom trawls (Lucchetti et al., 2016) and estimated from interview based approach for set nets (Lucchetti et al., 2017). Nevertheless, data on the extent of incidental catches of sea turtles in other gears in the Adriatic is still limited (Lazar and Tvrtkovic, 1995, Lazar and Tvrtkoviæ, 2003; Lazar et al., 2006; Fortuna et al., 2010).
Since 2006, an extensive monitoring program of bycatch of long-lived species like cetaceans, sea turtles, and elasmobranchs by Italian midwater pair trawlers has been conducted in the northern central Adriatic Sea (Fortuna et al., 2010; Sala et al., 2016, 2018). The information collected in its framework provides a unique opportunity to assess the operational details of capture events and the abundance trends of species over time (Bonanomi et al., 2018).
The present study provides a spatial and seasonal evaluation of the impact of midwater trawling on loggerhead turtles in the northern central Adriatic Sea with the aim to assess the effect of environmental variables influencing the presence of this species and provide a risk map of the interaction between turtles and fishery basing on fishery dependent data. The results of this study can contribute to improve spatial fisheries management in critical habitats and to develop measures aimed at reducing bycatch events of loggerhead turtle in northern central Adriatic Sea.
Materials and Methods
Study Area and Data Collection
The Italian midwater pair trawl fishery is based in the northern central Adriatic Sea, Geographical Sub-Area (GSA) 17 (Figure 1). The area is characterized by shallow waters, with an average depth of 35 m. The strong influence of the Po river discharge results in low salinity, low water temperature, and high nutrient concentration (Fonda Umani, 1996; Marini et al., 2008; Lipizer et al., 2014). GSA 17 includes the entire northern and central Adriatic Sea as far as the Gargano Promontory in Italy and the city of Kotor in Montenegro.
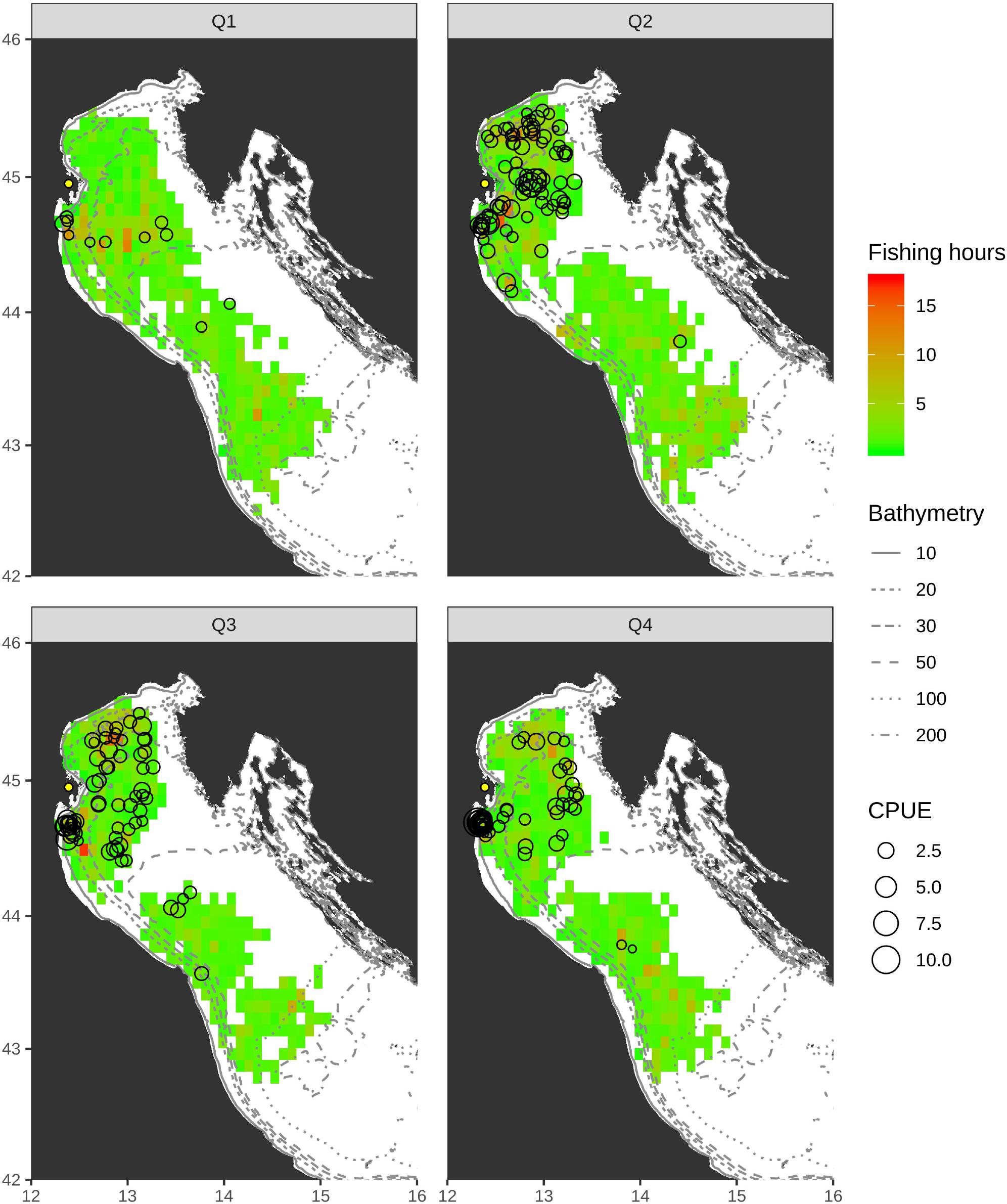
Figure 1. Distribution of hauls and bycatch events of Caretta caretta collected between 2006 and 2018 aggregated at quarterly base using a spatial grid with cell of 0.09° (about 10 km). The intensity of fishing hours is showed with a color gradient from green (0–5) to red (10–15). The size of circles indicates the magnitude of bycatch events (CPUE). The River Po delta is illustrated with a red point.
Between April 2006 and December 2018, a total of 15,975 hauls from 3,975 fishing trips, (see Table 1) conducted in the northern central Adriatic Sea, were monitored by independent observers who collected bycatch data of protected species (e.g., cetaceans, sea turtles) and species of conservation concern (e.g., elasmobranchs). The program took advantage of the mandatory monitoring program conducted under permit issued by the Italian Ministry of Agriculture, Food and Forestry, Fishery and Aquaculture directorate in compliance with the Italian obligations to the Council Regulation (EC) 812/2004 and with the EU Data Collection Framework. For each haul, observer recorded operational parameters including coordinates (latitude and longitude in WGS84), date and time of net setting and hauling, haul duration, trawling speed (nm) and water depth (m). For each haul, the observed Catch Per Unit Efforts (CPUEs) of loggerhead turtle were calculated as the number of individuals caught divided by the duration of the haul (in hours).
