- 1Department of Microbiology and Immunology, The University of British Columbia, Vancouver, BC, Canada
- 2Bigelow Laboratory for Ocean Sciences, Boothbay, ME, United States
- 3Woods Hole Oceanographic Institute, Woods Hole, MA, United States
- 4Terry Fox Laboratory, BC Cancer Agency, Vancouver, BC, Canada
- 5Department of Ocean and Earth Science, National Oceanography Centre, University of Southampton, Southampton, United Kingdom
- 6The Scientific Committee on Oceanographic Research, Working Group 144, Vancouver, BC, Canada
- 7Departamento de Oceanografía and Instituto Milenio de Oceanografía, Universidad Concepción, Concepción, Chile
- 8Department of Biological Oceanography, Leibniz Institute for Baltic Sea Research, Warnemünde, Germany
- 9Department of Marine Sciences and Convergent Technology, Hanyang University, Seoul, South Korea
- 10Department of Earth, Ocean and Atmospheric Sciences, The University of British Columbia, Vancouver, BC, Canada
- 11ECOSCOPE Training Program, The University of British Columbia, Vancouver, BC, Canada
- 12Graduate Program in Bioinformatics, The University of British Columbia, Vancouver, BC, Canada
- 13Peter Wall Institute for Advanced Studies, The University of British Columbia, Vancouver, BC, Canada
The Scientific Committee on Oceanographic Research (SCOR) Working Group 144 Microbial Community Responses to Ocean Deoxygenation workshop held in Vancouver, B.C on July 2014 had the primary objective of initiating a process to standardize operating procedures for compatible process rate and multi-omic (DNA, RNA, protein, and metabolite) data collection in marine oxygen minimum zones and other oxygen depleted waters. Workshop attendees participated in practical sampling and experimental activities in Saanich Inlet, British Columbia, a seasonally anoxic fjord. Experiments were designed to compare and cross-calibrate in situ versus bottle sampling methods to determine effects on microbial community structure and potential activity when using different filter combinations, filtration methods, and sample volumes. Resulting biomass was preserved for small subunit ribosomal RNA (SSU or 16S rRNA) and SSU rRNA gene (rDNA) amplicon sequencing followed by downstream statistical and visual analyses. Results from these analyses showed that significant community shifts occurred between in situ versus on ship processed samples. For example, Bacteroidetes, Alphaproteobacteria, and Opisthokonta associated with on-ship filtration onto 0.4 μm filters increased fivefold compared to on-ship in-line 0.22 μm filters or 0.4 μm filters processed and preserved in situ. In contrast, Planctomycetes associated with 0.4 μm in situ filters increased fivefold compared to on-ship filtration onto 0.4 μm filters and on-ship in-line 0.22 μm filters. In addition, candidate divisions and Chloroflexi were primarily recovered when filtered onto 0.4 μm filters in situ. Results based on rRNA:rDNA ratios for microbial indicator groups revealed previously unrecognized roles of candidate divisions, Desulfarculales, and Desulfuromandales in sulfur cycling, carbon fixation and fermentation within anoxic basin waters. Taken together, filter size and in situ versus on-ship filtration had the largest impact on recovery of microbial groups with the potential to influence downstream metabolic reconstruction and process rate measurements. These observations highlight the need for establishing standardized and reproducible techniques that facilitate cross-scale comparisons and more accurately assess in situ activities of microbial communities.
Introduction
Among the many environmental perturbations associated with global climate change is a decrease in dissolved oxygen (O2) concentrations in coastal and interior regions of the ocean. As dissolved O2 concentrations decline, oxygen minimum zones (OMZs) expand (Arrigo, 2005; Whitney et al., 2007; Diaz and Rosenberg, 2008; Stramma et al., 2008; Paulmier and Ruiz-Pino, 2009; Keeling et al., 2010; Schmidtko et al., 2017). The expansion of OMZs shifts energy away from higher trophic levels, impacting ecosystem functions and services through changes in food web structure and biodiversity (Diaz and Rosenberg, 2008; Stramma et al., 2010; Gruber, 2011; Bijma et al., 2013; Levin and Breitburg, 2015; Gallo and Levin, 2016). These changes are reflected in an increasing role for microbial metabolism in nutrient and energy cycling through the use of alternative terminal electron acceptors (TEAs) including nitrate (NO3-), sulfate (SO42-), and carbon dioxide (CO2) (Diaz and Rosenberg, 2008). Among other changes, the use of these TEAs in respiration results in fixed nitrogen loss and the production of climate active trace gasses including nitrous oxide (N2O) and methane (CH4) with potential feedback on the climate system (Lam et al., 2009; Ward et al., 2009; Canfield et al., 2010; Naqvi et al., 2010; Lam and Kuypers, 2011). Current research efforts are defining the interaction networks underlying microbial metabolism in OMZs and generating new insights into coupled biogeochemical processes driving nutrient and energy flow among and between trophic levels on local scales (Hawley et al., 2014; Cram et al., 2015; Louca et al., 2016; Torres-Beltrán et al., 2016). However, marine microbial responses at the individual, population and community levels to OMZ expansion, and the concomitant impact of these responses on global-scale nutrient and energy cycling remain poorly constrained due in part to inconsistent, and perhaps inadequate sampling methods that limit cross-scale comparisons between locations and may cloud our view of in situ microbial processes.
Over the past 20 years, oceanographic researchers have increasingly used multi-omic (DNA, RNA, protein, and metabolites) methods to determine microbial community structure, function and activity in relation to physical, chemical, and biological oceanographic processes (Supplementary Figure 1). While large-scale microbial community composition patterns appear to be consistent between studies with respect to major taxonomic groups and water column compartments, our ecological perspective is blurred by inconsistencies in marker gene selection and coverage.
Recent reports have begun to evaluate potential biases in OMZ microbial community structure, function, and activity with emphasis on sample collection and filtration methods. Water column sampling typically involves the use of collection bottles (Niskin or GO-FLO) and on-ship filtration to concentrate microbial biomass into two primary size fractions, a larger particle associated (>1–30 μm) and smaller free-living (<1–0.2 μm) fraction (Padilla et al., 2015). Size fractionation surveys conducted in the Eastern Tropical South Pacific (ETSP) and Eastern Tropical North Pacific (ETNP) showed differential microbial community structure and nitrogen cycling functional gene distribution and expression across size fractions (Ganesh et al., 2014, 2015). In addition to filter fractionation, Padilla et al. (2015) working in the Manzanillo Mexico OMZ observed variation in microbial community structure based on filtered water volume. Most recently, a study in the Cariaco Basin observed that particles sinking on timescales relevant to sample collection and filtration can influence microbial community structure in Niskin or GO-FLO bottles (Suter et al., 2016). A comparison of metatranscriptome data obtained from bathypelagic Mediterranean Sea samples collected using Niskin bottles followed by shipboard filtration vs. filtration and fixation in situ found significant shifts in gene expression for particular groups of microorganisms (Edgcomb et al., 2016). These findings reinforce the need for continued evaluation of the methods used for sample collection and processing. This is particularly relevant when conducting process rate measurements in which community structure variation due to bottle effects can result in potential rates that do not reflect in situ microbial activity (Stewart et al., 2012a).
The Scientific Committee on Oceanographic Research (SCOR) initiated Working Group 144 Microbial Community Responses to Ocean Deoxygenation to investigate and recommend community standards of practice for compatible multi-omic and process rate measurements in OMZs and other oxygen deficient waters in order to facilitate and promote future cross-scale comparisons that more accurately reflect in situ microbial community structure, function, and activity1. The inaugural workshop of SCOR Working Group 144 was held in British Columbia Canada during the week of July 14, 2014. During the workshop, attendees participated in practical sampling and experimental activities in Saanich Inlet (SI), a seasonally anoxic fjord on the coast of Vancouver Island, British Columbia. During spring and summer months, restricted circulation and high levels of primary production lead to progressive deoxygenation and the accumulation of methane (CH4), ammonium (NH4+), and hydrogen sulfide (H2S) in deep basin waters. In late summer and fall, oxygenated nutrient rich waters flow into the inlet from the Haro Strait “renewing” deep basin waters (Carter, 1932, 1934; Herlinveaux, 1962; Anderson and Devol, 1973; Zaikova et al., 2010; Walsh and Hallam, 2011; Torres-Beltrán et al., 2017). The seasonal pattern of water column anoxia and renewal makes the inlet a model ecosystem for evaluating changes in microbial community structure, function and activity in response to changing levels of water column deoxygenation. Saanich Inlet is thus a tractable environment to test different water sample collection and processing methods relevant to OMZs.
Experiments carried out during the workshop were designed to compare and cross-calibrate in situ sampling with conventional bottle sampling methods including the use of different filter combinations and sample volumes. Here, we describe the effect of these parameters on microbial community structure and potential activity and discuss community standards development to facilitate and promote future cross-scale comparisons that more accurately reflect in situ microbial community structure, function and activity in OMZs and other oxygen deficient waters.
Materials and Methods
Environmental Sampling
Sampling methods used during the workshop were similar to those previously described (Zaikova et al., 2010; Walsh and Hallam, 2011; Torres-Beltrán et al., 2017). In brief, waters for analysis of dissolved gasses and nutrients were collected aboard the MSV John Strickland at station SI03 (48° 35.500 N, 123° 30.300 W) on July 16, 2014 using 12 L GO-FLO bottles attached in series to a steel winch cable and closed at depth via messenger. A CTD was attached to the cable below the bottles and was used to measure temperature, salinity, PAR/Irradiance, fluorescence, conductivity, density, and dissolved O2 at 165 and 185 m depth intervals spanning anoxic (<1 μmol O2 kg1) and sulfidic water column compartments (Figures 1A,B and Supplementary Table 2).
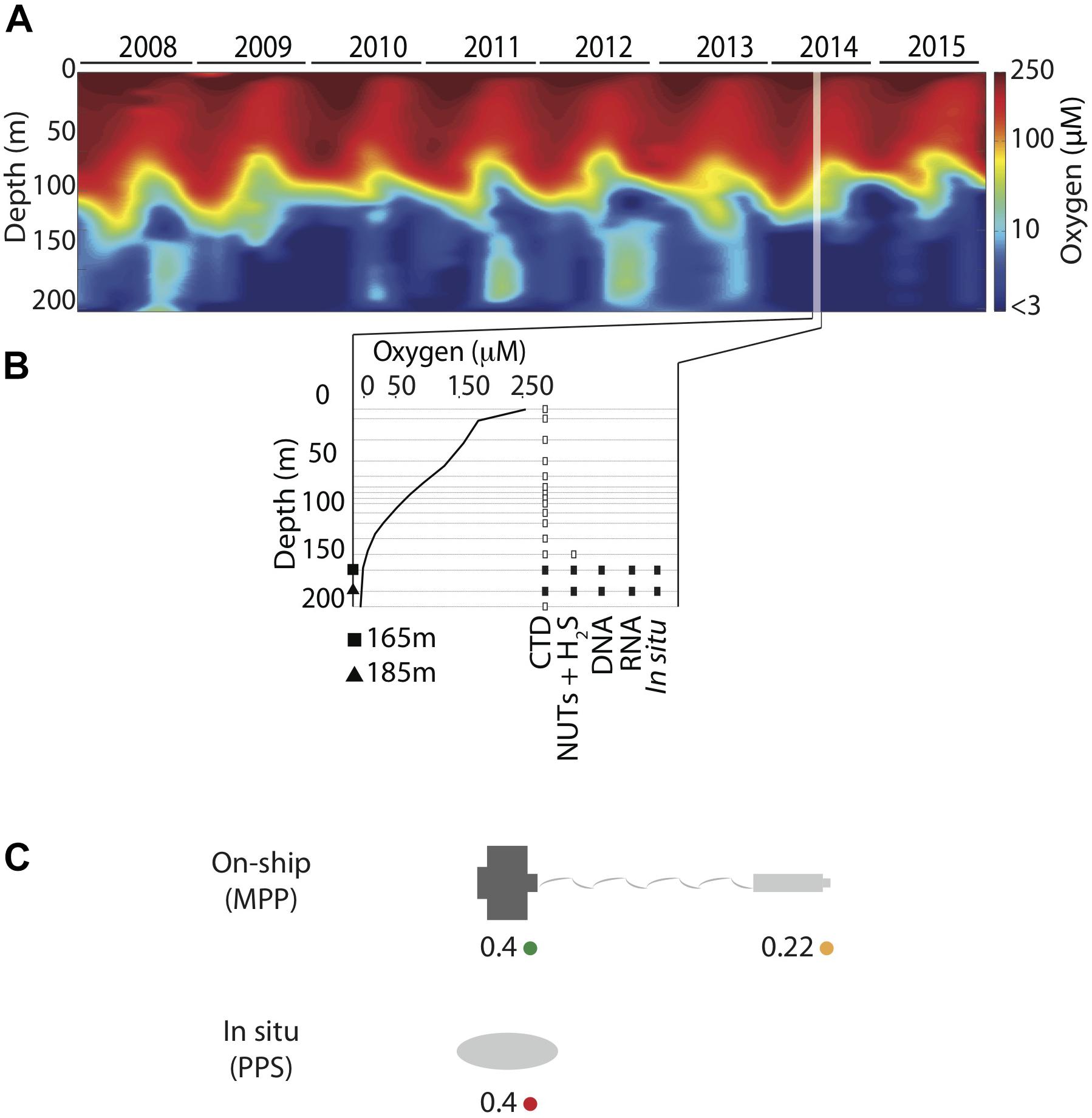
Figure 1. (A) Saanich Inlet time-series CTD oxygen (O2) concentration contour (2008–2015) showing seasonal water column stratification and deep-water renewal events. (B) Sample inventory for SCOR workshop held in July 2014 includes CTD, nutrients (NO3, NO2, NH4, PO4, and SiO2), hydrogen sulfide (H2S), and multi-omic on-ship and in situ data. Data shown in this survey is depicted as solid symbols (in situ and on ship DNA and RNA (rectangle) collected at 165 (square) and 185 (triangle) meters. (C) Schematic model for filter combinations and filtration methods showing on-ship [MasterFlex peristaltic pump (MPP)] filtration onto 0.4 μm pre-filters (green), filtration onto 0.22 μm filters with in-line pre-filtration using the 0.4 μm pre-filters (yellow), and in situ filtration (PPS) onto 0.4 μm filters without pre-filtration (red).
