- Plymouth Marine Laboratory, Plymouth, United Kingdom
Benthic macrofauna, as an element of rich and diverse benthic communities of the shelf seas, play a key role in marine biogeochemical cycles and support a wide range of ecosystem services. To better understand how macrofauna affects mass and energy fluxes within the seabed and between the bed and the pelagic, it is fundamental to characterise their structural and dynamic response to the quantity, quality and timing of food supply. To do so, we have combined long-term time-series of pelagic productivity and macrofaunal abundance with a model of benthic food web to: (1) estimate the characteristic response time scales of major groups of benthic macrofauna to food availability, (2) relate these to carbon fluxes within sediments and across the benthic–pelagic boundary, and (3) explore the mechanisms responsible. The model was designed as a canonical representation of the benthic system, retaining the key pathways that connect benthic macrofauna to pelagic environment, but aggregating variables and groups that were not explicitly observed. Both observations and model simulations revealed pronounced differences between deposit and suspension feeders in their rate of response to phytoplankton blooms: deposit feeders showed a dampened response lagging 26–125 days behind the peak in pelagic production, while suspension feeders responded rapidly, within only 5–7 days. The model parametrisation obtained during calibration relates this to differences in feeding modes, in (trophic) proximity to primary production and in rates of ingestion and losses. Specifically, suspension feeders are predicted to act as a gateway to pelagic productivity, controlling the quantity of organic carbon reaching sediment-dwelling fauna.
Introduction
Shelf seas are productive regions due to external and internal nutrient input and adequate light availability (Nixon et al., 1986; Joint and Pomroy, 1993; Jickells, 1998). With the majority of shelf sea areas being relatively shallow (water depths typically less than 200 m), considerable amounts of organic matter produced in the water column are rapidly deposited at the seafloor, sustaining rich and diverse benthic communities (e.g., Heip et al., 1992; Dauwe et al., 1998; Snelgrove, 1998, 1999; Martins et al., 2013). Inter-annual and seasonal variability in this pelagic food supply, specifically its quantity, quality and timing of deposition, directly affects many aspects of benthic community structure such as the abundance, biomass and species richness of benthic macrofauna (e.g., Gooday et al., 1990; Gili and Coma, 1998; Coma et al., 2000; Norkko et al., 2001; Ruhl and Smith, 2004). As a consequence, organic matter supply is a key driver in setting the dominant functional traits of benthic communities and can have a significant impact on biogeochemical cycles in sediments and benthic–pelagic coupling (e.g., Norling et al., 2007; Kristensen et al., 2014). Understanding the underlying mechanisms of macrofaunal response to food supply and availability is therefore crucial for quantifying and predicting particulate, solute and energy fluxes at the seabed and beyond, with implications for, but not limited to, impacts on nitrogen cycling, carbon sequestration and food provision, since benthic macrofauna play a significant role as an element in sustaining fish and fisheries, and directly as a food source for human consumption (Heip and Craeymeersch, 1995).
The response of coastal marine sediments and their biological communities to temporal variability in the pelagic environment has been a subject of several recent studies. For instance, Franco et al. (2007) observed shifts in benthic bacterial communities related to phytoplankton bloom deposition in the Belgian coastal sea. Similarly, Tait et al. (2015) reported a rapid response of benthic bacterial communities to a large phytoplankton bloom and showed a correlation between bacterial abundance and chlorophyll concentrations at station L4 in Western English Channel. Kitidis et al. (2017) studied the seasonality of microbially driven nitrogen cycling in the Celtic Sea during 2015 and reported increased process rates during the post-bloom period. In contrast to a relatively large number of microbially focussed studies, such as those just mentioned, fewer studies have focused specifically on the dynamics and time scales of response of benthic macrofauna to pelagic production in shelf seas. Those studies highlight the importance of pelagic production in fuelling benthic macrofaunal community change. For example, Austen et al. (1991) reported covariance of macrofauna in the Western and Eastern North Sea with phytoplankton colour and total zooplankton abundance in 1958–1988. In a later study, rapid and complex responses were observed in the majority of benthic taxa and functional groups to a large phytoplankton bloom (Zhang et al., 2015). However, the link between organic matter supply and some elements of the benthic community is not always as clear, as illustrated by Navarro-Barranco et al. (2017). These authors studied the response of amphipods to phytoplankton biomass over a 7-year period at the same location as the Zhang et al. (2015) study, and did not find any significant correlations with food supply, but did find close correlation with bottom water temperatures. This highlights the complexity and variability of response to pelagic production by various functional groups of benthic fauna.
The availability of complimentary physical, chemical and biological observational time-series data, collected concurrently from the same geographical location is fundamental for understanding and quantifying the response of benthic fauna to phytoplankton dynamics. Whilst multi-year coverage in measurements is necessary to study inter-annual variability, in order to understand intra-annual coupling seasonal sampling representative of successive ecosystem states is necessary. The sparsity of such observational data in terms of spatio-temporal coverage and the number of observed variables hinders our ability to discern connections and quantify fluxes both within the sediments and between pelagic and benthic environments. In this regard, the time-series data collected within the Western Channel Observatory (WCO) (Smyth et al., 2015), which include long-term weekly phytoplankton and nutrient measurements, as well as seasonal sampling of benthic macrofauna, present a unique opportunity to explore the connectivity between pelagic production and benthic community structure and function. Once integrated with marine biogeochemical models, such as the European Regional Seas Ecosystem Model (ERSEM) (Butenschön et al., 2016), these data allow for single coherent description of fluxes between ecosystem compartments, which together lead to the observed states.
In recent years, numerical models of different complexity have been developed and applied at various spatio-temporal scales in order to better understand, quantify and predict the dynamics of benthic environments and the links between the seabed and the overlying water column (e.g., Soetaert and Middelburg, 2009; Thullner et al., 2009; Stolpovsky et al., 2015; Capet et al., 2016; Dale et al., 2016; Yakushev et al., 2017, to name a few). These models are implemented either in isolation, forced by prescribed inputs, or dynamically coupled to models of the pelagic environment. Whilst serving their specific goals, the majority of benthic models do not explicitly represent biota within sediment. In case of macrofauna, their contribution to sediment particle displacement and mixing (bioturbation) is at most parameterised via non-local exchange or local biodiffusive modelling formalisms (Meysman et al., 2003), and to enhanced solute transport (bioirrigation) via model formulations of varying complexity (Meysman et al., 2006).
European Regional Seas Ecosystem Model, a generic model of marine biogeochemistry and the ecosystem dynamics of the lower trophic levels, includes explicit parameterisations of several functional groups of benthic biota: two types of macrofauna, namely deposit feeders and suspension feeders, meiofauna, and two types of benthic bacteria (Ebenhöh et al., 1995; Blackford, 1997; Butenschön et al., 2016). This makes ERSEM particularly applicable for studies of biological interactions within sediments and benthic–pelagic coupling (e.g., Lessin et al., 2016). Since the modularisation of ERSEM adopting Framework for Aquatic Biogeochemical Models (FABM) (Bruggeman and Bolding, 2014), the model structure can be modified and rescaled depending on specific research needs and in accordance with the complexity and level of understanding of particular ecosystems.
Here, we have constructed a canonical model of the benthic food web based on the modular components of ERSEM, and integrated it with sustained long-term observational data, with an aim to explore how pelagic production shapes the structure and temporal dynamics of benthic macrofaunal communities in a shallow shelf sea. This framework is applied to estimate the characteristic response times of different functional groups of benthic macrofauna to pelagic production and food availability, and to quantify their contributions to fluxes of carbon within the benthic environment and across benthic–pelagic interface. The implemented approach of combining modelling and observational data provides further insight into possible physiological and ecological mechanisms underlying differing response in macrofaunal functional groups.
Materials and Methods
Study Site
Our study site is station L4 (50°15.00’N, 4°13.02’W, depth 50 m), located 13 km southwest of Plymouth, United Kingdom (Figure 1). Station L4 is one of the main sampling sites of the WCO1: an oceanographic long-term time-series, a marine biodiversity reference site and one of the best-studied marine regions in Europe (Smyth et al., 2015). Observations of pelagic physical, biogeochemical and biological parameters have been made at L4 for more than 100 years, with considerably increased frequency and detail over the past 25 years. Starting in 2008, a regular benthic survey was initiated, which includes sampling of macrofauna.
Observational Data
As part of the regular sampling at L4, macrofauna samples are collected in four-five replicates using a 0.1 m2 USNL type boxcorer. These samples are sieved over a 0.5 mm mesh and preserved in 10% formalin. In the laboratory all macrofauna are extracted from the sample, identified to species level where possible and weighed (wet mass). Macrofauna dataset used within this study (Dashfield and McNeill, 2014), covering period 2008–2013, is available from the British Oceanographic Data Centre (BODC) database2.
