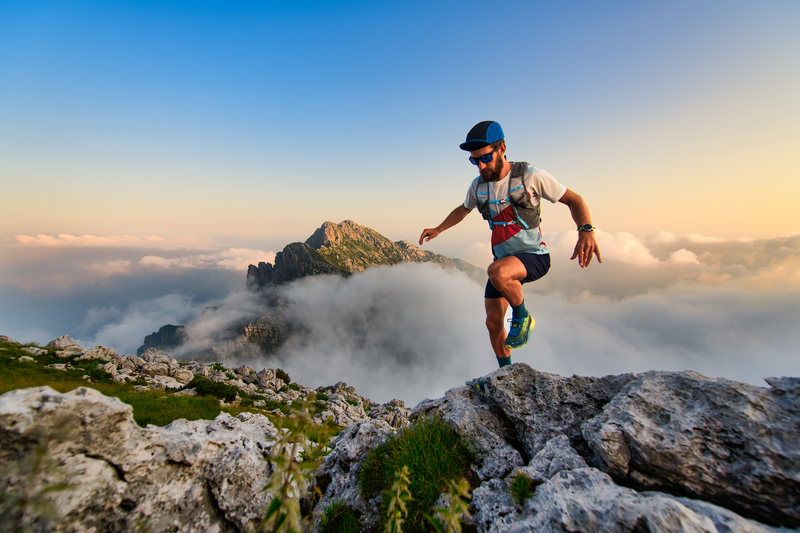
94% of researchers rate our articles as excellent or good
Learn more about the work of our research integrity team to safeguard the quality of each article we publish.
Find out more
REVIEW article
Front. Mar. Sci. , 12 February 2019
Sec. Marine Ecosystem Ecology
Volume 6 - 2019 | https://doi.org/10.3389/fmars.2019.00040
This article is part of the Research Topic Changing Plankton Communities: Causes, Effects and Consequences View all 14 articles
Trait-based approaches to investigate (short- and long-term) phytoplankton dynamics and community assembly have become increasingly popular in freshwater and marine science. Although the nature of the pelagic habitat and the main phytoplankton taxa and ecology are relatively similar in both marine and freshwater systems, the lines of research have evolved, at least in part, separately. We compare and contrast the approaches adopted in marine and freshwater ecosystems with respect to phytoplankton functional traits. We note differences in study goals relating to functional trait use that assess community assembly and those that relate to ecosystem processes and biogeochemical cycling that affect the type of characteristics assigned as traits to phytoplankton taxa. Specific phytoplankton traits relevant for ecological function are examined in relation to herbivory, amplitude of environmental change and spatial and temporal scales of study. Major differences are identified, including the shorter time scale for regular environmental change in freshwater ecosystems compared to that in the open oceans as well as the type of sampling done by researchers based on site-accessibility. Overall, we encourage researchers to better motivate why they apply trait-based analyses to their studies and to make use of process-driven approaches, which are more common in marine studies. We further propose fully comparative trait studies conducted along the habitat gradient spanning freshwater to brackish to marine systems, or along geographic gradients. Such studies will benefit from the combined strength of both fields.
Phytoplankton has been studied for a very long time, starting with the description and identification of the diverse plethora of species. The linkage between phenotypically-based taxonomy and evolutionary history led to an initial scientifically sound categorization. Since a number of traits are linked to phylogeny, this phylogenetic categorization inherently incorporated a (weak) trait-based component (Bruggeman, 2011; Narwani et al., 2015). Moreover, long before trait-based approaches became popular, what are currently considered phytoplankton traits (e.g., edible or motile) were commonly referred to, without explicitly using the term “trait.” A good example is the conceptual narrative PEG model for seasonal lake plankton community succession that emerged as early as the 1980s (Sommer et al., 1986). This oft-referred to lake model is essentially trait-based, without using the term trait. The roots of trait-based thinking about phytoplankton are in Ramon Margalef’s original work describing the “life-forms” and “functional morphologies” favorable to remaining in the water column under different nutrient conditions, irrespective of whether one considers freshwater or marine environments (Margalef, 1978). Margalef’s Mandala, as it has become known, underlies much thinking on phytoplankton community assembly based on traits. Margalef’s thinking formed a strong undercurrent in the pillar of the development of trait-based approaches to phytoplankton community assembly in freshwaters. Subsequently, in the 1980s, Colin Reynolds established a functional classification of lake phytoplankton assemblages based on observations from field data (summarized in Reynolds, 1997 and elaborated further in Reynolds et al., 2002). Using this classification scheme, characteristic phytoplankton communities comprised of key species were identified and related to specific environmental conditions, including season, lake trophy, lake morphometry, light availability. Within each assemblage, functionally different groups were usually represented, demonstrating that for a particular environment, complementarity through different ecological strategies or trait combinations enable thriving communities. It is from these sources that the application of trait-based thinking and theory with respect to phytoplankton has its roots, and from which it is currently rapidly expanding.
Traits for phytoplankton have been fairly well-explored and described for both marine and freshwater ecosystem types. In freshwaters, the focus has been on lake phytoplankton, and the conceptual link back to Reynold’s earlier work on functional groups (see previous section) can be directly traced in many cases. There are now essentially two schools of thought, although they are highly related. One “explicitly” defines traits as categorical, nominal or continuous and the other groups organisms by morphometric features into functional groups sometimes called “morphospecies,” continuing more directly Reynold’s classification. The first is common to both marine and freshwater studies while the morphospecies classification has been more commonly used only in freshwater studies, likely owing to the tradition of studying seasonal succession in these environments and less focus on biogeochemical cycling that preclude the need for high quality physiological processing rates.
Using explicitly defined traits lends itself well to the estimation of functional diversity indicators (such as functional dispersion, richness, diversity and evenness; Weithoff, 2003; Villéger et al., 2008; Laliberté and Legendre, 2010) as well as community weighted means to characterize functional composition of communities (e.g., Beisner and Longhi, 2013; Moser et al., 2017). It also enables the examination of trade-offs between different continuously measured traits such as those associated with nutrient uptake and storage (Litchman et al., 2007; Edwards et al., 2012). All of these indicators are relevant to questions of competitive interaction between phytoplankton, interaction with predators, and thus community assembly. Explicitly-defined traits will also characterize ecosystem functioning, including processes such as primary production, trophic transfer (biomass) and nutrient cycling and this has been addressed mainly in studies relating biodiversity to ecosystem function (BEF studies) (e.g., Zwart et al., 2015; Abonyi et al., 2018).
Generally, trait matrices are created for communities of interest whereby each species is characterized by a series of explicit traits. The traits used reflect both functions related to resource acquisition (bottom–up processes) and predation (top–down processes). In the explicit framework, commonly used traits are classified by type (Litchman and Klausmeier, 2008) as follows:
First: Morphological: size, biovolume, mucilage presence/absence, biological form (unicellular/colonial/coenobium/sincoenobium/filament/chains).
Second: Physiological: (a) presence/absence of silica demand, heterocysts (N2-fixation), mixotrophy, toxin production, resting stages; (b) dominant pigment type; (c) nutrient and/or light uptake parameters; (d) temperature optima.
Third: Behavioral: presence/absence of flagella, aerotypes.
Generally many of these traits are based on literature or expert knowledge to classify traits, although many gaps still exist. There are new and evolving techniques to fill the data gaps on traits, mainly through the use of phylogenetic relationships (e.g., Bruggeman, 2011).