The influence of environmental variables, potentially affecting the presence of loggerhead sea turtles caught during fishing operations, were investigated. Information regarding the distribution and extension of seabed substrates were obtained from European Seabed Habitat map provided by EMODnet portals1. Sea Surface Temperature (SST) and chlorophyll concentration (CHL) data were retrieved from the European Union’s Earth Observation Program2. Both variables were obtained from satellite-based observations and they were available at different temporal (daily or monthly) and spatial resolution (depending by variable and year). In this study, monthly values were considered for both variables, then, when they were available at daily scale they were averaged at monthly base. Furthermore, to uniform the spatial information, they were rescaled to the same spatial resolution (spatial grid of 0.089°, ∼10 km). For each hauls, the coordinates and date were used to extract the corresponding values of environmental variables.
CPUEs Modeling
Catch Per Unit Efforts represents a common method to summarize fishery data, providing a measure of relative abundance. However, multiple drivers, mainly related to operational and environmental factors, can affect catch rate limiting the relationship between CPUE and abundance (Walters, 2003; Maunder et al., 2006). Generally, CPUE data are modeled to address the effects of these factors affecting catch rate (Maunder and Punt, 2004) but also to analyze species distribution in relation to this fishery’s operations (Katsanevakis et al., 2009; Grüss et al., 2014; Parra et al., 2017; Orio et al., 2017). In the case non-target species, which are caught less frequency than target species, modeling problems are related to the excess of zero observation (no accidental captures), due to the low catch rate, rarity of the non-target specie and low interaction with fishing gears (Maunder and Punt, 2004; Minami et al., 2007; Wenger and Freeman, 2008). Furthermore, another modeling problem is related to the over dispersion of the data, mainly caused by the stochasticity of the magnitude of bycatch events (Brodziak and Walsh, 2013).
In this study, spatiotemporal assessment of loggerhead turtles CPUEs was modeled using Generalized Additive Models (GAM) (Hastie et al., 2001) in a delta modeling framework. This approach has been proposed as a useful tool in case of zero-inflated data (Maunder and Punt, 2004), and it was successfully used to assess spatiotemporal distribution of marine species worldwide (Punt et al., 2000; Rodríguez-Marín et al., 2003) and, it was applied in other study on the distribution of no-target species in this area (La Mesa et al., 2016). Delta modeling procedure allows to modeling the probability of species occurrence and magnitude of catch events (CPUEs) separately, involving two modeling components (Maunder and Punt, 2004). The first component uses the occurrence or non-occurrence of bycatch events to estimate the probability of encountering a loggerhead turtle. Bycatch probability was modeled using a binary response variable (coded as 1/0) with a binomial error distribution and logit link. The second component considers only the hauls with positive catch, to assess the magnitude of bycatch events (CPUE) using a Gamma error distribution model with log link function. The Gamma distribution was commonly used in skewed data, like those occurring in bycatch data (Punt et al., 2000; Maunder and Punt, 2004). Finally, the predicted probabilities of presence were multiplied by the predicted values of positive catch, to obtain predicted CPUEs.
Before proceeding, a preliminary analysis on raw data to select regular hauls (i.e., fishing operations that were successfully completed) and excluding those with erroneous and/or lacking values (e.g., position on land, missing depth values, etc.) was performed. Bycatch events with more than four individuals account for less the 1% of the cases (two events), thus events with more than four individuals were grouped together in extra-group signed as five individuals and CPUE were estimated again. An exploratory data analysis was performed to accommodate the spatio-temporal structure of the data and identify the set of variables to use in CPUEs modeling.
Delta modeling approach allows to model bycatch probability and CPUEs with different set of variables. The initial models used were as follows:
Where Y represents the encountering probability of bycatch event in (Eqs 1), or its magnitude (CPUEs) in (Eqs 2); β0 is an overall intercept, β1, β2are coefficients estimated for each level of the factors considered in the parametric component of the models; s is an isotropic smoothing function (thin-plate regression spline; Wood, 2003), te represents a tensor product smoothing function used for interaction between variables; fi are natural cubic line and 𝜖 are error terms.
Model selection was performed through a backward stepwise selection based the total explained Deviance and on statistical significance (Wood, 2006). Starting from the full models, the predictor with the lowest significant level, was excluded and the model was run again until all remaining predictors were significant. During the model selection procedures, different types of interaction between variables were tested in order to improve models fitting maintaining the initial ecological assumptions. Furthermore, the maximum degree of freedom of the smoothing functions (number of knots k) were limited for smoothers of single variables (k = 5) and for interactions (k = 25) to avoid overfitting. The χ2 test was used to estimate the statistical significance of each term. Spatial prediction of CPUEs was performed using a spatial grid of 0.089° (∼10 km) at seasonal scale considering annual quarter (Q1: winter; Q2: spring; Q3: summer, Q4: autum). Excluding depth, the environmental variables associated to grid were averaged in each cell considering the values observed along the whole period.
The statistical significance level assumed in all the analyses was 5%. Data exploration was carried out with R version 3.4.4 (R Development Core Team, 2008), the mgcv package (Wood, 2011) was used for modeling data.
Results
A summary of raw data is reported in Table 1. Between 2006 and 2018, 14,170 successful hauls were monitored during 3,865 fishing trips. During this period, a total of 291 loggerhead turtles were unintentionally caught; the overall observed annual average probability of occurrence of bycatch events was 0.016 per haul with a mean CPUE value of 0.03 individuals per hour. The 90% (n = 262) of loggerheads were released alive without showing any injuries. The geographical distribution of quarterly monitoring effort and bycatch events (with CPUE values) are illustrated in Figure 1. The southernmost bycatch event was recorded at 43.56° of latitude. Most events (about 90%) were recorded in the northernmost area of the Adriatic Sea (between 44.5° and 45.5°), in the southern area (below 44.5°) were recorded 10 events in the whole period.
The final model used to predict the probability of the occurrence of bycatch events (binomial) includes 6 variables with the following formula: Presence ∼ Year + s(Lon, Lat)Quarter + te(Lat, Depth) + te(Lon, Depth) + te(x, CHL) + f(SST) (Table 2). It explains about 18.4% of the total deviance and the Anova χ2 test indicates that all terms were significant, except the interaction term between hauls location in the third and fourth quarter. The model-derived effects of covariates used in the binomial model area reported in Supplementary Figure 1. All the interactions, latitude-depth, longitude-depth and longitude-CHL, were retained in the model, as well as the effect of SST. In the binomial model, the effect of the year was considered in the parametric component of the model. The final model used to predict abundance of bycatch events (Gamma) includes four variables with the following formula: log(CPUE) ∼ Quarter + f(Year) + s(Lon, Lat)CHL + s(Lon, Lat) (Table 2). It explains about 40.6% of the total deviance and the Anova F test indicates that all terms were significant. The model-derived effects of covariates used in the Gamma error distribution model and residual plots area reported in Supplementary Figures 2, 3. The interaction between hauls position, and longitude-latitude with chlorophyll concentration were retained in the model. The effect of the year was considered as a smoothing function, while the effect of quarter was considered in the parametric component of the model. In the abundance model the global effect of depth and sea surface temperature were not significant and they were discarded.