To allow for comparison with model results, we converted species-specific wet mass (WM) values of macrofauna into carbon mass (CM). Species-specific CM/WM ratios were based on the dataset from Brey et al. (2010), which was extrapolated to other species using PhyloPars (Bruggeman et al., 2009), a trait inference method that accounts for taxonomic relationships between species and correlations between traits. Subsequently, carbon masses and their associated variance estimates were summed over two groups of species: suspension feeders and deposit feeders. Species were classified as suspension- or deposit feeders based on their affinity for each of these feeding modes (highest wins); in turn, this affinity was estimated using PhyloPars to extrapolate from feeding modes of a subset of species3 to other species. Mass conversion coefficients and feeding mode affinities are available online at http://www.marine-ecosystems.org.uk/Trait_Explorer. Dominant classes and species of deposit- and suspension feeders at L4 are shown on Figure 2. Supplementary Material 1 contains a complete list of inferred trait values for the feeding mode and CM/WM ratios of all infaunal species sampled at L4, as well as details on PhyloPars method and its performance.
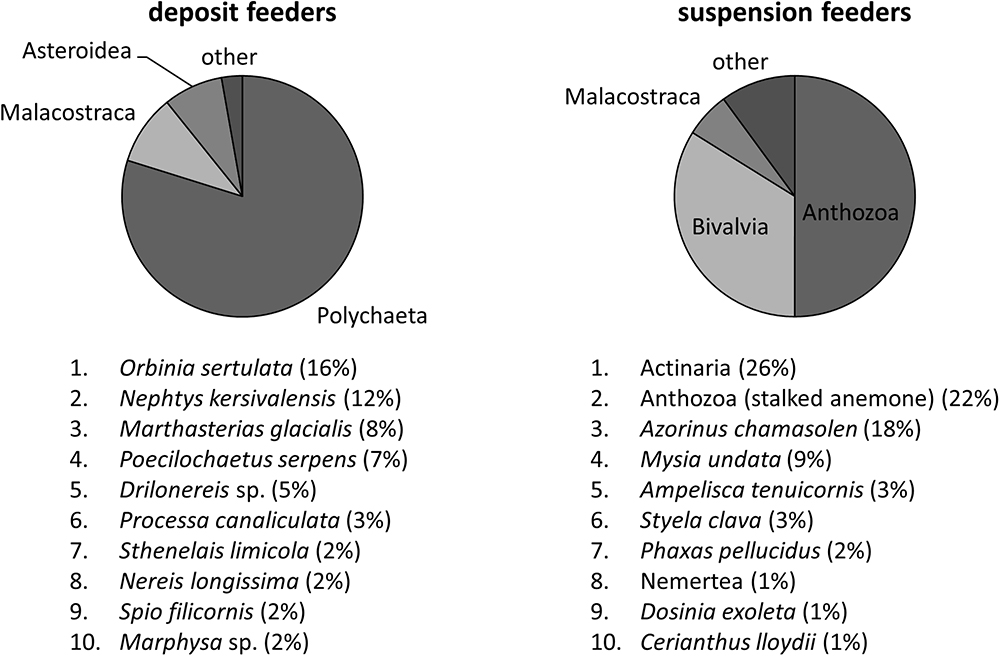
Figure 2. Dominant classes and species of deposit- and suspension feeders at L4. These were obtained by computing their relative contribution to biomass for every sample, and then averaging over all samples. It thus represents the expected fraction of a given class/species in a single sample, independent of its total biomass.
Times-series data of phytoplankton abundance measured at a water depth of 10 m (Widdicombe et al., 2010) were converted into carbon mass and used to drive the model. Time-series of in situ water temperature measured at 50 m depth were used as a proxy of the water temperature experienced by benthic fauna.
Model Design
To quantitatively describe the dynamics of benthic macrofaunal response to pelagic production, we constructed a canonical model of the benthic community food web using components of ERSEM (Butenschön et al., 2016). Our aim here was to retain those components of the macrofaunal food web for which observations are available, while simultaneously representing key pathways that connect benthic fauna to the pelagic environment. Below we present a conceptual description of the constructed canonical model; its detailed mathematical formulation and full list of parameters are given in the Supplementary Material 2.
The benthic model is driven by pelagic inputs, which on their own can be a source of considerable uncertainty in benthic modelling. Fortunately, we have detailed information on pelagic production in the form of a time-series of phytoplankton biomass and community composition at 10 m depth, at 1–2 weekly resolution. In this study, we have chosen to estimate deposition at the bed directly from this phytoplankton and a (calibrated) average sinking rate, instead of explicitly modelling pelagic processes with ERSEM components. This has two reasons. First, station L4 is highly dynamic, tidally influenced and episodically affected by inputs from nearby rivers (Smyth et al., 2015), which limits the ability to reproduce inter-annual dynamics and episodic changes in pelagic communities in idealised 1D model settings (Butenschön et al., 2016). Simulated pelagic production, even when calibrated against phytoplankton time-series, would therefore not be able to fully capture the key inter-annual and episodic changes in benthic inputs. Second, the connection between 10 m phytoplankton and the bed at 50 m depth involves considerable array of pelagic processes (e.g., aggregation, sinking) that are still poorly understood and thus subject to considerable uncertainty in their model formulation. Therefore, aiming to minimise the uncertainty associated with pelagic parametrisations, our benthic model was directly driven with an observed time-series of phytoplankton as a proxy of deposition of organic material. While other sources of carbon, such as microphytobenthos, seagrasses and macroalgae, may contribute to the pool of organic material available to benthic macrofauna (e.g., Smale et al., 2013), including them in this study would require detailed observational and experimental datasets describing inputs of non-planktonic material to sediment organic matter pool and its availability for macrofaunal uptake. These datasets will inform future model design and studies of benthic–pelagic interactions.
As we are particularly interested in the time scale at which benthic fauna respond to pelagic production, we differentiate two reservoirs of particulate organic carbon (POC), changing on very different time scales: first, a “fluff layer” at the sediment–water interface, spanning a continuum of near-bed suspended to newly deposited matter. Its operational definition is that it is supplied by phytoplankton sinking to the seabed, and easily resuspended again by tidal currents. Resuspension can be followed rapidly by sinking and deposition (i.e., no net change in fluff takes place), or by lateral transport taking material away from the site. The latter is included in the model by allowing resuspension that causes material to be removed from the system. The second POC reservoir represents a labile POC pool within the consolidated sediment, formed by benthic egestion and by direct incorporation of fluff.
We modelled two functional groups of benthic macrofauna: suspension- and deposit feeders. Both can feed on fluff, while deposit feeders are in addition allowed to utilise POC from within the sediment. Of all carbon ingested by benthic fauna, only part is used to produce new biomass. The remainder is either incorporated into POC within sediments through egestion and mortality, or is altogether lost to carbon pools not tracked by the model: it exits through respiration (as DIC), excretion (as DOC) or injection into the pelagic environment (e.g., through production of pelagic eggs or offspring, or consumption by pelagic predators). This loss to untracked carbon pools is represented by a bulk temperature-dependent and biomass-specific rate. Life stages of macrofauna, including the processes of reproduction and recruitment, are not explicitly considered in the model.
Aiming, wherever possible, to restrict the model structure to include variables with a corresponding observational equivalent, we aggregated those pools that are difficult to measure separately and that fulfil a similar role in relation to macrofauna. Specifically, benthic bacteria and meiofauna are difficult to distinguish from high quality POC by both experimental methods and by feeding macrofauna. Accordingly, these groups are not modelled separately but implicitly included as part of the POC pool. Observational data detailing seasonal and inter-annual dynamics of benthic bacterial and meiofaunal communities, as well as more experimental evidence for their functional linkages to macrofauna will allow for a more comprehensive resolution of the modelled food web.
Within the sediment, we considered one type of POC only. As this pool is a food source for the modelled deposit feeders, it is effectively organic matter of relatively high quality. Refractory material can play an important role in carbon cycling through interactions with benthic biota. Its mineralisation is enhanced due to exposure to oxygen during bioturbation (e.g., Andersen and Kristensen, 1992; Kristensen et al., 1992; Heilskov and Holmer, 2001). In some systems, as a result of niche partitioning, macrofauna may rely on uptake of more refractory material (Iken et al., 2010). However, in the absence of sufficient data on quality spectra of refractory material and its availability for direct uptake by macrofauna, for simplicity refractory compounds were not explicitly considered in our study. Both types of modelled organic matter (fluff and POC) are a subject to first-order temperature-dependent mineralisation.
A conceptual diagram representing model stocks and fluxes is shown in Figure 3. The modelled period covers years 2003–2013, where the first 5 years of simulation (prior to the beginning of WCO benthic survey) are regarded as a spin-up period and thus excluded from the consecutive analysis.