Workshop Microbial Biomass Collection
For comparison to Niskin bottle sampling, water samples were collected and preserved in situ in duplicate using a McLane Phytoplankton Sampler (PPS) system deployed at 165 and 185 m depth intervals spanning anoxic (<1 μmol O2 kg1) and sulfidic water column compartments (Figure 1B). Sample volumes of 2 L were filtered onto 0.4 μm GFF membrane filters (47 mm diameter). Filter biomass was directly frozen and stored at -80°C for downstream DNA and RNA extraction. On-ship samples were collected using Niskin bottles from 165 to 185 m as described above (Figure 1B) and concentrated for DNA and RNA extractions with a MasterFlex peristaltic pump (MPP) (∼60 mL min-1). Biomass was concentrated using different filter combinations (0.4 μm polycarbonate or 2.7 μm GF/D pre-filters in-line with a 0.22 μm Sterivex polycarbonate filter cartridge) (Figure 1C) and water volumes (250 ml, 500 ml, 1 and 2 L) (Table 1) to test significance of sample volume and filtration method, on community structure (see section “Statistical Analysis and Data Visualization”). Filtered biomass on Sterivex filters was preserved in 1.8 ml of sucrose lysis buffer (DNA analyses) or RNA later (RNA analyses) prior to storage at -80°C. Pre-filters (0.4 μm only) were also preserved in 1.8 ml of lysis buffer (DNA) or RNA later (RNA) prior to storage at -80°C.
Time-Series Microbial Biomass Collection
Time-series samples were collected as previously described (Walsh et al., 2009; Zaikova et al., 2010; Torres-Beltrán et al., 2016; Hawley et al., 2017). Briefly, large volume (10 L) samples were collected from February 2006 to February 2011 at six depths (10, 100, 120, 135, 150, and 200 m) and filtered with an in-line 2.7 μm GDF glass fiber pre-filter onto a 0.22 μm Sterivex polycarbonate cartridge filter. High-resolution (2 L) samples were collected from May 2008 to July 2010 from 16 depths (10 to 200 m) and filtered directly onto a 0.22 μm Sterivex polycarbonate cartridge filter. All time-series samples were preserved in 1.8 ml of sucrose lysis buffer (DNA) prior to storage at -80°C.
Nucleic Acid Extraction
Genomic DNA was extracted from Sterivex filters (Table 1) as previously described (Zaikova et al., 2010; Hawley et al., 2017). Briefly, after defrosting Sterivex on ice, 100 μl lysozyme (0.125 mg ml-1; Sigma) and 20 μl of RNAse (1 μl ml-1; Thermo Fisher) were added and incubated at 37°C for 1 h with rotation followed by addition of 50 μl Proteinase K (Sigma) and 100 μl 20% SDS and incubation at 55°C for 2 h with rotation. Lysate was removed by pushing through with a syringe into 15 mL falcon tube (Corning) and with an additional rinse of 1 mL of lysis buffer. Filtrate was subject to chloroform extraction (Sigma) and the aqueous layer was collected and loaded onto a 10 K 15 ml Amicon filter cartridge (Millipore), washed three times with TE buffer (pH 8.0) and concentrated to a final volume of between 150 and 400 μl. Total DNA concentration was determined by PicoGreen assay (Life Technologies) and genomic DNA quality determined by visualization on 0.8% agarose gel (overnight at 16V). Genomic DNA was extracted from 0.4 to 2.7 μm pre-filters (Table 1) as follows. The filter was cut in half using sterile scissors. One half was minced into smaller pieces and used for DNA extraction while the remaining half was stored at -80°C. Filter pieces were transferred to a 15 mL falcon tube followed by addition of 1.8 mL lysis buffer and 150 μL 20% SDS. In order to ensure biomass removal from the filter, 3 and 2 mm zirconium beads were added for bead beating using a vortex mixer at maximum speed. Filters were shaken for a total of 4 min, in two 2-min laps then subjected to chloroform extraction and processed as described above for Sterivex filters.
Total RNA was extracted from Sterivex filters (Table 1) using the mirVana Isolation kit (Ambion) (Shi et al., 2009; Stewart et al., 2010) protocol modified for Sterivex filters (Hawley et al., 2017). Briefly, after thawing the filter cartridge on ice, RNA later was removed by pushing through with a 3 ml syringe followed by rinsing with an additional 1.8 mL of Ringer’s solution and incubation at room temperature for 20 min with rotation. Ringer’s solution was evacuated with a 3 ml syringe followed by addition of 100 μl of 0.125 mg ml-1 lysozyme and incubation at 37°C for 30 min with rotation. Lysate was removed from the filter cartridge and subjected to organic extraction following the mirVana kit protocol. DNA removal and clean up and purification of total RNA were conducted following the TURBO DNA-free kit (Thermo Fisher) and the RNeasy MinElute Cleanup kit (Qiagen) protocols respectively. Total RNA concentration was determined by RiboGreen analysis (Life Technologies) prior to synthesis of first strand cDNA using the SuperScript III First-Strand Synthesis System for RT-qPCR (Invitrogen) according to manufacturer instructions. Total RNA was extracted from 0.4 to 2.7 μm filters (Table 1) as follows. The filter was cut in half using sterile scissors. One half was minced into smaller pieces and used for RNA extraction while the remaining half was stored at -80°C. Filter pieces were transferred to a 15 mL falcon tube followed by addition 1.8 ml MirVana Lysis Buffer and 100 μl of 0.125 mg ml-1 lysozyme. In order to ensure biomass removal from the filter, 3 and 2 mm zirconium beads were added for bead beating using a vortex mixer at maximum speed. Filters were shaken for 4 min in two, 2 min laps followed by incubation at 37°C for 30 min with rotation, then processed in the same way as described above for Sterivex filters.
Small Subunit Ribosomal RNA Sequencing
Extracted DNA and cDNA corresponding to 2.7, 0.4, and 0.22 μm filters from 165 to 185 m depth intervals (Table 1) was used to generate SSU rDNA and rRNA amplicon sequences with three domain resolution on the 454 pyrosequencing platform. PCR amplification procedures were carried out as previously described (Hawley et al., 2017). In brief, SSU rDNA and rRNA amplicon libraries (pyrotags) were generated by PCR amplification using multi-domain primers targeting the V6–V8 region of the SSU rRNA gene (Allers et al., 2013): 926F (5′-cct atc ccc tgt gtg cct tgg cag tct cag AAA CTY AAA KGA ATT GRC GG-3′) and 1392R (5′-cca tct cat ccc tgc gtg tct ccg act cag-<XXXXX>-ACG GGC GGT GTG TRC-3′). Primer sequences were modified by the addition of 454 A or B adapter sequences (lower case). In addition, the reverse primer included a 5 bp barcode designated<XXXXX> for multiplexing of samples during sequencing Twenty-five microliter PCR reactions were performed in triplicate and pooled to minimize PCR bias. Each reaction contained between 1 and 10 ng of target DNA, 0.5 μl Taq DNA polymerase (Bioshop, Inc.), 2.5 μL Bioshop 10x buffer, 1.5 μl 25 mM Bioshop MgCl2, 2.5 μL 10 mM dNTPs (Agilent Technologies) and 0.5 μL 10 mM of each primer. The thermal cycler protocol started with an initial denaturation at 95°C for 3 min and then 25 cycles of 30 s at 95°C, 45 s at 55°C, 90 s at 72°C and 45 s at 55°C. Final extension at 72°C for 10 min. PCR products were purified using the QiaQuick PCR purification kit (Qiagen), eluted in 25 μL, and quantified using the PicoGreen assay (Life Technologies). SSU rDNA and rRNA amplicons were pooled at 100 ng for each sample. Emulsion PCR and sequencing of the PCR amplicons was conducted on a Roche 454 GS FLX Titanium sequencer at the Department of Energy Joint Genome Institute (DOE-JGI), or the McGill University and Génome Québec Innovation Center.
A total of 1,027,601 small subunit ribosomal rDNA and rRNA pyrotags were processed together using the Quantitative Insights Into Microbial Ecology (QIIME) software package (Caporaso et al., 2010). Reads with length shorter than 200 bases, ambiguous bases, and homopolymer sequences were removed prior to chimera detection. Chimeras were detected and removed using chimera slayer provided in the QIIME software package. Sequences were then clustered into operational taxonomic units (OTUs) at 97% identity using UCLUST with average linkage algorithm. Prior to taxonomic assignment, singleton OTUs (OTUs represented by one read) were omitted, leaving 29,589 OTUs. Representative sequences from each non-singleton OTU were queried against the SILVA database release 111 using the BLAST algorithm (Altschul et al., 1990).
Statistical Analysis and Data Visualization
Statistical analyses were conducted using the R software package (R Core Team, 2013). Pyrotag data sets were normalized to the total number of reads per sample. Hierarchical cluster analysis (HCA) and non-metric multidimensional scaling (NMDS) were conducted to identify community compositional profiles associated with water column compartments using the pvclust (Suzuki and Shimodaira, 2015) and MASS (Venables and Ripley, 2002) packages with Manhattan Distance measures, and statistical significance to the resulting clusters as bootstrap score distributions with 1,000 iterations and NMDS stress value ≤ 0.05. Diversity indices (Shannon and alpha diversity) were calculated to identify changes in community structure based on filtration parameters using the vegan (Oksanen et al., 2015) package (Supplementary Figure 3 and Supplementary Table 1). Microbial community richness on HC selected samples was determined using the vegan (Oksanen et al., 2015) package. The OTU table was rarefied starting with 10 sequences to the total maximum number of sequences found in the dataset and 10 iterations per sample were calculated with 100 sequences between each step (Supplementary Figure 4). Non-parametric Friedman block tests were conducted to determine the significance of volume variation and filtration method on community structure using the base stats package (R Core Team, 2013). In addition, one-way ANOVA was conducted to test the significance of filter combinations on taxa relative abundance using the ggpbur package (Kassambara, 2017).
Multi-level indicator species analysis (ISA) was conducted to identify OTUs specifically associated with different experimental parameters based on groups resolved in HCA using the indicspecies package (De Caceres and Legendre, 2009). The ISA/multi-level pattern analysis calculates p-values with Monte Carlo simulations and returns indicator values (IVs) and p-values with α ≤ 0.05. The IVs range between 0 and 1, where indicator OTUs considered in the present study for further community analysis shown an IV ≥ 0.7 and p-value ≤ 0.001. ISA groups abundance was visualized as dot plots using the bubble.pl pearl script2. Taxonomic distribution of identified OTUs was visualized using the ggplot2 (Wickham, 2009) package. The total rRNA:rDNA ratios were calculated for the subset of matching samples (165 m 250 mL and 2 L on-ship 0.22 μm filters with in-line 0.4 μm pre-filtration, and 185 m 500 mL and 2 L on-ship 0.22 μm filters with in-line 0.4 μm pre-filtration) to account for variation in taxon abundance in the DNA pool (Frias-Lopez et al., 2008; Stewart et al., 2012b) and compared for a subset of microbial groups to explore how filtration parameters influence recovery of potentially active OTUs. We then selected OTUs based on ISA results and their shifts in abundance among filtering conditions.
Data Deposition
SSU rDNA and rRNA pyrotag sequences have been submitted to the National Center for Biotechnology Information (NCBI) under BioSample Nos. SAMN05392373–SAMN05392466.
Results
Water Column Conditions
Samples were collected during a stratification period characteristic of summer months (June–August) in SI (Carter, 1932, 1934; Herlinveaux, 1962; Zaikova et al., 2010). Below 150 m, water column CTD O2 concentrations were below < 3 μM, consistent with previous observations of water column anoxia during peak stratification (Figure 1A and Supplementary Table 2). In addition, increasing concentrations of H2S (13.95 μM) and NH4+ (6.1 μM) at 185 m were also observed, indicating anoxic and sulfidic conditions in deep basin waters. NO3- and NO2- concentrations peaked at 150 m reaching 12 and 0.6 μM, respectively. Phosphate concentrations ranged between 4.5 and 5.8 μM from 150 to 185 m, and SiO2 concentration peaked at 185 m reaching 110 μM (Supplementary Table 2).