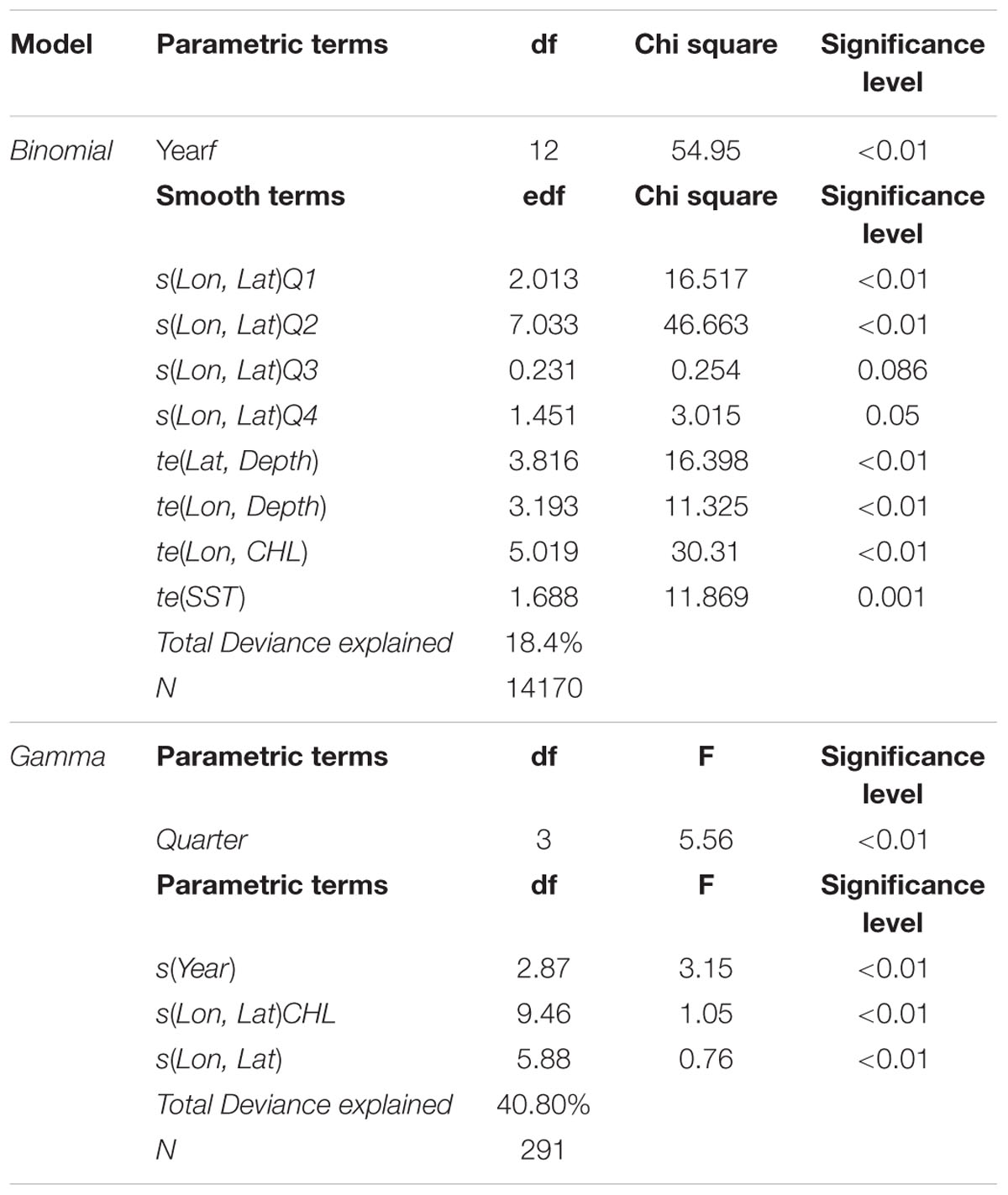
Table 2. Results of generalized additive models building for factors affecting the presence/absence and the abundance of loggerhead turtles in the northern central Adriatic Sea between 2006 and 2018.
The prediction of the final models on the spatial grid are showed at quarterly base in Figure 2. The predicted values were divided in four classes: from 0 to 0.009 (very low CPUE, <1 individual caught every 100 fishing hours), from 0.01 to 0.04 (low CPUE, between 1 and 4 individuals every 100 fishing hours), from 0.05 to 0.09 (Medium CPUE, between 5 to 9 individuals every 100 fishing hours) and between 0.1 to the maximum value (High CPUE, ≥10 individuals every 100 fishing hours). In winter (Q1), low CPUEs were predicted in cells in the eastern Adriatic Sea while high values were predicted in cell displaced south of the River Po delta. During spring (Q2), increasing values were predicted in the northern area (above 44° of latitude) with high values predicted in the cell of the western central and eastern central Adriatic. Medium and low values were predicted in cells in the northern and central Adriatic. In summer (Q3), high CPUEs were predicted in the cells south and north of the River Po delta and in a few cells in the western-central Adriatic. Very low values were predicted below 43.5° of latitude. During autumn (Q4), high and medium CPUEs were predicted in few cells in the area south and north of the River Po delta and in the eastern Adriatic, low values were predicted in cells of the northern area and in few cells toward western-central Adriatic.