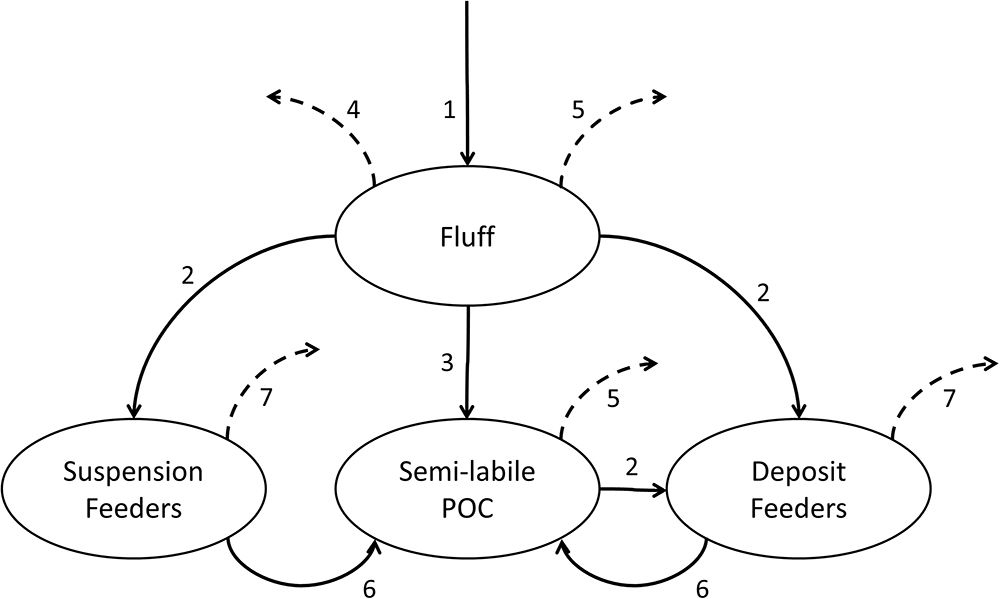
Figure 3. Conceptual diagram of the constructed canonical model of benthic macrofauna. The processes modelled are: phytoplankton deposition into fluff (1), uptake of fluff or semi-labile POC by benthic fauna (2), incorporation of fluff into sediment POC pool (3), fluff resuspension (4), mineralisation of fluff and semi-labile POC (5), egestion and mortality (6) and bulk losses from macrofauna (7). Fluxes into compartments not tracked within the model are indicated by dashed arrows.
Calibration
Forcing the model with the observed time-series of phytoplankton and temperature, we calibrated a subset of model parameters related to suspension- and deposit feeders’ food availability (phytoplankton deposition rate, fluff erosion rate, mineralisation rate of fluff and benthic POC), food uptake (maximum ingestion rates, food half-saturation concentrations, preference of deposit feeders for fluff), as well as rate of loss into compounds not tracked within the model. These parameters were optimised to obtain the best fit between modelled and observed biomasses of macrofaunal groups. The criterion for establishing the “best fit” was based on maximum likelihood, assuming a normal distribution of model-observation differences with a standard deviation that is variable- and sample- specific. In this case, maximum likelihood estimation is equivalent to minimising the sum of squares of model–observation differences, with each squared difference weighted by the square of the associated standard deviation. Standard deviations were taken equal to the standard deviation of each observed sample. Calibration of parameters was performed with the differential evolution (DE) optimisation algorithm (Storn and Price, 1997), assuming predefined search ranges for each parameter calibrated, as shown in Table 1. DE is a conservative optimisation method designed to find global optima. In our case, it took over 800,000 simulations, gradually narrowing the parameter search range to identify an optimal parameter set (Table 1), which produced the model-data fit shown on Figure 4.
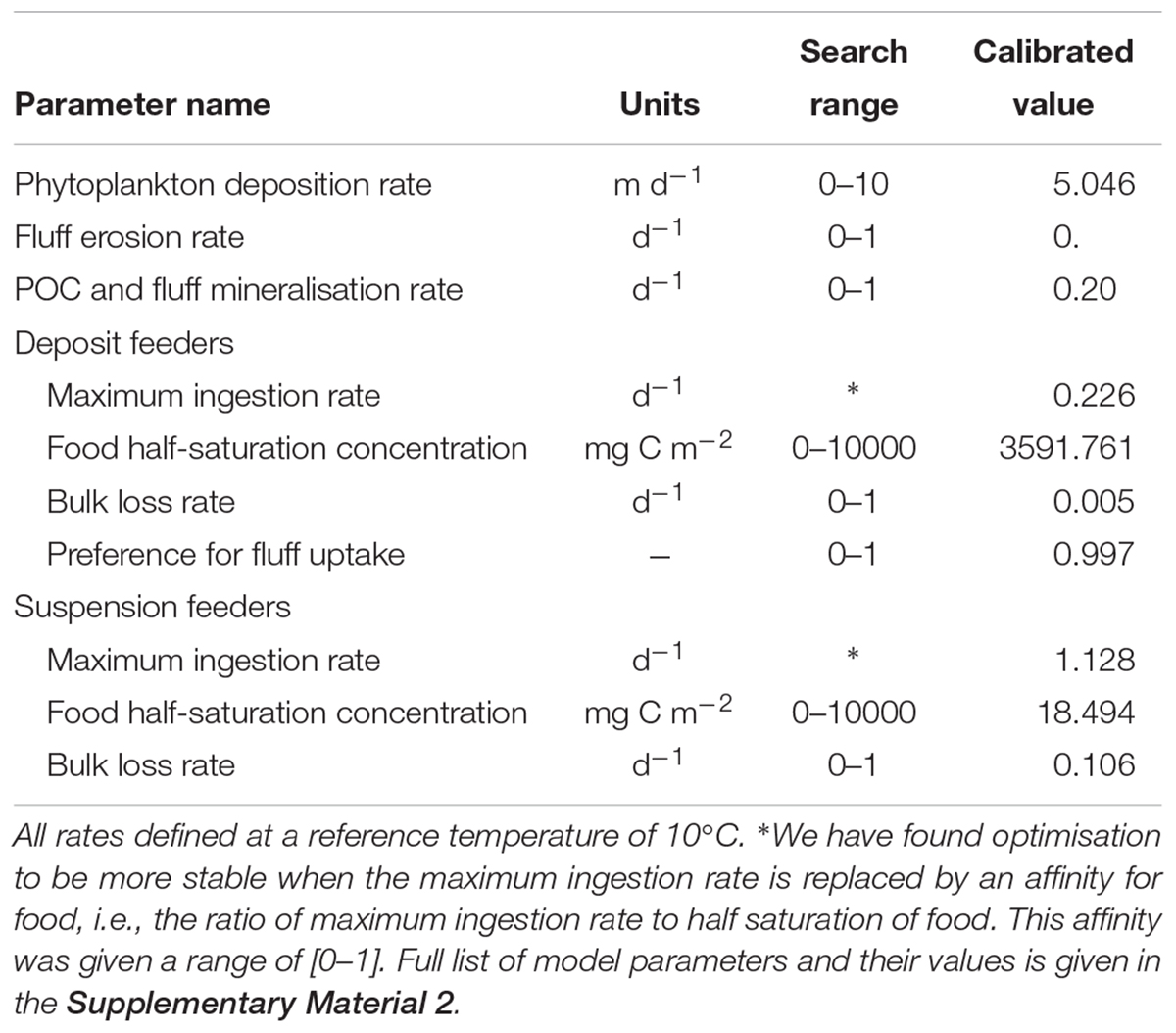
Table 1. Calibrated parameters of the model, their units and predefined search ranges applied for optimisation, as well as final calibrated values of these parameters.