Benchmarking Workshop and Saanich Inlet Time-Series Results
We evaluated microbial community structure using 521 time-series samples traversing the SI water column (Supplementary Figure 2) and 29 samples collected during the workshop using rDNA pyrotag sequences to compare and cross-calibrate in situ sampling with the McLane PPS system and bottle sampling methods. Different water volumes from the same depth interval and filtration method showed no significant difference (p = 0.1405 and p = 0.2545, respectively) in richness based on Friedman block test results and were therefore treated as pseudo-replicates. NMDS indicated workshop samples clustered together primarily with high-resolution suboxic and anoxic samples from 165 to 200 m depth intervals (0.22 μm Sterivex filters without pre-filtration) collected during summer months (Figure 2A). Similarities between time-series and workshop samples provided an internal check on experimental design and a rationale for examining more granular differences between community structure and potential activity resulting from different filtration parameters. Workshop samples collected at 165 and 185 m depth intervals formed three groups in NMDS analyses associated with on-ship 0.4 μm filters (group I), on-ship 0.22 μm filters with either 0.4 or 2.7 μm in-line pre-filtration (group II), and in situ 0.4 μm filters (group III) (Figure 2B). For the most part, samples within groups partitioned by depth. Consistent with NMDS, HCA resolved three groups (AU ≥ 70, 1000 iterations) associated with on-ship 0.4 μm filters (group I), on-ship 0.22 μm filters with in-line 0.4 μm pre-filters (group II), and in situ filtration 0.4 μm filters (group III) (Figure 3A). Within each filtration group, samples partitioned primarily by depth.
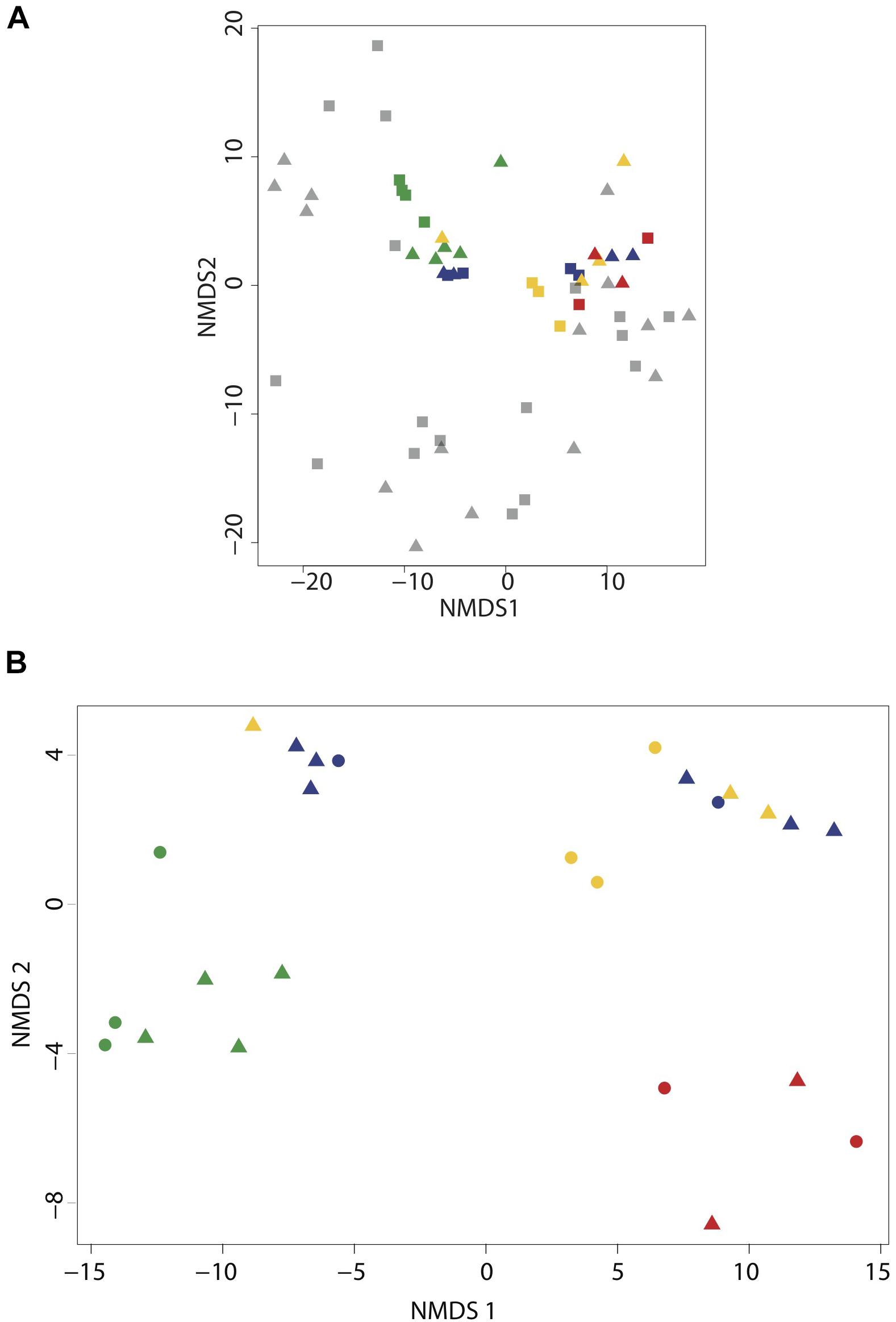
Figure 2. Cluster analysis of small subunit ribosomal RNA (SSU rDNA) gene pyrotag data for the Saanich Inlet time-series (2006–2011) and SCOR workshop samples collected at 165 and 185 m. (A) NMDS based on Manhattan distance (1000 iterations) of time-series and SCOR pyrotag data showing microbial community partitioning based on oxygen gradients spanning suboxic (20–1 μmolO2 kg-1) and anoxic-sulfidic (<1 μmol O2 kg1). (B) NMDS based on Manhattan distance (1000 iterations) of SCOR pyrotag data showing microbial community partitioning based on filtering conditions. In both biplots samples are depicted by depth (165 m = square and 185 m = triangle) and filter type [PPS in situ 0.4 μm (red), MPP on-ship 0.4 μm (green), 0.22 μm with in-line 0.4um prefilters (yellow), 0.22 μm with in-line 2.7 μm prefilters (blue), and time-series 0.22 μm (black)].
Size-Fractionation Effects on Community Structure
Based on NMDS and HCA results, we focused on changes in OTU relative abundance and taxon identity between groups. Microbial community structure was primarily comprised of OTUs (>0.1% relative abundance) affiliated with ubiquitous OMZ taxa including Marine Group A, SAR11, SAR324, SUP05 (Field et al., 1997; Fuhrman and Davis, 1997; Brown and Donachie, 2007; Tripp et al., 2008; Lam et al., 2009; Walsh et al., 2009; Zaikova et al., 2010; Walsh and Hallam, 2011; Wright et al., 2012) as well as Bacteroidetes, Desulfobacterales, and Euryarchaeota (Figure 3B). Interestingly, several of these groups were not detected in on-ship 0.4 μm pre-filter samples but were recovered from in-line 0.22 μm filters (group II). These included Marine Benthic Group E and Halobacteriales, SAR406 within the Marine Group A, Methylophilales within the Betaproteobacteria, Desulfuromonadales, and Desulfarculales within the Deltaproteobacteria and SUP05 within the Gammaproteobacteria. Conversely, Acidimicrobiales within the Actinobacteria, Cyanobacteria, Rhodobacterales within the Alphaproteobacteria, OM190 within the Planctomycetes, and eukaryotic phyla including Cnidaria and Arthropoda within the Opisthokonta and Diatoms within the Stramenopiles were detected in on-ship 0.4 μm filter samples but not recovered on in-line 0.22 μm filters or in situ 0.4 μm filters. Eukaryotic phyla affiliated with Alveolata were recovered on 0.22 μm filter samples with in-line 0.4 μm pre-filters (group I) and Phycisphaerales within the Planctomycetes were detected in in situ 0.4 μm filter samples (group III), respectively (Figure 3B).
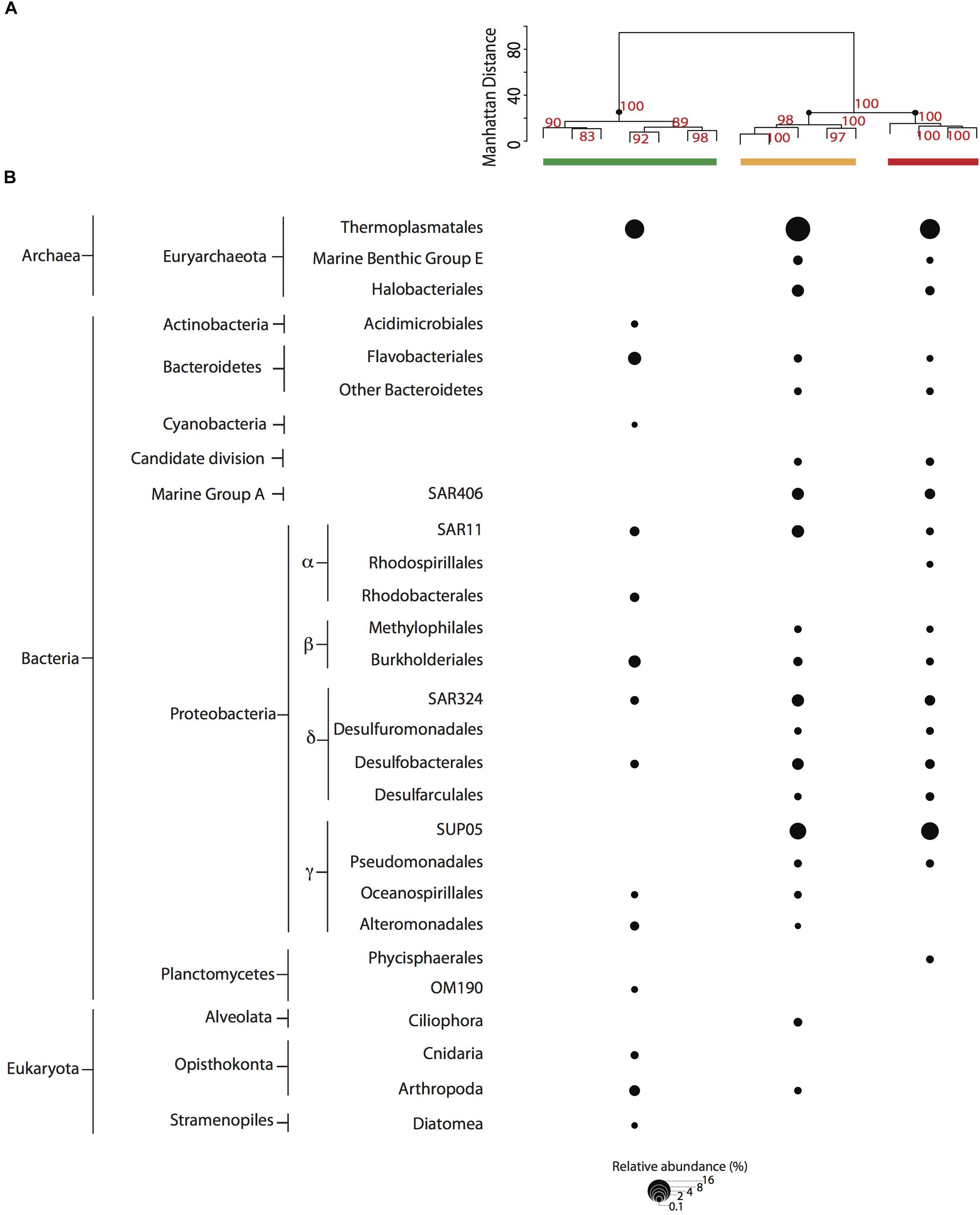
Figure 3. (A) Cluster analysis (AU > 70, 1000 iterations) for 0.4 μm filter fractions. Samples are depicted with a colored bar by filter type [PPS in situ 0.4 μm (red), and MPP on-ship 0.4 μm (green), and 0.22 μm with in-line 0.4 μm prefilters (yellow)]. (B) Abundant taxa (>0.1% relative abundance from total reads in sample) observed among filter combinations for PPS in situ 0.4 μm (red), and MPP on-ship 0.4 μm (green) and 0.22 μm with in-line 0.4 μm prefilters (yellow). The size of dots depicts the relative abundance for each taxa as indicated in key.