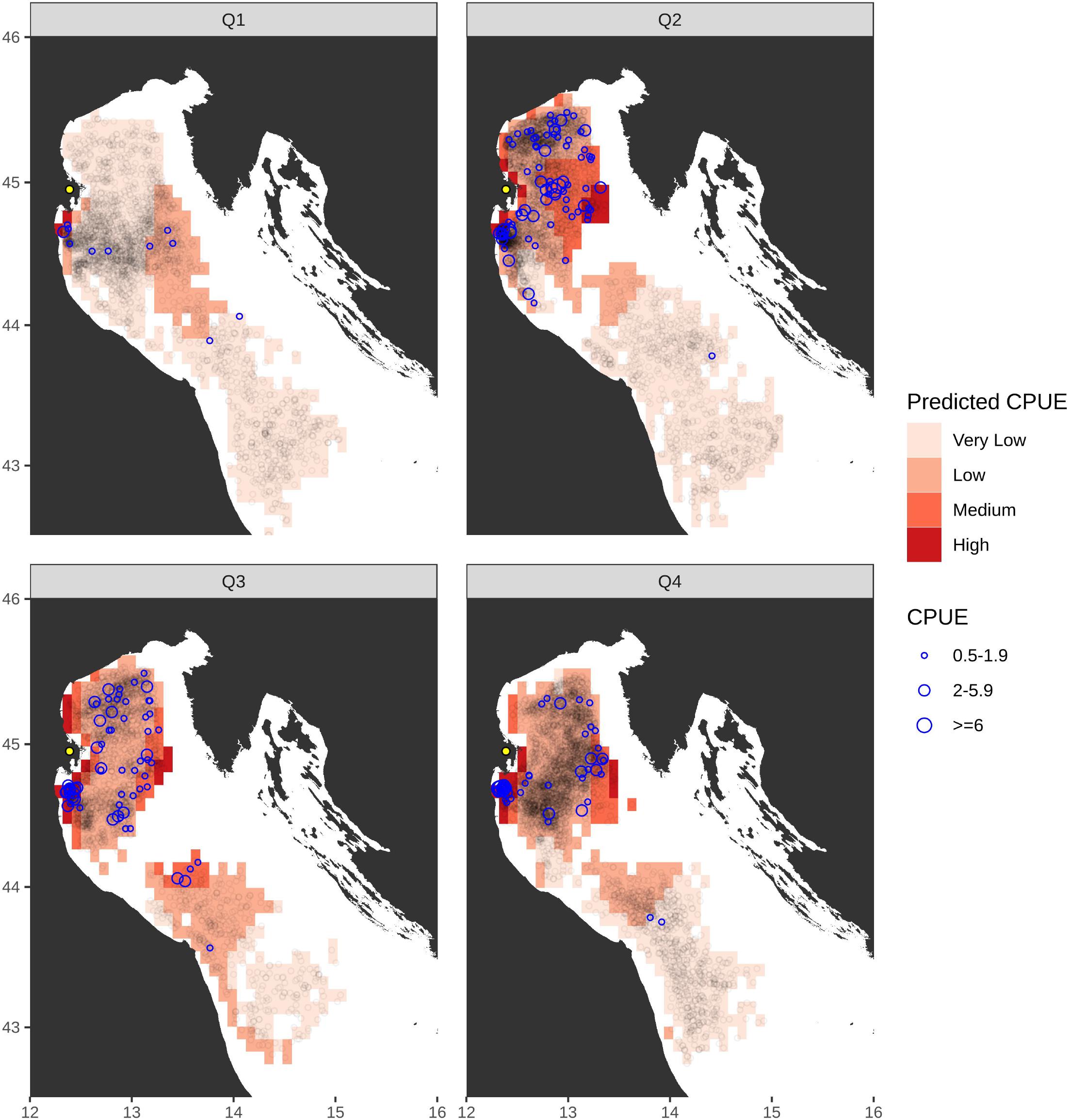
Figure 2. Distribution of predicted CPUEs aggregated at quarterly base using a spatial grid with cell of 0.09° (about 10 km). The predicted CPUEs are showed using tiles with a color a red gradient from light red (very low) to dark red (high). The observed CPUEs are represented with blue circles and their size indicates the magnitude. Position of fishing hauls with zero catches are represented with black circles. The River Po delta is illustrated with a yellow point.
Discussion
Robust data on spatial patterns of the risk of negative interactions between anthropogenic activities and protected species are required for Environmental Risk Assessments (see, for example, Azzellino et al., 2011). This must be true for fishery management frameworks too, which should be informed by a wide range of information, including spatial patterns of interactions and biological data on concerned species. However, the spatial element is seldom integrated in such frameworks, usually more oriented to consider only aspects related to species biology and population dynamics (e.g., ICES, 2014; Lucchetti et al., 2016). Bycatch Risk Assessments should incorporate the spatial dimension to inform area-based mitigation options, if they were to be effective tools to manage activities within marine regions (e.g., Azzellino et al., 2012). This study is among one of the first to provide an evaluation of the distribution of incidental capture of loggerhead turtle in a fishing métier for a Mediterranean sub-basin. It also provides the first maps of risk that are necessary to inform the process of designation and management of new Natura 2000 sites (Fortuna et al., 2018). In the study area, the interactions between loggerhead turtle and fishing gears have usually been ascribed to bottom trawlers (Casale et al., 2004; Lucchetti and Sala, 2010; Lucchetti et al., 2016), midwater pair trawlers (Casale et al., 2004; Fortuna et al., 2010), rapido (beam) trawlers (Lucchetti et al., 2018), and set nets (Lucchetti et al., 2017). The 13-year data in this study show that loggerheads were caught by midwater pair trawlers with a relative low rate (observed annual mean probability of 0.016 per haul). The monitoring activity demonstrated that a large number of individuals were incidentally caught and released alive without showing any injures. These findings indicate that interactions between loggerheads and midwater pair trawlers is moderate likely with very low mortality rate. In this study commercial CPUEs give an insight on the distribution of interactions between loggerhead turtles and fishing activities of midwater trawlers. They allow the assessment of the effect of operational and environmental variables on the interactions, by highlighting high and low risk areas. To accommodate the large amount of zero observations (about 98% of the records), data were analyzed by separately modeling the probability of occurrence of bycatch events (binomial model) and the positive catch (Gamma error distribution). Overall, the total Deviance explained by models was low and the explained variation was two times higher in the Gamma model. However, spatial and temporal assessment predicted by the final models seems to capture the global patterns present in the data. Different set of variables were used to modeling presence and the abundance patterns. The effects of operational variables, as location (i.e., coordinates of fishing operations) and time (year, quarter), resulted significant in both modeling components, as a consequence of the high spatiotemporal variability of the observed pattern. Depth and chlorophyll concentrations seem to be the most significant environmental drivers affecting the probability of bycatch events and their magnitude. Accordingly, the predicted spatial patterns of bycatch probability were concentrated in the northern Adriatic Sea, above 44.5° of latitude, nearby the River Po delta. This area is characterized by relative shallow waters (average depth of 30 m) and it is strongly influenced by the inter-annual freshwater discharge of the River Po, which is the primary source of nutrients in the whole basin (see Supplementary Figure 4; Degobbis and Gilmartin, 1990; de Wit and Bendoricchio, 2001) and makes the northern Adriatic one of the most productive area in the Mediterranean. Nutrient inflow, sustaining a high biodiversity community, makes this area important for turtles’ trophic interactions with abundant presence of preys like jellyfish, crustaceans and molluscs (Casale et al., 2012). The analyses presented in this study confirmed that the northernmost of Adriatic basin is a suitable foraging habitat for loggerheads according to previous studies (Lazar and Tvrtkovic, 1995, Lazar and Tvrtkoviæ, 2003; Lazar et al., 2006; Casale and Margaritoulis, 2010; Rees et al., 2017; Casale et al., 2018). In this area, the presence of a foraging ground identified nearby the Po delta, was probably the major ecological driver of the presence of this species, increasing the probability of unintentional catches particularly during spring and summer. Accordingly, in the southernmost area few individuals were accidentally caught.