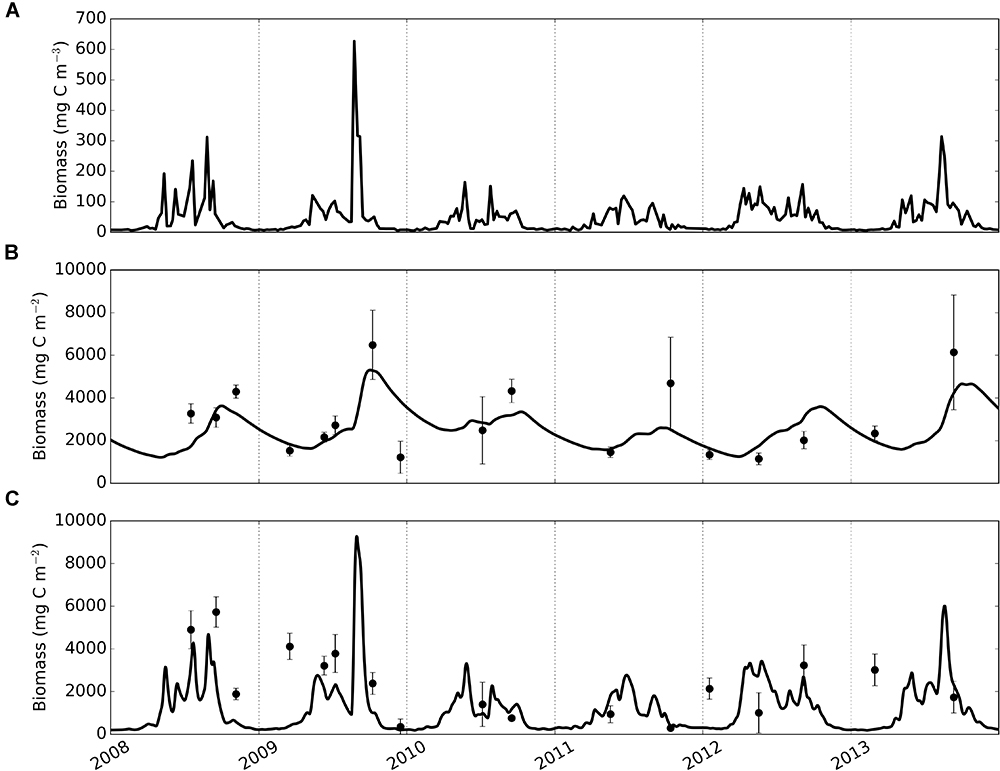
Figure 4. Time-series of phytoplankton (A), modelled and observed biomass of deposit feeders (B) and suspension feeders (C). Solid lines – model, dots and error bars – mean values and standard deviations of sample replicates in observational data.
Time Lag Analysis
The response time of macrofauna to changes in pelagic production was estimated from the cross-correlation of time-series of phytoplankton and benthic macrofauna biomass. Within entire time-series of 2008–2013, cross-correlations for time lags ranging from 1 to 365 days were evaluated in order to focus on the seasonal (rather than inter-annual) response. The cross-correlation analysis was performed twice: first we estimated the observed response time from the cross-correlation of observed phytoplankton and observed benthic macrofauna; subsequently we estimated the modelled response time from cross-correlation of observed phytoplankton (the model driver) and modelled macrofauna.
Results
Model-Data Comparison
Macrofaunal biomasses simulated by the calibrated model were compared with time-series of observed biomass (Figure 4). Generally, the model can be seen to track observations with smaller uncertainty more closely than observations with high uncertainty; this is a direct consequence of the fact that model-observation differences were weighted by the sample’s standard deviation during calibration. Modelled deposit feeders (Figure 4B) showed seasonal dynamics with biomasses steadily increasing post-bloom until autumn, and then declining to lower values (minimum biomass 1215 mg C m-2 during 2008–2013) prior to the following year’s spring phytoplankton bloom. Modelled suspension feeders (Figure 4C) exhibited pronounced seasonality with rapid biomass increase in spring, followed by a similarly fast decrease in autumn. The lowest biomasses of suspension feeders (down to a minimum value of 186 mg C m-2) are seen during winter months.
Modelled deposit feeders showed a clear response to phytoplankton blooms through a delayed but steady increase in biomass. This response pattern persisted through the seasons: compared with pelagic productivity, the trend in deposit feeder biomass was consistently dampened and offset in time. Conversely, modelled suspension feeders exhibited pronounced temporal variability and were predicted to closely track pelagic phytoplankton. For example, following the high spike in phytoplankton in 2009, the model showed a rapid increase of suspension feeders’ biomass, followed by a similarly quick decrease. There is a modest support in the observations for such close tracking: observed biomasses are generally lower in winter than in summer and there are no indications of suspension feeder biomass lagging noticeably behind peaks in phytoplankton productivity.
Time Lag of Macrofaunal Response to Pelagic Production
Cross-correlation of phytoplankton and observed deposit feeder biomass (Figure 5A, dashed line) peaked at a time lag of 50 days (correlation coefficient 0.75), and generally higher correlations were found at lags between 26 and 125 days (correlation coefficients higher than 0.55). Cross-correlation of phytoplankton and modelled deposit feeder biomass (Figure 5A, solid line) showed a similar pattern, with a maximum correlation at a time lag of 73 days (correlation coefficient 0.55).
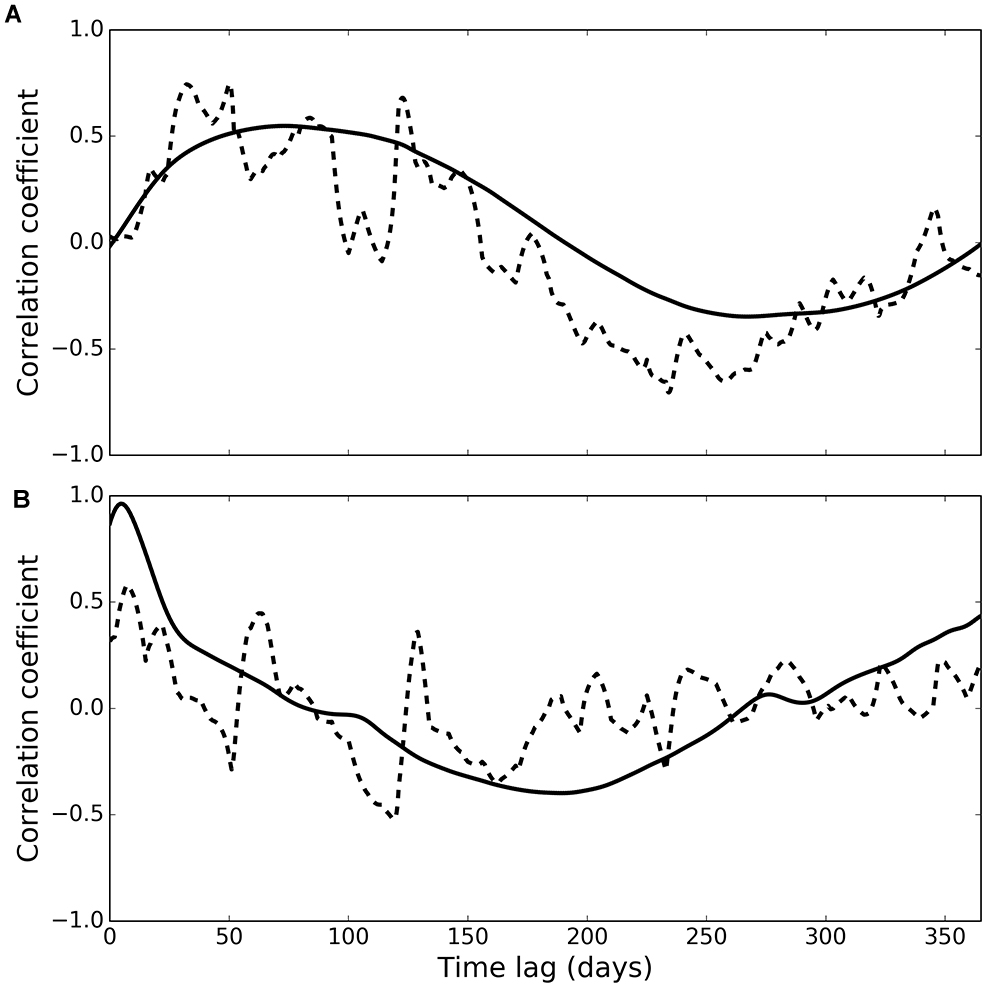
Figure 5. Cross-correlation of phytoplankton with observed (dashed line) and modelled (solid line) deposit feeders (A) and suspension feeders (B).
Cross-correlation of phytoplankton and observed suspension feeder biomass (Figure 5B, dashed line) showed the highest correlation at a very small time lag of only 7 days (correlation coefficient 0.58). This suggests suspension feeders respond near-instantaneously to changes in pelagic phytoplankton. Cross-correlation of phytoplankton and modelled suspension feeders (Figure 5B, solid line) showed similar pattern, but with even shorter time lag of 5 days and a stronger correlation (correlation coefficient 0.96).
Fluxes of Carbon From Pelagic and Within Benthic Environment
Daily mean fluxes of carbon between model compartments averaged over the simulation period (excluding spin-up) are shown in Figure 6. Following phytoplankton deposition into the fluff layer, the great majority was ingested by suspension feeders; only a very small fraction was ingested by sediment-dwelling deposit feeders or directly incorporated into the sediment. Of all carbon ingested by suspension feeders, 64% was lost to untracked reservoir. As a result, suspension feeders were responsible for most of the loss of deposited carbon. The remaining ingested carbon was incorporated in the sediment through egestion and mortality: it entered the pool of bioavailable POC. In turn, 59% of the POC was predicted to be subsequently ingested by deposit feeders; the remainder 41% was remineralised to inorganic carbon. Deposit feeders returned most of their ingested carbon (83%) back to the POC pool through egestion and mortality; the remainder 17% of its ingested carbon was lost from the system.