An important potential drawback with this approach, that assigns static traits to species, is that the actual expression of traits in phytoplankton may depend heavily on local (spatial and temporal) environmental conditions (but see section “Study of Communities vs. Selected Taxonomic Groups” on phenotypic plasticity). However (Violle et al., 2007), based on trait-based approaches in plants, argue that traits should be measurable on individuals independently of the environmental conditions. It is clear that such arguments do not necessarily apply in the case of the short-generation time and fast response times characteristic of phytoplankton. Notably, phytoplankton traits related to physiology may not always be expressed to the same degree, nor even active at all– for example classifying a species as a nitrogen-fixer does not characterize temporal variability in this trait that may occur in a changing environment. The next step in functional trait consideration of phytoplankton communities will be to better characterize in situ trait expression using genetic, biochemical or physiological probes. The challenge is doing so at the community level for the wide range of phytoplankton taxa and cells potentially present.
This approach is also commonly used, mainly in freshwater ecosystems in which it has roots (Reynolds et al., 2002), specifically in Reynolds (1997) trait-based “response group” classification of lake phytoplankton. Response group classification was based largely on how communities changed reliably year-to-year during seasonal succession. The morphospecies approach in particular counts, among other things, on the fact that shape (including size) affects buoyancy, uptake parameters and predator handling time and thus simplifies the original classification proposed by Reynolds (1988).
Morphospecies classification assumes that the empirical observation of the morphology of phytoplankton community constituents reflects the environmental and biotic interaction constraints on the community as well as the physiology of the organisms. It relies heavily on the idea that the morphometry of taxa reflect their autecology (physiology and functioning) (Kruk et al., 2010). Thus, like the response group approach of Reynolds (1988, 1997), it differs from the explicit trait approach in that it considers trait-mediated effects that arise from species interactions (by empirically assessing group composition) (Abonyi et al., 2018). Summarily, clusters of taxa based entirely on morphological features can be defined statistically and should represent meaningful functional phytoplankton groups.
In many ways, the original morphospecies classification resembles a reduced version of the explicitly-defined traits (Kruk et al., 2010). It was based originally on nine morphological traits including many that overlap with the previous classification: volume, maximum linear dimension, surface area, and the presence of mucilage, flagella, gas vesicles (or aero-topes), heterocysts or siliceous exoskeletal structures. Essentially, what is not found explicitly in the morphospecies approach is any explicit reference to physiological parameter rates – these are assumed (and were shown by Kruk et al., 2010) to be correlated with the morphological traits and group classification. The other difference from the explicitly-defined trait approach, is that instead of creating a species x trait matrix, then used to estimate functional composition or diversity, the focus here is on creating morphologically based functional groups (MBFGs) using clustering techniques. The groups thus created can then be related to environmental gradients or ecosystem functioning. In this approach, a potential short-coming however is the need to translate these morpho-groups into fitness (Naselli-Flores and Barone, 2011).
There has been a large focus on cell or body size as a “master” trait – so-called because of the large number of physiological and morphometric features that are regulated by body size. Also feedbacks with the environment are related to body size, as evident in the metabolic theory of ecology (e.g., Brown et al., 2004), which demonstrates the theoretical link between body size and ecosystem processes. Furthermore, it is recognized that body size and body size distribution (e.g., biomass size spectra) in phytoplankton communities can reflect many environmental factors such as vertical water dynamics and depth, trophic state, predation, and factors affecting growth (reviewed in Mouillot et al., 2006; Litchman and Klausmeier, 2008). It should be noted that the use of biomass size spectra has a long history in both marine and freshwater plankton studies: with older studies usually focused on estimating food web transfer efficiencies (e.g., Kerr, 1974; Gaedke, 1993; Sprules and Goyke, 1994) and not to prediction of other functional traits important for community assembly within specific groups like the phytoplankton themselves. More recent work has directly considered the relations between size spectra and effect traits such as nutrient recycling rates and in response to environmental conditions in aquatic ecosystems (e.g., Rodríguez et al., 2001) as is currently done in more explicit trait-based approaches. In phytoplankton body size is usually estimated as cell size along the maximum linear dimension (abbreviated as MLD or GALD: greatest axial linear dimension). The use of cell size is common to both the explicit trait and morphospecies frameworks and lends itself well to ecosystem modeling (e.g., Acevedo-Trejos et al., 2016).
Elemental stoichiometry of phytoplankton cells could be considered a type of “master trait” because, like size, these relationships are subject to biophysical rules that link growth rates with environments, food web interactions and thus biogeochemical cycles (Finkel et al., 2010). This consideration stems originally and largely from marine studies of phytoplankton (Finkel et al., 2010; Litchman et al., 2015), in large part due to the longer focus on biogeochemical cycles and feedbacks with phytoplankton (e.g., Follows and Dutkiewicz, 2011). Phytoplankton taxa have distinct stoichiometric ratios in both the macronutrients (e.g., C:P, N:P), but also in relation to micronutrients such as Cu, Fe, Zn, Co, Cd, and Mn; relationships that have evolved over time (reviewed in Litchman et al., 2015). Because of the strong potential effect of changes in stoichometry that would accompany changes in phytoplankton communities driven by environmental factors (other than biogeochemical ones), it is argued that the stoichiometric properties or traits of altered communities could inform on how biogeochemical processes are likely to be altered.
More recently, and in a different context, an interest in using phytoplankton to produce biofuel has lead to a fuller investigation of the stoichiometric traits in freshwater phytoplankton as well (Shurin et al., 2013, 2014). The goal has been to optimize long-term culture conditions for commercial-grade algal biofuels through the manipulation of C:N:P ratios that best optimize desired phytoplankton biomass production based on organismal requirements (stoichiometric traits).
There are a series of other trait types being developed, some focusing on more aggregate properties of communities (i.e., defined at the community and not individual level) and others focussing directly on the many individual phytoplankton present in a community. We will describe these briefly working from the aggregate community to the individual level.
In marine ecosystems, researchers have begun to use optical properties measured from satellites to estimate overall phytoplankton community structure. Essentially, from MERIS wavelengths, bio-optical traits (BOTs) have been derived to characterize phytoplankton functional types (PFTs) (Aiken et al., 2007, 2008). Such PFTs can then be linked to the global carbon cycle using bio-mechanistic models (Aiken et al., 2008). As with other trait-based methods, estimating the functional type (PFT) from remotely sensed oceanographic data requires that a specific BOT exist for each taxon. Bio-optical traits are derived more specifically from a suite of variables: Chl-a concentration; accessory pigments (Chl-b, Chl-c, carotenoids, phycobillins); pigment ratios (TChl-a/AP, TChl-a/TP, PPC/TC); phytoplankton absorption at 443 nm (aph443), and the spectral slope of aph.
Using high performance liquid chromatography (HPLC), it is possible to characterize phytoplankton communities by their pigments (e.g., Zhao and Quigg, 2014). Post-processing of HPLC results enables observation of changes in communities in terms of often highly phylogenetically conserved pigment groups. Because taxa with different pigments respond differently to the light environment, their pigment profiles can be considered as functional traits.
This method relies on the fact that there are conserved strategies in energy and C partitioning amongst phytoplankton species that can then be used to characterize their growth rate traits. Cell chemotyping can be done using Fourier transform infrared (FTIR)-spectroscopy to obtain biochemical signatures in phytoplankton cells (Fanesi et al., 2017). In this study, the authors demonstrated that the macromolecular (FTIR absorption peaks of proteins, lipids, carbohydrates, and phosphorylated compounds) composition of phytoplankton cells was able to better characterize details of cell physiology related to growth rates. In this way, a common set of physiological traits can be used to define species and it has the potential to apply to in situ estimates of functioning of cells, even in dynamic environments.