Analysis of the present dataset indicates that probability of bycatch events increases between April and September (Q2–Q3). In accordance with these results, increasing CPUEs were predicted in the northernmost area of the Adriatic Sea, and then in autumn their values decrease in the central zone. These patterns are in accordance with previous investigations suggesting seasonal migration patterns in the Adriatic toward the River Po delta (Casale et al., 2012; Luschi and Casale, 2014; Lucchetti et al., 2016). Based on these studies, along the Italian coast, loggerheads during spring swim from the southern Adriatic toward the Po delta; in summer they frequent the northern area and begin to migrate southward; in autumn loggerheads can be find in the whole Adriatic with a concentration in the coastal area south of the River Po delta. During winter the probability of bycatch events and CPUEs decrease because low temperatures can influence loggerheads behavior, frequenting the northernmost part of the basin, to move southward (Casale et al., 2012).
In addition, the model predicted relative high CPUEs from spring to autumn in the eastern-north of the study area. This predicted patterns, could be explained by factors related to seasonal movement of loggerheads in relation to marine currents. Although sea turtles can travel against sea currents (Meylan, 1995), favorable surface circulation may facilitate their migration. A prevailing surface current enters the Adriatic along the eastern coast moving to the north (Cushman-Roisin et al., 2013), this current may influence the drift of hatchlings and small juveniles, partially directed into the Adriatic (e.g., the eastern Adriatic is a migration corridor for individuals belonging to a population nesting in Greece, Lazar et al., 2004).
Similarly, a number of studies have documented the spatial and temporal impact of fisheries bycatch on sea turtles worldwide (Wallace et al., 2013; Swimmer et al., 2017). In the present study, the observed pattern of loggerhead CPUE is consistent with the one observed in Wallace et al. (2013), which estimated the value of CPUE of loggerheads of different fishing gears, including trawlers, around the world (see tables in Wallace et al., 2013 and references there in).
In this study, the stochasticity of the distribution of loggerheads, and the low interaction with midwater trawlers, make bycatch events very rare reducing the ability of the model to predict correctly the occurrence of an event. Despite delta modeling represents a valid approach to accommodate zero-inflated data (Maunder and Punt, 2004), other limitations of the analyzed dataset could have biased model performance causing the low level of explained deviance and reduced its ability to predict CPUEs in space, in terms of absolute values. However, low level of explained variation of the data, was also obtained in other studies regarding the distribution of different marine species (Grüss et al., 2014; Orio et al., 2017; Parra et al., 2017), particularly with bycatch species using fishery dependent data (La Mesa et al., 2016). An important confounding factor is related to the nature (fishery dependent) of the data collected. Fishery-dependent data suffer of intrinsic bias related to the displacement of monitoring effort in space and time (Fulton et al., 2005; Sims et al., 2008), making difficult to assess the real influence of environmental variables on CPUE. Indeed, the monitoring program that provided these data, lack of a well-defined sampling design and the distribution of monitored activity, in space and time, was dependent to fleets dynamic, that, in the case of midwater pair trawl fishery is high variable in space in the short run, depending on biological (distribution of the target species) and economic drivers (market, fuel price) (Russo et al., 2015). Following the considerations in Fortuna et al. (2010), a non-homogenous distribution of monitoring activity in space would affect both the observation coverage and estimation of bycatch events. The lower number of monitored fishing trips than in the northern area could have an effect on the observed patterns in the southernmost area. However, the great differences between the northern and the southern marine ecosystem (e.g., water depth and quality, species presence and distribution, etc.), which influence the way this fishing gear is used within the study area, seem to support an actual difference in bycatch rates. Annual and inter-annual variability of the monitoring effort, was also conditioned by bureaucratic and administrative delays (e.g., gaps within the end of a project and the following call for tender of up to 6 months, tenders for funding only 1-year or 2-year projects, renewal of all observers contracts every 1–1.5 year), which determine lack of data for certain period and/or areas, and increase the uncertainty around the observed pattern.
The results obtained in this study suggest that management should carefully considered spatial component of ecological drivers of species distribution to design ad hoc management measures and conservation strategies aimed to reduce accidental captures (Casale et al., 2018). Knowledge of the biology and ecology of loggerhead turtle is still scanty and comprehensive studies of the spatial pattern of bycatch is lagging behind. However, different technological mitigation measures (e.g., TED and UV-LED lamps) have been tested in the Mediterranean and they seem to be a valuable solutions applied in multispecies fisheries in critical areas and seasons (Lucchetti and Sala, 2010; Sala et al., 2011; Lucchetti et al., 2016; Virgili et al., 2018). Thus, more investigations are clearly needed to understand the real impact and ecological implications of incidental captures of sea turtles in midwater pair trawl fisheries.
Data Availability
All relevant data used in the present study are within the manuscript and within its Supplementary Material. Fishing data (all operational and bycatch information) are from a national monitoring program of protected species and species of conservation concern entitled “BYCATCH” and from the monitoring program of protected species required by the EU Data Collection Framework. These data can be obtained by contacting the General Directorate for Fisheries and Aquaculture of the Italian Ministry of Agriculture Food, and Forestry Policies.
Ethics Statement
The loggerhead turtle (Caretta caretta) is considered as Least Concern under current IUCN Red List criteria. The study was based on incidental catches of protected species and species of conservation concern recorded by qualified observers on board Italian pelagic trawlers in the Adriatic Sea. The data collection was conducted under permit issued by the Italian Ministry of Agriculture, Food and Forestry, Fishery and Aquaculture directorate in compliance with the Italian obligations to the Council Regulation (EC) 812/2004. No other authorization or ethics board approval was required to conduct the study.
Author Contributions
JP and SB developed the ideas within the manuscript. JP analyzed the data. FM curated and maintained the historical dataset. SB and JP wrote the manuscript with inputs from AC, CF, AL, and AS.
Funding
This research was supported by the Italian Ministry of Agriculture, Food and Forestry.
Conflict of Interest Statement
The authors declare that the research was conducted in the absence of any commercial or financial relationships that could be construed as a potential conflict of interest.
Acknowledgments
We are grateful to all independent observers and fishermen involved in the scientific and monitoring program funded in compliance with the Regulations (EC) 812/2004 and with the EU Data Collection Framework. We would also like to thank two reviewers for their constructive comments and criticism.