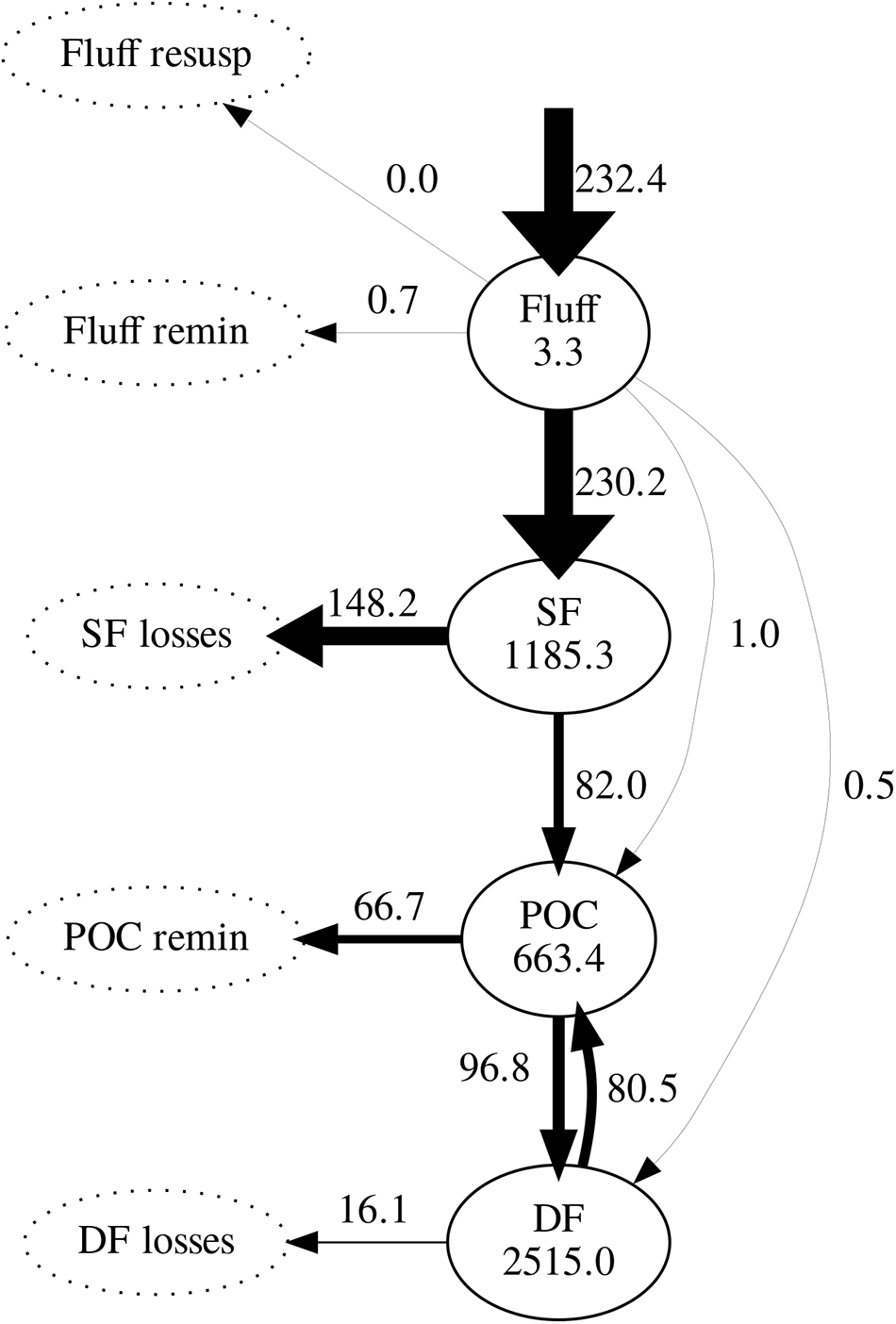
Figure 6. Modelled carbon stocks (mg C m-2) and fluxes (mg C m-2 d-1), averaged over the simulation period (2008–2013). Arrow widths are proportional to the value of maximum flux (phytoplankton deposition). Pools not tracked by the model are represented by dotted ellipses. SF, suspension feeders; DF, deposit feeders; POC, particulate organic carbon.
Discussion
On the Response of Deposit and Suspension Feeders to Food Availability
Both observations and model simulations show a pronounced difference in temporal response between deposit and suspension feeders: there are 26–125 days between the phytoplankton bloom and the peak in deposit feeder biomass, but only 5–7 days between the bloom and the peak in suspension feeder biomass. These lag times are found both in observations and model results (Figure 5). The differences in response are underlined by the emergent structure of the model food web (Figure 6): pelagic carbon flows via suspension feeders to deposit feeders. The strength and ordering of these carbon pathways is not prescribed: other connections (Figure 3; thin arrows on Figure 6) are theoretically possible and only weakened or inactivated during calibration to observations. During this process, the model recreates the observed lag between suspension and deposit feeders by placing suspension feeders in between pelagic deposition and deposit feeders. This could reflect differences in physical location (suspension feeders live close to the benthic–pelagic interface, deposit feeders deeper within sediments), as well as positioning within the food web (deposit feeders feed on waste of other benthic faunal groups, including suspension feeders). Thus, from the point of view of deposit feeders, suspension feeders are predicted to act as a gateway to pelagic productivity: they control the quantity of organic carbon reaching sediment-dwelling fauna. There is evidence for such a gateway role for benthic fauna: Tait et al. (2015) found that rapid removal of phytodetritus from the surface sediment at L4 is driven by macrofaunal consumption, and that organic material available for bacterial degradation within sediments is first processed by macrofauna. Moreover, some suspension feeder taxa produce pseudofaeces (Garrido et al., 2012), which is effectively another pathway dedicated to conversion of suspended matter into benthic deposits. The concept of suspension feeders having preferential access to pelagic food sources is not surprising and has been previously applied in modelling studies (e.g., Maar and Hansen, 2011); however, in those it was prescribed by the model structure rather than emerging as a result of model calibration, as is the case here.
The near-instantaneous response of suspension feeders to pelagic production deserves closer scrutiny. It is supported by high-frequency observations at L4: Zhang et al. (2015) found that suspension feeders more than doubled their biomass during the 2012 spring bloom, while biomass of deposit feeders remained relatively unchanged. However, the mechanism that underlies this rapid response is unclear. During calibration, the model recreates the close tracking of phytoplankton by suspension feeders by assigning them high ingestion and loss rates. The loss rate encapsulates a range of processes including respiration and DOC excretion, but potentially also the production of eggs or offspring and the loss of biomass to pelagic-dwelling predators such as fish.
Reproduction will be responsible for at least some loss of benthic biomass to reservoirs that are out of reach of our present observations, either because the released eggs or offspring are pelagic, or because they are too small to be sampled. Pelagic stages are characteristic for life cycles of many species of benthic macrofauna and can span a period from several days to few months, with seasonality and duration varying not only between, but also within species, depending on geographical location and environmental conditions (Widdows, 1991; Ansell et al., 1994). These life stages are not sampled as part of the benthic survey. Moreover, the smallest benthic life stages are not included in observed biomass due to the use of a 0.5 mm mesh during sampling. As a result, conversion of adult biomass into either planktonic life stages or small benthic offspring will manifest in the macrofauna time-series as a loss term that either directly decreases biomass or suppresses its growth. As a result, the differential responses of suspension and deposit feeders could relate to their reproductive strategies: deposit feeders might primarily respond to phytoplankton deposition by continuously releasing gametes or larvae, whereas suspension feeders could buffer ingested carbon only to spawn later in the season when deposited food becomes scarcer. Two observations point in this direction. First, in Zhang et al. (2015) study, deposit feeders responded to phytoplankton deposition by a rapid increase in abundance, but not biomass. This indicates the appearance of juveniles, and hence reproduction as primary response to enhanced food availability. Second, Lindeque et al. (2015) summarised seasonal contribution of meroplankton to total zooplankton at L4 during 1998–2010, and showed a typical increase in the relative prevalence of the offspring of (suspension feeding) bivalves toward autumn–winter.
Benthic macrofauna is an important food source for fish and large epifauna, and some part of its biomass will be lost to predation. The role of predation in shaping the structure of macrofaunal communities has been previously investigated by using manipulative field experiments (e.g., Virnstein, 1977), short-term (days) microcosm experiments (Service et al., 1992) and mid-term (weeks) flow-through systems (Nilsson et al., 1993), which confirm that predation pressure on benthic macrofauna can be significant. However, losses to predation in natural communities are more difficult to estimate. As it was summarised by Steven (1930), who extensively investigated bottom fauna and food eaten by fishes in waters off Plymouth (United Kingdom), availability of any animal for food, not taking into account considerations of size, will depend on habits and activity of the organism itself and of the fish, which will vary for the same organism with different fishes and for the different organisms with the same fish. Nevertheless, preferential predation on suspension feeders, which might contribute to rapid losses of their biomass, is expected due to their proximity to the sediment surface and, therefore, higher susceptibility to predation compared to deeper-dwelling deposit feeders. So, Steven (1930) found high, and occasionally even exclusive, predation on suspension feeders (Ampelisca) even in periods when they were scarce or seemingly absent. As Persson and Svensson (2006) showed for freshwater system, foraging bream significantly affected the community composition and vertical distribution of benthos, leading to an order of magnitude decrease in prey biomass in the upper (0–1 cm) sediment layer, whereas in the deepest layer (3–10 cm) there were no significant changes. Thus, proximity of suspension feeders’ habitat to sediment-water interface might reflect a trade-off between accessibility of food resources and exposure to predation (Compton et al., 2016).