Another recent method applies to characterizing individual cells in a community, rather than assigning traits to all individuals in the same taxon. Thus, the focus is on intra-specific variation. The idea is to scan communities using flow cytometry to obtain a series of parameter estimates (traits) for all individual cells present in a community (Fontana et al., 2014). These traits characterize morphology (length, area) as well as fluorescence characters (chlorophyll a, accessory and degraded pigments) on the individual level, thereby allowing for intra-specific variation in these traits to be estimated.
To better integrate the various ways in which traits have been defined and used for phytoplankton, recent developments have been underway to better harmonize data and allow for its interoperability (e.g., exchange between computer platforms or software). In particular the development of thesauri is occurring generally in ecology, to standardize the semantic properties for trait labels, including their definitions. For phytoplankton, the PhytoTraits thesaurus has recently been created1 (Rosati et al., 2017). This thesaurus creates a standard definition of phytoplankton traits and their measurements that will permit better data integration across studies. A thesaurus ensures the integration amongst different datasets that use different terms for the same concept (for example biomass, weight, or dry weight; Rosati et al., 2017). Thesaurus construction uses eco-informatics approaches to acquire, integrate and analyze trait terms, developing a standard terminology or “controlled vocabulary” to which the scientific community can refer. Each term or concept obtains its own Uniform Resource Identifier (URI that is a unique, persistent internet label). New terms can then be added to thesauri such as PhytoTrait via a portal and traits labeled differently but referring to the same concept can be identified and properly classified.
Due to the similar nature of marine and freshwater habitats, relevant phytoplankton traits are also very similar between marine and freshwater species. The master trait size is commonly used independently of the habitat under consideration, being a unifying trait (Litchman and Klausmeier, 2008). Other, less frequently used traits, are resource use traits, e.g., nitrate acquisition (N-fixation), mixotrophy or traits related to light harvesting (Barton et al., 2013; Edwards et al., 2013). In freshwater studies, size is often expanded to a more complex measure of shape, taking morpho-functional differences between species into account (e.g., Kruk et al., 2010), often in combination with phylogenetic/taxonomic relatedness (Huszar and Caraco, 1998; Cellamare et al., 2013; Segura et al., 2013). In studies investigating the vertical structure of lakes, the pigment composition (Beisner and Longhi, 2013) and the motility of cells (by gas vacuoles or flagella) are further relevant traits (e.g., Pomati et al., 2012), likely less important in the well-mixed surface layer of the open ocean.
To better compare how trait-based approaches have been used in phytoplankton ecological research in marine and freshwater studies, we performed a literature search using the criteria “phytoplankton” AND “trait∗” in the Web of Science (Clarivate Analytics) on March 7, 2018. A total of 631 entries matched our criteria; the oldest one is from 1976 (Vladimirskaya et al., 1976), taking 15 years until the next papers appeared. From that point onwards, the number of contributions increased rapidly with a total of 243 papers published in 2015–2017 on phytoplankton and trait∗. We discarded > 300 papers from our subsequent analysis because the term trait was not at all related to phytoplankton or because the study did not fit into the context of phytoplankton trait-based ecology. Another ca. 150 were also removed because of a lack of a community context, dealing with only a single trait or a single species, or a particular measurement technique. A total of 150 papers remained and these were grouped into marine or freshwater (lakes and rivers) studies, then classified according to study type (field observational surveys, experimental, mathematical modeling and conceptual/review) and according their research question (community assembly, response to environmental change or relations with biogeochemical cycling). A substantial number of the papers could not assigned clearly to each category, because research questions were overarching or the themes were only loosely related to our categories. Thus, the exact numbers we present are debatable, but they do indicate some overall tendencies. From the subset of 150 articles, we found an almost equal share of marine (67) and freshwater (76) studies (Figure 1 and Table 1). Furthermore, there were several modeling or conceptual/review studies that were either not specific to one habitat type or explicitly dealt with both. Grouping these articles into field surveys, experimental, mathematical modeling and conceptual/review studies, it became obvious that experimental studies were underrepresented, while field surveys and mathematical modeling studies dominate the literature. Mathematical modeling studies were more commonly applied to marine ecosystems, while field studies were more often conducted in freshwater studies. This might reflect the accessibility and higher sampling frequencies of freshwater sites, but also the goal of the respective studies (see section “Comparing Freshwater and Marine Trait Studies: Assembly vs. Biogeochemistry”). This literature survey better enabled us to determine the different ways in which traits have been incorporated into the study of phytoplankton ecology in both freshwater and marine environments, which we now elaborate with some examples.
Figure 1. Results of the literature search using “phytoplankton” AND “trait∗.” From a total 631 articles, 150 were selected that used a trait-based approach to study phytoplankton ecology. The remaining studies were assigned to four different types according to their predominant approach and according to ecosystem type. The conceptual/review articles were often not specific to a habitat type, so no further distinctions were made.
Table 1. Summary of the focus of the 73 trait-based studies examining entire (or a majority of groups within) phytoplankton communities.
Trait-based phytoplankton studies generally consider either the whole community or focus on responses in certain phylogenetic groups such as the diatoms or other major phyla. Whole community studies from freshwater sites typically analyze seasonal dynamics or a component of temporal variation, such as the spring bloom, using classical phylogenetic (taxonomic) approaches. A trait component, such as cell size as a master trait, or some measures of functional diversity based on functional traits is sometimes added to broaden the view. However, such studies do not focus on a trait-based approach per se making it difficult to group them unambiguously into our categories.
Another aspect in community studies is the analysis of spatial (mostly vertical) differences in communities from stratified environments (e.g., Longhi and Beisner, 2010; Santana et al., 2017). Often the response to environmental changes or a detailed analysis of the traits within a particular taxonomic group (representing a particular set of traits) has been the focus. For example, diatoms represent a phylogenetic group common to both marine and freshwater studies. They are abundant in lakes, rivers and oceans alike, and play an important role in food webs and the biogeochemical cycles. As a result, their trait distribution and the consequences for diatom dynamics have been commonly studied in both ecosystem types (e.g., Terseleer et al., 2014; B-Béres et al., 2017; Taherzadeh et al., 2017). Diatoms come with the added advantage that they preserve in sediments and can thus be the focus of longer-term paleo-limnological or paleo-oceanographic analyses (Smol et al., 2005; McKay et al., 2012). In marine studies dinoflagellates and coccolithophores represent other target groups. For dinoflagellates, important traits relate either to toxin production or to mixotrophy, for coccolithophores relevant traits relate to the tolerance to ocean acidification (see section “Genotypic Trait Plasticity”).
An important, though often neglected, aspect in the quantification of traits is the phenotypic and genotypic plasticity of traits. Plasticity or trait variation is difficult to measure, but it is important for the parametrization of mathematical models and for the understanding of community processes on timescales relevant for phytoplankton ecology.
Many traits can vary substantially according to environmental conditions. While some traits can be measured on individuals (e.g., body size), some are more amenable to measurement at the community level, representing the average of a population of individuals, such as nutrient ratios or physiological traits. Size, as a master trait (see section “Phytoplankton Trait Types”), is related to many ecological processes, but it can also be highly variable. To account for cell size variation within a phytoplankton population or community and over extended periods, modern techniques now exist, although not regularly utilized to assess size variation, including flow cytometry or related systems that also provide images (e.g., Flow-CAM, CytoBOT).