Supplementary Material
The Supplementary Material for this article can be found online at: https://www.frontiersin.org/articles/10.3389/fmars.2019.00365/full#supplementary-material
Footnotes
- ^ https://www.emodnet-seabedhabitats.eu/
- ^ http://marine.copernicus.eu/services-portfolio/access-to-products/
References
Azzellino, A., Lanfredi, C., D’amico, A., Pavan, G., Podestà, M., and Haun, J. (2011). Risk mapping for sensitive species to underwater anthropogenic sound emissions: model development and validation in two Mediterranean areas. Mar. Poll. Bull. 63, 56–70. doi: 10.1016/j.marpolbul.2011.01.003
Azzellino, A., Panigada, S., Lanfredi, C., Zanardelli, M., Airoldi, S., and di Sciara, G. N. (2012). Predictive habitat models for managing marine areas: spatial and temporal distribution of marine mammals within the Pelagos Sanctuary (Northwestern Mediterranean sea). Ocean Coast. Manag. 67, 63–74. doi: 10.1016/j.ocecoaman.2012.05.04
Bonanomi, S., Pulcinella, J., Fortuna, C. M., Moro, F., and Sala, A. (2018). Elasmobranch bycatch in the Italian adriatic pelagic trawl fishery. PLoS One 13:e0191647. doi: 10.1371/journal.pone.0191647
Brodziak, J., and Walsh, W. A. (2013). Model selection and multimodel inference for standardizing catch rates of bycatch species: a case study of oceanic whitetip shark in the Hawaii-based longline fishery. Can. J. Fish. Aquat. Sci. 70, 1723–1740. doi: 10.1139/cjfas-2013-0111
Casale, P. (2008). Incidental Catch of Marine Turtles in the Mediterranean Sea: Captures, Mortality, Priorities. Rome: WWF, doi: 10.1111/j.1467-2979.2010.00394.x
Casale, P. (2015). Caretta caretta (Mediterranean subpopulation). IUCN Red List Threatened Species 2015:e.T83644804A83646294. doi: 10.1093/jhered/esr089
Casale, P., Affronte, M., Scaravelli, D., Lazar, B., Vallini, C., and Luschi, P. (2012). Foraging grounds, movement patterns and habitat connectivity of juvenile loggerhead turtles (Caretta caretta) tracked from the Adriatic Sea. Mar. Biol. 159, 1527–1535. doi: 10.1007/s00227-012-1937-2
Casale, P., Broderick, A. C., Camiñas, J. A., Cardona, L., Carreras, C., Demetropoulos, A., et al. (2018). Mediterranean sea turtles: current knowledge and priorities for conservation and research. Endanger. Species Res. 36, 229–267. doi: 10.3354/esr00901
Casale, P., Laurent, L., and De Metrio, G. (2004). Incidental capture of marine turtles by the Italian trawl fishery in the north Adriatic Sea. Biol. Conserv. 119, 287–295. doi: 10.1016/j.biocon.2003.11.013
Casale, P., and Margaritoulis, D. (2010). Sea Turtles in the Mediterranean: Distribution, Threats and Conservation Priorities. Gland: IUCN.
Colloca, F., Scarcella, G., and Libralato, S. (2017). Recent trends and impacts of fisheries exploitation on Mediterranean stocks and ecosystems. Front. Mar. Sci. 4:244. doi: 10.3389/fmars.2017.00244
Cushman-Roisin, B., Gacic, M., Poulain, P. M., and Artegiani, A. (eds) (2013). Physical Oceanography of the Adriatic Sea: Past, Present and Future. Berlin: Springer Science & Business Media.
Davies, R. W. D., Cripps, S. J., Nickson, A., and Porter, G. (2009). Defining and estimating global marine fisheries bycatch. Mar. Policy 33, 661–672. doi: 10.1016/j.marpol.2009.01.003
de Wit, M. J. M., and Bendoricchio, G. (2001). Nutrient fluxes in the Po basin. Sci. Total Environ. 273, 147–161. doi: 10.1016/S0048-9697(00)00851-2
Degobbis, D., and Gilmartin, M. (1990). Nitrogen, phosphorus, and biogenic silicon budgets for the northern Adriatic Sea. Oceanol. Acta 13, 31–45. doi: 10.1002/2016JC012665
Fortuna, C. M., Cañadas, A., Holcer, D., Brecciaroli, B., Donovan, G. P., Lazar, B., et al. (2018). The coherence of the European Union marine natura 2000 network for wide-ranging charismatic species: a Mediterranean case study. Front. Mar. Sci. 5:356. doi: 10.3389/fmars.2018.00356
Fortuna, C. M., Vallini, C., Filidei, E. J., Ruffino, M., Consalvo, M., Di Muccio, S., et al. (2010). Bycatch of cetaceans and other species of conservation concern during pair trawl fishing operations in the Adriatic Sea (Italy). Chem. Ecol. 26, 65–76. doi: 10.1080/02757541003627662
Fulton, E. A., Smith, A. D., and Punt, A. E. (2005). Which ecological indicators can robustly detect effects of fishing? ICES J. Mar. Sci. 62, 540–551. doi: 10.1016/j.icesjms.2004.12.012
Giani, M., Djakovac, T., Degobbis, D., Cozzi, S., Solidoro, C., and Umani, S. F. (2012). Recent changes in the marine ecosystems of the northern Adriatic Sea. Estuar. Coast. Shelf Sci. 115, 1–13. doi: 10.1016/j.ecss.2012.08.023
Gray, C. A., and Kennelly, S. J. (2018). Bycatches of endangered, threatened and protected species in marine fisheries. Rev. Fish Biol. Fish. 28, 521–541. doi: 10.1007/s11160-018-9520-7
Grüss, A., Drexler, M., and Ainsworth, C. H. (2014). Using delta generalized additive models to produce distribution maps for spatially explicit ecosystem models. Fish. Res. 159, 11–24. doi: 10.1016/j.fishres.2014.05.005
Hastie, T., Tibshirani, R., and Friedman, J. (2001). The Elements of Statistical Learning: Data Mining, Inference, and Prediction. New York, NY: Springer-Verlag.
ICES (2014). Report of the Working Group on Bycatch of Protected Species (WGBYC),4–7 February. (2014), Copenhagen, Denmark. British Columbia: ICES.