Filtration methods, including the use of different pre-filters, resulted in a significant source of variation (p < 0.001) for the relative abundance of several bacterial phyla including Bacteroidetes, Deferribacteres, Alpha-, Delta- and Gamma-proteobacteria, Planctomycetes, archaeal phyla including Thaumarchaeota, and eukaryotic phyla including Opisthokonta and Rhizaria (Figure 4 and Table 2). For example, the relative abundance of Bacteroidetes (Flavobacteriales), Alphaproteobacteria (SAR11, Rhodobacterales and Rhodospirillales) and Opisthokonta (Maxillopoda) associated with on-ship 0.4 μm filters increased fivefold compared to 0.22 μm filters with in-line 0.4 μm pre-filters and in situ 0.4 μm filters, while the relative abundance of Deferribacteres, Deltaproteobacteria (SAR324, Desulfobacterales and Desulfarculales) and Gammaproteobacteria (Oceanospirillales mainly affiliated with SUP05, Pseudomonadales and Alteromonadales) associated with on-ship 0.4 μm filters decreased fivefold compared to 0.22 μm filters with in-line 0.4 μm pre-filters and in situ 0.4 μm filters (Figure 4). Conversely, the relative abundance of Planctomycetes (Phycisphaerales and OM190) associated with in situ 0.4 μm filters increased fivefold compared to on-ship 0.22 μm filters with in-line 0.4 μm pre-filters and 0.4 μm filters (Figure 4).
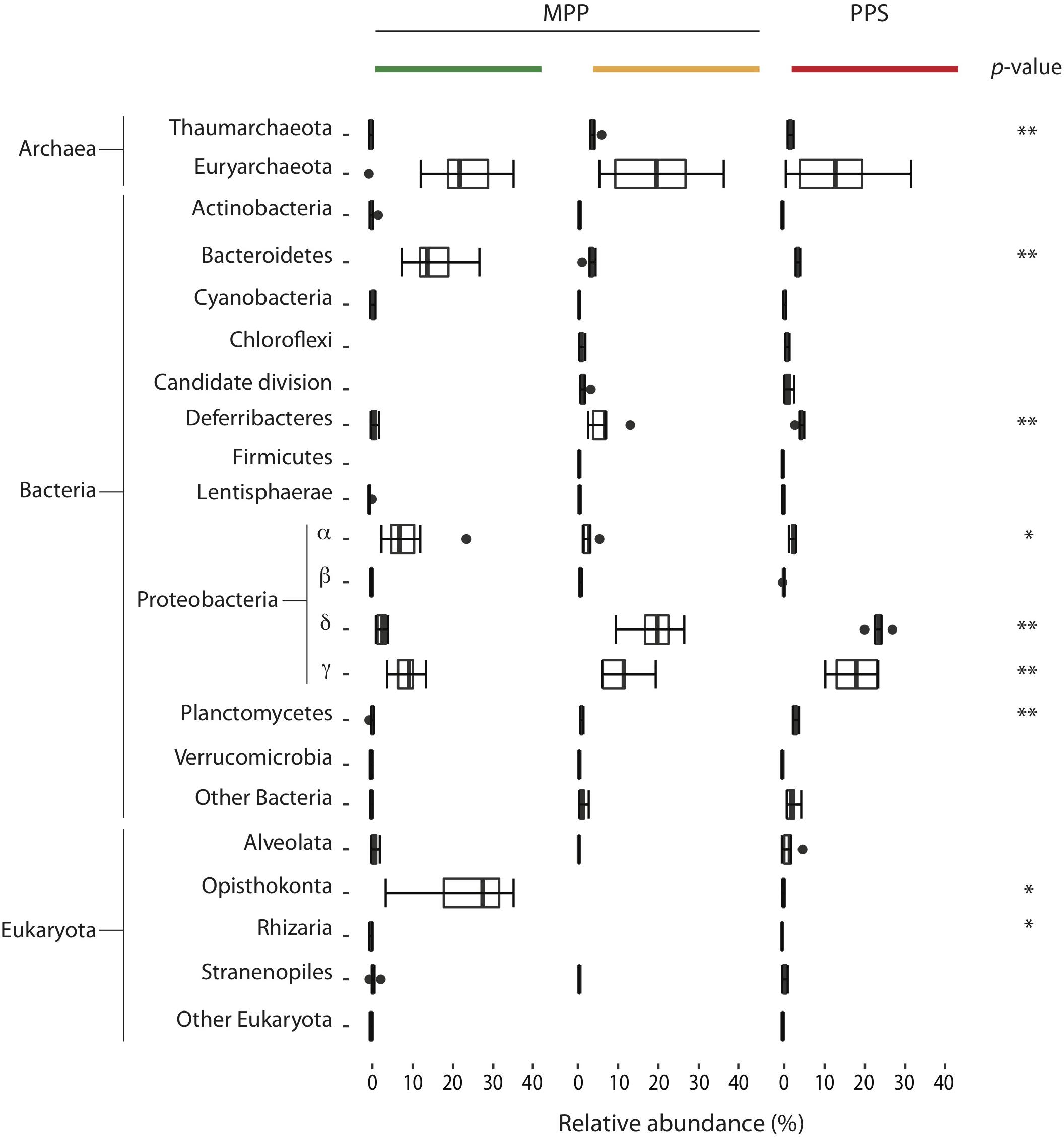
Figure 4. Community shifts occurred among and within filter combinations for PPS in situ 0.4 μm (red), and MPP on-ship 0.4 μm (green), and 0.22 μm with in-line 0.4 μm prefilters (yellow). The size of each box represents the average of the percentage of relative abundance throughout the water column over this period. For both plots, extended dashed lines (whiskers) represent at the base the lower and upper quartiles (25 and 75%) and at the end the minimum and maximum values encountered. The middle line represents the median. p-Values for significant relative abundance shifts among filter groups are depicted as ∗p < 0.01 and ∗∗p < 0.001 aside corresponding taxa.
Together these results indicate that filter selection and in-line positioning can introduce bias into microbial community structure data and reinforce the idea that filtration methods should be taken into consideration more carefully when interpreting microbial count data.
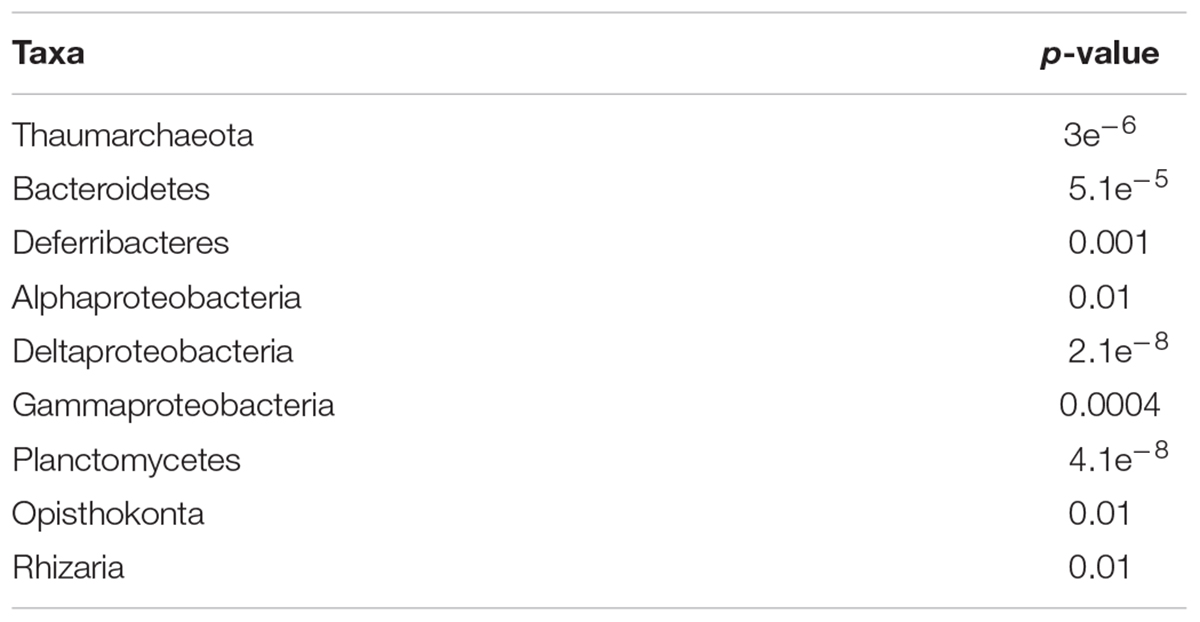
Table 2. p-values for taxa showing significant relative abundance shifts between filter conditions and filtration methods.
Size Fractionation Effects on Indicator OTUs (DNA Analyses)
To identify OTUs associated with specific filtration methods we conducted multi-level ISA on HCA groups I–III. As expected, resulting indicator OTUs varied with respect to filtration methods used (Figure 5). The largest differences with respect to indicators were detected between in situ and on-ship 0.4 μm filter samples. Indicator OTUs detected in on-ship 0.4 μm filter samples were mostly affiliated with bacterial phyla including Actinobacteria, Bacteroidetes, Cyanobacteria, Deferribacteres, Proteobacteria, and Verrucomicrobia, archaeal phyla including Euryarchaetoa, and eukaryotic phyla including Alveolata, Opisthokonta, Rhizaria, and Stramenopiles (Figure 5). Indicator OTUs detected in in situ 0.4 μm filter samples were mostly affiliated with bacterial phyla including Candidate divisions (WS3, OD1, and BRC1), Chloroflexi, Deferribacteres, Firmicutes, Lentisphareae, Nitrospirae, Alpha-, Beta-, Delta- and Gamma-proteobacteria, and Planctomycetes, archaeal phyla including Euryarchaeota, and eukaryotic phyla including Alveolata, Excavata, and Opisthokonta (Figure 5). Indicator OTUs detected in 0.22 μm filter samples with in-line 0.4 μm pre-filter were mostly affiliated with bacterial phyla including Deferribacteres and Bacteroidetes, and archaeal phyla including Euryarchaeota (Figure 5). The differences observed between in situ and on-ship indicator OTUs reinforce the effect of size fractionation on microbial community structure and raise important questions about metabolic reconstruction efforts based solely on on-ship filtration methods.
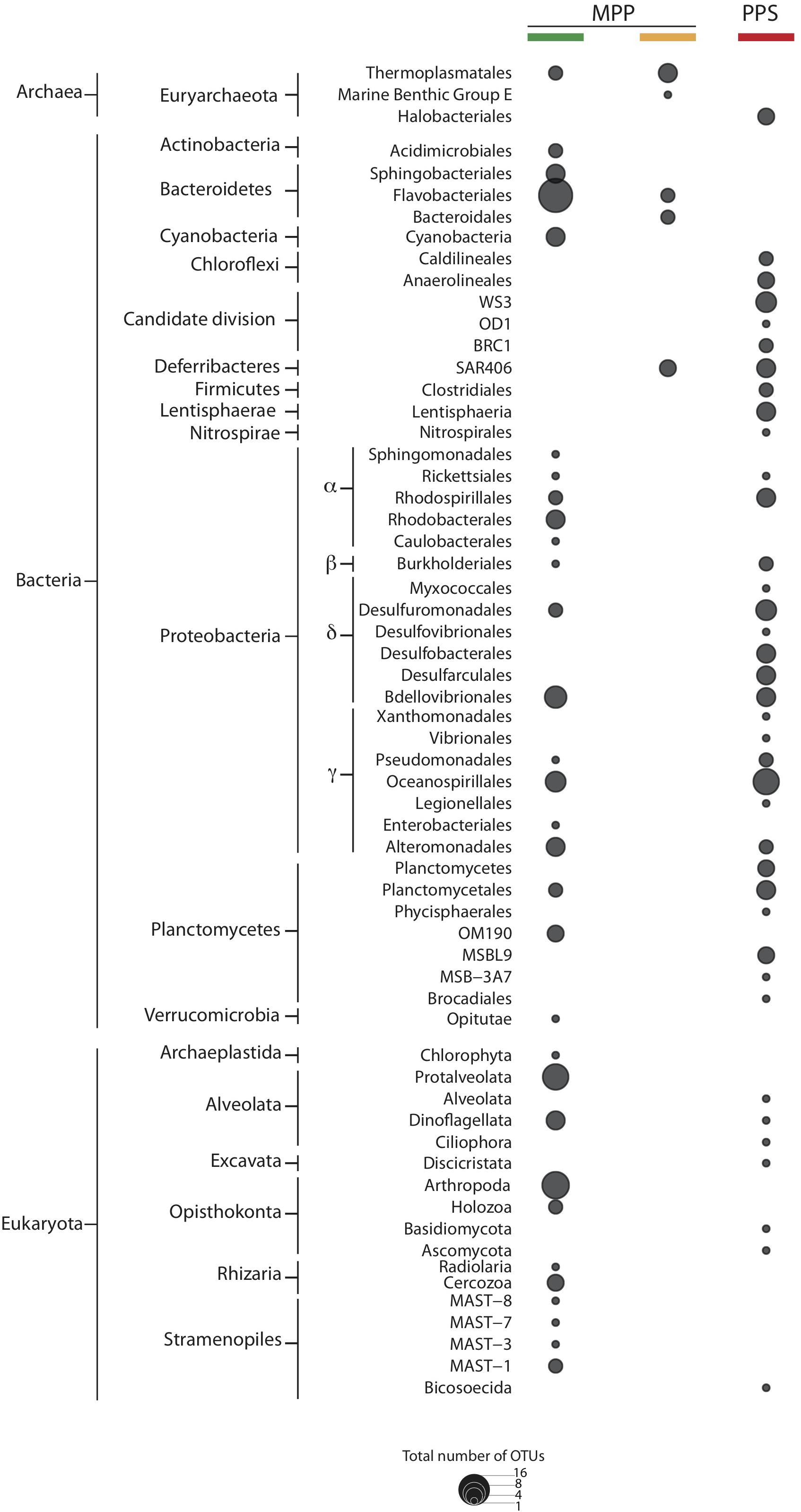
Figure 5. Indicator OTUs for filter groups PPS in situ 0.4 μm (red), and MPP on-ship 0.4 μm (green), and 0.22 μm with in-line 0.4 μm prefilters (yellow). The size of dots depicts the total number of indicator OTUs affiliated to specific taxa.