Body size variation, in addition to being easy to measure, is ecological very relevant in phytoplankton and thus often considered in ecological studies. For example, because zooplankton herbivory is often size-selective, a selective pressure on distinct prey size classes is introduced, thereby changing the size distribution of a population or community (Sommer et al., 2001; Lewandowska et al., 2014). Furthermore, in freshwater ecosystems, it has been observed that the mere presence of the herbivore Daphnia, can induce changes in the colony size of their algal prey driven by kairomones, even without any herbivory (Hessen and VanDonk, 1993). In another example, the physiological status of phytoplankton cells can also drive their body sizes and thus the variation observed in a population or community: during the regular cell growth cycle the size of the cells might vary by a factor of two or even more (Massie et al., 2010). With increasing nutrient limitation, the cell cycle arrests at a certain limiting nutrient concentration and it continues only under nutrient replete conditions, which may synchronize a population’s cell size to a smaller value (Massie et al., 2010). Body size in diatoms is a particularly interesting case of size variation. Diatoms reduce their average cell size through progressive population growth, because after cell division the newly-built theca is formed from the inner (smaller) part of the mother theca leading to a continuous cell size reduction of one of the daughter cells (Lee, 1999). Associated to a smaller body size is an increase in the surface to volume ratio, facilitating the nutrient uptake per unit of volume (Reynolds, 1997).
Physiological traits also vary in response to environmental changes. A common strategy is the optimization of resource use efficiency. This includes an increase in chlorophyll-a or other photosynthetic pigments by phytoplankton cells to better collect photons at low light availabilities or spectral discontinuities (Richardson et al., 1981; Falkowski and LaRoche, 1991). Another example is the production of the enzyme nitrogenase under nitrogen limiting conditions, which enables the fixation of elementary nitrogen. An increasing nitrogenase activity is typically found with increasing duration of nitrogen-depleted conditions (e.g., Paerl, 1988).
Traits and trait values not only vary in response to environmental factors but also among different genotypes within a population. Information on genotypic trait variation is surprisingly rare and often stems from research in other fields than trait-based ecology. We can draw on several examples involving phytoplankton. First, with respect to toxicity, different strains of potentially toxic algae have been analyzed to understand the mechanisms and physiological costs behind toxin production. In marine habitats, dinoflagellates (e.g., Alexandrium) are the most prominent toxic group, while cyanobacteria (e.g., Microcystis and others) are the main toxin producers in freshwaters. Within one species, the ability and magnitude of toxin production among different strains varies considerably, making it difficult to assign this trait to a species (Ichimi et al., 2002; Kardinaal et al., 2007; Touzet et al., 2008). Another source for information on trait plasticity comes from invasion biology: invasive species usually regarded as species with a high genotypic plasticity facilitating their establishment in newly invaded habitats. For the invasive cyanobacterium Cylindrospermopsis, high genotypic trait variability is notable (Willis et al., 2016; Bolius et al., 2017). Genotypic variation in phytoplankton also comes from global change research as it is related to ocean acidification. The response of coccolithophores to declining pH-levels has been shown to be strain-specific (Müller et al., 2015; Rickaby et al., 2016) and varies at different times scales (Meyer and Riebesell, 2015; Schlüter et al., 2016). Differentiating between such short-term strain-specific acclimation and long-term adaptation makes it difficult to predict community responses to ocean acidification.
For several applications of trait-based approaches, trait variability might not be a serious disadvantage, as for example with respect to aspects of community assembly in relatively stable environments. However, for modeling ecosystem processes or species interactions more directly and on shorter timescales, the variability in traits may be critical. However, not only does the variability itself complicate trait-based modeling (Coutinho et al., 2016), but the shape of the trait distribution also plays an important role (Gaedke and Klauschies, 2018). The shape of the trait distribution determines the potential for adaptive responses to environmental change. For example, when environmental forces act specifically on a certain trait and a distinct range of the trait values, a bimodal trait distribution might permit a more sustainable response option relative to a normal trait distribution, especially if the pressure is imposed around the mean. A great deal more work will be necessary to establish the distributions of highly variable individual phytoplankton traits to inform these modeling approaches.
Narrowing our literature survey subset of 150 articles further, by including only those studies that had either a broad spatial (many samples over a broader area) or temporal scale (time series data), as well as those that considered the entire (or a substantial part of the) phytoplankton community under a trait-based view, 73 articles remained. The majority were freshwater studies, with 29 dealing mostly with environmental factors driving community assembly or temporal dynamics when assessed using traits (perspective 1, Table 1 and Supplementary Table S1). Other studies at the community level examined trait distributions more directly by focusing on interactions between them and how this could influence community structure or dynamics (without explicit consideration of environmental variation). This group (perspective 2, Table 1) was more evenly split between marine and freshwater environments (6–7 per environment). On the other hand, studies that focused more directly on ecosystem consequences of traits (perspective 3, Table 1), including ecosystem biogeography and biogeochemical processes including stoichiometry, were much less numerous (total of 13), with the majority (8) from marine environments and another two combining freshwater and marine. Irrespective of research perspective, a total of seven studies used trait-based approaches in marine and freshwaters together, and three considered estuaries.
Generally speaking, for phytoplankton, the nature of the pelagic habitat as well as the main representative phyla and their ecology are relatively similar across both marine and freshwater ecosystems. Despite this similarity, research questions and approaches have evolved, at least in part, separately, in the two environments. One crucial difference between these ecosystem types is the accessibility and the possibility to take frequent samples. For many lakes, routine sampling campaigns are regularly conducted, either by local authorities or scientific institutes and universities. Thus, many data sets with some temporal resolution and good physico-chemical background data exist. In comparison, less data exists for coastal regions, and regular, high temporal resolution biological sampling in open ocean sites is very rare (but see Buitenhuis et al., 2013; Brun et al., 2015).
Traditionally, much ecological research was performed at field stations investigating the on-site environment. This is particularly the case for freshwater biological stations, many of which were established to combine monitoring and research, often in relation to water utilities and providers related to drinking water, fisheries or recreational uses. For these purposes, water quality and phytoplankton community composition has been extensively studied, with frequent and regular sampling of the pelagic food web, to ensure the management of drinking water quality and quantity or of fish harvest. At marine biological stations, local sites have also been sampled, although they have served primarily as entry points to understanding the open ocean, for which cruises are expensive and do not allow for regular sampling.
These different ecosystem accessibility and scientific histories are mirrored in the different research questions addressed in phytoplankton trait-based studies in freshwater and marine systems. Trait-based studies from the open ocean preferentially investigate biogeographic trait patterns (Acevedo-Trejos et al., 2013; Barton et al., 2013) or they have a strong biogeochemical focus aiming for the quantification of globally important processes related to primary production such as carbon-dioxide acquisition, nitrogen fixation (Breton et al., 2017), or oceanic stoichiometry and biochemistry (Litchman et al., 2007, 2015; Strom, 2008; Finkel et al., 2010; Bonachela et al., 2016), but also on phytoplankton dynamics (Alexander et al., 2015) (Figure 2). In oceanography, many studies model trait-based processes, aiming for broad-scale extrapolation of these globally (Smith et al., 2016; Vallina et al., 2017). From a conceptual point of view, marine research considers how traits and trait distribution drive processes determining the environmental conditions (e.g., biogeochemistry).
Figure 2. Schematic of the pre-dominant perspectives in marine and freshwater phytoplankton trait-based research. Line thickness indicates the relative number of studies.