Katsanevakis, S., Maravelias, C. D., Damalas, D., Karageorgis, A. P., Tsitsika, E. V., Anagnostou, C., et al. (2009). Spatiotemporal distribution and habitat use of commercial demersal species in the eastern Mediterranean Sea. Fish. Oceanogr. 18, 439–457. doi: 10.1111/j.1365-2419.2009.00523.x
La Mesa, G., Annunziatellis, A., Filidei, E. Jr., and Fortuna, C. M. (2016). Bycatch of myliobatid rays in the central mediterranean sea: the influence of spatiotemporal, environmental, and operational factors as determined by generalized additive modeling. Mar. Coast. Fish. 8, 382–394. doi: 10.1080/19425120.2016.1167795
Lauriano, G., Panigada, S., Casale, P., Pierantonio, N., and Donovan, G. P. (2011). Aerial survey abundance estimates of the loggerhead sea turtle Caretta caretta in the Pelagos Sanctuary, northwestern Mediterranean Sea. Mar. Ecol. Prog. Ser. 437, 291–302. doi: 10.3354/meps09261
Lazar, B., Margaritoulis, D., and Tvrtković, N. (2004). Tag recoveries of the loggerhead sea turtle Caretta caretta in the eastern Adriatic Sea: implications for conservation. J. Mar. Biol. Assoc. U.K. 84, 475–480. doi: 10.1017/S0025315404009488h
Lazar, B., and Tvrtkovic, N. (1995). Marine turtles in the eastern part of the Adriatic Sea: preliminary research. Oceanogr. Lit. Rev. 12:1106.
Lazar, B., and Tvrtkoviæ, N. (2003). “Marine turtles and fisheries in the Mediterranean: are we missing something?” in Proceedings of the 22nd Annual Symposium on Sea Turtle Biology and Conservation, 5- 6, ed. J. A. Seminoff (Miami: NOAA Technical Memorandum).
Lazar, B., Žiža, V., and Tvrtkoviæ, N. (2006). “Interactions of gillnet fishery with loggerhead sea turtles Caretta caretta in the northern Adriatic Sea,” in Book of Abstracts, 26th Annual Symposium on Sea Turtle Biology and Conservation, 352, eds M. Frick, A. Panagopoulou, A. F. Rees, and K. Williams (Athens: International Sea Turtle Society).
Lewison, R., Wallace, B., Alfaro-Shigueto, J., Mangel, J. C., Maxwell, S. M., and Hazen, E. L. (2013). “Fisheries bycatch of marine turtles: Lessons learned from decades of research and conservation,” in Biology of Sea Turtles, Vol. 3, eds J. Wyneken, K. J. Lohmann, and J. A. Musick (Boca Raton, FL: CRC Press), 329–351.
Lewison, R. L., Crowder, L. B., Wallace, B. P., Moore, J. E., Cox, T., Zydelis, R., et al. (2014). Global patterns of marine mammal, seabird, and sea turtle bycatch reveal taxa-specific and cumulative megafauna hotspots. Proc. Natl. Acad. Sci. U.S.A. 14, 5271–5276. doi: 10.1073/pnas.1318960111
Lipizer, M., Partescano, E., Rabitti, A., Giorgetti, A., and Crise, A. (2014). Qualified temperature, salinity and dissolved oxygen climatologies in a changing Adriatic Sea. Ocean Sci. 5:771. doi: 10.5194/os-10-771-2014
Lotze, H. K., Coll, M., and Dunne, J. (2011). Historical changes in marine resources, food-web structure and ecosystem functioning in the Adriatic Sea. Ecosystems 14, 198–222. doi: 10.1007/s10021-010-9404-8
Lucchetti, A., Angelini, V., Furii, G., Pari, S., Vasapollo, C., and Virgili, M. (2018). Evidence of loggerhead sea turtle (Caretta caretta, Linnaeus, 1758) injuries caused by Rapido (beam) trawling in the Mediterranean. Herpetol. J. 28, 134–136.
Lucchetti, A., Pulcinella, J., Angelini, V., Pari, S., Russo, T., and Cataudella, S. (2016). An interaction index to predict turtle bycatch in a Mediterranean bottom trawl fishery. Ecol. Indic. 60, 557–564. doi: 10.1016/j.ecolind.2015.07.007
Lucchetti, A., and Sala, A. (2010). An overview of loggerhead sea turtle (Caretta caretta) bycatch and technical mitigation measures in the Mediterranean Sea. Rev. Fish Biol. Fish. 20, 41–161. doi: 10.1007/s11160-009-91x26-1
Lucchetti, A., Vasapollo, C., and Virgili, M. (2017). An interview-based approach to assess sea turtle bycatch in Italian waters. PeerJ 5:e3151. doi: 10.7717/peerj.3151
Luschi, P., and Casale, P. (2014). Movement patterns of marine turtles in the Mediterranean Sea: a review. Ital. J. Zool. 81, 478–495. doi: 10.1080/11250003.2014.963714
Marini, M., Jones, B., Campanelli, A., Grilli, F., and Lee, C. (2008). Seasonal variability and Po River plume influence on biochemical properties along wester Adriatic Coast. J. Geophys. Res. 113:C05S90. doi: 10.1029/2007JC004370
Maunder, M. N., and Punt, A. E. (2004). Standardizing catch and effort data: a review of recent approaches. Fish. Res. 70, 141–159. doi: 10.1016/j.fishres.2004.08.002
Maunder, M. N., Sibert, J. R., Fonteneau, A., Hampton, J., Kleiber, P., and Harley, S. J. (2006). Interpreting catch per unit effort data to assess the status of individual stocks and communities. ICES J. Mar. Sci. 63, 1373–1385. doi: 10.1016/j.icesjms.2006.05.008
Meylan, A. B. (1995). “Sea turtle migration evidence from tag returns,” in Biology and Conservation of sea Turtles. Revised edition, ed. K. A. Bjorndal (Washington, DC: Smithsonian Institution Press), 91–100.