Implications for the Modelling of Benthic Communities
The model calibration procedure applied allowed us to constrain model parameters purely from observed forcing and field measurements. This contrasts with the more traditional approach to parameterisation, based on allometric relations and driven by a range of assumptions, as also applied in the original ERSEM benthic biological submodel (Ebenhöh et al. (1995), see discussion on model parameterisation therein). Compared to the original ERSEM parameterisation, our model calibration resulted in instantaneous response of suspension feeders, implying an almost linear, non-saturating response to food availability. This allowed the model to reproduce the highly dynamic response of benthic fauna to food variability. In the original ERSEM formulation, this response is much weaker (see Supplementary Material 2): it is strongly dampened by the choice of parameter values as well as formulation, notably, the presence of several density-dependent terms (e.g., a minimum prey threshold, reduction of ingestion due to crowding). Such mechanisms were deactivated in the present model to obtain a parameter-sparse formulation with a minimum of poorly defined processes. For instance, while stress from overcrowding can influence benthic communities (Peterson and Black, 1987, 1988), exact mechanisms of its functioning and its quantitative impact across wide ranges of species are uncertain. Based on the results obtained here, we see no reason to reintroduce such mechanisms in the model until more comprehensive datasets on this topic become available.
The process of model calibration resulted in fluff resuspension rate of 0 d-1. However, resuspension events are rather frequent at L4, and vary according to tidal and seasonal cycles (Cross et al., 2013). This apparent contradiction can be explained by the fact that episodic resuspension events do not necessitate removal of fluff as a food source of benthic macrofauna: this material either quickly settles back to the seafloor or remains available for uptake from suspension. Complete removal of matter from the site through resuspension and subsequent transport is presumably less likely, which can explain the calibration process deciding on zero net removal of fluff. More detailed description of resuspension dynamics should be prioritised by the follow-up modelling studies by (a) explicitly considering pelagic targets for resuspended material, and (b) vertically resolving structure of near-bed suspended matter, e.g., via Rouse profile, and backed by further observational evidence.
One key question is to what degree the benthic model and its parameter set are portable to different sites, in particular those that experience different levels of pelagic productivity and deposition. Such portability is by no means a given, since sites can further differ in resuspension regime, predatory pressure, trawling activities and type of substrate. Application and validation of the model at other geographical areas and ecosystems is not straightforward in particular because detailed time-series of benthic biomass such as the WCO provides are extremely rare. Perhaps the most promising way forward is the application of the proposed benthic food web model within 3D spatially resolved modelling frameworks, such as NEMO-ERSEM (Edwards et al., 2012). While attractive, this is beyond the scope of this study, as it requires a full mass-balanced representation of the pelagic and benthos that explicitly resolves all loss terms that were left implicit in the present study. This relates to the aforementioned fate of resuspended material, but also to the bulk loss flux from macrofauna, which has to be directed to either DIC (due to respiration), DOC (due to excretion), or various living and non-living pools of POC, both benthic and pelagic (due to e.g., spawning, recruitment, egestion, and predation).
Constructing the model so that it can be constrained by field observations required removal of explicit representation of several groups of biota (aerobic and anaerobic bacteria and meiofauna) which are part of the original configuration of ERSEM. For those groups and the related processes to be restored not only additional observations would be needed, but also experimental evidence on organisms’ specific functioning within ecosystems. In the case of meiofauna, for instance, there is currently no general agreement regarding the significance of their interactions with macrofauna (Giere, 2009).
In the model configuration applied, we restricted the diversity of macrofauna functional groups to suspension- and deposit feeders, in line with Butenschön et al. (2016) formulation of ERSEM. However, species assigned to any of these two groups can differ considerably, with suspension feeders for instance including members of Bivalvia, Malacostraca, and Anthozoa (Figure 2). These species are so different in physiology and ecology that their aggregation undoubtedly limits model skill. Moreover, single species are not always associated with a single feeding mode: in natural communities individual species can exert mixed and temporally varying feeding types. These issues can be addressed by further diversification of the modelled functional types of macrofauna, not only based on their feeding mode, but also considering other traits, e.g., reproductive strategies, feeding behaviour, vertical position within sediment, as well as by consideration of life stages, including juvenile macrofauna as a temporary component of meiofauna. However, model diversification will inevitably be combined with an increase in complexity and, as a result, increased uncertainty in process parameterisations due to insufficiently understood underlying mechanisms and the challenge of translating empirical research findings into mechanistic model formulations. To tackle the challenge of representing biodiversity in ecosystem models effective communication between modellers and empirical scientists is necessary (Queirós et al., 2015).
Conclusions
Our modelling study is a first attempt to uncover the mechanisms that explain why suspension and deposit feeders respond to pelagic production on different time scales. The model parametrisation obtained during calibration effectively implies that suspension and deposit feeders differ in (1) physical and/or trophic proximity to primary production, and (2) their rates of ingestion and losses. In the model, these differences explain why suspension feeders respond much more rapidly to pelagic production than deposit feeders. At this stage, these two mechanisms are merely model-inspired hypotheses. Whether these hypotheses can be confirmed experimentally will be clarified by the follow-up studies. To describe the precise mechanisms responsible for the observed patterns, among the key questions to be addressed by these studies are:
• Do suspension feeders act as gateway between pelagic production and sediment dwelling fauna? And if so, to what extent this can be explained by their vertical separation in physical space, or their preference for different food sources? Among other aspects, work toward answering this question could involve characterisation of the vertical location of biomass and feeding activity of the different types of fauna, and a comparison of their trophic levels.
• Is the near-instantaneous increase of suspension feeder biomass after a phytoplankton bloom consistently supported by high-frequency observations? And if so, what mechanisms allow them to appear so rapidly? And what mechanisms are responsible for dampened response of deposit feeders?
• Are there significant differences in the physiological rates (ingestion, respiration, and other losses) of suspension feeders and deposit feeders, and to what extent can they explain the difference in temporal response?
• Is there a difference in reproductive strategy between suspension and deposit feeders? Can we quantify the biomass fluxes between adult and reproductive life stages, as well as their timing?
• What is the impact of predation on macrofaunal biomass? How does this impact vary seasonally? What are the differences between predation on deposit and suspension feeders?
Answers to the above questions would advance our system understanding of benthic ecology and benthic–pelagic interactions, and simultaneously facilitate further model development, including better representation of the physiology, ecology and diversity of benthic communities and links between functionally and/or size-based different components of the benthic ecosystem. Accordingly, we regard our modelling study not as an endpoint – the model cannot itself answer any of the questions above – but as a fresh look at the ways we could direct further research.
Author Contributions
GL and JB conceived the idea for the study, designed the modelling framework, and analysed the results. CM analysed macrofauna samples. GL and JB wrote the manuscript. SW contributed to the discussions of the study and writing of the manuscript.
Funding
This work was funded by the Marine Ecosystems Research Programme (NE/L003066/1) and UK Shelf Seas Biogeochemistry programme (NE/K001876/1) of the Natural Environment Research Council (NERC), and the Department for Environment Food and Rural Affairs (Defra), as well as NERC National Capability in Marine Modelling. The Western Channel Observatory is part funded by NERC National Capability. This work was also funded by the UK Natural Environment Research Council through its National Capability Long-term Single Centre Science Programme, Climate Linked Atlantic Sector Science, grant number NE/R015953/1, and is a contribution to Theme 1.3 - Biological Dynamics.
Conflict of Interest Statement
The authors declare that the research was conducted in the absence of any commercial or financial relationships that could be construed as a potential conflict of interest.
Acknowledgments
We thank Claire Widdicombe for maintaining and providing the L4 phytoplankton time-series. We thank all those involved in the sample collection and analysis for the Western Channel Observatory, and specifically the crew of the RV Plymouth Quest.