In the more accessible coastal or freshwater sites such as lakes, the conceptual view is often in the opposite direction (Figure 2), considering instead how phytoplankton communities respond to the environment and which environmental factors drive the trait-based community assembly. Answers to these research questions rely on a sampling regime with a high temporal resolution. A prominent example for a coastal long-term sampling site is the Western Channel Observatory site in the English Channel off the coast of Plymouth, United Kingdom (Edwards et al., 2013; Mutshinda et al., 2017). Coastal sites that are influenced by the tide or estuaries are subjected to rapid environmental changes so that the response to such changes became into the focus of the investigations (Aubry et al., 2017; Klais et al., 2017; Moser et al., 2017). On a similar time-scale, lakes (and rivers) are also characterized by rapid environmental changes and studies on these systems thus often deal with trait-driven responses to these by the community. Longer-term studies that consider several months or years are highly suitable to these types of questions, especially when similar environmental changes are repeated during the investigation, representing a form of temporal replication (Pomati et al., 2012; Edwards et al., 2013; Tsai et al., 2014; Weithoff et al., 2015; Bortolini et al., 2016; Weithoff and Gaedke, 2017). Alternatively, instead of temporal replication, trait-based approaches also enable a space-for-time substitution in which many sites receiving a similar environmental signal (e.g., sites along a coastal or river stretch or lakes within the same region) are sampled only once or a very few times (Longhi and Beisner, 2010; Machado et al., 2016; Santana et al., 2017; Vadrucci et al., 2017). Trait-based approaches become particularly helpful in such cases, as they are ataxonomic, facilitating the detection of common response patterns within these potentially disparate communities. Another aspect of freshwater trait-based studies is the analysis of the vertical distribution of phytoplankton (Pomati et al., 2012; Beisner and Longhi, 2013; Santana et al., 2017), when steep physical-chemical gradients exist. One drawback we noted in several studies is that they lack a clear rationale as to why they use trait-based approaches in relation to the ecological goals (as also argued by Hébert et al., 2017 for zooplankton). Clearly outlined goals related to improved understanding of community assembly vs. contributions of phytoplankton to biogeochemical cycles should be a part of every study that uses trait-based approaches.
Similarities and differences in phytoplankton trait-based approaches between marine and freshwater studies can be identified by the ways in which traits are assigned and the focus of the primary research questions to which traits are applied. With respect to trait assignment, two related schools of thought exist, either “explicitly” defining several species’ traits simultaneously so that there is little overlap between taxa, or by restricting classification to “morphospecies” types, continuing more directly Reynold’s earlier classification. Explicitly-defined traits are commonly used in both marine and freshwater studies, while morphospecies classification has been more commonly used in freshwater habitats. We attribute this difference to the tradition of studying seasonal succession and community assembly under varying environmental conditions in highly accessible freshwater environments that preempts the need for higher resolution physiological processing rates. Marine studies often focus on globally relevant biogeochemical cycles, for which key process-related traits are studied on the most abundant taxa, especially coccolithophores, diatoms, dinoflagellates and prochlorophytes. In general, researchers are encouraged to better motivate, why they apply trait-based analyses to their studies. They will benefit from applying the strong process-driven approaches used in most marine studies to investigate the relationship between traits and biogeochemical processes. Marine researchers might benefit from the mechanistic approaches used by freshwater researchers to better understand community assembly using traits, if more resolved time-series data can be obtained through autonomous buoys or remote sensing. As another avenue of future research, we propose fully comparative trait studies conducted along the habitat gradient spanning freshwater to brackish to marine systems. Such studies can combine the strengths of both fields and would be of general ecological interest. Other comparative studies could analyze latitudinal, altitudinal, or trophic gradients using phytoplankton traits as a common currency to assess community structure. In some cases, data likely already exist to conduct these studies. Given the low number of experimental studies we noted in our literature survey, it would be very useful to test the field survey patterns more explicitly in manipulated experiments, such as in mesocosms, to ascertain mechanisms influencing trait distribution and expression. Last, but not least, a common challenge for future research in both systems remains to account for within-species trait variation, a critical component to trait-based studies in all aquatic habitats, especially in an era of rapid environmental change.
All authors listed have made a substantial, direct and intellectual contribution to the work, and approved it for publication.
BEB was supported by the Alexander von Humboldt-Stiftung.
The authors declare that the research was conducted in the absence of any commercial or financial relationships that could be construed as a potential conflict of interest.
The Supplementary Material for this article can be found online at: https://www.frontiersin.org/articles/10.3389/fmars.2019.00040/full#supplementary-material
TABLE S1 | List of articles that had either a broad spatial (many samples over a broader area) or temporal scale (time series data), as well as those that considered the entire (or a substantial part of the) phytoplankton community under a trait-based view; sorted by year.
Abonyi, A., Horvath, Z., and Ptacnik, R. (2018). Functional richness outperforms taxonomic richness in predicting ecosystem functioning in natural phytoplankton communities. Freshwat. Biol. 63, 178–186. doi: 10.1111/fwb.13051
Acevedo-Trejos, E., Brandt, G., Merico, A., and Smith, S. L. (2013). Biogeographical patterns of phytoplankton community size structure in the oceans. Glob. Ecol. Biogeogr. 22, 1060–1070. doi: 10.1038/srep08918