Size-Fractionation Effects on Expressed OTUs Within Specific Populations (rRNA Analyses)
To further evaluate the impact of size fractionation on detection of active microbial groups we compared SSU rRNA:rDNA ratios of OTUs between 0.4 μm filters collected and preserved in situ vs. on-ship 0.22 μm filters with in-line 0.4 μm pre-filters. We focused on OTUs exhibiting ratios ≥ 1 as a proxy for cellular activity (Blazewicz et al., 2013). Ratios for Candidate divisions, Desulfobacterales, SUP05, Phycisphaerae, and Halobacteria were highest in 0.4 μm in situ filter samples while SAR11, Rhodospirillales, Methylophilales and Burkholderiales within Betaproteobacteria, and Verrucomicrobia and eukaryotic phyla affiliated with Alveolata, Opisthokonta, Rhizaria, and Stramenopiles were highest in on-ship 0.22 μm filter samples with in-line 0.4 μm pre-filtration (Supplementary Figure 5).
We detected OTUs affiliated with SUP05, Marine Group A, SAR11 and SAR324 that showed ratios ≥ 1, with differential expression between in situ and on-ship filters. For instance, a total of 4 SUP05 OTUs with ratios ranging from 1 to 2, and 3 SAR324 OTUs with ratios equal to 3 were exclusively detected in 0.4 μm in situ filter samples (Figure 6). We also observed six Marine Group A OTUs with ratios equal to 2 in on-ship 0.22 μm filter samples with in-line 0.4 μm pre-filtration and 1 exclusively active in situ OTU (Figure 6). Interestingly, we observed eight SAR11 OTUs with ratios ranging from 1 to 3 exclusively in on-ship 0.22 μm filter samples with in-line 0.4 μm pre-filtration (Figure 6). Candidate divisions BCR1 and WS3, Delta- and Gammaproteobacteria, and Planctomycetes OTUs also manifested higher ratios in 0.4 μm in situ filter samples than in on-ship samples (Figure 7). These differences showed some depth specificity. For example, we observed BCR1 and WS3 OTUs with high ratio values at 165 m while Desulfovibrionales and Desulfarculales within the Deltaproteobacteria had the highest ratio values at 185 m (Figure 7). Similarly, most OTUs affiliated with Planctomycetes (Phycisphaerae, OM190, Brocadiales, and Plactomycetales) had the highest ratio values at 185 m (Figure 7). In contrast, Flavobacteriales within Bacteroidetes and Alphaproteobacteria had higher ratio values in on-ship 0.22 μm filter samples with in-line 0.4 μm pre-filtration than 0.4 μm in situ sampled at both 165 and 185 m (Figure 7).
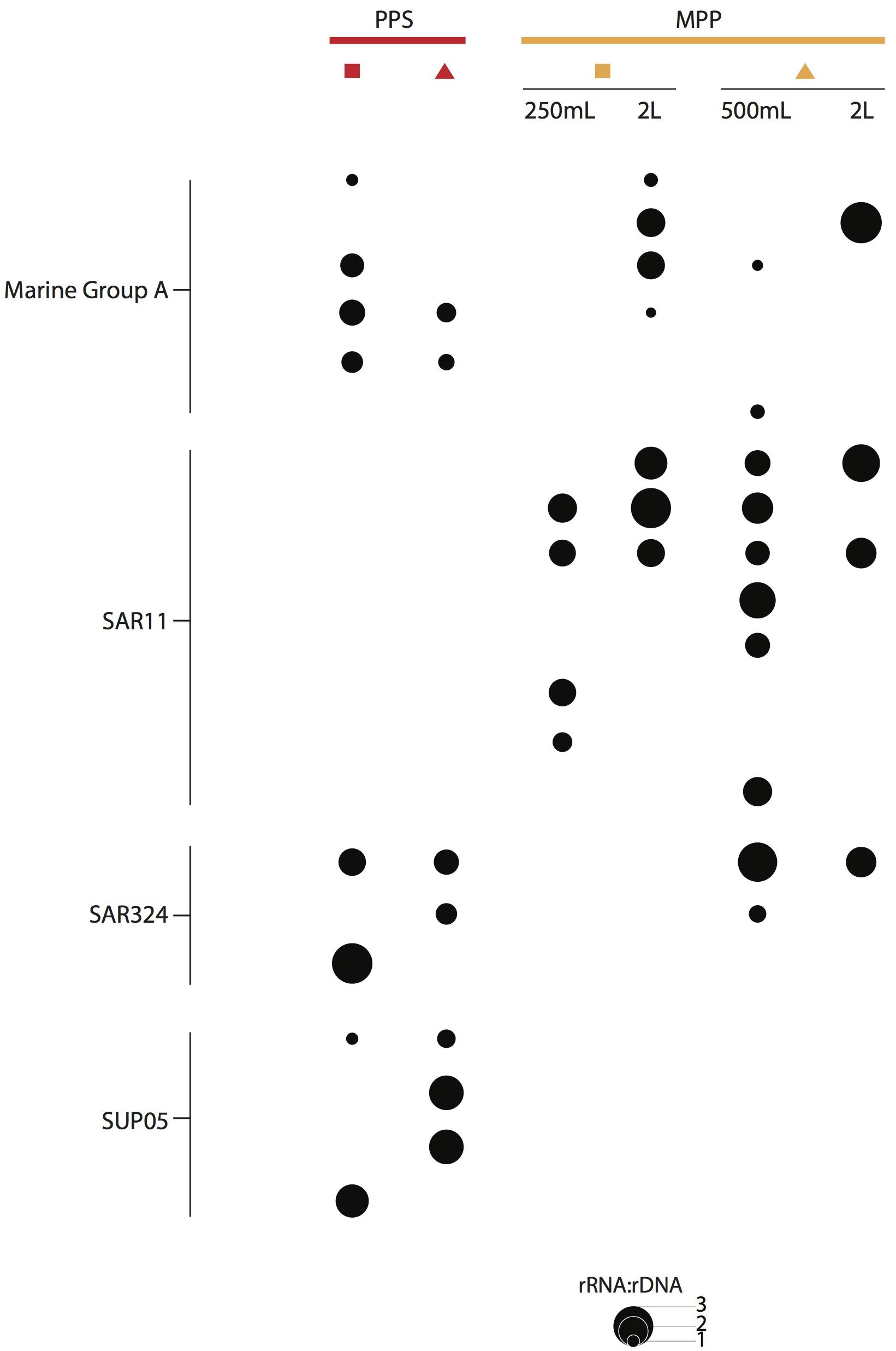
Figure 6. RNA:DNA ratio for abundant and ubiquitous taxa in OMZs, i.e., SUP05, SAR406, SAR11, and SAR324, observed at PPS in situ 0.4 μm (red) and MPP on-ship 0.22 μm with in-line 0.4 μm prefilters (yellow). The size of dots depicts ratio values for individual OTUs as indicated on plot size key.
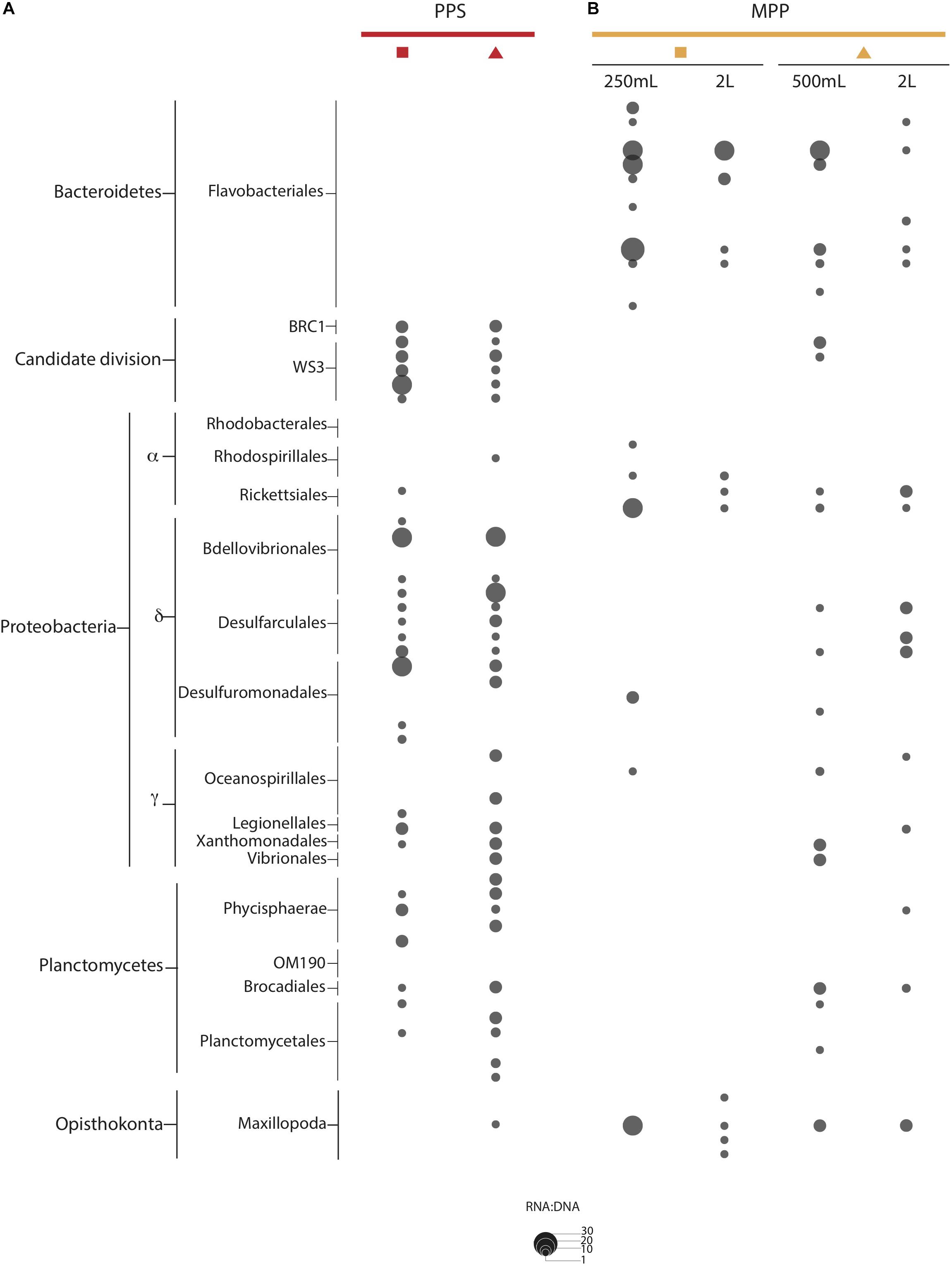
Figure 7. RNA:DNA ratio for indicator OTUs observed at PPS in situ 0.4 μm (A: red) and MPP on-ship 0.22 μm with in-line 0.4 μm prefilters (B: yellow). The size of dots depicts ratio values for individual OTUs as indicated on plot size key.
Discussion
In this study we used SSU rDNA and rRNA count data generated to determine the effects of collection and filtration methods on microbial community structure and potential activity in the anoxic water column of Saanich Inlet (SI). Observed differences in microbial community structure and potential activity associated with in situ versus on-ship size fractionation suggest potential sources of error when linking field processes to microbial agents based on genomic sequence information in isolation. In particular, in situ results detected several microbial groups implicated in the sulfur-cycle that are underrepresented in public amplicon and shotgun sequencing data sets. Overall, results from this study provide useful information on how different sampling methods can contribute to bias in experimental outcomes and reinforce the need for more integrated studies based on standardized sampling protocols that increasingly incorporate in situ measurements.