Supplementary Material
The Supplementary Material for this article can be found online at: https://www.frontiersin.org/articles/10.3389/fmars.2019.00015/full#supplementary-material
Footnotes
- ^http://www.westernchannelobservatory.org.uk
- ^https://www.bodc.ac.uk/
- ^http://www.marinespecies.org/traits/docs/reports_pilots/Benthos.pdf
References
Andersen, F. O., and Kristensen, E. (1992). The importance of benthic macrofauna in decomposition of microalgae in a coastal marine sediment. Limnol. Oceanogr. 37, 1392–1403. doi: 10.4319/lo.1992.37.7.1392
Ansell, A., Gibson, R., Barnes, M., and Press, U. (1994). Life—cycle and life—history diversity in marine invertebrates and the implications in community dynamics. Oceanogr. Mar. Biol. Ann. Rev. 32, 305–333.
Austen, M., Buchanan, J., Hunt, H., Josefson, A., and Kendall, M. (1991). Comparison of long-term trends in benthic and pelagic communities of the North Sea. J. Mar. Biol. Assoc. 71, 179–190. doi: 10.1017/S0025315400037498
Blackford, J. C. (1997). An analysis of benthic biological dynamics in a North Sea ecosystem model. J. Sea Res. 38, 213–230. doi: 10.1016/S1385-1101(97)00044-0
Brey, T., Müller-Wiegmann, C., Zittier, Z. M., and Hagen, W. (2010). Body composition in aquatic organisms—a global data bank of relationships between mass, elemental composition and energy content. J. Sea Res. 64, 334–340. doi: 10.1016/j.seares.2010.05.002
Bruggeman, J., and Bolding, K. (2014). A general framework for aquatic biogeochemical models. Environ. Model. Softw. 61, 249–265. doi: 10.1016/j.envsoft.2014.04.002
Bruggeman, J., Heringa, J., and Brandt, B. W. (2009). PhyloPars: estimation of missing parameter values using phylogeny. Nucleic Acids Res. 37(suppl_2), W179–W184. doi: 10.1093/nar/gkp370
Butenschön, M., Clark, J., Aldridge, J. N., Allen, J. I., Artioli, Y., Blackford, J., et al. (2016). ERSEM 15.06: a generic model for marine biogeochemistry and the ecosystem dynamics of the lower trophic levels. Geosci. Model Dev. 9, 1293–1339. doi: 10.5194/gmd-9-1293-2016
Capet, A., Meysman, F. J., Akoumianaki, I., Soetaert, K., and Grégoire, M. (2016). Integrating sediment biogeochemistry into 3D oceanic models: a study of benthic-pelagic coupling in the black sea. Ocean Model. 101, 83–100. doi: 10.1016/j.ocemod.2016.03.006
Coma, R., Ribes, M., Gili, J. M., and Zabala, M. (2000). Seasonality in coastal benthic ecosystems. Trends Ecol. Evol. 15, 448–453. doi: 10.1016/S0169-5347(00)01970-4
Compton, T. J., Bodnar, W., Koolhaas, A., Dekinga, A., Holthuijsen, S., ten Horn, J., et al. (2016). Burrowing behavior of a deposit feeding bivalve predicts change in intertidal ecosystem state. Front. Ecol. Evol. 4:19 doi: 10.3389/fevo.2016.00019
Cross, J., Nimmo-Smith, W. A. M., Torres, R., and Hosegood, P. J. (2013). Biological controls on resuspension and the relationship between particle size and the Kolmogorov length scale in a shallow coastal sea. Mar. Geol 343, 29-38. doi: 10.1016/j.margeo.2013.06.014
Dale, A. W., Boyle, R. A., Lenton, T. M., Ingall, E. D., and Wallmann, K. (2016). A model for microbial phosphorus cycling in bioturbated marine sediments: significance for phosphorus burial in the early Paleozoic. Geochim. Cosmochim. Acta 189, 251–268. doi: 10.1016/j.gca.2016.05.046
Dashfield, S. L., and McNeill, C. L. (2014). PML Benthic Survey Macrofauna Abundance And Biomass Data from Five Sites (Cawsand, L4, Rame, Eddystone And E1) And Megafauna From One Site (L4) In The Western English Channel Surveyed Between 2008 To 2013 Containing Data From Samples Analysed Up To And Including September 2013. Swindon: Natural Environment Research Council.
Dauwe, B., Herman, P., and Heip, C. (1998). Community structure and bioturbation potential of macrofauna at four North Sea stations with contrasting food supply. Mar. Ecol. Prog. Ser. 173, 67–83. doi: 10.3354/meps173067
Ebenhöh, W., Kohlmeier, C., and Radford, P. J. (1995). The benthic biological submodel in the European regional seas ecosystem model. Netherlands J. Sea Res. 33, 423–452. doi: 10.1016/0077-7579(95)90056-X
Edwards, K. P., Barciela, R., and Butenschön, M. (2012). Validation of the Nemo-Ersem operational ecosystem model for the North West European Continental Shelf. Ocean Sci. 8, 983–1000. doi: 10.5194/os-8-983-2012
Franco, M., De Mesel, I., Diallo, M. D., Van Der Gucht, K., Van Gansbeke, D., Van Rijswijk, P., et al. (2007). Effect of phytoplankton bloom deposition on benthic bacterial communities in two contrasting sediments in the southern North Sea. Aquat. Microb. Ecol. 48, 241–254. doi: 10.3354/ame048241
Garrido, M., Chaparro, O., Thompson, R., Garrido, O., and Navarro, J. (2012). Particle sorting and formation and elimination of pseudofaeces in the bivalves Mulinia edulis (siphonate) and Mytilus chilensis (asiphonate). Mar. Biol. 159, 987–1000. doi: 10.1007/s00227-012-1879-8
Giere, O. (2009). Meiobenthology: The Microscopic Motile Fauna of Aquatic Sediments. Berlin: Springer.
Gili, J. M., and Coma, R. (1998). Benthic suspension feeders: their paramount role in littoral marine food webs. Trends Ecol. Evol. 13, 316–321. doi: 10.1016/S0169-5347(98)01365-2
Gooday, A. J., Turley, C. M., and Allen, J. (1990). Responses by benthic organisms to inputs of organic material to the ocean floor: a review. Philos. Trans. R. Soc. Lond. Series A Math. Phys. Sci. 331 119–138. doi: 10.1098/rsta.1990.0060
Heilskov, A. C., and Holmer, M. (2001). Effects of benthic fauna on organic matter mineralization in fish-farm sediments: importance of size and abundance. ICES J. Mar. Sci. 58, 427–434. doi: 10.1006/jmsc.2000.1026
Heip, C., Basford, D., Craeymeersch, J. A., Dewarumez, J. M., Dörjes, J., de Wilde, P., et al. (1992). Trends in biomass, density and diversity of North Sea macrofauna. ICES J. Mar. Sci. 49, 13–22. doi: 10.1093/icesjms/49.1.13
Heip, C., and Craeymeersch, J. A. (1995). Benthic community structures in the North Sea. Helgol. Meeresuntersuchungen 49, 313–328. doi: 10.1007/bf02368359
Iken, K., Bluhm, B., and Dunton, K. (2010). Benthic food-web structure under differing water mass properties in the southern Chukchi Sea. Deep Sea Res. Part II Top. Stud. Oceanogr. 57, 71–85. doi: 10.1016/j.dsr2.2009.08.007
Jickells, T. (1998). Nutrient biogeochemistry of the coastal zone. Science 281, 217–222. doi: 10.1126/science.281.5374.217
Joint, I., and Pomroy, A. (1993). Phytoplankton biomass and production in the southern North Sea. Mar. Ecol. Prog. Ser., 99 169–182. doi: 10.3354/meps099169
Kitidis, V., Tait, K., Nunes, J., Brown, I., Woodward, E., Harris, C., et al. (2017). Seasonal benthic nitrogen cycling in a temperate shelf sea: the Celtic Sea. Biogeochemistry, 135, 103–119. doi: 10.1007/s10533-017-0311-3
Kristensen, E., Andersen, F. Ø., and Blackburn, T. H. (1992). Effects of benthic macrofauna and temperature on degradation of macroalgal detritus: the fate of organic carbon. Limnol. Oceanogr. 37, 1404–1419. doi: 10.4319/lo.1992.37.7.1404
Kristensen, E., Delefosse, M., Quintana, C. O., Flindt, M. R., and Valdemarsen, T. (2014). Influence of benthic macrofauna community shifts on ecosystem functioning in shallow estuaries. Front. Mar. Sci. 1:41. doi: 10.3389/fmars.2014.00041
Lessin, G., Artioli, Y., Queirós, A. M., Widdicombe, S., and Blackford, J. C. (2016). Modelling impacts and recovery in benthic communities exposed to localised high CO2. Mar. Pollut. Bull. 109, 267–280. doi: 10.1016/j.marpolbul.2016.05.071
Lindeque, P. K., Dimond, A., Harmer, R. A., Parry, H. E., Pemberton, K. L., and Fileman, E. S. (2015). Feeding selectivity of bivalve larvae on natural plankton assemblages in the Western English Channel. Mar. Biol. 162, 291–308. doi: 10.1007/s00227-014-2580-x