Acevedo-Trejos, E., Brandt, G., Smith, S. L., and Merico, A. (2016). PhytoSFDM version 1.0.0: phytoplankton size and functional diversity model. Geosci. Model. Dev. 9, 4071–4085. doi: 10.5194/gmd-9-4071-2016
Aiken, J., Fishwick, J. R., Lavender, S., Barlow, R., Moore, G. F., Sessions, H., et al. (2007). Validation of MERIS reflectance and chlorophyll during the BENCAL cruise October 2002: preliminary validation of new demonstration products for phytoplankton functional types and photosynthetic parameters. Internat. J. Rem. Sens. 28, 497–516. doi: 10.1080/01431160600821036
Aiken, J., Hardman-Mountford, N. J., Barlow, R., Fishwick, J., Hirata, T., and Smyth, T. (2008). Functional links between bioenergetics and bio-optical traits of phytoplankton taxonomic groups: an overarching hypothesis with applications for ocean colour remote sensing. J. Plankton Res. 30, 165–181. doi: 10.1093/plankt/fbm098
Alexander, H., Rouco, M., Haley, S. T., Wilson, S. T., Karl, D. M., and Dyhrman, S. T. (2015). Functional group-specific traits drive phytoplankton dynamics in the oligotrophic ocean. Proc. Natl. Acad. Sci. U.S.A. 112, E5972–E5979. doi: 10.1073/pnas.1518165112
Aubry, F. B., Pugnetti, A., Roselli, L., Stanca, E., Acri, F., Finotto, S., et al. (2017). Phytoplankton morphological traits in a nutrient-enriched, turbulent mediterranean microtidal lagoon. J. Plankton Res. 39, 564–576. doi: 10.1093/plankt/fbx008
Barton, A. D., Pershing, A. J., Litchman, E., Record, N. R., Edwards, K. F., Finkel, Z. V., et al. (2013). The biogeography of marine plankton traits. Ecol. Lett. 16, 522–534. doi: 10.1111/ele.12063
B-Béres, V., Török, P., Kókai, Z., Lukács, A., T-Krasznai, E., Tóthmérészc, B., et al. (2017). Ecological background of diatom functional groups: comparability of classification systems. Ecol. Indic. 82, 183–188. doi: 10.1016/j.ecolind.2017.07.007
Beisner, B. E., and Longhi, M. L. (2013). Spatial overlap in lake phytoplankton: relations with environmental factors and consequences for diversity. Limnol. Oceanogr. 58, 1419–1430. doi: 10.4319/lo.2013.58.4.1419
Bolius, S., Wiedner, C., and Weithoff, G. (2017). High local trait variability in a globally invasive cyanobacterium. Freshwat. Biol. 62, 1879–1890. doi: 10.1111/fwb.13028
Bonachela, J. A., Klausmeier, C. A., Edwards, K. F., Litchman, E., and Levin, S. A. (2016). The role of phytoplankton diversity in the emergent oceanic stoichiometry. J. Plankton Res. 38, 1021–1035. doi: 10.1093/plankt/fbv087
Bortolini, J. C., Moresco, G. A., de Paula, A. C. M., Jati, S., and Rodrigues, L. C. (2016). Functional approach based on morphology as a model of phytoplankton variability in a subtropical floodplain lake: a long-term study. Hydrobiologia 767, 151–163. doi: 10.1007/s10750-015-2490-z
Breton, E., Christaki, U., Bonato, S., Didry, M., and Artigas, L. F. (2017). Functional trait variation and nitrogen use efficiency in temperate coastal phytoplankton. Mar. Ecol. Prog. Ser. 563, 35–49. doi: 10.3354/meps11974
Brown, J. H., Gillooly, J. F., Allen, A. P., Savage, V. M., and West, G. B. (2004). Towards a metabolic theory in ecology. Ecology 85, 1771–1789. doi: 10.1890/03-9000
Bruggeman, J. (2011). A phylogenetic approach to the estimation of phytoplankton traits. J. Phycol. 47, 52–65. doi: 10.1111/j.1529-8817.2010.00946.x
Brun, P., Vogt, M., Payne, M. R., Gruber, N., O’Brien, C. J., Buitenhuis, E. T., et al. (2015). Ecological niches of open ocean phytoplankton taxa. Earth Syst. Sci. Data 5, 227–239. doi: 10.3389/fmicb.2012.00362
Buitenhuis, E. T., Vogt, M., Moriarty, R., Bednaršek, N., Dones, J. C., Leblanc, L., et al. (2013). MAREDAT: towards a world atlas to MARin ecosystem DATa. Earth Syst. Sci. Data 5, 227–239. doi: 10.5194/essd-5-227-2013
Cellamare, M., de Tezanos Pinto, P., Leitão, M., Coste, M., Boutry, S., and Haury, J. (2013). Using functional approaches to study phytoplankton communities in a temperate region exposed to tropical species dispersal. Hydrobiologia 702, 267–282. doi: 10.1007/s10750-012-1330-7
Coutinho, R. M., Klauschies, T., and Gaedke, U. (2016). Bimodal trait distributions with large variances question the reliability of trait-based aggregate models. Theor. Ecol. 9, 389–408. doi: 10.1007/s12080-016-0297-9
Edwards, K. F., Litchman, E., and Klausmeier, C. A. (2013). Functional traits explain phytoplankton community structure and seasonal dynamics in a marine ecosystem. Ecol. Lett. 16, 56–63. doi: 10.1111/ele.12012
Edwards, K. F., Thomas, M. K., Klausmeier, C. A., and Litchman, E. (2012). Allometric scaling and taxonomic variation in nutrient utilization traits and maximum growth rate of phytoplankton. Limnol. Oceanogr. 57, 554–566. doi: 10.4319/lo.2012.57.2.0554
Falkowski, P. G., and LaRoche, J. (1991). Acclimation to spectral irradiance in algae. J. Phycol. 27, 8–14. doi: 10.1111/j.0022-3646.1991.00008.x
Fanesi, A., Wagner, H., and Wilhelm, C. (2017). Phytoplankton growth rate modelling: can spectroscopic cell chemotyping be superior to physiological predictors? Proc. R. Soc. B. 284:20161956. doi: 10.1098/rspb.2016.1956
Finkel, Z. V., Beardall, J., Flynn, K. J., Quigg, A., Rees, T. A. V., and Raven, J. A. (2010). Phytoplankton in a changing world: cell size and elemental stoichiometry. J. Plankton Res. 32, 119–137. doi: 10.1093/plankt/fbp098
Follows, M. J., and Dutkiewicz, S. (2011). Modeling diverse communities of marine microbes. Ann. Rev. Mar. Sci. 3, 427–451. doi: 10.1146/annurev-marine-120709-142848
Fontana, S., Jokela, J., and Pomati, F. (2014). Opportunities and challenges in deriving phytoplankton diversity measures from individual trait-based data obtained by scanning flow-cytometry. Front. Microbiol. 5:324. doi: 10.3389/fmicb.2014.00324
Gaedke, U. (1993). Ecosystem analysis based on biomass size distribution – a case study of a plankton community in a large lake. Limnol. Oceanogr. 38, 112–127. doi: 10.4319/lo.1993.38.1.0112
Gaedke, U., and Klauschies, T. (2018). Analyzing the shape of observed trait distributions enables a data-based moment closure of aggregate models. Limnol. Oceanogr. Methods 15, 979–994. doi: 10.1002/lom3.10218
Hébert, M.-P., Beisner, B. E., and Maranger, R. (2017). Linking zooplankton communities to ecosystem functioning: toward an effect-trait framework. J. Plankton Res. 39, 3–12. doi: 10.1093/plankt/fbw068
Hessen, D. O., and VanDonk, E. (1993). Morphological changes in Scenedesmus induced by substances released by Daphnia. Arch. Hydrobiol. 127, 129–140.