Microbial Community Shifts Associated With Filter Type and Volume
For in situ sampling we used GFF filters certified with the instrument and the recommended filtration volume in duplicate. For on-ship sampling we adopted standard sampling protocols using filters available to us at the time including 0.4 μm polycarbonate or 2.7 μm GF/D pre-filters in-line with a 0.22 μm Sterivex polycarbonate filter cartridge. While we cannot strictly rule out potential membrane effects on the resulting microbial community composition profiles associated with GFF versus polycarbonate filters at the same size cut-off, a previous study by Djurhuus et al. (2017) suggests a minimal effect based on the nucleic acid extraction methods used. The use of different volumes filtered for a given depth and filter combination was intended to test the significance of volume variation on microbial community composition. We set the maximum volume to be 2 L in order to keep filtering time on ship to a minimum and for samples to be comparable to previous time-series environmental observations. Water volumes between 250 ml and 2 L from the same depth interval and filtration method showed no significant difference in richness based on Friedman block test results.
Microbial Community Shifts Associated With Size Fractionation
Understanding how microorganisms interact within the ocean at different scales is integral to linking microbial food webs to nutrient and energy flow processes (Azam and Malfatti, 2007). Particles play a salient role in structuring microbial community interactions and the interplay between “particle-associated” and “free-living” microbiota creates a dynamic metabolic network driving biogeochemical transformations (Smith et al., 1992; DeLong et al., 1993; Crump et al., 1999; Simon et al., 2002; Grossart, 2010; Ganesh et al., 2014). Previous observations from OMZ waters implicate particle maxima as hotspots for metabolic coupling (Garfield et al., 1983; Naqvi et al., 1993; Whitmire et al., 2009; Ganesh et al., 2014). However, the definition of “particle-associated” versus “free-living” can sometimes seem arbitrary and the degree to which microorganisms alternate between these two fractions in different water compartments is not firmly established. Typically, anything > 0.4 μm has been considered particle associated (Azam and Malfatti, 2007) although most studies use different combinations of 0.2–1.6 and 2.7 μm cut-offs to concentrate microbial biomass. Here, we compared 0.4 μm in situ filtration without a pre-filtration step to on-ship 0.22 μm filtration with in-line 0.4 μm pre-filtration to better evaluate “particle-associated” versus “free-living” fractions.
The core microbial community detected in situ versus on-ship was similar to time-series observations in suboxic-anoxic water column compartments (165–185 m) during summer months. However, at a more granular OTU level important differences in community structure and potential activity for a number of microbial groups were resolved that reinforce and expand on previous size fractionation studies in open ocean OMZs. For example, Padilla et al. (2015) have shown that mode and magnitude of sampling bias depends on filter type and pore size, particle load, and community complexity. Our results expand on these observations indicating that wire time and filtration duration likely impacted richness and particle size, as did potential bottle effects due to settling when processing on-ship samples (Supplementary Figures 3, 4). Several studies have shown that particles can settle in sampling bottles on timescales relevant to on-ship processing (Gardner, 1977; Suter et al., 2016).
With respect to size fractionation, community structure differences were driven by shifts in abundance and activity of many known microorganisms. For example, as observed in the ETNP OMZ, OTUs affiliated with Deferribacteres were enriched in the smaller size fraction (<0.4 μm) consistent with an autotrophic lifestyle (Ganesh et al., 2014). Similarly, indicator OTUs affiliated with Bacteroidetes (Bacteroidales and Flavobacteriales), Lentisphareae, Deltaproteobacteria (Myxococcales and Desulfobacterales), Planctomycetes, and Verrucomicrobia were enriched in the larger size fraction (>0.4 μm) as observed in both ETNP and ETSP OMZs, consistent with attachment to sinking aggregates or zooplankton (Crump et al., 1999; Simon et al., 2002; Eloe et al., 2011; Allen et al., 2012; Fuchsman et al., 2012; Ganesh et al., 2014; Padilla et al., 2015). These similarities transcended domain boundaries with eukaryotic phyla including Dinoflagellata (Alveolata), Radiolaria (Rhizaria), and Syndiniales (Stramenopiles) enriched in the 0.4 μm filter samples (Guillou et al., 2008; Duret et al., 2015).
With respect to in situ versus bottle collection methods, previous studies have identified changes in community gene expression profiles (Feike et al., 2012; Stewart et al., 2012a) and process rate measurements (Taylor and Doherty, 1990; Stewart et al., 2012a; Taylor et al., 2015; Edgcomb et al., 2016). Here we identified changes in microbial community richness and structure (Figure 4 and Supplementary Figure 4) that potentially explain variance in gene expression or process rates. For example, indicator OTUs detected in in situ samples suggests a potential unrecognized role for sulfate-reducers and candidate divisions WS3, OD1, and BRC1 in the SI water column. Previous studies have implicated WS3 and OD1 metabolism in sulfur cycling and methanogen provisioning (Kirkpatrick et al., 2006; Wrighton et al., 2012). Similarly, Desulfobacterales and Desulfovibrionales, are commonly underestimated in abundance in OMZs waters (Suter et al., 2016) as they are more prevalent on particles that likely settle during on-ship processing.
Working in the Cariaco Basin OMZ, Suter et al. (2016) provide a compelling description of bottle settling rates (approximately 12 min for a 1 mm particle to sink into below-spout space of an 8 L Niskin bottle or 18 min for a 12 L bottle) that can result in sampling bias. On-ship processing during the workshop took between 20 and 30 min. Shipboard processing times can often be even longer than this. In addition, wire-time and turbulence associated with sampling moment and vibration (Suter et al., 2016), and filtration across the membrane (Duret et al., 2015) can impact particle size and stability in bottles, e.g., production of smaller particles derived from larger aggregates. Based on sinking rates estimated in the Cariaco Basin it is possible that observed community structure differences between in situ and on-ship samples in SI could be explained by a combination of particle settling and turbulence associated with sample collection and filtration. This could affect how we perceive the microbial metabolic network with respect to transient spatial interactions that are altered or disrupted using on-ship methods.
Implications of Size Fractionation for Inferring Microbial Activity in OMZs
In contrast to only examining rDNA sequences, combining those with analysis of rRNA sequences can provide a robust proxy for past, and present or emerging cellular activities (Blazewicz et al., 2013) that can inform hypotheses related to life strategies and metabolic interactions within microbial communities (Lepp and Schmidt, 1998; Barnard et al., 2013). Here, we considered rRNA:rDNA ratio values ≥ 1 as an indicator for potentially active microbial community members. While, using ratios to infer activity at higher taxonomic levels, e.g., Phylum, Class, Order, can promote inconsistent results (Blazewicz et al., 2013; Ganesh et al., 2015), focusing on the OTU level can identify ecologically relevant populations with the potential to play integral roles in nutrient and energy cycling within the ecosystem under study. For example, SUP05 has been determined to be an abundant member of the SI microbial community comprising between 20 and 30% of total bacteria at 165 and 185 m, respectively (Walsh et al., 2009; Zaikova et al., 2010; Walsh and Hallam, 2011). We detected 85 OTUs affiliated with SUP05 based on rDNA sequences. However, only 4 had rRNA:rDNA ratio values > 1 indicating population level variation in potential activity.
Consistent with previous observations in the ETSP using metatranscriptomic data (Padilla et al., 2015), examination of the taxonomic affiliation of indicator OTUs produced using in situ versus on-ship methods identified differences between the active microbial community in samples. Some candidate divisions recovered in in situ samples have not been previously well-described in the SI water column based on rDNA sequences due to their low abundance (<0.1%). Interestingly, the rRNA:rDNA ratios observed for indicator WS3 and BCR1 OTUs (ratios equal to 7 and 2, respectively) were greater than those observed for OTUs affiliated with ubiquitous and abundant taxa, including SUP05. Similar observations were made for OTUs affiliated with Deltaproteobacteria, Chloroflexi, Firmicutes, Lentisphaera, Nitrospina and Marine Group A, reinforcing the idea that multi-omic sequences and process rate measurements sourced from on-ship samples have the potential to underestimate the contribution of some active microbial groups present in the water column. Such groups may be sensitive to settling, turbulence or other factors including oxygen exposure, necessitating in situ sampling to reveal their contributions to the metabolic network.
Hawley et al. (2014) used metaproteomics to develop a conceptual model of coupled carbon, nitrogen and sulfur cycling. Louca et al. (2016) incorporated these ideas into a numerical model integrating multi-omic sequence and geochemical information to predict metabolic fluxes and growth yields under near steady-state conditions. Both conceptual and numerical models were based on interactions between Thaurmarchaeota, SAR11, SUP05 and Planctomycetes (Walsh et al., 2009; Zaikova et al., 2010; Walsh and Hallam, 2011; Wright et al., 2012). Although, the metabolic potential of WS3, OD1 and sulfate-reducing Deltaproteobacteria in the SI water column remains to be determined, the potential role of these groups at the nexus of sulfur cycling and methanogenesis (Kirkpatrick et al., 2006) presents an opportunity for new hypothesis development and testing to integrate these groups into prevailing conceptual and numerical models for coupled biogeochemical cycling.
Conclusion
As the research community transitions away from descriptive studies of marine microorganisms to more quantitative comparisons at ecosystem scales integrating multi-omic information with process rates and modeling, the need for standards of practice that reduce sampling bias becomes increasingly important.
Currently most microbial community studies use a combination of bottle sampling and filtration to collect biomass for nucleic acid extraction and sequencing. However, the specific details of how samples are collected including wire and bottle time, filter type, and method can have a discernable impact on resulting microbial community composition profiles. This is an important consideration when comparing data sets between studies and when trying to link microbial agents to defined field processes, e.g., denitrification, sulfur oxidation, carbon fixation, etc. Based on our analysis of both in situ and on-ship sample collection and processing methods the following practical considerations can be identified. For amplicon-based studies, use a consistent filtration volume between 1 and 2 L and record the precise volume filtered to back calculate nucleic acid yield per unit volume of water collected. Depending on the size fraction you are interested in profiling consult the literature to determine a consensus filter type and method. For cross-scale studies use the same filtration method for all locations. Take advantage of current library production methods that allow for low-input samples, and use the coverage provided by next generation sequencing platforms to sample with depth and replication. When possible, consider using single-cell amplified genome (SAG) approaches when more functional information is required (Stepanauskas, 2015). Samples for SAG sequencing are easy to replicate and do not require more than a 1–2 ml per sample that can be stored for extended periods of time at -80°C (Rinke et al., 2014). Most important, minimize wire time including on-ship bottle sampling duration. Particles are settling as the waters in the bottle rise. Consider inverting the bottle before collecting waters for more even biomass sampling or filtering samples directly in the water column.
In situ sampling approaches have the potential to limit many biases by providing a more authentic representation of microbial activity than on-ship sampling methods. Several promising devices such as the PPS (Edgcomb et al., 2016), Environmental Sample Processor (ESP) (Jones et al., 2008; Preston et al., 2009; Ottesen et al., 2011; Robidart et al., 2014), Automatic Flow Injection Sampler (AFIS) (Feike et al., 2012), and the autonomous vertical sampling vehicle Clio (Jakuba et al., 2014) have been developed with the potential to support in situ sampling and direct fixation of samples under a variety of operational scenarios. For example, recent studies with the ESP have enabled dynamic intermittent sampling during light dark cycles in surface waters revealing conserved patters of gene expression on ocean basin scales (Ottesen et al., 2014; Aylward et al., 2015). Although community adoption of these new technologies remains in early stages due in part to accessibility, price point, and operating constraints, these devices and their “descendants” likely reflect the future of microbial sampling in the ocean given their autonomous and programmable designs extensible to time series or event response monitoring. Looking forward, we recommend replicated studies of different in situ sampling technologies that incorporate multi-omic sequencing and process rate measurements focused on coupled carbon, nitrogen, and sulfur cycling in coastal and open ocean OMZs.
Author Contributions
MT-B collected and processed samples, analyzed data, and wrote the manuscript under SH supervision. AM and MS collected and processed samples and assisted data analysis. MP, CT, and VE collected and processed PPS samples. KT processed samples for sequencing. CM collected and processed samples. PL, OU, J-HH, KJ, VE, SC, and SH organized the SCOR 144 working group workshop, collected and processed samples, and provided input for data analysis and manuscript development.
Funding
This work was performed under the auspices of the Scientific Committee on Oceanographic Research (SCOR), the United States Department of Energy (DOE) Joint Genome Institute, an Office of Science User Facility, supported by the Office of Science of the United States Department of Energy under Contract DE-AC02- 05CH11231, the G. Unger Vetlesen and Ambrose Monell Foundations, the Tula Foundation-funded Centre for Microbial Diversity and Evolution, the Natural Sciences and Engineering Research Council of Canada, Genome British Columbia, the Canada Foundation for Innovation, and the Canadian Institute for Advanced Research through grants awarded to SH. McLane Research Laboratories and Connie Lovejoy contributed access to instrumentation for field work. Ship time support was provided by NSERC between 2007 and 2014 through grants awarded to SC, SH and Philippe Tortell MT-B was funded by Consejo Nacional de Ciencia y Tecnología (CONACyT) and the Tula Foundation.
Conflict of Interest Statement
The authors declare that the research was conducted in the absence of any commercial or financial relationships that could be construed as a potential conflict of interest.