Maar, M., and Hansen, J. L. S. (2011). Increasing temperatures change pelagic trophodynamics and the balance between pelagic and benthic secondary production in a water column model of the Kattegat. J. Mar. Syst. 85, 57–70. doi: 10.1016/j.jmarsys.2010.11.006
Martins, R., Quintino, V., and Rodrigues, A. M. (2013). Diversity and spatial distribution patterns of the soft-bottom macrofauna communities on the Portuguese continental shelf. J. Sea Res. 83, 173–186. doi: 10.1016/j.seares.2013.03.001
Meysman, F. J., Boudreau, B. P., and Middelburg, J. J. (2003). Relations between local, nonlocal, discrete and continuous models of bioturbation. J. Mar. Res. 61, 391–410. doi: 10.1357/002224003322201241
Meysman, F. J., Galaktionov, O. S., Gribsholt, B., and Middelburg, J. J. (2006). Bioirrigation in permeable sediments: advective pore-water transport induced by burrow ventilation. Limnol. Oceanogr. 51, 142–156. doi: 10.4319/lo.2006.51.1.0142
Navarro-Barranco, C., McNeill, C. L., Widdicombe, C. E., Guerra-García, J. M., and Widdicombe, S. (2017). Long-term dynamics in a soft-bottom amphipod community and the influence of the pelagic environment. Mar. Environ. Res. 129, 133–146 doi: 10.1016/j.marenvres.2017.04.013
Nilsson, P., Sundbäck, K., and Jönsson, B. (1993). Effect of the brown shrimp Crangon crangon L. on endobenthic macrofauna, meiofauna and meiofaunal grazing rates. Netherlands J. Sea Res. 31, 95–106. doi: 10.1016/0077-7579(93)90021-J
Nixon, S., Oviatt, C., Frithsen, J., and Sullivan, B. (1986). Nutrients and the productivity of estuarine and coastal marine ecosystems. J. Limnol. Soc. S. Afr. 12, 43–71. doi: 10.1080/03779688.1986.9639398
Norkko, A., Hewitt, J. E., Thrush, S. F., and Funnell, T. (2001). Benthic-pelagic coupling and suspension-feeding bivalves: linking site-specific sediment flux and biodeposition to benthic community structure. Limnol. Oceanogr. 46, 2067–2072. doi: 10.4319/lo.2001.46.8.2067
Norling, K., Rosenberg, R., Hulth, S., Grémare, A., and Bonsdorff, E. (2007). Importance of functional biodiversity and species-specific traits of benthic fauna for ecosystem functions in marine sediment. Mar. Ecol. Progr. Ser. 332, 11–23. doi: 10.3354/meps332011
Persson, A., and Svensson, J. M. (2006). Vertical distribution of benthic community responses to fish predators, and effects on algae and suspended material. Aquat. Ecol. 40, 85–95. doi: 10.1007/s10452-005-9014-2
Peterson, C. H., and Black, R. (1987). Resource depletion by active suspension feeders on tidal fiats: influence of local density and tidal elevation. Limnol. Oceanogr. 32, 143–166. doi: 10.4319/lo.1987.32.1.0143
Peterson, C. H., and Black, R. (1988). Density-dependent mortality caused by physical stress interacting with biotic history. Am. Nat. 131, 257–270. doi: 10.1086/284788
Queirós, A. M., Bruggeman, J., Stephens, N., Artioli, Y., Butenschön, M., Blackford, J. C., et al. (2015). Placing biodiversity in ecosystem models without getting lost in translation. J. Sea Res. 98, 83–90. doi: 10.1016/j.seares.2014.10.004
Ruhl, H. A., and Smith, K. L. (2004). Shifts in deep-sea community structure linked to climate and food supply. Science 305, 513–515. doi: 10.1126/science.1099759
Service, S. K., Feller, R. J., Coull, B. C., and Woods, R. (1992). Predation effect of three fish species and a shrimp on macrobenthos and meiobenthos in microcosms. Estuar. Coast. Shelf Sci. 34, 277–293. doi: 10.1016/S0272-7714(05)80084-8
Smale, D. A., Burrows, M. T., Moore, P., O’Connor, N., and Hawkins, S. J. (2013). Threats and knowledge gaps for ecosystem services provided by kelp forests: a northeast Atlantic perspective. Ecol. Evol. 3, 4016–4038. doi: 10.1002/ece3.774
Smyth, T., Atkinson, A., Widdicombe, S., Frost, M., Allen, I., Fishwick, J., et al. (2015). The western channel observatory. Prog. Oceanogr. 137, (Part B), 335–341. doi: 10.1016/j.pocean.2015.05.020
Snelgrove, P. V. (1999). Getting to the bottom of marine biodiversity: sedimentary habitats: ocean bottoms are the most widespread habitat on earth and support high biodiversity and key ecosystem services. Bioscience 49, 129–138. doi: 10.2307/1313538
Snelgrove, P. V. R. (1998). The biodiversity of macrofaunal organisms in marine sediments. Biodivers. Conserv. 7, 1123–1132. doi: 10.1023/a:1008867313340
Soetaert, K., and Middelburg, J. J. (2009). Modeling eutrophication and oligotrophication of shallow-water marine systems: the importance of sediments under stratified and well-mixed conditions. Hydrobiologia 629, 239–254. doi: 10.1007/s10750-009-9777-x
Steven, G. (1930). Bottom fauna and the food of fishes. J. Mar. Biol. Assoc. 16, 677–706. doi: 10.1017/S0025315400073033
Stolpovsky, K., Dale, A. W., and Wallmann, K. (2015). Toward a parameterization of global-scale organic carbon mineralization kinetics in surface marine sediments. Global Biogeochem. Cycles 29, 812–829. doi: 10.1002/2015GB005087
Storn, R., and Price, K. (1997). Differential evolution – a simple and efficient heuristic for global optimization over continuous spaces. J. Global Optim. 11, 341–359. doi: 10.1023/a:1008202821328
Tait, K., Airs, R. L., Widdicombe, C. E., Tarran, G. A., Jones, M. R., and Widdicombe, S. (2015). Dynamic responses of the benthic bacterial community at the Western English Channel observatory site L4 are driven by deposition of fresh phytodetritus. Prog. Oceanogr. 137, 546–558. doi: 10.1016/j.pocean.2015.04.020
Thullner, M., Dale, A. W., and Regnier, P. (2009). Global-scale quantification of mineralization pathways in marine sediments: a reaction-transport modeling approach. Geochem. Geophys. Geosyst. 10. doi: 10.1029/2009GC002484
Virnstein, R. W. (1977). The importance of predation by crabs and fishes on benthic infauna in Chesapeake Bay. Ecology 58, 1199–1217. doi: 10.2307/1935076
Widdicombe, C. E., Eloire, D., Harbour, D., Harris, R. P., and Somerfield, P. J. (2010). Long-term phytoplankton community dynamics in the Western English Channel. J. Plankton Res. 32, 643–655. doi: 10.1093/plankt/fbp127
Widdows, J. (1991). Physiological ecology of mussel larvae. Aquaculture 94, 147–163. doi: 10.1016/0044-8486(91)90115-N
Yakushev, E. V., Protsenko, E. A., Bruggeman, J., Wallhead, P., Pakhomova, S. V., Yakubov, S. K., et al. (2017). Bottom RedOx Model (BROM v.1.1): a coupled benthic–pelagic model for simulation of water and sediment biogeochemistry. Geosci. Model Dev. 10, 453–482. doi: 10.5194/gmd-10-453-2017
Keywords: benthic macrofauna, benthic–pelagic coupling, phytoplankton blooms, ecosystem modelling, benthic food web, organic matter, ERSEM, Western Channel Observatory
Citation: Lessin G, Bruggeman J, McNeill CL and Widdicombe S (2019) Time Scales of Benthic Macrofaunal Response to Pelagic Production Differ Between Major Feeding Groups. Front. Mar. Sci. 6:15. doi: 10.3389/fmars.2019.00015
Received: 01 November 2018; Accepted: 14 January 2019;
Published: 30 January 2019.
Edited by:
Susana Carvalho, King Abdullah University of Science and Technology, Saudi ArabiaReviewed by:
Jeroen Ingels, Florida State University, United StatesKapuli Gani Mohamed Thameemul Ansari, Indian Institute of Science Education and Research Kolkata, India
Marie Maar, Aarhus University, Denmark
Copyright © 2019 Lessin, Bruggeman, McNeill and Widdicombe. This is an open-access article distributed under the terms of the Creative Commons Attribution License (CC BY). The use, distribution or reproduction in other forums is permitted, provided the original author(s) and the copyright owner(s) are credited and that the original publication in this journal is cited, in accordance with accepted academic practice. No use, distribution or reproduction is permitted which does not comply with these terms.
*Correspondence: Gennadi Lessin, gle@pml.ac.uk