Huszar, V. L. M., and Caraco, N. (1998). The relationship between phytoplankton composition and physical-chemical variables: a comparison of taxonomic and morphological-functional approaches in six temperate lakes. Freshwat. Biol. 40, 679–696. doi: 10.1046/j.1365-2427.1998.00369.x
Ichimi, K., Suzuki, T., and Ito, A. (2002). Variety of PSP toxin profiles in various culture strains of Alexandrium tamarense and change of toxin profile in natural A. tamarense population. J. Exp. Mar. Biol. Ecol. 273, 51–60. doi: 10.1016/S0022-0981(02)00137-5
Kardinaal, W. E. A., Tonk, L., Janse, I., Hol, S., Slot, P., Huisman, J., et al. (2007). Competition for light between toxic and nontoxic strains of the harmful cyanobacterium Microcystis. Appl. Environm. Microbiol. 73, 2939–2946. doi: 10.1128/AEM.02892-06
Kerr, S. R. (1974). Theory of size distribution in ecological communities. J. Fish. Res. Board Canada 31, 1859–1862. doi: 10.1139/f74-241
Klais, R., Norros, V., Lehtinen, S., Tamminen, T., and Olli, K. (2017). Community assembly and drivers of phytoplankton functional structure. Funct. Ecol. 31, 760–767. doi: 10.1111/1365-2435.12784
Kruk, C., Huszar, V. L. M., Peeters, E. T. H. M., Bonilla, S., Costa, L., Lürling, M., et al. (2010). A morphological classification capturing functional variation in phytoplankton. Freshwat. Biol. 55, 614–627. doi: 10.1111/j.1365-2427.2009.02298.x
Laliberté, E., and Legendre, P. (2010). A distance-based framework for measuring functional diversity from multiple traits. Ecology 91, 299–305. doi: 10.1890/08-2244.1
Lewandowska, A. M., Hillebrand, H., Lengfellner, K., and Sommer, U. (2014). Temperature effects on phytoplankton diversity - The zooplankton link. J. Sea Res. 85, 359–364. doi: 10.1016/j.seares.2013.07.003
Litchman, E., and Klausmeier, C. A. (2008). Trait-based community ecology of phytoplankton. Ann. Rev. Ecol. Evol. Syst. 39, 615–639. doi: 10.1146/annurev.ecolsys.39.110707.173549
Litchman, E., Klausmeier, C. A., Schofield, O. M., and Falkowski, P. G. (2007). The role of functional traits and trade-offs in structuring phytoplankton communities: scaling from cellular to ecosystem level. Ecol. Lett. 10, 1170–1181. doi: 10.1111/j.1461-0248.2007.01117.x
Litchman, E., Pinto, P. D., Edwards, K. F., Klausmeier, C. A., Kremer, C. T., and Thomas, M. K. (2015). Global biogeochemical impacts of phytoplankton: a trait-based perspective. J. Ecol. 103, 1384–1396. doi: 10.1111/1365-2745.12438
Longhi, M. L., and Beisner, B. E. (2010). Patterns in taxonomic and functional diversity of lake phytoplankton. Freshwat. Biol. 55, 1349–1366. doi: 10.1111/j.1365-2427.2009.02359.x
Machado, K. B., Teresa, F. B., Vieira, L. C. G., Huszar, V. L. D., and Nabout, J. C. (2016). Comparing the effects of landscape and local environmental variables on taxonomic and functional composition of phytoplankton communities. J. Plankton Res. 38, 1334–1346. doi: 10.1093/plankt/fbw062
Margalef, R. (1978). Life forms of phytoplankton as survival alternatives in an unstable environment. Oceanologia Acta 1, 493–509.
Massie, T. M., Blasius, B., Weithoff, G., Gaedke, U., and Fussmann, G. F. (2010). Cycles, phase synchronization, and entrainment in single-species phytoplankton populations. Proc. Natl. Acad. Sci. U.S.A. 107, 4236–4241. doi: 10.1073/pnas.0908725107
McKay, R., Naish, T., Carter, L., Riesselmann, C., Dunbar, R., Sjunneskog, C., et al. (2012). Antarctic and Southern Ocean influences on Late Pliocene global cooling. Proc. Natl. Acad. Sci. U.S.A. 109, 6423–6428. doi: 10.1073/pnas.1112248109
Meyer, J., and Riebesell, U. (2015). Reviews and syntheses: responses of coccolithophores to ocean acidification: a meta-analysis. Biogeosciences 12, 1671–1682. doi: 10.5194/bg-12-1671-2015
Moser, G. A. O., Piedras, F. R., Oaquim, A. B. J., Souza, D. S., Leles, S. G., de Lima, D. T., et al. (2017). Tidal effects on phytoplankton assemblages in a near-pristine estuary: a trait-based approach for the case of a shallow tropical ecosystem in Brazil. Mar. Ecol. 38:4. doi: 10.1111/maec.12450
Mouillot, D., Spatharis, S., Reizopoulou, S., Laugier, T., Sabetta, L., Basset, A., et al. (2006). Alternatives to taxonomic-based approaches to assess changes in transitional water communities. Aquat. Conserv. Mar. Freshw. Syst. 16, 469–482. doi: 10.1002/aqc.769
Müller, M. N., Trull, T. W., and Hallegraeff, G. M. (2015). Differing responses of three Southern Ocean Emiliania huxleyi ecotypes to changing seawater carbonate chemistry. Mar. Ecol. Prog. Ser. 531, 81–90. doi: 10.3354/meps11309
Mutshinda, C. M., Finkel, Z. V., Widdicombe, C. E., and Irwin, A. J. (2017). Phytoplankton traits from long-term oceanographic time-series. Mar. Ecol. Prog. Ser. 576, 11–25. doi: 10.3354/meps12220
Narwani, A., Alexandrou, M. A., Herrin, J., Vouaux, A., Zhou, C., Oakley, T. H., et al. (2015). Common ancestry is a poor predictor of competitive traits in freshwater green algae. PLoS One 10:9. doi: 10.1371/journal.pone.0137085
Naselli-Flores, L., and Barone, R. (2011). Fight on plankton! Or, phytoplankton shape and size as adaptive tools to get ahead in the struggle for life. Cryptogam. Algol. 32, 157–204. doi: 10.7872/crya.v32.iss2.2011.157
Paerl, H. W. (1988). “Growth and reproductive strategies of freshwater blue-green algae (Cyanobacteria),” in Growth and Reproductive Strategies in Freshwater Phytoplankton, ed. C. D. Sandgren (Cambridge: Cambridge University Press), 261–315.
Pomati, F., Matthews, B., Jokela, J., Schildknecht, A., and Ibelings, B. (2012). Effects of re-oligotrophication and climate warming on plankton richness and community structure in a deep mesotrophic lake. Oikos 121, 1317–1327. doi: 10.1111/j.1600-0706.2011.20055.x
Reynolds, C. S. (1988). “Functional morphology and the adaptive strategies of freshwater phytoplankton,” in Growth and Reproductive Strategies in Freshwater Phytoplankton, ed. C. D. Sandgren (Cambridge: Cambridge University Press), 388–433.
Reynolds, C. S. (1997). Vegetation Processes in the Pelagic: A Model for Ecosystem Theory. Luhe: Ecology Institute.
Reynolds, C. S., Huszar, V., Kruk, C., Naselli-Flores, L., and Melo, S. (2002). Towards a functional classification of the freshwater phytoplankton. J. Plankton Res. 24, 417–428. doi: 10.1093/plankt/24.5.417
Richardson, K., Beardall, J., and Raven, J. A. (1981). Adaptation of unicellular algae to irradiance: an analysis of strategies. New Phytol. 93, 157–191. doi: 10.1111/j.1469-8137.1983.tb03422.x
Rickaby, R. E. M., Hermoso, M., Lee, R. B. Y., Rae, B. D., Heureux, A. M. C., Balestreri, C., et al. (2016). Environmental carbonate chemistry selects for phenotype of recently isolated strains of Emiliania huxleyi. Deep Sea Res. II 127, 28–40. doi: 10.1016/j.dsr2.2016.02.010
Rodríguez, J., Tintoré, J., Allen, J. T., Blanco, J. M., Gomis, D., Reul, A., et al. (2001). Mesoscale vertical motion and the size structure of phytoplankton in the ocean. Nature 410, 360–363. doi: 10.1038/35066560
Rosati, I., Bergami, C., Stanca, E., Roselli, L., Tagliolato, P., Oggioni, A., et al. (2017). A thesaurus for phytoplankton trait-based approaches: development and applicability. Ecol. Inform. 42, 129–138. doi: 10.1016/j.ecoinf.2017.10.014
Santana, L. M., Weithoff, G., and Ferragut, C. (2017). Seasonal and spatial functional shifts in phytoplankton communities of five tropical reservoirs. Aquat. Ecol. 51, 531–543. doi: 10.1007/s10452-017-9634-3
Schlüter, L., Lohbeck, K. T., Gröger, J. P., Riebesell, U., and Reusch, T. B. H. (2016). Long-term dynamics of adaptive evolution in a globally important phytoplankton species to ocean acidification. Sci. Adv. 2:e1501660. doi: 10.1126/sciadv.1501660
Segura, A. M., Kruk, C., Calliari, D., and Fort, H. (2013). Use of morphology-based functional approach to model phytoplankton community succession in a shallow subtropical lake. Freshw. Biol. 58, 504–512. doi: 10.1111/j.1365-2427.2012.02867.x
Shurin, J. B., Abbott, R. L., Deal, M. S., Kwan, G. T., Litchman, E., McBride, R. C., et al. (2013). Industrial-strength ecology: trade-offs and opportunities in algal biofuel production. Ecol. Lett. 16, 1393–1404. doi: 10.1111/ele.12176
Shurin, J. B., Mandal, S., and Abbott, R. L. (2014). Trait diversity enhances yield in algal biofuel assemblages. J. Appl. Phycol. 51, 603–611. doi: 10.1111/1365-2664.12242
Smith, S. L., Pahlow, M., Merico, A., Acevedo-Trejos, E., Sasai, Y., Yoshikawa, C., et al. (2016). Flexible phytoplankton functional type (FlexPFT) model: size-scaling of traits and optimal growth. J. Plankton Res. 38, 977–992. doi: 10.1093/plankt/fbv038
Smol, J. P., Wolfe, A. P., Birks, H. J. B., Douglas, M. S. V., Jones, V. J., Korhola, A., et al. (2005). Climate-driven regime shift in the biological communities of arctic lakes. Proc. Natl. Acad. Sci. U.S.A. 102, 4397–4402. doi: 10.1073/pnas.0500245102
Sommer, U., Gliwicz, Z. M., Lampert, W., and Duncan, A. (1986). The PEG-model of seasonal succession of planktonic events in fresh waters. Arch. Hydrobiol. 106, 433–471.