Acknowledgments
We thank Captain Ken Brown and his crew for their engaged effort on every cruise aboard the RSV Strickland. We also thank past and present members of the Hallam, Crowe and Tortell labs and the many undergraduate trainees, aka minions, for contributions to cruise preparation and clean up, water filtration, DNA extraction and QC. We thank our sea-going technicians at UBC, Chris Payne and Larysa Pakhomova for their contributions to cruise preparation and sample analyses. Special thanks to Ed Urban at SCOR for administrative support and guidance and to the entire SCOR Working Group 144: Bess Ward (Chair), Sean Crowe, Virginia Edgcomb, Vernonique Garcon, Steven Hallam, KJ, Elsabe Julies, Phyllis Lam, Nagappa Ramaiah, Matthew Sullivan, OU and associate members: Mark Altabet, Annie Bourbonnais, Karen Casciotti, Francis Chan, David Conley, Robinson (Wally) Fulweiler, J-HH, David Karl, John Kaye, Konstantinos Kormas, SWA Naqvi, Nancy Rabalais, Mak Saito, Frank Stewart, RV-S, Jennifer Brum, and Jody Wright for their efforts to develop a community of practice for OMZ research.
Supplementary Material
The Supplementary Material for this article can be found online at: https://www.frontiersin.org/articles/10.3389/fmars.2019.00132/full#supplementary-material
Footnotes
- ^http://omz.microbiology.ubc.ca/page4/index.html
- ^http://hallam.microbiology.ubc.ca/LabResources/Software.html
References
Allen, L. Z., Allen, E. E., Badger, J. H., Mccrow, J. P., Paulsen, I. T., Elbourne, L. D. H., et al. (2012). Influence of nutrients and currents on the genomic composition of microbes across an upwelling mosaic. ISME J. 6, 1403–1414. doi: 10.1038/ismej.2011.201
Allers, E., Wright, J. J., Konwar, K. M., Howes, C. G., Beneze, E., Hallam, S. J., et al. (2013). Diversity and population structure of marine group A bacteria in the Northeast subarctic Pacific Ocean. ISME J. 7, 256–268. doi: 10.1038/ismej.2012.108
Altschul, S. F., Gish, W., Miller, W., Myers, E. W., and Lipman, D. J. (1990). Basic local alignment search tool. J. Mol. Biol. 215, 403–410. doi: 10.1016/S0022-2836(05)80360-2
Anderson, J. J., and Devol, A. H. (1973). Deep water renewal in Saanich Inlet, an intermittently anoxic basin. Estuar. Coast. Mar. Sci. 1, 1–10. doi: 10.1016/0302-3524(73)90052-2
Arrigo, K. R. (2005). Marine microorganisms and global nutrient cycles. Nature 437, 349–355. doi: 10.1038/nature04159
Aylward, F. O., Eppley, J. M., Smith, J. M., Chavez, F. P., Scholin, C. A., and Delong, E. F. (2015). Microbial community transcriptional networks are conserved in three domains at ocean basin scales. Proc. Natl. Acad. Sci. U.S.A. 112:5443. doi: 10.1073/pnas.1502883112
Azam, F., and Malfatti, F. (2007). Microbial structuring of marine ecosystems. Nat. Rev. Microbiol. 5, 966–966. doi: 10.1038/nrmicro1798
Barnard, R. L., Osborne, C. A., and Firestone, M. K. (2013). Responses of soil bacterial and fungal communities to extreme desiccation and rewetting. ISME J. 7, 2229–2241. doi: 10.1038/ismej.2013.104
Bijma, J., Pörtner, H.-O., Yesson, C., and Rogers, A. D. (2013). Climate change and the oceans – what does the future hold? Mar. Pollut. Bull. 74, 495–505. doi: 10.1016/j.marpolbul.2013.07.022
Blazewicz, S. J., Barnard, R. L., Daly, R. A., and Firestone, M. K. (2013). Evaluating rRNA as an indicator of microbial activity in environmental communities: limitations and uses. ISME J. 7, 2061–2068. doi: 10.1038/ismej.2013.102
Brown, M. V., and Donachie, S. P. (2007). Evidence for tropical endemicity in the Deltaproteobacteria marine group B/SAR324 bacterioplankton clade. Aquat. Microb. Ecol. 46, 107–115. doi: 10.3354/ame046107
Canfield, D. E., Stewart, F. J., Thamdrup, B., De Brabandere, L., Dalsgaard, T., Delong, E. F., et al. (2010). A cryptic sulfur cycle in oxygen-minimum-zone waters off the Chilean coast. Science 330, 1375–1378. doi: 10.1126/science.1196889
Caporaso, J. G., Kuczynski, J., Stombaugh, J., Bittinger, K., Bushman, F. D., Costello, E. K., et al. (2010). QIIME allows analysis of high-throughput community sequencing data. Nat. Methods 7, 335–336. doi: 10.1038/nmeth.f.303
Carter, N. M. (1932). The oceanography of the fjords of southern British Columbia. Fish. Res. Bd. Canada Prog. Rept. Pacific Coast. Sta. 12, 7–11.
Carter, N. M. (1934). Physiography and oceanography of some british columbia fjords. Proc. Fifth Pacific Sci. Cong. 1, 721–733.
Cram, J. A., Xia, L. C., Needham, D. M., Sachdeva, R., Sun, F., and Fuhrman, J. A. (2015). Cross-depth analysis of marine bacterial networks suggests downward propagation of temporal changes. ISME J. 9, 2573–2586. doi: 10.1038/ismej.2015.76
Crump, B. C., Armbrust, E. V., and Baross, J. A. (1999). Phylogenetic analysis of particle-attached and free-living bacterial communities in the columbia river, its estuary, and the adjacent coastal Ocean. Appl. Environ. Microbiol. 65, 3192–3204.
De Caceres, M., and Legendre, P. (2009). Associations between species and groups of sites: indices and statistical inference. Ecology 90, 3566–3574. doi: 10.1890/08-1823.1
DeLong, E. F., Franks, D. G., and Alldredge, A. L. (1993). Phylogenetic diversity of aggregate-attached vs. free-living marine bacterial assemblages. Limnol. Oceanogr. 38, 924–934. doi: 10.4319/lo.1993.38.5.0924
Diaz, R. J., and Rosenberg, R. (2008). Spreading dead zones and consequences for marine ecosystems. Science 321, 926–929. doi: 10.1126/science.1156401
Djurhuus, A., Port, J., Closek, C. J., Yamahara, K. M., Romero-Maraccini, O., Walz, K. R., et al. (2017). Evaluation of filtration and DNA extraction methods for environmental DNA biodiversity assessments across multiple trophic levels. Front. Mar. Sci. 4:314. doi: 10.3389/fmars.2017.00314
Duret, M. T., Pachiadaki, M. G., Stewart, F. J., Sarode, N., Christaki, U., Monchy, S., et al. (2015). Size-fractionated diversity of eukaryotic microbial communities in the Eastern Tropical North Pacific oxygen minimum zone. FEMS Microbiol. Ecol. 91:fiv037. doi: 10.1093/femsec/fiv037
Edgcomb, V. P., Taylor, C., Pachiadaki, M. G., Honjo, S., Engstrom, I., and Yakimov, M. (2016). Comparison of Niskin vs. in situ approaches for analysis of gene expression in deep Mediterranean Sea water samples. Deep Sea Res. Part II Top. Stud. Oceanogr. 129, 213–222. doi: 10.1016/j.dsr2.2014.10.020
Eloe, E. A., Shulse, C. N., Fadrosh, D. W., Williamson, S. J., Allen, E. E., and Bartlett, D. H. (2011). Compositional differences in particle-associated and free-living microbial assemblages from an extreme deep-ocean environment. Environ. Microbiol. Rep. 3, 449–458. doi: 10.1111/j.1758-2229.2010.00223.x
Feike, J., Jurgens, K., Hollibaugh, J. T., Kruger, S., Jost, G., and Labrenz, M. (2012). Measuring unbiased metatranscriptomics in suboxic waters of the central Baltic Sea using a new in situ fixation system. ISME J. 6, 461–470. doi: 10.1038/ismej.2011.94
Field, K. G., Gordon, D., Wright, T., Rappé, M., Urback, E., Vergin, K., et al. (1997). Diversity and depth-specific distribution of SAR11 cluster rRNA genes from marine planktonic bacteria. Appl. Environ. Microbiol. 63, 63–70.
Frias-Lopez, J., Shi, Y., Tyson, G. W., Coleman, M. L., Schuster, S. C., Chisholm, S. W., et al. (2008). Microbial community gene expression in ocean surface waters. Proc. Natl. Acad. Sci. U.S.A. 105, 3805–3810. doi: 10.1073/pnas.0708897105
Fuchsman, C. A., Staley, J. T., Oakley, B. B., Kirkpatrick, J. B., and Murray, J. W. (2012). Free-living and aggregate-associated Planctomycetes in the Black Sea. FEMS Microbiol. Ecol. 80, 402–416. doi: 10.1111/j.1574-6941.2012.01306.x
Fuhrman, J. A., and Davis, A. A. (1997). Widespread Archaea and novel bacteria from the deep sea as shown by 16S rRNA gene sequences. Mar. Ecol. Prog. Ser. 150, 275–285. doi: 10.3354/meps150275
Gallo, N. D., and Levin, L. A. (2016). “Chapter three - Fish ecology and evolution in the world’s oxygen minimum zones and implications of ocean deoxygenation,” in Advances in Marine Biology, ed. E. C. Barbara (Cambridge, MA: Academic Press), 117–198.
Ganesh, S., Bristow, L. A., Larsen, M., Sarode, N., Thamdrup, B., and Stewart, F. J. (2015). Size-fraction partitioning of community gene transcription and nitrogen metabolism in a marine oxygen minimum zone. ISME J. 9, 2682–2696. doi: 10.1038/ismej.2015.44
Ganesh, S., Parris, D. J., Delong, E. F., and Stewart, F. J. (2014). Metagenomic analysis of size-fractionated picoplankton in a marine oxygen minimum zone. ISME J. 8, 187–211. doi: 10.1038/ismej.2013.144
Gardner, W. D. (1977). Incomplete extraction of rapidly settling particles from water samplers1. Limnol. Oceanogr. 22, 764–768. doi: 10.4319/lo.1977.22.4.0764
Garfield, P. C., Packard, T. T., Friederich, G. E., and Codispoti, L. A. (1983). A subsurface particle maximum layer and enhanced microbial activity in the secondary nitrite maximum of the northeastern tropical Pacific Ocean. J. Mar. Res. 41, 747–768. doi: 10.1357/002224083788520496
Grossart, H.-P. (2010). Ecological consequences of bacterioplankton lifestyles: changes in concepts are needed. Environ. Microbiol. Rep. 2, 706–714. doi: 10.1111/j.1758-2229.2010.00179.x
Gruber, N. (2011). Warming up, turning sour, losing breath: ocean biogeochemistry under global change. Philos. Trans. A Math. Phys. Eng. Sci. 369:1980–1996. doi: 10.1098/rsta.2011.0003
Guillou, L., Viprey, M., Chambouvet, A., Welsh, R., Kirkham, A., Massana, R., et al. (2008). Widespread occurrence and genetic diversity of marine parasitoids belonging to Syndiniales (Alveolata). Environ. Microbiol. 10, 3349–3365. doi: 10.1111/j.1462-2920.2008.01731.x
Hawley, A. K., Brewer, H. M., Norbeck, A. D., Paša-Tolić, L., and Hallam, S. J. (2014). Metaproteomics reveals differential modes of metabolic coupling among ubiquitous oxygen minimum zone microbes. Proc. Natl. Acad. Sci. U.S.A. 111, 11395–11400. doi: 10.1073/pnas.1322132111
Hawley, A. K., Torres-Beltrán, M., Zaikova, E., Walsh, D. A., Mueller, A., Scofield, M., et al. (2017). A compendium of multi-omic sequence information from the Saanich Inlet water column. Sci. Data 4:170160. doi: 10.1038/sdata.2017.160
Herlinveaux, R. H. (1962). Oceanography of Saanich Inlet in Vancouver Island, British Columbia. J. Fish. Res. Board Can. 19, 1–37. doi: 10.1139/f62-001
Jakuba, M., Gomez-Ibanez, D., Saito, M. A., Dick, G., and Breier, J. A. Jr. (2014). Clio: An Autonomous Vertical Sampling Vehicle for Global Ocean Biogeochemical Mapping. Washington, DC: American Geophysical Union.
Jones, W. J., Preston, C. M., Marin Iii, R., Scholin, C. A., and Vrijenhoek, R. C. (2008). A robotic molecular method for in situ detection of marine invertebrate larvae. Mol. Ecol. Resour. 8, 540–550. doi: 10.1111/j.1471-8286.2007.02021.x
Kassambara, A. (2017). ggpubr: ’ggplot2’ Based Publication Ready Plots. R Package Version 0.1.5. Available at: https://rdrr.io/cran/ggpubr/.