Minami, M., Lennert-Cody, C. E., Gao, W., and Roman-Verdesoto, M. (2007). Modeling shark bycatch: the zero-inflated negative binomial regression model with smoothing. Fish. Res. 84, 210–221. doi: 10.1016/j.fishres.2006.10.019
Orio, A., Florin, A. B., Bergstrom, U., Sics, I., Baranova, T., and Casini, M. (2017). Modeling indices of abundance and size-based indicators of cod and flounder stocks in the Baltic Sea using newly standardized trawl survey data. ICES J. Mar. Sci. 74, 1322–1333. doi: 10.1093/icesjms/fsx005
Ortuño Crespo, G., and Dunn, D. C. (2017). A review of the impacts of fisheries on open-ocean ecosystems. ICES J. Mar. Sci. 74, 2283–2297. doi: 10.1093/icesjms/fsx084
Parra, H. E., Pham, C. K., Menezes, G. M., Rosa, A., Tempera, F., and Morato, T. (2017). Predictive modeling of deep-sea fish distribution in the Azores. Deep Sea Res. II Top. Stud. Oceanogr. 145, 49–60. doi: 10.1016/j.dsr2.2016.01.004
Punt, A. E., Walker, T. I., Taylor, B. L., and Pribac, F. (2000). Standardization of catch and effort data in a spatially-structured shark fishery. Fish. Res. 45, 129–145. doi: 10.1016/S0165-7836(99)00106-X
Rees, A. F., Carreras, C., Broderick, A. C., Margaritoulis, D., Stringell, T. B., and Godley, B. J. (2017). Linking loggerhead locations: using multiple methods to determine the origin of sea turtles in feeding grounds. Mar. Biol. 164:30. doi: 10.1007/s00227-016-3055-z
Rodríguez-Marín, E., Arrizabalaga, H., Ortiz, M., Rodríguez-Cabello, C., Moreno, G., and Kell, L. T. (2003). Standardization of bluefin tuna, Thunnus thynnus, catch per unit effort in the baitboat fishery of the Bay of Biscay (Eastern Atlantic). ICES J. Mar. Sci. 60, 1216–1231. doi: 10.1016/S1054-3139(03)00139-5
Romano, B., and Zullo, F. (2014). The urban transformation of Italy’s Adriatic coastal strip: fifty years of unsustainability. Land Policy 38, 26–36. doi: 10.1016/j.landusepol.2013.10.001
Russo, T., Pulcinella, J., Parisi, A., Martinelli, M., Belardinelli, A., Santojanni, A., et al. (2015). Modeling the strategy of mid-water trawlers targeting small pelagic fish in the Adriatic Sea and its drivers. Ecol. Model. 300, 102–113. doi: 10.1016/j.ecolmodel.2014.12.001
Sala, A., Bonanomi, S., Benetti, A., Cani, M. V., Ciofi, C., Colombelli, A., et al. (2018). Valutazione Delle Catture Accidentali di Specie protette nel traino pelagico BYCATCH 2016-2017. Relazione finale del progetto,109 pp.
Sala, A., Bonanomi, S., Colombelli, A., Filidei, E. J., Fortuna, C. M., Gaspari, S., et al. (2016). Valutazione delle catture accidentali di specie protette nel traino pelagico BYCATCH 2014-2015 (D.M. 68/14, Cap. 7043, Es. 2014). Relazione finale, 48 pp.
Sala, A., Lucchetti, A., and Affronte, M. (2011). Effects of turtle excluder devices on bycatch and discard reduction in the demersal fisheries of Mediterranean Sea. Aquat. Living Resour. 24, 183–192. doi: 10.1051/alr/2011109
Sims, M., Cox, T., and Lewison, R. (2008). Modeling spatial patterns in fisheries bycatch: improving bycatch maps to aid fisheries management. Ecol. Appl. 18, 649–661. doi: 10.1890/07-0685.1
Swimmer, Y., Gutierrez, A., Bigelow, K., Barceló, C., Schroeder, B., Keene, K., et al. (2017). Sea turtle bycatch mitigation in US longline fisheries. Front. Mar. Sci. 4:260. doi: 10.3389/fmars.2017.00260
Tudela, S. (2004). Ecosystem effects of fishing in the Mediterranean. An analysis of the major threats of fishing gear and practices to biodiversity and marine habitats. Stud. Rev. 74:160.
UNEP-MAP-RAC/SPA (2014). “Status and Conservation of Fisheries in the Adriatic Sea,” in Draft Internal Report for the Purposes of the Mediterranean Regional Workshop to Facilitate the Description of Ecologically or Biologically Significant Marine Areas, eds H. Farrugio and Alen Soldo (Malaga: UNEP). doi: 10.1016/j.gecco.2015.06.005
Veiga, P., Pita, C., Rangel, M., Gonçalves, J. M., Campos, A., Fernandes, P. G., et al. (2016). The EU landing obligation and European small-scale fisheries: what are the odds for success? Mar. Policy 64, 64–71. doi: 10.1016/j.marpol.2015.11.008
Virgili, M., Vasapollo, C., and Lucchetti, A. (2018). Can ultraviolet illumination reduce sea turtle bycatch in Mediterranean set net fisheries? Fish. Res. 199, 1–7. doi: 10.1016/j.fishres.2017.11.012
Wallace, B. P., Kot, C. Y., DiMatteo, A. D., Lee, T., Crowder, L. B., and Lewison, R. L. (2013). Impacts of fisheries bycatch on marine turtle populations worldwide: toward conservation and research priorities. Ecosphere 4:40. doi: 10.1890/ES12-00388.1
Walters, C. J. (2003). Folly and fantasy in the analysis of spatial catch rate data. Can. J. Fish. Aquat. Sci. 60, 1433–1436. doi: 10.1139/F03-152
Wenger, S. J., and Freeman, M. C. (2008). Estimating species occurrence, abundance, and detection probability using zero-inflated distributions. Ecology 89, 2953–2959. doi: 10.1890/07-1127.1
Wood, S. N. (2003). Thin-plate regression splines. J. R. Stat. Soc. 65, 95–114. doi: 10.1111/1467-9868.00374
Wood, S. N. (2006). Generalized Additive Models: An Introduction with R. Boca Raton, FL: Chapman and Hall.
Wood, S. N. (2011). Fast stable restricted maximum likelihood and marginal likelihood estimation of semiparametric generalized linear models. J. R. Stat. Soc. 73, 3–36. doi: 10.1111/j.1467-9868.2010.00749.x
Keywords: Adriatic Sea, bycatch, Caretta caretta, critical habitats, midwater pair trawl fishery
Citation: Pulcinella J, Bonanomi S, Colombelli A, Fortuna CM, Moro F, Lucchetti A and Sala A (2019) Bycatch of Loggerhead Turtle (Caretta caretta) in the Italian Adriatic Midwater Pair Trawl Fishery. Front. Mar. Sci. 6:365. doi: 10.3389/fmars.2019.00365
Received: 30 April 2019; Accepted: 12 June 2019;
Published: 04 July 2019.
Edited by:
Violin Stoyanov Raykov, Bulgarian Academy of Sciences, BulgariaReviewed by:
Dimitrios K. Moutopoulos, University of Patras, GreeceSanja Matic-Skoko, Institute of Oceanography and Fisheries, Croatia
Copyright © 2019 Pulcinella, Bonanomi, Colombelli, Fortuna, Moro, Lucchetti and Sala. This is an open-access article distributed under the terms of the Creative Commons Attribution License (CC BY). The use, distribution or reproduction in other forums is permitted, provided the original author(s) and the copyright owner(s) are credited and that the original publication in this journal is cited, in accordance with accepted academic practice. No use, distribution or reproduction is permitted which does not comply with these terms.
*Correspondence: Jacopo Pulcinella, amFjb3BvLnB1bGNpbmVsbGFAaXJiaW0uY25yLml0
†These authors have contributed equally to this work