Sommer, U., Sommer, F., Santer, B., Jamieson, C., Boersma, M., Becker, C., et al. (2001). Complementary impact of copepods and cladocerans on phytoplankton. Ecol. Lett. 4, 545–550. doi: 10.1046/j.1461-0248.2001.00263.x
Sprules, W. G., and Goyke, A. P. (1994). Size-based structure and production in the pelagia of Lakes Ontario and Michigan. Can. J. Fish. Aquat. Sci. 51, 2603–2611. doi: 10.1139/f94-260
Strom, S. L. (2008). Microbial ecology of ocean biogeochemistry: a community perspective. Science 320, 1043–1045. doi: 10.1126/science.1153527
Taherzadeh, N., Kerimoglu, O., and Wirtz, K. W. (2017). Can we predict phytoplankton community size structure using size scalings of eco-physiological traits? Ecol. Model. 360, 279–289. doi: 10.1016/j.ecolmodel.2017.07.008
Terseleer, N., Bruggeman, J., Lancelot, C., and Gypens, N. (2014). Trait-based representation of diatom functional diversity in a plankton functional type model of the eutrophied southern North Sea. Limnol. Oceanogr. 59, 1958–1972. doi: 10.4319/lo.2014.59.6.1958
Touzet, N., Franco, J. M., and Raine, R. (2008). Morphogenetic diversity and biotoxin composition of Alexandrium (Dinophyceae) in Irish coastal waters. Harmful Algae 7, 782–797. doi: 10.1016/j.hal.2008.04.001
Tsai, C.-H., Miki, T., Chang, C.-W., Ishikava, K., Ichise, S., Kumagai, M., et al. (2014). Phytoplankton functional group dynamics explain species abundance distribution in a directionally changing environment. Ecology 95, 3335–3343. doi: 10.1890/13-1946.1
Vadrucci, M. R., Barbone, E., Ungaro, N., Romano, A., and Bucci, R. (2017). Application of taxonomic and morpho-functional properties of phytoplankton communities to water quality assessment for artificial lakes in the Mediterranean Ecoregion. J. Plankton Res. 39, 550–563. doi: 10.1093/plankt/fbx011
Vallina, S. M., Cermeno, P., Dutkiewicz, S., Loreau, M., and Montoya, J. M. (2017). Phytoplankton functional diversity increases ecosystem productivity and stability. Ecol. Model. 361, 184–196. doi: 10.1016/j.ecolmodel.2017.06.020
Villéger, S., Mason, N. W. H., and Mouillot, D. (2008). New multidimensional functional indices for a multifaceted framework in functional ecology. Ecology 89, 2290–2301. doi: 10.1890/07-1206.1
Violle, C., Navas, M.-L., Vile, D., Kazakou, E., Fortunel, C., Hummel, I., et al. (2007). Let the concept of trait be functional! Oikos 116, 882–892. doi: 10.1111/j.0030-1299.2007.15559.x
Vladimirskaya, E. V., Makarov, R. R., Maslennikov, V. V., and Movchan, O. A. (1976). Some traits of phytoplankton distribution. Okeanologyia 16, 1069–1075.
Weithoff, G. (2003). The concepts of ‘plant functional types’ and ‘functional dversity’ in lake phytoplankton – a new understanding of phytoplankton ecology? Freshwat. Biol. 48, 1669–1675. doi: 10.1046/j.1365-2427.2003.01116.x
Weithoff, G., and Gaedke, U. (2017). Mean functional traits of lake phytoplankton reflect seasonal and inter-annual changes in nutrients, climate and herbivory. J. Plankton Res. 39, 509–517.
Weithoff, G., Rocha, M. R., and Gaedke, U. (2015). Comparing seasonal dynamics of functional and taxonomic diversity reveals the driving forces underlying phytoplankton community structure. Freshw. Biol. 60, 758–767. doi: 10.1111/fwb.12527
Willis, A., Chuang, A. W., Woodhouse, J. N., Neilan, B. A., and Burford, M. A. (2016). Intraspecific variation in growth, morphology and toxin quotas for the cyanobacterium, Cylindrospermopsis raciborskii. Toxicon 119, 307–310. doi: 10.1016/j.toxicon.2016.07.005
Zhao, Y., and Quigg, A. (2014). Nutrient Limitation in Northern Gulf of Mexico (NGOM): phytoplankton communities and photosynthesis respond to nutrient pulse. PLoS One 9:e88732. doi: 10.1371/journal.pone.0088732
Keywords: algae, functional traits, ocean, lake, biogeochemistry, community assembly
Citation: Weithoff G and Beisner BE (2019) Measures and Approaches in Trait-Based Phytoplankton Community Ecology – From Freshwater to Marine Ecosystems. Front. Mar. Sci. 6:40. doi: 10.3389/fmars.2019.00040
Received: 30 April 2018; Accepted: 28 January 2019;
Published: 12 February 2019.
Edited by:
Kalle Olli, University of Tartu, EstoniaReviewed by:
Alberto Basset, University of Salento, ItalyCopyright © 2019 Weithoff and Beisner. This is an open-access article distributed under the terms of the Creative Commons Attribution License (CC BY). The use, distribution or reproduction in other forums is permitted, provided the original author(s) and the copyright owner(s) are credited and that the original publication in this journal is cited, in accordance with accepted academic practice. No use, distribution or reproduction is permitted which does not comply with these terms.
*Correspondence: Guntram Weithoff, d2VpdGhvZmZAdW5pLXBvdHNkYW0uZGU=
Disclaimer: All claims expressed in this article are solely those of the authors and do not necessarily represent those of their affiliated organizations, or those of the publisher, the editors and the reviewers. Any product that may be evaluated in this article or claim that may be made by its manufacturer is not guaranteed or endorsed by the publisher.
Research integrity at Frontiers
Learn more about the work of our research integrity team to safeguard the quality of each article we publish.