Keeling, R. E., Kortzinger, A., and Gruber, N. (2010). Ocean deoxygenation in a warming world. Ann. Rev. Mar. Sci. 2, 199–229. doi: 10.1146/annurev.marine.010908.163855
Kirkpatrick, J., Oakley, B., Fuchsman, C., Srinivasan, S., Staley, J. T., and Murray, J. W. (2006). Diversity and distribution of planctomycetes and related bacteria in the suboxic zone of the black Sea. Appl. Environ. Microbiol. 72, 3079–3083. doi: 10.1128/AEM.72.4.3079-3083.2006
Lam, P., and Kuypers, M. M. M. (2011). Microbial nitrogen cycling processes in oxygen minimum zones. Annu. Rev. Mar. Sci. 3, 317–345. doi: 10.1146/annurev-marine-120709-142814
Lam, P., Lavik, G., Jensen, M. M., Van De Vossenberg, J., Schmid, M., Woebken, D., et al. (2009). Revising the nitrogen cycle in the Peruvian oxygen minimum zone. Proc. Natl. Acad. Sci. U.S.A. 106, 4752–4757. doi: 10.1073/pnas.0812444106
Lepp, P. W., and Schmidt, T. M. (1998). Nucleic acid content of Synechococcus spp. during growth in continuous light and light/dark cycles. Arch. Microbiol. 170, 201–207. doi: 10.1007/s002030050634
Levin, L. A., and Breitburg, D. L. (2015). Linking coasts and seas to address ocean deoxygenation. Nat. Clim. Change 5, 401–403. doi: 10.1038/nclimate2595
Louca, S., Hawley, A. K., Katsev, S., Torres-Beltran, M., Bhatia, M. P., Kheirandish, S., et al. (2016). Integrating biogeochemistry with multiomic sequence information in a model oxygen minimum zone. Proc. Natl. Acad. Sci. U.S.A. 113, E5925–E5933. doi: 10.1073/pnas.1602897113
Naqvi, S. W. A., Bange, H. W., Farías, L., Monteiro, P. M. S., Scranton, M. I., and Zhang, J. (2010). Marine hypoxia/anoxia as a source of CH4 and N2O. Biogeosciences 7, 2159–2190. doi: 10.5194/bg-7-2159-2010
Naqvi, S. W. A., Kumar, M. D., Narvekar, P. V., De Sousa, S. N., George, M. D., and D’silva, C. (1993). An intermediate nepheloid layer associated with high microbial metabolic rates and denitrification in the northwest Indian Ocean. J. Geophys. Res. 98, 16469–16479. doi: 10.1029/93JC00973
Oksanen, J., Blanchet, F. G., Kindt, R., Legendre, P., Minchin, P. R., O’hara, R. B., et al. (2015). vegan: Community Ecology Package. Available at: http://CRAN.R-project.org/package=vegan R package version 2.3-1
Ottesen, E. A., Marin, R., Preston, C. M., Young, C. R., Ryan, J. P., Scholin, C. A., et al. (2011). Metatranscriptomic analysis of autonomously collected and preserved marine bacterioplankton. ISME J. 5, 1881–1895. doi: 10.1038/ismej.2011.70
Ottesen, E. A., Young, C. R., Gifford, S. M., Eppley, J. M., Marin, R., Schuster, S. C., et al. (2014). Multispecies diel transcriptional oscillations in open ocean heterotrophic bacterial assemblages. Science 345:207. doi: 10.1126/science.1252476
Padilla, C. C., Ganesh, S., Gantt, S., Huhman, A., Parris, D. J., Sarode, N., et al. (2015). Standard filtration practices may significantly distort planktonic microbial diversity estimates. Front. Microbiol. 6:547. doi: 10.3389/fmicb.2015.00547
Paulmier, A., and Ruiz-Pino, D. (2009). Oxygen minimum zones (OMZs) in the modern ocean. Prog. Oceanogr. 80, 113–128. doi: 10.1016/j.pocean.2008.08.001
Preston, C. M., Marin, R., Jensen, S. D., Feldman, J., Birch, J. M., Massion, E. I., et al. (2009). Near real-time, autonomous detection of marine bacterioplankton on a coastal mooring in Monterey Bay, California, using rRNA-targeted DNA probes. Environ. Microbiol. 11, 1168–1180. doi: 10.1111/j.1462-2920.2009.01848.x
R Core Team (2013). R: A Language and Environment for Statistical Computing. Vienna: R Foundation for Statistical Computing.
Rinke, C., Lee, J., Nath, N., Goudeau, D., Thompson, B., Poulton, N., et al. (2014). Obtaining genomes from uncultivated environmental microorganisms using FACS–based single-cell genomics. Nat. Protoc. 9:1038. doi: 10.1038/nprot.2014.067
Robidart, J. C., Church, M. J., Ryan, J. P., Ascani, F., Wilson, S. T., Bombar, D., et al. (2014). Ecogenomic sensor reveals controls on N2-fixing microorganisms in the North Pacific Ocean. ISME J. 8:1175. doi: 10.1038/ismej.2013.244
Schmidtko, S., Stramma, L., and Visbeck, M. (2017). Decline in global oceanic oxygen content during the past five decades. Nature 542, 335–339. doi: 10.1038/nature21399
Shi, Y., Tyson, G. W., and Delong, E. F. (2009). Metatranscriptomics reveals unique microbial small RNAs in the ocean/’s water column. Nature 459, 266–269. doi: 10.1038/nature08055
Simon, M., Grossart, H.-P., Schweitzer, B., and Ploug, H. (2002). Microbial ecology of organic aggregates in aquatic ecosystems. Aquat. Microb. Ecol. 28, 175–211. doi: 10.3354/ame028175
Smith, D. C., Simon, M., Alldredge, A. L., and Azam, F. (1992). Intense hydrolytic enzyme activity on marine aggregates and implications for rapid particle dissolution. Nature 359, 139–142. doi: 10.1038/359139a0
Stepanauskas, R. (2015). Wiretapping into microbial interactions by single cell genomics. Front. Microbiol. 6:258. doi: 10.3389/fmicb.2015.00258
Stewart, F. J., Dalsgaard, T., Young, C. R., Thamdrup, B., Revsbech, N. P., Ulloa, O., et al. (2012a). Experimental incubations elicit profound changes in community transcription in OMZ bacterioplankton. PLoS One 7:e37118. doi: 10.1371/journal.pone.0037118
Stewart, F. J., Ulloa, O., and Delong, E. F. (2012b). Microbial metatranscriptomics in a permanent marine oxygen minimum zone. Environ. Microbiol. 14, 23–40. doi: 10.1111/j.1462-2920.2010.02400.x
Stewart, F. J., Ottesen, E. A., and Delong, E. F. (2010). Development and quantitative analyses of a universal rRNA-subtraction protocol for microbial metatranscriptomics. ISME J. 4, 896–907. doi: 10.1038/ismej.2010.18
Stramma, L., Johnson, G. C., Sprintall, J., and Mohrholz, V. (2008). Expanding oxygen-minimum zones in the tropical Oceans. Science 320:655. doi: 10.1126/science.1153847
Stramma, L., Schmidtko, S., Levin, L. A., and Johnson, G. C. (2010). Ocean oxygen minima expansions and their biological impacts. Deep Sea Res. Part I Oceanogr. Res. Pap. 57, 587–595. doi: 10.1016/j.dsr.2010.01.005
Suter, E. A., Scranton, M. I., Chow, S., Stinton, D., Medina Faull, L., and Taylor, G. T. (2016). Niskin bottle sample collection aliases microbial community composition and biogeochemical interpretation. Limnol. Oceanogr. 62, 606–617. doi: 10.1002/lno.10447
Suzuki, R., and Shimodaira, H. (2015). pvclust: Hierarchical Clustering with P-Values via Multiscale Bootstrap Resampling. R Package Version 2.0-0. Available at: http://CRAN.R-project.org/package=pvclust
Taylor, C. D., and Doherty, K. W. (1990). Submersible Incubation Device (SID), autonomous instrumentation for the in situ measurement of primary production and other microbial rate processes. Deep Sea Res. Part A Oceanogr. Res. Pap. 37, 343–358. doi: 10.1016/0198-0149(90)90132-F
Taylor, C. D., Edgcomb, V. P., Doherty, K. W., Engstrom, I., Shanahan, T., Pachiadaki, M. G., et al. (2015). Fixation filter, device for the rapid in situ preservation of particulate samples. Deep Sea Res. Part A Oceanogr. Res. Pap. 96, 69–79. doi: 10.1016/j.dsr.2014.09.006
Torres-Beltrán, M., Hawley, A., Capelle, D., Bhatia, M., Durno, E., Tortell, P., et al. (2016). Methanotrophic community dynamics in a seasonally anoxic fjord: saanich inlet, British Columbia. Front. Mar. Sci. 3:268. doi: 10.3389/fmars.2016.00268
Torres-Beltrán, M., Hawley, A. K., Capelle, D., Zaikova, E., Walsh, D. A., Mueller, A., et al. (2017). A compendium of geochemical information from the Saanich Inlet water column. Sci. Data 4:170159. doi: 10.1038/sdata.2017.159
Tripp, H. J., Kitner, J. B., Schwalbach, M. S., Dacey, J. W. H., Wilhelm, L. J., and Giovannoni, S. J. (2008). SAR11 marine bacteria require exogenous reduced sulphur for growth. Nature 452, 741–744. doi: 10.1038/nature06776
Venables, W. N., and Ripley, B. D. (2002). Modern Applied Statistics with S, 4th Edn. New York, NY: Springer. doi: 10.1007/978-0-387-21706-2
Walsh, D. A., and Hallam, S. J. (2011). “Bacterial Community Strucuture and Dynamics in a seasonally anoxic fjord: Saanich Inlet, British Columbia,” in Handbook of Molecular Microbial Ecology II: Metagenomics in Different Habitats, ed. F. J. De Brujin (Hoboken, NJ: Wiley-Blackwell), 253–267.
Walsh, D. A., Zaikova, E., Howes, C. G., Song, Y. C., Wright, J. J., Tringe, S. G., et al. (2009). Metagenome of a versatile chemolithoautotroph from expanding oceanic dead zones. Science 326, 578–582. doi: 10.1126/science.1175309
Ward, B. B., Devol, A. H., Rich, J. J., Chang, B. X., Bulow, S. E., Naik, H., et al. (2009). Denitrification as the dominant nitrogen loss process in the Arabian Sea. Nature 461, 78–81. doi: 10.1038/nature08276
Whitmire, A. L., Letelier, R. M., Villagrán, V., and Ulloa, O. (2009). Autonomous observations of in vivo fluorescence and particle backscattering in an oceanic oxygen minimum zone. Opt. Express 17, 21992–22004. doi: 10.1364/OE.17.021992
Whitney, F. A., Freeland, H. J., and Robert, M. (2007). Persistently declining oxygen levels in the interior waters of the eastern subarctic Pacific. Prog. Oceanogr. 75, 179–199. doi: 10.1016/j.pocean.2007.08.007
Wickham, H. (2009). ggplot2: Elegant Graphics for Data Analysis. New York, NY: Springer-Verlag. doi: 10.1007/978-0-387-98141-3
Wright, J. J., Konwar, K. M., and Hallam, S. J. (2012). Microbial ecology of expanding oxygen minimum zones. Nat. Rev. Microbiol. 10, 381–394. doi: 10.1038/nrmicro2778
Wrighton, K. C., Thomas, B. C., Sharon, I., Miller, C. S., Castelle, C. J., Verberkmoes, N. C., et al. (2012). Fermentation, hydrogen, and sulfur metabolism in multiple uncultivated bacterial phyla. Science 337:1661. doi: 10.1126/science.1224041
Keywords: microbial ecology, oxygen minimum zone, standards of practice, filtration methods, amplicon sequencing
Citation: Torres-Beltrán M, Mueller A, Scofield M, Pachiadaki MG, Taylor C, Tyshchenko K, Michiels C, Lam P, Ulloa O, Jürgens K, Hyun J-H, Edgcomb VP, Crowe SA and Hallam SJ (2019) Sampling and Processing Methods Impact Microbial Community Structure and Potential Activity in a Seasonally Anoxic Fjord: Saanich Inlet, British Columbia. Front. Mar. Sci. 6:132. doi: 10.3389/fmars.2019.00132
Received: 10 October 2018; Accepted: 04 March 2019;
Published: 22 March 2019.
Edited by:
Raquel Vaquer-Sunyer, Instituto Mediterráneo de Estudios Avanzados (IMEDEA), SpainReviewed by:
Dennis A. Bazylinski, University of Nevada, Las Vegas, United StatesJun Sun, Tianjin University of Science and Technology, China
Carolin Regina Löscher, University of Southern Denmark, Denmark
Copyright © 2019 Torres-Beltrán, Mueller, Scofield, Pachiadaki, Taylor, Tyshchenko, Michiels, Lam, Ulloa, Jürgens, Hyun, Edgcomb, Crowe and Hallam. This is an open-access article distributed under the terms of the Creative Commons Attribution License (CC BY). The use, distribution or reproduction in other forums is permitted, provided the original author(s) and the copyright owner(s) are credited and that the original publication in this journal is cited, in accordance with accepted academic practice. No use, distribution or reproduction is permitted which does not comply with these terms.
*Correspondence: Steven J. Hallam, c2hhbGxhbUBtYWlsLnViYy5jYQ==